the Creative Commons Attribution 4.0 License.
the Creative Commons Attribution 4.0 License.
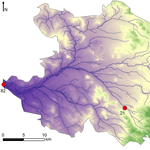
Seasonal streamflow forecasts in the Ahlergaarde catchment, Denmark: the effect of preprocessing and post-processing on skill and statistical consistency
Diana Lucatero
Henrik Madsen
Jens C. Refsgaard
Jacob Kidmose
Karsten H. Jensen
In the present study we analyze the effect of bias adjustments in both meteorological and streamflow forecasts on the skill and statistical consistency of monthly streamflow and yearly minimum daily flow forecasts. Both raw and preprocessed meteorological seasonal forecasts from the European Centre for Medium-Range Weather Forecasts (ECMWF) are used as inputs to a spatially distributed, coupled surface–subsurface hydrological model based on the MIKE SHE code. Streamflow predictions are then generated up to 7 months in advance. In addition to this, we post-process streamflow predictions using an empirical quantile mapping technique. Bias, skill and statistical consistency are the qualities evaluated throughout the forecast-generating strategies and we analyze where the different strategies fall short to improve them. ECMWF System 4-based streamflow forecasts tend to show a lower accuracy level than those generated with an ensemble of historical observations, a method commonly known as ensemble streamflow prediction (ESP). This is particularly true at longer lead times, for the dry season and for streamflow stations that exhibit low hydrological model errors. Biases in the mean are better removed by post-processing that in turn is reflected in the higher level of statistical consistency. However, in general, the reduction of these biases is not sufficient to ensure a higher level of accuracy than the ESP forecasts. This is true for both monthly mean and minimum yearly streamflow forecasts. We discuss the importance of including a better estimation of the initial state of the catchment, which may increase the capability of the system to forecast streamflow at longer leads.
Seasonal streamflow forecasting encompasses a variety of methods that range from purely data-based to entirely model-based or hybrid methods that exploit the benefits of each (Mendoza et al., 2017). Data-driven methods find empirical relationships between streamflow and a variety of predictors. These relationships are then used to derive forecasts for the upcoming seasons. Different predictors can be used depending on the relative importance they have for the regional hydroclimatic conditions. Predictors that have been used include large-scale climate indicators such as El Niño or the North Atlantic Oscillation, (Schepen et al., 2016; Shamir, 2017; Wang et al., 2009; Olsson et al., 2016), precipitation and land temperature (Córdoba-Machado et al., 2016), the state of the catchment in the form of streamflow, soil moisture, groundwater storages or snow storages that can be derived either by the use of a hydrological model, hence the term “hybrid” (Robertson et al., 2013; Rosenberg et al., 2011), or by means of observed antecedent conditions (Robertson and Wang, 2012).
Model-based systems include a hydrological model in the forecasting chain. Differences between forecasting frameworks may arise in the forcings, the initialization framework and/or the hydrological model structure and parameters. Focusing on the forcing, one can either use observed meteorology from previous years, a method that is commonly known as ensemble streamflow prediction (ESP) (Day, 1985), or outputs from general circulation models (GCMs) (Crochemore et al., 2016; Wood et al., 2002, 2005; Wood and Lettenmaier, 2006; Yuan et al., 2011, 2013, 2015, 2016). In principle, the latter should be more suitable in providing skillful forecasts as they are able to capture the evolving chaotic behavior of the atmosphere, whereas the ESP approach assumes that what has been observed in the past can be used as a proxy for what will happen in the future, an assumption that requires stationary climate conditions. On the other hand, the lack of reliability of GCMs in forecasting atmospheric patterns at long lead times precludes their use in weather-impacted sectors (Bruno Soares and Dessai, 2016; Weisheimer and Palmer, 2014). For example, a previous study on the skill of the European Centre for Medium-Range Weather Forecasts (ECMWF) System 4 in Denmark concluded that, in general, the precipitation forecast bias in the catchment area was in general around −25 % (Lucatero et al., 2017). This bias, together with the sharpness of forecasts, led to a mild positive skill limited to the first month lead time (Lucatero et al., 2017). These results are in accordance with skill studies with focus on a similar area (Crochemore, et al., 2017). This is the reason why preprocessing and post-processing should be performed when using GCM forecasts to force a hydrological model to eliminate biases intrinsic to climate and hydrological models. In the context of this study, preprocessing refers to any method that improves the forcings, i.e., precipitation and temperature, used in the hydrological forecasting system. Post-processing refers to the improvements achieved in the outputs of the hydrological model, e.g., streamflow. In this respect, post-processing also corrects errors in hydrological models that cannot be eliminated through calibration (Shi et al., 2008; Yuan et al., 2015; Yuan and Wood, 2012).
A couple of studies have quantified the effects on streamflow skill by preprocessing either seasonal (Crochemore et al., 2016) or medium-range (Verkade et al., 2013) forecasts. Other studies have assessed the efficiency of post-processing streamflow forecasts only (Bogner et al., 2016; Madadgar et al., 2014; Ye et al., 2015; Zhao et al., 2011; Wood and Schaake, 2008). To the best of our knowledge, only Roulin and Vannitsem (2015), Yuan and Wood (2012) and Zalachori et al. (2012) have compared the additional gain in skill of doing both preprocessing and post-processing. The previous studies have shown that improvements made by preprocessing the forcings do not necessarily translate into improvements in streamflow forecasts (Verkade et al., 2013; Zalachori et al., 2012). Improvements are larger when post-processing is done, and a combination of preprocessing and post-processing provides the best results (Yuan and Wood, 2012; Zalachori et al., 2012). To the best of our knowledge, only Yuan and Wood (2012) have made this evaluation in the context of seasonal forecasting.
The present study focuses on the following aspects: (i) the evaluation of the use of a GCM to generate seasonal streamflow forecasts, (ii) the study of the effect that preprocessing and post-processing have on streamflow forecasts 1–7 months ahead, and (iii) the effect of hydrological model biases in forecast skill evaluations. This is done by a combination of the following methodological choices. First, we make use of seasonal meteorological forecasts of ECMWF System 4 (Molteni, et al., 2011). Secondly, the hydrological simulations use an integrated physically based and spatially distributed model based on the MIKE SHE code (Graham and Butts, 2005). Thirdly, our evaluation focuses on three forecast qualities: bias, skill and statistical consistency. Skill is measured using ESP as a reference and focusing on both accuracy and sharpness. Finally, the focus here is to evaluate forecasts of monthly average streamflow throughout the year and minimum daily flows during the summer. The catchment serving as a basis of our study is groundwater-dominated and is located in a region where seasonal forecasting is a challenging endeavor (Lucatero et al., 2017). The following questions are then addressed.
-
How do GCM-generated forecasts compare to those of the ESP approach?
-
What is the effect of preprocessing and post-processing on streamflow forecasts in terms of bias, skill and statistical consistency? And more specifically, is there one single approach, or a combination of several, that reduces the bias and augments skill and statistical consistency?
-
What is the effect that hydrological model bias has on the evaluation of preprocessed and post-processed streamflow forecasts?
The following sections give a description of the methodology followed in this study. A graphical depiction of the steps carried out can be seen in Fig. 1.
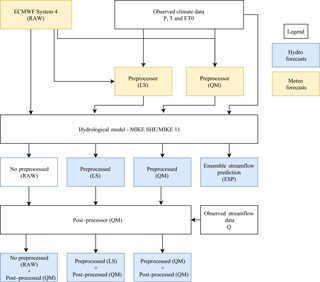
Figure 1Diagram of generation of forecasts and verification procedures. RAW refers to the uncorrected ECMWF System 4 forecasts, while LS and QM refer to forecasts (either meteorological or hydrological) that are corrected using the linear scaling/delta change or quantile mapping method, respectively, for precipitation (P), temperature (T) and reference evapotranspiration (ET0). Preprocessed refers to streamflow forecasts generated using corrected meteorological forecasts while post-processed refers to corrected streamflow forecasts.
