the Creative Commons Attribution 4.0 License.
the Creative Commons Attribution 4.0 License.
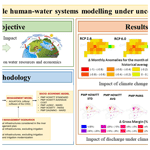
Actionable human–water system modelling under uncertainty
Laura Gil-García
Nazaret M. Montilla-López
Carlos Gutiérrez-Martín
Ángel Sánchez-Daniel
Pablo Saiz-Santiago
Josué M. Polanco-Martínez
Julio Pindado
Carlos Dionisio Pérez-Blanco
This paper develops an actionable interdisciplinary model that quantifies and assesses uncertainties in water resource allocation under climate change. To achieve this objective, we develop an innovative socio-ecological grand ensemble that combines climate, hydrological, and microeconomic ensemble experiments with a widely used decision support system for water resource planning and management. Each system is populated with multiple models (multi-model), which we use to evaluate the impacts of multiple climate scenarios and policies (multi-scenario, multi-forcing) across systems so as to identify plausible futures where water management policies meet or miss their objectives and to explore potential tipping points. The application of the methods is exemplified by a study conducted in the Douro River basin (DRB), an agricultural basin located in central Spain. Our results show how marginal climate changes can trigger non-linear water allocation changes in the decision support systems (DSSs) and/or non-linear adaptive responses of irrigators to water shortages. For example, while some irrigators barely experience economic losses (average profit and employment fall by < 0.5 %) under mild water allocation reductions of 5 % or lower, profit and employment fall by up to 12 % (∼ 24 ×) when water allocation is reduced by 10 % or less (∼ 2×). This substantiates the relevance of informing the potential natural and socio-economic impacts of adaptation strategies and related uncertainties for identifying robust decisions.
- Article
(4202 KB) - Full-text XML
-
Supplement
(56094 KB) - BibTeX
- EndNote
Complex socio-ecological systems, including coupled human–water systems, are inherently difficult to manage (UNDRR, 2019). Periods of relative stability and predictability are interspersed with periods of unexpected, and sometimes abrupt, change (UNDRR, 2021). These changes, even if small, can create ripples that cascade across systems and generate non-trivial environmental and socio-economic impacts that are difficult to foresee – thus leading to uncertainty. We define uncertainty as a situation where “(1) it is not possible to identify all plausible futures, or (2) assign a probability to each identified plausible future” (Walker et al., 2003), which excludes probabilistic risk. Note that, while point (2) refers to uncertainty in modelling that can be quantitatively assessed, point (1) cannot and is accordingly not considered in our study (Knightian uncertainty1; Knight, 1921). Conventional consolidative modelling based on point predictions and optimization of expected performance risks provides unrealistically precise information that gives a false appearance of uncertainty reduction (Hino and Hall, 2017). By ignoring (large parts of) the uncertainty inherent to modelling, the decision-making processes informed by conventional consolidative models can miss their objectives and under some conditions backfire and trigger crises (Anderies et al., 2006; Lempert, 2019). To allow humankind to embark on a sustainable and equitable development trajectory that delivers a satisfactory performance under multiple futures rather than a single plausible future (i.e. robust), a fundamental re-examination of the current approaches to modelling and uncertainty is necessary (IPCC, 2021). This calls for actionable forecasting methods that go beyond conventional consolidative models and point predictions and that thoroughly quantify and assess uncertainty so as to detect potential vulnerabilities of the system (including non-linearities) and identify robust adaptation policies and trajectories (UNEP, 2021).
The literature identifies three fundamental sources of uncertainty in models: (1) input uncertainty arising from scenario design and data inputs (Marchau et al., 2019), (2) parameter uncertainty associated with the data and methods used to calibrate model parameters (Tebaldi and Knutti, 2007), and (3) structural uncertainties associated with “the relationships between inputs and variables, among variables, and between variables and outputs” in models (Walker et al., 2003b). These uncertainties, which emerge within individual system models, can cascade across interconnected systems (UNDRR, 2019). Researchers have developed methods to quantify and assess scenario, parameter, and structural uncertainties in modelling, notably sensitivity analysis and multi-model ensemble experiments. Sensitivity analysis uses experiments representing the consequences of alternative sets of feasible assumptions (about scenario design, data, or parameter values) to discover their implications (Groves et al., 2015; Lempert and Groves, 2010), while multi-model ensemble experiments group multiple models with alternative structures to produce a range of forecasts rather than a single point prediction (CMIP6, 2023; ISIMIP, 2023). Sensitivity analyses and multi-model ensembles can be combined into “grand ensembles” that quantify input, parameter, and structural uncertainties within a system through the ensemble spread (Athey et al., 2019). This approach has been used in disciplines such as the climate sciences (e.g. Hagedorn et al., 2005; IPCC, 2014), economics (e.g. Krüger, 2017), and hydrology (e.g. Cloke et al., 2013). Recent research has combined grand ensembles over multiple ecological systems into multi-sector ensemble experiments such as the Coupled Model Intercomparison Project 6 (CMIP6) and the Inter-Sectoral Impact Model Intercomparison Project (ISIMIP) (CMIP6, 2023; ISIMIP, 2023). Echoing advances in socio-ecological research that have demonstrated the importance of considering the links between environmental change and human behaviour when designing and assessing solutions to complex climate, water, and other challenges (Pande and Sivapalan, 2017), multi-sector ensemble experiments have sought to incorporate human system aspects into their analyses. The conventional approach has been to exogenously model human systems through ensembles of macroeconomic models (typically integrated assessment models) and subsequently transform these simulation outcomes into scenarios of greenhouse gas emissions that can be used to force ensembles of climate system models (Ferrari et al., 2022). Alternatively, human systems can be endogenously represented in the socio-ecological ensemble by explicitly representing the impacts of ecological systems on human behaviour and responses and vice versa, e.g. using microeconomic models (Sapino et al., 2022b). Finally, acknowledging that model performance is conditional on its technical features as well as the modelling context and practices (Hamilton et al., 2019), research has paid attention to decision support systems (DSSs) and studied their design (Guillaume, 2022), output assessment (notably via robust decision-making, including multi-criteria evaluation; Groves et al., 2015; Maier et al., 2016; Marchau et al., 2019), and output interpretation (including the study of beliefs and biases, path dependence, incentives, politics and power, and information gaps and filtering; Cook et al., 2018; Peters and Nagel, 2020; Quiggin, 2012).
However, the application of these practices to human–water system modelling, management, and planning appears to be limited. In a recent literature review of uncertainty quantification in human–water system models (including DSSs used to support management and planning) across 198 studies, it was found that most studies focused on partial assessments of input (148 of 198 studies) or parameter uncertainties (40) through local sensitivity analysis, while structural uncertainties (7) were typically neglected (González-López et al., 2023). Few studies quantified two sources of uncertainty (31), and none quantified all three sources of uncertainty, which reflects the non-trivial computational costs of conducting multi-model and sensitivity analyses across multiple systems. Notably, 51 studies included a DSS or water resource management model such as WEAP or MIKE, of which 35 accounted for input uncertainty, 5 for parameter uncertainty, and 3 for structural uncertainty. Not a single study in the review, whether a DSS or another model, quantified uncertainties in both human and water systems (i.e. studies quantified uncertainties in either human or water systems). While integrated human–water system models (including DSSs) abound in the literature (Baccour et al., 2022; Graveline, 2020; Gil-García et al., 2023; Li et al., 2020; Martínez-Dalmau et al., 2023; Pande and Sivapalan, 2017; Ward, 2021) and examples of model intercomparison experiments (e.g. HEPEX, 2024) and sensitivity analyses do exist (Puy et al., 2022; Saltelli, 2019), particularly in water systems, in practice water resource modelling (including DSSs for planning and management) ignores uncertainties within and across water and/or human systems (OECD, 2021). This is also observed in the wider natural resource literature, where multi-system model intercomparison experiments to quantify structural uncertainties address ecological (and non-human) systems (AGMIP, 2023; CMIP6, 2023; ISIMIP, 2023).
We argue that to develop water policies that are sensitive to climate change and other key sources of uncertainty, including the adaptive responses by human agents, it is necessary to deliver actionable interdisciplinary modelling that quantifies and assesses uncertainty. To achieve this objective, we propose an innovative human–water system grand ensemble that combines climate, hydrological, and microeconomic ensemble experiments with a widely used DSS for water resource planning and management, named AQUATOOL (Andreu et al., 1991). The proposed modelling framework is illustrated with an application that quantifies structural uncertainties and input uncertainties via climate change scenarios, although it can be expanded to quantify parameter and other input uncertainties (with non-trivial computational costs; see Sect. 4). In the first step, we use surface hydrology forecasts under alternative climate scenarios (representative concentration pathways RCP2.6, RCP6.0, and RCP8.5) obtained from ISIMIP to force the DSS AQUATOOL, which yields information on water allocation to water users in the basin. In a second step, we assess the adaptive responses by irrigators to water allocation decisions and their repercussions in terms of income, employment, and water and land use changes. The grand ensemble adopts a modular approach where models at each system level operate independently in modules, which are subsequently interconnected through sets of protocols, i.e. rules designed to manage relationships among modules (Essenfelder et al., 2018). Each system is populated with multiple models (multi-model), which we use to evaluate the impacts of multiple climatic scenarios and policies (multi-scenario, multi-forcing) across systems. The uncertainty range provided through the ensemble spread can reveal relevant trade-offs and vulnerabilities, including potential non-linearities, thus providing valuable information that can be used to revise strategies and policies, including by adapting models to account for expert feedback until a robust policy is agreed upon (Marchau et al., 2019). The methods are exemplified by an application to the Spanish part of the Douro River basin (DRB).
