the Creative Commons Attribution 4.0 License.
the Creative Commons Attribution 4.0 License.
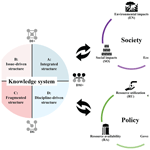
Impacts of science on society and policy in major river basins globally
Shuanglei Wu
Radical transformations of knowledge development are required to address the sustainability issues in the Anthropocene. This study developed a framework to understand the internal structures of knowledge development with two dimensions: degree of multidisciplinarity and degree of issue connectivity. Examining the knowledge development in 72 river basins globally from 1962 to 2017 using the Web of Science (WoS) dataset, it was found that the river basin knowledge systems were characterized by increasingly interconnected issues addressed by limited disciplines. Evaluating these structural characteristics against six impact indicators of society and policy, over 90 % of rivers were found to have knowledge structures that were strongly linked to society impacts, whereas only 57 % were linked to that of policy. Optimization analysis further found that about 35 % of the rivers studied mostly in Asia, Africa, and South America were prone to fragmented knowledge structures that had limited capacities to effectively address the issues with negative environmental impacts and resource depletion. Improving multidisciplinary research is the key to transforming the current knowledge structure to support more sustainable river basin development.
- Article
(12360 KB) - Full-text XML
- BibTeX
- EndNote
Science is often called upon to provide solutions to societal problems and acts as a common ingredient of policy making. However, the exponential development of science and technology, with its irreversible environmental and social side effects, is pushing the Earth's safe operating space close to its planetary boundaries (Steffen et al., 2015; Brey, 2018). Therefore, radical transformations of knowledge (science and technology) development are required to meet the rapidly changing societal needs in the Anthropocene (Norström et al., 2020; Hakkarainen et al., 2022).
Advancing knowledge management and assessment is a key to radical transformations of knowledge development. Current studies on knowledge management and assessment mainly rely on intellectualism-related indicators (e.g., research and development inputs and number of scientific papers and patents) with several evaluation tools (e.g., bibliometric studies, case study analysis, and patent benchmarking) (Penfield et al., 2013). They tend to focus on the quality of scientific outputs, i.e., the credible, legitimate, and relevant criteria of good science (Cash et al., 2003; Posner and Cvitanovic, 2019). While these studies have provided fruitful insights into how science has produced impacts, on the one hand, due to a lack of generalized findings, they have limited applicability beyond their case study areas; on the other hand, due to the large negligence of the structural dynamics of the knowledge system, they have failed to answer how different disciplinary knowledge interacts to address increasingly complex issues that may significantly impact the society and policy-making (Weichselgartner and Kasperson, 2010; Hakkarainen et al., 2020). Without understanding and addressing the possible structural failure of knowledge development, we would not be in a position to direct knowledge transformations (Wu et al., 2021; Wei et al., 2022; Newig and Rose, 2020).
This study developed a framework to understand the internal structure of knowledge development and evaluated the impacts of these structural dynamics on society and policy with this framework, thus contributing to structurally reconfiguring the knowledge systems for addressing complex sustainability issues. The framework was empirically applied to knowledge development on 72 river basins across the world from 1962 to 2017 using publications from the Web of Science (WoS) dataset. Knowledge development in river basins was chosen as an example because water is a key input for almost all economic activities, with broad impacts on both society and policy (Rodríguez et al., 2021), and river basins are logical spatial units which help understand the water cycle within the Earth system (Warner et al., 2008).
2.1 A network-based framework to measure the structure of a knowledge system
Built on the Science of Science (SoS) theory (Zeng et al., 2017), a knowledge system is understood as a dynamic system, consisting of knowledge from different disciplines and issues studied, with complex and co-evolving relationships between them; as Latour (1987) described it, it is “knitting, weaving and knotting together into an overarching scientific fabric” (Latour, 1987; Shi et al., 2015). We adopt a network-based framework to evaluate such interactions (Wei et al., 2022; Wu et al., 2021; Sayles and Baggio, 2017; Coccia, 2020). We characterize the knowledge system as a discipline–issue network, where connections are established between issues and the disciplines used to address the issues (Noyons, 2001; Callon et al., 1983). To further examine the impacts of knowledge development, the discipline–issue networks are projected into issue networks, where issues are connected if they are studied by the same discipline. We use two dimensions to capture the topological structure of a knowledge system (Wasserman and Faust, 1994; Borgatti, 2005; Zeng et al., 2017). The first is the degree of multidisciplinarity (DM), which indicates the proportion of disciplines engaged in different issues and is measured as the density of the discipline–issue network (the ratio between the actual number of connections and the maximum possible number of connections in the network) (Eq. 1). The following applies for any discipline–issue network i:
where DMi is the degree of multidisciplinarity value of a discipline–issue network i, Cd is the total number of existing connections between any issue and discipline d in the network, and n is the total number of disciplines in the network. This dimension recognizes the importance of disciplinary diversity in sustainability issues (Norström et al., 2020; Cockburn, 2022; Stirling, 2007). The higher the DM, the more disciplines involved and the more multidisciplinary the knowledge system.
The second is the degree of issue connectivity (DI). It indicates how many different issues are studied in an interconnected manner and is measured as the degree centrality (Wasserman and Faust, 1994; Borgatti, 2005) of the issue network (Eq. 2). The following applies for any issue network i:
where DIi is the degree of issue connectivity of an issue network i, Cm is the number of adjacent connections to any specific issue m, and n is the total number of specific issues in the network. This dimension recognizes the increasing complexity in sustainability issues and the importance of understanding these issues in an interactive manner (Burmaoglu et al., 2019; Okamura and Nishijo, 2020). The greater the DI, the more interconnected the issues and the more centralized the knowledge system.
To compare the relative differences in DM and DI among rivers, the z scores for DM and DI () in any river k are calculated by subtracting the means () and then dividing by the standard deviation (σk) of all rivers. Four types of knowledge structures are defined as follows (Fig. 1): (A) integrated knowledge structure (; ), with diverse disciplines engaged in interconnected issues; (B) issue-driven knowledge structure (; ), with limited disciplines engaged in interconnected issues; (C) fragmented knowledge structure (; ), with limited disciplines engaged in isolated issues; and (D) discipline-driven knowledge structure (; ), with diverse disciplines engaged in isolated issues. An integrated knowledge structure is considered ideal in studying highly interconnected issues with diverse disciplines, while a fragmented structure is at the other end of the spectrum, where both issues and disciplines are working in silos. An issue-driven knowledge structure tends to provide disciplinary-specific solutions for interconnected issues, which are often cost-effective in the short term but may lead to unintended or unexpected outcomes in the long term due to the narrow perspective of the limited number of disciplines. A discipline-driven knowledge structure tends to provide trans-disciplinary solutions for key issues of focus, which are often not cost-effective in the short term, as it often takes a long time and requires large investments to find a solution, but are more sustainable in the long term. In time, knowledge development may demonstrate different structural pathways – for example, moving from the under-developed fragmented structure to a discipline-driven structure and/or from an issue-driven structure towards an integrated one.
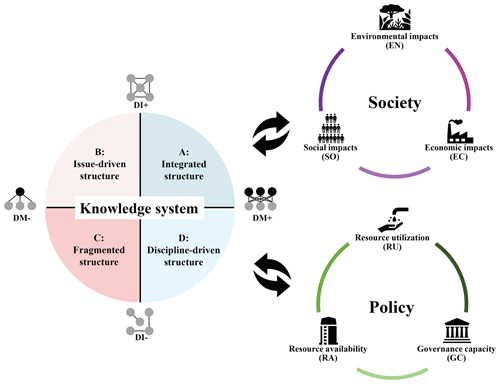
Figure 1A framework to understand the knowledge system and its impacts on society and policy in natural resources management.
We apply our framework to evaluate the impacts of knowledge development. The commonly recognized triple-bottom-line framework is adopted to define the impacts of the knowledge system on society (Reyers and Selig, 2020), which include the social (SO), economic (EC), and environmental (EN) dimensions. We then uniquely define the impacts of the knowledge system on policy according to the whole-of-system characteristics in natural resources management, covering resource availability (RA), resource utilization (RU), and governance capacity (GC) (Wei et al., 2018; Ostrom, 2009). Resource availability refers to the supply capacity of natural resources, resource utilization reflects the extent to which a resource is used, and governance capacity indicates the government's regulation of the supply and demand of a resource (Fig. 1).