2.1 Area of study, observational data and hydrological model
The present study is carried out for the Ahlergaarde catchment located in West Jutland, Denmark (Fig. 2), which has a size of 1044 km2. It is located in one of the most irrigated zones in Denmark, with 55 % of the area covered with agricultural crops such as barley, grass, wheat, maize and potatoes. The remaining area is distributed in categories as follows: grass (30 %), forest (7 %), heath (5 %), urban (2 %) and other (1 %) (Jensen and Illangasekare, 2011).
The climatology of the area is shown in Fig. 3. Climate in the Ahlergaarde region is mainly influenced by its proximity to the sea towards the west. The mean annual precipitation, reference evapotranspiration and discharge for the period 1990–2013 is 983, 540 and 500 mm, respectively. The hydrology of the catchment is groundwater-dominated due to the high permeability of the top geological layer, which consists mainly of sand and gravel. Another consequence of the geological composition of the surface layer is that overland flow rarely happens (Jacob Kidmose, personal communication, 2014). Daily precipitation (P), temperature (T) and reference evapotranspiration (ET0) data are retrieved from the Danish Meteorological Institute (DMI; Scharling and Kern-Hansen, 2012). The dataset spatial domain covers Denmark with a 10 km grid resolution for P and a 20 km resolution for T and ET0. P is corrected for systematic under-catch due to wind effects (Stisen et al., 2011, 2012), and ET0 is derived using the Makkink formulation (Hendriks, 2010). Finally, daily streamflow observations are retrieved from the Danish Hydrological Observatory (HOBE) (Jensen and Illangasekare, 2011) datasets.
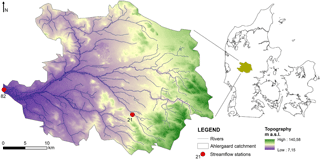
Figure 2Location and topography of the Ahlergaarde catchment. The outlet station (82) and the upstream sub-catchment (21) are used in the study.
The hydrological simulations for this study are grounded on a physically based, spatially distributed, coupled surface–subsurface model that simulates the main hydrological processes such as evapotranspiration, overland flow, unsaturated, saturated and streamflows and their interactions. The model is based on the MIKE SHE code (Graham and Butts, 2005). Groundwater flow is described by the governing equation for three-dimensional groundwater flow based on Darcy's law. Drain flow is considered when the groundwater table exceeds a drain level. Surface water flow in streams is simulated by a one-dimensional channel flow model based on kinematic routing, while a two-dimensional diffusive wave approximation of the St. Venant equations is used for overland flow routing. Finally, a two-layer approach is used for the simulations of unsaturated flow and evapotranspiration (Graham and Butts, 2005). Snow is not an important process in the study area; therefore, the model takes snowmelt into account by using a simple degree-day model formulation. The horizontal numerical discretization is 200 m, whereas the vertical discretization is based on six numerical layers whose dimension depends on the geological stratigraphy. Model parameters were calibrated against groundwater head and discharge using an automated optimizer, PEST (parameter estimation) version 11.8 (Doherty, 2016) for the 2006–2009 period. Parameters to be calibrated were selected based on a sensitivity analysis study. These are hydraulic conductivities for 10 geological units, specific yield, specific storage, drain time constant, detention storage, river-groundwater conductance and root depth of 10 vegetation types. The reader is referred to Zhang et al. (2016) for further details on the calibration procedure.
2.2 Forecast generation: GCM-based and ESP
As seen in Fig. 1, P, T and ET0 forecasts are taken from the ECMWF System 4 (RAW), preprocessed ECMWF System 4 (linear scaling, LS, and quantile mapping, QM), and historical observations (ESP). The European Centre for Medium-Range Weather Forecasts (ECMWF) offers a seasonal forecasting product that currently is in its version number 4 (Molteni et al., 2011). An attempt to reduce the biases intrinsic in ECMWF System 4 led to what we refer to as preprocessed forecasts. The reader is referred to Lucatero et al. (2017) for details of the evaluation of both ECMWF System 4 and preprocessed forecasts for Denmark. The spatial resolution of the raw forecasts is 0.7∘ in latitude and longitude. Forecasts were interpolated to a 10 km grid to match the resolution of the observed grid. For the Ahlergaarde catchment, forecast–observation data for the 1990–2013 period are extracted for 24 grid points covering the study area, leading to a sample size of 24 years. Finally, ET0 is computed using the Makkink formulation (Hendriks, 2010) that takes T and incoming shortwave solar radiation from the ECMWF System 4 forecasts as inputs.
Daily raw and preprocessed forecasts are initialized on the first day of each calendar month with a 7-month lead time. The number of ensemble members varies by month, 15 for January, March, April, June, July, September, October and December, and 51 for the remaining months. The number of ensembles is higher for February, May, August and November to aid in improving forecasts for the most predictable seasons. ESP forcings are taken from the observation record, with each year acting as an ensemble member. Values are taken from the start of each calendar month, with a 7-month lead time in order to match the lead time of the ECMWF System 4 forecasts. Since the year to be forecasted is left out of the ensemble, the number of ensemble members for the ESP is 23. Both the ECMWF System 4-generated forecasts and ESP share the same hydrological initial conditions for forecasts initiated in the same month. These are computed from a spin-up run starting in January 1990 and up until 2013. Initial states are saved on the first day of each calendar month. Forecasts are then run on a daily basis up to 7 months.
2.3 Preprocessor and post-processor
Preprocessed forcings for the hydrological model were retrieved from data of the companion paper, Lucatero et al. (2017). The authors used two well-known bias correction techniques, LS and QM. In LS the ensemble is adjusted with a scaling factor, either by multiplication (for P and ET0) or addition (T). The scaling factor is computed as the ratio or difference between the averages of the ensemble mean and the observed mean for a specific month, lead time and location, with the sole purpose of adjusting the mean.
QM (Zhao et al., 2017) matches the quantiles of the ensemble distribution with the quantiles of the observed distribution in the following way:
where G and F represent the observed and the ensemble distribution functions, respectively, for forecast–observation pair i, for , with M being the number of forecast–observation pairs. fk,i represents ensemble member k, , where N is the ensemble size and represents the corrected ensemble member k. F is an empirical distribution function trained with all ensemble members in a given month for a given lead time and location. G and F are fitted on a leave-one-out cross-validation mode, i.e., the forecast–observation pair i is left out of the sample. For example, for a forecast of the target month April, initialized in February, F is computed using all ensemble members, comprising 30 (days) times 23 (number of years in the training sample minus the year to be corrected), times the ensemble size of that particular month (15 or 51). The same is done for G. Linear extrapolation is applied to approximate the values between the bins of F and G and to map ensemble values and quantiles that are outside the training sample.
QM is the only method used for post-processing in the present study as no striking differences in either bias or skill were found between LS and QM in Lucatero et al. (2017). Moreover, QM shows more satisfactory results for the correction of forecasts in the lower tail of the distribution and for the correction of forecasts that also exhibit underdispersion (Lucatero et al., 2017).
2.4 Performance metrics
The performance of raw, preprocessed and post-processed forecasts is evaluated. Our main focus is the following four qualities: bias, skill in regards to accuracy and sharpness and statistical consistency. Bias is the measure of under- or overestimation of the mean of the ensemble in comparison with the observed values (Yapo et al., 1996):
where and yi represent, respectively, the ensemble mean and the observed values for forecast–observation pair i of a particular month, lead time and location. If the value in Eq. (2) is negative, we have an underprediction, and conversely an overprediction, if the value is positive.
