the Creative Commons Attribution 4.0 License.
the Creative Commons Attribution 4.0 License.
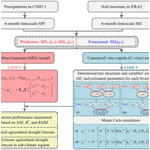
Comparison between canonical vine copulas and a meta-Gaussian model for forecasting agricultural drought over China
Haijiang Wu
Vijay P. Singh
Te Zhang
Jixia Qi
Shengzhi Huang
Agricultural drought mainly stems from reduced soil moisture and precipitation, and it causes adverse impacts on the growth of crops and vegetation, thereby affecting agricultural production and food security. In order to develop drought mitigation measures, reliable agricultural drought forecasting is essential. In this study, we developed an agricultural drought forecasting model based on canonical vine copulas in three dimensions (3C-vine model) in which antecedent meteorological drought and agricultural drought persistence were utilized as predictors. Furthermore, a meta-Gaussian (MG) model was selected as a reference to evaluate the forecast skill. The agricultural drought in China in August of 2018 was selected as a typical case study, and the spatial patterns of 1- to 3-month lead forecasts of agricultural drought utilizing the 3C-vine model resembled the corresponding observations, indicating the good predictive ability of the model. The performance metrics – the Nash–Sutcliffe efficiency (NSE), the coefficient of determination (R2), and the root-mean-square error (RMSE) – showed that the 3C-vine model outperformed the MG model with respect to forecasting agricultural drought in August for diverse lead times. Moreover, the 3C-vine model exhibited excellent forecast skill with respect to capturing the extreme agricultural drought over different selected typical regions. This study may help to guide drought early warning, drought mitigation, and water resource scheduling.
- Article
(8243 KB) - Full-text XML
- BibTeX
- EndNote
Agriculture is the source of livelihood for over 2.5 billion people worldwide, but this sector also sustains 82 % of all drought impacts (FAO, 2021). Thus, a cascade of impacts from droughts, such as crop reduction and failure, increased human and tree mortality, and ecological disturbance, has attracted considerable attention (FAO, 2021; Lu et al., 2012; Modanesi et al., 2020; Su et al., 2018; Q. Zhang et al., 2018, 2019; Zscheischler et al., 2020). Droughts reduced global crop production by about 9 %–10 % in the 1964–2007 period (Lesk et al., 2016). Additionally, droughts have caused overall crop and livestock production loss of USD 37 billion over the least-developed and lower-middle-income countries (FAO, 2021). Agricultural drought forecasting, therefore, lies at the core of overall drought risk management and is critical for food security, early warning, and drought preparedness and mitigation.
Agricultural drought is generally referred to as soil moisture shortage, which adversely affects crop yield and vegetation health (Modanesi et al., 2020; Zhang et al., 2016; T. Zhang et al., 2021). Under natural conditions, atmospheric precipitation is a paramount source for the replenishment of soil moisture (Wu et al., 2021b). Therefore, reduced soil moisture (agricultural drought) mainly arises from a precipitation deficit (meteorological drought) (Modanesi et al., 2020; Orth and Destouni, 2018). Moreover, soil moisture has a good drought memory due to the time-integration effects (Long et al., 2019), i.e., agricultural drought persistence. Hence, previous meteorological drought and antecedent agricultural drought can be taken into consideration as predictors of subsequent agricultural drought.
In hydrology, some physically based hydrological models (e.g., the Distributed Time-Variant Gain Hydrological Model – DTVGM, Ma et al., 2018, and the Soil and Water Assessment Tool – SWAT, Wu et al., 2019) are widely used in hydrological simulation and prediction, including the prediction of droughts. However, physically based hydrological models typically only apply at the catchment or subregional scale, and they generally require numerous hydrometeorological variables to achieve more accurate real-time predictions (Liu et al., 2021a; L. Xu et al., 2021). Traditional methods, such as regression models, machine learning models, and hybrid models (considering both statistical and dynamical predictions) (Hao et al., 2016), have been extensively employed to forecast drought. However, these models tend to be limited with respect to considering complex nonlinear factors (e.g., regression models), explicit physical mechanisms, and overfitting (e.g., machine learning models) as well as the demand of massive hydroclimatic data input (e.g., hybrid models). The copula functions, first introduced by Sklar (1959), overcome the limitations of the abovementioned conventional statistical methods, with the application of copulas in hydrology and geosciences stretching back to the 2000s (e.g., De Michele and Salvadori, 2003; Favre et al., 2004; Salvadori and De Michele, 2004). As copulas are flexible, joining arbitrary marginal distributions of variables, they have been widely employed by the hydrological research community in applications such as frequency analysis and risk assessment (De Michele et al., 2013; Hao et al., 2017; Liu et al., 2021b; Sarhadi et al., 2016; Y. Xu et al., 2021; T. Zhang et al., 2021, 2022; Zhou et al., 2019), flood and runoff forecasting (Bevacqua et al., 2017b; Hemri et al., 2015; Liu et al., 2018; Zhang and Singh, 2019), and drought forecasting (Ganguli and Reddy, 2014; Wu et al., 2021b). However, when bivariate copulas are extended to higher-dimensional (≥ three-dimensional) cases, they are restricted due to the nonexistence of analytical expressions (Liu et al., 2021a). Symmetric Archimedean copulas and nested Archimedean copulas have partially addressed the issues of dimensionality, but characterizing the various dependence structures with single-parameter and Archimedean class copulas is difficult (Aas and Berg, 2009; Hao et al., 2016; Wu et al., 2021b). Fortunately, vine copulas, developed by Joe (1996) and Bedford and Cooke (2002), can be adopted to address these limitations.
Vine copulas are flexible with respect to decomposing any multidimensional joint distribution into a hierarchy of bivariate-copula or pair-copula constructions (Aas et al., 2009; Bedford and Cooke, 2002; Liu et al., 2021a; Vernieuwe et al., 2015; Xiong et al., 2014). These copulas have been extensively applied in the hydrological field (Bevacqua et al., 2017b; Liu et al., 2021b; Vernieuwe et al., 2015; Wu et al., 2021a, b). For instance, Xiong et al. (2014) derived annual runoff distributions using canonical vine copulas; Liu et al. (2018) developed a framework to investigate compound floods based on canonical vine copulas; Wang et al. (2019) utilized regular vine copulas with historical streamflow and climate drivers to simulate monthly streamflow for the headwater catchment of the Yellow River basin; Liu et al. (2021a) developed a hybrid ensemble forecast model, using Bayesian model averaging combined canonical vine copulas, to forecast water level; and Wu et al. (2021b) proposed an agricultural drought forecast model based on vine copulas using four-dimensional scenarios.
The meta-Gaussian (MG) model, a popular statistical model in the hydrometeorological community, has explicit conditional distributions, which are apt for forecasting and risk assessment purposes (Hao et al., 2016, 2019a; Wu et al., 2021c; Y. Zhang et al., 2021). The forecast skill of the MG model for drought or compound dry–hot events, for example, has outperformed persistence-based or random forecast models (Hao et al., 2016, 2019a; Wu et al., 2021c). However, the MG model only depicts the linear relationship among explanatory variables (predictors) and the forecasted variable via a covariate matrix, and it cannot characterize the nonlinear or tail dependence existing in the variables (Hao et al., 2016). Fortunately, vine copulas can flexibly combine multiple variables via bivariate copula to characterize numerous or complex dependencies. To date, there has been rather limited investigation, to our knowledge, into model comparisons between vine copulas and the MG model with respect to agricultural drought forecasting under the same conditions. Therefore, comparative investigations into drought forecasting skill between vine copulas and the MG model are needed to obtain more reliable drought forecasts.
With the abovementioned facts in mind, the objective of this study was to compare the ability of canonical vine copulas in three dimensions (i.e., the 3C-vine model) and the MG model to forecast agricultural drought in August of every year in the 1961–2018 period using a three-dimensional scenario. Thus, the remainder of this paper is structured as follows: in Sect. 2, we briefly describe the study area and data used; the MG and 3C-vine models and performance metrics utilized are presented in Sect. 3; the results of the 3C-vine model application and assessment are displayed in Sect. 4; and, finally, the discussion and conclusions are presented in Sect. 5.
