the Creative Commons Attribution 4.0 License.
the Creative Commons Attribution 4.0 License.
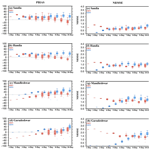
Ensemble streamflow prediction considering the influence of reservoirs in Narmada River Basin, India
Urmin Vegad
Developing an ensemble hydrological prediction system is essential for reservoir operations and flood early warning. However, efforts to build hydrological ensemble prediction systems considering the influence of reservoirs have been lacking in India. We examine the potential of the Extended Range Forecast System (ERFS, 16 ensemble members) and Global Ensemble Forecast System (GEFS, 21 ensemble members) forecast for streamflow prediction in India using the Narmada River Basin as a test bed. We use the variable infiltration capacity (VIC) with reservoir operations (VIC-Res) scheme to simulate the daily river flow at four locations in the Narmada Basin. Streamflow prediction skills of the ERFS forecast were examined for the period 2003–2018 at 1–32 d lead. We compared the streamflow forecast skills of raw meteorological forecasts from ERFS and GEFS at a 1–10 d lead for the summer monsoon (June–September) 2019–2020. The ERFS forecast underestimates extreme precipitation against the observations compared to the GEFS forecast during the summer monsoon of 2019–2020. However, both forecast products show better skills for minimum and maximum temperatures than precipitation. Ensemble streamflow forecast from the GEFS performs better than the ERFS during 2019–2020. The performance of GEFS-based ensemble streamflow forecast declines after 5 days lead. Overall, the GEFS ensemble streamflow forecast can provide reliable skills at a 1–5 d lead, which can be utilized in streamflow prediction. Our findings provide directions for developing a flood early warning system based on ensemble streamflow prediction considering the influence of reservoirs in India.
- Article
(8837 KB) - Full-text XML
-
Supplement
(1341 KB) - BibTeX
- EndNote
Floods are one of India's most destructive and frequently occurring natural disasters. Floods accounted for about 47 % of natural disasters in India during the last 100 years (Tripathi, 2015). Riverine floods occur during the summer monsoon season affecting approximately 5 million people annually (Luo et al., 2015). Singh and Kumar (2013) reported an increase in the frequency of floods in India. About 20 % of the total flood-prone area gets affected every year (Ray et al., 2019). Floods in 2018 caused an economic loss of more than 12 billion dollars (USD) and resulted in the loss of 1808 lives (Joshi, 2020). In addition, climate warming is projected to increase the frequency and intensity of riverine floods (Field et al., 2011; Luo et al., 2015; Nanditha and Mishra, 2022; Ali et al., 2019).
Preparedness for disasters like floods can help in mitigating economic loss and reducing flood mortality (Jain et al., 2018). While losses due to floods are projected to rise under the warming climate, human mortality can be reduced with flood early warning systems and effective communication (Dipti, 2017; Nanditha and Mishra, 2021). Therefore, developing a robust flood prediction system is necessary for early warning and preparedness. Streamflow prediction is an essential component of flood forecasting, which helps in planning and decision making (Georgakakos et al., 2012; Alfieri et al., 2013). Most of the streamflow prediction systems in India are based on the deterministic approach (Harsha, 2020a; Todini, 2017; Nanditha and Mishra, 2021), which do not account for perturbations in initial conditions to quantify the uncertainty (Bowler et al., 2008). Uncertainty quantification in streamflow prediction can reduce the risk of false alarms based on deterministic forecast (Todini, 2017). In addition, ensemble streamflow prediction is essential for the probabilistic flood forecast. The probabilistic approach performs better than the deterministic approach by quantifying uncertainties associated with flood prediction and early warning systems (Krzysztofowicz, 2001). Previous studies used ensemble streamflow prediction in flood forecasting (Cloke and Pappenberger, 2009; Wu et al., 2020) using ensemble meteorological forecast and hydrological models (Zhang et al., 2020). Ensemble weather forecast provides multiple members at the same location and time that can be used for probabilistic hydrological prediction. However, several challenges are associated with the operational ensemble streamflow forecast, including computational limitations, explanation of ensemble forecasts to non-experts, and up-gradation in the policy to use the forecast for decision making (Demeritt et al., 2010; Arnal et al., 2020). Despite these challenges, ensemble flood forecasts consider the uncertainty that can be used for preparedness and planning compared to the deterministic forecast approach (Pappenberger et al., 2012; Cloke and Pappenberger, 2009).
Indian river basins are considerably affected by human interventions including the presence of reservoirs, water withdrawal for irrigation, and interbasin and intrabasin water transfer (Nanditha and Mishra, 2021; Madhusoodhanan et al., 2016; Gosain et al., 2006). India has more than 5000 large dams while about 450 are currently under construction (NRLD, 2017). Reservoirs and irrigation can considerably modulate terrestrial water and energy budgets in India (Shah et al., 2019). For instance, Shah et al. (2019) showed that evapotranspiration and latent heat flux are increased under the presence of irrigation and reservoirs in Indian river basins compared to their natural conditions. Dong et al. (2022) reported that reservoirs can significantly (∼ 25 %) contribute to the variation of terrestrial water storage in China. In addition, the presence of reservoirs can considerably affect streamflow variability in the downstream regions (Zajac et al., 2017; Yun et al., 2020; Chai et al., 2019). Reservoirs in India are multipurpose as these store water for the dry season, generate hydropower, and attenuate floods in the downstream regions (Tiwari and Mishra, 2022). Reservoirs store water during the summer monsoon season and release water during the dry season for irrigation. Similarly, based on the reservoir rule curve, a buffer storage is kept during the wet season to accommodate high inflow so that flood risk can be minimized in the downstream region. Therefore, there are several challenges associated with the streamflow forecast in the river basins that are affected by reservoirs. Most often hydrological model-based flood and streamflow forecasts do not consider the influence of reservoirs that could lead to underestimation or overestimation of flow depending on the season (Nanditha and Mishra, 2021; Dang et al., 2020a). Incorporating reservoir influence in hydrological models is essential as reservoirs significantly affect the magnitude and timing of streamflow (Zajac et al., 2017; Yassin et al., 2019; Dang et al., 2020a). Several efforts have been made to incorporate the influence of reservoirs in the hydrological models (Boulange and Hanasaki, 2013; Dang et al., 2020a; Hanasaki et al., 2018). However, most of the previous studies on flood forecasts and early warnings in India did not consider the influence of reservoirs (Goswami et al., 2018; Sikder and Hossain, 2019).
The Central Water Commission (CWC) manages flood forecast systems in India. The flood forecast network monitors 325 stations across India. The CWC observes real-time water levels and discharges along the major rivers of India during the designated flood period. The flood forecast is performed using statistical correlation methods from gauge to gauge. Moreover, quantitative precipitation forecast (QPF) from the India Meteorological Department (IMD) is used to forecast floods at a 3 d lead time (Teja and Umamahesh, 2020). The current model-based flood forecast approach used by CWC is deterministic, which lacks the incorporation of uncertainties in the forecast and early warning systems. An ensemble forecast system can help in flood early warning and decision making (Harsha, 2020b; Nanditha and Mishra, 2021). Various ensemble forecast products are available from the IMD and the Indian Institute of Tropical Meteorology (IITM). However, the utility of these forecast products for streamflow prediction and flood early warning at the river basin scale has not been examined. In addition, despite the advantages of ensemble hydrological prediction, India's current hydrological forecast systems are mainly deterministic. Given the increasing flood damage in India, the overarching aim of this work is to explore the utility of ensemble forecast products for streamflow prediction in India. We considered the Narmada River basin as a test bed to examine the potential of ensemble hydrological prediction. We used the variable infiltration capacity (VIC) with reservoir operations (VIC-Res) scheme, which incorporates the effect of reservoirs (Dang et al., 2020a). Extended Range Forecast System (ERFS) and Global Ensemble Forecast System (GEFS) ensemble forecasts developed by the IITM are used to examine the hydrological prediction skills at the selected gauge stations in the Narmada basin.