Secondly, we compute the continuous rank probability score CRPS (Hersbach, 2000) as a general measure of the accuracy of the forecasts. The computation of the score is as follows:
where Pi(x) represents the cumulative distribution function (CDF) of the ensemble for forecast–observation pair i, H(x−yi) is the Heaviside function that takes the value 0 when x<yi or 1 otherwise. yi is the verifying observation of forecast–observation pair i. Sharpness for forecast–observation pair i is measured as the difference between the 25 and the 75 % percentiles. The average of these differences along the forecast–observation record is then used as a measure of sharpness. Both the CRPS and sharpness scores are then given in the units of the variable of interest, i.e., m−3 s−1 for streamflow. Both scores are positive oriented; i.e., the lower the value, the more accurate or sharper a forecast. A skill score can then be computed in the following manner:
where, for the present study, Scoresys is the score of streamflow forecasts generated either using raw, preprocessed ECMWF System 4 or post-processed forecasts. Scoreref is the score value of our reference system, the ESP. The range of the skill score in Eq. (4) is from −∞ to 1, and values closer to 1 are preferred. Negative values indicate that, on average, the system being evaluated does not perform better than the ESP used as reference. Hereafter, we denote the skill with respect to accuracy as CRPSS and the skill in terms of sharpness as SS. In order to evaluate the statistical significance of the differences of skill between GCM-generated forecasts and ESP, we use a two-sided Wilcoxon–Mann–Whitney test (WMW test) at the 5 % significance level (see Hollander et al., 2014).
Since the number of ensemble members varies from month to month, the value of the skill scores for months with a larger ensemble size will be more favorable. Although the purpose of the present study is not to make an in-depth analysis of the effect of changing ensemble size, we utilized a bootstrapping technique to make the reader aware of the possible gains in skill due to increased ensemble size. This is accomplished by computing the skill scores of a random selection of 15 of the 51 ensemble members for February, May, August and November as in Jaun et al. (2008). This step is performed 1000 times. The final value of the skill score of interest is then the average of these. Note that the bootstrapping is not applied to the ESP forecasts with an ensemble size of 23 members.
Finally, in order to evaluate the statistical consistency between predictive and observed distribution functions, we use the probability integral transform (PIT) diagram. The PIT diagram is the CDF of , where zi is the value of the cumulative distribution function that the observed value attains within the ensemble distribution for each forecast–observation pair i. Note that the PIT diagram is the continuous equivalent of the rank histograms (Friederichs and Thorarinsdottir, 2012) and it is mainly used to evaluate statistical consistency of a continuous predictive CDF. However, in this study, the s are based on the empirical CDF of the ensemble members at a given lead time. Note that the evaluation of the appropriateness of the choice of PIT diagrams over rank histograms for ensemble forecasts is beyond the scope of the present study. For a forecasting system to be statistically consistent, meaning that the observations can be seen as a draw of the predictive CDF, the CDF of the s should be close to the CDF of a uniform distribution in the [0, 1] range. Deviations from the uniform distribution signify bias in the ensemble mean and spread (see Laio and Tamea, 2007). Finally, in order to make the test for uniformity formal, we make use of the Kolmogorov confidence bands. The bands are two straight lines, parallel to the 1 : 1 diagonal and at a distance , where q(α) is a coefficient that depends on the significance level of the test, i.e., (see Laio and Tamea, 2007; D'Agostino and Stephens, 1986), and M is again the number of forecast–observation pairs. The test for uniformity is not rejected if the CDF of the s lies within these bands.
2.5 Forecasts of minimum daily flow within a year
Annual minimum daily flow forecasts can be used for optimizing groundwater extractions for irrigation. The years for which the predicted minimum daily flows are above the prescribed minimum can be exploited and utilized for crops with a higher irrigation demand that may increase economic returns. Here we focus on forecasts initiated in April. For the purposes of this study, minimum daily flows are defined as the flow of the day with the minimum yearly discharge (m−3 s−1) that usually happens during July to September (Fig. 3). Note that timing errors are not an issue here due to the computation choice of minimum daily flows. Observed minimum daily flow is computed as the flow of the day with the minimum discharge over the 7-month forecasting period (April–October). Forecasted minimum daily flow (for each ensemble) is computed in the same manner. Timing errors will only be visible if forecasted minimum daily flow was chosen to be the discharge values of the day where minimum daily flow was observed, which is not the case here. Studies that have focused their attention on situations of low flow or hydrological drought in the context of seasonal forecasting exist (Fundel et al., 2013; Demirel et al., 2015; Trambauer et al., 2015), documenting the possibility of extracting skillful forecasts months ahead for low flow/drought scenarios. Finally, minimum daily flow forecasts are evaluated using the same skill scores as for monthly flow forecasts, i.e., using ESP as a reference forecast.
3.1 Hydrological model evaluation
Figure 4 shows the results for simulated streamflow at the upstream station 21 and the downstream station 82. The focus of the evaluation is done for daily values during the period from 2000 to 2003. As a preliminary evaluation, we computed the percent bias (PBias) and the Nash–Sutcliffe model efficiency coefficient (NSE) for the complete observed–simulated record (1990–2013). There is, in general, a good agreement in timing between observed and simulated values. The visual inspection of the hydrographs reveal, however, an amplitude error that is more pronounced at the upstream station 21, especially during the winter season. Evidence for this is also reflected by the high values of bias and the negative NSE for this station (NSE = −0.85). Furthermore, a scatterplot of simulated and observed minimum daily flows for the 24 years shows an overestimation of the minimum daily flows that is more pronounced at the upstream station (Fig. 4). At the outlet station 82 there is a better behavior in terms of bias and NSE, with an overestimation of only 1.7 % and a NSE of 0.73. Moreover, for this station there is a better agreement in both the high and low flows through the year. The latter can be verified by looking at the scatterplot of the minimum daily flows (Fig. 4), with the majority of points lying close to the 1 : 1 diagonal.
Due to the poor performance at the upstream station 21, in the following Sect. 3.2–3.4 we will discuss the skill and consistency of the different approaches for forecast improvement, with a focus on the outlet station only. The large biases in the upstream station, combined with the structural biases of the meteorological forecasts, seem to inflate the skill of the streamflow forecasts. This will be further discussed in Sect. 3.5.
3.2 Streamflow forecasts forced with raw meteorological forecasts
The bias and skill of the monthly streamflow forecasts forced with raw ECMWF forecasts are shown in the first row of Fig. 5. The x axis represents the different lead times in months, while the y axis represents the target month. For example, the bias of November with a lead time of 2 represents the value of bias for a forecast initiated on 1 October for the target month of November. This bias is in the [−30, −20 %] range. In general, the absolute bias increases with lead time, and usually moves from an overprediction (or mild underprediction) to a large negative bias at longer lead times.
Figure 5 also shows the skill of accuracy and sharpness. The months with statistically significant differences in skill between the ESP and ECMWF System 4 forecasts are represented with a black circle. There is a connection between bias and skill of accuracy in the sense that months with a higher bias tend to be the ones with lower or nonexistent skill (e.g., September, October, November). The opposite also holds; i.e., months with milder bias tend to be the months when the forecast is improving over the reference forecast to a higher degree (e.g., December, January, February). This is by no means surprising, as the CRPS penalizes forecasts that have biases.
The CRPSS is negative, except for some months during winter and at short lead times for which a forecast generated using raw ECMWF System 4 forcings improves accuracy up to 40 % compared to ESP. As for the case of bias, skill depends on lead time, reaching its most negative values for forecasts generated 7 months in advance. One important feature is the high skill that a forecast generated using ECMWF raw forcings has in terms of sharpness (SS). Figure 5 shows that this quality is present in the majority of target months and lead times. Note, however, that sharpness is only a desirable property when biases are low. In our case study, the width of the raw forecasts is smaller than that of the ESP, indicating overconfidence when biases are high.