2.2 Data collection and processing
2.2.1 The river basin knowledge system
The river basin knowledge system was represented by peer-reviewed articles indexed in the Web of Science (WoS) dataset. Archiving over 21 000 high-quality scholarly journals, the WoS is one of the largest databases that documents knowledge development since 1900. It provides up-to-date, consistent classifications of knowledge under the Master Journal List (https://mjl.clarivate.com/home, last access: 22 June 2024), which classifies articles according to their source journals into 254 disciplines under five research areas: arts and humanities, life sciences and biomedicine, physical sciences, social sciences, and technology (Web of Science, 2021).
Articles with “drainage basin” OR “river basin” OR “valley” OR “hydrographic basin” OR “watershed” OR “catchment” OR “wetland” in their titles, abstracts, and keywords were collected from 1900 to 2017. Four types of information were extracted from each article: the disciplines, year of publication, keywords, and river basin studied. The discipline and year of publication for each article were automatically assigned based on their source journals. For journals with multiple disciplines, only the first, most dominant discipline was assigned. A total of 215 disciplines were identified (see Table A1 for a full list).
The keywords were extracted, filtered, and tokenized from the titles, abstracts, and keywords of the articles using the natural language processing (NLP) module in the Derwent Data Analyzer (https://clarivate.com/derwent/zh-hans/solutions/derwent-data-analyzer-automated-ip-intelligence/, last access: 16 July 2021). Those keywords related to the methodologies of the articles were removed, and those remaining were manually regrouped into the 94 issue groups that broadly represent major topics of river basin research and management (e.g., agriculture, pollution, and climate change; see Table A2 for a full list and also refer to Wei and Wu, 2022, for more details on the grouping of the keywords).
Each article was also assigned a river basin on which it was used as a case study. All articles without a clear indication of case river basins and duplicate articles were removed. Initially, the top 100 most-published-about river basins were selected. Removing those with ambiguous river basin names and those river basins with unenclosed coastal shorelines that lack country-level data, a total of 72 river basins, covering major river basins in the world, were finally selected. The river basins were selected based on the volumes of scientific publications to ensure that major river basins with high socio-economic and environmental significance were covered. At least one river basin in each of the continents was included for the spatial representativeness of the study. Exactly 165 044 discipline–issue connections, with the number of articles counted as the weight of connections, were also identified. These connections were used to construct the discipline–issue network and the issue network for each of the 72 river basins for analysis.
2.2.2 Indicators to represent society and policy
We chose the indicators for society and policy based on the following principles: (1) quantitative expression, (2) reflection on system processes rather than end states, (3) data availability, and (4) specific focus on impacts related to water resources. For society, the economic impact was defined by water productivity, which stands for the economic value generated by water resource use. The societal impact was represented by the population to show the total size of human demand for water resources, and the environmental impact was a negative indicator of water stress. Greater water stress indicated greater negative impacts on the environment. For the policy, resource availability was represented by the percentage of cultivated land. While precipitation and runoff are commonly recognized as key indicators of water resource availability, we selected cultivated land as its change was more influenced by water resources management. It was a negative indicator, meaning that increasing cultivated land increased water resource use, thus reducing the availability of water resources. Resource utilization was represented by total freshwater withdrawals to indicate the size of water use, and governance capacity was represented by a normalized government effectiveness index that gauged the abilities of policy implementation.
Data on the indicators for both the society and the policy were collected from the AQUASTAT database by the Food and Agriculture Organization (FAO), the World Bank, and the Socioeconomic Data and Applications Centre (SEDAC). In particular, population and water withdrawal data have been improved by Yan et al. (2022) by combining FAO and SEDAC databases and local government archives with extended temporal and spatial scales, which was adopted in this study. The chosen indicators, with brief descriptions and corresponding temporal and spatial scales, are summarized in Table 1.
To aggregate the different spatial scales of data into a unified river basin scale, the boundaries of the 72 river basins were defined. Boundaries of 26 river basins were identified as transboundary and collected from the Transboundary Waters Assessment Programme (TWAP). The basin boundaries of the remaining 46 river basins located entirely within single countries were collected from corresponding national records (e.g., the US Geological Survey and the Murray–Darling Basin Authority). For each transboundary river basin, a basin area ratio was calculated as the weighted proportion of the river basin area to the population for each country found within the boundary of the river basin. The country-level indicators were multiplied by the basin area ratio and then aggregated by the average values for all spanning countries in the basins. For river basins located entirely within single countries, the country-level indicators were assumed to be the same within the basin boundaries. All gridded level indicators were clipped based on the basin boundaries and averaged across the basin area using ArcGIS Pro 3.0. Finally, missing values at the country level in time were imputed by linearly interpolating the missing values based on the regression relationship between the existing values in the time series. For the government effectiveness index, which was not available before 1996, values were assumed to be the same as in the first available year.
The study period from 1962 to 2017 at 5-year intervals was used. This study period was chosen to reflect the history of water resources development, which is closely tied to rapid socio-economic development, environmental deterioration, and a governance system transitioning from technocratic top-down control to collaborative integrated management (Molle, 2009). Also, there was limited data availability on society and policy on a global scale before 1962.
2.3 Approaches to analysis
2.3.1 Time-trend analysis for indicators of the knowledge system and its impacts
The Mann–Kendall test was used to test if there exist statistically significant, monotonically increasing or decreasing trends in the time series for the knowledge system and its impacts (Mann, 1945; Kendall, 1975). Significant trends were identified with a two-sided t test with a p value of <0.05.
Sen's slopes (Sen, 1968) were then used to measure the magnitudes of the trends as follows:
where is the median value separating the higher 50 % from the lower 50 % of the indicator value x in the time series, i and j are adjacent time points, and n is the total number of time points. As a non-parametric measure, Sen's slope is insensitive to outliers and autocorrelations in the time series and does not require data that satisfy the normality assumption, thus providing a robust measure of the time trends for indicators with varying scales and limited amounts of data (Wang et al., 2020; Fernandes and Leblanc, 2005).
2.3.2 Measuring the knowledge system impacts
To compare the impacts on society and policy on different scales, z scores were calculated to normalize the values of the knowledge indicators (i.e., DM and DI) and the society and policy indicators over their time series (Eq. 4).
The following applies for any river basin k and any knowledge, societal, and policy indicator x:
where is the z score of any knowledge, societal, and policy indicator of xk; is the mean value; and σk is the standard deviation.
Generalized linear regression models were used to quantify the relationships between the normalized society and policy indicators as dependent variables and the knowledge system indicators as independent variables using the following equations, Eqs. (5) and (6):
where α and β are the normalized coefficients representing the partial influences which DM and DI have on river basin k relate to a particular society indicator, x, or a particular policy indicator, y, and ε is the random error term that captures the biased values. Models that failed to pass the two-sided t test with a p value of >0.05 and/or with adjusted R2<0.3 were rejected (Royston, 2007; Ratner, 2009). This threshold value was selected to ensure that at least weak regression relationships were identified between the knowledge structural indicators and the society and policy indicators and has been commonly adopted in studies on correlations between knowledge and environmental practices (Afroz and Ilham, 2020; Alias, 2019; Hernanda et al., 2023).
We recognized that the society and policy indicators can be influenced by a wide range of factors. Therefore, these statistical models were not developed for causal inferences. We rather focused on the comparative knowledge impacts associated with different river basin biophysical and socio-political contexts.
2.3.3 Determining the patterns of knowledge impact
To identify the different interacting patterns between knowledge and society and between knowledge and policy, the river basins were grouped based on their regression coefficients (α and β) for the society and policy indicators, respectively. Firstly, river basins with more than two statistically non-significant linear models regarding the three society indicators and those regarding the three policy indicators were grouped separately. These river basins were identified to have knowledge systems with unclear impact patterns. Secondly, the remaining river basins were grouped using agglomerative hierarchical clustering (AHC) based on the Euclidean distances and Ward's agglomerative criterion, which was chosen as it was less prone to the randomness of clustering initiation and provided stable groupings of rivers (Murtagh and Legendre, 2014). Rivers were first clustered based on the six coefficients in the linear models with the society indicators (i.e., α and β for social, economic, and environmental impacts) and then clustered separately based on the six coefficients in models with the policy indicators (i.e., α and β for resource availability, utilization, and governance). The number of clusters was chosen to be two for the society and policy clustering, respectively, which was determined by maximizing the sum of square errors between different groups and minimizing the errors within groups.