2.1 Study region and datasets
The Narmada is the fifth biggest and the largest west-flowing river in India. The Narmada River Basin falls in two states, Gujarat and Madhya Pradesh. Many tributaries contribute to the river through its way to the Arabian Sea, with the Tawa River being its longest tributary. The catchment area of the river basin at the outlet is approximately 98 796 km2. The upper portion of the basin falls in Madhya Pradesh. The mean annual rainfall in the Narmada Basin is 1064 mm. Most of the total annual precipitation occurs during the summer monsoon season (June–September). We used observed daily streamflow at four stations: Sandia, Handia, Mandleshwar, and Garudeshwar (Fig. 1). There are several ongoing hydropower and irrigation projects in the Narmada Basin. Our hydrological modeling framework has considered four dams: Bargi, Tawa, Indira Sagar, and Sardar Sarovar (Table 1). The Bargi and Tawa reservoirs were primarily constructed for irrigation purposes (Table 1). At the same time, Indira Sagar (0.975 billion cubic meters, BCM) and Sardar Sarovar (5.8 BCM) are the two largest reservoirs that are used for multiple purposes.
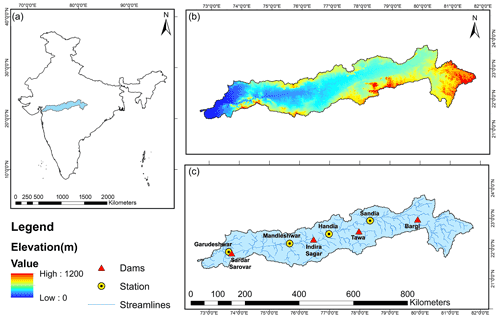
Figure 1Basic information about (a) location in India, (b) topography, (c) streamlines, location of streamflow gauge stations and reservoirs.
We used 0.25∘ (approximate spatial resolution; ∼ 27.5 × 27.5 km) gridded daily precipitation from IMD for the 1951–2020 period (Pai et al., 2014). The daily gridded precipitation product is developed using observations from 6955 rain gauge stations (Pai et al., 2015). Pai et al. (2015) examined daily rainfall trends, long-term climatology, and variability over the central Indian region. The high resolution (0.25∘) gridded precipitation captures spatial variability in better manner compared to previous coarse-gridded rainfall products. We obtained daily 1∘ gridded maximum and minimum temperatures from IMD (Srivastava et al., 2009). Srivastava et al. (2009) developed the gridded temperature dataset using observations from 395 stations. We used bilinear interpolation to convert the 1∘ gridded temperature to 0.25∘ resolution to make it consistent with the gridded precipitation. The VIC model also requires daily wind speed as an input. We obtained the wind speed from the National Centers for Environmental Prediction (NCEP)-National Center for Atmospheric Research (NCAR) (https://psl.noaa.gov/data/gridded/data.ncep.reanalysis.pressure.html, last access: 4 October 2022). The wind speed at a coarser (1.875∘ × 1.905∘) resolution was interpolated using bilinear interpolation to 0.25∘ to make it consistent with the other meteorological datasets. The VIC model vegetation parameters were obtained from the Advanced Very High-Resolution Radiometer (AVHRR) global land cover, which is available at 1 km spatial resolution (Sheffield and Wood, 2007). Soil parameters at 0.25∘ were developed using the Harmonized World Soil Database (HWSD version 1.2) (Gao et al., 2010). We used digital elevation model data from Shuttle Radar Topography Mission (SRTM) at 90 m spatial resolution (Jarvis, 2008). The hydrological model considers sub-grid variability of topography and vegetation (Gao et al., 2010). Therefore, the high-resolution vegetation and elevation datasets were used to extract values for different tiles within a grid.
We obtained observed daily streamflow, reservoir water level, and reservoir live storage data from the India Water Resources Information System (IWRIS; https://indiawris.gov.in/wris/, last access: 4 October 2022), which is a joint venture of the CWC, the Ministry of Jal Shakti, and the Indian Space Research Organization (ISRO). Streamflow and reservoir levels are monitored at various locations in the Narmada Basin by CWC. We selected the gauge stations (Sandia, Handia, Mandleshwar, and Garudeshwar) that have observed flow data for at least 15 years. The reservoir storage and water level data were obtained for different periods depending on the data availability.
We obtained the ERFS meteorological forecast for the 2003–2020 period. In addition, GEFS meteorological forecast was obtained for the summer monsoon season (July–September) of 2019–2020 from the IITM. Both the ERFS and GEFS forecast products are developed at IITM and are currently being used for the operational weather forecast by the IMD. In June 2018, the high-resolution GEFS forecast was developed and then transferred to the IMD for operational forecasting (Mukhopadhyay et al., 2018). The GEFS dataset has a horizontal resolution of T1534 (∼ 12.5 km) and consists of 21 ensemble members (1 control and 20 perturbed). The dynamic core of the model is based on semi-Lagrangian framework, which reduces considerable computational requirements. The initial conditions (IC) for meteorological forecasts are obtained from Global Data Assimilation System (GDAS). The GEFS is being run operationally for the 10-day lead forecast using daily ICs during the summer monsoon period. The GEFS forecast successfully predicted the 2018 Kerala extreme rainfall at 2–3 d lead and showed reasonable forecast skills at 5–7 d lead (Mukhopadhyay et al., 2018).
The ERFS multimodel system consists of four (CFSv2T382, CFSv2T126, GFSbcT382 and GFSbcT126) suites, each having four ensemble members (one control and three perturbed). Therefore, 16 ensemble members are available for the ERFS forecast. The model is being run operationally for 32 d lead based on the initial conditions of every Wednesday. Atmospheric and oceanic initial conditions from the National Center for Medium-Range Weather Forecasting (NCMWRF) and the Indian National Centre for Ocean Information Services (INCOSIS) assimilation system are used by the models in ERFS. We used the 16 ensemble meteorological forecasts to simulate the daily streamflow at 1–32 d leads at selected stations in the Narmada River Basin. Shah et al. (2017) reported that ERFS performed better than the Global Ensemble Forecast System v2 (GEFSv2) and Climate Forecast System v2 (CFSv2) in precipitation forecast during the summer monsoon season over India.
2.2 The VIC-Res hydrological model
We used the VIC-Res hydrological model (Dang et al., 2020a), a novel variant of the VIC model (Liang et al., 1994), to simulate streamflow. A combination of the VIC model and the routing model developed by Dang et al. (2020a) was used to simulate streamflow at the selected locations in the basin. Dang et al. (2020a) incorporated the effect of reservoirs by considering the reservoir storage dynamics and operating rules within the streamflow routing model in the VIC-Res model. The rainfall-runoff model generates water and energy fluxes within each grid using climate forcing, soil parameters, land use and land cover, and the digital elevation model. The model uses vegetation cover for each tile and three soil layers for each grid cell. The upper two soil layers control runoff, infiltration, and evaporation, while the bottom layer governs baseflow. The routing model uses water fluxes (runoff and baseflow) from each grid to simulate streamflow at selected gauge stations using the linearized Saint-Venant equations. The routing model uses flow direction, fractional area within a grid, and station location as input to generate streamflow. In addition, the VIC-Res model requires reservoir parameters and location as inputs. The reservoir parameters include full reservoir level (FRL), dead water level, storage capacity, dead storage, rated head, and the year when the reservoir became operational. The VIC-Res considers a grid as a reservoir and the incoming streamflow to that reservoir is considered as the inflow. In addition to the reservoir parameters, the observed seasonal cycle is also required as input to the routing scheme. The model implements mass balance equations at each time step to calculate storage, outflow and release from the reservoir. The VIC-Res model simulates daily reservoir inflow, outflow, live storage, and water level. Dang et al. (2020a) reported that even the model without a reservoir exhibits almost the same level of accuracy. However, as the parameterization is inappropriate when the model is calibrated using the observed flow that is affected by reservoirs, hydrological processes simulated by the model can be erroneous.
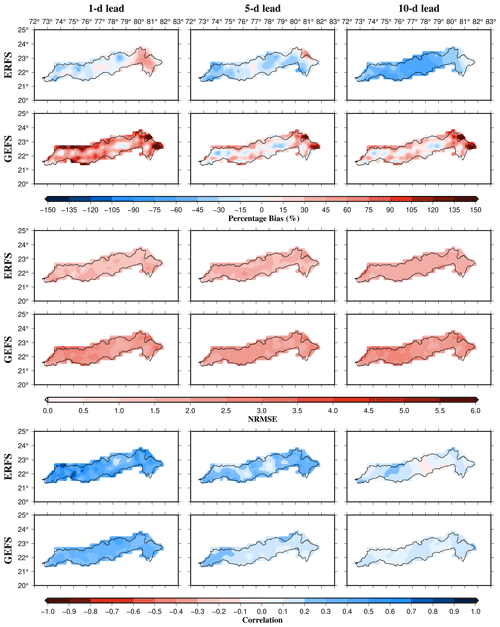
Figure 4Comparison of the precipitation forecast skills from ERFS and GEFS for the summer monsoon period during 2019–2020. Forecast skills were evaluated using bias, NRMSE, and correlation for each ensemble member of ERFS and GEFS and the median skill is presented.