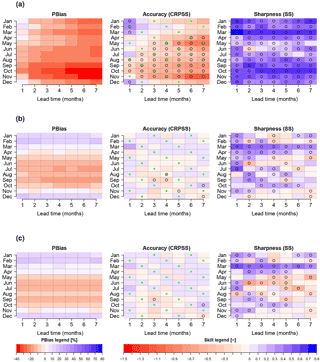
Figure 5PBias and skill in terms of accuracy and sharpness of monthly means of daily streamflow of raw and preprocessed forecasts at station 82. Streamflow forecasts are generated using (a) raw meteorological forecasts and preprocessed meteorological forecasts with the (b) linear scaling/delta change (LS) and (c) quantile mapping (QM) methods. The y axis represents the target month, and the x axis represents the different lead times at which target months are forecasted. Values in the blue range show a positive bias/skill and values in red a negative bias/skill. Circles represent the cases where the distribution of the accuracy and/or sharpness for ESP differs from that of the ECMWF System 4-generated forecasts at a 5 % significance level using the WMW test. Green crosses represent the months/lead times for which the ensemble size is 51.
The results of the bootstrapping procedure for the computation of the skill score due to accuracy (CRPSS) indicate that, by reducing the ensemble size to 15, there is a reduction of skill as expected (not shown). However, this reduction does not change the main conclusion. Months with positive skill due to accuracy remain, in general, positive. For example, the CRPSS of February streamflow forecasts at a lead time of 1 is 0.31 for 51 ensemble members. After the bootstrapping experiment with the reduction to 15 ensemble members, the skill score is mildly reduced to 0.29. In order to make the reader aware of the possible increase of skill due to increased ensemble size, green crosses in Fig. 5 (and subsequent figures dealing with skill due to accuracy) represent the target months and lead times with 51 ensemble members.
Statistical consistency of the raw forecasts is visualized on the first column of the PIT diagrams in Fig. 6 for winter (December, January and February) and summer (June, July and August) (first and second row, respectively) at a lead time of 1. Kolmogorov confidence bands are also plotted for a graphical test of uniformity at the α=0.05 level. For the sake of brevity, the remaining seasons and lead times are not shown. For the particular seasons and lead time shown, statistical consistency seems to be achieved only for the wettest months (December–February). The explanation for this particular behavior will be given in Sect. 3.5. Early spring and November forecasts are also able to pass the uniformity test at a lead time of 1 month (not shown). Summer forecasts together with late spring and autumn months (May, September and October, not shown) show a significant underprediction, which prevents them from passing the uniformity test. Statistical consistency worsens as the lead time increases, in accordance with the deterioration of the bias in Fig. 5.
3.3 Streamflow forecasts forced with preprocessed meteorological forecasts
The second and third rows of Fig. 5 show the bias and skill of streamflow forecasts generated using preprocessed forcings from ECMWF System 4 using the LS and the QM method, respectively.
Several conclusions can be drawn when comparing forecasts using the preprocessed and raw forcings. First, biases are clearly improved, especially for longer lead times. For example, for October forecasts from a lead time of 3 to 7 months, biases are reduced from the [−40, −30 %] to the [−20, 10 %] range for LS and to the [−15, 20 %] range for QM. There are, however, no obvious differences between the two preprocessing methods, which seem to perform equally well in reducing biases. Secondly, three features of accuracy are seen. The first one is that, also for accuracy, there are no obvious differences in skill between the two preprocessing methods. Furthermore, there seems to be a reduction of skill for the winter months and March in the first month lead time. These months are the only ones with a statistically significant skill using the raw forecasts. This feature is a consequence of the reduction of the forcing biases, a situation that will be further discussed in Sect. 3.5. The last feature is that the improvement of the forcings can help to reduce the negative skill in streamflow forecasts. For example, April to November forecasts at longer lead times, generated using raw ECMWF System 4 forcings, exhibit a highly negative and statistically significant skill, sometimes lower than −1.0. Streamflow forecasts generated using preprocessed forcings for those months tend to have a neutral skill. This in turn implies that their accuracy is not different from the accuracy of ESP forecasts. The final conclusion is related to sharpness. As we can see in Fig. 5, streamflow forecasts generated using preprocessed forcings have an ensemble range that is wider than the reference ESP forecasts. This indicates that preprocessing the forcings also leads to a reduction of sharpness in comparison to forecasts generated using raw forcings (Sect. 3.2.).
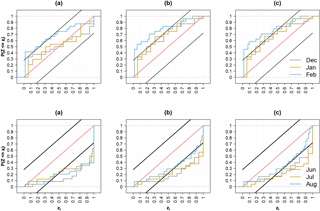
Figure 6PIT diagrams of monthly means of daily streamflow forecasts for winter (upper row) and summer (bottom row) of station 82 for (a) raw meteorological forecasts and preprocessed meteorological forecasts with (b) linear scaling/delta change (LS) and (c) quantile mapping (QM). The lead time is 1 month. Different colors represent different months in the season. The black lines parallel to the 1 : 1 diagonal are the Kolmogorov bands at the 5 % significance level.
The second and third columns in Fig. 6 show the PIT diagrams of streamflow forecasts generated using preprocessed forcings for the winter and summer forecasts in the first month lead time. The statistical consistency for the winter months is worse than the consistency of forecasts generated using raw forcings. The same degree of deterioration is seen for both preprocessing methods. This is caused by compensational errors that will be further discussed in Sect. 3.5. Besides that particular season, improvements in consistency after preprocessing can be seen during the autumn (not shown) and August, although to a lesser degree. For spring (not shown) and early summer forecasts, the same level of consistency is observed for both the raw and preprocessed forecasts. At longer lead times, the benefit of preprocessing for statistical consistency is clearer, with most of the months passing the uniformity test (not shown).
3.4 Post-processed streamflow forecasts
The final step in the analysis is the post-processing of streamflow forecasts generated using raw and preprocessed ECMWF System 4 forcings. Figure 7 shows the verification results that can be directly compared to the results in Fig. 5.
The first column in Fig. 7 shows a clear reduction of the absolute bias compared to the raw and preprocessed generated forecasts. Bias lies within the range [−10, 10 %], for all months and lead times. Furthermore, the majority of the CRPSS values for all months and lead times are positive, while a small negative skill is seen during the autumn. Note, however, that the differences in accuracy between ESP and the post-processed forecasts are only significant at the 5 % level for few target months and lead times. In general, there seems to be a worsening of the sharpness after post-processing (Fig. 5). However, this deterioration is lower when comparing preprocessed versus post-processed forecasts. Furthermore, the degree of the deterioration varies according to the target month. For example, summer months (June and July) exhibit a larger deterioration of sharpness; i.e., the forecast spread is larger than that of the ESP. On the other hand, forecasts for late autumn and early December appear to be narrower than ESP forecasts after post-processing.
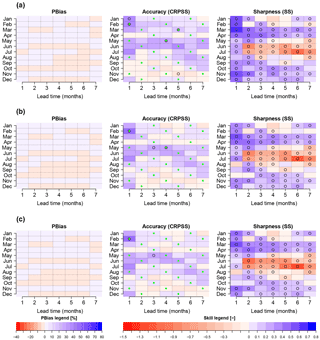
Figure 7PBias and skill (sharpness and accuracy) of daily monthly mean streamflow forecasts for post-processed forecasts using the quantile mapping (QM) method for predictions generated using raw (a) and preprocessed meteorological forcings with the linear scaling/delta change (b) and quantile mapping (c) methods. Legend is the same as Fig. 5. Green crosses represent the months/lead times were the ensemble size is 51.
Figure 8 shows the PIT diagrams for the months of the summer and winter seasons in the first month lead time of post-processed streamflow forecasts. The plot can be directly compared to Fig. 6. As seen from the PIT diagram, all months in those seasons pass the uniformity test, indicating that after post-processing, the observations can be considered as random samples of the predictive distribution. The remaining PIT diagrams for spring and autumn and lead times of 2–7 months (not shown in Fig. 8) show that statistical consistency is present for all months and lead times. At longer lead times, the CDFs of the s are closer to the 1 : 1 diagonal. This is achieved due to two factors: (i) the additional reduction of bias after post-processing and (ii) the worsening of sharpness for long lead times, when the larger ensemble spread encloses a larger portion of observed values.
