the Creative Commons Attribution 4.0 License.
the Creative Commons Attribution 4.0 License.
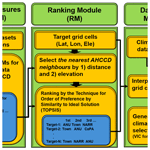
Hybrid climate datasets from a climate data evaluation system and their impacts on hydrologic simulations for the Athabasca River basin in Canada
Hyung-Il Eum
Anil Gupta
A reliable climate dataset is the backbone for modelling the essential processes of the water cycle and predicting future conditions. Although a number of gridded climate datasets are available for the North American content which provide reasonable estimates of climatic conditions in the region, there are inherent inconsistencies in these available climate datasets (e.g., spatially and temporally varying data accuracies, meteorological parameters, lengths of records, spatial coverage, temporal resolution, etc.). These inconsistencies raise questions as to which datasets are the most suitable for the study area and how to systematically combine these datasets to produce a reliable climate dataset for climate studies and hydrological modelling. This study suggests a framework called the REFerence Reliability Evaluation System (REFRES) that systematically ranks multiple climate datasets to generate a hybrid climate dataset for a region. To demonstrate the usefulness of the proposed framework, REFRES was applied to produce a historical hybrid climate dataset for the Athabasca River basin (ARB) in Alberta, Canada. A proxy validation was also conducted to prove the applicability of the generated hybrid climate datasets to hydrologic simulations. This study evaluated five climate datasets, including the station-based gridded climate datasets ANUSPLIN (Australia National University Spline), Alberta Township, and the Pacific Climate Impacts Consortium's (PCIC) PNWNAmet (PCIC NorthWest North America meteorological dataset), a multi-source gridded dataset (Canadian Precipitation Analysis; CaPA), and a reanalysis-based dataset (North American Regional Reanalysis; NARR). The results showed that the gridded climate interpolated from station data performed better than multi-source- and reanalysis-based climate datasets. For the Athabasca River basin, Township and ANUSPLIN were ranked first for precipitation and temperature, respectively. The proxy validation also confirmed the utility of hybrid climate datasets in hydrologic simulations compared with the other five individual climate datasets investigated in this study. These results indicate that the hybrid climate dataset provides the best representation of historical climatic conditions and, thus, enhances the reliability of hydrologic simulations.
- Article
(5932 KB) - Full-text XML
- BibTeX
- EndNote
A reliable historical climate dataset is essential to understanding the climatic and hydrological characteristics of a watershed, as it is crucial forcing input data for simulating key processes of the water and energy cycles in impact models (Deacu et al., 2012; Essou et al., 2016; Wong et al., 2017). Although climate monitoring networks have advanced over the last decades, poor network density still exists, especially in western mountainous and northern parts of Canada. Moreover, climate observations are often spatially interpolated to cover ungauged regions, which may cause unexpected erroneous model predictions as a consequence of the sparse measurement network, especially for mountainous areas affected by orographic effects (Rinke et al., 2004; Wang and Lin, 2015).
As advances in numerical hydrologic and hydrodynamic modelling have increased the capability and reliability in simulating complex natural processes to detect anthropogenic and natural climate changes, a need for temporally and spatially reliable climate data has also grown to accommodate the requirements of input data for numerical models (Shen et al., 2010; Shrestha et al., 2012; Islam and Déry, 2017). For instance, process-based distributed hydrologic models have a grid-based structure that requires input data for each grid cell. However, a simple spatial interpolation of observational station data to all model grid cells may not produce a reliable input forcing dataset for hydrologic models, particularly in a region with a sparse gauging network. A reliable historical climate dataset is also crucial in climate change studies when used for statistical downscaling techniques that employ the relationships between observations and outputs of global (or regional) climate models to produce climate forcing at regional or local scales. Since the resolution of products from a statistical downscaling technique usually corresponds to that of the historical climate dataset (Werner and Cannon, 2016; Eum and Cannon, 2017), the availability of temporally and spatially reliable historical climate data is essential for climate-related impact studies (Christensen and Lettenmaier, 2007; Kay et al., 2009; Gutmann et al., 2014; Eum et al., 2016).
A number of high-resolution gridded climate datasets have been developed for various applications such as intercomparison studies (Eum et al., 2014a; Wong et al., 2017) and hydrologic modelling (Choi et al., 2009; Eum et al., 2016). There are various types of gridded climate datasets available for the North American region: (1) station-based interpolated, (2) station-based multi-source, and (3) reanalysis-based multi-source (Wong et al., 2017). By interpolation of observational station data, long-term gridded climate datasets have been produced over various domains defined by stations incorporated such as the Canada-wide Australia National University Spline (ANUSPLIN, Hutchison et al., 2009), the Alberta Township data (Shen et al., 2001), and the Pacific Climate Impacts Consortium (PCIC) NorthWest North America meteorological (PNWNAmet) datasets (Werner et al., 2019). The Canadian Precipitation Analysis (CaPA) system, a multi-source-based climate dataset, has been developed to produce near-real-time precipitation analyses (6 h accumulated precipitation) over North America at 15 km resolution which has been further improved to 10 km resolution (Lespinas et al., 2015). North American Regional Reanalysis (NARR), one of the reanalysis-based datasets derived from a regional climate model (∼32 km), has been tested as an alternative climate dataset (Choi et al., 2009; Praskievicz and Bartlein, 2014; Essou et al., 2016; Islam and Déry, 2017).
In most of the large-scale modelling studies, multiple climate datasets were combined to cover the entire modelling domain for all the required climate variables, usually without evaluating the performance of different climate datasets for the modelled regions (Faramarzi et al., 2015; R. R. Shrestha et al., 2017; Wong et al., 2017). The lack of performance indicators for available climate datasets may cause the inappropriate application of these datasets for various large-scale studies, resulting in unreliable outputs, e.g., considerable bias in statistical downscaling studies. Therefore, selecting reliable gridded climate data for a study area is crucial for hydrological or climate-related studies (Werner and Cannon, 2016; Eum et al., 2014a, 2017). Eum et al. (2014a) intercompared three gridded climate datasets (ANUSPLIN, NARR, and CaPA) for the Athabasca River basin (ARB) and found that data accuracy varies spatially and temporally over the basin mainly due to the heterogeneity of spatial density of the observational climate network in the basin and limited data assimilation. Wong et al. (2017) also intercompared gridded precipitation datasets derived from different data sources over Canada. Few studies have attempted to incorporate spatially varied performance measures of various climate datasets to produce a complete long-term historical climate dataset for a study region (Faramarzi et al., 2015; R. R. Shrestha et al., 2017). In addition, no systematic framework has been developed yet that could be employed by climatic and hydrologic studies.
Therefore, this study provides a framework, called the REFerence Reliability Evaluation System (REFRES), to systematically determine the ranking of multiple climate datasets based on their performance and generate a hybrid climate dataset for a study region by extracting the best candidate (based on the ranking) from multiple climate datasets available in a repository. Several performance measures were identified and calculated by comparing this to the Adjusted and Homogenized Canadian Climate Data (AHCCD) over western Canada. Based on the performance measures, the climate datasets were ranked to generate a hybrid climate dataset for the area of interest (target area). A hybrid dataset for two climate variables, precipitation and temperature, key forcing for hydrological modelling, was produced for a period of the record that is fully covered by the multiple climate datasets. To validate the applicability of the hybrid climate dataset, a proxy validation approach was employed by comparing simulated streamflows derived from the generated hybrid climate data and other available climate datasets to recorded streamflows at various hydrometric stations in the Athabasca River basin. Streamflows were simulated using a hydrologic model (Variable Infiltration Capacity; VIC) calibrated and forced by individual climate datasets and the generated hybrid climate dataset. Therefore, the aims of this study are (1) to develop a methodology (i.e., REFRES) to compare and rank multiple gridded climate datasets based on the proposed performance measures and to generate the hybrid climate dataset and (2) to validate the hybrid climate dataset using the proxy validation approach for the Athabasca River basin as a case study to confirm the applicability of the hybrid climate dataset to hydrologic simulations.
2.1 Adjusted and Homogenized Canadian Climate Data (AHCCD)
Climate station observations in Canada are available from the national climate data and information archive of Environment and Climate Change Canada (ECCC, http://climate.weather.gc.ca/, last access: 11 December 2019). Besides the variable number of observations due to frequent changes in operations including the discontinuation of stations, the observations are also subject to various errors from the undercatch of solid precipitation, orographic effects, and malfunctions while taking measurements (Mekis and Hogg, 1999; Rinke et al., 2004).
Mekis and Vincent (2011) adjusted daily rainfall and snowfall data, considering wind undercatch, evaporation, and wetting losses corresponding to the types of gauges for 450 stations in Canada. The most recent version released in 2016 provides the adjusted precipitation observations, expanded to 464 precipitation stations. Vincent et al. (2012) produced the second generation of homogenized daily temperature data by adjusting the time series at 120 synoptic stations to account for a nation-wide change in observing time and homogenizing discontinuities over 338 temperature (daily minimum and maximum) stations in Canada. The adjusted and homogenized Canadian Climate Data (AHCCD) are available through Environment and Climate Change Canada (https://open.canada.ca/data/en/dataset/9c4ebc00-3ea4-4fe0-8bf2-66cfe1cddd1d, last access: 11 December 2019).
Considering that archived raw station data were used to produce the historical gridded climate datasets used in our study, the evaluation of performance at the AHCCD stations is more meaningful because the AHCCD data were adjusted to account for the known measurement issues in the raw station data. For example, the adjusted precipitation data are higher by 5 % to 20 %, varying with topographic characteristics (Mekis and Vincent, 2011). Therefore, the AHCCD dataset is recognized as the best estimate of actual climate variables in Canada, and consequently it is used in a number of climate-related studies (Asong et al., 2015; Eum et al., 2014a; Shook and Pomeroy, 2012; Wong et al., 2017). As large-scale watersheds in Alberta cross the province, e.g., the Peace River and Athabasca River basins, this study evaluated the performance of the historical gridded climate datasets at the AHCCD stations within British Columbia (BC), Alberta (AB), and Saskatchewan (SK) (190 and 129 stations for precipitation and temperature, respectively, in Fig. 1). The AHCCD stations have different record lengths. For example, the longest record period is from 1840 to 2016, while the shortest period is from 1967 to 2004. As the data lengths are different at each AHCCD station, we selected a common period between each AHCCD station and climate dataset to estimate performance measures.
