the Creative Commons Attribution 4.0 License.
the Creative Commons Attribution 4.0 License.
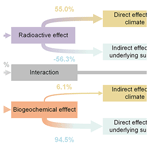
Future response of ecosystem water use efficiency to CO2 effects in the Yellow River Basin, China
Siwei Chen
Yuxue Guo
Lu Wang
Ecosystem water use efficiency (WUE) is pivotal for understanding carbon–water cycle interplay. Current research seldom addresses how WUE might change under future elevated CO2 concentrations, limiting our understanding of regional ecohydrological effects. We present a land–atmosphere attribution framework for WUE in the Yellow River basin (YRB), integrating the Budyko model with global climate models (GCMs) to quantify the impacts of climate and underlying surface changes induced by CO2. Additionally, we further quantitatively decoupled the direct and secondary impacts of CO2 radiative and biogeochemical effects. Attribution results indicate that WUE in the YRB is projected to increase by 0.36–0.84 gC kg−1H2O in the future, with climate change being the predominant factor (relative contribution rate of 77.9 %–101.4 %). However, as carbon emissions intensify, the relative importance of land surface changes becomes increasingly important (respective contribution rates of −1.4 %, 14.9 %, 16.9 %, and 22.1 % in SSP126, SSP245, SSP370, and SSP585). Typically, WUE is considered a reflection of an ecosystem's adaptability to water stress. Thus, we analyzed the response of WUE under different scenarios and periods and various drought conditions. The results show a distinct “two-stage” response pattern of WUE to drought in the YRB, where WUE increases under moderate–severe drought conditions but decreases as drought intensifies across most areas. Furthermore, GCM projections suggest that plant adaptability to water stress may improve under higher-carbon-emission scenarios. Our findings enhance the understanding of regional ecohydrological processes and provide insights for future predictions of drought impacts on terrestrial ecosystems.
- Article
(6750 KB) - Full-text XML
- BibTeX
- EndNote
Ecosystem water use efficiency (WUE) is commonly defined as the ratio of the ecosystem's gross primary productivity (GPP) to water evapotranspiration (ET), i.e., WUE = , which reflects the carbon gain per unit of water lost (Keenan et al., 2013; Li et al., 2023a; Naeem et al., 2023). This metric couples the water and carbon cycle processes, serving as a key characteristic indicator for ecosystem function (Liu et al., 2020). Investigating the spatiotemporal characteristics and driving factors of WUE holds substantial practical importance for exploring the response mechanisms of ecosystems under changing environmental conditions (Huang et al., 2017; Tan et al., 2023; Zhou et al., 2017).
The increase in atmospheric CO2 concentrations brings profound impacts on regional WUE, GPP, and ET through radiative effects and biogeochemical effects (Naeem et al., 2023; Yang et al., 2022). The interactions between land and atmosphere further complicate the changes associated with rising CO2 levels (De Kauwe et al., 2021; He et al., 2023; Zhan et al., 2022). Regarding radiative effects, elevated CO2 directly affects hydro-atmospheric processes, altering global and regional precipitation and evaporation patterns (Bintanja and Andry, 2017; L. Gu et al., 2023; Yin et al., 2018). The biogeochemical effects are primarily manifested through the impacts of CO2 fertilization and changes in plant stomatal conductance on the underlying vegetation structure. Fertilization effect refers to the increased CO2 concentrations enhancing photosynthesis rates, thereby increasing vegetation productivity and promoting plant growth (Chen et al., 2024; Sun et al., 2018). He et al. (2023) demonstrated that CO2 was the dominant driver of the increase in forest carbon sinks over the past few decades. The growth in plant biomass can lead to an increase in evapotranspiration (Xie et al., 2020). However, elevated CO2 concentrations can also contribute to a reduction in leaf stomatal conductance, potentially resulting in a decrease in transpiration (De Kauwe et al., 2021; Zhu et al., 2011). Beyond these direct climatic changes through radiative and direct biogeochemical effects on the land surface, the climate and land surface are also subject to secondary effects. For example, climate change can further impact vegetation cover (Berg et al., 2017); changes in the structure of surface vegetation will alter the water–energy exchange process, thereby affecting precipitation and other processes (Zhou et al., 2022). Few studies have focused on the relative contributions of CO2's direct and secondary effects on WUE. However, understanding these contributions is crucial for exploring the mechanisms of WUE changes and accurately predicting future changes in WUE.
Conducting a joint analysis of hydrological variables using conceptual models and global climate models (GCMs) serves as an effective method for attributing land–atmosphere processes (Zhou et al., 2023). Since its inception, the Budyko model (Budyko, 1974) has been extensively employed to evaluate the relationships between water–energy exchanges and changes in surface characteristics under different climate scenarios (Fang et al., 2020; Fathi et al., 2019; Roderick and Farquhar, 2011). As a conceptual framework, the Budyko model is characterized by its simplicity and excellent performance, elucidating the relationship between () and () (Cheng et al., 2011; Choudhury, 1999; Xu et al., 2022). Yang et al. (2015) applied the Budyko framework to the carbon cycle, revealing the relationship between GPP and energy exchange. Fang et al. (2020) explored the relationship between GPP, WUE, and water–energy relations in semi-arid basins through the Budyko model, investigating the correlations between WUE and underlying surface parameters. Despite previous efforts to extend the scope of the Budyko model from water–energy relations to water–carbon–energy relations, there have been few descriptions of how these relationships may change in the future along with the relative contribution of elements to the studied variables.
The complexity of drought events can have significant impacts on the regional economy and environment (Yin et al., 2023; Yuan et al., 2023). Variations in WUE under drought conditions have been recognized as an indicator of ecosystems' adaptability to drought stress (Huang et al., 2017; Ponce-Campos et al., 2013). Ponce-Campos et al. (2013) suggested that ecosystems increased their WUE in response to water stress. However, observations have shown considerable variability in WUE responses to drought events across different regions (Huang et al., 2017; Lu and Zhuang, 2010; M. Wang et al., 2021; Xie et al., 2016; Yang et al., 2021). This indicates the need for more in-depth research into the WUE–drought relationship. According to Pokhrel et al. (2021), in the context of climate change, the risk of drought in most regions worldwide is expected to continue increasing, which raises the question of how WUE will respond to drought in the future. This study aims to explore the future response mechanisms of WUE to drought to better assess the resilience of ecosystems under climate change. The drought severity index (TWSA-DSI) based on the terrestrial water storage anomaly (TWSA) has been widely used in drought monitoring and analysis (Yin et al., 2022, 2023; Zhao et al., 2017). This index effectively monitors regional drought characteristics and development paths (Yin et al., 2022). Compared to other traditional drought indicators such as the Palmer drought severity index (PDSI), soil moisture drought index (SMI), standardized precipitation index (SPI), standardized runoff index (SRI), etc., TWSA-DSI is better able to detect overall regional drought conditions and to explore the effects of drought on hydrological systems and vegetation growth (A et al., 2017; Du et al., 2019; Zhao et al., 2017). This study chooses to use TWSA-DSI to identify regional drought events, allowing for a more nuanced understanding of how WUE may adapt or respond to these conditions in various ecosystems.
The Yellow River basin (YRB) is an important basin in China, situated in the arid and semi-arid regions. The ecosystem within the basin is complex and vulnerable to drought (Huang et al., 2015). Changes in the ecohydrological processes of the YRB in the future will profoundly impact China's socioeconomic development. This paper selects the YRB as the study area, trying to deepen the understanding of the spatiotemporal variation mechanisms of WUE in this region. This study aims to answer the following three research questions over both historical and future timescales:
- a.
What changes will occur in the spatiotemporal distribution of WUE within the basin?
- b.
How can the impact of elevated atmospheric CO2 concentrations on WUE changes be quantitatively explained?
- c.
How does the response mechanism of WUE to drought within the basin evolve under future scenarios?
The reminder of this paper is organized as follows. Section 2 describes the study area and the data used. Section 3 defines the methodology. Section 4 and Sect. 5 give the results and discussion of the study, respectively. Finally, Sect. 6 concludes the paper.
2.1 Study area
The Yellow River is the second-longest river in China and the fifth-longest river in the world, with a total length of about 5464 km. The YRB (32–41° N, 95–119° E) is the second-largest drainage basin in China (Fig. 1), with an approximate area of 795 000 km2 (including a 42 000 km2 inland flow area). The YRB spans the Tibetan Plateau, the Inner Mongolian Plateau, the Loess Plateau, and the North China Plain. The average altitude of the basin exceeds 3000 m, and the terrain becomes progressively lower from west to east. The sub-basins are characterized by a wide range of climatic and geomorphologic variations. Overall, the basin has a largely semi-humid climate in the southeast and an arid/semi-arid climate in the center and the northwest. The YRB is one of the earliest origins of human civilization, and the Yellow River is also known as the “mother river” of China. The YRB has a population of more than 120 million people, a gross national product of about 8 trillion RMB (≈ 1.1 trillion USD), and a cultivated agricultural area of about 200 000 km2. The YRB has been regarded as one of the most important basins in China because of its important role in socioeconomic development, ecological resource protection, and agricultural food production.
2.2 Data collection
2.2.1 Climate and GPP data
The monthly precipitation, potential evapotranspiration, and evapotranspiration and GPP datasets during 1997–2016 were both retrieved from National Tibetan Plateau Data Center (https://data.tpdc.ac.cn/, last access: 1 February 2024). The precipitation and potential evapotranspiration dataset are based on the Climatic Research Unit (CRU) time series (TS) v4.02 dataset and WorldClim v2.0 data to generate data with high spatial resolution and accuracy (Ding and Peng, 2020, 2021). The evapotranspiration dataset employed a calibration-free nonlinear complementary relationship model, and the evaluation suggests that the evapotranspiration dataset has a good performance (Ma et al., 2019a; Ma and Szilagyi, 2019). The GPP dataset was developed based on the satellite-based near-infrared reflectance of vegetation (NIRv) (S. Wang et al., 2021). The accuracy and reliability have been validated by many studies (Cai et al., 2023; Feng et al., 2023; P. Li et al., 2023; Peng et al., 2023; Xing et al., 2023). For consistency, the above data were bilinearly interpolated to 0.25°×0.25°. To avoid uncertainties introduced by extremely small values, this study calculated only grid-point monthly WUE with GPP > 10 gC m2 and ET > 10 mm following the approach used by Naeem et al. (2023).
2.2.2 Vegetation indicator
In this study, the normalized difference vegetation index (NDVI) and the leaf area index (LAI) were selected as vegetation indicators to characterize the underlying surface conditions. The GIMMS3g NDVI and GIMMS4g LAI data, acquired from the Advanced Very High Resolution Radiometer (AVHRR) sensor from NOAA's series of weather satellites, provide high-spatiotemporal-resolution and high-accuracy observed vegetation indicators (Pinzon and Tucker, 2014; Cao et al., 2023a). These data are freely available on NASA's website (https://daac.ornl.gov/VEGETATION/guides/Global_Veg_Greenness_GIMMS_3G.html, last access: 1 February 2024; https://doi.org/10.5281/zenodo.8281930, Cao et al., 2023b). To match the other data, the vegetation data were processed into monthly 0.25° × 0.25° gridded data.