The DRB in Spain covers an area of 78 889 km2 and stretches over eight regions (NUTS22), of which Castile and León is the most relevant (98.25 % of the basin's total area). The region experiences an average annual rainfall of 450–500 mm, with lower figures in the central part, where most of the agricultural area is situated, and higher precipitation in the mountainous areas surrounding the basin. Low rainfall values are complemented in agriculture with an expanding irrigation supply that, while representing 10 % of the total agricultural land, already claimed 89 % of the total water use of 4366×106 m3 yr−1 in 2021 – which is expected to increase to 4692 and 4688 ×106 m3 yr−1 by 2027 and 2033, respectively, and which is mainly driven by irrigation expansion. Water supply on the other hand decreased from 14 231 ×106 m3 yr−1 over 1940–2005 to 12 777 ×106 m3 yr−1 over 1980–2005, and although on average this is still sufficient to meet the growing demand, drought spells are increasing in both frequency and intensity (Field et al., 2014). Agriculture, the main user and the one generating the lowest market added value from water, suffers most from water allocation restrictions as per the Spanish use priority rules established in the Drought Management Plans (DRBA, 2018).
Agricultural lands represent more than half of the Douro River basin's total area (5.7 ×106 ha) and include rainfed crops such as wheat (26 %), barley (23 %), rye (2 %), sunflower (6 %), and vineyards (2 %). Irrigated crops include cereals such as maize (4 %), alfalfa (2 %), vegetables (1 %), and sugar beet (1 %). Surface water resources serve as the primary irrigation water source, representing on average 82 % of the basin's water supply (DRBA, 2022). The relevant administrative units for irrigation in the DRB (and in other regions in Spain) are the agricultural water demand units (AWDUs), which are also the agents in the microeconomic models.
We build a socio-ecological grand ensemble around AQUATOOL, a widely used DSS for watershed planning and management of water resource systems with applications to real planning cases in Spain, Ecuador, Brazil, Italy, Algeria, Mexico, Bosnia, Chile, Peru, Argentina, and Morocco, inter alia. The grand ensemble comprises four modules, each of which represents a key system in the human–water conundrum: the climate system, modelled by ISIMIP (2023) through an ensemble of global circulation models (GCMs); the natural water system, also modelled by ISIMIP (2023) through an ensemble of global hydrological models (GHMs); the water management system, modelled through an ensemble comprising alternative setups of the DSS AQUATOOL; and the human system, modelled through an ensemble of microeconomic mathematical programming models. The coupling among the modules is implemented in three steps (Fig. 2):
-
Step 1 is done externally to our model by ISIMIP Protocol 2b (2023) and includes the simulation of discharge data by forcing the ensemble of GHMs with climate change forecasts obtained from the ensemble of GCMs under alternative climate scenarios.
-
Step 2 imports discharge outputs from the GHM ensemble into the AQUATOOL ensemble and produces data on water allocations under alternative water management scenarios.
-
Step 3 uses water allocation data to force an ensemble of microeconomic models that represent human behaviour and responses and that simulate changes in land use, water use, income, and employment.
The upshot of this coupling process is a database of plausible futures that assesses the repercussions of climate change and adaptation scenarios for the water and human systems while accounting for input (climate and management scenarios), structural and parameter uncertainties in modelling, and cascading uncertainties across coupled ecological and human systems.
The following sub-sections describe the components of the grand ensemble, i.e. the climate scenarios (Sect. 3.1), the management scenarios (Sect. 3.2), and the modules, including the climate system (Sect. 3.3), natural water system (Sect. 3.4), water management system (Sect. 3.5), and human system modules (Sect. 3.6).
3.1 Climate scenarios
The modelling exercise encompasses three of the original four RCP scenarios. ISIMIP Protocol 2b (2023) considers RCP2.6, RCP6.0, and RCP8.5, which outline trajectories used by the IPCC (2021, 2014) to depict various potential climate futures based on future greenhouse gas emissions. In our simulations, for all the scenarios, we assumed present-day socio-economic conditions (2005 economic development, population levels, land use, and management consistent with the management scenarios in AQUATOOL).
-
RCP2.6 outlines a climate scenario where CO2 emissions decline to zero by 2100, with methane emissions at 50 % of 2020 levels and sulfur dioxide emissions at 10 % of 1980–1990 levels. Negative CO2 emissions (e.g. via tree CO2 sequestration) averaging 2 Gt CO2 yr−1 are incorporated. This pathway aims to keep global temperature rise below 2 °C by 2100.
-
RCP6.0 foresees a peak in emissions by 2080, followed by a decline. It involves initially high greenhouse gas emissions and stabilization of radiative forcing post-2100, leading to a projected 3–4 °C temperature rise with CO2 reaching 670 ppm.
-
RCP8.5 depicts a scenario where emissions keep increasing throughout the 21st century, which is typically regarded as unlikely but still possible. Initially viewed as a worst-case scenario with overestimated coal emissions, it continues to be employed today to predict mid-century and earlier emissions based on existing policies.
3.2 Management scenarios
The water management ensemble comprises three different setups of the AQUATOOL model. Each model setup corresponds to one alternative management scenario with specific developments of reservoirs, canals, irrigated land, and irrigation infrastructure. These management scenarios are the outcome of the public consultation process led by the basin authority and implemented during the third river basin planning cycle (2022–2027), which crystalized in the DRB Management Plan (DRBA, 2022). In management scenario 1 (M01), all new developments proposed in the river basin plan are implemented. In management scenario 2 (M02), all new developments proposed in the river basin plan, excluding irrigation expansion, are developed. In management scenario 3 (M03), all new developments proposed in the river basin plan, excluding irrigation expansion and irrigation modernization, are developed. The specific developments carried out in each management scenario are detailed in Appendix A.
3.3 Climate system module
Climate change forecasts are produced by ISIMIP Protocol 2b (2023) by simulating the impacts of the three climate change scenarios (Sect. 3.1) using four GCMs. Each of the four GCMs is combined with each of the three RCP scenarios, thus generating 12 climate scenarios. The outputs from the GCMs are used, in turn, to force GHMs (see the next sub-section). The four GCMs are the following:
-
GFDL-ESM2M combines atmospheric and oceanic circulation models, land dynamics, and biogeochemical processes like the carbon cycle. This model, a collaborative effort involving various institutions under the leadership of the Geophysical Fluid Dynamics Laboratory of the NOAA, aims to study climate and ecosystem interactions, both natural and human-induced. It includes components for the atmosphere, land, and oceans, tracking factors such as aerosols, precipitation, and sea ice dynamics. The model also monitors chemical and ecological tracers that impact nutrient cycles, plant growth, and more. By integrating these components, GFDL-ESM2M provides comprehensive understanding of how Earth's ecosystems interact with the climate system. For additional details and a mathematical statement, the reader is referred to Dunne et al. (2013, 2012).
-
HadGEM2-ES is part of the broader HadGEM2 model family involving diverse model setups that vary in complexity while sharing a unified physical structure. This version of the HadGEM is the second generation and includes, among other features, a well-resolved stratosphere. The HadGEM is developed in the Hadley Center and the Met Office (UK) and is one of the most well-known full global climate models. For additional details and a mathematical statement, the reader is referred to Collins et al. (2011).
-
The IPSL-CM5A-LR model is a comprehensive and full Earth system model (ESM) and is developed at the Institut Pierre-Simon Laplace (IPSL) (France). The model offers a versatile platform for addressing diverse scientific questions. It comprises two sets of physical models, including ocean extensions. The model's configurations can vary in terms of physical parameterizations, resolution, components (ranging from the atmosphere and land to a full ESM), and processes (covering physical, chemical, aerosol, and carbon cycle processes). At its core, IPSL-CM5 integrates components for the land surface, atmosphere sea ice, and ocean, along with biogeochemical processes, including stratospheric and tropospheric chemistry, aerosols, and terrestrial and oceanic carbon cycles. For additional details and a mathematical statement, the reader is referred to Dufresne et al. (2013).
-
MIROC5 (Model for Interdisciplinary Research on Climate version 5) is an atmospheric and oceanic GCM developed at the Atmosphere and Ocean Research Institute of the University of Tokyo (Japan). MIROC is an advanced climate model designed to better simulate the average climate, variability, and climate change resulting from human-induced radiative forcing. This model was tested through a 100-year long control experiment with specific atmospheric and oceanic resolutions, and its performance was compared to observations and a previous model version with varying spatial resolutions. For additional details and a mathematical statement of the model, the reader is referred to Watanabe et al. (2010).
3.4 Natural water system module
Water discharge forecasts are produced by ISIMIP Protocol 2b (ISIMIP, 2023) forcing eight GHMs with the simulation outputs of the four GCMs. GHMs provide spatially aggregated information within standardized grids of 0.5° × 0.5°. The eight GHMs are the following:
-
CLM4.5 explores the cycling of water, trace gases, chemical elements, and energy. The model components include biogeophysics, the hydrological cycle, dynamic vegetation, and biogeochemistry. The land surface is categorized into glacier, lake, wetland, urban, and vegetated areas, with further sub-divisions for plant functional types. For additional details and a mathematical formulation, the reader is referred to Oleson et al. (2013).
-
H08 represents a global hydrological model organized by grid cells, featuring six sub-models designed to explicitly replicate the interplay between the natural water cycle and human activities worldwide. The model maintains a nearly complete water balance. In 2016, water abstraction schemes were improved, and a groundwater scheme was added. CLM4.5 in ISIMP Protocol 2b is run using inputs from the climate models: IPSL-CM5A-LR, HadGEM2-ES, GFDL-ESM2M, and MIROC5. For additional details and a mathematical statement of the model, the reader is referred to Hanasaki et al. (2018).