2.2 Historical gridded climate datasets
In general, the available historical gridded climate dataset can be divided into three categories: (1) station-based, (2) multi-source-based, and (3) reanalysis-based. In this study, five high-resolution gridded climate datasets available for Alberta were selected (Table 1) to evaluate their performance and include in the generation of a hybrid climate dataset for Alberta.
Table 1High-resolution gridded historical climate datasets used in this study.
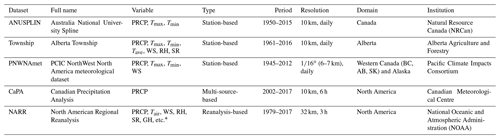
PRCP: precipitation, Tmax: maximum temperature, Tmin: minimum temperature,
Tave: average temperature, Tair: air temperature, WS: wind speed, RH:
relative humidity, SR: solar radiation, GH: geopotential height.
∗ Refer to https://www.esrl.noaa.gov/psd/data/gridded/data.narr.monolevel.html (last access: 13 December 2019) for
details.
2.2.1 Station-based datasets
Hutchinson et al. (2009) produced a Canada-wide daily climate dataset at 10 km resolution from 1961 to 2003 by using the Australia National University trivariate thin-plate smoothing spline technique to model the complex spatial patterns (e.g., large variations in ground elevation and station density over Canada) of daily weather data. Hopkinson et al. (2011) updated the existing ANUSPLIN dataset by reducing residuals and extended the daily weather data from 1950 to 2011. Recently, ANUSPLIN data were extended until 2015 for three climate variables, i.e., daily precipitation and minimum and maximum air temperature, which were interpolated with 7514 surface-based observations (archive data) of Environment Canada. However, the numbers of stations included in interpolation varied year to year, ranging from 2000 to 3000 for precipitation and from 1500 to 3000 for air temperature. The ANUSPLIN data generated by Natural Resource Canada (NRCan) have been used as the source data to compare climate products (Eum et al., 2014a; Wong et al., 2017), evaluate the accuracy of regional climate models (Eum et al., 2012), and model hydrologic regimes (Islam and Déry, 2017; Eum et al., 2017; Dibike et al., 2018).
Similar to the ANUSPLIN dataset, the Pacific Climate Impacts Consortium also generated data for daily precipitation, minimum and maximum air temperature, and wind speed from 1945 to 2012 at 1∕16∘ (6–7 km) resolution using a thin-plate smoothing spline technique over northwestern North America called the PCIC NorthWest North America meteorological (PNWNAmet, Werner et al., 2019) dataset (https://data.pacificclimate.org/portal/gridded_observations/map/, last access: 11 December 2019). While ANUSPLIN utilized a varying number of gauge stations depending on availability of observations in a given year, PNWNAmet set a common period from 1945 to 2012 for all stations included in the interpolation over regularly spaced grid cells within the domain. The PNWNAmet dataset was developed to produce forcing data for an updated version of the Variable Infiltration Capacity model with glaciers (VIC-GL). In addition to precipitation and minimum and maximum temperature, PNWNAmet includes wind speed, which considerably affects vital hydrologic processes, especially evapotranspiration, sublimation, and snow transport (i.e., snow blowing). Because the AHCCD dataset provides only daily precipitation and temperature, wind speed was excluded in this study.
Alberta Agriculture and Forestry (AF) produced the Alberta Township data (http://agriculture.alberta.ca/acis/township-data-viewer.jsp, last access: 11 December 2019) from 1961 to 2016 at approximately 10 km (Alberta Township grid) resolution using a hybrid inverse distance weighting (IDW) process (Shen et al., 2001) for daily precipitation, minimum and maximum temperature, relative humidity, wind speed, and solar radiation. The archive (raw) station data collected by ECCC, Alberta Environment and Parks (AEP), and AF over Alberta were used in producing the Township dataset. The Township data used various effective radiuses (60 to 200 km) to ensure a sufficient number of gauge stations in the IDW process. When there is no station within 200 km, it is assumed that the nearest station represents the climate conditions of the Township center. The Township data domain covers most of Alberta except the mountainous regions, while both ANUSPLIN and PNWNAmet cover all of western Canada (refer to Table 1). Therefore, one of the limitations of the Township dataset is its application to a large watershed spanning Alberta and other neighbouring provinces.
2.2.2 Multi-source-based dataset
As an operational system, the Meteorological Service of Canada initiated the Canadian Precipitation Analysis (CaPA) in 2003 to produce superior gridded precipitation data over North America at 10 km resolution (Lespinas et al., 2015), especially for regions with poor observational networks (Mahfouf et al., 2007). CaPA employs an optimum interpolation technique that requires properties of error statistics among observations and a first guess, i.e., the background field (Garand and Grassotti, 1995). A short-term forecast of 6 h accumulated precipitation from the Canadian Meteorological Centre (CMC) regional Global Environmental Multiscale (GEM) model (Côté et al., 1998a, b) is used in CaPA as the background field. The assimilated precipitation from the Canadian weather radar network, and 33 US radars near the border are used as additional observations to generate analysis error among multiple sources of observations and the background precipitation. Zhao (2013) tested the applicability of CaPA for hydrologic modelling in the Canadian Prairies and proved its usefulness in data-sparse regions and the winter season. In addition, CaPA has been widely used in agricultural and hydrologic applications (Deacu et al., 2012; NIDIS, 2015). Eum et al. (2014a) further addressed some of the limitations of CaPA, i.e., the lack of air temperature which is one of the primary drivers in hydrologic modelling and shorter data length (only from 2002 to 2017), for model calibration and validation. Using 6 h accumulated precipitation CaPA products, in this study, daily accumulated precipitation was generated over western Canada by adjusting the time zone from coordinated universal time (UTC) to mountain time (MT).
2.2.3 Reanalysis-based dataset
Reanalysis products are another common type of gridded dataset used in climate and hydrologic studies. The WATer and global CHange (WATCH) Forcing Data methodology applied to the ERA-Interim (WFDEI) dataset provides reanalysis data from 1979 to 2016 globally at 0.5∘ ( ∼50 km), which are bias-corrected by the Climatic Research Unit (CRU) and the Global Precipitation Climatology Centre (GPCC) monthly precipitation data (Weedon et al., 2014). Another representative reanalysis dataset in the North America is the North American Regional Reanalysis (NARR) that has been developed to create a long-term set of dynamically consistent 3-hourly climate data from 1979 to 2003 at a regional scale ( ∼ 32 km) for the North American domain (Mesinger et al., 2006). By utilizing advanced land surface modelling and data assimilation through the Eta Data Assimilation System (EDAS), NARR improved the National Centers for Environmental Prediction/National Center for Atmospheric Research (NCEP/NCAR) global reanalysis data. NARR cycled every 3 h to produce a climate dataset from 1979 to present. Choi et al. (2009) tested the applicability of NARR for hydrologic modelling in Manitoba for a region with poor monitoring network density. However, the NARR dataset after 2004 is not consistent with that of prior years (i.e., 1979 to 2003) because assimilation of precipitation observations was discontinued in 2003 (Eum et al., 2014a). Wong et al. (2017) found that WFDEI performed better than NARR over Canada. However, their study focused on only precipitation at the Canada-wide scale. In addition, WFDEI is not an operational system but is updated when GPCC and CRU are available for the bias correction of monthly values. Furthermore, WFDEI provides rain and snow separately, which requires another process to obtain total precipitation. On the contrary, the NARR data provide total precipitation rate and are available from 1979 to the current with a delay of half a month as an operating system. In other words, NARR is operationally updated every half month. Therefore, this study selected NARR to provide a more recent climate dataset through the REFRES. Using the 3 h NARR climate data, daily precipitation and minimum and maximum temperature were calculated by adjusting the time zone to MT from the original NARR dataset (UTC zone).
3.1 REFerence Reliability Evaluation System (REFRES)
This study suggests a REFerence Reliability Evaluation System that consists of three main modules (refer to Fig. 2): (1) a performance measure module (PMM) to evaluate various performance measures for each climate dataset, (2) a ranking module (RM) to identify the most reliable climate data for a target grid cell using a multi-criteria decision-making technique based on the performance measures provided by PMM, and (3) a data generation module (DGM) to produce a hybrid climate dataset by selecting the most reliable climate dataset based on the ranking provided by the RM. These three modules are seamlessly integrated and exchange the required data and information to generate a hybrid climate dataset. The next section provides further details on each module.
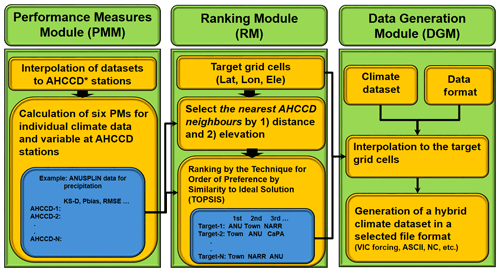
Figure 2Structure of REFRES comprised of three modules: (1) performance measure module (PMM), (2) ranking module (RM), and (3) data generation module (DGM). Lat: latitude; Lon: longitude; Ele: elevation. ANU: ANUSPLIN; Town: Alberta Township. KS-D: Kolmogorov-Smirnov D statistic; NC: NetCDF.