2.3.4 Optimizing the knowledge system for its impacts
We represented the four types of knowledge–impact relationships by calculating the average of coefficients (αavg, βavg, εavg) for the linear models of the corresponding rivers in each knowledge–impact pattern group. These relationships were then used as the objective functions for multi-objective optimizations using a Non-dominated Sorting Genetic Algorithm (NSGA-II; Deb et al., 2002; Coello Coello et al., 2020) to identify the optimal DM and DI values ( and ) that simultaneously achieve the objectives specified in Table 2.
The NSGA-II algorithm was selected because it searches for the global Pareto optimality for the multiple counteractive objectives in this study (Edgeworth, 1881; Deb and Gupta, 2005). It provides a set of effective solutions that are at least as good as the other possible solutions for each objective and strictly better for at least one objective (Halffmann et al., 2022). Combining random numbers and information from previous search interactions over all potential solution points, this algorithm has been effectively used to solve multi-objective problems, particularly in engineering and decision-making optimization (Marler and Arora, 2004). Initially, 100 pairs of potential DM and DI values were randomly generated and modeled over 1000 iterations to search for the optimum values. Finally, we evaluated the trade-offs and synergies of different objectives achieved by different optimized DM and DI values to recommend tailored management strategies for future knowledge system development.
The above analyses were conducted using R version 4.2.3 (R Core Team, 2023) with the following packages: igraph (Csardi and Nepusz, 2006), imputeTS (Moritz and Bartz-Beielstein, 2017), factoextra (Kassambara and Mundt, 2020), and nsga2R (Deb et al., 2002).
3.1 Knowledge systems characterized by increasingly interconnected issues addressed by limited disciplines
The knowledge systems of the 72 river basins were characterized by a limited increase in scientific disciplines engaged (low and stabilizing DM) but an increase in interconnections among issues studied (increasing DI). Of the river basins, 47 % had positive temporal trends for DM, but only eight were statistically significant (p<0.05), most of which are located in Asia (e.g., the Nakdong River and the Yangtze River). About 40 % had negative Sen's slopes, of which only nine were statistically significant, spreading across North America, Europe, and Oceania. Moreover, both positive and negative average significant Sen's slopes only varied between 0.02 % and 0.05 % per 5-year period, with obvious stabilization of the absolute DM values between 0 and 0.25 (i.e., no more than 25 % of the different disciplines and issues was connected) for all river basins in 2017. Multidisciplinary research for global river basin studies was highly constrained within the biophysical disciplines, with over 70 % of interactions being among the environmental sciences, water resources, ecology, multidisciplinary geosciences, and marine and freshwater biology. Only about 10 % of interactions were contributed by social sciences such as human geography, economics, and management (Fig. 2a, Table A1).
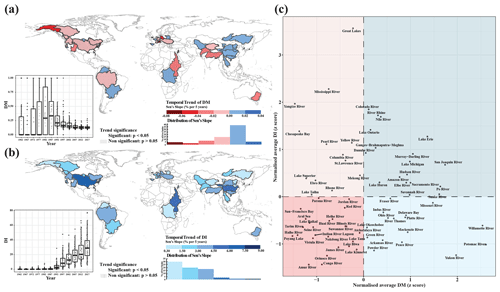
Figure 2(a) The temporal trends (Sen's slope) and the absolute values (in inset) of the degree of multidisciplinary (DM) for the 72 river basins, (b) the temporal trends (Sen's slope) and the absolute values (in inset) of the degree of issue connectivity (DI) for the 72 river basins, and (c) the knowledge system classification for the 72 river basins by their normalized average DM and DI. Dots in the box plots indicate individual DM and DI values, the box boundaries indicate the 25th and 75th percentiles, the center line indicates median values, and the whiskers indicate a value that is 1.5 times that of the interquartile range.
On the other hand, all river basins demonstrated statistically significant increasing trends for DI (p<0.05). The top five river basins with the greatest positive trends were the Great Lakes, the Mississippi River, the Yangtze River, the Nile River, and the Chesapeake Bay, with an average Sen's slope increase of 6 % per 5-year period, which was about 12 times greater than the bottom five river basins. The Murray–Darling River had an increasing trend of 3.8 % per 5-year period as the only river basin studied in Oceania, followed by the European river basins with an average increasing trend of 2.7 %. We found that 50 % of the river basins had absolute DI values between 20 and 40 (i.e., the average number of issue interconnections in the knowledge system), and their highest DI value reached nearly 80 (i.e., the Great Lakes) in 2017. About 40 % of issue connections were between ecological degradation and restoration and pollution and treatments, followed by similar connections between management and control, agriculture and irrigation, flood and drought management, and climate change and population, each at about 4 %–5 % (Fig. 2b, Table A2).
Classifying the knowledge structures of river basins based on their normalized DM and DI values indicates that 35 % of the river basins, located mostly in Asia, had fragmented knowledge structures with low DM and low DI. Furthermore, 25 % of the river basins had integrated knowledge systems with relatively high DM and DI values, including the Murray–Darling River, the Colorado River, the Amazon River, the Nile River, and most of the European rivers. Most of the discipline-driven rivers are located in North America, whereas there are major Asian and North American river basins (e.g., the Yellow River, the Yangtze River, the Mekong River, the Mississippi River, and the Columbia River) with issue-driven knowledge systems (Fig. 2c).
3.2 Unequal development of the society and policy indicators among the 72 river basins
We then examined the development of the society and policy indicators in the 72 river basins by investigating the change trends in absolute values and their relative proportions among the three society indicators and among the three policy indicators, respectively. The impacts of SO (i.e., increasing populations) dominated among the society indicators (over 60 % of the relative proportions) of the African and South American river basins. These basins also had the greatest increases in the absolute SO values on average (an average Sen's slope of 25 % per 5-year period, same thereafter), and the South American river basins also had the greatest increase in the EN values on average (13.7 %) (i.e., increasing water stress). For Asian river basins, SO contributed to over 50 % of proportions among the society indicators and less than 20 % was contributed by EC (i.e., increasing water productivity), yet, mostly by the Yangtze River, the Pearl River, and the Yellow River (average 100 % increase), they had the greatest absolute EC increase at over 60 % per 5-year period on average, whereas other basins like the Ganges–Brahmaputra–Meghna Basin, the Mekong River, and the Jordan River only increased by 10 % on average. Most of the European and North American river basins had relatively stable development of their society indicators, characterized by low SO (0 %–40 %) and balanced EC (30 %–60 %) and EN (50 %–70 %). European river basins demonstrated the lowest absolute increase in SO at less than 3 %, and all European and North American river basins studied had decreasing trends (−2.4 % and −1.2 % on average) in EN (i.e., reducing waster stress) (Figs. 3a and B1).
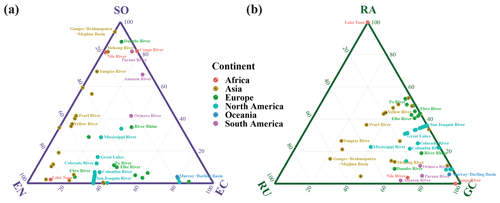
Figure 3(a) The relative average values of the social (SO), economic (EC), and environmental (EN) indicators and (b) the relative average values of the resource availability (RA), utilization (RU), and governance (GC) indicators for the 72 river basins. Only the top five most-published-about river basins in Asia, Europe, and North America and all rivers in Africa, South America, and Oceania were labeled.
Lake Tana in Africa demonstrated the greatest relative proportions in RA (i.e., area of cultivated area to indicate reduced water availability) among the three policy indicators, whereas the greatest absolute increases in RA were observed for the South American (9.1 %) river basins. Most river basins studied (African, European, North American, South American, and the Murray–Darling Basin in Oceania) had similarly lower RU (i.e., freshwater withdrawal amount) (0 %–40 % of relative proportions) and higher GC (i.e., governance effectiveness) (50 %–100 %). Among them, the African river basins had the greatest absolute RU increase at 43 % on average, whereas non-significant change trends in GC were observed for over 60 % of the rivers. Although the Asian river basins had comparatively lower GC (20 %–50 %), a significant increase of 2.4 % per 5-year period on average was identified (Figs. 3b and B2).