China stretches across a vast area that covers diverse climate regimes and is a major agricultural producer (Wu et al., 2021b; Zhang et al., 2015). For the convenience of analyzing the spatial patterns of agricultural drought, the climate of China was divided into seven subclimate regions, based on of Zhao (1983) and Yao et al. (2018), as shown in Fig. 1. For each subclimate region, the combined temperature and moisture conditions are roughly similar, and the soil and vegetation types have a certain common characteristic (Zhao, 1983).
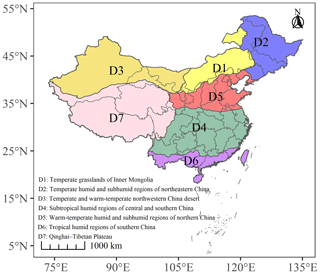
Figure 1The seven subclimate regions over China used in this work. Specific information on climate regions D1–D7 is listed in the bottom left of the panel.
In this study, the gridded monthly precipitation at a 0.25∘ × 0.25∘ spatial resolution was obtained from the CN05.1 data set for the 1961–2018 period over mainland China (excluding the Taiwan Province), which was provided by the Climate Change Research Center, Chinese Academy of Sciences (available at http://ccrc.iap.ac.cn/resource/detail?id=228, last access: 17 August 2021). The Copernicus Climate Change Service (C3S) at the European Center for Medium-Range Weather Forecasts (ECMWF) has begun the release of the ERA5 “back extension” data covering the 1950–1978 period on the Climate Data Store (CDS). Therefore, the gridded monthly soil moisture at a 0.25∘ × 0.25∘ spatial resolution corresponding to three soil depths (0–7 cm, 7–28 cm, and 28–100 cm) is available from the ECMWF ERA5 reanalysis data sets for the 1961–1978 period (https://cds.climate.copernicus.eu/cdsapp#!/dataset/reanalysis-era5-single-levels-monthly-means-preliminary-back-extension?tab=overview, last access: 14 May 2021) and the 1979–2018 period (https://cds.climate.copernicus.eu./cdsapp#!/dataset/reanalysis-era5-single-levels-monthly-means?tab=overview, last access: 14 May 2021). The CN05.1 and ERA5 reanalysis data sets have been extensively utilized in numerous studies for applications such as drought monitoring and forecasting (Wu et al., 2021b; T. Zhang et al., 2021), long-term climatic analysis (He et al., 2021; Wu et al., 2017), and flash-drought attribution analysis (Wang and Yuan, 2021).
The standardized precipitation index (SPI; based on monthly precipitation) and the standardized soil moisture index (SSI; based on monthly cumulative soil moisture at the top three soil depths) are leveraged to characterize meteorological drought and agricultural drought at a 6-month timescale, respectively. The empirical Gringorten plotting position formula (Gringorten, 1963) was used to obtain the empirical cumulative probabilities of these two indexes, which were then transformed into standardized variables via normal quantile transformation. As meteorological drought is a source of other drought types (e.g., agricultural drought), the antecedent precipitation deficiency (i.e., meteorological drought) has a stronger effect on the subsequent soil moisture deficiency (i.e., agricultural drought). Moreover, soil moisture has a good memory for prior drought (i.e., agricultural drought persistence), which is attributed to the soil porosity characteristics and time-integration effects (Long et al., 2019; Wu et al., 2021b).
We attempted to use the prior meteorological drought (SPIt−i, where t denotes the target month (e.g., August), and i indicates the lead time (month)) and agricultural drought persistence (SSIt−i) to forecast the subsequent agricultural drought (SSIt) based on canonical vine copulas using three-dimensional scenarios (3C-vine model). We selected the meta-Gaussian (MG) model as a reference model to assess the agricultural drought forecast performance of the 3C-vine model. Here, the 6-month timescale SPI (SSI) in August, which is calculated using the cumulative precipitation (soil moisture) from March to August, can indirectly reflect water surplus or deficit conditions in the spring (March–April–May) and summer (June–July–August) seasons. Furthermore, August is a key growth period for crops (e.g., anthesis, fruiting, and seed filling) and vegetation and is also a period with frequent droughts (Wu et al., 2021b). Undoubtedly, agricultural drought forecast can be implemented in any month of interest using the 3C-vine and MG models. More detailed information is given in the following.
3.1 The meta-Gaussian model using three-dimensional scenarios
The meta-Gaussian (MG) model can effectively combine multiple hydrometeorological variables, which have gained attention with respect to drought forecasting and risk assessment (Hao et al., 2019a, b; Wu et al., 2021c; Y. Zhang et al., 2021). Thus, if the series of SPIt−i, SSIt−i, and SSIt correspond to random variables Y1, Y2, and Y3, respectively, the predictand y3, under the given conditions of y1 and y2 based on the MG model, can be expressed as follows (Wilks, 2020):
where N signifies the Gaussian distribution function, denotes the conditional mean, and represents the conditional covariate matrix.
Furthermore, we remove the forecast values in a specific year of y1, y2, and y3, which denote , , and , respectively. Under this circumstance, the covariate matrix Σ regarding , , and can be written as follows:
where Covmn= Cov(, ) denotes the covariance between and (m= 1, 2, 3; n= 1, 2, 3). The forecast of specific years (i.e., ) can be derived as follows (Wilks, 2020):
where , , and represent the mean of , , and , respectively; and denote that y1 and y2 provided the forecast information at time t−i in a specific year. More details about forecasting agricultural drought based on the MG model can be found in Fig. 3.
3.2 The canonical vine copulas model using three-dimensional scenarios
Copulas can effectively combine multiple variables without the restriction of marginal distributions (Nelsen, 2006; Sarhadi et al., 2016; Wang et al., 2019; Xiong et al., 2014). They were initially utilized for deriving joint distributions of two-dimensional variables, as parameters are easy to assess and the analytical solution is easy to obtain (Liu et al., 2021a; Sadegh et al., 2017). However, in higher-dimensional (e.g., d≥ 3) scenarios, owing to the limitations of a great deal of parameters and complexity, the copulas (mainly referring to bivariate copulas) are difficult to promote and apply (Joe, 2014; Liu et al., 2018, 2021a; Sadegh et al., 2017). To overcome these limitations, Joe (1996) and Aas et al. (2009) developed vine copulas, which are a hierarchy of pair-copula constructions, for multidimensional cases. Vine copulas possess two subclasses: canonical vine copulas (C-vine copulas) and drawable vine copulas (D-vine copulas). Here, we mainly employed C-vine copulas to establish the forecast model of agricultural drought under three-dimensional conditions. Undoubtedly, the application of a similar scheme would be possible using D-vine copulas.
C-vine copulas may have numerous tree structures, especially in the case of higher dimensions, which are associated with the quantity and ordering of variables (Aas et al., 2009; Liu et al., 2018, 2021a; Wu et al., 2021b). Moreover, the different ordering of variables affects the estimation of the parameters of C-vine copulas (Liu et al., 2021a; Wang et al., 2019). Given the ordering of variables Y1, Y2, and Y3 for the three-dimensional C-vine copula model (hereinafter referred to as the 3C-vine model; Fig. 2a), the joint probability density function (PDF), g123, can be expressed as follows (Aas et al., 2009):
where g1, g2, and g3 correspond to the margin density functions of g1(y1), g2(y2), and g3(y3), respectively; c is the bivariate-copula density; and c12, c13, and c23|1 signify the abbreviation of c1,2[G1(y1), G2(y2)], c1,3[G1(y1), G3(y3)], and [G(y2|y1), G(y3|y1)], respectively. The term Gm(ym) corresponds to the cumulative density function (CDF) of the ym, and G(y2|y1) denotes the conditional probability distribution of y2 under known conditions of y1, which is similar for G(y3|y1). The Gaussian (or normal), Student t, Clayton, and Frank copulas as well as their rotated (survival) forms (Dißmann et al., 2013; Liu et al., 2021b) are utilized to obtain the optimal internal bivariate copulas for distinct trees in the 3C-vine model based on the Akaike information criterion (AIC). With the help of the “CDVineCondFit” R function in the “CDVineCopulaConditional” R package (Bevacqua, 2017a), based on the AIC, we selected the optimal tree structures (i.e., detected the suitable variable ordering; as seen in Fig. 2).