-
LPJmL is a model that focuses on water balance and irrigation processes, with the latest version distinguishing between different irrigation systems. It is designed to study the impact of replacing natural vegetation with agroecosystems due to rising CO2 levels and climate change. Additionally, it plays a key role in assessing future ecosystem services, considering factors like climate, CO2 levels, land management, and land use change. LPJmL in ISIMP Protocol 2b is run using inputs from the climate models: IPSL-CM5A-LR, HadGEM2-ES, GFDL-ESM2M, and MIROC5. For additional details and a mathematical statement of the model, the reader is referred to Bondeau et al. (2007).
-
MATSIRO is meant to work with a climate system research model. It is used for climate studies covering various timescales and resolutions. MATSIRO focuses on representing essential land–atmosphere water and energy exchange processes in a physically based yet straightforward manner, making it a valuable tool for climate research. MATSIRO in ISIMP Protocol 2b is run using inputs from the climate models: IPSL-CM5A-LR, HadGEM2-ES, GFDL-ESM2M, and MIROC5. For additional details and a mathematical formulation of this model, the reader is referred to Takata et al. (2003).
-
MPI-HM is a model that focuses solely on calculating water fluxes, excluding any considerations for energy balance calculations. MPI-HM is used for high-resolution river routing in hydrological research. MPI-HM in ISIMP Protocol 2b is run using inputs from the climate models: IPSL-CM5A-LR, GFDL-ESM2M, and MIROC5. For additional details and a mathematical statement, the reader is referred to Stacke and Hagemann (2012).
-
PCR-GLOBWB is a model that simulates water dynamics for each grid cell on a daily basis. It tracks water storage in soil and groundwater layers as well as exchanges like infiltration, percolation, and capillary rise. The model includes atmospheric interactions, such as rainfall and evapotranspiration, and connects water use in agriculture, industry, and households to daily hydrological processes. The simulated runoff and water flow are subsequently routed through river networks interconnected with water allocation and reservoir operation schemes. PCR-GLOBWB in ISIMP Protocol 2b is run using inputs from the climate models: IPSL-CM5A-LR, HadGEM2-ES, GFDL-ESM2M, and MIROC5. For additional details and a mathematical statement, the reader is referred to van Beek and Bierkens (2009).
-
WaterGAP2 and WaterGAP2-2C are global freshwater models that assess water flows and storage across continents, factoring in human impact from water abstractions and reservoirs. It helps analyse water scarcity, droughts, floods, and the influence of human actions on groundwater, wetlands, streamflow, and sea level rise. The model relies on climate data, surface water information, land characteristics, and more for its inputs. WaterGAP2 and WaterGAP2-2C in ISIMP Protocol 2b are run using inputs from the climate models: IPSL-CM5A-LR, HadGEM2-ES, GFDL-ESM2M, and MIROC5. For additional details and a mathematical statement of the model, the reader is referred to Alcamo et al. (2003).
3.5 Water management system module
AQUATOOL serves as a DSS designed for editing, implementing, reviewing, and analysing hydrologic models, with a specific emphasis on integrated watershed management. It provides detailed data on the qualitative and quantitative conditions of water bodies as well as water allocation, across both space and time. AQUATOOL is structured into various modules, each with its own model or software. In our application to the DRB, we utilize the AQUATOOL module to conduct a comprehensive longitudinal and spatial analysis of the impacts of climate change and discharge variations in alternative management scenarios on water bodies and water allocation. The impacts of climate change models and management scenarios on surface water bodies are simulated according to continuity or equilibrium principles, while both unicellular and multicellular models are used for groundwater bodies. The water allocation, on the other hand, is determined by relying on a network optimization algorithm. The model is calibrated following a positive approach that aims to minimize the difference between simulated and observed water allocations, observed discharges, and reservoir levels (PUV, 2020).
The data inputs for setting up AQUATOOL in the DRB are accessible online (Mírame-IDEDUERO, 2023), excluding the discharge series under natural conditions for the model baseline conditions (no climate change), which must be generated. To obtain these discharge series under natural conditions, daily precipitation series from 1940 to 2018 are processed using the EVALHID tool (Lerma et al., 2017), in addition to SIMPA (Sistema Integrado para la Modelación del proceso Precipitación Aportación) (CEDEX, 2020).
AQUATOOL is openly accessible for academics and practitioners, while private for-profit companies have to pay a fee.
3.6 Human system module
The human system module is comprised of an ensemble of five positive mathematical programming (PMP) models. PMP modelling also adapts a positive calibration approach capable of reproducing the choices of the reference year without error. PMP was first formalized by Howitt (1995) and has since been the dominant technique for calibrating mathematical decision-making models in the agricultural sector. In general, these models include a non-linear component within the objective function, which can be yield or cost. The original parameter, yield (yi) or cost (ci), is replaced with a crop-area-dependent function ( or ), so that when the area of a crop (xi) expands, its yield decreases (its cost increases) and vice versa, with B0i, B1i, αi, and βi being the calibrating parameters (intercept and slope) for yield and cost linear functions.
Five alternative different PMP calibration techniques have been included in the human system ensemble, i.e. the standard approach of Howitt (1995), the average cost approach (Heckelei et al., 2000), the Paris (1988) approach, the Júdez et al. (2001) approach, and the Dagnino and Ward (2012) approach, which we briefly introduce below. For a detailed description and mathematical statement of the model, the reader is referred to the original papers. While all of these approaches reproduce the reference or calibration year without error, the objective function and agent responses during the simulations do differ, often significantly.
-
Standard approach (Howitt, 1995). The original work included a yield function, which in this case has been replaced by a cost function. This method needs two stages to calibrate. First, the dual values (μi) of some calibration constraints are obtained using a linear model. From these dual values, the observed cost () and the observed area (), the calibration coefficients of the cost function (αi and βi) are obtained. As noted by Heckelei et al. (2000), a key problem with the standard approach is the under-determination of the calibration parameters.
-
Average cost approach (Heckelei et al., 2000). The average cost approach is similar to Howitt (1995), but the calibration parameters are determined in such a way that, for the reference year, the value of the cost function coincides with the observed average cost.
-
Paris (1988) eliminates the first calibration parameter.
-
Judez et al. (2001) skip the first phase of Howitt's method and rely on external information to calibrate the model, land rent (LandRent), and average income per crop (AverageIncomei).
-
Dagnino and Ward (2012) also skip the first phase and directly calibrate a yield function with the parameters B0i (intercept) and B1i (slope) from the observed yield (), average income (AverageIncomei), and price per crop (pricei).
All the models maximize a quadratic objective function where the only relevant attribute is profit, as measured by the profit, subject only to soil and water constraints. The data used for the calibration of the five PMP models are available in Table S1 in the Supplement.
3.7 Results
We conduct a set of simulations in three steps, following the hierarchy detailed in Fig. 2. Step 1, which is performed externally to our model by ISIMIP (2023) Protocol 2b, produces discharge data by forcing eight GHMs with climate change forecasts produced by an ensemble of four GCMs in three climate change scenarios. This results in 86 plausible futures (note that not all GHMs can run simulations using the outputs produced by the GCMs, as explained in Sect. 3.4). Discharge data are produced in regular grids of 0.5° × 0.5° and are transformed into discharge anomalies (%) by comparing GHM forecasts under climate change (2006–2040 and 2006–2070 periods) to simulations using historical data (45 years in historical series from 1961 to 2005). Figure 3 illustrates longitudinal discharge anomalies for a critical section of the basin on the border between Portugal and Spain, using a 12-month moving average. Most combinations of models and scenarios in Fig. 3 forecast a reduction in discharge. Discharge reductions exhibit a more significant impact during the 2040–2070 period compared to the earlier 2006–2040 period, which is exacerbated by the peak in greenhouse gas concentrations and the worsening effects of climate change on the water cycle.
In Step 2, anomalies in discharge reported by GHMs are imported into the water management system ensemble to obtain longitudinal series of water allocation for each AWDU in three alternative management scenarios (see Sect. 3.2). To this end, we follow the approach by MAGRAMA (2017) to adjust the discharge series under natural conditions in AQUATOOL using the discharge anomalies obtained in Step 1. The resulting water allocations for each of the 150 AWDUs in the Castile and León region and every year in the series are reported in Table S2 in the Supplement. The integration of the ensembles of the climate system, natural water system, and water management system further amplifies the database of plausible futures to 258.
Finally, in Step 3, each of these 258 plausible futures and related water allocations to AWDUs is used to force the human system ensemble and produce longitudinal forecasts of the impacts of climate change and water management strategies on the income and employment for each of the AWDUs in the DRB. The box–whisker plot in Fig. 4 quantifies the uncertainty across the entire basin for each RCP scenario (RCP2.6, RCP6.0, and RCP8.5) for both profit and employment. In Fig. 4a, the change in profit shows greater dispersions and outliers in the RCP2.6 scenario, while the RCP6.0 and RCP8.5 scenarios display distributions that are more concentrated around the median. In all cases, the median is negative, indicating a reduction in profits in each scenario. In Fig. 4b, changes in employment also exhibit a negative trend in the median across all the RCP scenarios. The data dispersion is greater in the RCP8.5 scenario, followed by RCP6.0 and RCP2.6, suggesting increased variability in employment changes as greenhouse gas concentrations rise. Figure 4a and b reflect an adverse impact on both profit and employment as the RCP scenarios progress, with more pronounced effects in scenarios with higher greenhouse gas concentrations (RCP8.5).