3.3 Knowledge structures are more strongly linked to society than to policy indicators
The structural characteristics of the knowledge systems have been strongly linked to the society indicators with over 90 % river basins had acceptable regression model fits, but the link with the policy indicators is much weaker as only 41 river basins had two or more linear models that validated the relationships between their knowledge systems and the policy indicators (adjusted R2>0.3; statistical significance p<0.05).
Additionally, 69 % river basins mostly in North America, Europe, and the Murray–Darling River in Oceania were identified to have a pattern of knowledge for environment (KFE), of which increases in DM and DI corresponded to decreases in the EN (an inverse indicator of water stress). For river basins with this pattern, relationships with the SO were generally positive (median DM=0.10 and DI=0.74 and the same thereafter), and trade-off relationships between the DM (−0.02) and DI (0.92) with the EC were also identified. Also, 21 river basins, located mostly in Asia, Africa and South America, were identified to have a pattern of knowledge against environment (KAE). These river basins had strong positive relationships of DM (0.12) and DI (0.93) with EN, SO (DM=0.28; DI=0.72), and EC (DM=0.02; DI=0.90). Only the DM and DI of Lake Kinneret had insignificant correlations with the EN and EC indicators, and the lake was grouped into a separate group identified as “unclear knowledge–society interaction” (Figs. 4a and b and B3).
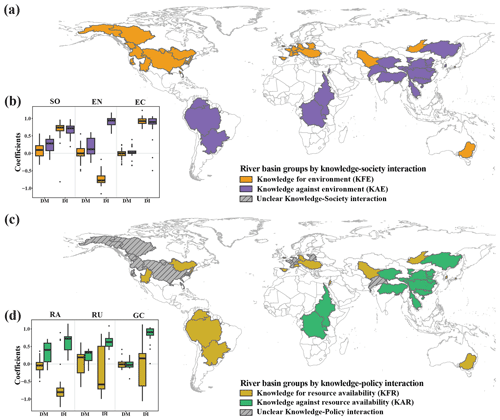
Figure 4(a) The 72 river basins classified based on the coefficients of the linear models between the knowledge structural indicators and the society indicators and (b) the distributions of the DM and DI coefficients for valid linear models. (c) The 72 river basins classified based on the coefficients of the linear models between the knowledge structural indicators and the policy indicators and (d) the distributions of the DM and DI coefficients for valid linear models. Dots in the box plots indicate individual DM and DI coefficients in the linear models, the box boundaries indicate the 25th and 75th percentiles, the center line indicates the median value, and the whiskers indicate a value that is 1.5 times that of the interquartile range.
In total, 25 river basins spreading across North America, Asia, South America, and Oceania had a pattern of knowledge for resource availability (KFR). These rivers demonstrated negative relationships of DM (−0.04) and DI (−0.80) with RA (an inverse indicator of cultivated land). There were also trade-off relationships of DM and DI with RU (DM=0.19; ) and with GC (; DI=0.16). However, 16 rivers in Asia and Africa had a pattern of knowledge against resource availability (KAR), which tended to have strong positive relationships of DM and DI with RA (DM=0.40; DI=0.72) and RU (DM=0.32; DI=0.63) and trade-off relationships (; DI=0.91) with GC. The remaining 31 river basins, located mostly in North America, were identified to have “unclear knowledge–policy interaction”. Further, the impacts of DI were generally stronger and statistically significant, whereas the impacts of DM were much weaker and tended to be insignificant (Figs. 4c and d and B4).
3.4 Optimizing the knowledge structures for improved society and policy impacts
Mapping the river basins' knowledge system classifications with their society and policy impact patterns, it was found that river basins with integrated knowledge structures tended to have KFE (83 % of rivers with integrated structures, same thereafter) and KFR (50 %) patterns. The issue-driven river basins tended to have KFE (61 %) and KAR (38 %) patterns, whereas the discipline-driven river basins were dominated by the KFE (94 %) and unclear knowledge–policy (75 %) patterns. River basins with fragmented knowledge structures were prone to the KAE (36 %) and KAR (48 %) patterns (Fig. 5a).
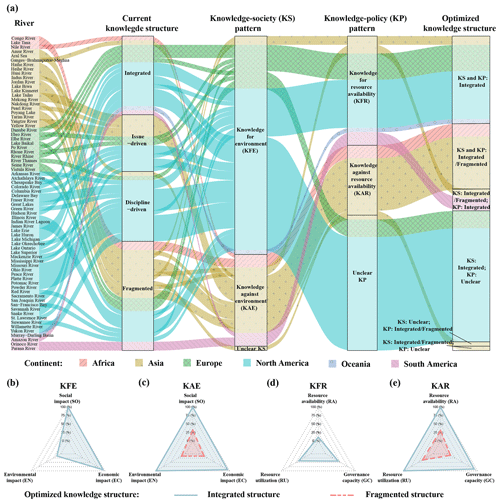
Figure 5(a) The current knowledge structure, patterns of knowledge impacts on society and policy, and the suggested knowledge structures after the optimization for the 72 river basins. The optimized society indicators for the patterns of (b) knowledge for environment (KFE) and (c) knowledge against environment (KAE) and the optimized policy indicators for the patterns of (d) knowledge for resource availability (KFR) and (e) knowledge against resource availability (KAR).
We further identified the optimal DM and DI values for river basins with each of the KFE, KAE, KFR, and KAR patterns, with the objective to maximize their positive and minimize their negative society and policy impacts (see Table C1 in Appendix C for criteria of optimization). For the KFE river basins, an integrated knowledge structure (DM=1; DI=1) should be targeted, which maximizes the SO (normalized value of 1) and EC (0.94) indicators while minimizing the negative EN indicators (0.13) (Fig. 5a and b). On the other hand, there exist trade-offs for the KAE river basins to select the optimal knowledge structure. While the integrated knowledge structure (DM=1; DI=1) could maximize the SC (1) and EC (0.93), it also maximizes the negative EN (1). A fragmented knowledge structure is optimal for minimizing the negative EN impact (0.13) but reduces positive SC (0.33) and EC (0.13) impacts (Fig. 5a and c).
For river basins with the KFR pattern, an integrated structure is optimal for minimizing the negative RA (0.14) and maintaining a balanced RU (0.41) and GC (0.43) (Fig. 5a and d). For the river basins with the KAR patterns, an integrated knowledge structure could maximize RA (1), RU (1), and GC (0.89). A fragmented knowledge structure minimizes the negative RA (0.32) and yet trades off low RU (0.36) and GC (0.10). It should also be noted that the knowledge systems for rivers with unclear knowledge–society interaction (1) and unclear knowledge–policy interaction (31) could not be optimized (Fig. 5a and e).
This study developed a framework to measure the knowledge development of 72 river basins from a quantifiable network perspective using scientific publications in the Web of Science (WoS) dataset and evaluated the impacts of knowledge on society and policy from 1962 to 2017. Our findings shed light on and help better understand river basin knowledge development.
4.1 Insufficient development of multidisciplinary research
Current knowledge structures in the 72 main river basins in the world were characterized by increasing degree of issue connectivity (DI), whereas the degree of multidisciplinarity (DM) was low and had limited growth (Fig. 2). We identified that even for river basins with discipline-driven knowledge structures, they had low values of DM and their interconnections were concentrated among biophysical disciplines, indicating the domination of natural sciences in multidisciplinary research for most river basins across the world. Additionally, the impacts of DM tended to be statistically insignificant with both society and policy indicators (Figs. 4, B3, and B4), coupled with booming populations (SO) for resource demand (i.e., large RA indicating low water availability) and low economic and resource productivity (EC and RU), along with deteriorating environment (EN) (Fig. 3). This implies that current practices of multidisciplinary research were not sufficient to solve the complex sustainability issues. Addressing many sustainability issues requires more knowledge from the human perspective to comprehend the human–nature interactions (Krausmann and Fischer-Kowalski, 2013; Jerneck et al., 2011). Drawing knowledge from social sciences (e.g., political science, sociology, management, and psychology) is the key to improving multidisciplinary research to transform the current knowledge systems of river basins. Knowledge systems for river basins could benefit from strengthening these governance-related disciplines to reconcile the relationship between individual behaviors and collective management decisions for water and coordinate the interactive relationships between socio-economic development and environmental sustainability.