3.1.1 Performance measure module (PMM)
AHCCD is a point (station) dataset, while the other climate datasets used in this study (refer to Table 1) are regularly spaced gridded datasets with varying time periods, spatial resolution, and coverage (i.e., domain). Therefore, the inverse distance-squared weighting method was applied to obtain the values at the AHCCD stations from all the gridded climate datasets. Then, performance measures were calculated by comparing the interpolated values with the data collected at AHCCD stations. The choice of the performance measures is vital in REFRES, as the ranking of climate datasets entirely depends on included performance measures. In this study, performance measures were selected based on three criteria: (1) distribution, (2) sequencing, and (3) spatial pattern. Distribution-related performance is assessed by the Kolmogorov–Smirnov D statistic (DKS) and standard deviation ratio (σratio). Sequence-related performance is assessed by the percentage of bias (Pbias), root mean square error (RMSE), and temporal correlation coefficient (TCC). Spatial-pattern-related performance is evaluated by the pattern correlation coefficient (PCC) as shown in Eqs. (1) to (5). The equations of TCC and PCC are identical, but TCC is calculated with the daily time series of climate variables, and PCC is obtained by the mean annual precipitation and temperature of the AHCCD stations over a target domain. Therefore, PCC varies with the user-specified target domain.
where σG and σO are the standard deviation of gridded and observed climate datasets; Gi and Oi represent gridded and observed climate datasets at an ith time step, respectively; F is the empirical distribution function of a climate dataset; σ is standard deviation; and represent the mean of gridded and observed climate datasets, respectively; and N is a total number of data points. These six performance measures were calculated for all the selected climate datasets and variables at each AHCCD station. Figure 2 (blue box in the PMM) shows an example of six PMs (performance measures) calculated for the precipitation variable using the ANUSPLIN gridded data. Thus, 15 tables (five climate datasets with three variables) were generated by the PMM and transferred to the RM.
3.1.2 Ranking module (RM)
The function of the ranking module is to select the appropriate AHCCD stations for a given target grid cell and to rank all the gridded datasets based on the six performance measures calculated in the previous module. For a given target cell, AHCCD stations are selected based on two criteria: distance and elevation. Firstly, 20 % of all AHCCD stations are selected based on the nearest-distance criteria, which are then again reduced by the five nearest stations based on the minimum-elevation-difference criteria. Then the performance measures are averaged over the selected AHCCD stations to represent the skill of each climate dataset for the given target grid cell.
As multiple performance measures are employed in this study, there are situations when a climate dataset may perform well for some measures but not for others. Therefore, a multi-criteria decision-making (MCDM) technique is required to systematically rank all of the climate datasets while considering multiple performance measures. This study applied a multi-criteria decision-making technique called the Technique for Order of Preference by Similarity to Ideal Solution (TOPSIS; Hwang and Yoon, 1981) to systematically determine the order of preference for all climate datasets at each target grid cell. TOPSIS calculates the geometric distance between alternatives and an ideal solution defined by the best performance on each criterion from the alternatives, and it then determines the best and worst alternatives based on the distance. TOPSIS has been successfully applied to watershed management for multi-criteria problems (Jun et al., 2013; Lee et al., 2013). TOPSIS starts with the averaged performance measures, (xij)m×n for the ith alternative (climate dataset in this study) and jth criterion (i.e., a performance measure). A weighted normalized decision matrix, (tij)m×n, is given by
where m and n are the total number of alternatives and criteria, respectively, nij is the matrix normalized by Eq. (7), and wj represents weighting on the jth criterion. Under the assumption that all performance measures are important, this study used an equal weighting. Then, Euclidean distances (dib and diw) of climate datasets from the best (Ab) and worst (Aw) conditions were calculated respectively by Eqs. (8) to (11).
where tbj and twj are the best and worst decision matrices determined by Eqs. (8) and (9), respectively, and J+ and J− represent criteria that have a positive and a negative impact on performance. For example, TCC and PCC are in J+, while DKS , σratio, Pbias, and RMSE are in J−. Using the Euclidean distances, the order of preference for all climate datasets was determined by the similarity (Siw) to the worst condition in Eq. (12).
siw=1 when the alternative is equal to the best condition (Ab), and siw=0 if the alternative is equal to the worst condition (Aw). In other words, a higher siw represents higher preference among alternatives. As we evaluate the performance measures (criteria) for individual climate variables, TOPSIS can be applied to decide the preference of climate datasets considering the performance measures for either individual or multiple variables. In this study, TOPSIS provides two types of ranking information by using performance measures from (i) individual climate variables and (ii) all climate variables. That is, one is the ranking for precipitation and temperature separately (Rind), and the other is the ranking for multiple variables (Rmul). For example, in this study, Rind was determined by a 5×6 decision matrix (five climate datasets and six performance measures) for precipitation and temperature individually, while Rmul was determined by a 4×18 decision matrix (four climate datasets excluding CaPA that provides only precipitation and 18 performance measures from three variables). To alleviate the erroneous output that minimum temperature is higher than maximum temperature on a certain day when producing the hybrid climate dataset by the ranking of temperature values individually, the performance measures of both minimum and maximum temperature are employed together to rank the climate datasets for temperature.
3.1.3 Data generation module (DGM)
The DGM extracts the most reliable climate data for a user-specified target region based on the ranking information obtained from the RM. The tool is flexible enough to provide output in various common formats, i.e., NetCDF (Network Common Data Form), ASCII (American Standard Code for Information Interchange; text), or in the specific format of a numerical model. As all of the historical gridded climate datasets have been tested and employed in numerous climatic and hydrologic studies, an assumption was made in generating the hybrid climate dataset that all of the climate datasets are equally qualified for inclusion, but the final selection can be determined by the proven superiority evaluated through the performance measures. Under this assumption, the available datasets can be combined systematically based on the rank (performance) of each dataset at target grid cells. As each climate dataset has different data periods shown in Table 1, the first-ranked dataset cannot fully cover a whole target period to be extracted from a set of climate data candidates. The DGM provides a systematic procedure to identify the most reliable dataset for a target region and extracts the data from the inventory of climate datasets considering the ranking and availability of each dataset for a desired period. For instance, if CaPA and ANUSPLIN ranked first and second for precipitation and the desired period is 1950 to 2016, the DGM would start searching for the availability of precipitation in 1950. As CaPA is only available from 2002 to 2016, the DGM reorders the rank to select ANUSPLIN as the best climate dataset available in 1950. In this way, a hybrid dataset over the period 1950 to 2016 is generated by extracting from ANUSPLIN from 1950 to 2001 and CaPA from 2002 to 2016 in this particular case. Once the best climate datasets are extracted over all the target grid cells (study domain), the hybrid climate dataset is produced in a user-defined format. This study generated the hybrid climate datasets in the form of the VIC forcing input format to be directly employed into the hydrologic model.
3.2 Proxy validation
Although the AHCCD dataset has been adjusted to provide better estimates of actual precipitation and temperature, it contains statistical artifacts that include inevitable errors from sequential data processes that can be propagated in the derived hybrid climate dataset. Given that the AHCCD stations, the reference dataset for the performance measures, are not regularly distributed and have an especially poor density in the northern parts of the study area (refer to Fig. 1), it is questionable if the hybrid climate dataset can represent a historical climate better than the individual gridded climate dataset. Utilizing a proxy validation approach (Klyszejko, 2007), this study applied streamflow records to validate the utility of the derived hybrid climate dataset over other existing climate datasets in hydrologic simulations. In this study, the proxy validation was conducted using an existing hydrologic model (Eum et al., 2017), Variable Infiltration Capacity (Liang et al., 1994), for the Athabasca River basin. The VIC model was further refined at 1∕32∘ (2–3 km) for a finer spatial resolution and to better simulate the complex river network in the Lower Athabasca River basin. Five of the catchment areas listed in Table 2 were selected for the proxy validation based on three criteria: (i) hydrometric record length, (ii) location defined by upper, middle, and lower reaches (refer to http://www.ramp-alberta.org/river/geography/basin+landscape.aspx, last access: 11 December 2019), and (iii) the number of gridded climate datasets used to generate a hybrid climate dataset for the catchment area of the selected hydrometric station. In other words, a higher number of gridded climate datasets contributing to the hybrid climate dataset within a catchment was selected to evaluate the utility of the hybrid climate data relative to the existing gridded climate datasets. Figure 3 shows the geographical information on the selected five sub-basins. Hinton is located near the headwaters of the ARB, which are characterized by mountainous topography and snowmelt- and glacier-ice-melt-dominated hydrologic regimes. Pembina is one of the major rivers in the middle reach. The other three stations (Christina, Clearwater above Christina, and Firebag) are located in the lower reach, which is a water-limited (dry) region due to a higher amount of evapotranspiration (Eum et al., 2014b). The sub-basins of Hinton, Firebag, and Clearwater include a partial area outside of the Township data domain, thus inducing a higher or lower number of climate datasets in the derived hybrid dataset.
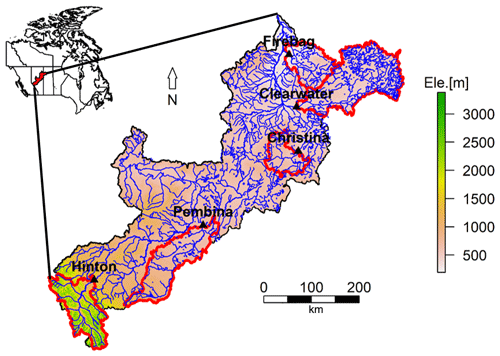
Figure 3Geographical information on the five sub-basins (red line) selected in the Athabasca River basin for the proxy validation.