2.2.3 TWSA datasets
To assess long-term changes in drought conditions in the basin, monthly reconstructed TWSA datasets from 1997–2016 were used in this study (Humphrey and Gudmundsson, 2019a). This dataset is based on two different Gravity Recovery and Climate Experiment (GRACE)/Gravity Recovery and Climate Experiment Follow-On (GRACE-FO) satellite products and three different meteorological forcing datasets, producing six reconstructed TWSA datasets with 100 ensemble members each. Its performance is effectively validated (H. Gu et al., 2023; Yin et al., 2023; Zhong et al., 2023). In this study, we calculated the mean results of the six datasets and thus bilinearly interpolated them to 0.25° × 0.25°.
2.2.4 GCM output data
In order to project future climate scenarios, we selected seven GCMs in CMIP6 (Table 1). These models were chosen because they encompass most or even all of the variables required for our analysis. Furthermore, they incorporate the experimental settings needed for our research and integrate the dynamic response of vegetation to climate change. In comparison to CMIP5, CMIP6 uses the matrix framework of the Shared Socioeconomic Pathway (SSP) and the Representative Concentration Pathway (RCP). In this study, we utilized simulated monthly data for GPP (gpp), evapotranspiration (evspsbl), precipitation (pr), and terrestrial water storage (mrtws), where the terms in parentheses represent the variable names within CMIP6. These data encompassed the historical period from 1985–2014 and projected future scenarios, including SSP126 (sustainable development; 2015–2100), SSP245 (moderate development; 2015–2100), SSP370 (regional rivalry development; 2015–2100), and SSP585 (conventional development; 2015–2100). These scenarios represent a range of possible futures from sustainable, low-emission trajectories to high-emission pathways based on varying degrees of socioeconomic development and environmental policy implementation. To assess the impact of rising CO2 concentration on WUE, we also used data from three CO2 sensitivity experiments – namely, 1ptCO2, 1ptCO2-bgc, and 1ptCO2-rad. In 1ptCO2, the concentration of CO2 in the atmosphere increases by 1 % per year. In 1ptCO2-bgc and 1ptCO2-rad, the growth rate of CO2 is the same as that in 1ptCO2, but the ones ending in -rad is only coupled with the atmospheric part (CO2 radiative forcing), while that ending in -bgc is only coupled with the land part (CO2 biogeochemically forcing), and the other part maintained the fixed CO2 concentration level of pre-industrialization. It is notable that in all three experiments, the CO2 concentration rises at the same rate as in SSP585.
Table 1CMIP6 GCMs used in this study. The eighth column shows the model outputs for the three CO2 sensitivity experiments (1ptCO2, 1ptCO2-bgc, and 1ptCO2-rad). GPP, ET, PET, P, and TWSA are represented by red, blue, yellow, green, and black crosses, respectively.
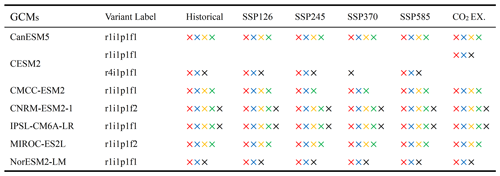
To obtain the TWSA series from CMIP6, the common practice is to select the same baseline period (2004–2009) as GRACE/GRACE-FO satellite data and deduct the series mean value during this period to obtain the TWSA series (Pokhrel et al., 2021; Yin et al., 2022). In this study, we also used this method. Since there is no direct output of potential evapotranspiration from CMIP6, we used near-surface air temperature (Tas), near-surface wind speed (sfcWind), relative humidity (hur), surface pressure (ps), heat flux, net surface radiation, and other data to calculate potential evapotranspiration with the Penman–Monteith equation (Eq. 1), which is the only standard method proposed by the Food and Agriculture Organization of the United Nations (FAO) (Allan et al., 1998). All GCM outputs were bilinearly interpolated to a common spatial resolution of 0.25° × 0.25°.
where PET is the potential evapotranspiration (mm), Δ is the slope of the saturation vapor pressure curve at temperature T (kPa °C−1), Rn is net surface radiation (MJ m−2), G is the soil heat flux (MJ m−2), γ is the psychrometric constant (kPa °C−1), T is the near-surface air temperature (°C), μ2 is the near-surface wind speed (m s−1), and (es−ea) is the vapor pressure deficit (kPa).
3.1 Trend-preserving bias correction
Bias correction is the adjustment of GCM simulation data to reduce their systematic deviation as compared to observations. Compared to traditional bias correction methods, the trend-preserving bias correction method enables more robust bias adjustments for extremes and more accurately maintains trends across quantiles. The main steps of the trend-preserving bias correction are as below (Lange, 2019).
-
Step 1. Detrend observation data (, GCM outputs during historical period (, GCM outputs during the future period or CO2 experiment period ( to obtain three new series, , and , respectively.
-
Step 2. Transfer the change signals between the simulation series ( and to the observation series ( to obtain the pseudo-observation series, .
-
Step 3. Adjust the distribution of based on by quantile mapping. The formula is as follows:
-
Step 4. Add the results from Step 3 to the trend values previously subtracted to get the corrected results.
This study took into account the variations in the frequency distribution functions of TWSA across different periods, thereby implementing corrections for individual months separately. The results indicate that this month-specific correction approach yields better outcomes than a correction approach that does not differentiate by month.
3.2 WUE attribution framework
Many studies applied the Budyko model to isolate the effects of climate change and land surface properties on evapotranspiration or runoff at the basin/catchment scale (Roderick and Farquhar, 2011; Yang and Yang, 2011; Xu et al., 2022). The general Budyko model contemplates the interconnections and feedbacks between water and energy cycles while also considering the impact of basin characteristics. The expression of the model is as follows:
where E and P, respectively, represent evapotranspiration (mm) and precipitation (mm) and c is the basin underlying surface parameter.
In this study, we analyzed the relationship between hydrological elements and vegetation structure. After a selection process, we employed the linear Budyko function structure for WUE as proposed in previous studies (Cheng et al., 2011; Fang et al., 2020). The formula is expressed as follows:
where WUE is the water use efficiency (g C kg−1 H2O) and m and n represent the underlying surface conditions of the basin. The Spearman correlation coefficient and its significance were used to study the relationships between variables in Eq. (3). The model regression results and the coefficient of determination (R2) were derived from data analysis to evaluate the accuracy of the model.
To avoid the effects of climate variability and seasonal changes in the basin underlying parameters in the Budyko model, this study focused on a timescale of about 30 years (Ning et al., 2019).
In traditional attribution analysis based on the Budyko model, the total differentiation method is widely used. Therefore, based on Eq. (3), it can be formulated as follows:
In Eqs. (5) and (6), the terms on the left side of the equality represent the impact of climate change on WUE. Meanwhile, in Eq. (7), the term on the left represents the influence of land surface changes. In accordance with Zhou et al. (2016), we assume that there is such an equation that holds: . In other words, the impact of land surface changes can be represented by the calculated result of . The proof proceeds as follows:
In practical applications, Eq. (4) is commonly expressed as the first-order Taylor approximation for attribution analysis:
where ΔWUE, ΔP, ΔPET, Δm, and Δn, respectively, represent the actual changes in WUE; precipitation; potential evapotranspiration; and two underlying surface parameters, i.e., m and n. It is noteworthy that in Eq. (9), there is consistently a residual term, O, which represents the higher-order terms in the Taylor expansion. The value of O will affect the accuracy of the attribution results.
Zhou et al. (2015) have proven that if the function structure between a dependent variable Z and N independent variables (X1, X2, …, XN) is
the sum of the elasticity coefficients is then unity, i.e. the following:
Demonstrating that a similar complementary relationship exists in is straightforward; namely, the following applies:
Therefore, according to previous studies (Zhou et al., 2016; Yang et al., 2023; Zhang et al., 2023), if such a complementary relationship (Eq. 12) exists, the difference in dependent variable Z (ΔWUE) can be expressed in the following form without any residual term:
where subscripts 1 and 2, respectively, represent the initial- and final-state quantities; Δ denotes the difference between the two states; and α is the weighting factor, with a value range of 0 to 1.
Considering Eqs. (4) and (8), ΔWUE can be further written as changes due to the climate (ΔWUEc) and due to the underlying surface (ΔWUEu):
From Eqs. (14)–(16), the impacts of climate and underlying surface changes on WUE can be precisely decomposed within the Budyko model. However, attribution solely through the Budyko model is insufficient to decouple the effects of CO2 on land and atmosphere. The CO2 sensitivity experiments of GCMs mentioned in Sect. 2.2.4 had been applied to explore the atmosphere–surface interactions caused by elevated CO2 (Piao et al., 2007; Fowler et al., 2019). In the 1ptCO2-rad experiment, the increase in CO2 concentration has a direct radiative effect on the climate and an indirect effect on vegetation physiology; conversely, in the 1ptCO2-bgc experiment, the increase in CO2 concentration has a direct biogeochemical impact on vegetation and then indirectly affects the climate. The difference between the combined results of the -rad and -bgc experiments and the results of the 1ptCO2 experiment are attributed to land–atmosphere interactions. By applying the Budyko attribution method in the three CO2 experiments (Zhou et al., 2023), the impact of land–atmosphere coupling on WUE can be quantitatively interpreted.
3.3 TWSA-DSI
The method proposed by Zhao et al. (2017) was employed to identify terrestrial drought conditions using the TWSA-DSI index. TWSA-DSI is capable of comparing drought characteristics across regions and periods, and it can also sensitively capture the available water amount affecting vegetation growth. The calculation is as follows:
where and TWSAi,j represent the TWSA-DSI and TWSA data for the ith year and jth month and and σj, respectively, denote the average and standard deviation of TWSA for the jth month within the study period.
In this study, the TWSA-DSI index was calculated for each grid point within the basin for each study period (experiment). Based on these results, different levels of terrestrial wetness/dryness were classified as shown in Table 2 (Yin et al., 2022). Three main categories of terrestrial wet and dry conditions were investigated in this study: non-drought (TWSA-DSI > −0.8), moderate–severe drought (−1.6 < TWSA-DSI ≤ −0.8), and extreme–exceptional drought (TWSA-DSI ≤ −1.6).
3.4 Conditional probability
The impact of different drought levels on WUE can be assessed by considering the conditional probability distribution of the drought index. Referring to previous studies (Feng et al., 2019; Wu and Jiang, 2022), WUE is transformed into a standardized form using a meta-Gaussian model specifically as the standardized water use efficiency index (SWI). The differences in the distribution of SWI under different drought conditions (with TWSA-DSI as the drought index) were used to evaluate the response of WUE to different drought severity levels. As TWSA-DSI is also a standardized normal random variable (X), the bivariate conditional distribution of SWI (Y) given X can be straightforwardly expressed as follows:
where μY|X is the conditional mean and ΣY|X is the conditional variance.