4.2 Challenges of the knowledge–policy interface
Over 90 % of the river basins had knowledge structures that are strongly linked to the society indicators, but only 57 % of rivers had statistically significant relationships with the policy indicators (Fig. 4). This is closely related to the challenge knowledge transfer imposes on decision-making at the science–policy interface (Nguyen et al., 2017; Louder et al., 2021). Such a challenge has been widely recognized as policy and practice decisions are informed by diverse values and beliefs and multiple sources of knowledge and are shaped by cognitive factors and power dynamics beyond the direct influence of research activities (Hakkarainen et al., 2020; Pitt et al., 2018; Posner and Cvitanovic, 2019). We propose developing “boundary spanners” as a potential solution (Edwards and Meagher, 2020). These spanners could be creditable academic organizations for the policy community, individual or groups of scientists, or professional consultants who facilitate knowledge and information across otherwise disconnected communities and synthesize different values and insights to facilitate collective sense-making (Stovel and Shaw, 2012; Bodin, 2017). They can not only bridge disciplinary silos of natural and social scientists but are also, more importantly, able to coordinate scientists with local stakeholders and policy-makers with different levels of management power and contexts. Additionally, although beyond the scope of this study, we recognize the interactions between society and policy. In particular, the SO in society indicators and the RU in policy indicators were most strongly positively correlated (r=0.81; p<0.05) (Fig. B5), which indicates a need to recognize the connections between policy and society development and their spillover effects on knowledge in future study.
4.3 Tailored knowledge strategies based on knowledge–society–policy patterns
The integrated knowledge structure was identified to be most desirable, which links with the patterns of knowledge for environment (KFE) and the knowledge for resource availability (KFA). Issue-driven knowledge structures were identified to have similar optimized society and policy impacts to the integrated knowledge structure, whereas discipline-driven knowledge structure was not effective in optimizing multiple society and policy indicators at the same time (Figs. 5 and C1). About 15 % of the river basins studied in America, Europe and Oceania (e.g., the Amazon River, the Colorado River, the Danube River, and the Murray–Darling Basin) with integrated knowledge structures demonstrated more balanced impacts on society and policy (Fig. 5). They provide good examples for other river basins in achieving a holistic integration of science, society, and policy. On the other hand, river basins with the patterns of knowledge against environment (KAE) and the knowledge against resource availability (KAR) are considered less desirable, as optimizing the current knowledge structure to reduce the negative environmental impacts or improving resource availabilities would be traded off for socio-economic development and governance capacities (Fig. 5). Rivers with fragmented knowledge structures comprising 35 % of the river basins studied, mostly in Asia, Africa, and South America, were most prone to these impact patterns (Fig. 3). It reflects the inevitable concerns and interests of these river basins with greater development pressures and inequalities. A more balanced and integrated knowledge development approach could be supported by raising awareness of human impacts on river basins and targeted research funding that facilitate bridging the gap between science and policy (Matsumoto et al., 2020; Jabbour, 2022).
Our network-based framework contributes to advancing the Science of Science theory (Zeng et al., 2017) and transforming knowledge for more sustainable river basin development. It provides a method of explicitly measuring the structure of knowledge as a discipline–issue network system, which guides future knowledge development by identifying explicitly where and what to change or connect between disciplinary knowledge and issues at hand, therefore assisting in more suitable, more precise, and more predictable knowledge development. Moreover, our framework links the structural configurations of knowledge systems with developments in society and policy, thus contributing to better evaluation of research outcomes and action-oriented research for specifying “credible, legitimate, and relevant” criteria in good governance (Kim, 2019; Cash et al., 2003). Finally, this framework will contribute to river basin management by enabling comparisons of knowledge development for river basins with varying management issues regarding focus and context and thus also enabling the design of tailored management strategies and co-learning according to different patterns of connections among river basin knowledge, society, and policy development.
The limitations in this study and future research directions are also recognized. Regarding the data source, only scientific publications indexed in the WoS that were written in English were studied. While the WoS provides a consistent, systematic documentation of scientific knowledge development across a broad range of disciplines for a long time frame, gray literature focusing on practice-driven knowledge (e.g., conference paper, government reports) also contributes to the river basin knowledge development and can be included in future studies (Ramírez-Castañeda, 2020). Selecting case river basins based on scientific publications also led to potential bias towards large river basins with societal and natural significance, and selections of the indicators to represent the society and policy of the river basins were also bounded by the temporal and spatial data availability. Additionally, classifications of disciplines in this study were conducted based on journal assignments. It should be recognized that boundaries between disciplines are becoming increasingly blurred when used in the context of research evaluation. Most importantly, further research efforts should be made to reveal the mechanisms behind the interactive dynamics between the knowledge system and its impacts on society and policy.
To conclude, this study developed a systemic framework to evaluate the impacts of science on society and policy at a global river basin scale. Rather than using input- or output-based knowledge proxies, it directly measured the knowledge structure using network-based dimensions: degree of multidisciplinarity and degree of issue connectivity, which recognizes the diversity and complexity of sustainability issues in the Anthropocene. It was found that the river basin knowledge systems were characterized by increasingly interconnected issues addressed by limited disciplines, which were more strongly linked to society impacts than to policy. Integrated knowledge structures were more desirable for balanced development for society and policy, while over 35 % of river basins, located mostly in Asia, Africa, and South America, faced challenges in effective knowledge transformation for more sustainable development. By determining the structural configurations suitable for specific society and policy impacts, this study can assist in transforming knowledge for more sustainable river basins.
This appendix provides information on the 215 disciplines and the 94 issues grouped based on keywords collected from the Web of Science database and used to construct the discipline–issue networks and the issue networks for the 72 river basins studied. Tables A1 and A2 summarize the total number of connections for each discipline and issue in the networks.
This appendix provides additional statistical details on the Results and Discussions sections. For Sect. 3.2, the temporal trends and corresponding absolute indicator values of the social impact (SO), economic impact (EC), and environmental impact (EN) for the society indicators (Fig. B1) and the temporal trends and absolute indicator valuesof the resource availability (RA), resource utilization (RU), and governance capacity (GC) for the policy indicators are shown (Fig. B2).
For Sect. 3.3, the regression coefficients and levels of significance between the knowledge structural indicators and the society indicators (Fig. B3) and between the knowledge structural indicators and the policy indicators are shown (Fig. B4).
Figure B5 provides correlations between the society indicators and the policy indicators to support the Discussions section.
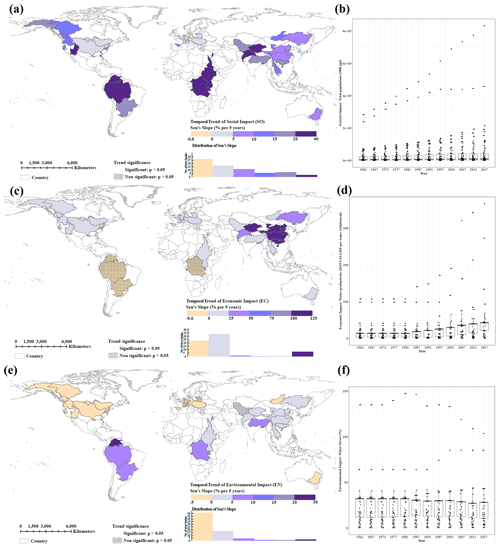
Figure B1(a) The temporal trends (Sen's slope) and (b) the absolute values of the social indicators, (c) the temporal trends (Sen's slope) and (d) the absolute values of the economic indicators, and (e) the temporal trends (Sen's slope) and (f) the absolute values of the environmental indicators for the 72 river basins. Dots in the box plots indicate individual DM and DI values, the box boundaries indicate the 25th and 75th percentiles, the center line indicates median values, and the whiskers indicate a value that is 1.5 times that of the interquartile range.