A total of seven climate datasets (five individual and two hybrid climate datasets from Rind and Rmul) are available to calibrate the VIC hydrologic model parameter set related to soil properties and routing. The calibration period is 1985–1997 as in Eum et al. (2017), except for CaPA that uses the period of 2003–2009 for calibration, as CaPA covers the period from 2002 to 2016. The remaining period of total record length for each climate dataset is used for validation. More details on calibration can be found in Eum et al. (2017). Under the assumption of REFRES that all of the existing climate datasets are of equal quality for hydrologic simulations, all of the calibrated parameter sets can be considered as mostly plausible parameter sets for the selected sub-basins. However, as mentioned above, intrinsic biases exist temporally and spatially in all of the gridded climate datasets, e.g., discrepancies in the amount and spatial distribution of precipitation between the gridded climate datasets and observations. Therefore, the similarity of the gridded climate datasets in terms of magnitude, sequence, and spatial distribution of climate events relative to observations is crucial to reproduce historically observed streamflows. In addition to climate forcings, streamflows are mainly affected by geographic characteristics and physical land surface processes (e.g., infiltration and evapotranspiration), which are represented by model parametrization related to infiltration and soil properties (Demaria et al., 2007). In a hydrologic simulation, the biases in climate datasets can be compromised by model parameters that adjust hydrologic processes to observations (Harpold et al., 2017; Kirchner, 2006). That is, a calibrated parameter set may imply biases in a climate dataset. Under the assumption that the calibrated parameter sets are suitable for hydrologic simulations in each sub-basin, this study applied a multiset-parameter hydrologic-simulation approach that employs all parameter sets calibrated by the seven climate datasets and the same climate dataset as forcing input data to assess the sensitivity of the climate dataset to all feasible parameter sets. From the multiset-parameter hydrologic simulations, the bias in a climate dataset can be estimated indirectly by quantifying the variability in hydrologic simulations derived from the feasible calibrated parameter sets under a climate forcing dataset. In other words, lower variability in the hydrologic simulations indicates higher reliability in the climate forcing dataset. The suitability of the hybrid climate dataset for improving historical hydrologic simulations was also tested by directly comparing the performances of calibration and validation for each climate dataset. Proxy validations were carried out by conducting 49 hydrologic simulations (seven climate forcing and seven parameter sets) for the Pembina and Christina catchment areas, whereas only 36 simulation runs were possible for the Hinton, Firebag, and Clearwater sub-basins, as one of the gridded datasets (i.e., Township) did not cover the entire catchment areas of these three hydrometric stations.
4.1 Precipitation performance measures in Alberta
Although the performance measures were calculated for 190 AHCCD stations in western Canada, the target area of this study is in Alberta, where only 45 stations are located. Therefore, the results for the 45 AHCCD stations are given in this study. Table 3 shows spatially averaged performance measures for precipitation. The Township data outperformed other climate datasets for all performance measures except Pbias. ANUSPLIN is the second-best climate dataset for Alberta. All climate datasets underestimate the standard deviation of observed daily precipitation (i.e., negative σratio), especially PNWNAmet and CaPA, which underestimated it by 34 % and 39 %, respectively. Interestingly, two station-based gridded climate datasets, ANUSPLIN and Township, show negative Pbias, while PNWNAmet, CaPA, and NARR datasets have positive Pbias. This indicates that ANUSPLIN and Township may underestimate extreme precipitation, as they employed the raw station data instead of the adjusted precipitation data which are higher than the raw station data by 5 %–20 %. In contrast, other climate datasets (especially multiple sources and reanalysis data) overestimate extreme precipitation. These results are consistent with findings in Eum et al. (2014a) that CaPA and NARR overestimate extreme precipitation events by overly reflecting the orographic effects on precipitation in western Alberta.
Figure 4 shows the temporal correlation coefficient data averaged over the AHCCD stations in Alberta in order to investigate the similarity between historical precipitation datasets employed in this study. As expected, station-based climate datasets (i.e., ANUSPLIN, PNWNAmet, and Township) showed better TCCs than CaPA and NARR. The TCC between ANUSPLIN and Township was the highest among climate datasets except for the observations (i.e., OBS), even though they incorporated different interpolation techniques. PNWNAmet showed the highest TCC with ANUSPLIN because they both are based on thin-plate spline interpolation. TCCs between CaPA and other climate datasets are similar, as CaPA is produced from multiple sources such as GEM's outputs and weather radar networks in Canada and the US. NARR, the reanalysis-based climate dataset, showed a higher TCC value with CaPA than with other datasets, as it is assimilated with multiple sources of observations.
Maps of each performance measure are shown in Fig. 5. It is evident from the spatial variability that the ANUSPLIN and Township datasets outperformed the other datasets in DKS throughout Alberta. In the mountainous region of southwest Alberta, most of the climate datasets performed poorly in Pbias, σratio, RMSE, and PCC, resulting mainly from the sparse observation network and inconsistent observations near the Canada–US border. PNWNAmet highly overestimates the mean annual precipitation in the mountainous area (e.g., 300 mm yr−1 higher than that observed at station ID 3050519), which may considerably affect simulated streamflows originating in mountainous headwaters and further downstream.
4.2 Air temperature performance measures in Alberta
The performance measures for air temperature averaged over 37 AHCCD stations in Alberta are presented in Table 4. As CaPA provides only precipitation, it was excluded in the assessment for temperature. All of the performance measures for temperature are better than those for precipitation except Pbias. NARR is highly biased as it underestimates minimum and maximum temperatures, which might be an attribute of the discontinuation of the observation assimilation since 2003 (Eum et al., 2014a). ANUSPLIN and Township showed an almost perfect linear relationship (TCC) with the observations (i.e., >0.97 for all of the climate datasets). The performance measures for maximum temperature are better than those for minimum temperature, as maximum temperature is dominated by mainly large-scale heat waves, while minimum temperature is affected by local physical processes, e.g., topography and surface conditions (Eum et al., 2012). NARR showed less skill in capturing these local effects due to the coarse spatial resolution (∼32 km) compared to other station-based climate datasets. As with precipitation, the maps of performance measures for minimum and maximum temperature presented in Figs. 6 and 7 showed that data from the mountainous areas performed poorly in most of the performance measures. NARR showed positive and negative Pbias for minimum and maximum temperature, respectively, in the mountainous region, indicating that NARR has a warm bias in extreme cold temperatures and a cold bias in extreme warm temperatures.
Table 4Performance measures averaged over the AHCCD stations in Alberta for minimum and maximum temperature.
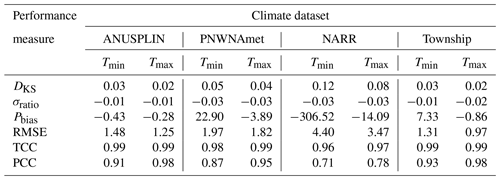
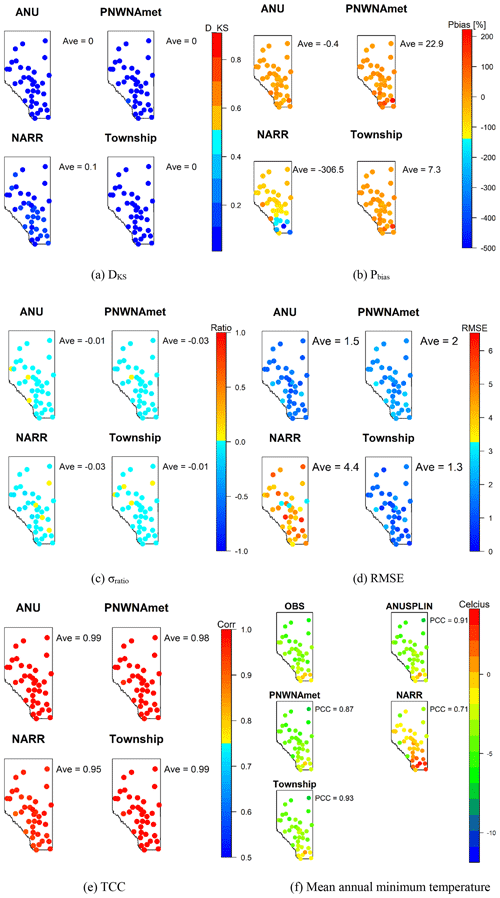
Figure 6Maps of performance measures for minimum temperature over the AHCCD stations in Alberta. (a) DKS, (b) Pbias, (c) σratio, (d) RMSE, (e) TCC, (f) mean annual minimum temperature.
4.3 Ranking of climate datasets in the ARB
The geospatial information (i.e., latitude, longitude, and elevation) of 22 372 grid cells within the ARB was extracted from the Canadian digital elevation data provided by Natural Resources Canada (refer to https://open.canada.ca/data/dataset/7f245e4d-76c2-4caa-951a-45d1d2051333, last access: 11 December 2019). Using this information, the RM in REFRES ranked the five climate datasets by TOPSIS for each grid cell. Table 5 presents the first-ranked number of grid cells and their percentage for each climate dataset according to the performance measures of individual variables (Case A and Case B) and multi-variables (Case C), i.e., precipitation and (minimum and maximum) temperature in this study.
Table 5First-ranked number of grid cells in the five sub-basins and the whole Athabasca River basin (ARB) and their percentages for each climate dataset, considering the performance measures of the cases with individual (Case A and B) and multi-variables (Case C, i.e., precipitation and temperature in this study). Total number of grid cells is 22 372 at 1∕32∘ (2–3 km).
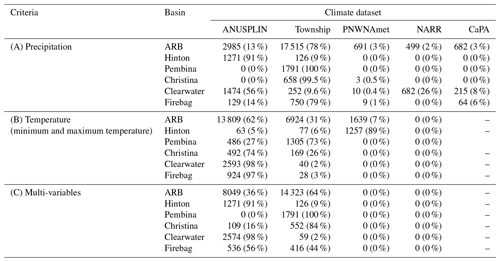
For precipitation, the Alberta Township dataset was ranked first in most of the grid cells within the basin (78 %) for the whole ARB, followed by ANUSPLIN (13 %), PNWNAmet (3 %), CaPA (3 %), and NARR (2 %). However, the Township data domain covers only 83 % of the ARB within Alberta; the remaining 17 % of the watershed area that lies outside the province is not covered (Fig. 8). The Township dataset was ranked first for almost 95 % of grid cells within its domain, indicating that the Township dataset overwhelmingly outperformed other climate datasets for precipitation. Township was dominantly ranked first for the sub-basins (Pembina and Christina) within the Township domain.