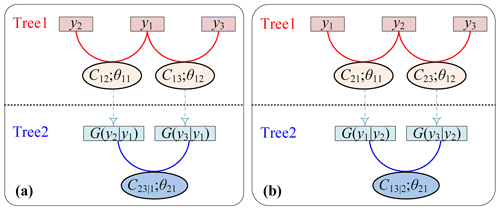
Figure 2Different schematics (two types) for C-vine copulas using three-dimensional scenarios. For the first type (a), the ordering variables are y1, y2, and y3; for the second type (b), they are y2, y1, and y3. C12(C21), C13(C23), and denote bivariate copulas with parameters of θ11, θ12, and θ21, respectively. Here, θij signifies the parameters of the jth edge with respect to the ith tree. G() represents conditional distribution functions.
A conditional copula density needs to be addressed in Eq. (4), i.e., G(y|w), where w is a d-dimensional vector w= (w1, …, wd). Here, regarding the conditional distribution of y given the conditions w, we introduced the h function, h(y, w; θ), to indicate G(y| w) as follows (Aas et al., 2009; Joe, 1996):
where θ denotes the parameter(s) of the bivariate-copula function , wj represents an arbitrary component of w, and w−j indicates the excluding element wj from the vector w.
If we set the ordering variables as y1, y2, and y3, the conditional variables as y1 and y2, and the predictand as y3, G(y3|y1, y2), based on Eq. (5), can be expressed as follows:
Here, θij (where i denotes a tree and j is an edge) represents the parameters of different conditional copulas in the 3C-vine model (Fig. 2a), and uk (k= 1, 2, 3) is the marginal CDF of yk. The CDF for each variable is substituted by the corresponding empirical Gringorten cumulative probability (Bevacqua et al., 2017b; Genest et al., 2009; Wu et al., 2021b).
Next, we introduced the τth copula–quantile curve (Chen et al., 2009; Liu et al., 2018) to simulate u3 based on Eq. (6) and derived its inverse distribution function as follows:
where N−1 and h−1 signify the inverse forms of the Gaussian distribution and the h function, respectively; y3 is the forecasted agricultural drought at time t (i.e., SSIt); and y1 and y2 are the predictors corresponding to the antecedent meteorological drought and agricultural drought persistence at time t−i (i.e., SPIt−i and SSIt−i, respectively). The “BiCopHfunc” and “BiCopHinv” R functions in the “VineCopula” R package (Nagler et al., 2021) were utilized to model the h function and its inverse form for Eq. (7), respectively.
The tree structure is related to the ordering variables; thus, when the ordering variables are y2, y1, and y3 (conditional variables are y1 and y2; Fig. 2b), Eqs. (6) and (7) can be changed analogously as follows:
For agricultural drought forecast via the 3C-vine model (see Fig. 3 for details), we first selected the best 3C-vine model (i.e., selected the best model from Eqs. (7) and (9) according to the minimum AIC). A sample size of 1,000 uniformly distributed random values was then generated over the [0, 1] interval using Monte Carlo simulation. Finally, the best 3C-vine model was utilized to obtain 1000 simulations (or estimations) of . The best forecast of was finally calculated using the mean value of these simulations. Note that leave-one-out cross-validation (LOOCV) (Wilks, 2020) is applied to forecast agricultural drought for each grid cell in August of every year during 1961–2018 based on the 3C-vine model or MG model, namely, each time one sample (or observation) was left for validation, and the rest were used to establish the 3C-vine model or MG model and obtain the corresponding parameters of these models. In other words, this process was repeated 58 times (the length of years used in this study) for a specific grid cell.
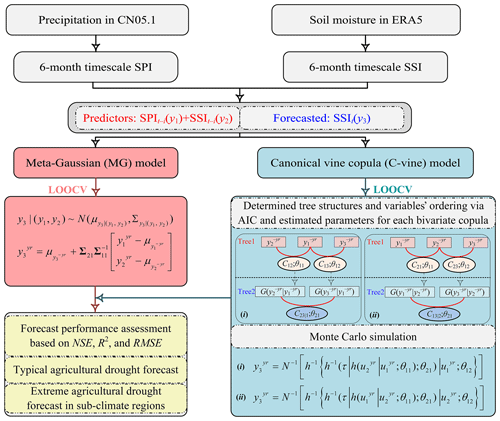
Figure 3Flowchart of agricultural drought forecasting based on the canonical vine copulas (3C-vine) and meta-Gaussian (MG) models using three-dimensional scenarios. Here, t denotes the target month (e.g., August), i signifies the lead times (1–3 months), LOOCV stands for leave-one-out cross-validation, indicates the series after removing a sample ( for a specific year, and is the agricultural drought forecast value for the target month of a specific year. Note that the optimal tree structure (i or ii on the right-hand side of this figure) is selected based on the AIC to forecast agricultural drought.
3.3 Performance metrics
Three evaluation metrics – the Nash–Sutcliffe efficiency (NSE), the coefficient of determination (R2), and the root-mean-square error (RMSE) – were utilized to assess the forecast performance of the 3C-vine and MG models. These metrics can be expressed as follows:
Here, n is the number of forecast periods; AOi and APi are the ith observed and forecasted agricultural droughts (i.e., SSI), respectively; and and denote the mean of the SSI observations and forecasts in the target month (e.g., August), respectively. Moreover, a more positive NSE and R2 value and a lower RMSE value indicate good forecast performance for the 3C-vine or MG models.
4.1 Correlation patterns of agricultural drought with potential predictors
The dependence between variables can be measured by the correlation coefficient, which indirectly characterizes the quantity of common information between two variables. We employed Kendall's correlation coefficient (τk) to measure the dependence of agricultural drought at current time t (SSIt, where t is August in this work) on the previous meteorological drought (SPIt−i, where i indicates the 1- to 3-month lag or lead times in this work) and agricultural drought persistence (SSIt−i). It should be mentioned that the widely used significant correlation threshold may overestimate or overinterpret the dependence between variables (Wilks, 2016). Therefore, we adopted the maximum false discovery rate (FDR) of 0.1 to correct τk at the 0.05 significance level (Benjamini and Hochberg, 1995; Röthlisberger and Martius, 2019; Wilks, 2016).
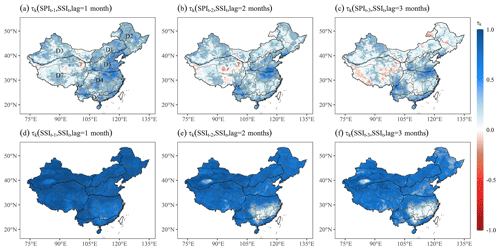
Figure 4Spatial patterns of 1- to 3-month lag times for the Kendall's correlation coefficient (τk) between SPIt−i and SSIt (where t denotes August, and i is the 1- to 3-month lag time) (top row) and between SSIt−i and SSIt (bottom row) for August during 1961–2018 over China. Note that the stippling indicates where τk is at a 0.05 significance level, which is corrected via the false discovery rate (FDR) of 0.1.
Figure 4 summarizes the 1- to 3-month lag τk between an antecedent SPI (SSI) and succedent SSI for August during 1961–2018 over China. For most regions of China, the previous meteorological drought or agricultural drought persistence (memory) showed significant positive correlations (i.e., stippling in Fig. 4) with the target agricultural drought when using 1- to 3-month lag times. Moreover, we found perfect agricultural drought memory over many regions of China (excluding D4, a humid climate region; Fig. 4e, f), as overlapping information existed in SSIt and SSIt−i. Additionally, the dependency pattern varied both temporally and spatially, and this phenomenon evidently occurred with the lag (or lead) time extended, especially between SPIt−i and SSIt (Fig. 4a, b, c). Overall, the prior meteorological drought and agricultural drought memory provided reliable and useful forecast information for subsequent agricultural drought for most areas of China.