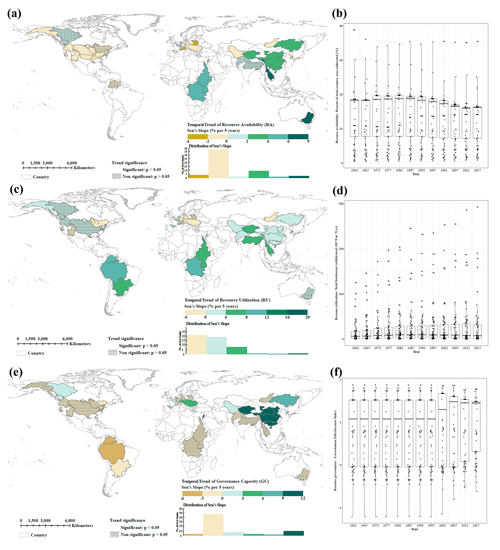
Figure B2(a) The temporal trends (Sen's slope) and (b) the absolute values of the resource availability indicators, (c) the temporal trends (Sen's slope) and (d) the absolute values of the resource utilization indicators, and (e) the temporal trends (Sen's slope) and (f) the absolute values of the governance capacity indicators for the 72 river basins. Dots in the box plots indicate individual DM and DI values, the box boundaries indicate the 25th and 75th percentiles, the center line indicates median values, and the whiskers indicate a value that is 1.5 times that of the interquartile range.
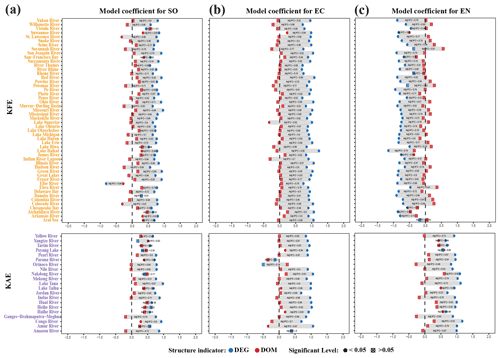
Figure B3The model coefficients for each river basin's linear models between the structural indicators and the (a) SO indicators, (b) EC indicators, and (c) EN indicators.
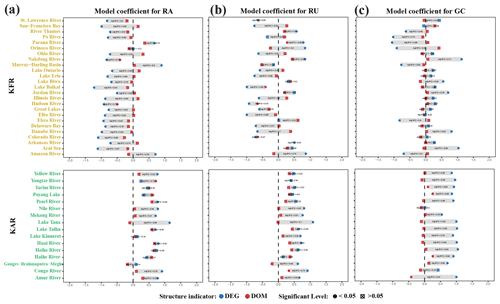
Figure B4The model coefficients for each river basin's linear models between the structural indicators and the (a) RA indicators, (b) RU indicators, and (c) GC indicators.
This appendix provides additional details on the optimization analysis conducted in Sect. 3.4.
The clustering analysis of river basins based on the regression models for the society indicators (i.e., SO, EC, and EN) resulted in three knowledge–society interaction patterns for the 72 river basins: the patterns of knowledge for environment (KFE), knowledge against environment (KAE), and unclear knowledge–society interaction. Similarly, the regression models for the policy indicators (i.e., RA, RU, and GC) resulted in three knowledge–policy interaction patterns, namely of knowledge for resource availability (KFR), knowledge against resource availability (KAR), and unclear knowledge–policy interaction. This means that each of the 72 river basins have one knowledge–society interaction pattern and one knowledge–policy interaction pattern.
To identify the knowledge structures (i.e., DM and DI) that optimize the society indicators, we first removed the rivers with the unclear knowledge–society interaction pattern (n=1) and then calculated the average regression coefficients for river basins under the KFE and KAE patterns, respectively. Similarly, to identify the DM and DI values for optimized policy indicators, rivers with the unclear knowledge–policy interaction pattern were removed (n=31), and the average regression coefficients for each of the KFR and KAR patterns were calculated. This resulted in 12 regression relationships (two for each of SO, EC, EN, RA, RU, and GC), as summarized in Table C1.
For the KFE, KAE, KFR, and KAR patterns, these relationships were used as objective functions for multi-objective optimizations using a NSGA-II genetic algorithm (Deb et al., 2002; Coello Coello et al., 2020) to identify the optimal DM and DI values ( and ). Initially, 100 pairs of potential DM and DI values were randomly generated and modeled over 1000 iterations to search for the optimum values that achieve the objectives as outlined in Table 2.
The global Pareto optimality for each pattern was identified when the Pareto front = 1, which indicated the set of effective solutions that were at least as good as the other possible solutions for each objective and strictly better for at least one objective (Halffmann et al., 2022). Sets of optimal DM and DI values that resulted in society and policy indicators for each pattern were identified, as shown in Fig. C1.
The darker lines highlight the boundary values for the SO, EC, EN, RA, RU, and GC indicators and their corresponding DM and DI values that were selected as the optimal solutions discussed in the main text. The lighter lines represent the other possible values on the Pareto front.
It should also be noted that as we conducted optimizations based on the average coefficients in the linear models, these exact optimal DM and DI values were not directly related to any specific rivers in each knowledge–impact pattern group. Therefore, we referred to the corresponding knowledge structures (i.e., integrated, issue-driven, discipline-driven, and fragmented) that these structural values represented as the optimal knowledge structures that achieved the society and policy objectives.
Data pertaining to this work are available publicly as cited in the paper, and code used to analyze the data is deposited at https://doi.org/10.5281/zenodo.13207837 (SLWU423, 2023).
SW contributed to conceptualization, data curation, methodology, data analysis, writing the original draft, and reviewing and editing the paper, and YW contributed to conceptualization, methodology, data validation, and reviewing and editing the paper.
At least one of the (co-)authors is a member of the editorial board of Hydrology and Earth System Sciences. The peer-review process was guided by an independent editor, and the authors also have no other competing interests to declare.
Publisher's note: Copernicus Publications remains neutral with regard to jurisdictional claims made in the text, published maps, institutional affiliations, or any other geographical representation in this paper. While Copernicus Publications makes every effort to include appropriate place names, the final responsibility lies with the authors.
This study is supported by the Australian Research Council Special Research Initiative (grant no. SR200200186) and The University of Queensland Research Stimulus (UQ RS) Fellowship.
This research has been supported by the Australian Research Council (grant no. SR200200186).
This paper was edited by Nadia Ursino and reviewed by two anonymous referees.
Afroz, N. and Ilham, Z.: Assessment of Knowledge, Attitude and Practice of University Students towards Sustainable Development Goals (SDGs), The Journal of Indonesia Sustainable Development Planning, 1, 31–44, https://doi.org/10.46456/jisdep.v1i1.12, 2020.
Alias, N. A.: Correlation between knowledge, attitude, and behavior towards river pollution, International Journal of Modern Trends in Social Sciences, 2, 31–38, 2019.
Bodin, Ö.: Collaborative environmental governance: Achieving collective action in social-ecological systems, Science, 357, eaan1114, https://doi.org/10.1126/science.aan1114, 2017.
Borgatti, S. P.: Centrality and network flow, Soc. Networks, 27, 55–71, https://doi.org/10.1016/j.socnet.2004.11.008, 2005.
Brey, P.: The strategic role of technology in a good society, Technol. Soc., 52, 39–45, https://doi.org/10.1016/j.techsoc.2017.02.002, 2018.
Burmaoglu, S., Sartenaer, O., and Porter, A.: Conceptual definition of technology emergence: A long journey from philosophy of science to science policy, Technol. Soc., 59, 101126, https://doi.org/10.1016/j.techsoc.2019.04.002, 2019.
Callon, M., Courtial, J.-P., Turner, W. A., and Bauin, S.: From translations to problematic networks: An introduction to co-word analysis, Information (International Social Science Council), 22, 191–235, https://doi.org/10.1177/053901883022002003, 1983.
Cash, D. W., Clark, W. C., Alcock, F., Dickson, N. M., Eckley, N., Guston, D. H., Jäger, J., and Mitchell, R. B.: Knowledge systems for sustainable development, P. Natl. Acad. Sci. USA, 100, 8086–8091, https://doi.org/10.1073/pnas.1231332100, 2003.
Coccia, M.: The evolution of scientific disciplines in applied sciences: dynamics and empirical properties of experimental physics, Scientometrics, 124, 451–487, https://doi.org/10.1007/s11192-020-03464-y, 2020.
Cockburn, J.: Knowledge integration in transdisciplinary sustainability science: Tools from applied critical realism, Sustain. Dev., 30, 358–374, https://doi.org/10.1002/sd.2279, 2022.