4.1 Assessment of bias correction performance
We applied bias correction to the monthly GCM data using the method described in Sect. 3.1 and compared the correction results for the overlapping period (1997–2014) with observation data. It is noteworthy that different GCMs include a varying number of variables. As a result, even though we have endeavored to select the same models, the ensemble GCM members for different variables are not exactly the same. The performance of correction was presented in the form of Taylor diagrams (Fig. 2). The correction results for GPP and ET were substantially better than those for P, PET, and TWSA. The correlation coefficients (CCs) for GPP and ET after correction were all above 0.97, with multi-model average root mean square errors (RMSEs) of 9.90 gC m−2 and 7.35 mm, respectively. The inter-model differences were also small. Thus, it can be assumed that the WUE calculated from the corrected output data was credible. For P, PET, and TWSA, the models with the best correction effects were IPSL-CM6A-LR, CMCC-ESM2, and CESM2, respectively. Their CC reaches 0.89, 0.99, and 0.82, with RMSE values of 18.13, 8.35, and 10.16 mm. In comparison, CMCC-ESM2 performed relatively poor for P with a CC of 0.74. CNRM-ESM2-1 exhibited suboptimal correction performance for PET and TWSA, with CC values of 0.78 and 0.70, respectively. Overall, the outputs of all GCMs showed good performance after bias correction (with CCs all greater than or equal to 0.70). The trend-preserving bias correction method effectively eliminated systematic errors between GCMs and observation data. The corrected data can be used for the assessment and attribution of future climate scenarios.
4.2 Changes in WUE over YRB
Figure 3 illustrated the time series of multi-model annual averages for WUE, GPP, and ET under historical and different future scenarios. During the historical period, WUE and GPP in the YRB showed an increasing trend, while ET exhibited a slight rise with fluctuations. From 2015 to 2100, the changes in each indicator varied in different scenarios, but the trend patterns of WUE and GPP are relatively similar (Fig. 3a and b). Under the low-emission pathway scenario (SSP126), WUE and GPP first experienced a slight increase in the future (peaking around 1.68 gC kg−1H2O and 675 gC m−2, respectively), which was then followed by a decline. In the moderate-emission scenario (SSP245), WUE and GPP increased before stabilizing at the end of the century (2070–2099), with values of 1.55 gC kg−1H2O and 771 gC m−2, respectively. Both WUE and GPP exhibited a continuous upward trend in the high-emission scenarios (SSP370 and SSP585), and SSP585 showed a higher growth rate compared to SSP370. For ET (Fig. 3c), there was a subtle increase under the low-emission scenario (SSP126) and a slight decrease towards the end of the century. Guo et al. (2022) observed that temperature and precipitation demonstrate similar developmental trends in the YRB under SSP126 as the low-emission pathway aims to limit global warming. Given that temperature and precipitation are crucial drivers of evapotranspiration, it is logical to see ET following this pattern of development. In other scenarios, ET showed an overall upward trend, and with increasing carbon emissions, ET in the YRB increased more rapidly. Additionally, it can be observed from the box plots that the outputs for WUE, GPP, and ET from the multi-model ensemble were more concentrated during the historical period, whereas they were more dispersed in the future periods. Furthermore, the degree of dispersion increased with the rise in carbon emission concentrations.
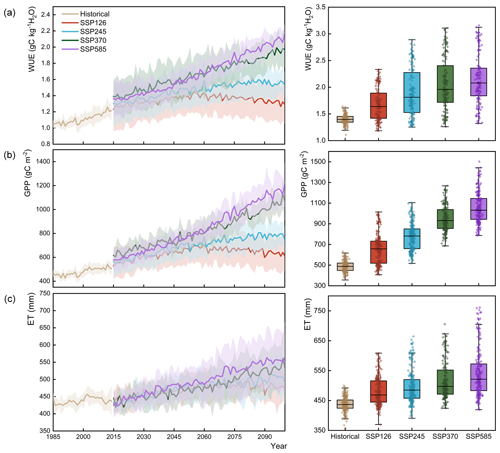
Figure 3Annual averaged time series and box plots during historical-period (1985–2014) and future-period (2070–2099) (a) WUE, (b) GPP, and (c) ET from the CMIP6 multi-model ensemble in the YRB.
The first column in Fig. 4 displays the spatial distribution of annual WUE in the YRB during the historical period (1985–2014) and the future period (2070–2099) under different SSPs. The multi-model average results indicated that during the historical period, the multi-year average WUE in the YRB predominantly exhibited a pattern of higher values in the east and lower values in the west, with higher values in the south and lower values in the north. High WUE values were observed in the upstream areas and downstream coastal areas, while low values appeared in the northwest part of the basin, specifically the Inner Mongolia Plateau. Under different scenarios, the future distribution characteristics of WUE remained similar. As shown in the first column of Fig. 5, there was a predominant upward trend in WUE in the middle-downstream region in the future, and the relative growth increased with increasing carbon emissions. In some areas, the growth exceeded 200 %. However, the Hetao region in the northwest part of the Yellow River consistently experienced a downward trend in WUE under different scenarios, with the greater decline as carbon emissions increased. In the SSP585 scenario, this region experienced a negative growth of close to 20 %. The upstream areas of the YRB showed relatively consistent changes under different scenarios except for a noticeable decrease in the southwestern of the basin, i.e., the Qinghai–Tibet Plateau region, while changes in other regions were less evident.
To further investigate the spatial distribution characteristics of WUE, we plotted the spatial distribution and relative changes in GPP and ET (different columns in Figs. 4 and 5). The spatial distribution characteristics of GPP closely resembled those of WUE (the first and second column in Fig. 4), with the locations of high and low values aligning well. Under different scenarios, the entire YRB experienced an increase in GPP, though the degree varies (the second column in Fig. 5). Overall, there was relatively little change in GPP in the source area of the YRB, while significant growth was observed in the Loess Plateau and the intra-basin areas. The growth in GPP increased sequentially from SSP126 to SSP585. In SSP585, most regions in the basin, including the Loess Plateau and Ningxia Plain, exhibited a 260 % growth in GPP. Extremely low values ET were observed in the Hetao area, with ET increasing in a radial pattern, indicating higher ET values at locations increasingly distant from this region (the third column in Fig. 4). Throughout different periods and scenarios, the minimum values consistently appeared in the northwest part of the basin, specifically the Inner Mongolia Plateau, all below 60 mm. The maximum values occurred along the Qinling (which also serves as the boundary between northern and southern China), where ET exceeds 780 mm. The relative changes in ET in the YRB exhibited the opposite trend, increasing from southeast to northwest (the third column in Fig. 5). In the low-emission scenario (SSP126), ET showed minimal variation within the basin. However, with increasing carbon emissions, the growth in ET became more drastic, especially in the Inner Mongolia Plateau. In SSP585, the growth in this area and its vicinity reached 52 %. In comparison, ET changes in other regions are not significant except for in the source area, where ET also increased.
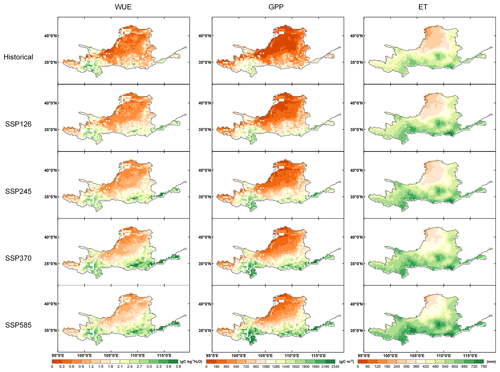
Figure 4Spatial distribution of multi-model average results in the YRB under different scenarios. The three columns represent the multi-year average of WUE (the first column), GPP (the second column), and ET (the third column) during the historical (the first row) period (1985–2014) and future periods (2070–2099) under the SSP126 (the second row), SSP245 (the third row), SSP370 (the fourth row), and SSP585 (the fifth row) scenarios.
4.3 Attribution of WUE changes over the YRB
We constructed a Budyko-type model of water–energy exchange for WUE based on the method outlined in Sect. 3.2. Figure 6a illustrates the Spearman correlation coefficients between the underlying surface parameters of the Budyko model, WUE, and vegetation indices based on observation data. WUE showed a strong positive correlation with LAI and NDVI, with correlation coefficients reaching 0.97 and 0.95, respectively. The correlation with the underlying land surface parameter, m, was also significant (p<0.01), with a coefficient of 0.56. Although the negative correlation between WUE and n was relatively weaker (−0.26), it was still statistically significant (p<0.01). The basin underlying surface parameters (m and n) exhibited good correlations with vegetation indices (LAI and NDVI) at 0.53, 0.65, −0.30, and −0.33, respectively. In general, an increase in LAI and NDVI will cause an increase in m and a decrease in n, consequently resulting in an increase in WUE.
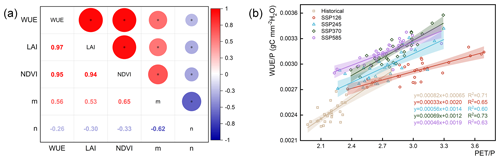
Figure 6Performance of the Budyko model. (a) Cross-correlation coefficients among WUE, Budyko model parameters, and vegetation indices based on observation data (* indicates a significance of p<0.01). (b) Budyko model performance for WUE in the historical period (1985–2014) and under different SSPs (2070–2099). The shading represents the 95 % confidence interval.
Furthermore, we established Budyko models for WUE using the multi-model average data under different periods and scenarios to assess the combined impacts of climate and vegetation patterns on WUE. As depicted in Fig. 6b, the selected linear Budyko models demonstrated good performance across various periods and scenarios. It exhibited the best fits in SSP370 and historical scenarios, with R2 values of 0.73 and 0.71, respectively, while performing relatively less well in SSP245, with an R2 of 0.60. Overall, the models' performance had been satisfactorily validated in the YRB (R2 consistently exceeding 0.60), providing confidence for subsequent attribution analyses.
Based on the established Budyko models, we calculated the changes in WUE and the attribution results for the YRB (Fig. 7a). The results indicated an increase trend in the multi-year average WUE for the basin in the future period (2070–2099) compared to the historical period (1985–2014). Moreover, with intensifying carbon emissions, the increase in WUE became more substantial, with average increases in WUE sequentially from SSP126 to SSP585 by 0.36, 0.54, 0.75, and 0.84 gC kg−1 H2O. SSP585 exhibited the highest growth in WUE, although we observed a slowdown in the increase from SSP370 to SSP585. According to the attribution results, climate change consistently remained the predominant factor influencing WUE changes, especially in SSP126, where almost the entire variation in WUE was attributable to climate change (101.4 %). In SSP126, SSP245, SSP370, and SSP585, the proportions dominated by underlying change were −1.4 %, 14.9 %, 16.9 %, and 22.1 %, respectively. However, we also observed that with increasing carbon emissions, the impacts of underlying surface changes on WUE became more significant. In the low-emission scenario (SSP126), the influence of land surface changes in the basin was minimal, only playing a slightly negative role (−1.4 %). But in SSP245, SSP370, and SSP585, the promoting effects of land surface factors on WUE became more pronounced. In SSP585, nearly a quarter of the WUE growth was attributed to changes in the underlying surface. These phenomena could be explained by the fertilization effect of carbon dioxide. In the SSP126 scenario, atmospheric CO2 concentrations for the future period (2070–2099) were projected to be lower than in the historical period (1985–2014), which could be detrimental to plant growth and especially GPP. Therefore, under the low-emission scenario, changes in the land surface might have a slight impact on WUE. With the increase in carbon emissions, the growth of vegetation was further promoted, and the changes in vegetation productivity due to different carbon emission scenarios might be more pronounced compared to the impacts of climate change. Thus, the role of underlying land surface changes became increasingly important in the variation in WUE.