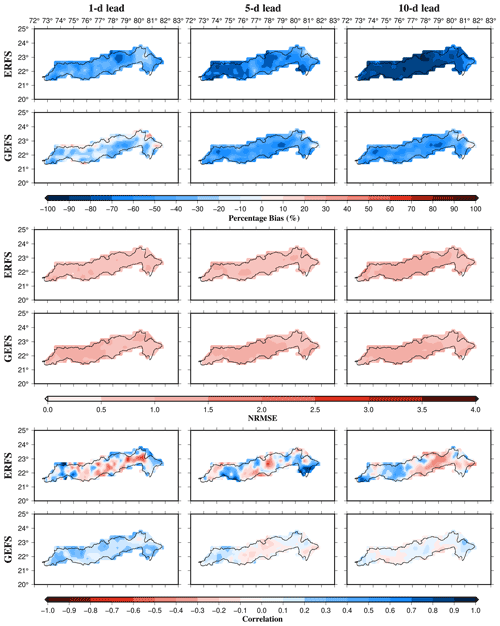
Figure 5Comparison of the extreme precipitation (exceeding 75th percentile) forecast skills from ERFS and GEFS for the summer monsoon period during 2019–2020. Forecast skills were evaluated using bias, NRMSE, and correlation for each ensemble member of ERFS and GEFS and the median skill is presented.
We used observed daily precipitation, maximum and minimum temperatures from IMD, and wind speed from NCEP-NCAR reanalysis as meteorological forcing. We used reservoir storage observations to input the seasonal cycle for each reservoir into the model. An autocalibration module developed by Dang et al. (2020b) was used to calibrate soil parameters of the VIC-Res model for the Narmada River Basin. The autocalibration module uses the ε-NSGAII multiobjective evolutionary algorithm (Reed et al., 2013) to adjust the values of sensitive soil parameters. The autocalibration module can be used to calibrate model parameters at the outlet of different sub-basins within a river basin. First, we used autocalibration to calibrate parameters of upstream basins, then the parameters for the downstream basins were calibrated for the grids that are not part of the upstream basins. We used five soil parameters (Binf, Ds, Dsmax, Ws, and depth of three soil layers) to calibrate daily streamflow at the selected gauge stations in the basin as described in Mishra et al. (2010), where Binf is the variable infiltration curve parameter, Dsmax is the maximum velocity of baseflow, Ds is a fraction of Dsmax where non-linear baseflow begins and Ws is a fraction of maximum soil moisture where non-linear baseflow occurs (Liang et al., 1994). Further details of the calibration parameters can be obtained from Mishra et al. (2010). The autocalibration module optimizes the model's performance in simulating streamflow at selected stations considering reservoir dynamics. We set our objective to maximize Nash-Sutcliffe Efficiency (NSE) (Dawson et al., 2007; Nash and Sutcliffe, 1970). The model performance was evaluated for daily streamflow, the water level of reservoirs, and the live storage of reservoirs using NSE and the coefficient of determination (R2). Daily streamflow was calibrated and evaluated at Sandia, Handia, Mandleshwar, and Garudeshwar. We selected different periods for the calibration and evaluation of the VIC-Res model based on the availability of observed streamflow. For instance, we selected the years 1986–2000, 1986–2000, 1998–2005, 1998–2005 as the calibration period, while the years 2001–2018, 2001–2018, 2015–2018, 2015–2018 as the evaluation period for stations Sandia, Handia, Mandleshwar, and Garudeshwar, respectively. The VIC-Res model performance was also evaluated against water level and live storage for Bargi, Tawa, Indira Sagar, and Sardar Sarovar reservoirs.
We first generated daily meteorological forcing of both ERFS and GEFS forecasts. The ERFS forecast is available for the extended range (1–32 d lead), while the GEFS forecast is available at 1–10 d lead. We developed observed initial conditions for each forecast date by forcing the long-term (20 years) observed meteorological forcing from IMD into the calibrated VIC-Res model. Therefore, the model spin-up is considered in the observed initial state. We simulated a daily streamflow forecast at all the four selected gauge stations using the meteorological forcing and initial conditions. The VIC-Res simulations were run for all the ensemble members for ERFS and GEFS forecasts. The ensemble streamflow forecasts were simulated for 1–32 d lead and 10 d lead for ERFS and GEFS datasets. The ERFS forecast simulations were run for 1–32 d lead with the initial conditions of every Wednesday generated from the VIC-Res model using the observed forcings. Similarly, GEFS streamflow forecast simulations were performed for 1–10 d lead with initial conditions 1 d before the forecast.
2.3 Forecast skill evaluation
We evaluated the skills of the streamflow forecast generated using the ERFS and GEFS meteorological forecast by comparing the simulated streamflow forecast to the observed daily streamflow at each of the four locations. The model-simulated streamflow forecast was evaluated against the VIC-Res model-simulated daily streamflow using the observed forcing due to the unavailability of the observed streamflow for the years 2019–2020. The ERFS meteorological forcing was used to run the VIC-Res model for 1–32 d from each forecast date using the initial conditions generated using the observed forcing from IMD. Similarly, we ran the GEFS ensemble members for a 1–10 d lead for each forecast date. We used bias and normalized root mean square error (NRMSE) to evaluate the performance of individual ensemble forecast members, which can be estimated as follows:
where = mean of observations.
where Qobs,i and Qsim,i are observed and simulated streamflow, respectively. Bias provides a measure of correspondence between the mean of observations and the mean of the VIC-Res model simulations, while NRMSE represents the relative magnitude of the squared error. We also evaluated the skills of ERFS forecast using the continuous ranked probability score (CRPS) (Hersbach, 2000), which measures the closeness between the distributions of forecast and observations. The CPRS can be estimated as follows:
where F(x) is the cumulative distribution function (CDF) associated with probabilistic forecast and H(x) is the Heaviside function (H(x)=1 for x≥0 and zero otherwise). The unit of CRPS is the same the of observations. Gneiting and Raftery (2007) suggested CPRS as a direct measure to compare deterministic and probabilistic forecasts.
3.1 Skill evaluation of ERFS and GEFS meteorological forecasts
First, we evaluated ERFS precipitation and temperature forecast skills for 1, 7, 15, and 31 d leads. We used bias, NRMSE, and the correlation coefficient (r) to estimate the forecast skills. The forecast skill was evaluated for the period 2003–2018. We estimated the forecast skill for each ensemble member and then calculated the median of the forecast skill of all the 16 members for each grid in the Narmada River Basin. Precipitation forecast from ERFS shows a negative bias indicating an underestimation compared to observed rainfall. The dry bias in precipitation forecast increases with the lead time (Fig. 2). For the 1 d lead, precipitation forecast from ERFS showed a moderate positive correlation (median ∼ 0.49), which declines with the lead time. Similarly, NRMSE in the precipitation forecast is large (>2.0) over the river basin. We also estimated bias in the precipitation forecast exceeding the 90th percentile (Fig. 3). The extreme rainfall in the raw ERFS forecast dataset exhibited a weaker correlation with the observed extreme precipitation. Moreover, a considerable dry bias in the extreme precipitation forecast was found. We also evaluated forecast skills for maximum and minimum temperatures against the observed temperatures from IMD for the 2003–2018 period (Figs. S1 and S2 in the Supplement). The daily temperature forecast showed a relatively higher positive correlation with the observed temperatures from IMD. Moreover, a lower NRMSE was noted for the temperature forecast than the observed maximum and minimum temperatures. However, a positive bias of ∼ 1.5 ∘C (median of all grids in the basin) was found in the minimum temperature forecast at all the lead times.