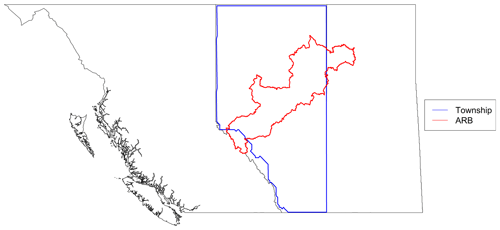
Figure 8Domain of the Township dataset (blue line) and the boundary of the Athabasca River basin (red line).
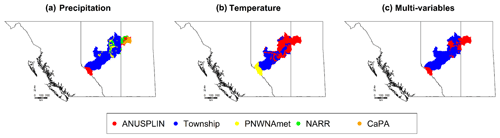
Figure 9Maps of the first-ranked climate datasets in the ARB for the individual variable (a, b) and multi-variables (c).
For temperature, ANUSPLIN was ranked first (in 62 % grid cells) for the whole ARB, followed by Township (31 %) and PNWNAmet (7 %). In the upper and middle reaches, i.e., Hinton and Pembina, PNWNAmet and Township were mostly ranked first, respectively, while ANUSPLIN outperformed other climate datasets for the sub-basins in the lower reach. When considering the performance measures for multiple variables simultaneously, the Township dataset was ranked first, followed by ANUSPLIN for 64 % and 36 % of the grid cells for the whole ARB. Figure 9 shows maps of the first-ranked climate datasets for each case in Table 5, i.e., cases with individual (Case A and B) and multi-variables (Case C). Due to the limited spatial coverage of the Township dataset, other climate datasets were ranked first in the headwaters of the ARB and the area of the river basin in Saskatchewan. For instance, ANUSPLIN and PNWNAmet were ranked first in the headwaters, while no specific climate dataset dominated in Saskatchewan for precipitation (refer to Fig. 9a). For temperature, ANUSPLIN outperformed in the northern part (middle and lower reaches of the ARB) due to the outstanding performance of the Pbias performance measure for minimum temperature as shown in Table 4 and Fig. 6b. For multi-variables, Township was mostly ranked first within its domain, and ANUSPLIN was ranked first outside the Township dataset domain and also for a small part of the lower reach area in the ARB.
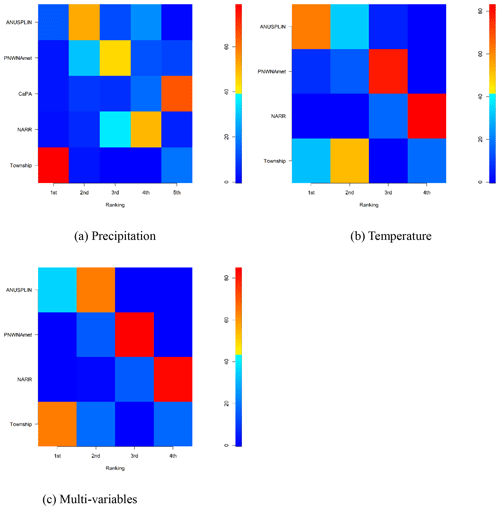
Figure 10Percentage of climate datasets on each rank for Rind and Rmul. (a) Precipitation, (b) temperature, (c) multi-variables.
Figure 10 shows the percentage of each climate dataset at each rank for the three cases (e.g., A, B, and C in Table 5). For precipitation (Case A), Township overwhelmed other climate datasets. The second alternative was ANUSPLIN in the majority of grid cells in the ARB. PNWNAmet, NARR, and CaPA were mostly ranked third, fourth, and fifth, respectively. For temperature (Case B), ANUSPLIN was ranked mostly first, and Township was a distinct second choice in the majority of grid cells, followed by PNWNAmet and NARR. For multi-variables (Case C), Township and ANUSPLIN were the first and second choices in the majority of grid cells in the ARB, respectively.
As two different hybrid climate datasets were generated using the ranking information from single- and multi-variable approaches, i.e., Hybrid(Rind) and Hybrid(Rmul), further investigation is required to identify which hybrid climate dataset may provide better performance and consequently will be recommended for future climate-related studies. A proxy validation approach was applied using both generated hybrid climate datasets to validate the utility of one dataset over the other.
4.4 Proxy validation of generated hybrid climate datasets
In addition to the five gridded climate datasets, the two hybrid climate datasets were implemented for proxy validation using the VIC model. In contrast to the station-based climate datasets, both CaPA and NARR were produced from climate models and multiple sources of observations, consequently showing a higher correlation with each other as shown in Fig. 4. Since CaPA also provides only precipitation, this study combined precipitation of CaPA with the NARR temperature to prepare the CaPA climate forcing dataset for the proxy validation. Table 6 presents the Nash–Sutcliffe efficiency (NSE) for the calibration and validation periods at the selected hydrometric stations (Hinton, Pembina, Christina, Clearwater, and Firebag) in the ARB to assess the suitability of each climate dataset as climate forcing input data for hydrologic simulations. Over the five hydrometric stations, most of the climate datasets performed well with the exception of NARR in the Pembina catchment. Most of the NSE values in calibration for Christina and Firebag were above 0.50, which was considered as a threshold of satisfactory performance in hydrologic models as suggested by Moriasi et al. (2007). However, model performance is not satisfactory for Christina and Firebag during the validation period. Such an underperformance at the lower reach of the Athabasca River basin may be attributed to (1) relatively poor forcing datasets within the drainage area of each hydrometric station, caused by the lack of observational stations in the northern part of Alberta (refer to Fig. 1) and (2) anthropogenic activities that were not reflected in the VIC model simulations especially during the validation period when land cover changes and water withdrawals mainly induced by oil-sand development have occurred. Table 7 shows the NSE values of hydrologic models applied for the Athabasca River basin in the literature. All of the NSE values were obtained from the simulations for calibration and validation periods. The NSE values of the current study were obtained from the VIC simulation forced by Hybrid(Rind) for comparison to the literature. It needs to be noted that the VIC model was calibrated for the entire ARB watershed to simulate historical flow over the ARB. The results of the VIC simulation for the entire Athabasca River basin were included in the Discussion section. The VIC model's performance in this study was better than or comparable to the literature for all stations in the ARB. In particular, this study considerably improved the performance of streamflow simulation for the Firebag catchment. Comparing to the NSE values presented in Table 6, in addition, the NSE values of all cases for Firebag and Christina were better than (or comparable to) those of the literature. Overall, the quality of hydrologic simulations in this study was considerably improved (or comparable) compared to the results of the literature. Consequently, the VIC model performance is acceptable at all of the hydrometric stations for the proxy validation. The two hybrid climate datasets performed well, with comparably good and better NSE values than other climate datasets, especially at Pembina, Clearwater, and Firebag, located in the middle and lower reaches.
Table 6Nash–Sutcliffe efficiency (NSE) for the calibration (“Cal.”) and validation (“Val.”) periods at five sub-basins in the ARB for the climate datasets investigated in this study.
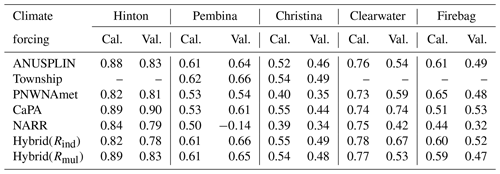
Table 7NSE values between the current study and literature for the Athabasca River basin. The NSE values were obtained for calibration and validation periods. For comparison of the current study to the literature, the NSE values of the current study were obtained from the VIC simulation for the hybrid climate dataset (Rind).
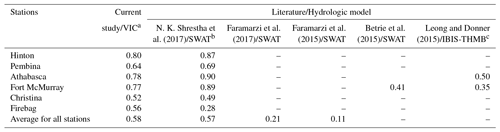
a Variable Infiltration Capacity. b Soil and Water Assessment Tool (SWAT). c Integrated BIosphere Simulator – Terrestrial Hydrology Model with Biogeochemistry (IBIS-THMB).
Figure 11 presents the boxplots of NSEs obtained through the multiset-parameter VIC simulations. The NSE ranges were obtained from multiple VIC simulations, with each climate dataset used as climate forcing for all the plausible model parameter sets, which were calibrated with seven climate datasets, individually. The values above each boxplot represent the averaged value of the NSEs over the multiset-parameter hydrologic simulations. A narrower range of NSE values represents a higher precision for a climate dataset, and a higher averaged NSE value means higher accuracy. Therefore, a climate dataset showing both a higher averaged NSE and a narrow range of NSEs indicates that it is a relatively more appropriate and reliable climate forcing dataset for hydrologic simulations.
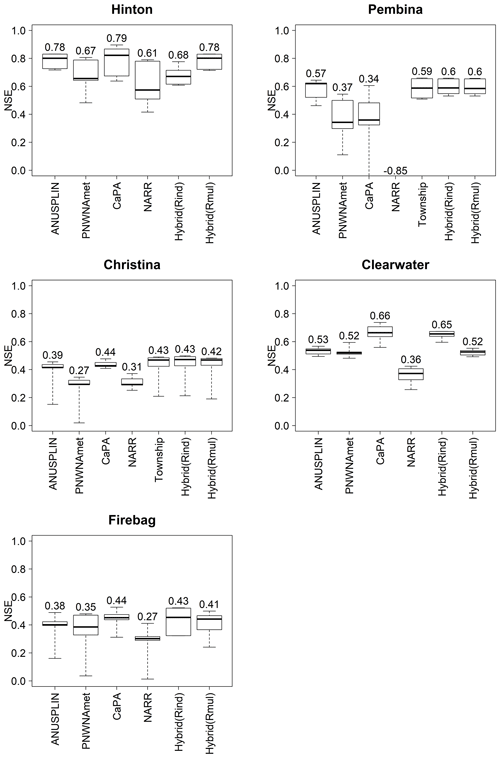
Figure 11Boxplots of the NSEs of the proxy validation at the five sub-basins in the ARB. The values above each boxplot represent the average over NSEs of the proxy validation.