3.5 Effect of hydrological model bias in skill evaluations
As mentioned in Sect. 3.1., hydrological model biases, which are larger for the upstream station 21 (Fig. 4), combined with structural biases in GCMs, can lead to a situation with a high skill resulting from compensational errors providing “the right forecast for the wrong reasons”. In order to illustrate this point, Fig. 9a and b show the CRPSS for, respectively, station 21 with a large bias (PBias = 48 %, Fig. 4) and station 82 with a small bias (PBias = 1.7 %, Fig. 4). The figure shows CRPSS for forecasts generated using raw ECMWF forcings and preprocessed forcings with the LS method for the target months January–December at a lead time of 4 (e.g., January forecasts initiated in October). In addition to the computation of bias and accuracy of ECMWF-based streamflow forecasts and ESP forecasts using observed streamflow, we also include a computation of bias and accuracy against simulated streamflows (continuous run of the Ahlergaarde model with observed meteorological forcings, Fig. 4). This is done in order to remove the effect of hydrological model bias and hence focus the analyses on the biases coming from forcings alone.
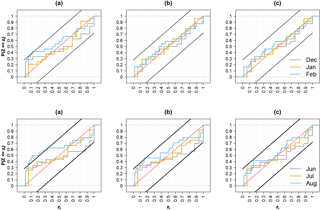
Figure 8PIT diagrams of daily monthly mean streamflow post-processed forecasts for summer (upper row) and winter (bottom row) of station 82. Streamflow forecasts are post-processed using the quantile mapping (QM) method for predictions generated using raw (a) and preprocessed forcings with the linear scaling/delta change (b) and quantile mapping (c) methods. Lead time 1 month. The black lines parallel to the 1 : 1 diagonal are the Kolmogorov bands at the 5 % significance level.
The high skill against observed streamflows is more visible during the wettest months (November–April) for station 21 where hydrological model biases are highest (Fig. 4). Once the comparison is made against simulated streamflows, the high positive skill becomes highly negative (Fig. 9a). The deterioration of skill when compared against simulated streamflows is also seen at station 82 for December–March, although to a lesser extent (Fig. 9b). To illustrate why this happens, Fig. 9c and d show the monthly streamflow forecasts for all 24 years for the target month of December of forecasts initialized in September (lead time 4). Both ESP and raw (Fig. 9c) and preprocessed (Fig. 9d) forecasts are shown, along with their respective skill scores of accuracy, when the comparison is made against observed (CRPSS) and simulated (CRPSS.s) values.
Figure 9c shows two issues. First, the large hydrological model bias causes ESP to have a deviation from the observations, leading to a high CRPS for the reference forecast in Eq. (4). Secondly, for the winter months, precipitation from the raw ECMWF System 4 forecasts exhibits a negative bias of around −25 % (Lucatero et al., 2017). This compensates the biased streamflow forecasts and results in a low CRPSSys value in Eq. (4). The CRPSS then becomes positive and large (0.54). However, when the comparison is done against simulated values, the skill score becomes highly negative (CRPSS.s = −0.41). Once the biases in the forcings are removed (Fig. 9d), then the hydrological model bias takes over, leading these forecasts to the same level as the ESP, increasing its CRPS, which in turn reduces the skill score (CRPSS = −0.04).
Note that the opposite situation arises, i.e., “the wrong forecast for the wrong reason”, when the hydrological model error is small and precipitation forecast bias is large. Biases in precipitation forecasts will propagate through streamflow forecasts, leading to a streamflow bias of equal sign and of similar magnitude as the precipitation bias. The streamflow bias is then reduced when the meteorological forecast bias is removed (Fig. 5, second and third row). This situation appears during summer or autumn (Fig. 5, first row), when hydrological model errors are smaller than in winter.
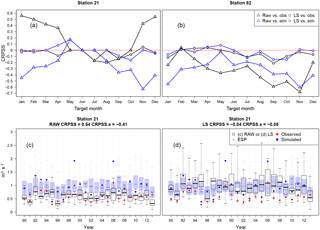
Figure 9(a, b) Skill of accuracy (CRPSS) for upstream station 21 and outlet station 82 for target months January–December at a lead time of 4. Triangles and circles represent the forecasts generated using raw ECMWF System 4 forcings and preprocessed with LS, respectively, whereas black and blue lines represent the comparison against observed and simulated streamflow, respectively. The second row shows the monthly forecasts of December streamflow initialized in September (4-month lead time) for predictions using raw (c) and preprocessed (d) forcings for all years in the record (1990–2013) for station 21.
The apparent skill trend along target months of raw GCM-based streamflow forecasts (Fig. 5, first row) is a product of the above explained error interactions, rather than the existence (or lack) of predictability during the given months. Further analysis linking concurrent and/or previous hydrometeorological processes (i.e., accumulation of snowpack) to streamflow forecast skill would require additional research as discussed later. Moreover, the preprocessed meteorological forecasts' bias is invariant along lead time, and in terms of forecast accuracy, only mild improvements are found over ensemble climatology during the first month lead time (Lucatero et al., 2017). This situation, together with the reduction of error interactions negatively affecting streamflow forecasts at longer leads, produces the flattening of the trend in skill along lead time (Fig. 5, second and third row).
Stations like 21 could benefit the most from post-processing, removing hydrological model biases that calibration alone could not remove. This is illustrated with the visualization of the CRPSS of the different forecasts in Fig. 10a–d. The comparison is made against observations. Figure 10b shows a reduction of skill after raw forcings have been preprocessed, as a result of the compensation errors discussed above. However, once the hydrological biases are removed with post-processing (Fig. 10c and d), the skill is positive and significant throughout November to April. Note, however, that the high skill at this particular station is mainly driven by the poor performance of the reference ESP, due to the large bias of the hydrological model (Fig. 4). It is also worth noting the lack of differences in skill between Fig. 10c and d, showing that, for this particular location, a combination of preprocessing plus post-processing is just as good as post-processing of the forecasts generated using raw forcings alone.
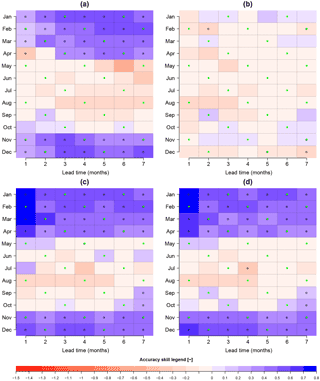
Figure 10CRPSS of station 21 for forecasts generated using raw (a) and preprocessed (b) forcings, in addition to the post-processed streamflow forecasts using the quantile mapping method (QM) for raw meteorological (c) and preprocessed meteorological forcings using the linear scaling/delta change method (d).
3.6 Forecasts of the minimum daily flow within a year
In addition to the evaluation of the monthly streamflow forecasts, we have assessed whether the use of GCM forecasts can add value to the forecasting of annual minimum daily flows compared to ESP. Figure 11 shows forecasts of the minimum daily flow in each year of the study period, considering forecasts issued on 1 April for the next 7 months. Forecasts are for the outlet station 82 for both raw forecasts and the different preprocessing and post-processing strategies. Black box plots represent the forecast generated using the raw outputs of the ECMWF System 4 (Fig. 11a), the preprocessed forecasts (Fig. 11c and e) and the post-processed forecasts (Fig. 11b, d, f). The box plots in the background (blue) represent the ESP forecasts and the red dots represent the yearly observed minimum discharges. When we look at Fig. 10a, several features can be highlighted. First, despite the underprediction of the raw generated forecasts and, to a lesser extent, the ESP forecasts of the highest minimum daily flows in the 2000s, the year-to-year variability is replicated well. Secondly, even though the raw generated forecasts are sharper than the ESP by about 10 % (SS = 0.11), they do not manage to perform better than ESP in terms of skill of accuracy (CRPSS = −0.14); i.e., they are overconfident.