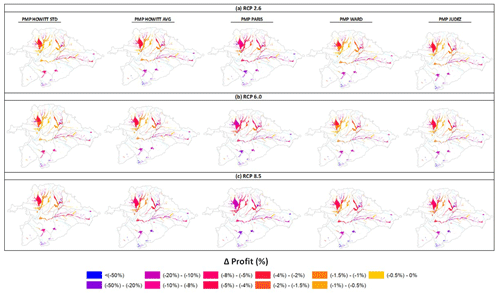
Figure 5Spatially disaggregated impacts (best estimates) of discharge anomalies under climate change (a RCP2.6, b RCP6.0, c RCP8.5) on profit in the AWDUs of the Douro River basin for the 2006–2070 periods, considering the management scenario M03. Changes in profit are obtained as the difference between simulated and observed values in the year 2017. It is important to note that the GHM MPI-HM yields discharge forecasts that are markedly lower than those obtained with the other models, leading to outliers in the employment and profit predictions, which decrease by nearly 100 % in most of the years in the series. This outlier is excluded from the best estimates reported in this figure.
The presented box–whisker plot lacks spatial disaggregation of the results, thus constraining our understanding of spatial variations within the basin. To address this limitation, Fig. 5 exemplifies the modelling potential in delivering spatially distributed profit outcomes. This figure presents the impacts of climate change on profit for each of the five PMP models, considering one adaptive management strategy (M03) and three climate scenarios (RCP2.6, RCP6.0, and RCP8.5) over the period 2006–2070 and for each of the AWDUs in the DRB. Such spatial representation allows for the identification of regional patterns and trends that remain elusive in the aggregate analysis, which is of value for local planning and management by pinpointing specific areas necessitating attention or adaptation. Detailed results of the climate change impacts on profit and employment for each AWDU, management strategy, and climatic scenario are available in Appendix B. Additionally, Table S3 in the Supplement provides longitudinal projections of profit and employment for each ensemble model and AWDU.
For all AWDUs, all combinations of models and scenarios predict a reduction in profit and employment due to climate change. Profit and employment losses are higher for 2040–2070, when greenhouse gas concentrations peak and discharge reductions are more marked. Notably, employment and profit losses are significantly higher in management scenario M01 than in M02, which in turn also displays higher employment and profit losses than M03. This indicates that the expansion of irrigation (incorporated into M01) and its modernization (M01, M02) negatively affect both profit and employment. This is due to the reallocation of water resources from downstream to upstream users, resulting in a cascade of negative and sometimes non-linear repercussions that diminish overall profit and employment losses.
AWDUs in the DRB initially manage decreases in water allocations by replacing irrigated crops at the margin (wheat) with rainfed crops, so as to maintain the surface area dedicated to valuable irrigated crops like sugar beet, vegetables, maize, and fruits. As water allocations continue to decline, AWDUs are constrained to decrease the surface area of increasingly valuable irrigated crops, resulting in more abrupt profit reductions. Due to the labour-intensive nature of these crops, employment also undergoes sudden changes. This explains why marginal decreases in water allocation can lead to substantial and sometimes disproportionately larger decreases in profit and employment, contributing to a considerable degree of non-linear change. For instance, AWDUs in the Carrión sub-basin (AWDUs 2000063, 2000064, 2000065, 2000082, 2000083, 2000084, 2000085, 2000086, 2000097, 2000099, and 2000105 – see Table S3) can initially manage reductions in water allocation of up to 10 % with low to moderate economic losses (< 5 %). However, they experience abrupt decreases in profit and employment of up to 40 % under more stringent water allocation reductions of around 20 %. Similarly, AWDUs in the Arlanza sub-basin (AWDUs 2000077, 2000078, 2000079, 2000080, 2000235, 2000320, 2000338, and 2000603 – see Table S3), with minimal economic losses (< 0.5 %) under mild water allocation reductions of 5 % or lower, experience abrupt decreases in profit and employment of 12 % when water allocation is decreased by 10 %. These complex interactions between human behaviour and the water system, including non-linear responses by economic agents to environmental change, cannot be fully understood or modelled without considering the economic system. To this end, coupled socio-ecological modelling is necessary – although this can further amplify uncertainty, especially if more than one human system model is used.
This paper introduces a modular hierarchy comprising ensembles of socio-economic and ecological systems (multi-system ensemble). Each ensemble incorporates multiple models (multi-model ensemble) employed to evaluate the repercussions of climate change and management scenarios for water availability, profit, and employment (multi-model ensemble). Using this modelling approach, a comprehensive database of simulations is generated, wherein each result represents the socio-economic and environmental implications of a distinct combination of scenarios and models, thus quantifying parameter, structural, and scenario uncertainties. By integrating human system dynamics into the modelling framework, the resulting grand ensemble accounts for non-linearities emerging across both human and water systems as well as their cascading impacts, thus providing valuable data for informing robust strategies.
The grand ensemble is built around a commonly used DSS model, AQUATOOL, thus contributing to the generation of actionable science that can be readily adopted by decision-makers and other stakeholders. The coupling framework is intentionally crafted to be reproducible and adaptable, with the ability to incorporate alternative climate, hydrological, DSS, and microeconomic models of farmers that may better represent climate, water, and/or human systems in different regions. Accordingly, our coupling approach and modelling framework can be applied widely and at a relatively low cost by exploiting existing data and/or models. To better inform the replication of our framework elsewhere, we exemplify below which models can be used to populate our framework at each system level.
-
Climate system and natural water system: climate forecasts for alternative climate scenarios are available in climate ensemble experiments, including global (CMIP6, 2023; ISIMIP, 2023) and downscaling (EURO-CORDEX, 2023) experiments at a regional level, which offer simulation outputs for key climate change scenarios such as RCP2.6 or RCP6.0. Managing these simulation outputs requires skills in big data, knowledge of NetCDF and related software, knowledge of the format in which simulations are reported, and elemental knowledge of climate model simulation. Climate ensemble experiments can provide relevant data on water discharge, a key input for the water management system. Water discharge data can alternatively be produced using regionally calibrated models, which requires ad hoc data-gathering efforts (although some of these models also provide databases for their calibration) and modelling skills in the specific software to be used. Also, a list of the hydrological models included in Pérez-Blanco et al. (2022) could be incorporated: the Soil and Water Assessment Tool – SWAT (Arnold et al., 1998); the Annualized Agricultural Non-Point Source Pollution Model – AnnAGNPS (Young et al., 1989); the Areal Nonpoint Source Watershed Environment Response Simulation – ANSWERS 2000 (Bouraoui and Dillaha, 2000); the Agricultural Policy/Environmental eXtender model – APEX (Gassman et al., 2009); the US Army Corps of Engineers Hydrologic Engineering Center Hydrologic Modeling System – HEC-HMS (US Army Corps of Engineers, 2015); and the Soil and Water Integrated Model – SWIM (Krysanova et al., 2005).
-
Water management system: the data inputs necessary to run the relevant DSS in a given basin are typically accessible to the competent authority, either directly or through a consulting company. Some widely used DSSs that could be incorporated into our modelling framework include AQUATOOL, WEAP, TOPKAPI, MIKE, RIBASIM, or LISFLOOD. Critically, DSSs are often profusely edited to account for the unique features of the basin at hand, and thus their management requires support from an expert. In our illustrative example with AQUATOOL, the competent authority was the DRB, which typically relies on an external consultant to run hydrological simulations of the management and allocation of water resources. For this research, USAL collaborated with the consultant to develop the coupling and run the simulations, leveraging funding provided by the DRB.
-
Human system: the human system can be populated by any mathematical programming model of agricultural water use available in the literature. The data necessary to run these models are provided in Table S1. In the case of EU river basins, all necessary data are publicly available, although the granularity may differ. For example, in the case of the Portuguese part of the DRB, a similar database to the one used in this paper is publicly available, although the granularity is significantly lower (regional scale rather than AWDU scale).
The current and previous (Gil-García et al., 2023; Pérez-Blanco et al., 2021a, b) versions of the AQUATOOL-based human–water system DSS presented in this paper have already been used by stakeholders in the context of financial and economic viability assessments of new water works proposed in the Douro River Basin Plan under climate change and uncertainty, including La Rial reservoir, Los Morales reservoir, the Lastras de Cuéllar reservoir (assessed with previous versions of the model with a focus on input uncertainty), and the Las Cuezas reservoirs (assessed with the current version of the model that includes structural uncertainties in models). All of these assessments were commissioned by the river basin authority.
Future scientific research offers several avenues to further develop and expand the proposed hierarchical coupling framework for understanding the intricate interactions between human actions, water resources, and related uncertainties. Firstly, it is possible to introduce improvements to the individual models within each module by integrating recent scientific advancements within each discipline. For instance, recent developments in microeconomic modelling decouple land use choices from water use choices, allowing for two decision variables rather than one decision variable, as is usually the case in conventional PMP and other mathematical programming models, where agents only decide on land use (Graveline and Merel, 2014; Loch et al., 2020; Sapino et al., 2022a). This allows for the representation and assessment of adaptation measures at the intensive margin (such as deficit or supplementary irrigation), going beyond the extensive (transition to less water-intensive crops) and super-extensive (transition to rainfed crops) adaptations examined in conventional PMP and other mathematical programming models.
Secondly, additional protocols could be introduced across systems to bolster the framework and its interactions. For instance, this might entail incorporating distinct protocols for water use decisions independent of land use decisions in the coupling between the human and hydrological modules.