At Hinton, all of the climate datasets showed satisfactory NSE values for accuracy, while ANUSPLIN, Hybrid(Rind), and Hybrid(Rmul) showed better precision. The validation period of CaPA is only 6 years from 2010 to 2016, as CaPA data are only available between 2002 and 2016. This might be a reason why CaPA produced the highest NSE (accuracy) among the climate datasets used in this study. Therefore, the results of CaPA need to be considered carefully; otherwise they might be misleading. In this context, the CaPA dataset was excluded from further assessment of the precision and accuracy even though all of the results of CaPA were included in Fig. 11 for reference only. Hybrid(Rmul) and ANUSPLIN showed the highest accuracy as forcing data, followed by Hybrid(Rind), PNWNAmet, and NARR. In the Pembina and Christina catchments, the Hybrid(Rind), Hybrid(Rmul), and Township datasets had the highest precision and accuracy. NARR produced negative NSEs at Pembina, indicating it is not reliable or suitable as a forcing dataset. For Clearwater, Hybrid(Rind) is the top performer, followed by Hybrid(Rmul), ANUSPLIN, PNWNAmet, and NARR. Clearwater had the highest number of climate datasets combined in the hybrid climate dataset within the basin for precipitation as shown in Fig. 9. Interestingly, the precision of NARR is similar to that of CaPA because they shared the temperature data from NARR. For Firebag, Hybrid(Rind) also showed top performance in both precision and accuracy, followed by Hybrid(Rmul), ANUSPLIN, PNWNAmet, and NARR. Overall, Hybrid(Rind) showed the best accuracy and precision at all hydrometric stations, indicating that it has the potential not only to improve historical hydrologic simulations but also to be used as reference data for statistical downscaling of climate change projections in the province.
Among the station-based gridded climate datasets, the Township dataset outperformed other station-based gridded climate datasets. As PNWNAmet set a common period from 1945 to 2012 for all stations included in the interpolation, many stations might be left out in the data generation processes. While ANUSPLIN used the Canada-wide archive (raw) station data collected only by ECCC, the Alberta Township data have been produced on the basis of the archive (raw) station data collected by ECCC, AEP, and AF over Alberta. Therefore, one of the possible reasons for the Township dataset outperforming the others might be the difference in the numbers of stations (i.e., station density) employed to produce the gridded climate datasets. In addition, PNWNAmet showed a positive Pbias for precipitation, especially in the mountainous areas, while ANUSPLIN, which employs similar thin-plate spline interpolation, generated negative Pbias. PNWNAmet overestimated precipitation over the mountainous area, which considerably affects simulated low flows at Hinton in the ARB. Figure 12 shows the observed and simulated hydrographs from gridded climate datasets at (a) Hinton and (b) Pembina. It clearly shows that PNWNAmet highly overestimated the low and high, which is caused by overestimated precipitation in the drainage area of the sub-basins. As with PNWNAmet, NARR also overestimated the low and high flows, which is induced by the combined effects of overestimating precipitation and warm biases in cold temperatures. The temperature bias of NARR is thus further confirmed and is consistent with the earlier finding of Eum et al. (2014a) and Islam and Déry (2017).
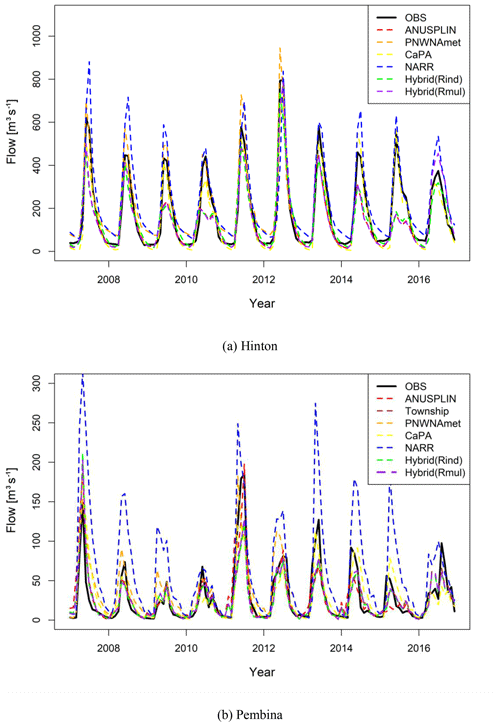
Figure 12Monthly observed and simulated hydrographs from the gridded climate datasets at (a) Hinton and (b) Pembina.
Table 8Comparison of NSE values for hydrologic simulations forced by ANUSPLIN and the hybrid climate datasets at the main stream of the ARB.
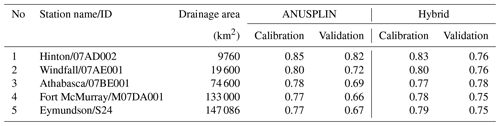
In Fig. 12, the hybrid climate datasets underestimated the peak flows (in 2009, 2010, 2014, and 2015) at Hinton, and the hydrograph is similar to the hydrograph produced by the ANUSPLIN dataset that dominantly ranked first in this watershed. On the contrary, the hydrograph of the hybrid climate datasets at Pembina is similar to that of Township, which is dominantly ranked first in Pembina (refer to Table 5). These results indicate that the hybrid climate dataset has the intrinsic limitation that the performance of the hybrid dataset for a basin may closely resemble that of the climate dataset that is dominantly ranked first for the basin. However, the utility of the hybrid climate dataset can be clearly found at a whole-basin scale for a large watershed, as the added values of the hybrid climate dataset in sub-basins can be cumulated to the main stem downstream in the watershed. To further validate the utility of the hybrid climate dataset, the VIC model was calibrated for the entire ARB to produce a long-term historical hydrologic simulation for the ARB. Table 8 presents the NSE values of hydrologic simulations forced by ANUSPLIN and Hybrid(Rind) at the hydrometric stations in the main stream of the ARB. The result shows that as the size of the watershed increases, the hybrid climate dataset starts performing better than ANUSPLIN used in Eum et al. (2017). In other words, the hybrid climate dataset improved the historical hydrologic simulation for the ARB. This is mainly due to the fact that as the watershed area increases, the derived hybrid climate dataset is no longer dominated by a single gridded climate dataset.
Among the station-based gridded climate datasets, ANUSPLIN and Township employed a different number of stations depending on their periods of record. Therefore, there is an inconsistency in these climate datasets over time. For example, the Township dataset employed only 300–400 stations in the 1960s, but that has increased to 400–500 since 1970. A change-point analysis of these datasets may provide some useful information to end users with respect to when and where changes occurred, which will help in establishing spatial and temporal accuracies of these datasets (Eum et al., 2014a). Further, PNWNAmet employed the same number of stations over time to avoid the abovementioned inconsistency, but this study found that it induced the overestimation of precipitation in data-poor regions such as mountainous regions in Alberta. As the hybrid climate datasets are generated from the multiple historical gridded datasets, they may also have the same inconsistencies identified in other datasets. The proxy validation, however, demonstrated that the generated hybrid climate datasets can improve the performance of hydrologic simulations.
This study identified the preference order of all gridded climate datasets based on the performance measures evaluated at the AHCCD stations, therefore the ranking somewhat relies on the spatial distribution of the AHCCD stations. As shown in Fig. 1, the density of AHCCD stations varies across western Canada, and it is low in the cold climates of mountainous and northern areas. Therefore, the ranking could further be improved with a more uniform density of AHCCD stations over western Canada.
The literature has demonstrated that NARR, a reanalysis-based climate dataset, can be an alternative as a climate forcing dataset for hydrologic simulations in data-sparse regions (Choi et al., 2009; Praskievicz and Bartlein, 2014; Islam and Déry, 2017). In this study, the NARR dataset performed quite well in high-elevation regions (Hinton in this study), while it did not perform so well in the middle and lower reaches, i.e., lower-elevation watersheds. NARR performed especially poorly in the Pembina sub-basin, a region where hydrologic simulations are highly sensitive to model parameters (Eum et al., 2014b). In Fig. 11b, however, the NARR parameter set produced fair NSE values in hydrologic simulations forced by the other climate datasets except for CaPA and PNWNAmet. Such result indicates that (1) all of the parameter sets used in this study were calibrated reasonably and (2) climate forcing input data play a more crucial role in hydrologic simulations, as no parameter sets produced a fair NSE value from NARR in Pembina. CaPA was more suitable than NARR for the selected sub-basins in this study, which indicates that CaPA might be a better alternative in low station-density regions such as the ARB. However, since the validation period in this study is only 7 years from 2010 to 2016, a longer data period is necessary to validate the suitability of CaPA as indicated in Eum et al. (2014a) and Wong et al. (2017).
In the proxy validation, Hybrid(Rind) performed well in the Clearwater sub-basin, where the highest number of climate datasets were combined in the generated hybrid climate datasets. The Township dataset, which mostly ranked first within its spatial domain, partially covers the drainage area of Clearwater so that the generated hybrid climate dataset, Hybrid(Rind), is composed of many climate datasets in this sub-basin. In a traditional approach to hydrological modelling for Clearwater, either the Township dataset might be completely excluded (as it does not cover the entire Clearwater watershed) or potentially combined with other gridded climate datasets to cover the entire watershed. However, combining different climate datasets to construct the climate forcing for a larger region requires an evaluation of the datasets to identify the order of preference for such aggregation when multiple choices are available. Therefore, this study suggested the REFRES methodology to systematically compare all available climate datasets for a region to produce a hybrid climate dataset that covers a desired period of the record and spatial domain by considering the order of preference for combining various climate datasets at each grid cell. The proxy validation approach also confirmed the utility of a generated hybrid climate dataset over other datasets, especially in hydrologic simulations.