Next, we compared the ERFS and GEFS ensemble forecast skills for the summer monsoon (June–September) of the 2019–2020 period. We limit the comparison to the 2 years as the GEFS ensemble forecast is available only for 2019–2020. We evaluated forecast skills for 1, 5, and 10 d leads (Fig. 4). Our results show that the ERFS precipitation forecast has a dry bias across the river basin and all the leads (Fig. 4). The GEFS precipitation forecast showed a positive (wet) bias in the majority of the Narmada River Basin. The forecast products (ERFS and GEFS) underestimate extreme rainfall in the Narmada Basin (Fig. 5). The dry bias in extreme rainfall increases with lead time in the ERFS and GEFS forecasts (Fig. 5). The forecast products showed a poor correlation with the observed extreme precipitation in the Narmada River Basin (Fig. 5). However, both the forecast products demonstrated relatively better skills for maximum and minimum temperatures than precipitation (Figs. S3 and S4).
3.2 Calibration and evaluation of the VIC-Res model
We performed calibration of reservoir level and storage and calibration of daily streamflow. Daily storage and water levels were used to calibrate the VIC-Res model for four major reservoirs (Bargi, Tawa, Indira Sagar and Sardar Sarovar) in the Narmada Basin. The upstream catchment area of all the gauge locations and calibration parameters are shown in Supplement Fig. S5. We evaluated the VIC-Res model's performance using the coefficient of determination (R2) and NSE (Fig. 6). The VIC-Res model simulates daily streamflow at the selected stations in the basin. The R2 and NSE values were above 0.65 at Sandia, Handia, and Mandleshwar stations for the calibration period, while at Garudeshwar the VIC-Res model performed comparatively weaker (R2=0.55 and NSE = 0.53) for the calibration period.
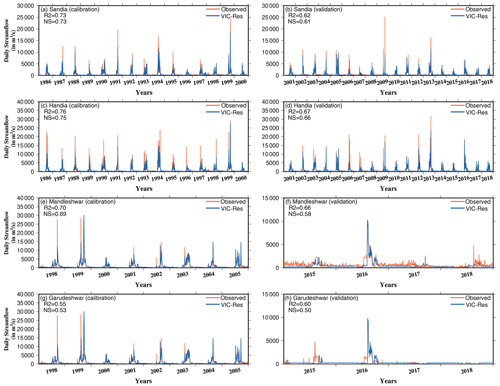
Figure 6Calibration and evaluation of the VIC-Res model against observed daily streamflow at gauge stations at Sandia, Handia, Mandleshwar and Garudeshwar. The performance of the VIC-Res model in simulating daily streamflow was evaluated using the R2 and NSE.
We considered the influence of major reservoirs on the simulated daily streamflow. Therefore, the VIC-Res model's performance in simulating daily reservoir storage and the water level was evaluated against the streamflow observations. We selected 2000–2016, 2000–2016, 2007–2016, and 2008–2013 as evaluation periods for Bargi, Tawa, Indira Sagar, and Sardar Sarovar reservoirs, respectively, based on the availability of observations. We estimated R2 and NSE to evaluate the model's performance (Fig. 7). The model performed well in simulating all the reservoirs' water levels and storage (R2>0.78 and NSE >0.62). We also compared the seasonal cycle of the observed and simulated reservoir storage for all the four major reservoirs (Fig. 8). The monthly seasonal cycle of reservoir storage simulated by the model compares well with the observed storage for all the dams with R2 of more than 0.77. We find that the model underestimates storage for the Bargi reservoir, which can be due to the relatively smaller upstream catchment area that might not capture the spatial variability of rainfall. Overall, we found that the VIC-Res model can evaluate the ensemble streamflow forecast in the Narmada River Basin.
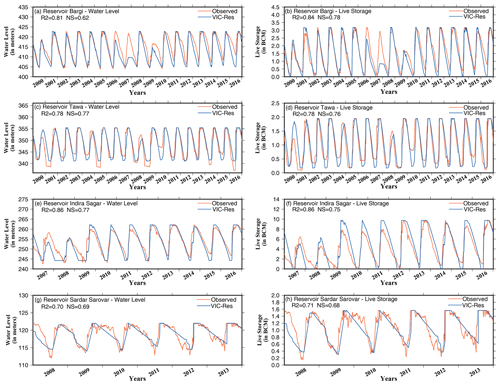
Figure 7Evaluation of the VIC-Res model in simulating daily water level and daily live storage at four major reservoirs Bargi, Tawa, Indira Sagar and Sardar Sarovar.
3.3 Evaluation of ensemble streamflow forecast skills of ERFS
We estimated forecast skills of daily streamflow for 2003–2018 generated from each ensemble member of ERFS for the 12 lead times (1–10, 15, and 31 d). We selected a 1–10 d lead as the GEFS forecast is also available with the same lead. In addition, 2 other lead times (15 and 31 d) were selected to evaluate the skill of streamflow forecast from all the 16 members of ERFS (Fig. 9). Both bias and NRMSE showed a relatively lesser spread for the shorter lead (1–3 d) streamflow forecast from all the ensemble members of ERFS (Fig. 9). However, uncertainty in streamflow forecast due to different ensemble members increases with the lead time. The NRMSE of streamflow forecast from ERFS also rises with the lead at all the stations. Ensemble streamflow forecast from ERFS showed a positive bias for Sandia, Handia, and Garudeshwar, while a negative bias was found for Mandleshwar station (Fig. 9). We estimated the CRPS, which is higher for a 1 d lead compared to 3 d leads and increases with the lead time (Fig. S6).
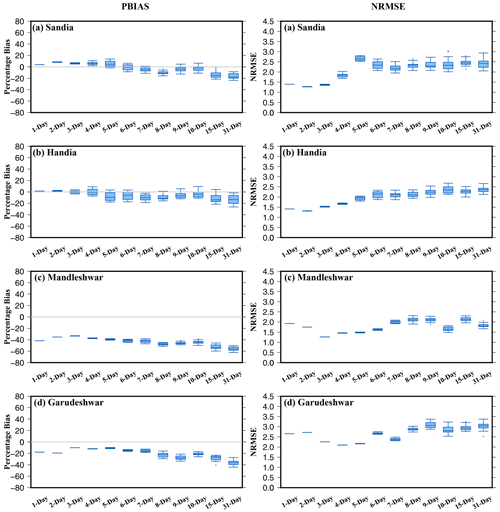
Figure 9Ensemble streamflow forecast skill based on the ERFS forecast for 2003–2018. The forecast was evaluated using bias (%) and NRMSE. Box and whisker plots show the skill for all 16 ensemble members at leads 1–10, 15 and 31 d at 4 gauge stations.
We estimated the forecast skill in streamflow exceeding certain thresholds (50th, 70th, 80th, 90th, and 95th percentiles) (Fig. 10). We find less spread in bias among different ensemble members for 1 d lead streamflow forecast from ERFS. However, the spread of bias in streamflow forecast due to different ensemble members increases with the lead time (Fig. 10). Moreover, bias in streamflow forecast remains stable for all the selected percentile thresholds at a 1 d lead at all 4 gauge stations. On the other hand, bias in streamflow forecast increases for higher percentiles at longer lead times. For instance, dry bias in streamflow forecast in all the ensemble members is higher for the 95th percentile than for the 50th percentile. Therefore, our results show that regardless of the spread among the ensemble members from ERFS, almost all the ensemble members underestimate the high flow at all the gauge stations in the Narmada River Basin (Fig. 10).
3.4 Comparison of ensemble streamflow forecast skills ERFS and GEFS
We compared the streamflow forecast skills of 16 ensemble members from ERFS and 21 ensemble members from GEFS. As GEFS meteorological forecast is available only for 2019–2020, we compared the summer monsoon season of these 2 years. The ERFS forecast is available weekly for 1–32 d, while the GEFS forecast is generated every day. Therefore, we compared the daily streamflow forecast from both the products for the weeks for which the ERFS forecast was available for the summer monsoon of the 2019–2020 period. We compared the streamflow forecast skills for all the ensemble members at 1–10 d leads at Sandia, Handia, Mandleshwar, and Garudeshwar (Fig. 11). We find that the GEFS forecast has a better skill for the short lead time (∼ 1–5 d) with less bias and NRMSE. On the other hand, the ERFS ensemble forecast showed higher bias and NRMSE at shorter leads for most of the selected locations in the Narmada Basin. Streamflow forecast skill of GEFS declines rapidly after the 3–4 d lead time for most of the locations in the Narmada Basin. The forecast products showed a larger spread among the streamflow forecast ensemble members after 5 days lead. For short to medium range (∼ 1–5 d), the streamflow forecast from GEFS performed better with low NRMSE and bias for streamflow exceeding the 75th percentile of the summer monsoon period (Fig. S7). Moreover, streamflow forecast skill from the ERFS was considerably lower than the GEFS at most of the locations for flow exceeding 75th percentiles (Fig. S7).