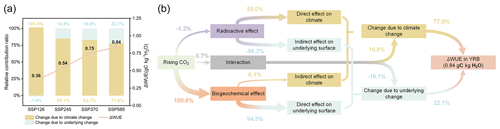
Figure 7Attribution results for WUE changes. (a) Changes in WUE in the YRB under different SSPs along with the relative contributions of climate factors and underlying surface factors. The stacked chart represents the relative contribution rates of climate change and underlying changes to WUE variations under different scenarios. The line graph depicts the average WUE changes (during 1985–2014 and 2070–2099) across the basin under various scenarios. (b) Land–atmosphere decoupling attribution results based on SSP585 and CO2 experiments. The percentages represents the relative contribution rates.
Due to the consistent increase in CO2 concentration in the three CO2 sensitivity experiments at the same rate as in SSP585, we combined the CO2 experiments with the attribution in SSP585 to decouple the direct and secondary effects of CO2-induced radiative and biogeochemical impacts. To ensure the validity of the results, we selected the first 30 years and the subsequent 30 years of the CO2 experiment, with a time span consistent with SSP585. As shown in Fig. 7b, compared to the radiative effect (−1.3 %), the biogeochemical effect of CO2 overwhelmingly dominated the change in WUE (100.6 %). This was understandable as the majority of the effects from the biogeochemical experiment directly acted on land surface (94.5 %) and specifically on the vegetation structure. Climate-induced feedback on changes in vegetation structure also played a positive role in WUE growth (6.1 %). Analyzing the attribution results from the radiative experiment, we found that although the radiative effect had a minimal negative impact on WUE (−1.3 %), this outcome was due to the significant weakening or even surpassing of the indirect response of the land surface vegetation structure to the climate (−56.3 %) compared to the direct radiative forcing effect of CO2 on the climate (55.0 %). The mutual offsetting of these two results led to the radiative effect having a very small negative impact on WUE changes. However, the direct forcing of the radiative effect on climate (55.0 %), the indirect feedback in the biogeochemical experiment (6.1 %), and the interaction between the two experiments (16.8 %) ultimately made climate change the dominant factor (77.9 %) in the growth of the YRB.
4.4 WUE response to drought
In order to investigate how WUE responds to different levels of drought under various scenarios, we calculated the anomaly of WUE at each pixel at different drought levels and averaged the anomaly results across different GCMs. To obtain a sufficient number of event samples at the grid scale and derive more universally applicable conclusions, we focused only on the moderate–severe drought (−1.6 < TWSA-DSI ≤ −0.8) and extreme–exceptional drought (TWSA-DSI ≤ −1.6) categories by treating all other conditions as non-drought situations (TWSA-DSI > −0.8) (Yin et al., 2022). In the analysis of future drought responses, particular attention was given to the potential influence of long-term trends on the calculated values of WUE and TWSA. To mitigate this effect, we calculated the mean and standard deviation of WUE and TWSA for the same time spans separately to get the WUE anomaly and TWSA-DSI, which ensures that our analysis focuses on the specific variations and conditions within each period. As shown in Fig. 8 (the first and second columns), in most regions of YRB, the response of WUE to drought can be divided into two stages: an increase in WUE during moderate–severe drought and a decrease in WUE during extreme–exceptional drought. The area percentages of the two-stage model in different periods and scenarios are 98.0 % in historical, 96.8 % in SSP126, 97.6 % in SSP245, 97.0 % in SSP370, and 99.2 % in SSP585. The spatial characteristics of WUE response patterns were also similar across different periods and scenarios.
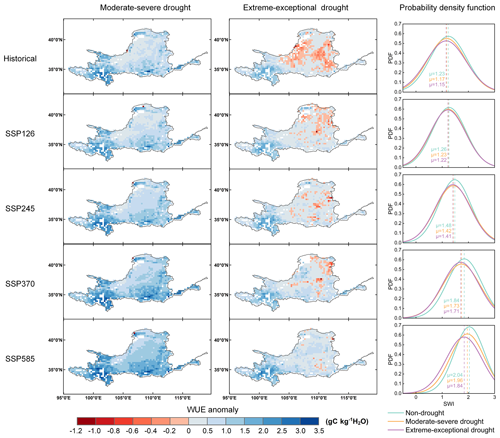
Figure 8Responses of WUE to different levels of drought. The first column shows the anomalies of WUE during moderate–severe drought. The second column shows the anomalies of WUE during extreme–exceptional drought. The third column illustrates the conditional probability function of SWI under different drought levels.
In the historical period (1985–2014), WUE generally increased during moderate–severe drought, with a widespread increase, and only a few areas (specifically, two pixels in the Ningxia Plain) showed a decrease. The middle reaches of the YRB showed a relatively small increase, while more significant increases were observed in the upstream source area and downstream estuary. As drought intensified to extreme–exceptional levels, there was a large-scale decrease in WUE across the basin. The areas with increased WUE compared to the previous stage also experienced a decline in this stage. Similar to moderate–severe drought, the middle reaches of the YRB saw a significant decrease in WUE, while the source and downstream areas showed a slight increase.
Looking into the future (2070–2099), WUE's positive response to drought became more prominent under all SSPs. This was evident in the significantly higher increase in WUE during moderate–severe drought compared to the historical period. Moreover, the areas with a decrease in WUE during extreme–exceptional drought became smaller. With increasing carbon emissions, the positive response of WUE to drought became more pronounced. This was due to the fact that CO2 not only promoted the growth of the plant, but also reduced stomatal conductance, allowing water to be better retained in the body during dry periods. In SSP126, the WUE response pattern to drought was similar to that of the historical period. However, in SSP585, almost all regions within the basin showed a positive response of WUE to moderate–severe drought, with WUE anomalies reaching around 1.0 gC kg−1H2O. Even during extreme–exceptional drought, there were very few areas where WUE decreased across the basin.
Figure 8 (the third column) quantitatively described the conditional probability distribution of SWI under different dry and wet conditions. Across different periods and SSPs, the mean (μ) of SWI under extreme–exceptional drought conditions was lower than that under moderate–severe drought conditions. Combining the previously mentioned two-stage response characteristics, this is understandable because as drought intensified, the WUE response in the basin tended to shift towards negative values. However, we also observe that μ under non-drought conditions was generally higher than that under drought conditions. A reasonable explanation was that, compared to the wet season, the basin tended to have lower WUE during seasons or months prone to drought. Therefore, even with a positive response during drought, the value of μ remained lower than in non-drought periods. Additionally, compared to the historical period, the future μ under different scenarios showed varying degrees of growth. This growth was less pronounced in the low-emission scenario (SSP126) but became more significant with increasing carbon emissions, reaching its maximum in SSP585. This was consistent with the results in Sect. 4.2.
Furthermore, we observed that with the increase in carbon emissions, the gap between μ under non-drought conditions and that under drought conditions became more apparent. The difference in μ (Δμ) represents the difference in the mean values of WUE across varying intensities of drought within the same period. This measure, utilized to analyze the differential impacts of drought levels, also became larger (from SSP126 to SSP585, Δμ is 0.01, 0.01, 0.02, and 0.12, respectively). This implied that a high-carbon-emission scenario would exacerbate the numerical differences in WUE between dry and wet seasons in the YRB while also amplifying the response differences in WUE to different drought levels. Moreover, in SSP585, these differences were significantly greater than in other scenarios.
5.1 Spatiotemporal variation characteristics of WUE in the YRB
Unlike many studies that focus solely on the historical trends of WUE (Kim et al., 2021; Huang et al., 2017; Lu and Zhuang, 2010), this research systematically projected the development trends of WUE, GPP, and ET in the YRB for both historical and future periods. GPP, as a key variable in calculating WUE, exhibits significant differences among various GPP datasets, such as NIRv, GIMMS, FLUXCOM (based on upscaled eddy covariance flux tower measurements), LUE (based on the light-use efficiency model), and TRENDY (a recent model intercomparison project) (S. Wang et al., 2021). Compared to NIRv GPP data, GIMMS ignores the impact of CO2 on LUE (De Kauwe et al., 2016), and FLUXCOM GPP lacks consideration of the CO2 fertilization effect (Anav et al., 2015), thus underestimating the interannual sensitivity of GPP to climate change. A recent study has improved the traditional LUE method using eddy covariance, which produced a revised global GPP product (EC-LUE GPP) (Zheng et al., 2020). However, satellite-based APAR (absorbed photosynthetic radiation) data used may lead to inaccuracies in some regions due to saturation (Zhang et al., 2020). Furthermore, the GPP trends in TRENDY vary significantly between different models and offer lower spatial resolution (Zheng et al., 2020). Considering all these factors, we opted for the advanced GPP dataset based on NIRv with high temporal and spatial resolutions. Our results also indicate that these data are reasonable and accurate. During the historical period, the average results from multiple GCMs closely align with previous findings (H. Li et al., 2023; Sun et al., 2022; Zhao et al., 2022b), which indirectly demonstrated the excellent performance of bias correction done in this study. According to Fig. 3, WUE, GPP, and ET were projected to increase to varying degrees in the future. The growth rate was higher under high-emission scenarios, while under the SSP126 scenario, all three showed an initial increase followed by a decline. F. Li et al. (2023) suggested that global WUE had been approaching saturation in recent years and may maintain the saturated state in the future. According to our results, under SSP245 (which is also most consistent with the Chinese context), WUE in the YRB is expected to reach a relatively stable “saturated” state by the end of this century, with a value of around 1.55 gC kg−1H2O. The spatial distribution of WUE in the YRB can be summarized as “higher in the south and lower in the north, higher in the east and lower in the west” (Fig. 4). This spatial pattern and the regions where extreme values occur align well with the study of Sun et al. (2022). We also observed that the future spatial distribution of WUE was very similar to GPP (Fig. 4) (Liu et al., 2020). Combining these results with those in Fig. 2, despite the general increase in ET, WUE in most regions of the YRB is still on the rise. Therefore, we conclude that in most areas of the YRB, GPP's relative contribution to WUE change is higher than that of ET. In other words, GPP dominates the future changes in WUE in the basin, which is in good agreement with other scholars' findings (Naeem et al., 2023; Tan et al., 2023). However, ET remains a primary driving factor for WUE in arid regions (Yang et al., 2016), a point especially evident in the Yellow River source region. In the upstream source region of the YRB, ET shows an increasing trend under different SSPs, while GPP's growth is relatively slow. This asymmetrical growth between GPP and ET has led to a decline in WUE in the source region.
5.2 Interpretation of Budyko model parameters and attribution results
In this study, we employed the linear Budyko model to describe the relationship between WUE and the water–energy nexus in the YRB. In Eq. (3), m and n are the underlying surface parameters of the basin. To validate the rationality of these parameters, we conducted a correlation analysis between m, n, and vegetation indices as shown in Fig. 6a. The results indicate good correlations between underlying surface parameters and LAI, NDVI. This suggests that the chosen parameters can effectively reflect the underlying surface vegetation conditions closely related to WUE, further validating the physical significance of the established Budyko model. Additionally, we demonstrated a strong positive correlation between WUE and both LAI and NDVI due to the significant enhancement of the region's GPP by increased vegetation factors. In the mostly arid areas of the YRB, the impacts of these vegetation changes on ET is minimal (Liu et al., 2020; Yang et al., 2022). Moreover, our results also support the viewpoint that there is a positive correlation between WUE and m (Fang et al., 2020). Previous studies have shown that WUE is closely related to precipitation, vapor pressure deficit, temperature, wind speed, humidity, et cetera (F. Li et al., 2023; Lin et al., 2020; Liu et al., 2020; Yang et al., 2022). This study employed the Penman–Monteith equation to calculate PET, incorporating the aforementioned factors; hence, P and the calculated PET can be selected as the climatic attribution factors for WUE.