This study suggested a framework called the REFerence Reliability Evaluation System to systematically generate a performance-based hybrid climate dataset from multiple climate datasets for a region. The hybrid dataset was found to more reliable for hydrological modelling. The REFRES is composed of three modules: (1) performance measures, (2) ranking, and (3) data generation. The suggested framework was applied to the ARB as a test bed and generated two hybrid climate datasets from single- (Rind) and multi-variable (Rmul) approaches by evaluating the performance of five available gridded climate datasets: station-based gridded climate datasets (i.e., ANUSPLIN, Alberta Township, and PNWNAmet), a multi-source dataset (CaPA), and a reanalysis-based dataset (NARR). A hydrologic-modelling-based proxy validation approach was applied to demonstrate the applicability of the hybrid climate dataset generated for the five sub-basins in the ARB. The results showed the following:
-
Among the five climate datasets, the station-based climate datasets performed better than multi-source- and reanalysis-based datasets. The Township dataset, in particular, outperformed other climate datasets in the selected performance measures over northern Alberta.
-
Most of the climate datasets performed poorly in the mountainous areas of southwest Alberta, due to a sparse observation network, orographic effects, topographic complexity, and inconsistencies in observation between Canada and the US.
-
As a result of REFRES' application for the ARB, the Township and ANUSPLIN datasets are mostly ranked the highest among the five climate datasets for precipitation and temperature, respectively.
-
In the proxy validation, two hybrid climate datasets, Hybrid(Rind) and Hybrid(Rmul), performed better in terms of precision and accuracy as forcing data for hydrologic simulations.
-
Hybrid(Rind) especially outperformed other climate datasets in the Clearwater sub-basin where the highest number of climate datasets were combined in generating Hybrid(Rind) for precipitation. This indicates that the hybrid climate dataset generated by REFRES may lead to more reliable hydrologic simulations, resulting in improved hydrologic predictions.
This study provided the preference order of climate datasets available in Alberta, which may be useful for modellers and decision-makers as to which climate dataset is the most suitable for their studies and projects. Furthermore, this study demonstrated that the hybrid climate dataset produced by REFRES is more representative of historical climatic conditions. Therefore, the hybrid climate dataset is recommended to be used as a reference dataset for statistical downscaling and hydrologic-model forcing, resulting in more reliable high-resolution climatic and hydrologic projections.
The REFRES package is available upon request by contacting hyung.eum@gov.ab.ca. The Variable Infiltration Capacity (VIC) model is also freely available at https://github.com/UW-Hydro/VIC (Liang et al., 1994).
ANUSPLIN can be accessed at ftp://ftp.nrcan.gc.ca/pub/outgoing/canada_daily_grids (Hopkinson et al., 2011), and PNWNAmet is available at https://data.pacificclimate.org/portal/gridded_observations/map/ (Werner et al., 2019). The Alberta Township data can be downloaded from http://agriculture.alberta.ca/acis/township-data-viewer.jsp (Shen et al., 2001; Alberta Agriculture and Forestry, contact Ralph Wright at +1 (780) 427-3556 for details). The archives of CaPA can be accessed at http://collaboration.cmc.ec.gc.ca/science/outgoing/capa.grib/ (Lespinas et al., 2015) and http://collaboration.cmc.ec.gc.ca/science/outgoing/capa.grib/hindcast/ (Lespinas et al., 2015), and the last 30 d of CaPA data are available at http://dd.weather.gc.ca/analysis/precip/rdpa/grib2/polar_stereographic (Lespinas et al., 2015). The NARR dataset is available at https://www.esrl.noaa.gov/psd/data/gridded/data.narr.monolevel.html (Mesinger et al., 2006). The hybrid climate dataset for Alberta is also available upon request by contacting hyung.eum@gov.ab.ca.
HIE conceived and designed the study, carried out the development of REFRES, hydrologic simulations, and all analyses, and prepared the first draft. AG contributed to the analysis and interpretation of the results. All authors contributed to writing and editing the paper.
The authors declare that they have no conflict of interest.
The authors would like to thank Natural Resources Canada, Alberta Agriculture and Forest, the Pacific Climate Impacts Consortium, Environment and Climate Change Canada, and NOAA/OAR/ESRL PSD for providing the historical gridded climate datasets.
This research has been supported by Alberta Environment and Parks (grant no. MSDEA5).
This paper was edited by Xing Yuan and reviewed by two anonymous referees.
Asong, Z. E., Khaliq, M. N., and Wheater, H. S.: Regionalization of precipitation characteristics in the Canadian Prairie Provinces using large-scale atmospheric covariates and geophysical attributes, Stoch. Env. Res. Risk A., 29, 875–892, 2015.
Betrie, G. D., Deng, B., and Wang, J.: Integrated modeling of the Athabasca River Basin using SWAT, Proceedings of Science and Technology Innovations, Faculty of Science and Technology, Athabasca University, Alberta, Canada, 27–38, ISBN 978-1-987973-00-6, 2015.
Choi, W., Kim, S. J., Rasmussen, P. F., and Moore, A. R.: Use of the North American Regional Reanalysis for hydrological modeling in Manitoba, Can. Water Resour. J., 34, 13–36, 2009.
Christensen, N. S. and Lettenmaier, D. P.: A multimodel ensemble approach to assessment of climate change impacts on the hydrology and water resources of the Colorado River Basin, Hydrol. Earth Syst. Sci., 11, 1417–1434, https://doi.org/10.5194/hess-11-1417-2007, 2007.
Côté, J., Desmarais, J.-G., Gravel, S., Méthot, A., Patoine, A., Roch, M., and Staniforth, A.: The operational CMC–MRB global environmental multiscale (GEM) model. Part II: Results, Mon. Weather Rev., 126, 1397–1418, 1998a.
Côté, J., Gravel, S., Méthot, A., Patoine, A., Roch, M., and Staniforth, A.: The operational CMC–MRB global environmental multiscale (GEM) model. Part I: Design considerations and formulation, Mon. Weather Rev., 126, 1373–1395, 1998b.
Deacu, D., Fortin, V., Klyszejko, E., Spence, C., and Blanken, P. D.: Predicting the Net Basin Supply to the Great Lakes with a Hydrometeorological Model, J. Hydrometeorol., 13, 1739–1759, https://doi.org/10.1175/JHM-D-11-0151.1, 2012.
Demaria, E. M., Nijssen, B., and Wagener, T.: Monte Carlo sensitivity analysis of land surface parameters using the variable infiltration capacity model, J. Geophys. Res., 112, D11113, https://doi.org/10.1029/2006JD007534, 2007.
Dibike, Y., Eum, H.-I., and Prowse, T.: Modelling the Athabasca watershed snow response to a changing climate, J. Hydrol., 15, 134–148, https://doi.org/10.1016/j.ejrh.2018.01.003, 2018.
Essou, G. R. C., Sabarly, F., Lucas-Picher, P., Brissette, F., and Poulin, A.: Can Precipitation and Temperature from Meteorological Reanalyses Be Used for Hydrological Modeling?, J. Hydrometeorol., 17, 1929–1950, https://doi.org/10.1175/JHM-D-15-0138.1, 2016.
Eum, H.-I. and Cannon, A. J.: Intercomparison of projected changes in climate extremes for South Korea: application of trend preserving statistical downscaling methods to the CMIP5 ensemble, Int. J. Climatol., 37, 3381–3397, https://doi.org/10.1002/joc.4924, 2017.
Eum, H.-I., Gachon, P., Laprise, R., and Ouarda, T.: Evaluation of regional climate model simulations versus gridded observed and regional reanalysis products using a combined weighting scheme, Clim. Dynam., 38, 1433–1457, https://doi.org/10.1007/s00382-011-1149-3, 2012.
Eum, H.-I., Dibike, Y., Prowse, T., and Bonsal, B.: Inter-comparison of high-resolution gridded climate data sets and their implication on hydrological model simulation over the Athabasca Watershed, Canada, Hydrol. Process., 28, 4250–4271, https://doi.org/10.1002/hyp.10236, 2014a.
Eum, H.-I., Dibike, Y., and Prowse, T.: Uncertainty in modelling the hydrologic responses of a large watershed: a case study of the Athabasca River basin, Canada, Hydrol. Process., 28, 4272–4293, https://doi.org/10.1002/hyp.10230, 2014b.
Eum, H.-I., Dibike, Y., and Prowse, T.: Comparative evaluation of the effects of climate and land-cover changes on hydrologic responses of the Muskeg River, Alberta, Canada, J. Hydrol., 8, 198–221, https://doi.org/10.1016/j.ejrh.2016.10.003, 2016.
Eum, H.-I., Dibike, Y., and Prowse, T.: Climate-induced alteration of hydrologic indicators in the Athabasca River Basin, Alberta, Canada, J. Hydrol., 544, 327–342, https://doi.org/10.1016/j.jhydrol.2016.11.034, 2017.
Faramarzi, M., Srinivasan, R., Iravani, M., Bladon, K. D., Abbaspour, K. C., Zehnder, A. J. B., and Goss, G. G.: Setting up a hydrological model of Alberta: Data discrimination analyses prior to calibration, Environ. Modell. Softw., 74, 48–65, https://doi.org/10.1016/j.envsoft.2015.09.006, 2015.
Faramarzi, M., Abbaspour, K. C., Adamowicz, W. L., Lu, W., Fennell, J., Zehnder, A. J. B., and Goss, G. G.: Uncertainty based assessment of dynamic freshwater scarcity in semi-arid watersheds of Alberta, Canada, J. Hydrol., 9, 48–68, https://doi.org/10.1016/j.ejrh.2016.11.003, 2017.
Garand, L. and Grassotti, C.: Toward an objective analysis of rainfall rate combining observations and short-term forecast model estimates, J. Appl. Meteorol., 34, 1962–1977, 1995.
Gutmann, E., Pruitt, T., Clark, M. P., Brekke, L., Arnold, J. R., Raff, D. A., and Rasmussen, R. M.: An intercomparison of statistical downscaling methods used for water resource assessments in the United States, Water Resour. Res., 50, 7167–7186, https://doi.org/10.1002/2014WR015559, 2014.
Harpold, A. A., Kaplan, M. L., Klos, P. Z., Link, T., McNamara, J. P., Rajagopal, S., Schumer, R., and Steele, C. M.: Rain or snow: hydrologic processes, observations, prediction, and research needs, Hydrol. Earth Syst. Sci., 21, 1–22, https://doi.org/10.5194/hess-21-1-2017, 2017.