Preprocessing meteorological forecasts seems to have a positive effect on minimum daily flow forecasting, reducing the CRPSS from −0.14 to −0.01 when using the LS preprocessor. This happens because of the loss of sharpness (from 0.11 to −0.11), which allows the forecasts to better capture the higher minimum daily flows during the 00s. However, it is still difficult to outperform the ESP. Post-processing seems to have a similar effect: a loss of sharpness and decrease in bias that allow the forecasts to capture the high minimum daily flows in the 2000s and 2010s. This situation, however, leads to a loss in skill in forecasting minimum daily flows in the 1990s, leveling out the skill to a similar score (CRPSS = −0.12) as the forecasts generated using raw ECMWF forcings (CRPSS = −0.14). Thus, it seems that an attempt to reduce meteorological and hydrological biases through processing the forcings and/or the streamflow will result in only a modest increase in skill of minimum daily flow predictions on average. ESP remains a reference forecast system difficult to outperform.
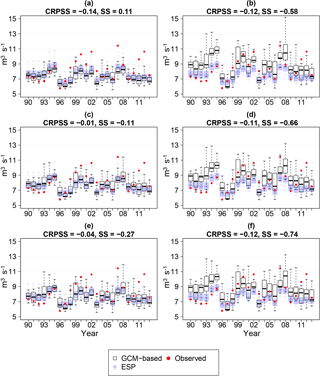
Figure 11Forecasts of minimum daily flows for each year of the period 1990–2013, considering a forecast issued on 1 April for the next 7 months. Forecasts are generated using raw forcings (a), preprocessed forcings with the linear scaling/delta change (c) and the quantile mapping (e) methods and post-processed streamflow for forecasts generated using raw (b) and preprocessed inputs (d and f). Blue shaded box plots are ESP forecasts. CRPSS and SS are computed using Eq. (4) with ESP as reference.
Monthly streamflow forecasts derived for raw, preprocessed meteorology and post-processed streamflow in general show limited skill beyond a 1-month lead time for the Ahlergaarde catchment in Denmark. This is not a surprising result, given the limited skill of meteorological forecasts in the region (Lucatero et al., 2017). Similar results have been documented in Wood et al. (2005), Yuan et al. (2013) and, more recently, Crochemore et al. (2016) for France. GCM-based streamflow forecasts could then be of potential use if the end user is interested in gaining accuracy of forecasts for the next month only. Moreover, we were able to demonstrate that, at least for a groundwater-dominated catchment located in a region with temperate climate, the GCM ability to improve forecasts of minimum daily flows within a year is also limited, regardless of any attempts to correct forcings and/or streamflow forecasts. Further research could focus on the usefulness of GCM forecasts for drought forecasting, i.e., magnitude, duration and severity (Fundel, 2013) in comparison to forecasts generated using the ESP method.
Furthermore, caution must be taken when hydrological model errors are large, as it may lead to erroneous evaluations of skill when hydrological model biases are neutralized by opposite GCM errors, e.g., forecasts of monthly streamflow during the winter in the study region. This is an issue somewhat underexplored in studies of forecast skill and should be evaluated especially when calibration objective functions focus on attributes that differ from the ones looked for in the final forecast quantity of interest, and when no attempts to remove biases in meteorological forecasts are made.
In our study, preprocessing of the forcings alone helped to reduce streamflow biases and reduce the negative skill at longer lead times. The reduction of the under- or overestimation led to forecasts with a higher statistical consistency for most of the months and lead times considered. This rather mild enhancement was also found by Crochemore et al. (2016). Moreover, post-processing alone does a better job in removing biases in the mean, which, in turn, helps to ameliorate issues with the statistical consistency. Ye et al. (2015) and Zalachori et al. (2012) also report the above behavior, whereas Yuan and Wood (2012) found a better correction of statistical consistency after both preprocessing and post-processing. The removal of biases of both forcings and hydrological model did not ensure a higher level of accuracy than the ESP, as demonstrated by the nonsignificant differences of accuracy between GCM-based forecasts and the ESP forecasts. This is also true for forecasts of minimum daily flows in a year, as mentioned above.
The methods used here for preprocessing (LS and QM) and post-processing (QM) were chosen because of their simplicity. However, post-processing in general is a field that has been gaining traction over the last decade, with a variety of methods that differ in their mathematical sophistication. The reader is referred to Li et al. (2017) for a detailed and updated literature review on the subject. Moreover, QM disadvantages have been widely discussed in Zhao et al. (2017) and references therein. The main issue discussed concerns the fact that when the forecast–observation linear relationship is weak, or nonexistent, QM has difficulties creating forecasts that are consistent (i.e., that have skill at least as good as the reference forecast). Other methods could have been used that allow for correction of both statistical consistency together with consistency. However, the benefits of the more sophisticated methods might be dampened due to the limited sample size, which is often the case in hydrometeorological forecasting. Nevertheless, our present study could be extended by analyzing the added skill gained by the increased complexity of processing methods, using the same reforecast dataset, such as the case of Mendoza et al. (2017), although with its application focused on statistical forecasting.
Another obvious omission of the study is the exploitation of storages in the form of snow, soil moisture or/and groundwater and taking advantage of the hydrological memory that may increase skill at longer leads. This has been the routine for snow-dominated catchments in the western United States by means of ESP (Wood and Lettenmaier, 2006). However, a preliminary evaluation of the relationship between groundwater levels in winter and minimum daily flows during the summer in the Ahlergaarde catchment studied here showed that relatively high correlations exist in large parts of the catchment (Jacob Kidmose, personal communication, 2014). This correlation can be further explored in the forecasting mode to extend the positive skill lead time by means of data assimilation (Zhang et al., 2016) or by statistical post-processing of streamflow forecasts (Mendoza et al., 2017). Moreover, predictability attribution studies exist that quantify the sensitivity of the skill of a forecasting system relative to different degrees of uncertainty, either in the forcing or the initial conditions. Wood et al. (2016) developed a framework to detect where to concentrate on improvements, e.g., either the initial conditions, usually by means of data assimilation (Zhang et al., 2016), or the seasonal climate forecasts. This might shed light on, and possibly reinforce, the hypothesis that for groundwater-dominated catchments and forecasting of low flows, initial conditions will have a higher influence on forecast skill at longer lead times (Paiva et al., 2012; Fundel et al., 2013).
Seasonal forecasts of streamflows initiated in each calendar month for the 1990–2013 period were generated for a groundwater-dominated catchment located in a region where seasonal atmospheric forecasting is a challenge. We analyzed the bias and statistical consistency of monthly streamflow forecasts forced with ECMWF System 4 seasonal forecasts along all calendar months throughout the year. In addition to this, we evaluated their accuracy and sharpness relative to that of the forecasts generated using an ensemble of historical meteorological observations, the ESP. Monthly streamflow forecasts generated using raw ECMWF System 4 forcings show skill only during the winter months in the first month lead time. Nevertheless, it was shown that the apparent large skill can be an effect of compensational errors between meteorological forecasts and the hydrological model. Due to biases of GCM-based meteorological seasonal forecasts and errors in the hydrological model that calibration alone cannot defuse, both preprocessing and post-processing using two popular and simple correction techniques were used to remove them: LS and QM. Finally, we also estimated the skill that the different forecast generation approaches have on forecasting the minimum yearly daily discharge. Our results show that post-processing streamflow allows for the most gain in skill and statistical consistency. However, monthly streamflow and annual minimum daily discharge forecasts generated using forcings from GCM still show difficulties in outperforming ESP forecasts, especially at lead times longer than 1 month.