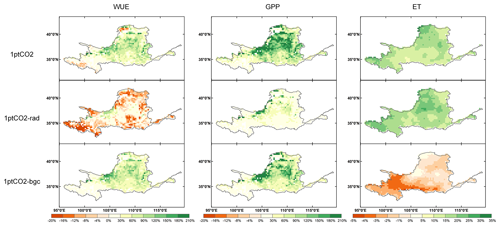
Figure 9Spatial distribution of the relative changes in WUE (the first column), GPP (the second column), and ET (the third column) across different CO2 sensitivity experiments.
To further elucidate the attribution results shown in Fig. 7b, we plotted the relative changes in WUE, GPP, and ET across different CO2 sensitivity experiments (Fig. 9). Over the same time span, the spatial distribution pattern of relative changes in WUE, GPP, and ET within the 1ptCO2 scenario aligns substantially with that of the SSP585 scenario. In the 1ptCO2-rad experiment, WUE showed a declining trend in the peripheral areas of the YRB but an increase in the central region, thus presenting a slight negative contribution overall (−1.3 %) as depicted in Fig. 7b. Conversely, in the 1ptCO2-bgc experiment, WUE exhibited a comprehensive upward trend across the basin, serving as the primary driving factor for the increase in WUE observed in the comprehensive experiment (1ptCO2) (100.6 %). To explain the difference in WUE performance, we further analyzed the relative changes in GPP and ET across different CO2 sensitivity experiments. In the 1ptCO2-rad experiment, due to the absence of CO2's direct biogeochemical effect on plants, the change in GPP was not as sharp as that in the 1ptCO2-bgc. Moreover, the radiative effects of CO2 increase atmospheric evaporative demand, i.e., PET (Milly and Dunne, 2016, 2017), which is the most likely reason for the widespread increase in ET and the broad decline in WUE within the YRB. However, combining the analysis with Fig. 7b, such changes encompass CO2's direct impact on climate and the indirect feedback from the underlying surface, with the latter possibly exerting a greater influence on ET and GPP variations. However, due to the minimal numerical changes in variables in 1ptCO2-rad, the uncertainty is also higher. Under the 1ptCO2-bgc experiment conditions, owing to the fertilization effect of CO2, plant growth was stimulated (He et al., 2023; Zhao et al., 2022a), resulting in a significant widespread increase in GPP across the basin. The increased plant biomass also led to a rise in ET (Mankin et al., 2019; Piao et al., 2007; Zhan et al., 2022), while the elevated CO2 concentrations further reduced plant stomatal conductance, potentially diminishing transpiration (Guerrieri et al., 2019; F. Li et al., 2023; Mathias and Thomas, 2021; Zhang et al., 2022). We found that with the combined effects of these factors, changes in ET within the YRB are minimal, generally ranging between −5 % and 5 % in 1ptCO2-bgc experiment. Consequently, in 1ptCO2-bgc, WUE across various regions of the basin exhibits varying degrees of increase.
Table 3Model parameter settings for simulating stomatal conductance in the GCMs.
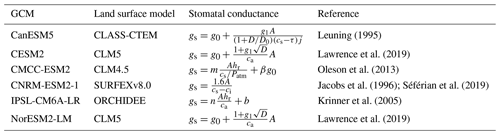
CLASS-CTEM: coupled Canadian Land Surface Scheme and the Canadian Terrestrial Ecosystem Model. CLM5: Community Land Model version 5. CLM4.5: Community Land Model version 4.5. SURFEXv8.0: Surface Externalisée version 8.0. ORCHIDEE: Organizing Carbon and Hydrology in Dynamic Ecosystems Land Surface Model. gs is the stomatal conductance; g0 is the minimum stomatal conductance as assimilation rate reaches zero; g1, D0, b, m, n, and β are the empirical coefficients; A is the net photosynthesis rate; D is the humidity deficit; cs is the CO2 concentration at the leaf surface; τ is the CO2 compensation point; ca is the atmospheric CO2 concentration; hr is the relative air humidity; Patm is the atmospheric pressure; and ci is the CO2 concentration in the intercellular spaces.
5.3 Two-stage response pattern of WUE to drought
WUE can reflect the impacts of local water availability on the ecosystem's carbon sequestration capacity to a certain extent and can serve as one of the reference indicators for measuring ecosystem resilience (Ponce-Campos et al., 2013). Therefore, we considered the response of WUE in the YRB to drought stress. Many scholars have suggested that WUE will continue to increase or decrease under drought conditions in most regions of the world (Liu et al., 2023; Ponce-Campos et al., 2013; Xie et al., 2016). However, these studies have not considered the differential responses of WUE to various drought intensities. Our results (Fig. 8) illustrate that WUE exhibits a two-stage response pattern during drought; i.e., it increases in moderate–severe drought but begins to decrease as the drought intensifies. This finding is similar to the phenomenon observed by Lu and Zhuang (2010) over most of the US. This means that under the moderate–severe drought condition, plants physiologically adapt to water stress, thereby maintaining a certain level of GPP under limited water conditions (Vicente-Serrano et al., 2010). However, as drought intensifies, plant physiology is damaged, thereby reducing WUE, which is consistent with findings by Yang et al. (2021) in semi-arid/semi-humid regions of the world. As mentioned before, the increase in atmospheric CO2 concentration directly leads to a reduction in plant leaf stomatal conductance. In Table 3, it is evident that although different GCMs employ various models for stomatal conductance, the physical implications across these models consistently align with this principle. It is the change in stomatal conductance that is suggested to help alleviate the stress of drought on plants (De Kauwe et al., 2021; Leakey et al., 2006; Swann, 2018). Additionally, we note that with the intensification of carbon emission scenarios (from SSP126 to SSP585), the future response of WUE in the basin to drought becomes more positive, indicating an enhanced adaptability of plants to water stress. The results also indirectly prove that plants are more likely to benefit from drought stress in the future (De Kauwe et al., 2021).
WUE, due to its unique connotation, has become an important indicator for ecosystem health, reflecting the tradeoff between regional carbon assimilation and water loss. In this study, after applying trend-preserving bias correction to CMIP6 data informed by satellite-monitored reanalysis datasets, we investigated spatiotemporal variations in WUE, GPP, and ET spanning from 1985 to 2100. Our construction of a Budyko model, underpinned by GCMs, offers a robust framework for dissecting the intricacies of WUE evolution, revealing a predominantly climate-driven narrative, albeit with an escalating influence from underlying surface modifications under severe carbon emission trajectories.
The spatial dynamics of WUE across the YRB have historically been characterized by higher values in the east and the south contrasted with lower values in the west and the north. Looking forward, our analysis projects a universal uptick in WUE under varying future climate scenarios, with increases ranging from 0.36 to 0.84 gC kg−1 H2O across SSP126 to SSP585. The constructed Budyko model displays strong performance in different periods and under different scenarios (R2= 0.60–0.73). The land–atmosphere attribution framework underscores the pivotal role of climate change as the primary driver of WUE alterations, overshadowing underlying surface changes. Nevertheless, the impact of biogeochemical CO2 effects emerges as a dominant force, illustrating the complex interplay of carbon dioxide in shaping WUE trajectories. Besides, our findings delineate a distinctive two-stage response pattern of WUE to drought conditions in the YRB and forecast enhanced ecosystem resilience to drought stress in future scenarios, particularly under heightened carbon emissions.
While this study advances our understanding of ecohydrological processes in the YRB, it is not without its constraints. The reliance on reanalysis datasets, despite their robust performance, introduces a layer of uncertainty when compared against actual measurements. Moreover, the complexity of vegetation responses to elevated CO2 levels coupled with the variability in dynamic global vegetation models (DGVMs) across different GCMs calls for a deeper exploration into the quantification and assessment of uncertainties surrounding CO2's impact on WUE.
By charting the interdependencies of CO2 dynamics, climate change, and land surface alterations, this study concerns the future of ecohydrological sustainability in the YRB, inviting further inquiry into the resilience of terrestrial ecosystems under anthropogenic stress.
The precipitation and potential evapotranspiration dataset are available at https://doi.org/10.5281/zenodo.3114194 (Peng, 2019) and https://www.tpdc.ac.cn/en/data/8b11da09-1a40-4014-bd3d-2b86e6dccad4/ (Peng, 2022). The evapotranspiration dataset is available at https://doi.org/10.11888/AtmosPhys.tpe.249493.file (Ma et al., 2019b). The GPP dataset is available at https://doi.org/10.6084/m9.figshare.12981977.v2 (Wang and Zhang, 2020). The LAI data are available in Cao et al. (2023b). The NDVI data are available at https://doi.org/10.3334/ORNLDAAC/2187 (Pinzon et al., 2013). The TWSA data are available at https://doi.org/10.6084/m9.figshare.7670849 (Humphrey and Gudmundsson, 2019b). The future data were acquired from CMIP6 (https://pcmdi.llnl.gov/CMIP6/, last access: 1 February 2024).
Conceptualization: SC, YPX, and YG. Funding acquisition: YPX. Methodology: SC, YPX, and YG. Project administration: YPX. Writing-original draft: SC. Supervisor: YPX. Writing-review and editing: YPX, YG, and LW.
At least one of the (co-)authors is a member of the editorial board of Hydrology and Earth System Sciences. The peer-review process was guided by an independent editor, and the authors also have no other competing interests to declare.
Publisher's note: Copernicus Publications remains neutral with regard to jurisdictional claims made in the text, published maps, institutional affiliations, or any other geographical representation in this paper. While Copernicus Publications makes every effort to include appropriate place names, the final responsibility lies with the authors.
We are grateful to the China Meteorological Administration, Yellow River Hydrological Bureau, and National Tibetan Plateau Data Center for providing meteorological and hydrological data used in this study.
This research has been supported by the National Key Research and Development Program of China (grant no. 2021YFC3201105) and the National Natural Science Foundation of China (grant no. 52009121).
This paper was edited by Fuqiang Tian and reviewed by two anonymous referees.
A, G., Velicogna, I., Kimball, J. S., Du, J., Kim, Y., Colliander, A., and Njoku, E.: Satellite-observed changes in vegetation sensitivities to surface soil moisture and total water storage variations since the 2011 Texas drought, Environ. Res. Lett., 12, 054006, https://doi.org/10.1088/1748-9326/aa6965, 2017.
Allan, R., Pereira, L., and Smith, M.: Crop evapotranspiration: guidelines for computing crop water requirements, FAO Irrigation and Drainage Paper No. 56, FAO, Rome, Italy, 1998.
Anav, A., Friedlingstein, P., Beer, C., Ciais, P., Harper, A., Jones, C., Murray-Tortarolo, G., Papale, D., Parazoo, N. C., Peylin, P., Piao, S., Sitch, S., Viovy, N., Wiltshire, A., and Zhao, M.: Spatiotemporal patterns of terrestrial gross primary production: A review, Rev. Geophys., 53, 785–818, https://doi.org/10.1002/2015RG000483, 2015
Berg, A., Sheffield, J., and Milly, P. C. D.: Divergent surface and total soil moisture projections under global warming, Geophys. Res. Lett., 44, 236–244, https://doi.org/10.1002/2016GL071921, 2017.
