the Creative Commons Attribution 4.0 License.
the Creative Commons Attribution 4.0 License.
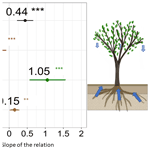
Root water uptake patterns are controlled by tree species interactions and soil water variability
Andrew J. Guswa
Janett Filipzik
Johanna Clara Metzger
Christine Römermann
Root water uptake depends on soil moisture which is primarily fed by throughfall in forests. Several biotic and abiotic elements shape the spatial distribution of throughfall. It is well documented that throughfall patterns result in reoccurring higher and lower water inputs at certain locations. However, how the spatial distribution of throughfall affects root water uptake patterns remains unresolved. Therefore, we investigate root water uptake patterns by considering spatial patterns of throughfall and soil water in addition to soil and neighboring tree characteristics. In a beech-dominated mixed deciduous forest in a temperate climate, we conducted intensive throughfall sampling at locations paired with soil moisture sensors during the 2019 growing season. We employed a linear mixed-effects model to understand controlling factors in root water uptake patterns. Our results show that soil water patterns and interactions among neighboring trees are the most significant factors regulating root water uptake patterns. Temporally stable throughfall patterns did not influence root water uptake patterns. Similarly, soil properties were unimportant for spatial patterns of root water uptake. We found that wetter locations (rarely associated with throughfall hotspots) promoted greater root water uptake. Root water uptake in monitored soil layers also increased with neighborhood species richness. Ultimately our findings suggest that complementarity mechanisms within the forest stand, in addition to soil water variability and availability, govern root water uptake patterns.
- Article
(3237 KB) - Full-text XML
-
Supplement
(778 KB) - BibTeX
- EndNote
Root water uptake depends on soil moisture, which is replenished by precipitation. At the same time, the vegetation canopy intercepts and redirects precipitation into throughfall and stemflow, collectively referred to as below-canopy precipitation. Thus, even before soil water can be taken up by roots, it has already been influenced by the canopy.
Throughfall is typically the largest component of below-canopy precipitation (Levia and Frost, 2006; Sadeghi et al., 2020). For instance, in temperate forests, about 70 % of above-canopy precipitation ends up as throughfall (Levia and Frost, 2003; Sadeghi et al., 2020). Below-canopy precipitation is modified by several biotic and abiotic factors (Levia and Frost, 2006; Levia et al., 2011), including vegetation type, canopy architecture (Crockford and Richardson, 2000; Pypker et al., 2011; Levia et al., 2017), and forest structure (Rodrigues et al., 2022), and meteorological elements such as wind speed (Staelens et al., 2008; Van Stan et al., 2011; Fan et al., 2015), precipitation intensity, and event size (Dunkerley, 2014; Magliano et al., 2019; Zhang et al., 2016; Staelens et al., 2008). As a result, throughfall inherently varies across space and time. However, previous studies have shown that the spatial distribution of throughfall persists over time (Keim et al., 2005; Staelens et al., 2006; Guswa and Spence, 2012; Carlyle-Moses et al., 2014; Metzger et al., 2017; Van Stan et al., 2020).
Throughfall patterns have been hypothesized to affect the spatial variation in water uptake (Bouten et al., 1992; Coenders-Gerrits et al., 2013) and soil moisture distribution (Raat et al., 2002; Blume et al., 2009; Zimmermann et al., 2009; Zehe et al., 2010; Bachmair et al., 2012; Rosenbaum et al., 2012; Zhang et al., 2016). However, empirical evidence is scarce. A decade ago, Coenders-Gerrits et al. (2013) proposed that throughfall patterns are translated into soil wetting dynamics with a model based on combined hillslope topographic and throughfall data collected in a beech-dominated catchment. However, in this model, the effect of throughfall patterns on soil moisture patterns rapidly ceased and became more similar to the bedrock topography. Regarding the latter result, the model and reality differ, as the correlation between the measured bedrock topography and soil moisture is low (Tromp-van Meerveld and McDonnell, 2006), which Coenders-Gerrits et al. (2013) attributed to root water uptake. Metzger et al. (2017) showed through field observations that, although throughfall spatial variation strongly increases shortly after rainfall, it drops again quickly in the drained state, so the impact rapidly disappears. Later, Fischer-Bedtke et al. (2023) confirmed at the same field site that recurring throughfall patterns left a notable imprint on the soil moisture response to rainfall, yet the effect on the absolute values of the soil water content in the drained state was rather weak. Additionally, Zhu et al. (2021) observed that stable throughfall patterns were weakly related to the spatial distribution of soil moisture since this relationship was restricted only to relatively wet soil locations and throughfall hotspots. They also showed that throughfall patterns had a weaker influence on the temporal dynamics of the soil water content compared to soil bulk density and litter layer properties.