ECMWF seasonal reforecasts are available under a range of licences; for more information visit http://www.ecmwf.int (last access: 27 June 2018). The hydrological model forcing data (temperature, precipitation and reference evapotranspiration) are from the Danish Meteorological Institute (https://www.dmi.dk/vejr/arkiver/vejrarkiv/, last access: 27 June 2018). The streamflow data are available on the HOBE data platform (http://www.hobe.dk/index.php/data/live-data, last access: 27 June 2018). A more detailed description of the data usage can be found on the Hydrocast project website (http://hydrocast.dhigroup.com/, last access: 27 June 2018) and the HOBE project website (http://hobe.dk/, last access: 27 June 2018).
The authors declare that they have no conflict of interest.
This article is part of the special issue “Sub-seasonal to seasonal hydrological forecasting”. It is not associated with a conference.
This study was supported by the project “HydroCast – Hydrological
Forecasting and Data Assimilation”, contract no. 0603-00466B
(http://hydrocast.dhigroup.com/, 27 June 2018), funded by the
Innovation Fund Denmark. Special thanks to Florian Pappenberger for providing
the ECMWF System 4 reforecast and Andy Wood and Pablo Mendoza for hosting the
first author at NCAR. We also express our gratitude to Massimiliano Zappa and
one anonymous reviewer for their comments that improved the quality of this
paper.
Edited by: Maria-Helena
Ramos
Reviewed by: Massimiliano Zappa and one anonymous referee
Bogner, K., Liechti, K., and Zappa, M.: Post-processing of stream flows in Switzerland with an emphasis on low flows and floods, Water (Switzerland), 8, 115, https://doi.org/10.3390/w8040115, 2016.
Bruno Soares, M. and Dessai, S.: Barriers and enablers to the use of seasonal climate forecasts amongst organisations in Europe, Clim. Change, 137, 89–103, https://doi.org/10.1007/s10584-016-1671-8, 2016.
Córdoba-Machado, S., Palomino-Lemus, R., Gámiz-Fortis, S. R., Castro-Díez, Y., and Esteban-Parra, M. J.: Seasonal streamflow prediction in Colombia using atmospheric and oceanic patterns, J. Hydrol., 538, 1–12, https://doi.org/10.1016/j.jhydrol.2016.04.003, 2016.
Crochemore, L., Ramos, M.-H., and Pappenberger, F.: Bias correcting precipitation forecasts to improve the skill of seasonal streamflow forecasts, Hydrol. Earth Syst. Sci., 20, 3601–3618, https://doi.org/10.5194/hess-20-3601-2016, 2016.
D'Agostino, R. B. and Stephens, A. M.: Goodness-of-fit techniques, Dekker, New York, 1986.
Day, G. N.: Extended stream flow forecasting Using NWSRFS, J. Water Res. Pl., 111, 157–170, 1985.
Demirel, M. C., Booij, M. J., and Hoekstra, A. Y.: The skill of seasonal ensemble low-flow forecasts in the Moselle River for three different hydrological models, Hydrol. Earth Syst. Sci., 19, 275–291, https://doi.org/10.5194/hess-19-275-2015, 2015.
Doherty, J.: PEST, Model-independent parameter estimation, User manual: 5th Edn., Watermark Numerical Computing, 2010.
Friederichs, P. and Thorarinsdottir, T. L.: Forecast verification for extreme value distributions with an application to probabilistic peak wind prediction, Environmetrics, 23, 579–594, https://doi.org/10.1002/env.2176, 2012.
Fundel, F., Jörg-Hess, S., and Zappa, M.: Monthly hydrometeorological ensemble prediction of streamflow droughts and corresponding drought indices, Hydrol. Earth Syst. Sci., 17, 395–407, https://doi.org/10.5194/hess-17-395-2013, 2013.
Graham, D. N. and Butts, M. B.: Flexible, integrated watershed modelling with MIKE SHE, in: Watershed Models, edited by: Singh, V. P. and Frevert D. K., 245–272, CRC Press, Florida, 2005.
Hendriks, M.: Introduction to Physical Hydrology, Oxford University Press, Oxford, 2010.
Hersbach, H.: Decomposition of the Continuous Ranked Probability Score for Ensemble Prediction Systems, Weather Forecast., 15, 559–570, https://doi.org/10.1175/1520-0434(2000)015<0559:DOTCRP>2.0.CO;2, 2000.
Hollander, M., Wolfe, D. A., and Chicken, E.: Nonparametric statistical methods, 3 Edn., Wiley Series in Probability and Statistics, Hoboken, New Jersey, 2014.
Jaun, S., Ahrens, B., Walser, A., Ewen, T., and Schär, C.: A probabilistic view on the August 2005 floods in the upper Rhine catchment, Nat. Hazards Earth Syst. Sci., 8, 281–291, https://doi.org/10.5194/nhess-8-281-2008, 2008.
Jensen, K. H. and Illangasekare, T. H.: HOBE: A Hydrological Observatory, Vadose Zone J., 10, 1–7, https://doi.org/10.2136/vzj2011.0006, 2011.
Laio, F. and Tamea, S.: Verification tools for probabilistic forecasts of continuous hydrological variables, Hydrol. Earth Syst. Sci., 11, 1267–1277, https://doi.org/10.5194/hess-11-1267-2007, 2007.
Li, W., Duan, Q., Miao, C., Ye, A., Gong, W., and Di, Z.: A review on statistical postprocessing methods for hydrometeorological ensemble forecasting, WIREs Water, 4, e1246, https://doi.org/10.1002/wat2.1246, 2017.
Lucatero, D., Madsen, H., Refsgaard, J. C., Kidmose, J., and Jensen, K. H.: On the skill of raw and postprocessed ensemble seasonal meteorological forecasts in Denmark, Hydrol. Earth Syst. Sci. Discuss., https://doi.org/10.5194/hess-2017-366, in review, 2017.
Madadgar, S., Moradkhani, H., and Garen, D.: Towards improved post-processing of hydrologic forecast ensembles, Hydrol. Process., 28, 104–122, https://doi.org/10.1002/hyp.9562, 2014.
Mendoza, P. A., Wood, A. W., Clark, E., Rothwell, E., Clark, M. P., Nijssen, B., Brekke, L. D., and Arnold, J. R.: An intercomparison of approaches for improving operational seasonal streamflow forecasts, Hydrol. Earth Syst. Sci., 21, 3915–3935, https://doi.org/10.5194/hess-21-3915-2017, 2017.
Molteni, F., Stockdale, T., Balmaseda, M., Balsamo, G., Buizza, R., Ferranti, L., Magnusson, L., Mogensen, K., Palmer, T., and Vitart, F.: The new ECMWF seasonal forecast system (System 4), ECMWF Technical Memorandum 656, November, 49, 2011.
Olsson, J., Uvo, C. B., Foster, K., and Yang, W.: Technical Note: Initial assessment of a multi-method approach to spring-flood forecasting in Sweden, Hydrol. Earth Syst. Sci., 20, 659–667, https://doi.org/10.5194/hess-20-659-2016, 2016.
Paiva, R. C. D., Collischonn, W., Bonnet, M. P., and de Gonçalves, L. G. G.: On the sources of hydrological prediction uncertainty in the Amazon, Hydrol. Earth Syst. Sci., 16, 3127–3137, https://doi.org/10.5194/hess-16-3127-2012, 2012.
Robertson, D. E. and Wang, Q. J.: A Bayesian Approach to Predictor Selection for Seasonal Streamflow Forecasting, J. Hydrometeorol., 13, 155–171, https://doi.org/10.1175/JHM-D-10-05009.1, 2012.
Robertson, D. E., Pokhrel, P., and Wang, Q. J.: Improving statistical forecasts of seasonal streamflows using hydrological model output, Hydrol. Earth Syst. Sci., 17, 579–593, https://doi.org/10.5194/hess-17-579-2013, 2013.
Rosenberg, E. A., Wood, A. W., and Steinemann, A. C.: Statistical applications of physically based hydrologic models to seasonal streamflow forecasts, Water Resour. Res., 47, 3, https://doi.org/10.1029/2010WR010101, 2011.