Bintanja, R. and Andry, O.: Towards a rain-dominated Arctic, Nat. Clim. Change, 7, 263–267, https://doi.org/10.1038/nclimate3240, 2017.
Budyko, M. I.: Climate and Life, 508 pp., Academic Press, New York, ISBN 0121394506, 1974.
Cai, X., Li, L., Fisher, J. B., Zeng, Z., Zhou, S., Tan, X., Liu, B., and Chen, X.: The responses of ecosystem water use efficiency to CO2, nitrogen deposition, and climatic drivers across China, J. Hydrol., 622, 129696, https://doi.org/10.1016/j.jhydrol.2023.129696, 2023.
Cao, S., Li, M., Zhu, Z., Wang, Z., Zha, J., Zhao, W., Duanmu, Z., Chen, J., Zheng, Y., Chen, Y., Myneni, R. B., and Piao, S.: Spatiotemporally consistent global dataset of the GIMMS leaf area index (GIMMS LAI4g) from 1982 to 2020, Earth Syst. Sci. Data, 15, 4877–4899, https://doi.org/10.5194/essd-15-4877-2023, 2023a.
Cao, S., Li, M., Zhu, Z., Wang, Z., Zha, J., Zhao, W., Duanmu, Z., Chen, J., Zheng, Y., Chen, Y., Myneni, R. B., and Piao, S.: Spatiotemporally consistent global dataset of the GIMMS Leaf Area Index (GIMMS LAI4g) from 1982 to 2020 (V1.2), Zenodo [data set], https://doi.org/10.5281/zenodo.8281930, 2023b.
Chen, Z., Wang, W., Forzieri, G., and Cescatti, A.: Transition from positive to negative indirect CO2 effects on the vegetation carbon uptake, Nat. Commun., 15, 1500, https://doi.org/10.1038/s41467-024-45957-x, 2024.
Cheng, L., Xu, Z., Wang, D., and Cai, X.: Assessing interannual variability of evapotranspiration at the catchment scale using satellite-based evapotranspiration data sets, Water Resour. Res., 47, W09509, https://doi.org/10.1029/2011WR010636, 2011.
Choudhury, B.: Evaluation of an empirical equation for annual evaporation using field observations and results from a biophysical model, J. Hydrol., 216, 99–110, https://doi.org/10.1016/S0022-1694(98)00293-5, 1999.
De Kauwe, M. G., Keenan, T. F., Medlyn, B. E., Prentice, I. C., and Terrer, C.: Satellite based estimates underestimate the effect of CO2 fertilization on net primary productivity, Nat. Clim. Change, 6, 892–893, https://doi.org/10.1038/nclimate3105, 2016.
De Kauwe, M. G., Medlyn, B. E., and Tissue, D. T.: To what extent can rising [CO2] ameliorate plant drought stress?, New Phytol., 231, 2118–2124, https://doi.org/10.1111/nph.17540, 2021.
Ding, Y. and Peng, S.: Spatiotemporal Trends and Attribution of Drought across China from 1901–2100, Sustainability, 12, 477, https://doi.org/10.3390/su12020477, 2020.
Ding, Y. and Peng, S.: Spatiotemporal change and attribution of potential evapotranspiration over China from 1901 to 2100, Theor. Appl. Climatol., 145, 79–94, https://doi.org/10.1007/s00704-021-03625-w, 2021.
Du, J., Kimball, J. S., Velicogna, I., Zhao, M., Jones, L. A., Watts, J. D., and Kim, Y.: Multicomponent Satellite Assessment of Drought Severity in the Contiguous United States From 2002 to 2017 Using AMSR-E and AMSR2, Water Resour. Res., 55, 5394–5412, https://doi.org/10.1029/2018WR024633, 2019.
Fang, Q., Wang, G., Liu, T., Xue, B., Sun, W., and Shrestha, S.: Unraveling the sensitivity and nonlinear response of water use efficiency to the water–energy balance and underlying surface condition in a semiarid basin, Sci. Total Environ., 699, 134405, https://doi.org/10.1016/j.scitotenv.2019.134405, 2020.
Fathi, M. M., Awadallah, A. G., Abdelbaki, A. M., and Haggag, M.: A new Budyko framework extension using time series SARIMAX model, J. Hydrol., 570, 827–838, https://doi.org/10.1016/j.jhydrol.2019.01.037, 2019.
Feng, H., Kang, P., Deng, Z., Zhao, W., Hua, M., Zhu, X., and Wang, Z.: The impact of climate change and human activities to vegetation carbon sequestration variation in Sichuan and Chongqing, Environ. Res., 238, 117138, https://doi.org/10.1016/j.envres.2023.117138, 2023.
Feng, S., Hao, Z., Zhang, X., and Hao, F.: Probabilistic evaluation of the impact of compound dry-hot events on global maize yields, Sci. Total Environ., 689, 1228–1234, https://doi.org/10.1016/j.scitotenv.2019.06.373, 2019.
Fowler, M. D., Kooperman, G. J., Randerson, J. T., and Pritchard, M. S.: The effect of plant physiological responses to rising CO2 on global streamflow, Nat. Clim. Change, 9, 873–879, https://doi.org/10.1038/s41558-019-0602-x, 2019.
Gu, H., Xu, Y.-P., Liu, L., Xie, J., Wang, L., Pan, S., and Guo, Y.: Seasonal catchment memory of high mountain rivers in the Tibetan Plateau, Nat. Commun., 14, 3173, https://doi.org/10.1038/s41467-023-38966-9, 2023.
Gu, L., Yin, J., Gentine, P., Wang, H.-M., Slater, L. J., Sullivan, S. C., Chen, J., Zscheischler, J., and Guo, S.: Large anomalies in future extreme precipitation sensitivity driven by atmospheric dynamics, Nat. Commun., 14, 3197, https://doi.org/10.1038/s41467-023-39039-7, 2023.
Guerrieri, R., Belmecheri, S., Ollinger, S. V., Asbjornsen, H., Jennings, K., Xiao, J., Stocker, B. D., Martin, M., Hollinger, D. Y., Bracho-Garrillo, R., Clark, K., Dore, S., Kolb, T., Munger, J. W., Novick, K., and Richardson, A. D.: Disentangling the role of photosynthesis and stomatal conductance on rising forest water-use efficiency, P. Natl. Acad. Sci. USA, 116, 16909–16914, https://doi.org/10.1073/pnas.1905912116, 2019.
Guo, Y., Yu, X., Xu, Y.-P., Wang, G., Xie, J., and Gu, H.: A comparative assessment of CMIP5 and CMIP6 in hydrological responses of the Yellow River Basin, China, Hydrol. Res., 53, 867–891, https://doi.org/10.2166/nh.2022.001, 2022.
He, Y., Liu, Y., Lei, L., Terrer, C., Huntingford, C., Peñuelas, J., Xu, H., and Piao, S.: CO2 fertilization contributed more than half of the observed forest biomass increase in northern extra-tropical land, Glob. Change Biol., 29, 4313–4326, https://doi.org/10.1111/gcb.16806, 2023.
Huang, L., He, B., Han, L., Liu, J., Wang, H., and Chen, Z.: A global examination of the response of ecosystem water-use efficiency to drought based on MODIS data, Sci. Total Environ., 601–602, 1097–1107, https://doi.org/10.1016/j.scitotenv.2017.05.084, 2017.
Huang, S., Chang, J., Leng, G., and Huang, Q.: Integrated index for drought assessment based on variable fuzzy set theory: A case study in the Yellow River basin, China, J. Hydrol., 527, 608–618, https://doi.org/10.1016/j.jhydrol.2015.05.032, 2015.
Humphrey, V. and Gudmundsson, L.: GRACE-REC: a reconstruction of climate-driven water storage changes over the last century, Earth Syst. Sci. Data, 11, 1153–1170, https://doi.org/10.5194/essd-11-1153-2019, 2019.
Humphrey, V. and Gudmundsson, L.: GRACE-REC: a reconstruction of climate-driven water storage changes over the last century, figshare [data set], https://doi.org/10.6084/m9.figshare.7670849, 2019.
Jacobs, C. M. J., van den Hurk, B. M. M., and de Bruin, H. A. R.: Stomatal behaviour and photosynthetic rate of unstressed grapevines in semi-arid conditions, Agr. Forest Meteorol., 80, 111–134, 1996.
Keenan, T. F., Hollinger, D. Y., Bohrer, G., Dragoni, D., Munger, J. W., Schmid, H. P., and Richardson, A. D.: Increase in forest water-use efficiency as atmospheric carbon dioxide concentrations rise, Nature, 499, 324–327, https://doi.org/10.1038/nature12291, 2013.
Kim, D., Baik, J., Umair, M., and Choi, M.: Water use efficiency in terrestrial ecosystem over East Asia: Effects of climate regimes and land cover types, Sci. Total Environ., 773, 145519, https://doi.org/10.1016/j.scitotenv.2021.145519, 2021.
Krinner, G., Viovy, N., De Noblet-Ducoudré, N., Ogée, J., Polcher, J., Friedlingstein, P., Ciais, P., Sitch, S., and Prentice, I. C.: A dynamic global vegetation model for studies of the coupled atmosphere-biosphere system, Global Biogeochem. Cy., 19, 2003GB002199, https://doi.org/10.1029/2003GB002199, 2005.
Lange, S.: Trend-preserving bias adjustment and statistical downscaling with ISIMIP3BASD (v1.0), Geosci. Model Dev., 12, 3055–3070, https://doi.org/10.5194/gmd-12-3055-2019, 2019.
Lawrence, D. M., Fisher, R. A., Koven, C. D., Oleson, K. W., Swenson, S. C., Bonan, G., Collier, N., Ghimire, B., Van Kampenhout, L., Kennedy, D., Kluzek, E., Lawrence, P. J., Li, F., Li, H., Lombardozzi, D., Riley, W. J., Sacks, W. J., Shi, M., Vertenstein, M., Wieder, W. R., Xu, C., Ali, A. A., Badger, A. M., Bisht, G., Van Den Broeke, M., Brunke, M. A., Burns, S. P., Buzan, J., Clark, M., Craig, A., Dahlin, K., Drewniak, B., Fisher, J. B., Flanner, M., Fox, A. M., Gentine, P., Hoffman, F., Keppel-Aleks, G., Knox, R., Kumar, S., Lenaerts, J., Leung, L. R., Lipscomb, W. H., Lu, Y., Pandey, A., Pelletier, J. D., Perket, J., Randerson, J. T., Ricciuto, D. M., Sanderson, B. M., Slater, A., Subin, Z. M., Tang, J., Thomas, R. Q., Val Martin, M., and Zeng, X.: The Community Land Model Version 5: Description of New Features, Benchmarking, and Impact of Forcing Uncertainty, J. Adv. Model Earth Sy., 11, 4245–4287, https://doi.org/10.1029/2018MS001583, 2019.