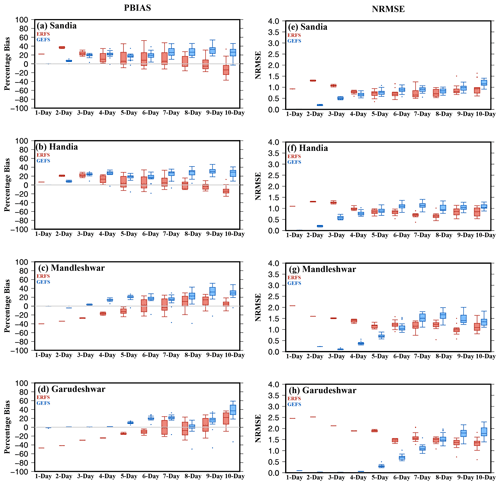
Figure 11Comparison of ensemble streamflow forecast skills from ERFS and GEFS for 2019–2020. The forecast skill was evaluated considering the VIC-Res simulated streamflow with the observed forcing from IMD due to unavailability of observed flow.
We examined the daily streamflow forecast skill at 3, 5 d, and 10-leads from ERFS and GEFS forecasts for the summer monsoon season of 2019 and 2020 against VIC-Res simulated streamflow using the observed meteorological forcing at all the four gauge stations (Figs. 12 and S8). As observed daily streamflow was unavailable for skill assessment, the comparison was made against the VIC-Res model simulated flow with the observed meteorological forcing (Figs. 12 and S8). The GEFS forecast successfully captured streamflow peaks in both 2019 and 2020 at a 3 d lead. In 2019, GEFS forecasts overestimated streamflow peaks at 3 and 5 d leads during the summer monsoon. On the other hand, the ensemble streamflow forecast developed using the ERFS meteorological forecast showed a higher spread than GEFS (Figs. 12, S8). The spread in ensemble streamflow forecast increases for both ERFS and GEFS forecast at a 10 d lead. However, the ERFS streamflow forecast showed a better skill at the 10 d lead. Despite having fewer ensemble members than the GEFS, the ERFS forecast showed a broader spread in streamflow prediction, highlighting a higher uncertainty in prediction. We find that GEFS overestimates while ERFS underestimates streamflow at most of the locations and lead times.
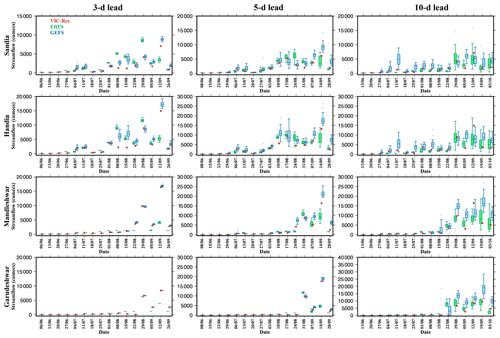
Figure 12Comparison of ensemble streamflow simulated using the VIC-Res model with ERFS and GEFS forecast products during the summer monsoon of 2019. The forecast skill was evaluated considering the VIC-Res simulated streamflow with the observed forcing from IMD due to unavailability of observed flow.
We examined the streamflow forecast generated by all the ensemble members of ERFS and GEFS for a few events using the VIC-Res model (Fig. 13). The ensemble streamflow prediction was compared considering the model simulated streamflow with the observed forcing from IMD. In 2019, the ensemble mean streamflow from all the ensemble members of ERFS considerably underestimated the peak flow (Fig. 13). However, a few ensemble members of the ERFS forecast captured the peak flow at the four locations of the Narmada River Basin (Fig. 13). At Handia station, 1 out of 16 ensemble members exceeded the observed streamflow. Moreover, GEFS forecasts at short leads (3–5 d) performed well in capturing peaks (Fig. 13). However, GEFS forecasts showed a smaller spread in ensemble streamflow at the short lead time (1–5 d). Overall, we find that ensemble forecasts can be used for probabilistic streamflow prediction.
Streamflow forecast plays an essential role in efficient reservoir operations and flood mitigation (Chen et al., 2016; Mediero et al., 2007). A reliable streamflow forecast can reduce uncertainty in reservoir operations and enhance the development of a flood early warning system. Notwithstanding the considerable progress in an operational meteorological forecast from different agencies, efforts to establish an ensemble streamflow forecast system at river basin scales have been limited for India. Moreover, it remains unclear if other meteorological forecast products have different streamflow forecast skills. We used the two meteorological ensemble forecast products from IMD to examine streamflow forecast skills in the Narmada River Basin. The presence of reservoirs influences the water budget and streamflow (Shah et al., 2019; Zajac et al., 2017; Yun et al., 2020; Chai et al., 2019). Hydrological model parameters calibrated without considering the role of reservoirs can be erroneous and lead to errors and uncertainty in simulated hydrological processes (Dang et al., 2020a). Therefore, we used the ensemble streamflow prediction approach to generate the daily streamflow simulations considering the influence of reservoirs in the Narmada River Basin. We compared the performance of ERFS and GEFS ensembles for the summer monsoon period of 2019–2020. We also assessed the skills of the ERFS dataset solely for a more extended period from 2003 to 2018.
The ERFS ensemble forecast is available once a week at 1–32 d lead times. On the other hand, GEFS ensemble forecasts are available daily at 1–10 d leads for the summer monsoon period of 2019–2020. Hagedorn et al. (2005) reported that bias correction of the raw forecast does not necessarily increase the forecast skill. Moreover, statistical correction of the raw forecast is inappropriate, which can lose its effect by propagating through the hydrological model (Zalachori et al., 2012; Crochemore et al., 2016; Benninga et al., 2017; Hagedorn et al., 2005). Therefore, we did not correct the raw meteorological ensemble forecasts from ERFS and GEFS for bias. The skills of ERFS and GEFS precipitation and temperature (minimum and maximum) forecasts were estimated for 1, 5 and 10 d leads. The GEFS raw forecast showed better skills than the ERFS forecast for mean and extreme precipitation. As precipitation plays a vital role in streamflow forecast (Meaurio et al., 2017; Demargne et al., 2014; Pappenberger et al., 2005), our results show that GEFS forecast provides better skills for streamflow prediction in the Narmada River Basin. The postprocessing of streamflow data can significantly improve performance (Tiwari et al., 2021; Muhammad et al., 2018), which can be used in the future to examine the improvements in streamflow prediction. Moreover, a multimodel approach can be used to reduce the errors and uncertainty in streamflow forecasts that could arise due to the parameterization of hydrological models (Velázquez et al., 2011; Zarzar et al., 2018; Muhammad et al., 2018).
The skills of ERFS and GEFS ensemble forecasts were estimated for 1, 5 and 10 d leads. The GEFS raw forecasts illustrated better skills than ERFS forecasts for overall rainfall and extreme precipitation. Studies showed that rain plays a vital role in streamflow forecast (Demargne et al., 2014; Meaurio et al., 2017; Pappenberger et al., 2005) and we also observed the same results. The ensemble forecast with better skills performed well in predicting daily streamflow. Correcting the bias of the input forecast may shrink the variability range of the result. However, ensemble forecasts aim to capture uncertainties. Studies suggest that the postprocessing of streamflow data can significantly improve performance (Muhammad et al., 2018; Tiwari et al., 2021). A multimodel approach, where more than one hydrological model is used, can generalize the uncertainty introduced by the hydrological model. Various studies have reported improved forecast skills using the multimodel approach (Muhammad et al., 2018; Velázquez et al., 2011; Zarzar et al., 2018). Also, our analysis is based only on 2019–2020 as the GEFS hindcast is available only for this period. Availability of longer hindcast from the GEFS can help to understand the forecast skills for hydrological extremes (drought and floods). Moreover, we did not examine the forecast skill of reservoir storage, which can provide a better understanding of the impacts of storage during the floods.