Thirdly, the modelling framework could benefit from the integration of extra modules, including the linkage with macroeconomic or crop models. This integration would allow for the evaluation of climate change impacts on crop yields (using crop models) and prices (using macroeconomic models). Multi-model ensembles of global gridded crop models are available in ISIMIP (2023) and could be coupled following a similar procedure to the one described here for the natural water systems or GHMs, while the integration of macroeconomic models such as computable general equilibrium (CGE) models (Hertel and Liu, 2016) into water system research has already been done (Parrado et al., 2020; Pérez-Blanco et al., 2021a; Pérez-Blanco and Gutiérrez-Martín, 2017; Ronneberger et al., 2009). By adding these and other new system modules, uncertainty could further cascade across systems. This can help us identify new vulnerabilities and further underpin robust decision-making.
On the other hand, as the number of modules, protocols, structures, and other modelling factors (inputs, parameters, structures) considered in the analysis grows, other issues may arise that may reduce the tractability of the problem. These issues are dealt with in our last three recommendations for improvement.
Fourthly, having incorporated multiple uncertainties, the model output may vary “so wildly as to be of no practical use” (Saltelli et al., 2008). However, as noted by Saltelli et al. (2008) and in line with previous work by Beven and Binley (1992) and Beven and Freer (2001) that introduced the equifinality concept (i.e. distinct configurations of model components such as inputs, parameters, or structures can lead to similar or equally acceptable representations of the real-world process of interest), this “trade-off may not be as dramatic as one might expect, and increasing the number of input factors does not necessarily lead to an increased variance in model output.” (Beven and Freer, 2001). Typically, a few inputs create almost all the uncertainty, and the majority make a marginal contribution.
Fifthly, computational costs may pose a challenge to conducting uncertainty quantification and analyses, where (1) each model run demands a considerable amount of time stretching from minutes to hours or even longer (especially in the case of highly intricate models) and/or (2) the model encompasses numerous uncertain inputs, which expand the computational cost exponentially with the increase in the number of inputs – a phenomenon known as the curse of dimensionality. Addressing computational expenses is crucial in many practical sensitivity analyses and model intercomparison projects. Strategies to mitigate this burden include employing emulators or meta-models driven by machine learning techniques that are particularly suitable for large models (Storlie et al., 2009) and employing screening methods to reduce the dimensionality of the problem, e.g. high-dimensional model representations (Li et al., 2006).
Sixthly, at some point, modellers must decide on the boundaries for the uncertainty quantification, i.e. the inputs and models, which will condition the outputs of the modelling exercise. This involves defining some limits to not generate computational costs we cannot afford through model selection and other techniques. For example, techniques for model selection can be employed to assign weights to the models in the ensemble based on their performance in calibration and forecasting errors. This could help us not only reduce computational costs (e.g. by discarding some models) but also reduce potential biases, such as the simulation outputs from GHM MPI-HM, which yields discharge forecasts that are markedly lower than the other models (with reductions close to or equal to 100 %), shifting the ensemble spread downwards and with important implications for human system forecasting (see Fig. 5). It should be noted that, while model selection techniques based on forecasting errors can be implemented for GHMs and GCMs, the measurement of forecasting errors in human system models is significantly more challenging: information on agent crop choices in the DRB has only been available since 2004, which leads to a significantly more reduced data series that complicates the implementation of rolling origins or other techniques to measure forecasting errors. On the other hand, the adoption of model selection techniques on the basis of calibration errors can be misleading, since models with higher calibration errors may show better predictive performance (Pindyck, 2015).
The convenience of adopting model selection techniques is a question for debate, since weighting can significantly affect modelling results and condition stakeholder choices and decision-making. On the other hand, it has been contended that, whenever model selection techniques are not considered, each potential simulation outcome is equally important, “which can also be interpreted as an implicitly equal weighting” (Taner et al., 2019). This is more so the case when results are explicitly reported using best estimates as done in Figs. 3–5. While this statistical treatment is a key step in making results understandable to users, it may introduce non-trivial biases through the processing and communication of modelling results, which has to be explicitly addressed by the use of dispersion measures (Fig. 5) and by the development of adequate processing techniques for modelling outputs (e.g. serious games that convey the economic and environmental repercussions of water extremes). On the other hand, a similar critique can be made of weighting. The decision whether to assign weights to simulation outcomes or leave them open for interpretation remains a subject of debate among academics (Taner et al., 2019).
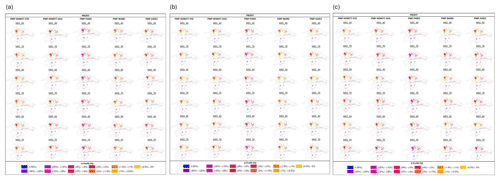
Figure B1Spatially disaggregated impacts (best estimates) of discharge anomalies under climate change (a RCP2.6, b RCP6.0, (c) RCP8.5) on profit in the AWDUs of the Douro River basin for the 2006–2040 and 2040–2070 periods. Changes in profit are obtained as the difference between simulated values under alternative climate change and management scenarios and observed values in the year 2017. It is important to note that the GHM MPI-HM yields discharge forecasts that are markedly lower than those obtained with the other models, leading to outliers in the employment and profit predictions, which decrease by nearly 100 % in most of the years in the series. This outlier is excluded from the best estimates reported in this figure.
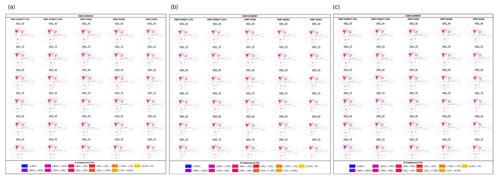
Figure B2Spatially disaggregated impacts (best estimates) of discharge anomalies under climate change (a RCP2.6, b RCP6.0, c RCP8.5) on employment in the AWDUs of the Douro River basin for the 2006–2040 and 2040–2070 periods. Changes in employment are obtained as the difference between simulated values under alternative climate change and management scenarios and observed values in the year 2017. It is important to note that the GHM MPI-HM yields discharge forecasts that are markedly lower than those obtained with the other models, leading to outliers in the employment and profit predictions, which decrease by nearly 100 % in most of the years in the series. This outlier is excluded from the best estimates reported in this figure.
The data that support the findings of this study are available from the corresponding author upon reasonable request.
Supplement 1 contains data input of the PMP models (see Excel file “Table S1” attached to the submission for the database). Supplement 2: data of water allocations for each AWDU (see Excel file “Table S2” attached to the submission. Two sheets – first: monthly allocation, second: yearly water allocation deficit; input for the micro-model) Supplement 3: data of the impacts on profit and employment for the AWDUs (see Excel file “Table S3” attached to the submission) The supplement related to this article is available online at: https://doi.org/10.5194/hess-28-4501-2024-supplement.
LGG: data curation, formal analysis, software, investigation, visualization, writing – original draft, writing – review and editing. NMML: data curation, software, investigation, writing – review and editing. CGM: supervision, software, investigation, writing – review and editing. ÁSD: data curation, software. PSS: data curation, software. JMPM: data curation, writing – review and editing. JP: writing – review and editing. CDPB: conceptualization, funding acquisition, project administration, supervision, investigation, methodology, resources, writing – original draft, writing – review and editing.
The contact author has declared that none of the authors has any competing interests.
Publisher's note: Copernicus Publications remains neutral with regard to jurisdictional claims made in the text, published maps, institutional affiliations, or any other geographical representation in this paper. While Copernicus Publications makes every effort to include appropriate place names, the final responsibility lies with the authors.
This article is part of the special issue “Drought, society, and ecosystems (NHESS/BG/GC/HESS inter-journal SI)”. It is not associated with a conference.
This research is part of project no. TED2021-131066B-I00 funded by MCIN/AEI/10.13039/501100011033, by the European Union “NextGenerationEU”/PRTR, by project WADECO (PID2023-146274OB-I00) and by the PRIMA Foundation's TALANOA-WATER project (Talanoa Water dialogue for Transformational Adaptation to Water Scarcity under Climate Change).
This paper was edited by Giuliano Di Baldassarre and reviewed by Adam Loch and one anonymous referee.
AGMIP: Agricultural Model Intercomparison and Improvement Project, Columbia University, https://agmip.org/ (last access: 1 September 2023), 2023.
Alcamo, J., Döll, P., Henrichs, T., Kaspar, F., Lehner, B., Rösch, T., and Siebert, S.: Development and testing of the WaterGAP 2 global model of water use and availability, Hydrolog. Sci. J., 48, 317–337, https://doi.org/10.1623/hysj.48.3.317.45290, 2003.
Anderies, J. M., Ryan, P., and Walker, B. H.: Loss of Resilience, Crisis, and Institutional Change: Lessons from an Intensive Agricultural System in Southeastern Australia, Ecosystems, 9, 865–878, https://doi.org/10.1007/s10021-006-0017-1, 2006.
Andreu, J., Capilla, J., and Sanchis, E.: AQUATOOL: A Computer-Assisted Support System for Water Resources Research Management Including Conjunctive Use, in: Decision Support Systems, edited by: Loucks, D. P. and Da Costa, J. R., Springer Berlin Heidelberg, Berlin, Heidelberg, https://doi.org/10.1007/978-3-642-76048-8_15, 333–355, 1991.
Arnold, J. G., Srinivasan, R., Muttiah, R. S., and Williams, J. R.: LARGE AREA HYDROLOGIC MODELING AND ASSESSMENT PART I: MODEL DEVELOPMENT, J. Am. Water Resour. As., 34, 73–89, https://doi.org/10.1111/j.1752-1688.1998.tb05961.x, 1998.
Athey, S.,Bayati, M., Imbens, G., and Qu, Z.: Ensemble Methods for Causal Effects in Panel Data Settings (Working Paper No. 25675), Nat. Bur. Ec., AEA Papers and Proceedings, 109, 65–70, https://doi.org/10.3386/w25675, 2019.