Roulin, E. and Vannitsem, S.: Post-processing of medium-range probabilistic hydrological forecasting: Impact of forcing, initial conditions and model errors, Hydrol. Process., 29, 1434–1449, https://doi.org/10.1002/hyp.10259, 2015.
Scharling, M. and Kern-Hansen, C.: Climate Grid Denmark – Dateset for use in research and education, DMI Tech. Rep., 1–12, available at: https://www.dmi.dk/vejr/arkiver/ vejrarkiv/ (last access: 27 June 2018), 2012.
Schepen, A., Zhao, T., Wang, Q. J., Zhou, S., and Feikema, P.: Optimising seasonal streamflow forecast lead time for operational decision making in Australia, Hydrol. Earth Syst. Sci., 20, 4117–4128, https://doi.org/10.5194/hess-20-4117-2016, 2016.
Shamir, E.: The value and skill of seasonal forecasts for water resources management in the Upper Santa Cruz River basin, southern Arizona, J. Arid Environ., 137, 35–45, https://doi.org/10.1016/j.jaridenv.2016.10.011, 2017.
Shi, X., Wood, A. W., and Lettenmaier, D. P.: How Essential is Hydrologic Model Calibration to Seasonal Streamflow Forecasting?, J. Hydrometeorol., 9, 1350–1363, https://doi.org/10.1175/2008JHM1001.1, 2008.
Stisen, S., Sonnenborg, T. O., Højberg, A. L., Troldborg, L., and Refsgaard, J. C.: Evaluation of Climate Input Biases and Water Balance Issues Using a Coupled Surface-Subsurface Model, Vadose Zone J., 10, 37–53, https://doi.org/10.2136/vzj2010.0001, 2011.
Stisen, S., Højberg, A. L., Troldborg, L., Refsgaard, J. C., Christensen, B. S. B., Olsen, M., and Henriksen, H. J.: On the importance of appropriate precipitation gauge catch correction for hydrological modelling at mid to high latitudes, Hydrol. Earth Syst. Sci., 16, 4157–4176, https://doi.org/10.5194/hess-16-4157-2012, 2012.
Trambauer, P., Werner, M., Winsemius, H. C., Maskey, S., Dutra, E., and Uhlenbrook, S.: Hydrological drought forecasting and skill assessment for the Limpopo River basin, southern Africa, Hydrol. Earth Syst. Sci., 19, 1695–1711, https://doi.org/10.5194/hess-19-1695-2015, 2015.
Verkade, J. S., Brown, J. D., Reggiani, P., and Weerts, A. H.: Post-processing ECMWF precipitation and temperature ensemble reforecasts for operational hydrologic forecasting at various spatial scales, J. Hydrol., 501, 73–91, https://doi.org/10.1016/j.jhydrol.2013.07.039, 2013.
Wang, Q. J., Robertson, D. E., and Chiew, F. H. S.: A Bayesian joint probability modeling approach for seasonal forecasting of streamflows at multiple sites, Water Resour. Res., 45, 1–18, https://doi.org/10.1029/2008WR007355, 2009.
Weisheimer, A. and Palmer, T. N.: On the reliability of seasonal climate forecasts, J. R. Soc. Interface, 11, 20131162, https://doi.org/10.1098/rsif.2013.1162, 2014.
Wood, A. W. and Lettenmaier, D. P.: A test bed for new seasonal hydrologic forecasting approaches in the western United States, B. Am. Meteorol. Soc., 87, 1699–1712, https://doi.org/10.1175/BAMS-87-12-1699, 2006.
Wood, A. W. and Schaake, J. C.: Correcting Errors in Streamflow Forecast Ensemble Mean and Spread, J. Hydrometeorol., 9, 132–148, https://doi.org/10.1175/2007JHM862.1, 2008.
Wood, A. W., Maurer, E. P., Kumar, A., and Lettenmaier, D. P.: Long-range experimental hydrologic forecasting for the eastern United States, J. Geophys. Res.-Atmos., 107, 1–15, https://doi.org/10.1029/2001JD000659, 2002.
Wood, A. W., Kumar, A., and Lettenmaier, D. P.: A retrospective assessment of National Centers for Environmental prediction climate model-based ensemble hydrologic forecasting in the western United States, J. Geophys. Res.-Atmos., 110, 1–16, https://doi.org/10.1029/2004JD004508, 2005.
Wood, A. W., Hopson, T., Newman, A., Brekke, L., Arnold, J., and Clark, M.: Quantifying Streamflow Forecast Skill Elasticity to Initial Condition and Climate Prediction Skill, J. Hydrometeorol., 17, 651–668, https://doi.org/10.1175/JHM-D-14-0213.1, 2016.
Yapo, P. O., Gupta, H. V., and Sorooshian, S.: Automatic calibration of conceptual rainfall-runoff models: sensitivity to calibration data, J. Hydrol., 181, 23–48, https://doi.org/10.1016/0022-1694(95)02918-4, 1996.
Ye, A., Duan, Q., Schaake, J., Xu, J., Deng, X., Di, Z., Miao, C., and Gong, W.: Post-processing of ensemble forecasts in low-flow period, Hydrol. Process., 29, 2438–2453, https://doi.org/10.1002/hyp.10374, 2015.
Yuan, X.: An experimental seasonal hydrological forecasting system over the Yellow River basin – Part 2: The added value from climate forecast models, Hydrol. Earth Syst. Sci., 20, 2453–2466, https://doi.org/10.5194/hess-20-2453-2016, 2016.
Yuan, X. and Wood, E. F.: Downscaling precipitation or bias-correcting streamflow? Some implications for coupled general circulation model (CGCM)-based ensemble seasonal hydrologic forecast, Water Resour. Res., 48, 1–7, https://doi.org/10.1029/2012WR012256, 2012.
Yuan, X., Wood, E. F., Luo, L., and Pan, M.: A first look at Climate Forecast System version 2 (CFSv2) for hydrological seasonal prediction, Geophys. Res. Lett., 38, 1–7, https://doi.org/10.1029/2011GL047792, 2011.
Yuan, X., Wood, E. F., Roundy, J. K., and Pan, M.: CFSv2-Based seasonal hydroclimatic forecasts over the conterminous United States, J. Clim., 26, 4828–4847, https://doi.org/10.1175/JCLI-D-12-00683.1, 2013.
Yuan, X., Roundy, J. K., Wood, E. F., and Sheffield, J.: Seasonal forecasting of global hydrologic extremes: System development and evaluation over GEWEX basins, B. Am. Meteorol. Soc., 96, 1895–1912, https://doi.org/10.1175/BAMS-D-14-00003.1, 2015.
Zalachori, I., Ramos, M.-H., Garçon, R., Mathevet, T., and Gailhard, J.: Statistical processing of forecasts for hydrological ensemble prediction: a comparative study of different bias correction strategies, Adv. Sci. Res., 8, 135–141, https://doi.org/10.5194/asr-8-135-2012, 2012.
Zhang, D., Madsen, H., Ridler, M. E., Kidmose, J., Jensen, K. H., and Refsgaard, J. C.: Multivariate hydrological data assimilation of soil moisture and groundwater head, Hydrol. Earth Syst. Sci., 20, 4341–4357, https://doi.org/10.5194/hess-20-4341-2016, 2016.
Zhao, L., Duan, Q., Schaake, J., Ye, A., and Xia, J.: A hydrologic post-processor for ensemble streamflow predictions, Adv. Geosci., 29, 51–59, https://doi.org/10.5194/adgeo-29-51-2011, 2011.
Zhao, T., Bennett, J., Wang, Q. J., Schepen, A., Wood, A., Robertson D., and Ramos, M.-H.: How suitable is quantile mapping for postprocessing GCM precipitation forecasts? J. Clim., 30, 3185–3196. https://doi.org/10.1175/JCLI-D-16-0652.1, 2017.