Coello Coello, C. A., González Brambila, S., Figueroa Gamboa, J., Castillo Tapia, M. G., and Hernández Gómez, R.: Evolutionary multiobjective optimization: open research areas and some challenges lying ahead, Complex & Intelligent Systems, 6, 221–236, https://doi.org/10.1007/s40747-019-0113-4, 2020.
Csardi, G. and Nepusz, T.: The igraph software package for complex network research, InterJournal, Complex Systems, https://igraph.org/ (last access: 22 June 2024), 2006.
Deb, K. and Gupta, H.: Searching for Robust Pareto-Optimal Solutions in Multi-objective Optimization, in: edited by: Coello Coello, C. A., Hernández Aguirre, A., and Zitzler, E., Evolutionary Multi-Criterion Optimization. EMO 2005. Lecture Notes in Computer Science, Springer, Berlin, Heidelberg, 3410, 150–164, https://doi.org/10.1007/978-3-540-31880-4_11, 2005.
Deb, K., Pratap, A., Agarwal, S., and Meyarivan, T.: A fast and elitist multiobjective genetic algorithm: NSGA-II, IEEE T. Evolut. Comput., 6, 182–197, https://doi.org/10.1109/4235.996017, 2002.
Edgeworth, F. Y.: Mathematical psychics: An essay on the application of mathematics to the moral sciences, CK Paul, ISBN-10: 9333349391, ISBN-13: 978-9333349390, 1881.
Edwards, D. M. and Meagher, L. R.: A framework to evaluate the impacts of research on policy and practice: A forestry pilot study, Forest Policy Econ., 114, 101975, https://doi.org/10.1016/j.forpol.2019.101975, 2020.
Fernandes, R. and Leblanc, S. G.: Parametric (modified least squares) and non-parametric (Theil–Sen) linear regressions for predicting biophysical parameters in the presence of measurement errors, Remote Sens. Environ., 95, 303–316, https://doi.org/10.1016/j.rse.2005.01.005, 2005.
Hakkarainen, V., Daw, T. M., and Tengö, M.: On the other end of research: exploring community-level knowledge exchanges in small-scale fisheries in Zanzibar, Sustain. Sci., 15, 281–295, https://doi.org/10.1007/s11625-019-00750-4, 2020.
Hakkarainen, V., Soini, K., Dessein, J., and Raymond, C. M.: Place-embedded agency: Exploring knowledge–place connections for enabling plurality in governance of social–ecological systems, People and Nature, 4, 1141–1158, https://doi.org/10.1002/pan3.10365, 2022.
Halffmann, P., Schäfer, L. E., Dächert, K., Klamroth, K., and Ruzika, S.: Exact algorithms for multiobjective linear optimization problems with integer variables: A state of the art survey, Journal of Multi-Criteria Decision Analysis, 29, 341–363, https://doi.org/10.1002/mcda.1780, 2022.
Hernanda, T., Absori, Azhari, A. F., Wardiono, K., and Arlinwibowo, J.: Relationship Between Knowledge and Affection for the Environment: A Meta-Analysis, European Journal of Educational Research, 12, 1071–1084, https://doi.org/10.12973/eu-jer.12.2.1069, 2023.
Jabbour, J.: Global sustainability governance: Integrated scientific assessment at a critical inflection point, PhD dissertation, TU München, https://doi.org/10.14279/depositonce-15477, 2022.
Jerneck, A., Olsson, L., Ness, B., Anderberg, S., Baier, M., Clark, E., Hickler, T., Hornborg, A., Kronsell, A., Lövbrand, E., and Persson, J.: Structuring sustainability science, Sustain. Sci., 6, 69–82, https://doi.org/10.1007/s11625-010-0117-x, 2011.
Kassambara, A. and Mundt, F.: Extract and visualize the results of multivariate data analyses, CRAN [code], https://doi.org/10.32614/CRAN.package.factoextra, 2020.
Kendall, M. G.: Rank correlation methods, 4th edn., Charles Griffin, London, ISBN-10: 0852641990, ISBN-13: 978-0852641996, 1975.
Kim, R. E.: Is Global Governance Fragmented, Polycentric, or Complex? The State of the Art of the Network Approach, Int. Stud. Rev., 22, 903–931, https://doi.org/10.1093/isr/viz052, 2019.
Krausmann, F. and Fischer-Kowalski, M.: Global Socio-metabolic Transitions, in: Long Term Socio-Ecological Research: Studies in Society-Nature Interactions Across Spatial and Temporal Scales, edited by: Singh, S. J., Haberl, H., Chertow, M., Mirtl, M., and Schmid, M., Springer Netherlands, Dordrecht, 339–365, https://doi.org/10.1007/978-94-007-1177-8_15, 2013.
Latour, B.: Science in action: How to follow scientists and engineers through society, Harvard University Press, ISBN-10: 0674792912, ISBN-13: 978-0674792913,, 1987.
Louder, E., Wyborn, C., Cvitanovic, C., and Bednarek, A. T.: A synthesis of the frameworks available to guide evaluations of research impact at the interface of environmental science, policy and practice, Environ. Sci. Policy, 116, 258–265, https://doi.org/10.1016/j.envsci.2020.12.006, 2021.
Mann, H. B.: Nonparametric tests against trend, Econometrica, 13, 245–259, 1945.
Marler, R. T. and Arora, J. S.: Survey of multi-objective optimization methods for engineering, Struct. Multidiscip. O., 26, 369–395, https://doi.org/10.1007/s00158-003-0368-6, 2004.
Matsumoto, I., Takahashi, Y., Mader, A., Johnson, B., Lopez-Casero, F., Kawai, M., Matsushita, K., and Okayasu, S.: Mapping the Current Understanding of Biodiversity Science–Policy Interfaces, in: Managing Socio-ecological Production Landscapes and Seascapes for Sustainable Communities in Asia, edited by: Saito, O., Subramanian, S. M., Hashimoto, S., and Takeuchi, K., Springer Singapore, Singapore, 147–170, https://doi.org/10.1007/978-981-15-1133-2_8, 2020.
Molle, F.: River-basin planning and management: The social life of a concept, Geoforum, 40, 484–494, https://doi.org/10.1016/j.geoforum.2009.03.004, 2009.
Moritz, S. and Bartz-Beielstein, T.: imputeTS: time series missing value imputation in R, R Journal, 9, 207–218, https://doi.org/10.32614/RJ-2017-009, 2017.
Murtagh, F. and Legendre, P.: Ward's Hierarchical Agglomerative Clustering Method: Which Algorithms Implement Ward's Criterion?, J. Classif., 31, 274–295, https://doi.org/10.1007/s00357-014-9161-z, 2014.
Newig, J. and Rose, M.: Cumulating evidence in environmental governance, policy and planning research: towards a research reform agenda, J. Environ. Pol. Plan., 22, 667–681, https://doi.org/10.1080/1523908X.2020.1767551, 2020.
Nguyen, V. M., Young, N., and Cooke, S. J.: A roadmap for knowledge exchange and mobilization research in conservation and natural resource management, Conserv. Biol., 31, 789–798, https://doi.org/10.1111/cobi.12857, 2017.
Norström, A. V., Cvitanovic, C., Löf, M. F., West, S., Wyborn, C., Balvanera, P., Bednarek, A. T., Bennett, E. M., Biggs, R., de Bremond, A., Campbell, B. M., Canadell, J. G., Carpenter, S. R., Folke, C., Fulton, E. A., Gaffney, O., Gelcich, S., Jouffray, J.-B., Leach, M., Le Tissier, M., Martín-López, B., Louder, E., Loutre, M.-F., Meadow, A. M., Nagendra, H., Payne, D., Peterson, G. D., Reyers, B., Scholes, R., Speranza, C. I., Spierenburg, M., Stafford-Smith, M., Tengö, M., van der Hel, S., van Putten, I., and Österblom, H.: Principles for knowledge co-production in sustainability research, Nature Sustainability, 3, 182–190, https://doi.org/10.1038/s41893-019-0448-2, 2020.
Noyons, E.: Bibliometric mapping of science in a science policy context, Scientometrics, 50, 83–98, https://doi.org/10.1023/A:1005694202977, 2001.