Baccour, S., Ward, F. A., and Albiac, J.: Climate adaptation guidance: New roles for hydroeconomic analysis, Sci. Total Environ., 835, 155518, https://doi.org/10.1016/j.scitotenv.2022.155518, 2022.
Beven, K. and Binley, A.: The future of distributed models: Model calibration and uncertainty prediction, Hydrol. Process., 6, 279–298, https://doi.org/10.1002/hyp.3360060305, 1992.
Beven, K. and Freer, J.: Equifinality, data assimilation, and uncertainty estimation in mechanistic modelling of complex environmental systems using the GLUE methodology, J. Hydrol., 249, 11–29, https://doi.org/10.1016/S0022-1694(01)00421-8, 2001.
Bondeau, A., Smith, P. C., Zaehle, S., Schaphoff, S., Lucht, W., Cramer, W., Gerten, D., Lotze-Campen, H., Müller, C., Reichstein, M., and Smith, B.: Modelling the role of agriculture for the 20th century global terrestrial carbon balance, Glob. Change Biol., 13, 679–706, https://doi.org/10.1111/j.1365-2486.2006.01305.x, 2007.
Bouraoui, F. and Dillaha, T. A.: ANSWERS-2000: Non-Point-Source Nutrient Planning Model, J. Environ. Eng., 126, 1045–1055, https://doi.org/10.1061/(ASCE)0733-9372(2000)126:11(1045), 2000.
CEDEX: SIMPA. Sistema Integrado para la Modelación del proceso Precipitación Aportación, Ministerio para la Transición Ecológica y el Reto Demográfico, http://ceh-flumen64.cedex.es/Hidrologia/pub/proyectos/simpa.htm (last access: 1 September 2023), 2020.
Cloke, H. L., Pappenberger, F., van Andel, S. J., Schaake, J., Thielen, J., and Ramos, M. H.: Hydrological ensemble prediction systems, Hydrol. Process., 27, 1–4, https://doi.org/10.1002/hyp.9679, 2013.
CMIP6: Detailed and up-to-date description of the CMIP6 experiments protocol, https://wcrp-cmip.org/cmip6/ (last access: 1 September 2023), 2023.
Collins, W. J., Bellouin, N., Doutriaux-Boucher, M., Gedney, N., Halloran, P., Hinton, T., Hughes, J., Jones, C. D., Joshi, M., Liddicoat, S., Martin, G., O'Connor, F., Rae, J., Senior, C., Sitch, S., Totterdell, I., Wiltshire, A., and Woodward, S.: Development and evaluation of an Earth-System model – HadGEM2, Geosci. Model Dev., 4, 1051–1075, https://doi.org/10.5194/gmd-4-1051-2011, 2011.
Cook, J., Ellerton, P., and Kinkead, D.: Deconstructing climate misinformation to identify reasoning errors, Environ. Res. Lett., 13, 024018, https://doi.org/10.1088/1748-9326/aaa49f, 2018.
Dagnino, M. and Ward, F. A.: Economics of Agricultural Water Conservation: Empirical Analysis and Policy Implications, Int. J. Water Resour. D., 28, 577–600, https://doi.org/10.1080/07900627.2012.665801, 2012.
DRBA: Plan Especial de Sequía, Douro River Basin Authority, Valladolid, Spain, https://www.chduero.es/documents/20126/99746/PlandeSequia_CHDuero_Memoria.pdf (last access: 1 May 2023), 2018.
DRBA: Plan Hidrológico de la Cuenca del Duero 2022–2027, Douro River Basin Authority, Valladolid, Spain, https://www.chduero.es/documents/20126/1883851/PHD22-27_000_00_Memoria-v08.pdf/8e3cd7e5-6e16-9c6c-f15d-5f65788ba883?t=1666613605946 (last access: 1 May 2023), 2022.
Dufresne, J.-L., Foujols, M.-A., Denvil, S., Caubel, A., Marti, O., Aumont, O., Balkanski, Y., Bekki, S., Bellenger, H., Benshila, R., Bony, S., Bopp, L., Braconnot, P., Brockmann, P., Cadule, P., Cheruy, F., Codron, F., Cozic, A., Cugnet, D., De Noblet, N., Duvel, J.-P., Ethé, C., Fairhead, L., Fichefet, T., Flavoni, S., Friedlingstein, P., Grandpeix, J.-Y., Guez, L., Guilyardi, E., Hauglustaine, D., Hourdin, F., Idelkadi, A., Ghattas, J., Joussaume, S., Kageyama, M., Krinner, G., Labetoulle, S., Lahellec, A., Lefebvre, M.-P., Lefevre, F., Levy, C., Li, Z. X., Lloyd, J., Lott, F., Madec, G., Mancip, M., Marchand, M., Masson, S., Meurdesoif, Y., Mignot, J., Musat, I., Parouty, S., Polcher, J., Rio, C., Schulz, M., Swingedouw, D., Szopa, S., Talandier, C., Terray, P., Viovy, N., and Vuichard, N.: Climate change projections using the IPSL-CM5 Earth System Model: from CMIP3 to CMIP5, Clim. Dynam., 40, 2123–2165, https://doi.org/10.1007/s00382-012-1636-1, 2013.
Dunne, J. P., John, J. G., Adcroft, A. J., Griffies, S. M., Hallberg, R. W., Shevliakova, E., Stouffer, R. J., Cooke, W., Dunne, K. A., Harrison, M. J., Krasting, J. P., Malyshev, S. L., Milly, P. C. D., Phillipps, P. J., Sentman, L. T., Samuels, B. L., Spelman, M. J., Winton, M., Wittenberg, A. T., and Zadeh, N.: GFDL's ESM2 Global Coupled Climate–Carbon Earth System Models. Part I: Physical Formulation and Baseline Simulation Characteristics, J. Climate, 25, 6646–6665, https://doi.org/10.1175/JCLI-D-11-00560.1, 2012.
Dunne, J. P., John, J. G., Shevliakova, E., Stouffer, R. J., Krasting, J. P., Malyshev, S. L., Milly, P. C. D., Sentman, L. T., Adcroft, A. J., Cooke, W., Dunne, K. A., Griffies, S. M., Hallberg, R. W., Harrison, M. J., Levy, H., Wittenberg, A. T., Phillips, P. J., and Zadeh, N.: GFDL's ESM2 Global Coupled Climate–Carbon Earth System Models. Part II: Carbon System Formulation and Baseline Simulation Characteristics, J. Climate, 26, 2247–2267, https://doi.org/10.1175/JCLI-D-12-00150.1, 2013.
Essenfelder, A. H., Pérez-Blanco, C. D., and Mayer, A. S.: Rationalizing Systems Analysis for the Evaluation of Adaptation Strategies in Complex Human–Water Systems, Earths Future, 6, 1181–1206, https://doi.org/10.1029/2018EF000826, 2018.
EURO-CORDEX: Coordinated Downscaling Experiment – European Domain, Climate Service Center Germany, Germany, https://www.euro-cordex.net/ (last access: 1 September 2023), 2023.
Eurostat: Eurostat Database, Eurostat Database, http://epp.eurostat.ec.europa.eu/portal/page/portal/statistics/themes, (last access: 10 May 2013), 2020.
Ferrari, L., Carlino, A., Gazzotti, P., Tavoni, M., and Castelletti, A.: From optimal to robust climate strategies: expanding integrated assessment model ensembles to manage economic, social, and environmental objectives, Environ. Res. Lett., 17, 084029, https://doi.org/10.1088/1748-9326/ac843b, 2022.
Field, C. B., Barros, V. R., Dokken, D. J., Mach, K. J., and Mastrandrea, M. D.: Summary for Policymakers, in: Climate Change 2014 Impacts, Adaptation, and Vulnerability, Cambridge University Press, Cambridge, https://doi.org/10.1017/CBO9781107415379.003, 1–32, 2014.
Gassman, P., Williams, J., and Wang, X.: The Agricultural Policy Environmental Extender (APEX) model: An emerging tool for landscape and watershed environmental analyses (Report No. 41), CARD Technical Reports, CARD, Iowa Sate University, Ames, https://www.card.iastate.edu/products/publications/synopsis/?p=1101 (last access: 1 June 2023), 2009.
Gil-García, L., González-López, H., and Pérez-Blanco, C. D.: To dam or not to dam? Actionable socio-hydrology modeling to inform robust adaptation to water scarcity and water extremes, Environ. Sci. Policy, 144, 74–87, https://doi.org/10.1016/j.envsci.2023.03.012, 2023.
González-López, H., Pérez-Blanco, C. D., Gil-García, L., Foster, T., Mysiak, J., and Pulido-Velázquez, M.: Report Deliverable 3.1: WHITE PAPER – METHODS & MODELS FOR ASSESSING POLICY PERFORMANCE UNDER DEEP UNCERTAINTY, Universidad de Salamanca, https://transcend.usal.es/deliverable-3-1-white-paper-methods-models-for-assessing-policy-performance-under-deep-uncertainty/ (last access: 1 February 2024), 2023.
Graveline, N.: Combining flexible regulatory and economic instruments for agriculture water demand control under climate change in Beauce, Water Resour. Econ., 29, 100143, https://doi.org/10.1016/j.wre.2019.100143, 2020.
Graveline, N. and Merel, P.: Intensive and extensive margin adjustments to water scarcity in France's Cereal Belt, Eur. Rev. Agric. Econ., 41, 707–74, https://doi.org/10.1093/erae/jbt039, 2014.