4.2 Forecast performance comparison between the 3C-vine and MG models
We leveraged the MG model as a reference model to measure the performance of the 3C-vine model with respect to forecasting agricultural drought for the 1961–2018 period over China. Figure 5a–i show the difference in the NSE, R2, and RMSE between the 3C-vine and MG models (i.e., ΔNSE = NSE3C − NSEMG; − ; and ΔRMSE = RMSE3C −RMSEMG) for respective 1- to 3-month lead times for August. In terms of the spatial extent of ΔNSE > 0, ΔR2 > 0, and ΔRMSE < 0, the agricultural drought forecast ability of the 3C-vine model was superior to the MG model, which occupied 65 %, 68 %, and 58 % of land areas in China, respectively, for the 1-month lead SSI forecast (Fig. 5a, d, g). The relationship between the predictors and the forecasted variable was simple at a 1-month lead time; thus, the MG model better showed their connection. However, when using a prolonged lead time, the forecast skill of the 3C-vine model was superior to the MG model for most regions of China, accounting for 72 % and 74 % of land areas in China for ΔR2 > 0 for 2- and 3-month lead times, respectively (e.g., Fig. 5e, f). This indicates the 3C-vine model sufficiently utilized the forecasted information on previous meteorological drought and agricultural drought persistence, in contrast with the MG model under the same conditions.
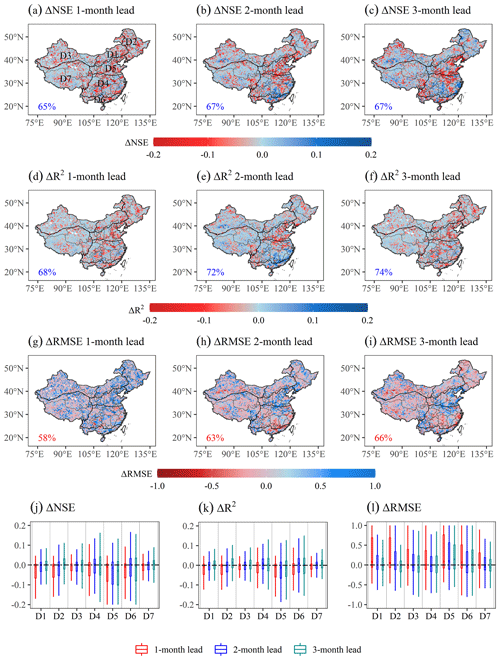
Figure 5Forecast performance based on (a)–(c) ΔNSE (the difference in NSE between the 3C-vine and MG models, NSE3C − NSEMG), (d–f) ΔR2 ( − ), and (g–i) ΔRMSE (RMSE3C − RMSEMG) for the 1- to 3-month lead times in August during 1961–2018 over China. The corresponding box plots of (j) ΔNSE, (k) ΔR2, and (l) ΔRMSE relative to a threshold of zero (horizontal black dash line) for agricultural drought forecast in August for 1- and 3-month lead times in climate regions D1–D7 over China are also shown. The percentages of ΔNSE > 0, ΔR2 > 0, and ΔRMSE < 0 are listed in the bottom left of the corresponding subfigures.
The forecast ability of the 3C-vine model, compared with the MG model, is limited over climate region D5 (e.g., Fig. 5b, c). This may be related to the fact that D5 is a crucial grain-producing region in China (Lu et al., 2012; Xiao et al., 2019; Zhang et al., 2016), and the related intensive anthropogenic activities (e.g., irrigation and urbanization) in the area may alter the linkage between meteorological drought and agricultural drought as well as the strength of the agricultural drought memory (AghaKouchak et al., 2021). To ensure food security, if D5 had experienced a drought event at the previous stage, agricultural managers and policymakers would have mitigated the drought through irrigation in a variety of ways, such as groundwater exploitation and reservoir operation (Zhang et al., 2016). However, in this case, the soil water obtaining the supplement from the irrigation water would affect the performance of agricultural drought forecast. Thus, in contrast with the MG model, the 3C-vine model yielded a better forecast performance for August under 1- to 3-month lead times for agricultural drought across most areas of China, except for the climate region D5.
4.3 Case study and subclimate region assessment
A severe drought hit most regions of China in summer 2018, especially southern and northern China, due to the abnormal impact of the western North Pacific subtropical high (Liu and Zhu, 2019; Zhang et al., 2020; Y. Zhang et al., 2018). We chose the agricultural drought that occurred in August of 2018 as a case study to investigate the forecast ability of the 3C-vine model. The MG model was selected as a benchmark model. Figure 6 presents the SSI observations and the 1- to 3-month lead time SSI forecasts for this agricultural drought using the 3C-vine and MG models. Obviously, the 1- to 3-month lead time SSI forecasts from the 3C-vine model resembled the observations (Fig. 6a, b, c, d), which captured the droughts that emerged in southern China, northern China, and northeastern China (i.e., climate regions D1–D2 and D4–D6). Comparing the 3C-vine model with the MG model using 2- to 3-month lead times (Fig. 6a, b, c, and d versus Fig. 6f and g), we observed the deteriorating forecast skill of the MG model in climate region D5, which tended toward a non-drought state (i.e., SSI > 0), but the 3C-vine model better forecasted the agricultural drought for these regions under the same conditions, although the severity of agricultural drought had some decrement. The above analyses indicated that the 3C-vine model, using previous meteorological drought and agricultural drought persistence as two predictors, had the ability to reliably forecast drought over many regions of China.
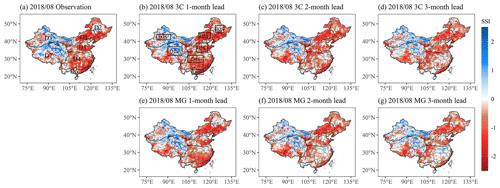
Figure 6SSI observations in August of 2018 (a) as well as the corresponding SSI forecasts for 1- to 3-month lead times utilizing the 3C-vine model (b–d) and the MG model (e–g) over China. The black rectangles (as shown in b) denote the typical areas (corresponding to D1S–D7S) selected in climate regions D1–D7.
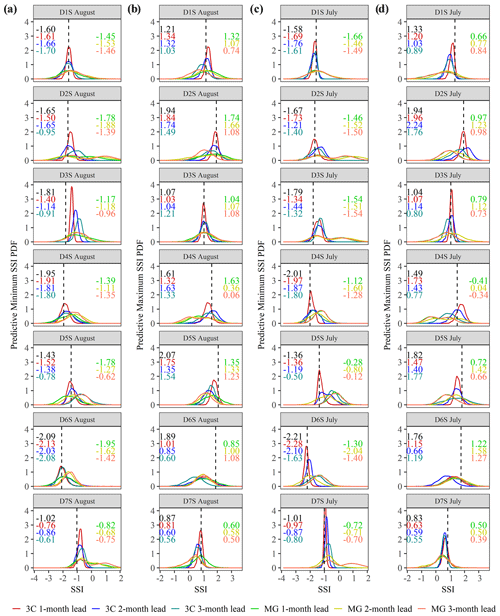
Figure 7Probability density function (PDF) curve of minimum (a, c) and maximum (b, d) SSI for 1- to 3-month lead times for August (a, b) and July (c, d) during the 1961–2018 period over seven selected typical areas in the climate regions D1–D7 (i.e., the black rectangles in Fig. 6b signify regions D1S–D7S; see labels). The black dashed line and text indicate the minimum and maximum SSI observations in August and July over D1S–D7S. The red (green), blue (yellow), and cyan (coral) text on the left (right) of each subfigure denote SSI forecasts for 1- to 3-month lead times in August or July from the 3C-vine model (MG model), which correspond to the abscissa projected by the peak point of each PDF.