Hopkinson, R. F., McKenney, D. W., Milewska, E. J., Hutchinson, M. F., Papadopol, P., and Vincent, L. A.: Impact of Aligning Climatological Day on Gridding Daily Maximum–Minimum Temperature and Precipitation over Canada, J. Appl. Meteorol. Clim., 50, 1654–1665, https://doi.org/10.1175/2011JAMC2684.1, 2011 (data available at: ftp://ftp.nrcan.gc.ca/pub/outgoing/canada_daily_grids, last access: 11 December 2019).
Hutchinson, M. F., McKenney, D. W., Lawrence, K., Pedlar, J. H., Hopkinson, R. F., Milewska, E., and Papadopol, P.: Development and Testing of Canada-Wide Interpolated Spatial Models of Daily Minimum–Maximum Temperature and Precipitation for 1961–2003, J. Appl. Meteorol. Clim., 48, 725–741, https://doi.org/10.1175/2008JAMC1979.1, 2009.
Hwang, C. L. and Yoon, K.: Multiple attribute decision making: methods and applicasions, Springer, New York, USA, 1981.
Islam, S. U. and Déry, S. J.: Evaluating uncertainties in modelling the snow hydrology of the Fraser River Basin, British Columbia, Canada, Hydrol. Earth Syst. Sci., 21, 1827–1847, https://doi.org/10.5194/hess-21-1827-2017, 2017.
Jun, K. S., Chung, E.-S., Kim, Y.-G., and Kim, Y.: A fuzzy multi-criteria approach to flood risk vulnerability in South Korea by considering climate change impacts, Expert Systems with Applications, 40, 1003–1013, 2013.
Kay, A. L., Davies, H. N., Bell, V. A., and Jones, R. G.: Comparison of uncertainty sources for climate change impacts: flood frequency in England, Climatic Change, 92, 41–63, https://doi.org/10.1007/s10584-008-9471-4, 2009.
Kirchner, J. W.: Getting the right answers for the right reasons: Linking measurements, analyses, and models to advance the science of hydrology: getting the right answers for the right reasons, Water Resour. Res., 42, W03S04, https://doi.org/10.1029/2005WR004362, 2006.
Klyszejko, E. S.: Hydrologic Validation of Real-Time Weather Radar VPR Correction Methods, Master Thesis, University of Waterloo, Ontario, Canada, 263 pp., 2007.
Lee, G., Jun, K.-S., and Chung, E.-S.: Integrated multi-criteria flood vulnerability approach using fuzzy TOPSIS and Delphi technique, Nat. Hazards Earth Syst. Sci., 13, 1293–1312, https://doi.org/10.5194/nhess-13-1293-2013, 2013.
Leong, D. N. S. and Donner, S. D.: Climate change impacts on streamflow availability for the Athabasca Oil Sands, Climatic Change, 133, 651–663, https://doi.org/10.1007/s10584-015-1479-y, 2015.
Lespinas, F., Fortin, V., Roy, G., Rasmussen, P., and Stadnyk, T.: Performance Evaluation of the Canadian Precipitation Analysis (CaPA), J. Hydrometeorol., 16, 2045–2064, https://doi.org/10.1175/JHM-D-14-0191.1, 2015 (data available at: http://dd.weather.gc.ca/analysis/precip/rdpa/grib2/polar_stereographic, http://collaboration.cmc.ec.gc.ca/science/outgoing/capa.grib/, and http://collaboration.cmc.ec.gc.ca/science/outgoing/capa.grib/hindcast/, last access: 11 December 2019).
Liang, X., Lettenmaier, D. P., Wood, E. F., and Burges, S. J.: A simple hydrologically based model of land surface water and energy fluxes for general circulation model, J. Geophys. Res., 99, 14415–14428, https://doi.org/10.1029/94JD00483, 1994 (data available at: https://github.com/UW-Hydro/VIC, last access: 11 December 2019).
Mahfouf, J.-F., Brasnett, B., and Gagnon, S.: A Canadian Precipitation Analysis (CaPA) Project: Description and Preliminary Results, Atmos.-Ocean, 45, 1–17, https://doi.org/10.3137/ao.v450101, 2007.
Mekis, E. and Hogg, W. D.: Rehabilitation and analysis of Canadian daily precipitation time series, Atmos.-Ocean, 37, 53–85, https://doi.org/10.1080/07055900.1999.9649621, 1999.
Mekis, É. and Vincent, L. A.: An Overview of the Second Generation Adjusted Daily Precipitation Dataset for Trend Analysis in Canada, Atmos.-Ocean, 49, 163–177, https://doi.org/10.1080/07055900.2011.583910, 2011.
Mesinger, F., DiMego, G., Kalnay, E., Mitchell, K., Shafran, P. C., Ebisuzaki, W., Jović, D., Woollen, J., Rogers, E., Berbery, E. H., Ek, M. B., Fan, Y., Grumbine, R., Higgins, W., Li, H., Lin, Y., Manikin, G., Parrish, D., and Shi, W.: North American Regional Reanalysis, B. Am. Meteorol. Soc., 87, 343–360, https://doi.org/10.1175/BAMS-87-3-343, 2006 (data available at: https://www.esrl.noaa.gov/psd/data/gridded/data.narr.monolevel.html, last access: 11 December 2019).
Moriasi, D. N., Arnold, J. G., Van Liew, M. W., Bingner, R. L., Harmel, R. D., and Veith, T. L.: Model evaluation guidelines for systematic quantification of accuracy in watershed simulations, T. ASABE, 50, 885–900, 2007.
NIDIS: U.S. Drought Portal. NOAA, available at: http://www.drought.gov (last access: 11 December 2019), 2015.
Praskievicz, S. and Bartlein, P.: Hydrologic modeling using elevationally adjusted NARR and NARCCAP regional climate-model simulations: Tucannon River, Washington, J. Hydrol., 517, 803–814, https://doi.org/10.1016/j.jhydrol.2014.06.017, 2014.
Rinke, A., Marbaix, P., and Dethloff, K.: Internal variability in Arctic regional climate simulations: case study for the SHEBA year, Clim. Res., 27, 197–209, https://doi.org/10.3354/cr027197, 2004.
Shen, S. S., Dzikowski, P., Li, G., and Griffith, D.: Interpolation of 1961–97 daily temperature and precipitation data onto Alberta polygons of ecodistrict and soil landscapes of Canada, J. Appl. Meteorol., 40, 2162–2177, https://doi.org/10.1175/1520-0450(2001)040<2162:IODTAP>2.0.CO;2, 2001 (data available at: http://agriculture.alberta.ca/acis/township-data-viewer.jsp, last access: 11 December 2019).
Shen, Y., Xiong, A., Wang, Y., and Xie, P.: Performance of high-resolution satellite precipitation products over China, J. Geophys. Res., 115, D02114, https://doi.org/10.1029/2009JD012097, 2010.
Shook, K. and Pomeroy, J.: Changes in the hydrological character of rainfall on the Canadian prairies, Hydrol. Process., 26, 1752–1766, 2012.
Shrestha, N. K., Du, X., and Wang, J.: Assessing climate change impacts on fresh water resources of the Athabasca River Basin, Canada, Sci. Total Environ., 601, 425–440, 2017.
Shrestha, R. R., Schnorbus, M. A., Werner, A. T., and Berland, A. J.: Modelling spatial and temporal variability of hydrologic impacts of climate change in the Fraser River basin, British Columbia, Canada, Hydrol. Process., 26, 1840–1860, 2012.
Shrestha, R. R., Cannon, A. J., Schnorbus, M. A., and Zwiers, F. W.: Projecting future nonstationary extreme streamflow for the Fraser River, Canada, Climatic Change, 145, 289–303, https://doi.org/10.1007/s10584-017-2098-6, 2017.
Vincent, L. A., Wang, X. L., Milewska, E. J., Wan, H., Yang, F., and Swail, V.: A second generation of homogenized Canadian monthly surface air temperature for climate trend analysis: homogenized canadian temperature, J. Geophys. Res.-Atmos., 117, D18110, https://doi.org/10.1029/2012JD017859, 2012.
Wang, X. L. and Lin, A.: An algorithm for integrating satellite precipitation estimates with in situ precipitation data on a pentad time scale: blended pentad precipitation data, J. Geophys. Res.-Atmos., 120, 3728–3744, https://doi.org/10.1002/2014JD022788, 2015.
Weedon, G. P., Balsamo, G., Bellouin, N., Gomes, S., Best, M. J., and Viterbo, P.: The WFDEI meteorological forcing data set: WATCH Forcing Data methodology applied to ERA-Interim reanalysis data, Water Resour. Res., 50, 7505–7514, 2014.
Werner, A. T. and Cannon, A. J.: Hydrologic extremes – an intercomparison of multiple gridded statistical downscaling methods, Hydrol. Earth Syst. Sci., 20, 1483–1508, https://doi.org/10.5194/hess-20-1483-2016, 2016.
Werner, A. T., Schnorbus, M., Shrestha, R., Cannon, A., Zwiers, F., Dayon, G., and Anslow, F.: A long-term, temporally consistent, gridded daily meteorological dataset for northwestern North America, Scientific Data, 6, 180299, https://doi.org/10.1038/sdata.2018.299, 2019 (data available at: https://data.pacificclimate.org/portal/gridded_observations/map/, last access: 11 December 2019).
Wong, J. S., Razavi, S., Bonsal, B. R., Wheater, H. S., and Asong, Z. E.: Inter-comparison of daily precipitation products for large-scale hydro-climatic applications over Canada, Hydrol. Earth Syst. Sci., 21, 2163–2185, https://doi.org/10.5194/hess-21-2163-2017, 2017.
Zhao, K.: Validation of the Canadian Precipitation Analysis (CaPA) for hydrological modelling in the Canadian Prairies, Master Thesis, University of Manitoba, Manitoba, Canada, 163 pp., 2013.