Groves, D. G., Evan, B., Lempert, R. J., Fischbach, J. R., Nevills, J., and Goshi, B.: Developing Key Indicators for Adaptive Water Planning, J. Water Res. Plan. Man., 141, 05014008, https://doi.org/10.1061/(ASCE)WR.1943-5452.0000471, 2015.
Guillaume, J.: Designing a knowledge system for managing deep uncertainty?, International Congress on Environmental Modelling and Software, Brussels, Belgium, 7 June 2022, 12, https://scholarsarchive.byu.edu/iemssconference/2022/Stream-D/12 (last access: 1 September 2023), 2022.
Hagedorn, R., Doblas-Reyes, F. J., and Palmer, T. N.: The rationale behind the success of multi-model ensembles in seasonal forecasting – I. Basic concept, Tellus A., 57, 219–233, https://doi.org/10.1111/j.1600-0870.2005.00103.x, 2005.
Hamilton, S. H., Fu, B., Guillaume, J. H. A., Badham, J., Elsawah, S., Gober, P., Hunt, R. J., Iwanaga, T., Jakeman, A. J., Ames, D. P., Curtis, A., Hill, M. C., Pierce, S. A., and Zare, F.: A framework for characterising and evaluating the effectiveness of environmental modelling, Environ. Modell. Softw., 118, 83–98, https://doi.org/10.1016/j.envsoft.2019.04.008, 2019.
Hanasaki, N., Yoshikawa, S., Pokhrel, Y., and Kanae, S.: A global hydrological simulation to specify the sources of water used by humans, Hydrol. Earth Syst. Sci., 22, 789–817, https://doi.org/10.5194/hess-22-789-2018, 2018.
Heckelei, T., Britz, W., Heckelei, T., and Britz, W.: Positive Mathematical Programming with Multiple Data Points: A Cross-Sectional Estimation Procedure, Cah. Econ. Sociol., 57, 27–50, https://doi.org/10.3406/reae.2000.1649, 2000.
HEPEX: HEPEX. A global community in hydrological ensemble prediction, INRAE, France, https://hepex.org.au/ (last access: 1 September 2023), 2024.
Hertel, T. W. and Liu, J.: Implications of water scarcity for economic growth (OECD Environment Working Papers), Organisation for Economic Co-operation and Development, Paris, https://doi.org/10.1787/5jlssl611r32-en, 2016.
Hino, M. and Hall, J. W.: Real Options Analysis of Adaptation to Changing Flood Risk: Structural and Nonstructural Measures, ASCE-ASME Journal of Risk and Uncertainty in Engineering Systems, Part A: Civil Engineering, 3, 04017005, https://doi.org/10.1061/AJRUA6.0000905, 2017.
Howitt, R. E.: Positive Mathematical Programming, Am. J. Agr. Econ., 77, 329–342, https://doi.org/10.2307/1243543, 1995.
IPCC: IPCC Sixth Assessment Report (AR6) (No. WGII), Intergovernmental Panel on Climate Change, Geneva, Switzerland, https://www.ipcc.ch/assessment-report/ar6/ (last access: 1 October 2023), 2021.
IPCC: IPCC Fifth Assessment Report (AR5) (No. WGII), Intergovernmental Panel on Climate Change, Geneva, Switzerland, https://www.ipcc.ch/assessment-report/ar5/ (last access: 1 October 2023), 2014.
ISIMIP: The Inter-Sectoral Impact Model Intercomparison Project, The Inter-Sectoral Impact Model Intercomparison Project, https://www.isimip.org/gettingstarted/data-access/ (last access: 1 September 2023), 2023.
Júdez, L., Chaya, C., Martínez, S., and González, A. A.: Effects of the measures envisaged in “Agenda 2000” on arable crop producers and beef and veal producers: an application of Positive Mathematical Programming to representative farms of a Spanish region, Agr. Syst., 67, 121–138, https://doi.org/10.1016/S0308-521X(00)00051-2, 2001.
Knight, F. H.: Risk, Uncertainty and Profit, University of Illinois at Urbana–Champaign's Academy for Entrepreneurial Leadership Historical Research Reference in Entrepreneurship, https://ssrn.com/abstract=1496192 (last access: 1 November 2023), 1921.
Krüger, F.: Survey-based forecast distributions for Euro Area growth and inflation: ensembles versus histograms, Empir. Econ., 53, 235–246, https://doi.org/10.1007/s00181-017-1228-3, 2017.
Krysanova, V., Hattermann, F., and Wechsung, F.: Development of the ecohydrological model SWIM for regional impact studies and vulnerability assessment, Hydrol. Process., 19, 763–783, https://doi.org/10.1002/hyp.5619, 2005.
Lempert, R. J.: Robust Decision Making (RDM), in: Decision Making under Deep Uncertainty: From Theory to Practice, edited by: Marchau, V. A. W. J., Walker, W. E., Bloemen, P., and Popper, S. W., Springer, Cham, Switzerland, https://doi.org/10.1007/978-3-030-05252-2, 2019.
Lempert, R. J. and Groves, D. G.: Identifying and evaluating robust adaptive policy responses to climate change for water management agencies in the American west, Technol. Forecast. Soc., 77, 960–974, https://doi.org/10.1016/j.techfore.2010.04.007, 2010.
Li, G., Hu, J., Wang, S.-W., Georgopoulos, P. G., Schoendorf, J., and Rabitz, H.: Random Sampling-High Dimensional Model Representation (RS-HDMR) and Orthogonality of Its Different Order Component Functions, J. Phys. Chem. A, 110, 2474–2485, https://doi.org/10.1021/jp054148m, 2006.
Lerma, N., Paredes, J., Solera, A., and Andreu, J.: Herramienta EvalHid para la evaluación de recursos hídricos (Manual No. 1.1), Polytechnic University of Valencia, Valencia, Spain, https://aquatool.webs.upv.es/files/manuales/evalhid/Manual_Usuario_EvalHid.pdf (last access: 1 May 2023), 2017.
Li, T., Dong, Y., and Liu, Z.: A review of social-ecological system resilience: Mechanism, assessment and management, Sci. Total Environ., 723, 138113, https://doi.org/10.1016/j.scitotenv.2020.138113, 2020.
Loch, A., Adamson, D., and Auricht, C.: (g)etting to the point: The problem with water risk and uncertainty, Water Resour. Econ., 32, 100154, https://doi.org/10.1016/j.wre.2019.100154, 2020.
MAGRAMA: Evaluación del impacto del cambio climático en los recursos hídricos y sequías en España (Report), Ministerio de Agricultura y Pesca, Alimentación y Medio Ambiente, Madrid, Spain, https://www.miteco.gob.es/content/dam/miteco/es/cambio-climatico/publicaciones/publicaciones/Memoria_encomienda_CEDEX_tcm30-178474.pdf (last access: 1 February 2023), 2017.
Maier, H. R., Guillaume, J. H. A., Van Delden, H., Riddell, G. A., Haasnoot, M., and Kwakkel, J. H.: An uncertain future, deep uncertainty, scenarios, robustness and adaptation: How do they fit together?, Environ. Modell. Softw., 81, 154–164, https://doi.org/10.1016/j.envsoft.2016.03.014, 2016.
Marchau, V. A. W. J., Walker, W. E., Bloemen, P., and Popper, S. W.: Decision Making under Deep Uncertainty: From Theory to Practice, 2019th ed., Springer, Cham, Switzerland, https://doi.org/10.1007/978-3-030-05252-2, 2019.
Martínez-Dalmau, J., Gutiérrez-Martín, C., Expósito, A., and Berbel, J.: Analysis of Water Pricing Policy Effects in a Mediterranean Basin Through a Hydroeconomic Model, Water Resour. Manag., 37, 1599–1618, https://doi.org/10.1007/s11269-023-03446-8, 2023.
Mírame-IDEDUERO: Visor Mírame-IDEDUERO, Valladolid, España, https://mirame.chduero.es/chduero/viewer (last access: 1 October 2023), 2023.
OECD: Toolkit for Water Policies and Governance: Converging Towards the OECD Council Recommendation on Water (Report), OECD Publishing, Paris (France), ISBN 978-92-64-87648-4, 2021.
Oleson, K. W., Lawrence, D. M., Bonan, G. B., Drewniak, B., Huang, M., Levis, S., Li, F., Riley, W. J., Swenson, S. C., Thornton, P. E., Bozbiyik, A., Fisher, R., Heald, C. L., Kluzek, E., Lamarque, F., Lawrence, P. J., Leung, L. R., Muszala, S., Ricciuto, D. M., Sacks, W., Sun, Y., Tang, J., and Yang, Z.-L.: Technical Description of version 4.5 of the Community Land Model (CLM), Ncar Technical Note NCAR/TN-503+STR, National Center for Atmospheric Research, Boulder, CO, 422 pp., https://doi.org/10.5065/D6RR1W7M, 2013.
Pande, S. and Sivapalan, M.: Progress in socio-hydrology: a meta-analysis of challenges and opportunities, WIREs Water 4, 1193, https://doi.org/10.1002/wat2.1193, 2017.
Paris, Q.: PQP, PMP, parametric programming and comparative statics, in: Lecture for notes for AE, edited by: Department of Agricultural Economics, University of California, Davis, 1988.
Parrado, R., Pérez-Blanco, C. D., Gutiérrez-Martín, C., and Gil-García, L.: To charge or to cap in agricultural water management. Insights from modular iterative modeling for the assessment of bilateral micro-macro-economic feedback links, Sci. Total Environ., 742, 140526, https://doi.org/10.1016/j.scitotenv.2020.140526, 2020.
Pérez-Blanco, C. D. and Gutiérrez-Martín, C.: Buy me a river: Use of multi-attribute non-linear utility functions to address overcompensation in agricultural water buyback, Agr. Water Manage., 190, 6–20, https://doi.org/10.1016/j.agwat.2017.05.006, 2017.