Leakey, A. D. B., Uribelarrea, M., Ainsworth, E. A., Naidu, S. L., Rogers, A., Ort, D. R., and Long, S. P.: Photosynthesis, Productivity, and Yield of Maize Are Not Affected by Open-Air Elevation of CO2 Concentration in the Absence of Drought, Plant Physiol., 140, 779–790, https://doi.org/10.1104/pp.105.073957, 2006.
Leuning, R.: A critical appraisal of a combined stomatal-photosynthesis model for C3 plants, Plant Cell Environ., 18, 339–355, https://doi.org/10.1111/j.1365-3040.1995.tb00370.x, 1995.
Li, F., Xiao, J., Chen, J., Ballantyne, A., Jin, K., Li, B., Abraha, M., and John, R.: Global water use efficiency saturation due to increased vapor pressure deficit, Science, 381, 672–677, https://doi.org/10.1126/science.adf5041, 2023.
Li, H., He, Y., Zhang, L., Cao, S., and Sun, Q.: Spatiotemporal changes of Gross Primary Production in the Yellow River Basin of China under the influence of climate-driven and human-activity, Global Ecol. Conserv., 46, e02550, https://doi.org/10.1016/j.gecco.2023.e02550, 2023.
Li, P., Tedersoo, L., Crowther, T. W., Dumbrell, A. J., Dini-Andreote, F., Bahram, M., Kuang, L., Li, T., Wu, M., Jiang, Y., Luan, L., Saleem, M., De Vries, F. T., Li, Z., Wang, B., and Jiang, J.: Fossil-fuel-dependent scenarios could lead to a significant decline of global plant-beneficial bacteria abundance in soils by 2100, Nature Food, 4, 996–1006, https://doi.org/10.1038/s43016-023-00869-9, 2023.
Lin, S., Wang, G., Hu, Z., Huang, K., Sun, J., and Sun, X.: Spatiotemporal Variability and Driving Factors of Tibetan Plateau Water Use Efficiency, J. Geophys. Res.-Atmos., 125, e2020JD032642, https://doi.org/10.1029/2020JD032642, 2020.
Liu, X., Feng, X., and Fu, B.: Changes in global terrestrial ecosystem water use efficiency are closely related to soil moisture, Sci. Total Environ., 698, 134165, https://doi.org/10.1016/j.scitotenv.2019.134165, 2020.
Liu, Y., Ding, Z., Chen, Y., Yan, F., Yu, P., Man, W., Liu, M., Li, H., and Tang, X.: Restored vegetation is more resistant to extreme drought events than natural vegetation in Southwest China, Sci. Total Environ., 866, 161250, https://doi.org/10.1016/j.scitotenv.2022.161250, 2023.
Lu, X. and Zhuang, Q.: Evaluating evapotranspiration and water-use efficiency of terrestrial ecosystems in the conterminous United States using MODIS and AmeriFlux data, Remote Sens. Environ., 114, 1924–1939, https://doi.org/10.1016/j.rse.2010.04.001, 2010.
Ma, N. and Szilagyi, J.: The CR of Evaporation: A Calibration-Free Diagnostic and Benchmarking Tool for Large-Scale Terrestrial Evapotranspiration Modeling, Water Resour. Res., 55, 7246–7274, https://doi.org/10.1029/2019WR024867, 2019.
Ma, N., Szilagyi, J., Zhang, Y., and Liu, W.: Complementary-Relationship-Based Modeling of Terrestrial Evapotranspiration Across China During 1982–2012: Validations and Spatiotemporal Analyses, J. Geophys. Res.-Atmos., 124, 4326–4351, https://doi.org/10.1029/2018JD029850, 2019a.
Ma, N., Jozsef, S., Zhang, Y., and Liu, W.: Terrestrial evapotranspiration dataset across China (1982–2017), National Tibetan Plateau/Third Pole Environment Data Center [data set], https://doi.org/10.11888/AtmosPhys.tpe.249493.file, 2019b.
Mankin, J. S., Seager, R., Smerdon, J. E., Cook, B. I., and Williams, A. P.: Mid-latitude freshwater availability reduced by projected vegetation responses to climate change, Nat. Geosci., 12, 983–988, https://doi.org/10.1038/s41561-019-0480-x, 2019.
Mathias, J. M. and Thomas, R. B.: Global tree intrinsic water use efficiency is enhanced by increased atmospheric CO2 and modulated by climate and plant functional types, P. Natl. Acad. Sci. USA, 118, e2014286118, https://doi.org/10.1073/pnas.2014286118, 2021.
Milly, P. C. D. and Dunne, K. A.: Potential evapotranspiration and continental drying, Nat. Clim. Change, 6, 946–949, https://doi.org/10.1038/nclimate3046, 2016.
Milly, P. C. D. and Dunne, K. A.: A Hydrologic Drying Bias in Water-Resource Impact Analyses of Anthropogenic Climate Change, J. Am. Water Resour. As., 53, 822–838, https://doi.org/10.1111/1752-1688.12538, 2017.
Naeem, S., Zhang, Y., Zhang, X., Rehman, A. U., Tang, Z., Xu, Z., Li, C., and Azeem, T.: Recent change in ecosystem water use efficiency in China mainly dominated by vegetation greening and increased CO2, Remote Sens. Environ., 298, 113811, https://doi.org/10.1016/j.rse.2023.113811, 2023.
Ning, T., Zhou, S., Chang, F., Shen, H., Li, Z., and Liu, W.: Interaction of vegetation, climate and topography on evapotranspiration modelling at different time scales within the Budyko framework, Agr. Forest Meteorol., 275, 59–68, https://doi.org/10.1016/j.agrformet.2019.05.001, 2019.
Oleson, K. W., Lawrence, D. M., Bonan, G. B., Drewniak, B., Huang, M., Koven, C. D., Levis, S., Li, F., Riley, W. J., Subin, Z. M., Swenson, S. C., Thornton, P. E., Bozbiyik, A., Fisher, R., Kluzek, E., Lamarque, J.-F., Lawrence, P. J., Leung, L. R., Lipscomb, W., Muszala, S., Ricciuto, D. M., Sacks, W., Sun, Y., Tang, J., and Yang, Z.-L.: Technical Description of version 4.5 of the Community Land Model (CLM). Ncar Technical Note NCAR/TN-503+STR, National Center for Atmospheric Research, Boulder, CO, 422 pp., https://doi.org/10.5065/D6RR1W7M, 2013.
Peng, S.: High-spatial-resolution monthly precipitation dataset over China during 1901–2017, Zenodo [data set], https://doi.org/10.5281/zenodo.3114194, 2019.
Peng, S.: 1-km monthly potential evapotranspiration dataset for China (1901–2023) National Tibetan Plateau/Third Pole Environment Data Center [data set], https://www.tpdc.ac.cn/en/data/8b11da09-1a40-4014-bd3d-2b86e6dccad4/, 2022.
Peng, D., Lyu, J., Song, Z., Huang, S., Zhang, P., Gao, J., and Zhang, Y.: Mercury budgets in the suspended particulate matters of the Yangtze River, Water Res., 243, 120390, https://doi.org/10.1016/j.watres.2023.120390, 2023.
Piao, S., Friedlingstein, P., Ciais, P., De Noblet-Ducoudré, N., Labat, D., and Zaehle, S.: Changes in climate and land use have a larger direct impact than rising CO2 on global river runoff trends, P. Natl. Acad. Sci. USA, 104, 15242–15247, https://doi.org/10.1073/pnas.0707213104, 2007.
Pinzon, J. E. and Tucker, C. J.: A Non-Stationary 1981–2012 AVHRR NDVI3g Time Series, Remote Sensing, 6, 6929–6960, https://doi.org/10.3390/rs6086929, 2014.
Pinzon, J. E., Pak, E. W., Tucker, C. J., Bhatt, U. S., Frost, G. V., and Macander, M. J.: Global Vegetation Greenness (NDVI) from AVHRR GIMMS-3G+, 1981–2022, ORNL DAAC, Oak Ridge, Tennessee, USA [data set], https://doi.org/10.3334/ORNLDAAC/2187, 2023.
Pokhrel, Y., Felfelani, F., Satoh, Y., Boulange, J., Burek, P., Gädeke, A., Gerten, D., Gosling, S. N., Grillakis, M., Gudmundsson, L., Hanasaki, N., Kim, H., Koutroulis, A., Liu, J., Papadimitriou, L., Schewe, J., Müller Schmied, H., Stacke, T., Telteu, C.-E., Thiery, W., Veldkamp, T., Zhao, F., and Wada, Y.: Global terrestrial water storage and drought severity under climate change, Nat. Clim. Change, 11, 226–233, https://doi.org/10.1038/s41558-020-00972-w, 2021.
Ponce-Campos, G. E., Moran, M. S., Huete, A., Zhang, Y., Bresloff, C., Huxman, T. E., Eamus, D., Bosch, D. D., Buda, A. R., Gunter, S. A., Scalley, T. H., Kitchen, S. G., McClaran, M. P., McNab, W. H., Montoya, D. S., Morgan, J. A., Peters, D. P. C., Sadler, E. J., Seyfried, M. S., and Starks, P. J.: Ecosystem resilience despite large-scale altered hydroclimatic conditions, Nature, 494, 349–352, https://doi.org/10.1038/nature11836, 2013.
Roderick, M. L. and Farquhar, G. D.: A simple framework for relating variations in runoff to variations in climatic conditions and catchment properties, Water Resour. Res., 47, W00G07, https://doi.org/10.1029/2010WR009826, 2011.
Séférian, R., Nabat, P., Michou, M., Saint-Martin, D., Voldoire, A., Colin, J., Decharme, B., Delire, C., Berthet, S., Chevallier, M., Sénési, S., Franchisteguy, L., Vial, J., Mallet, M., Joetzjer, E., Geoffroy, O., Guérémy, J.-F., Moine, M.-P., Msadek, R., Ribes, A., Rocher, M., Roehrig, R., Salas-y-Mélia, D., Sanchez, E., Terray, L., Valcke, S., Waldman, R., Aumont, O., Bopp, L., Deshayes, J., Éthé, C., and Madec, G.: Evaluation of CNRM Earth System Model, CNRM-ESM2-1: Role of Earth System Processes in Present-Day and Future Climate, J. Adv. Model. Earth Sy., 11, 4182–4227, https://doi.org/10.1029/2019MS001791, 2019.
Sun, H., Chen, L., Yang, Y., Lu, M., Qin, H., Zhao, B., Lu, M., Xue, J., and Yan, D.: Assessing Variations in Water Use Efficiency and Linkages with Land-Use Changes Using Three Different Data Sources: A Case Study of the Yellow River, China, Remote Sensing, 14, 1065, https://doi.org/10.3390/rs14051065, 2022.
Sun, S., Song, Z., Wu, X., Wang, T., Wu, Y., Du, W., Che, T., Huang, C., Zhang, X., Ping, B., Lin, X., Li, P., Yang, Y., and Chen, B.: Spatio-temporal variations in water use efficiency and its drivers in China over the last three decades, Ecol. Indic., 94, 292–304, https://doi.org/10.1016/j.ecolind.2018.07.003, 2018.
Swann, A. L. S.: Plants and Drought in a Changing Climate, Current Climate Change Reports, 4, 192–201, https://doi.org/10.1007/s40641-018-0097-y, 2018.