Flood forecasting using the available meteorological forecast products can help in mitigating the losses through early warnings. To account for the uncertainty arising from initial state and model parameterization, the individual members of the ensemble weather forecast can provide better information than their ensemble mean (Saleh et al., 2019). The probabilistic approach over the deterministic method provides the range of variability, which can help determine the probability of exceeding a specific threshold of streamflow (Hsiao et al., 2013). The shift from the existing flood forecast system to the ensemble-based probabilistic forecast requires modifications in the current flood forecast practice. The transition is expected to change various aspects of the existing decision-making process. The forecasters need to adequately train the on duty officers and the authorities on probabilistic forecasts. We evaluated the streamflow forecast skills at 1–32 d leads in the Narmada River Basin. The increased lead time in streamflow forecast can assist in developing efficient methods for communication of information (Arnal et al., 2020; Ramos et al., 2010). Moreover, ensemble streamflow forecast at longer leads can be effectively used in optimizing reservoir operations (Alemu et al., 2011). Our results show that while the mean of the ensemble members failed to capture the high flows, a few individual ensemble members performed better in capturing peak flow, which can be used to develop probabilistic early warning systems.
Based on our findings, the following conclusions can be made.
-
The raw precipitation forecast from both GEFS and ERFS datasets showed moderate skills (bias, NRMSE and correlation) against observations from IMD at 1, 5 and 10 d lead times. While both (ERFS and GEFS) forecast products underestimated extreme precipitation, dry bias in the ERFS forecast was more prominent than the GEFS forecast. For instance, raw precipitation forecast from ERFS showed negative bias across the Narmada River Basin. On the other hand, the raw precipitation forecast from GEFS exhibited both negative and positive bias. Both the forecast products showed better skills for maximum and minimum temperatures than precipitation.
-
We calibrated and evaluated the VIC-Res model to simulate streamflow, considering the influence of reservoirs at four gauge stations in the Narmada River Basin. The model reproduced daily streamflow, reservoir water level, and storage reasonably well against the observations.
-
Comparing the streamflow forecast skills of both ensemble forecasts showed that GEFS forecasts performed better than the ERFS at all the locations in the basin. However, both of the forecast products underestimated the extremes, which can be due to dry bias in extreme precipitation. The spread in streamflow due to different ensemble members increased with the forecast lead time. Overall, an ensemble forecast can be used to develop a probabilistic forecast-based flood early warning system.
All the datasets used in this study can be obtained from the corresponding author.
The supplement related to this article is available online at: https://doi.org/10.5194/hess-26-6361-2022-supplement.
VM designed the study. UV conducted simulations and wrote the first draft. UV and VM discussed the results and prepared the final version.
The contact author has declared that neither of the authors has any competing interests.
Publisher's note: Copernicus Publications remains neutral with regard to jurisdictional claims in published maps and institutional affiliations.
This article is part of the special issue “Representation of water infrastructures in large-scale hydrological and Earth system models”. It is not associated with a conference.
The work was supported by the Monsoon Mission, Ministry of Earth Sciences. The authors acknowledge the data availability from India Meteorological Department (IMD) and India-WRIS. ERFS and GEFS forecast products were obtained from the Indian Institute of Tropical Meteorology (IITM), Pune.
This research has been supported by the Ministry of Earth Sciences (Monsoon Mission).
This paper was edited by Stefano Galelli and reviewed by two anonymous referees.
Alemu, E. T., Palmer, R. N., Polebitski, A., and Meaker, B.: Decision Support System for Optimizing Reservoir Operations Using Ensemble Streamflow Predictions, J. Water Resour. Plan Manag., 137, 72–82, https://doi.org/10.1061/(asce)wr.1943-5452.0000088, 2011.
Alfieri, L., Burek, P., Dutra, E., Krzeminski, B., Muraro, D., Thielen, J., and Pappenberger, F.: GloFAS – global ensemble streamflow forecasting and flood early warning, Hydrol. Earth Syst. Sci., 17, 1161–1175, https://doi.org/10.5194/hess-17-1161-2013, 2013.
Ali, H., Modi, P., and Mishra, V.: Increased flood risk in Indian sub-continent under the warming climate, Weather Clim. Extrem., 25, 100212, https://doi.org/10.1016/j.wace.2019.100212, 2019.
Arnal, L., Anspoks, L., Manson, S., Neumann, J., Norton, T., Stephens, E., Wolfenden, L., and Cloke, H. L.: “Are we talking just a bit of water out of bank? Or is it Armageddon?” Front line perspectives on transitioning to probabilistic fluvial flood forecasts in England, Geosci. Commun., 3, 203–232, https://doi.org/10.5194/gc-3-203-2020, 2020.
Benninga, H.-J. F., Booij, M. J., Romanowicz, R. J., and Rientjes, T. H. M.: Performance of ensemble streamflow forecasts under varied hydrometeorological conditions, Hydrol. Earth Syst. Sci., 21, 5273–5291, https://doi.org/10.5194/hess-21-5273-2017, 2017.
Boulange, J. and Hanasaki, N.: A global flood risk analysis with explicit representation of major dams, in: Japan Society of Hydrology and Water Resources 2019 Annual Meeting, https://doi.org/10.11520/jshwr.32.0_12, 2013.
Bowler, N. E., Arribas, A., Mylne, K. R., Robertson, K. B., and Beare, S. E.: The MOGREPS short-range ensemble prediction system, Q. J. Roy. Meteor. Soc., 134, 703–722, https://doi.org/10.1002/qj.234, 2008.
Chai, Y., Li, Y., Yang, Y., Zhu, B., Li, S., Xu, C., and Liu, C.: Influence of Climate Variability and Reservoir Operation on Streamflow in the Yangtze River, Sci. Rep., 9, 5060, https://doi.org/10.1038/s41598-019-41583-6, 2019.
Chen, L., Singh, V. P., Lu, W., Zhang, J., Zhou, J., and Guo, S.: Streamflow forecast uncertainty evolution and its effect on real-time reservoir operation, J. Hydrol., 540, 712–726, https://doi.org/10.1016/j.jhydrol.2016.06.015, 2016.
Cloke, H. L. and Pappenberger, F.: Ensemble flood forecasting: A review, J. Hydrol., 375, 613–626, https://doi.org/10.1016/j.jhydrol.2009.06.005, 2009.
Crochemore, L., Ramos, M.-H., and Pappenberger, F.: Bias correcting precipitation forecasts to improve the skill of seasonal streamflow forecasts, Hydrol. Earth Syst. Sci., 20, 3601–3618, https://doi.org/10.5194/hess-20-3601-2016, 2016.
Dang, T. D., Chowdhury, A. F. M. K., and Galelli, S.: On the representation of water reservoir storage and operations in large-scale hydrological models: implications on model parameterization and climate change impact assessments, Hydrol. Earth Syst. Sci., 24, 397–416, https://doi.org/10.5194/hess-24-397-2020, 2020a.
Dang, T. D., Vu, D. T., Chowdhury, A. F. M. K., and Galelli, S.: A software package for the representation and optimization of water reservoir operations in the VIC hydrological model, Environ. Model. Softw., 126, 104673, https://doi.org/10.1016/j.envsoft.2020.104673, 2020b.
Dawson, C. W., Abrahart, R. J., and See, L. M.: HydroTest: A web-based toolbox of evaluation metrics for the standardised assessment of hydrological forecasts, Environ. Modell. Softw., 22, 1034–1052, https://doi.org/10.1016/j.envsoft.2006.06.008, 2007.
Demargne, J., Wu, L., Regonda, S. K., Brown, J. D., Lee, H., He, M., Seo, D. J., Hartman, R., Herr, H. D., Fresch, M., Schaake, J., and Zhu, Y.: The science of NOAA's operational hydrological ensemble forecast service, B. Am. Meteorol. Soc., 95, 79–98, https://doi.org/10.1175/BAMS-D-12-00081.1, 2014.
Demeritt, D., Pappenberger, F., Centre, E., Weather, R., and Rg, R.: Challenges in communicating and using ensembles in operational flood forecasting, Meteorol. Appl., 17, 209–222, https://doi.org/10.1002/met.194, 2010.
Dipti, J.: What is the impact of floods on India's GDP?, https://www.livemint.com/Politics/M1cZ2bfYHSG7yCdHHvUozN/Are-floods-causing-more-damage-these-days.html (last access: 4 October 2022), 2017.