Furthermore, to explore the skill of the 3C-vine model with respect to capturing the extrema of agricultural drought (i.e., minimum and maximum SSIs), we randomly selected a typical area (black rectangles in Fig. 6b) in each climate region. Note that these extreme SSI values were calculated using the spatial average in each typical region. Figure 7a and b show the probability density function (PDF) curves of the minimum and maximum SSIs for these selected typical regions (D1S–D7S) using the 3C-vine and MG models for 1- to 3-month lead times in August. Here, the vertical black dashed line denotes the SSI observation in each subplot. The x-axis value of the peak point (i.e., high probability) for each PDF curve is regarded as the best estimation of the SSI for diverse lead times. Using the 3C-vine model as an example (as for the MG model), for a minimum SSI with 1- to 2-month lead times, the differences between the forecasted SSI and the observed SSI were slight (except for D3S), and all cases reflected the drought state for these typical regions (Fig. 7a). The deteriorated skill of the 3C-vine and MG models in region D3S may be attributed to the lengthy response time existing between precipitation deficiency and soil moisture shortage, which is caused by limited precipitation that cannot effectively replenish soil moisture depletion due to the incrassation of the vadose zone. For the 3-month lead time, poor forecasts were produced in region D5S for the minimum SSI. This phenomenon may result in the agricultural manager utilizing irrigation to mitigate the effect of drought on crop growth; thus, the response relationship between meteorological drought and agricultural drought accordingly would change (Y. Xu et al., 2021).
For the maximum SSI forecasted utilizing the 3C-vine model (as for the MG model) over diverse regions, excellence forecast ability is displayed for the 1- to 3-month lead times (Fig. 7b), excluding regions D5S and D6S (PDF curve shifted left). Due to abundant precipitation and a higher soil moisture content in D6S, the shortened response time between precipitation and soil moisture (Y. Xu et al., 2021) may cause inferior forecasts for the 3C-vine model for the target month.
To display the robustness of the 3C-vine model with respect to forecasting agricultural drought in any month of interest, we further forecasted extreme agricultural drought in July for D1S–D7S (Fig. 7c, d). The difference between the forecasted and observed extreme SSIs for the MG model is larger than that for the 3C-vine model in distinct typical regions, e.g., the forecasted maximum SSI in July for D4S (Fig. 7d). The width of the PDF curve qualitatively provides an estimation of the forecast uncertainty of the 3C-vine and MG models. As shown in Fig. 7, in comparison with the 3C-vine model, we found that the width of the PDF curves of the MG model are broadened, indicating that the MG model produced more pronounced uncertainty with respect to agricultural drought forecast. Furthermore, the skill of the MG model tended to deteriorate over many selected typical regions, especially for 2- to 3-month lead times in July and August. Generally, compared with the MG model using different lead times, agricultural drought forecasts made by the 3C-vine model are more accurate across different typical regions, in terms of predictive uncertainty (i.e., the width of PDF curve) as well as the difference between observed and forecasted extreme SSIs (Fig. 7).
Moreover, to assess the forecast performance (according to the NSE, R2, and RMSE) of the 3C-vine model over each climate region, we counted the pixels contained in each climate region and constructed box plots for these performance metrics (Fig. 5j, k, l). We still selected the MG model as the reference model and obtained the difference between these two models (i.e., ΔNSE, ΔR2, and ΔRMSE). The forecast performance of the 3C-vine and MG models was generally consistent for a 1-month lead time for August over climate regions D1–D7 (the median percentiles of ΔNSE, ΔR2, and ΔRMSE were all around the zero line; Fig. 5j, k, l), indicating that the improved skill of the 3C-vine model was limited under the same conditions. Obviously, the median percentiles of ΔNSE and ΔR2 were greater than zero and ΔRMSE was lower than zero for the 2- to 3-month lead time SSI forecasts for August in the different climate regions D1–D7 (except for D5), indicating that the 3C-vine model shows better performance than the MG model with respect to forecasting agricultural drought over diverse climate regions of China.
In conclusion, based the ability of typical agricultural drought forecasts (Fig. 6), the agricultural drought extrema captured in selected typical regions (Fig. 7), and the comprehensive forecast performance shown in diverse climate regions (Fig. 5j, k, l), the 3C-vine model had a good forecast skill for 1- to 3-month lead times for agricultural drought in August over most areas of China.
This study developed a C-vine copula model to forecast agricultural drought over China in three dimensions in which antecedent meteorological drought and agricultural drought persistence were employed as two predictors. We selected the MG model as a reference, in terms of the difference in NSE, R2, and RMSE between the 3C-vine and MG models, in order to evaluate the forecast performance of the 3C-vine model. These performance metrics all showed that the 3C-vine model, especially for 2- to 3-month lead times, outperformed the MG model in many climate regions over China (except for D5, which lies in the humid and subhumid regions of northern China) (Fig. 5). Compared with the MG model, the 3C-vine model yielded good forecast skill for the selected typical agricultural droughts (Fig. 5). Furthermore, the nearly perfect forecast of extrema agricultural drought in typical regions (Fig. 7) further certified the excellent ability of the 3C-vine model.
Heterogeneous topography and anthropogenic activities (e.g., irrigation and urbanization) have certainly impacted precipitation interpolation and soil moisture simulation, which may depart from the actual precipitation or soil moisture conditions; notwithstanding, the precipitation of CN05.1 and the soil moisture of ERA5 show good performance with respect to drought monitoring and forecasting over China (Wang and Yuan, 2021; Wu et al., 2021b; Xu et al., 2009; T. Zhang et al., 2021; X. Zhang et al., 2019). The abovementioned factors can also influence the response (propagation) time from meteorological drought to agricultural drought as well as agricultural drought memory and can, thus, lead to the 3C-vine model falling short in some climate regions. To address this issue, we can comprehensively utilize multiple reanalysis data sets, e.g., the precipitation and soil moisture data in Global Land Data Assimilation System (GLDAS) and ERA5, to reduce the uncertainty resulting from a single data source (Wang and Yuan, 2021; Wu et al., 2021b). Currently, it is a challenge to consider irrigation activities in agricultural drought forecasting, especially at large spatial scales. In addition to the antecedent precipitation deficit, air temperature, relative humidity, and evapotranspiration may influence the soil moisture budget. Moreover, from the perspective of driving mechanisms, the effects of certain atmospheric circulation anomalies (e.g., the El Niño–Southern Oscillation, ENSO; the Pacific Decadal Oscillation, PDO; the and North Arctic Oscillation, NAO) on agricultural drought at regional and global scales can also be considered as predictors (Y. Zhang et al., 2021). Therefore, a more efficient space can be established by leveraging these predictors for forecasting agricultural drought.
In recent years, a myriad of extreme events, such as heat waves and flash droughts, have swept many regions around the globe (Wu et al., 2021a). These extreme events have a rapid onset of a few days or weeks and lead to devastating impacts on agricultural production, water resource security, and human well-being (Wang and Yuan, 2021; Yuan et al., 2019; Zscheischler et al., 2020). Therefore, agricultural drought forecasting at finer temporal scales (e.g., weekly) is essential for agricultural managers and policymakers to manage and plan water use. However, with the limited spatiotemporal resolution and the length of model samples, agricultural drought forecasting has not been carried out at sub-monthly or pentad temporal scales.
The limitation of this study is that we choose a “best” model from two C-vine copula candidate models (i.e., Fig. 2) as the ideal forecast. However, due to the inherent structural differences (i.e., ordering variables are different), the utilized best model may underestimate the forecast uncertainty (Liu et al., 2021a). Therefore, to reduce the predictive uncertainty and improve the forecast performance, a multi-model combination technique (e.g., Bayesian model averaging; Liu et al., 2021a; Long et al., 2017) could be considered to merge different C-vine copula candidate models. Moreover, as we only focused on C-vine copulas and several bivariate-copula functions, other D-vine copulas or regular vine copulas as well as a multitude of bivariate-copula families (Sadegh et al., 2017) could be investigated to establish the forecast model for agricultural drought in following work.