Tan, X., Jia, Y., Niu, C., Yang, D., Lu, W., and Hao, C.: Response of water-use efficiency to phenology in the natural forest and grassland of the Loess Plateau in China, Science China Earth Sciences, 66, 2081–2096, https://doi.org/10.1007/s11430-022-1124-0, 2023.
Vicente-Serrano, S. M., Beguería, S., and López-Moreno, J. I.: A Multiscalar Drought Index Sensitive to Global Warming: The Standardized Precipitation Evapotranspiration Index, J. Climate, 23, 1696–1718, https://doi.org/10.1175/2009JCLI2909.1, 2010.
Wang, S. and Zhang, Y.: Long-term (1982–2018) global gross primary production dataset based on NIRv, National Tibetan Plateau/Third Pole Environment Data Center [data set], https://doi.org/10.6084/m9.figshare.12981977.v2, 2020.
Wang, M., Ding, Z., Wu, C., Song, L., Ma, M., Yu, P., Lu, B., and Tang, X.: Divergent responses of ecosystem water-use efficiency to extreme seasonal droughts in Southwest China, Sci. Total Environ., 760, 143427, https://doi.org/10.1016/j.scitotenv.2020.143427, 2021.
Wang, S., Zhang, Y., Ju, W., Qiu, B., and Zhang, Z.: Tracking the seasonal and inter-annual variations of global gross primary production during last four decades using satellite near-infrared reflectance data, Sci. Total Environ., 755, 142569, https://doi.org/10.1016/j.scitotenv.2020.142569, 2021.
Wu, X. and Jiang, D.: Probabilistic impacts of compound dry and hot events on global gross primary production, Environ. Res. Lett., 17, 034049, https://doi.org/10.1088/1748-9326/ac4c5b, 2022.
Xie, J., Chen, J., Sun, G., Zha, T., Yang, B., Chu, H., Liu, J., Wan, S., Zhou, C., Ma, H., Bourque, C. P.-A., Shao, C., John, R., and Ouyang, Z.: Ten-year variability in ecosystem water use efficiency in an oak-dominated temperate forest under a warming climate, Agr. Forest Meteorol., 218–219, 209–217, https://doi.org/10.1016/j.agrformet.2015.12.059, 2016.
Xie, S., Mo, X., Hu, S., and Liu, S.: Contributions of climate change, elevated atmospheric CO2 and human activities to ET and GPP trends in the Three-North Region of China, Agr. Forest Meteorol., 295, 108183, https://doi.org/10.1016/j.agrformet.2020.108183, 2020.
Xing, X., Wu, M., Zhang, W., Ju, W., Tagesson, T., He, W., Wang, S., Wang, J., Hu, L., Yuan, S., Zhu, T., Wang, X., Ran, Y., Li, S., Wang, C., and Jiang, F.: Modeling China's terrestrial ecosystem gross primary productivity with BEPS model: Parameter sensitivity analysis and model calibration, Agr. Forest Meteorol., 343, 109789, https://doi.org/10.1016/j.agrformet.2023.109789, 2023.
Xu, X., Li, X., He, C., Tia, W., and Tian, J.: Development of a simple Budyko-based framework for the simulation and attribution of ET variability in dry regions, J. Hydrol., 610, 127955, https://doi.org/10.1016/j.jhydrol.2022.127955, 2022.
Yang, H. and Yang, D.: Derivation of climate elasticity of runoff to assess the effects of climate change on annual runoff, Water Resour. Res., 47, W07526, https://doi.org/10.1029/2010WR009287, 2011.
Yang, L., Feng, Q., Wen, X., Barzegar, R., Adamowski, J. F., Zhu, M., and Yin, Z.: Contributions of climate, elevated atmospheric CO2 concentration and land surface changes to variation in water use efficiency in Northwest China, CATENA, 213, 106220, https://doi.org/10.1016/j.catena.2022.106220, 2022.
Yang, S., Zhang, J., Han, J., Wang, J., Zhang, S., Bai, Y., Cao, D., Xun, L., Zheng, M., Chen, H., Xu, C., and Rong, Y.: Evaluating global ecosystem water use efficiency response to drought based on multi-model analysis, Sci. Total Environ., 778, 146356, https://doi.org/10.1016/j.scitotenv.2021.146356, 2021.
Yang, X., Wang, C., Du, J., Qiu, S., and Liu, J.: Dynamic evolution of attribution analysis of runoff based on the complementary Budyko equation in the source area of Lancang river, Front. Earth Sci., 11, 1160520, https://doi.org/10.3389/feart.2023.1160520, 2023.
Yang, Y., Donohue, R. J., McVicar, T. R., and Roderick, M. L.: An analytical model for relating global terrestrial carbon assimilation with climate and surface conditions using a rate limitation framework, Geophys. Res. Lett., 42, 9825–9835, https://doi.org/10.1002/2015GL066835, 2015.
Yang, Y., Wu, Q., Yun, H., Jin, H., and Zhang, Z.: Evaluation of the hydrological contributions of permafrost to the thermokarst lakes on the Qinghai–Tibet Plateau using stable isotopes, Global Planet. Change, 140, 1–8, https://doi.org/10.1016/j.gloplacha.2016.03.006, 2016.
Yin, J., Gentine, P., Zhou, S., Sullivan, S. C., Wang, R., Zhang, Y., and Guo, S.: Large increase in global storm runoff extremes driven by climate and anthropogenic changes, Nat. Commun., 9, 4389, https://doi.org/10.1038/s41467-018-06765-2, 2018.
Yin, J., Guo, S., Yang, Y., Chen, J., Gu, L., Wang, J., He, S., Wu, B., and Xiong, J.: Projection of droughts and their socioeconomic exposures based on terrestrial water storage anomaly over China, Science China Earth Sciences, 65, 1772–1787, https://doi.org/10.1007/s11430-021-9927-x, 2022.
Yin, J., Gentine, P., Slater, L., Gu, L., Pokhrel, Y., Hanasaki, N., Guo, S., Xiong, L., and Schlenker, W.: Future socio-ecosystem productivity threatened by compound drought–heatwave events, Nat. Sustain., 6, 259–272, https://doi.org/10.1038/s41893-022-01024-1, 2023.
Yuan, X., Wang, Y., Ji, P., Wu, P., Sheffield, J., and Otkin, J. A.: A global transition to flash droughts under climate change, Science, 380, 187–191, https://doi.org/10.1126/science.abn6301, 2023.
Zhan, C., Orth, R., Migliavacca, M., Zaehle, S., Reichstein, M., Engel, J., Rammig, A., and Winkler, A. J.: Emergence of the physiological effects of elevated CO2 on land–atmosphere exchange of carbon and water, Glob. Change Biol., 28, 7313–7326, https://doi.org/10.1111/gcb.16397, 2022.
Zhang, Y., Piao, S., Sun, Y., Rogers, B. M., Li, X., Lian, X., Liu, Z., Chen, A., and Peñuelas, J.: Future reversal of warming-enhanced vegetation productivity in the Northern Hemisphere, Nat. Clim. Change, 12, 581–586, https://doi.org/10.1038/s41558-022-01374-w, 2022.
Zhang, Y., He, Y., and Song, J.: Effects of climate change and land use on runoff in the Huangfuchuan Basin, China, J. Hydrol., 626, 130195, https://doi.org/10.1016/j.jhydrol.2023.130195, 2023.
Zhang, Z., Zhang, Y., Zhang, Y., Gobron, N., Frankenberg, C., Wang, S., and Li, Z.: The potential of satellite FPAR product for GPP estimation: An indirect evaluation using solar-induced chlorophyll fluorescence, Remote Sens. Environ., 240, 111686, https://doi.org/10.1016/j.rse.2020.111686, 2020.
Zhao, F., Wu, Y., Ma, S., Lei, X., and Liao, W.: Increased Water Use Efficiency in China and Its Drivers During 2000–2016, Ecosystems, 25, 1476–1492, https://doi.org/10.1007/s10021-021-00727-4, 2022a.
Zhao, F., Ma, S., Wu, Y., Qiu, L., Wang, W., Lian, Y., Chen, J., and Sivakumar, B.: The role of climate change and vegetation greening on evapotranspiration variation in the Yellow River Basin, China, Agr. Forest Meteorol., 316, 108842, https://doi.org/10.1016/j.agrformet.2022.108842, 2022b.
Zhao, M., A, G., Velicogna, I., and Kimball, J. S.: Satellite Observations of Regional Drought Severity in the Continental United States Using GRACE-Based Terrestrial Water Storage Changes, J. Climate, 30, 6297–6308, https://doi.org/10.1175/JCLI-D-16-0458.1, 2017.
Zheng, Y., Shen, R., Wang, Y., Li, X., Liu, S., Liang, S., Chen, J. M., Ju, W., Zhang, L., and Yuan, W.: Improved estimate of global gross primary production for reproducing its long-term variation, 1982–2017, Earth Syst. Sci. Data, 12, 2725–2746, https://doi.org/10.5194/essd-12-2725-2020, 2020.
Zhong, Y., Tian, B., Vishwakarma, B. D., Feng, W., Wu, Y., Bai, H., and Zhong, M.: Reinterpreting Global GRACE Trends Based on Century-Long GRACE-REC Data, Water Resour. Res., 59, e2023WR035817, https://doi.org/10.1029/2023WR035817, 2023.
Zhou, S., Yu, B., Huang, Y., and Wang, G.: The complementary relationship and generation of the Budyko functions, Geophys. Res. Lett., 42, 1781–1790, https://doi.org/10.1002/2015GL063511, 2015.
Zhou, S., Yu, B., Zhang, L., Huang, Y., Pan, M., and Wang, G.: A new method to partition climate and catchment effect on the mean annual runoff based on the Budyko complementary relationship: Partitioning the climate and catchment effect on runoff, Water Resour. Res., 52, 7163–7177, https://doi.org/10.1002/2016WR019046, 2016.
Zhou, S., Yu, B., Schwalm, C. R., Ciais, P., Zhang, Y., Fisher, J. B., Michalak, A. M., Wang, W., Poulter, B., Huntzinger, D. N., Niu, S., Mao, J., Jain, A., Ricciuto, D. M., Shi, X., Ito, A., Wei, Y., Huang, Y., and Wang, G.: Response of Water Use Efficiency to Global Environmental Change Based on Output From Terrestrial Biosphere Models, Global Biogeochem. Cy., 31, 1639–1655, https://doi.org/10.1002/2017GB005733, 2017.
Zhou, S., Keenan, T. F., Williams, A. P., Lintner, B. R., Zhang, Y., and Gentine, P.: Large Divergence in Tropical Hydrological Projections Caused by Model Spread in Vegetation Responses to Elevated CO2, Earths Future, 10, e2021EF002457, https://doi.org/10.1029/2021EF002457, 2022.
Zhou, S., Yu, B., Lintner, B. R., Findell, K. L., and Zhang, Y.: Projected increase in global runoff dominated by land surface changes, Nat. Clim. Chang., 13, 442–449, https://doi.org/10.1038/s41558-023-01659-8, 2023.
Zhu, Q., Jiang, H., Peng, C., Liu, J., Wei, X., Fang, X., Liu, S., Zhou, G., and Yu, S.: Evaluating the effects of future climate change and elevated CO2 on the water use efficiency in terrestrial ecosystems of China, Ecol. Model., 222, 2414–2429, https://doi.org/10.1016/j.ecolmodel.2010.09.035, 2011.