Dong, N., Wei, J., Yang, M., Yan, D., Yang, C., Gao, H., Arnault, J., Laux, P., Zhang, X., Liu, Y., Niu, J., Wang, H., Wang, H., Kunstmann, H., and Yu, Z.: Model Estimates of China's Terrestrial Water Storage Variation Due To Reservoir Operation, Water Resour. Res., 58, WR031787, https://doi.org/10.1029/2021WR031787, 2022.
Field, C. B., Stocker, T. F., Barros, V. R., Qin, D., Ebi, K. L., and Midgley, P. M.: IPCC special report on managing the risks of extreme events and disasters to advance climate change adaptation, in: AGU Fall Meeting Abstracts, San Francisco, California, 5–9 December 2011, NH12A–02, https://ui.adsabs.harvard.edu/abs/2011AGUFMNH12A..02F/abstract (last access: 10 June 2022), 2011.
Gao, H., Tang, Q., Shi, X., Zhu, C., Bohn, T., Su, F., Pan, M., Sheffield, J., Lettenmaier, D., and Wood, E.: Water budget record from Variable Infiltration Capacity (VIC) model, 120–173, 2010.
Georgakakos, A. P., Yao, H., Kistenmacher, M., Georgakakos, K. P., Graham, N. E., Cheng, F. Y., Spencer, C., and Shamir, E.: Value of adaptive water resources management in Northern California under climatic variability and change: Reservoir management, J. Hydrol., 412–413, 34–46, https://doi.org/10.1016/j.jhydrol.2011.04.038, 2012.
Gneiting, T. and Raftery, A. E.: Strictly Proper Scoring Rules, Prediction, and Estimation, J. Am. Stat. Assoc., 102, 359–378, https://doi.org/10.1198/016214506000001437, 2007.
Gosain, A. K., Rao, S., and Basuray, D.: Climate change impact assessment on hydrology of Indian river basins, Current Science, 90, 346–353, https://www.jstor.org/stable/24091868 (last access: 4 October 2022), 2006.
Goswami, S. B., Bal, P. K., and Mitra, A. K.: Use of rainfall forecast from a high-resolution global NWP model in a hydrological stream flow model over Narmada river basin during monsoon, Model Earth Syst. Environ., 4, 1029–1040, https://doi.org/10.1007/s40808-018-0436-y, 2018.
Hagedorn, R., Doblas-Reyes, F. J., and Palmer, T. N.: The rationale behind the success of multi-model ensembles in seasonal forecasting – I. Basic concept, Tellus A, 57, 219–233, https://doi.org/10.3402/tellusa.v57i3.14657, 2005.
Hanasaki, N., Yoshikawa, S., Pokhrel, Y., and Kanae, S.: A global hydrological simulation to specify the sources of water used by humans, Hydrol. Earth Syst. Sci., 22, 789–817, https://doi.org/10.5194/hess-22-789-2018, 2018.
Harsha, J.: Fighting floods with insufficient warning, https://www.thestatesman.com/opinion/fighting-floods-insufficient-warning-1502924062.html (last access: 4 October 2022), 2020a.
Harsha, J.: Playing catch up in flood forecasting technology, https://www.thehindu.com/opinion/lead/playing-catch-up-in-flood-forecasting-technology/article32797281.ece (last access: 4 October 2022), 2020b.
Hersbach, H.: Decomposition of the continuous ranked probability score for ensemble prediction systems, Weather Forecast., 15, 559–570, https://doi.org/10.1175/1520-0434(2000)015<0559:DOTCRP>2.0.CO;2, 2000.
Hsiao, L. F., Yang, M. J., Lee, C. S., Kuo, H. C., Shih, D. S., Tsai, C. C., Wang, C. J., Chang, L. Y., Chen, D. Y. C., Feng, L., Hong, J. S., Fong, C. T., Chen, D. S., Yeh, T. C., Huang, C. Y., Guo, W. D., and Lin, G. F.: Ensemble forecasting of typhoon rainfall and floods over a mountainous watershed in Taiwan, J. Hydrol., 506, 55–68, https://doi.org/10.1016/j.jhydrol.2013.08.046, 2013.
Jain, S. K., Mani, P., Jain, S. K., Prakash, P., Vijay, P., Tullos, D., Kumar, S., Agarwal, S. P., and Dimri, A. P.: A Brief review of flood forecasting techniques and their applications, Intl. J. River Basin Management, 16, 329–344, https://doi.org/10.1080/15715124.2017.1411920, 2018.
Jarvis, A.: Hole-filled seamless SRTM data V4, International Centre for Tropical Agriculture (CIAT), https://cir.nii.ac.jp/crid/1572824499120022016 (last access: 10 June 2022), 2008.
Joshi, H.: Floods across the country highlight need for a robust flood management structure, https://india.mongabay.com/2020/08/floods-across-the-country-highlight-need-for-a-robust-flood-management-structure/ (last access: 4 October 2022), 2020.
Krzysztofowicz, R.: The case for probabilistic forecasting in hydrology, J. Hydrol., 249, 2–9, https://doi.org/10.1016/S0022-1694(01)00420-6, 2001.
Liang, X., Lettenmaier, D. P., Wood, E. F., and Burges, S. J.: A simple hydrologically based model of land surface water and energy fluxes for general circulation models, J. Geophys. Res.-Atmos., 99, 14415–14428, https://doi.org/10.1029/94JD00483, 1994.
Luo, T., Maddocks, A., Iceland, C., Ward, P., and Winsemius, H.: World's 15 countries with the most people exposed to river floods, https://www.wri.org/insights/worlds-15-countries-most-people-exposed-river-floods (last access: 4 October 2022), 2015.
Madhusoodhanan, C. G., Sreeja, K. G., and Eldho, T. I.: Climate change impact assessments on the water resources of India under extensive human interventions, Ambio, 45, 725–741, https://doi.org/10.1007/s13280-016-0784-7, 2016.
Meaurio, M., Zabaleta, A., Boithias, L., Epelde, A. M., Sauvage, S., Sánchez-Pérez, J. M., Srinivasan, R., and Antiguedad, I.: Assessing the hydrological response from an ensemble of CMIP5 climate projections in the transition zone of the Atlantic region (Bay of Biscay), J. Hydrol., 548, 46–62, https://doi.org/10.1016/j.jhydrol.2017.02.029, 2017.
Mediero, L., Garrote, L., and Martín-Carrasco, F.: A probabilistic model to support reservoir operation decisions during flash floods, Hydrolog. Sci. J., 52, 523–537, https://doi.org/10.1623/hysj.52.3.523, 2007.
Mishra, V., Cherkauer, K. A., Niyogi, D., Lei, M., Pijanowski, B. C., Ray, D. K., Bowling, L. C., and Yang, G.: A regional scale assessment of land use/land cover and climatic changes on water and energy cycle in the upper Midwest United States, Int. J. Climatol., 30, 2025–2044, https://doi.org/10.1002/joc.2095, 2010.
Muhammad, A., Stadnyk, T. A., Unduche, F., and Coulibaly, P.: Multi-model approaches for improving seasonal ensemble streamflow prediction scheme with various statistical post-processing techniques in the Canadian Prairie Region, Water, 10, 1604, https://doi.org/10.3390/w10111604, 2018.
Mukhopadhyay, P., Krishna, R. P. M., Deshpande, M., Ganai, M., Tirkey, S., Goswami, T., Sarkar, S., Malviya, S., Kanase, R., Roy, K., and Prasad, V. S.: High Resolution (12.5 km) Ensemble Prediction system based on GEFS: Evaluation of extreme precipitation events over Indian region, http://www.bom.gov.au/research/workshop/abstracts/bm_abs_20181126T1145_mukhopadhyay.docx (last access: 12 April 2022), 2018.
Nanditha, J. S. and Mishra, V.: On the need of ensemble flood forecast in India, Water. Secur., 12, 100086, https://doi.org/10.1016/j.wasec.2021.100086, 2021.
Nanditha, J. S. and Mishra, V.: Multiday Precipitation Is a Prominent Driver of Floods in Indian River Basins, Water Resour. Res., 58, e2022WR032723, https://doi.org/10.1029/2022WR032723, 2022.
Nash, J. E. and Sutcliffe, J. V.: River flow forecasting through conceptual models part I – A discussion of principles, J. Hydrol., 10, 282–290, 1970.
National Register of Large Dams (NRLD): Central Water Commission (CWC), Ministry of Water Resources, Government of India, http://cwc.gov.in/sites/default/files/NRLD_04012017.pdf (last access: 4 October 2022), 2017.