The gridded monthly CN05.1 precipitation data with a 0.25∘ spatial resolution were provided by the Climate Change Research Center, Chinese Academy of Sciences, for the 1961–2018 period (http://ccrc.iap.ac.cn/resource/detail?id=228, Wu and Gao, 2021). The gridded monthly soil moisture data at three soil depths (0–7, 7–28, and 28–100 cm) from the European Center for Medium-Range Weather Forecasts (ECMWF) ERA5 reanalysis data sets are available at https://cds.climate.copernicus.eu/cdsapp#!/dataset/reanalysis-era5-single-levels-monthly-means-preliminary-back-extension?tab=overview (Bell et al., 2020) for the 1961–1978 period and at https://doi.org/10.24381/cds.f17050d7 (Hersbach et al., 2019) for the 1979–2018 period.
HW was responsible for conceptualizing the study, developing the methodology and software, creating the figures, and writing the manuscript. XS contributed to reviewing and editing the manuscript, curating and validating the data, carrying out the investigation and formal analysis, acquiring funding, and supervising the study. VPS reviewed and edited the manuscript and supervised the study. TZ was responsible for carrying out the formal analysis and investigation. JQ curated data and carried out the investigation. SH contributed to reviewing and editing the paper and carrying out the investigation.
The contact author has declared that none of the authors has any competing interests.
Publisher’s note: Copernicus Publications remains neutral with regard to jurisdictional claims in published maps and institutional affiliations.
The authors would like to thank Mohammad Nazeri Tahroudi, the anonymous reviewer, and the editor (Carlo De Michele) for their constructive comments and suggestions which contributed to improving the quality of the paper. This study was financially supported by the National Natural Science Foundation of China (grant nos. 51879222 and 52079111).
This research has been supported by the National Natural Science Foundation of China (grant nos. 51879222 and 52079111).
This paper was edited by Carlo De Michele and reviewed by Mohammad Nazeri Tahroudi and one anonymous referee.
Aas, K. and Berg, D.: Models for construction of multivariate dependence – a comparison study, Eur. J. Financ., 15, 639–659, https://doi.org/10.1080/13518470802588767, 2009.
Aas, K., Czado, C., Frigessi, A., and Bakken, H.: Pair-copula constructions of multiple dependence, Insur. Math. Econ., 44, 182–198, https://doi.org/10.1016/j.insmatheco.2007.02.001, 2009.
AghaKouchak, A., Mirchi, A., Madani, K., Di Baldassarre, G., Nazemi, A., Alborzi, A., Anjileli, H., Azarderakhsh, M., Chiang, F., Hassanzadeh, E., Huning, L. S., Mallakpour, I., Martinez, A., Mazdiyasni, O., Moftakhari, H., Norouzi, H., Sadegh, M., Sadeqi, D., Van Loon, A. F., and Wanders, N.: Anthropogenic Drought: Definition, Challenges, and Opportunities, Rev. Geophys., 59, e2019RG000683, https://doi.org/10.1029/2019rg000683, 2021.
Bedford, T. and Cooke, R. M.: Vines – A new graphical model for dependent random variables, Ann. Stat., 30, 1031–1068, https://doi.org/10.1214/aos/1031689016, 2002.
Bell, B., Hersbach, H., Berrisford, P., Dahlgren, P., Horányi, A., Muñoz Sabater, J., Nicolas, J., Radu, R., Schepers, D., Simmons, A., Soci, C., and Thépaut, J.-N.: ERA5 monthly averaged data on single levels from 1950 to 1978 (preliminary version). Copernicus Climate Change Service (C3S) Climate Data Store (CDS) [data set], https://cds.climate.copernicus.eu/cdsapp#!/dataset/reanalysis-era5-single-levels-monthly-means-preliminary-back-extension?tab=overview (last access: 14 May 2022), 2020.
Benjamini, Y. and Hochberg, Y.: Controlling the false discovery rate: A practical and powerful approach to multiple testing, J. R. Stat. Soc. Ser. B-Met., 57, 289–300, https://doi.org/10.1111/j.2517-6161.1995.tb02031.x, 1995.
Bevacqua, E.: CDVineCopulaConditional: Sampling from conditional C- and D-vine copulas, R package, version 0.1.1, https://CRAN.R-project.org/package=CDVineCopulaConditional (last access: 15 April 2022), 2017a.
Bevacqua, E., Maraun, D., Hobæk Haff, I., Widmann, M., and Vrac, M.: Multivariate statistical modelling of compound events via pair-copula constructions: analysis of floods in Ravenna (Italy), Hydrol. Earth Syst. Sci., 21, 2701–2723, https://doi.org/10.5194/hess-21-2701-2017, 2017b.
Chen, X., Koenker, R., and Xiao, Z.: Copula-based nonlinear quantile autoregression, Econom. J., 12, S50–S67, https://doi.org/10.1111/j.1368-423X.2008.00274.x, 2009.
De Michele, C. and Salvadori, G.: A Generalized Pareto intensity-duration model of storm rainfall exploiting 2-Copulas. J. Geophys. Res., 108, 4067, https://doi.org/10.1029/2002jd002534, 2003.
De Michele, C., Salvadori, G., Vezzoli, R., and Pecora, S.: Multivariate assessment of droughts: Frequency analysis and dynamic return period, Water Resour. Res., 49, 6985–6994, https://doi.org/10.1002/wrcr.20551, 2013.
Dißmann, J., Brechmann, E. C., Czado, C., and Kurowicka, D.: Selecting and estimating regular vine copulae and application to financial returns, Comput. Stat. Data Anal., 59, 52–69, https://doi.org/10.1016/j.csda.2012.08.010, 2013.
FAO: The impact of disasters and crises on agriculture and food security, Food and Agriculture Organization of the United Nations, Rome, https://doi.org/10.4060/cb3673en, 2021.
Favre, A.-C., El Adlouni, S., Perreault, L., Thiémonge, N., and Bobée, B.: Multivariate hydrological frequency analysis using copulas. Water Resour. Res., 40, W01101, https://doi.org/10.1029/2003wr002456, 2004.
Ganguli, P. and Reddy, M. J.: Ensemble prediction of regional droughts using climate inputs and the SVM-copula approach, Hydrol. Process., 28, 4989–5009, https://doi.org/10.1002/hyp.9966, 2014.
Genest, C., Rémillard, B., and Beaudoin, D.: Goodness-of-fit tests for copulas: A review and a power study, Insur. Math. Econ., 44, 199–213, https://doi.org/10.1016/j.insmatheco.2007.10.005, 2009.
Gringorten, I. I.: A plotting rule for extreme probability paper, J. Geophys. Res., 68, 813–814, https://doi.org/10.1029/JZ068i003p00813, 1963.
Hao, Z., Hao, F., Singh, V. P., Sun, A. Y., and Xia, Y.: Probabilistic prediction of hydrologic drought using a conditional probability approach based on the meta-Gaussian model, J. Hydrol., 542, 772–780, https://doi.org/10.1016/j.jhydrol.2016.09.048, 2016.
Hao, Z., Hao, F., Singh, V. P., and Ouyang W.: Quantitative risk assessment of the effects of drought on extreme temperature in eastern China, J. Geophys. Res.-Atmos., 122, 9050–9059, https://doi.org/10.1002/2017JD027030, 2017.
Hao, Z., Hao, F., Singh, V. P., and Zhang, X.: Statistical prediction of the severity of compound dry-hot events based on El Niño-Southern Oscillation, J. Hydrol., 572, 243–250, https://doi.org/10.1016/j.jhydrol.2019.03.001, 2019a.
Hao, Z., Hao, F., Xia, Y., Singh, V. P., and Zhang, X.: A monitoring and prediction system for compound dry and hot events, Environ. Res. Lett., 14, 114034, https://doi.org/10.1088/1748-9326/ab4df5, 2019b.