Okamura, A. and Nishijo, K.: Constructing vision-driven indicators to enhance the interaction between science and society, Scientometrics, 125, 1575–1589, https://doi.org/10.1007/s11192-020-03598-z, 2020.
Ostrom, E.: A General Framework for Analyzing Sustainability of Social-Ecological Systems, Science, 325, 419–422, https://doi.org/10.1126/science.1172133, 2009.
Penfield, T., Baker, M. J., Scoble, R., and Wykes, M. C.: Assessment, evaluations, and definitions of research impact: A review, Res. Evaluat., 23, 21–32, https://doi.org/10.1093/reseval/rvt021, 2013.
Pitt, R., Wyborn, C., Page, G., Hutton, J., Sawmy, M. V., Ryan, M., and Gallagher, L.: Wrestling with the complexity of evaluation for organizations at the boundary of science, policy, and practice, Conserv. Biol., 32, 998–1006, https://doi.org/10.1111/cobi.13118, 2018.
Posner, S. M. and Cvitanovic, C.: Evaluating the impacts of boundary-spanning activities at the interface of environmental science and policy: A review of progress and future research needs, Environ. Sci. Policy, 92, 141–151, https://doi.org/10.1016/j.envsci.2018.11.006, 2019.
Ramírez-Castañeda, V.: Disadvantages in preparing and publishing scientific papers caused by the dominance of the English language in science: The case of Colombian researchers in biological sciences, PLoS ONE, 15, e0238372, https://doi.org/10.1371/journal.pone.0238372, 2020.
Ratner, B.: The correlation coefficient: Its values range between , or do they?, Journal of Targeting, Measurement and Analysis for Marketing, 17, 139–142, https://doi.org/10.1057/jt.2009.5, 2009.
R Core Team: R: A language and environment for statistical computing, Version 4.2.3, R Foundation for Statistical Computing [code], Vienna, Austria, https://www.r-project.org/ (last access: 22 June 2024), 2023.
Reyers, B. and Selig, E. R.: Global targets that reveal the social–ecological interdependencies of sustainable development, Nat. Ecol. Evol., 4, 1011–1019, https://doi.org/10.1038/s41559-020-1230-6, 2020.
Rodríguez, D. J., Paltán, H. A., García, L. E., Ray, P., and St. George Freeman, S.: Water-related infrastructure investments in a changing environment: a perspective from the World Bank, Water Policy, 23, 31–53, https://doi.org/10.2166/wp.2021.265, 2021.
Royston, P.: Profile Likelihood for Estimation and Confidence Intervals, Stata J., 7, 376–387, https://doi.org/10.1177/1536867x0700700305, 2007.
Sayles, J. S. and Baggio, J. A.: Social–ecological network analysis of scale mismatches in estuary watershed restoration, P. Natl. Acad. Sci. USA, 114, E1776–E1785, https://doi.org/10.1073/pnas.1604405114, 2017.
Sen, P. K.: Estimates of the Regression Coefficient Based on Kendall's Tau, J. Am. Stat. Assoc., 63, 1379–1389, https://doi.org/10.1080/01621459.1968.10480934, 1968.
Shi, F., Foster, J. G., and Evans, J. A.: Weaving the fabric of science: Dynamic network models of science's unfolding structure, Soc. Networks, 43, 73–85, https://doi.org/10.1016/j.socnet.2015.02.006, 2015.
SLWU423: SLWU423/Code-for-global-river-basin-science-policy-society-impact: Pub, Zenodo [code], https://doi.org/10.5281/zenodo.13207837, 2023.
Steffen, W., Richardson, K., Rockström, J., Cornell, S. E., Fetzer, I., Bennett, E. M., Biggs, R., Carpenter, S. R., de Vries, W., de Wit, C. A., Folke, C., Gerten, D., Heinke, J., Mace, G. M., Persson, L. M., Ramanathan, V., Reyers, B., and Sörlin, S.: Planetary boundaries: Guiding human development on a changing planet, Science, 347, 1259855, https://doi.org/10.1126/science.1259855, 2015.
Stirling, A.: A general framework for analysing diversity in science, technology and society, J. R. Soc. Interface, 4, 707–719, https://doi.org/10.1098/rsif.2007.0213, 2007.
Stovel, K. and Shaw, L.: Brokerage, Annu. Rev. Sociol., 38, 139–158, https://doi.org/10.1146/annurev-soc-081309-150054, 2012.
Wang, F., Shao, W., Yu, H., Kan, G., He, X., Zhang, D., Ren, M., and Wang, G.: Re-evaluation of the power of the Mann-Kendall test for detecting monotonic trends in hydrometeorological time series, Front. Earth Sci., 8, 14, 2020.
Warner, J., Wester, P., and Bolding, A.: Going with the flow: river basins as the natural units for water management?, Water Policy, 10, 121–138, https://doi.org/10.2166/wp.2008.210, 2008.
Wasserman, S. and Faust, K.: Social Network Analysis: Methods and Applications, Cambridge University Press, ISBN-10: 0521387078, ISBN-13: 978-0521387071, 1994.
Web of Science: List of Subject Classifications for All Databases, https://images.webofknowledge.com/images/help/WOS/hp_research_areas_easca.html (last access: 16 July 2021), 2021.
Wei, Y. and Wu, S.: The gulf of cross-disciplinary research collaborations on global river basins is not narrowed, Ambio, 51, 1994–2006, https://doi.org/10.1007/s13280-022-01716-0, 2022.
Wei, Y., Ison, R., Western, A., and Lu, Z.: Understanding ourselves and the environment in which we live, Curr. Opin. Env. Sust., 33, 161–166, https://doi.org/10.1016/j.cosust.2018.06.002, 2018.
Wei, Y., Wu, S., Lu, Z., Wang, X., Wu, X., Xu, L., and Sivapalan, M.: Ageing Knowledge Structure in Global River Basins, Frontiers in Environmental Science, 10, 821342, https://doi.org/10.3389/fenvs.2022.821342, 2022.
Weichselgartner, J. and Kasperson, R.: Barriers in the science-policy-practice interface: Toward a knowledge-action-system in global environmental change research, Global Environ. Chang., 20, 266–277, https://doi.org/10.1016/j.gloenvcha.2009.11.006, 2010.
Wu, S., Wei, Y., and Wang, X.: Structural gaps of water resources knowledge in global river basins, Hydrol. Earth Syst. Sci., 25, 5381–5398, https://doi.org/10.5194/hess-25-5381-2021, 2021.
Yan, D., Zhang, X., Qin, T., Li, C., Zhang, J., Wang, H., Weng, B., Wang, K., Liu, S., Li, X., Yang, Y., Li, W., Lv, Z., Wang, J., Li, M., He, S., Liu, F., Bi, W., Xu, T., Shi, X., Man, Z., Sun, C., Liu, M., Wang, M., Huang, Y., Long, H., Niu, Y., Dorjsuren, B., Gedefaw, M., Li, Y., Tian, Z., Mu, S., Wang, W., and Zhou, X.: A data set of distributed global population and water withdrawal from 1960 to 2020, Scientific Data, 9, 640, https://doi.org/10.1038/s41597-022-01760-1, 2022.
Zeng, A., Shen, Z., Zhou, J., Wu, J., Fan, Y., Wang, Y., and Stanley, H. E.: The science of science: from the perspective of complex systems, Phys. Rep., 714–715, 1–73, https://doi.org/10.1016/j.physrep.2017.10.001, 2017.
- Abstract
- Introduction
- Methods
- Results
- Discussions
- Conclusions
- Appendix A: List of scientific disciplines and issues in the global river basin knowledge network
- Appendix B: Additional statistical details
- Appendix C: Additional methods and results on optimizing the knowledge structures for improved society and policy
- Code and data availability
- Author contributions
- Competing interests
- Disclaimer
- Acknowledgement
- Financial support
- Review statement
- References
- Abstract
- Introduction
- Methods
- Results
- Discussions
- Conclusions
- Appendix A: List of scientific disciplines and issues in the global river basin knowledge network
- Appendix B: Additional statistical details
- Appendix C: Additional methods and results on optimizing the knowledge structures for improved society and policy
- Code and data availability
- Author contributions
- Competing interests
- Disclaimer
- Acknowledgement
- Financial support
- Review statement
- References