Pérez-Blanco, C. D., Gil-García, L., and Saiz-Santiago, P.: An actionable hydroeconomic Decision Support System for the assessment of water reallocations in irrigated agriculture. A study of minimum environmental flows in the Douro River Basin, Spain, J. Environ. Manage., 298, 113432, https://doi.org/10.1016/j.jenvman.2021.113432, 2021a.
Pérez-Blanco, C. D., González-López, H., and Hrast-Essenfelder, A.: Beyond piecewise methods: Modular integrated hydroeconomic modeling to assess the impacts of adaptation policies in irrigated agriculture, Environ. Modell. Softw., 136, 104943, https://doi.org/10.1016/j.envsoft.2020.104943, 2021b.
Pérez-Blanco, C. D., Parrado, R., Essenfelder, A. H., Bodoque, J., Gil-García, L., Gutiérrez-Martín, C., Ladera, J., and Standardi, G.: Assessing farmers' adaptation responses to water conservation policies through modular recursive hydro-micro-macro-economic modeling, J. Clean. Prod., 360, 132208, https://doi.org/10.1016/j.jclepro.2022.132208, 2022.
Peters, B. G. and Nagel, M. L.: Zombie Ideas: Why Failed Policy Ideas Persist, 1st edn., Cambridge University Press, Cambridge New York Port Melbourne New Delhi Singapore, https://doi.org/10.1017/9781108921312, 2020.
Pindyck, R.: The Use and Misuse of Models for Climate Policy (No. w21097), Nat. Bur. Ec., Cambridge, MA, https://doi.org/10.3386/w21097, 2015.
PUV: Manuals – AquaTool, Universidad Politécnica de Valencia, https://aquatool.webs.upv.es/aqt/en/manuals/ (last access: 1 September 2021), 2020.
Puy, A., Sheikholeslami, R., Gupta, H. V., Hall, J. W., Lankford, B., Lo Piano, S., Meier, J., Pappenberger, F., Porporato, A., Vico, G., and Saltelli, A.: The delusive accuracy of global irrigation water withdrawal estimates, Nat. Commun., 13, 3183, https://doi.org/10.1038/s41467-022-30731-8, 2022.
Quiggin, J.: Zombie economics: how dead ideas still walk among us, Pbk, Princeton University Press, Princeton, ISBN 978-1-4008-4208-7, 2012.
Ronneberger, K., Berrittella, M., Bosello, F., and Tol, R. S. J.: KLUM@GTAP: Introducing Biophysical Aspects of Land-Use Decisions into a Computable General Equilibrium Model a Coupling Experiment, Environ. Model Assess., 14, 149–168, https://doi.org/10.1007/s10666-008-9177-z, 2009.
Saltelli, A.: A short comment on statistical versus mathematical modelling, Nat. Commun., 10, 3870, https://doi.org/10.1038/s41467-019-11865-8, 2019.
Saltelli, A., Ratto, M., Andres, T., Campolongo, F., Cariboni, J., Gatelli, D., Saisana, M., and Tarantola, S.: Global Sensitivity Analysis, The Primer, 1st edn., Wiley, 237–275, https://doi.org/10.1002/9780470725184, 2008.
Sapino, F., Pérez-Blanco, C. D., Gutiérrez-Martín, C., García-Prats, A., and Pulido-Velazquez, M.: Influence of crop-water production functions on the expected performance of water pricing policies in irrigated agriculture, Agr. Water Manage., 259, 107248, https://doi.org/10.1016/j.agwat.2021.107248, 2022a.
Sapino, F., Pérez-Blanco, C. D., and Saiz-Santiago, P.: A Hydro-Economic Model to Calculate the Resource Costs of Agricultural Water Use and the Economic and Environmental Impacts of Their Recovery, Water Economics and Policy, 08, 2240012, https://doi.org/10.1142/S2382624X22400124, 2022b.
Stacke, T. and Hagemann, S.: Development and evaluation of a global dynamical wetlands extent scheme, Hydrol. Earth Syst. Sci., 16, 2915–2933, https://doi.org/10.5194/hess-16-2915-2012, 2012.
Storlie, C. B., Swiler, L. P., Helton, J. C., and Sallaberry, C. J.: Implementation and evaluation of nonparametric regression procedures for sensitivity analysis of computationally demanding models, Reliab. Eng. Syst. Safe., 94, 1735–1763, https://doi.org/10.1016/j.ress.2009.05.007, 2009.
Takata, K., Emori, S., and Watanabe, T.: Development of the minimal advanced treatments of surface interaction and runoff, Global Planet. Change, 38, 209–222, https://doi.org/10.1016/S0921-8181(03)00030-4, 2003.
Taner, M.Ü., Ray, P., and Brown, C.: Incorporating Multidimensional Probabilistic Information Into Robustness-Based Water Systems Planning, Water Resour. Res., 55, 3659–3679, https://doi.org/10.1029/2018WR022909, 2019.
Tebaldi, C. and Knutti, R.: The use of the multi-model ensemble in probabilistic climate projections, Philos. T. R. Soc. A, 365, 2053–2075, https://doi.org/10.1098/rsta.2007.2076, 2007.
UNDRR: Global Assessment Report on Disaster Risk Reduction (GAR), United Nations Office for Disaster Risk Reduction, Geneva, Switzerland, ISBN 978-92-1-004180-5, 2019.
UNDRR: GAR Special Report on Drought 2021 – World (Special Report on Drought), United Nations Office for Disaster Risk Reduction, Geneva, Switzerland, ISBN 9789212320274, 2021.
UNEP: Adaptation Gap Report 2021, No. 2021, United Nations Environment Programme, New York, USA, ISBN 978-92-807-3895-7, 2021.
US Army Corps of Engineers: HEC-HMS Hydrologic Modeling System. User's Manual – Version 4.1, User's manual No. CPD-74A v. 4.1, US Army Corps of Engineers, Davis, California, USA, https://www.hec.usace.army.mil/software/hec-hms/documentation/HEC-HMS_Users_Manual_4.1.pdf (last access: 1 September 2023), 2015.
van Beek, L. and Bierkens, M. M.: The global hydrological model PCR-GLOBWB: conceptualization, parameterizaction and verification, Report Department of Physical Geography, Utrecht University, Utrecht, the Netherlands, http://vanbeek.geo.uu.nl/suppinfo/vanbeekbierkens2009.pdf (last access: 1 May 2023), 2009.
Walker, W. E., Harremoës, P., Rotmans, J., Van Der Sluijs, J. P., Van Asselt, M. B. A., Janssen, P., and Krayer Von Krauss, M. P.: Defining Uncertainty: A Conceptual Basis for Uncertainty Management in Model-Based Decision Support, Integrat. Ass., 4, 5–17, https://doi.org/10.1076/iaij.4.1.5.16466, 2003.
Ward, F. A.: Hydroeconomic Analysis to Guide Climate Adaptation Plans, Front. Water, 3, 681475, https://doi.org/10.3389/frwa.2021.681475, 2021.
Watanabe, M., Suzuki, T., O'ishi, R., Komuro, Y., Watanabe, S., Emori, S., Takemura, T., Chikira, M., Ogura, T., Sekiguchi, M., Takata, K., Yamazaki, D., Yokohata, T., Nozawa, T., Hasumi, H., Tatebe, H., and Kimoto, M.: Improved Climate Simulation by MIROC5: Mean States, Variability, and Climate Sensitivity, J. Climate, 23, 6312–6335, https://doi.org/10.1175/2010JCLI3679.1, 2010.
Young, R. A., Onstad, C. A., Bosch, D. D., and Anderson, W. P.: AGNPS: A nonpoint-source pollution model for evaluating agricultural watersheds, J. Soil Water Conserv., 44, 168–173, 1989.
Walker et al. (2003) identify different levels across the uncertainty spectrum: (1) determinism (where point predictions are reliable), (2) probabilistic risk (we know which plausible futures lie ahead of us as well as the associated probabilities), (3) (deep) uncertainty type 1 (we do not know which inputs, parameters, and/or model structures are right, nor do we know their probability, but we can anticipate how the system will react to these), (4) (deep) uncertainty type 2 (we know we do not know), and (5) complete ignorance (we are not aware of what we do not know). Knightian uncertainty would fall into levels 4–5, which precludes modelling. Deep uncertainty type 1 can be modelled and has been modelled by model intercomparison projects such as AGMIP (2023), CMIP6 (2023), HEPEX (2024), and ISIMIP (2023).
The European Union (EU) uses a system called NUTS (Nomenclature des Unités Territoriales Statistiques) to categorize its economic regions. In Spain, NUTS2 levels correspond to regional divisions (Eurostat, 2020).
- Abstract
- Introduction
- Case study area: the Douro River basin
- Methods: a modular hierarchy of socio-ecological ensembles
- Discussion and conclusions
- Appendix A: New infrastructures in the Douro River Basin Plan
- Appendix B: Spatial distribution of profit and employment for each ensemble model
- Data availability
- Author contributions
- Competing interests
- Disclaimer
- Special issue statement
- Financial support
- Review statement
- References
- Supplement
- Abstract
- Introduction
- Case study area: the Douro River basin
- Methods: a modular hierarchy of socio-ecological ensembles
- Discussion and conclusions
- Appendix A: New infrastructures in the Douro River Basin Plan
- Appendix B: Spatial distribution of profit and employment for each ensemble model
- Data availability
- Author contributions
- Competing interests
- Disclaimer
- Special issue statement
- Financial support
- Review statement
- References
- Supplement