He, L., Hao, X., Li, H., and Han, T.: How Do Extreme Summer Precipitation Events Over Eastern China Subregions Change?, Geophys. Res. Lett., 48, e2020GL091849, https://doi.org/10.1029/2020GL091849, 2021.
Hemri, S., Lisniak, D., and Klein, B.: Multivariate postprocessing techniques for probabilistic hydrological forecasting, Water Resour. Res., 51, 7436–7451, https://doi.org/10.1002/2014wr016473, 2015.
Hersbach, H., Bell, B., Berrisford, P., Biavati, G., Horányi, A., Muñoz Sabater, J., Nicolas, J., Peubey, C., Radu, R., Rozum, I., Schepers, D., Simmons, A., Soci, C., Dee, D., and Thépaut, J.-N.: ERA5 monthly averaged data on single levels from 1959 to present, Copernicus Climate Change Service (C3S) Climate Data Store (CDS) [data set], https://doi.org/10.24381/cds.f17050d7, 2019.
Joe, H.: Families of m-variate distributions with given margins and bivariate dependence parameters, Institute of Mathematical Statistics Lecture Notes – Monograph Series, 28, 120–141, https://doi.org/10.1214/lnms/1215452614, 1996.
Joe, H.: Dependence modeling with copulas, Chapman and Hall/CRC, ISBN 9780429103186, https://doi.org/10.1201/b17116, 2014.
Lesk, C., Rowhani, P., and Ramankutty, N.: Influence of extreme weather disasters on global crop production, Nature, 529, 84–87, https://doi.org/10.1038/nature16467, 2016.
Liu, B. and Zhu, C.: Extremely Late Onset of the 2018 South China Sea Summer Monsoon Following a La Niña Event: Effects of Triple SST Anomaly Mode in the North Atlantic and a Weaker Mongolian Cyclone, Geophys. Res. Lett., 46, 2956–2963, https://doi.org/10.1029/2018gl081718, 2019.
Liu, Z., Cheng, L., Hao, Z., Li, J., Thorstensen, A., and Gao, H.: A Framework for Exploring Joint Effects of Conditional Factors on Compound Floods, Water Resour. Res., 54, 2681–2696, https://doi.org/10.1002/2017wr021662, 2018.
Liu, Z., Cheng, L., Lin, K., and Cai, H.: A hybrid bayesian vine model for water level prediction, Environ. Modell. Softw., 142, 105075, https://doi.org/10.1016/j.envsoft.2021.105075, 2021a.
Liu, Z., Xie, Y., Cheng, L., Lin, K., Tu, X., and Chen, X.: Stability of spatial dependence structure of extreme precipitation and the concurrent risk over a nested basin, J. Hydrol., 602, 126766, https://doi.org/10.1016/j.jhydrol.2021.126766, 2021b.
Long, D., Pan, Y., Zhou, J., Chen, Y., Hou, X., Hong, Y., Scanlon, B. R., and Longuevergne, L.: Global analysis of spatiotemporal variability in merged total water storage changes using multiple GRACE products and global hydrological models, Remote Sens. Environ., 192, 198–216, https://doi.org/10.1016/j.rse.2017.02.011, 2017.
Long, D., Bai, L., Yan, L., Zhang, C., Yang, W., Lei, H., Quan, J., Meng, X., and Shi, C.: Generation of spatially complete and daily continuous surface soil moisture of high spatial resolution, Remote Sens. Environ., 233, 111364, https://doi.org/10.1016/j.rse.2019.111364, 2019.
Lu, Y., Wu, K., Jiang, Y., Guo, Y., and Desneux, N.: Widespread adoption of Bt cotton and insecticide decrease promotes biocontrol services, Nature, 487, 362–365, https://doi.org/10.1038/nature11153, 2012.
Ma, F., Luo, L., Ye, A., and Duan, Q.: Seasonal drought predictability and forecast skill in the semi-arid endorheic Heihe River basin in northwestern China, Hydrol. Earth Syst. Sci., 22, 5697–5709, https://doi.org/10.5194/hess-22-5697-2018, 2018.
Modanesi, S., Massari, C., Camici, S., Brocca, L., and Amarnath, G.: Do Satellite Surface Soil Moisture Observations Better Retain Information About Crop-Yield Variability in Drought Conditions?, Water Resour. Res., 56, e2019WR025855, https://doi.org/10.1029/2019wr025855, 2020.
Nagler, T., Schepsmeier, U., Stoeber, J., Brechmann, E. C., Graeler, B., Erhardt, T., Almeida, C., Min, A., Czado, C., Hofmann, M., Killiches, M., Joe, H., and Vatter, T.: VineCopula: Statistical Inference of Vine Copulas, R Package Version 2.4.2, https://CRAN.R-project.org/package=VineCopula (last access: 15 April 2022), 2021.
Nelsen, R. B.: An Introduction to Copulas, Springer, NY, https://doi.org/10.1007/0-387-28678-0, 2006.
Orth, R. and Destouni, G.: Drought reduces blue-water fluxes more strongly than green-water fluxes in Europe, Nat. Commun., 9, 3602, https://doi.org/10.1038/s41467-018-06013-7, 2018.
Röthlisberger, M. and Martius, O.: Quantifying the Local Effect of Northern Hemisphere Atmospheric Blocks on the Persistence of Summer Hot and Dry Spells, Geophys. Res. Lett., 46, 10101–10111, https://doi.org/10.1029/2019gl083745, 2019.
Sadegh, M., Ragno, E., and AghaKouchak, A.: Multivariate Copula Analysis Toolbox (MvCAT): Describing dependence and underlying uncertainty using a Bayesian framework, Water Resour. Res., 53, 5166–5183, https://doi.org/10.1002/2016wr020242, 2017.
Salvadori, G. and De Michele, C.: Frequency analysis via copulas: Theoretical aspects and applications to hydrological events, Water Resour. Res., 40, W12511, https://doi.org/10.1029/2004wr003133, 2004.
Sarhadi, A., Burn, D. H., Concepción Ausín, M., and Wiper, M. P.: Time-varying nonstationary multivariate risk analysis using a dynamic Bayesian copula, Water Resour. Res., 52, 2327–2349, https://doi.org/10.1002/2015wr018525, 2016.
Sklar, A.: Fonctions de Répartition à Dimensions et Leurs marges, 8, Thesis, Publications de l'Institut de Statistique de L'Université de Paris, Paris, France, 1959.
Su, B., Huang, J., Fischer, T., Wang, Y., Kundzewicz, Z. W., Zhai, J., Sun, H., Wang, A., Zeng, X., Wang, G., Tao, H., Gemmer, M., Li, X., and Jiang, T.: Drought losses in China might double between the 1.5 ∘C and 2.0 ∘C warming, P. Natl. Acad. Sci. USA, 115, 10600–10605, https://doi.org/10.1073/pnas.1802129115, 2018.
Vernieuwe, H., Vandenberghe, S., De Baets, B., and Verhoest, N. E. C.: A continuous rainfall model based on vine copulas, Hydrol. Earth Syst. Sci., 19, 2685–2699, https://doi.org/10.5194/hess-19-2685-2015, 2015.
Wang, W., Dong, Z., Lall, U., Dong, N., and Yang, M.: Monthly Streamflow Simulation for the Headwater Catchment of the Yellow River Basin With a Hybrid Statistical-Dynamical Model, Water Resour. Res., 55, 7606–7621, https://doi.org/10.1029/2019wr025103, 2019.
Wang, Y. and Yuan, X.: Anthropogenic Speeding Up of South China Flash Droughts as Exemplified by the 2019 Summer-Autumn Transition Season, Geophys. Res. Lett., 48, e2020GL091901, https://doi.org/10.1029/2020gl091901, 2021.
Wilks, D. S.: Statistical methods in the atmospheric sciences, Elsevier, https://doi.org/10.1016/C2017-0-03921-6, 2020.