Pai, D. S., Sridhar, L., Rajeevan, M., Sreejith, O. P., Satbhai, N. S., and Mukhopadhyay, B.: Development of a new high spatial resolution (0.25∘ × 0.25∘) long period (1901–2010) daily gridded rainfall data set over India and its comparison with existing data sets over the region, Mausam, 65, 1–18, 2014.
Pai, D. S., Sridhar, L., Badwaik, M. R., and Rajeevan, M.: Analysis of the daily rainfall events over India using a new long period (1901–2010) high resolution (0.25∘ × 0.25∘) gridded rainfall data set, Clim. Dynam., 45, 755–776, https://doi.org/10.1007/s00382-014-2307-1, 2015.
Pappenberger, F., Beven, K. J., Hunter, N. M., Bates, P. D., Gouweleeuw, B. T., Thielen, J., and de Roo, A. P. J.: Cascading model uncertainty from medium range weather forecasts (10 days) through a rainfall-runoff model to flood inundation predictions within the European Flood Forecasting System (EFFS), Hydrol. Earth Syst. Sci., 9, 381–393, https://doi.org/10.5194/hess-9-381-2005, 2005.
Pappenberger, F., Stephens, E., Thielen, J., Salamon, P., Demeritt, D., Jan, S., Wetterhall, F., and Al, L.: Visualizing probabilistic flood forecast information : expert preferences and perceptions of best practice in uncertainty communication, Hydrol. Process., 27, 132–146, https://doi.org/10.1002/hyp.9253, 2012.
Ramos, M. H., Mathevet, T., Thielen, J., and Pappenberger, F.: Communicating uncertainty in hydro-meteorological forecasts: Mission impossible?, Meteorol. Appl., 17, 223–235, https://doi.org/10.1002/met.202, 2010.
Ray, K., Pandey, P., Pandey, C., Dimri, A. P., and Kishore, K.: On the recent floods in India, Curr. Sci., 117, 204–218, https://doi.org/10.18520/cs/v117/i2/204-218, 2019.
Reed, P. M., Hadka, D., Herman, J. D., Kasprzyk, J. R., and Kollat, J. B.: Evolutionary multiobjective optimization in water resources: The past, present, and future, Adv. Water Resour., 51, 438–456, https://doi.org/10.1016/j.advwatres.2012.01.005, 2013.
Saleh, F., Ramaswamy, V., Georgas, N., Blumberg, A. F., and Pullen, J.: Inter-comparison between retrospective ensemble streamflow forecasts using meteorological inputs from ECMWF and NOAA/ESRL in the Hudson River sub-basins during Hurricane Irene (2011), Hydrol. Res., 50, 166–186, https://doi.org/10.2166/nh.2018.182, 2019.
Shah, H. L., Zhou, T., Sun, N., Huang, M., and Mishra, V.: Roles of Irrigation and Reservoir Operations in Modulating Terrestrial Water and Energy Budgets in the Indian Subcontinental River Basins, J. Geophys. Res.-Atmos., 124, 12915–12936, https://doi.org/10.1029/2019JD031059, 2019.
Shah, R., Sahai, A. K., and Mishra, V.: Short to sub-seasonal hydrologic forecast to manage water and agricultural resources in India, Hydrol. Earth Syst. Sci., 21, 707–720, https://doi.org/10.5194/hess-21-707-2017, 2017.
Sheffield, J. and Wood, E. F.: Characteristics of global and regional drought, 1950–2000: Analysis of soil moisture data from off-line simulation of the terrestrial hydrological cycle, J. Geophys. Res.-Atmos. 112, D17115, https://doi.org/10.1029/2006JD008288, 2007.
Sikder, M. S. and Hossain, F.: Improving operational flood forecasting in monsoon climates with bias-corrected quantitative forecasting of precipitation, International Journal of River Basin Management, 17, 411–421, https://doi.org/10.1080/15715124.2018.1476368, 2019.
Singh, O. and Kumar, M.: Flood events, fatalities and damages in India from 1978, Nat. Hazards, 69, 1815–1834, https://doi.org/10.1007/s11069-013-0781-0, 2013.
Srivastava, A. K., Rajeevan, M., and Kshirsagar, S. R.: Development of a high resolution daily gridded temperature data set (1969–2005) for the Indian region, Atmos. Sci. Lett., 10, 249–254, https://doi.org/10.1002/asl.232, 2009.
Teja, K. N. and Umamahesh, N. V.: Application of Ensemble Techniques for Flood Forecasting in India. Roorkee Water Conclave, https://www.iitr.ac.in/rwc2020/pdf/papers/RWC_67_Nikhil_Teja_et_al.pdf (last access: 4 October 2022), 2020.
Tiwari, A. D. and Mishra, V.: Sub-Seasonal Prediction of Drought and Streamflow Anomalies for Water Management in India, J. Geophys. Res.-Atmos., 127, e2021JD035737, https://doi.org/10.1029/2021JD035737, 2022.
Tiwari, A. D., Mukhopadhyay, P., and Mishra, V.: Influence of bias correction of meteorological and streamflow forecast on hydrological prediction in India, J. Hydrometeorol., 23, 1171–1192, https://doi.org/10.1175/jhm-d-20-0235.1, 2021.
Todini, E.: Flood Forecasting and Decision Making in the new Millennium. Where are We?, 31, 3111–3129, https://doi.org/10.1007/s11269-017-1693-7, 2017.
Tripathi, P.: Flood Disaster in India : An Analysis of trend and Preparedness Flood Disaster in India : An Analysis of trend and Preparedness, Interdisciplinary Journal of Contemporary Research, 2, 91–98, https://www.researchgate.net/publication/292980782 (last access: 4 October 2022), 2015.
Velázquez, J. A., Anctil, F., Ramos, M. H., and Perrin, C.: Can a multi-model approach improve hydrological ensemble forecasting? A study on 29 French catchments using 16 hydrological model structures, Adv. Geosci., 29, 33–42, https://doi.org/10.5194/adgeo-29-33-2011, 2011.
Wu, W., Emerton, R., Duan, Q., Wood, A. W., Wetterhall, F., and Robertson, D. E.: Ensemble flood forecasting: Current status and future opportunities, Wiley Interdisciplinary Reviews: Water, 7, e1432, https://doi.org/10.1002/wat2.1432, 2020.
Yassin, F., Razavi, S., Elshamy, M., Davison, B., Sapriza-Azuri, G., and Wheater, H.: Representation and improved parameterization of reservoir operation in hydrological and land-surface models, Hydrol. Earth Syst. Sci., 23, 3735–3764, https://doi.org/10.5194/hess-23-3735-2019, 2019.
Yun, X., Tang, Q., Wang, J., Liu, X., Zhang, Y., Lu, H., Wang, Y., Zhang, L., and Chen, D.: Impacts of climate change and reservoir operation on streamflow and flood characteristics in the Lancang-Mekong River Basin, J. Hydrol., 590, 125472, https://doi.org/10.1016/j.jhydrol.2020.125472, 2020.
Zajac, Z., Revilla-Romero, B., Salamon, P., Burek, P., Feyera, A., and Beck, H.: The impact of lake and reservoir parameterization on global streamflow simulation, J. Hydrol., 548, 552–568, https://doi.org/10.1016/j.jhydrol.2017.03.022, 2017.
Zalachori, I., Ramos, M.-H., Garçon, R., Mathevet, T., and Gailhard, J.: Statistical processing of forecasts for hydrological ensemble prediction: a comparative study of different bias correction strategies, Adv. Sci. Res., 8, 135–141, https://doi.org/10.5194/asr-8-135-2012, 2012.
Zarzar, C. M., Hosseiny, H., Siddique, R., Gomez, M., Smith, V., Mejia, A., and Dyer, J.: A Hydraulic MultiModel Ensemble Framework for Visualizing Flood Inundation Uncertainty, J. Am. Water Resour. Assoc., 54, 807–819, https://doi.org/10.1111/1752-1688.12656, 2018.
Zhang, J., Chen, J., Li, X., Chen, H., Xie, P., and Li, W.: Combining Postprocessed Ensemble Weather Forecasts and Multiple Hydrological Models for Ensemble Streamflow Predictions, J. Hydrol. Eng., 25, 04019060, https://doi.org/10.1061/(asce)he.1943-5584.0001871, 2020.