Wilks, D. S.: “The Stippling Shows Statistically Significant Grid Points”: How Research Results are Routinely Overstated and Overinterpreted, and What to Do about It, B. Am. Meteorol. Soc., 97, 2263–2273, https://doi.org/10.1175/bams-d-15-00267.1, 2016.
Wu, H., Su, X., and Singh, V. P.: Blended Dry and Hot Events Index for Monitoring Dry–Hot Events Over Global Land Areas, Geophys. Res. Lett., 48, e2021GL096181, https://doi.org/10.1029/2021GL096181, 2021a.
Wu, H., Su, X., Singh, V. P., Feng, K., and Niu, J.: Agricultural Drought Prediction Based on Conditional Distributions of Vine Copulas, Water Resour. Res., 57, e2021WR029562, https://doi.org/10.1029/2021wr029562, 2021b.
Wu, H., Su, X., and Zhang, G.: Prediction of agricultural drought in China based on Meta-Gaussian model, Acta Geogr. Sin., 76, 525–538, https://doi.org/10.11821/dlxb202103003, 2021c.
Wu, J. and Gao, X.: A CN05.1 high resolution gridded observation dataset over China region, National Climate Center, China Meteorological Administration, Climate Change Research Center, Chinese Academy of Sciences [data set], http://ccrc.iap.ac.cn/resource/detail?id=228, last access: 17 August 2021.
Wu, J., Chen, X., Yu, Z., Yao, H., Li, W., and Zhang, D.: Assessing the impact of human regulations on hydrological drought development and recovery based on a “simulated-observed” comparison of the SWAT model, J. Hydrol., 577, 123990, https://doi.org/10.1016/j.jhydrol.2019.123990, 2019.
Wu, J., Gao, X., Giorgi, F., and Chen, D.: Changes of effective temperature and cold/hot days in late decades over China based on a high resolution gridded observation dataset, Int. J. Climatol., 37, 788–800, https://doi.org/10.1002/joc.5038, 2017.
Xiao, G., Zhao, Z., Liang, L., Meng, F., Wu, W., and Guo, Y.: Improving nitrogen and water use efficiency in a wheat-maize rotation system in the North China Plain using optimized farming practices, Agr. Water Manage., 212, 172–180, https://doi.org/10.1016/j.agwat.2018.09.011, 2019.
Xiong, L., Yu, K.-x., and Gottschalk, L.: Estimation of the distribution of annual runoff from climatic variables using copulas, Water Resour. Res., 50, 7134–7152, https://doi.org/10.1002/2013wr015159, 2014.
Xu, L., Chen, N., Chen, Z., Zhang, C., and Yu, H.: Spatiotemporal forecasting in earth system science: Methods, uncertainties, predictability and future directions, Earth-Sci. Rev., 222, 103828, https://doi.org/10.1016/j.earscirev.2021.103828, 2021.
Xu, Y., Gao, X., Shen, Y., Xu, C., Shi, Y., and Giorgi, F.: A daily temperature dataset over China and its application in validating a RCM simulation, Adv. Atmos. Sci., 26, 763–772, https://doi.org/10.1007/s00376-009-9029-z, 2009.
Xu, Y., Zhang, X., Hao, Z., Singh, V. P., and Hao, F.: Characterization of agricultural drought propagation over China based on bivariate probabilistic quantification, J. Hydrol., 598, 126194, https://doi.org/10.1016/j.jhydrol.2021.126194, 2021.
Yao, N., Li, Y., Lei, T., and Peng, L.: Drought evolution, severity and trends in mainland China over 1961–2013, Sci. Total Environ., 616–617, 73–89, https://doi.org/10.1016/j.scitotenv.2017.10.327, 2018.
Yuan, X., Wang, L., Wu, P., Ji, P., Sheffield, J., and Zhang, M.: Anthropogenic shift towards higher risk of flash drought over China, Nat. Commun., 10, 4661, https://doi.org/10.1038/s41467-019-12692-7, 2019.
Zhang, J., Mu, Q., and Huang, J.: Assessing the remotely sensed Drought Severity Index for agricultural drought monitoring and impact analysis in North China, Ecol. Indic., 63, 296–309, https://doi.org/10.1016/j.ecolind.2015.11.062, 2016.
Zhang, L. and Singh, V. P.: Copulas and their applications in water resources engineering, Cambridge University Press, ISBN 9781108474252, https://doi.org/10.1017/9781108565103, 2019.
Zhang, L., Zhou, T., Chen, X., Wu, P., Christidis, N., and Lott, F. C.: The late spring drought of 2018 in South China, B. Am. Meteorol. Soc., 101, S59–S64, https://doi.org/10.1175/BAMS-D-19-0202.1, 2020.
Zhang, Q., Qi, T., Singh, V. P., Chen, Y. D., and Xiao, M.: Regional Frequency Analysis of Droughts in China: A Multivariate Perspective, Water Resour. Manag., 29, 1767–1787, https://doi.org/10.1007/s11269-014-0910-x, 2015.
Zhang, Q., Li, Q., Singh, V. P., Shi, P., Huang, Q., and Sun, P.: Nonparametric integrated agrometeorological drought monitoring: Model development and application, J. Geophys. Res.-Atmos., 123, 73–88, https://doi.org/10.1002/2017JD027448, 2018.
Zhang, Q., Yu, H., Sun, P., Singh, V. P., and Shi, P.: Multisource data based agricultural drought monitoring and agricultural loss in China, Global Planet. Change, 172, 298–306, https://doi.org/10.1016/j.gloplacha.2018.10.017, 2019.
Zhang, T., Su, X., and Feng, K.: The development of a novel nonstationary meteorological and hydrological drought index using the climatic and anthropogenic indices as covariates, Sci. Total Environ., 786, 147385, https://doi.org/10.1016/j.scitotenv.2021.147385, 2021.
Zhang, T., Su, X., Zhang, G., Wu, H., Wang, G., and Chu, J.: Evaluation of the impacts of human activities on propagation from meteorological drought to hydrological drought in the Weihe River Basin, China, Sci. Total Environ., 819, 153030, https://doi.org/10.1016/j.scitotenv.2022.153030, 2022.
Zhang, X., Su, Z., Lv, J., Liu, W., Ma, M., Peng, J., and Leng, G.: A Set of Satellite-Based Near Real-Time Meteorological Drought Monitoring Data over China, Remote Sens., 11, 453, https://doi.org/10.3390/rs11040453, 2019.
Zhang, Y., Wang, Z., Sha, S., and Feng, J.: Drought Events and Its Causes in Summer of 2018 in China, J. Arid Meteoro., 36, 884–892, https://doi.org/10.11755/j.issn.1006-7639(2018)-05-0884, 2018.
Zhang, Y., Hao, Z., Feng, S., Zhang, X., Xu, Y., and Hao, F.: Agricultural drought prediction in China based on drought propagation and large-scale drivers, Agr. Water Manage., 255, 107028, https://doi.org/10.1016/j.agwat.2021.107028, 2021.
Zhao, S.: A new scheme for comprehensive physical regionalization in China, Acta Geogr. Sin., 38, 1–10, 1983.
Zhou, S., Williams, A. P., Berg, A. M., Cook, B. I., Zhang, Y., Hagemann, S., Lorenz, R., Seneviratne, S. I., and Gentine, P.: Land-atmosphere feedbacks exacerbate concurrent soil drought and atmospheric aridity, P. Natl. Acad. Sci. USA, 116, 18848–18853, https://doi.org/10.1073/pnas.1904955116, 2019.
Zscheischler, J., Martius, O., Westra, S., Bevacqua, E., Raymond, C., Horton, R. M., van den Hurk, B., AghaKouchak, A., Jézéquel, A., Mahecha, M. D., Maraun, D., Ramos, A. M., Ridder, N. N., Thiery, W., and Vignotto, E.: A typology of compound weather and climate events, Nature Reviews Earth & Environment, 1, 333–347, https://doi.org/10.1038/s43017-020-0060-z, 2020.