Taken together, several studies have searched for patterns of throughfall in soil moisture spatial variation. As comparatively weak relationships were found, some previous studies have suggested that root water uptake (Bouten et al., 1992; Schwärzel et al., 2009) could be the cause. Specifically, based on a one-dimensional soil-water model, Bouten et al. (1992) proposed that throughfall patterns alter and localize root water uptake and promote fast drainage. As a result, spatial variation in root water uptake could diminish the effect of throughfall patterns into spatiotemporal variation of soil water. However, other researchers suggested that other factors, such as soil properties (Metzger et al., 2017), preferential flow (Jost et al., 2004; Blume et al., 2009; Molina et al., 2019; Fischer-Bedtke et al., 2023), and litter layer processes (Raat et al., 2002), may be at the heart of the weak and short-term effects of throughfall patterns on soil moisture variability.
However, to the best of our knowledge, the feedback mechanism of throughfall patterns on root water uptake variation has not yet been investigated empirically. More common are studies related to soil water distribution. Soil water availability, which could potentially be enhanced by throughfall, affects root water uptake patterns even more than root abundance (Kühnhammer et al., 2020; Guderle et al., 2018). On the flip side, root water uptake can amplify or homogenize soil water variability (Hupet and Vanclooster, 2005; Teuling and Troch, 2005; Ivanov et al., 2010; Baroni et al., 2013; Martínez García et al., 2014). Moreover, variations in soil water content reflect on root water uptake (Hupet et al., 2002; Schume et al., 2004; Schwärzel et al., 2009; Guderle and Hildebrandt, 2015; Jackisch et al., 2020).
Next to water input, soil properties can alter root water uptake patterns (Nadezhdina et al., 2007; Kirchen et al., 2017). Also, they control soil water redistribution (Grayson et al., 1997; Cosh et al., 2008; Jarecke et al., 2021) and water availability for root structures (Vereecken et al., 2007; Cai et al., 2018). For a given evaporative demand, water uptake at a particular location is a function of water transport resistance between root and soil in addition to the soil-water potential (Cardon and Letey, 1992; Shani and Dudley, 1996; Lhomme, 1998). Both characteristics depend on local soil properties and soil water status, and the latter in turn is affected by the local water uptake rate.
Finally, plant individual and ecosystem processes affect uptake: root networks can connect wetter and drier locations in a variety of ecosystems (e.g., Emerman and Dawson, 1996; Katul and Siqueira, 2010; Yu and D'Odorico, 2015; Priyadarshini et al., 2016; Hafner et al., 2017). In addition, tree size, age, neighboring tree species, and ecosystem structure affect the spatiotemporal variation in root water uptake (Volkmann et al., 2016; Spanner et al., 2022; Kostner et al., 2002; Dawson, 1996; Brinkmann et al., 2019; Gaines et al., 2016; Silvertown et al., 2015; Guo et al., 2018; Brum et al., 2019; Krämer and Hölscher, 2010).
Taken together, throughfall and soil water variability, soil properties, and root water uptake patterns form complex and intertwined interactions in the terrestrial hydrological cycle. It has not yet been shown empirically how root water uptake patterns are affected by throughfall and spatial distribution of soil water content. In line with previous modeling results (Bouten et al., 1992; Coenders-Gerrits et al., 2013), we hypothesize that throughfall hotspots enhance water availability at certain locations that elevate root water uptake. Furthermore, we investigate the role of soil water variation in combination with soil properties and neighboring tree characteristics in root water uptake patterns. We pose the following questions to test the main hypothesis and guide the investigation.
- i.
How do throughfall patterns influence root water uptake patterns?
- ii.
How do soil moisture and its variation, along with soil properties, control variation in root water uptake?
- iii.
What is the role of biotic factors, i.e., size, distance, number, and species richness of neighboring trees, in root water uptake patterns?
Here, we address these questions by employing a linear mixed-effects model based on weekly throughfall sampling at locations paired with intensive soil moisture measurements in a beech-dominated unmanaged forest. We estimate root water uptake using a water balance method applied at a soil moisture measurement point. This method dissects soil water flow and water uptake by exploring the differences in soil water content change per time between day and night (Guderle and Hildebrandt, 2015; Jackisch et al., 2020). While other methods exist, such as using isotopic tracers (Rothfuss and Javaux, 2017; Zarebanadkouki et al., 2013), daily fluctuations in soil water allow for estimation of the spatial distribution of ecosystem evapotranspiration using standard measurements of soil water content (Guderle and Hildebrandt, 2015) without the need for additional infrastructure. In addition, we incorporate data on field capacity, bulk density, and neighboring tree characteristics, i.e., size and species.
2.1 Research site and field sampling
2.1.1 Research site
The research site is located in the forested upper hill region of the Hainich low mountain range in Thuringia, Germany, as a part of the Hainich Critical Zone Exploratory (CZE) (Küsel et al., 2016). The altitude at the research site ranges from 362 m to 368 m a.s.l. The mean annual air temperature varies between 7.5 and 9.5 °C, and the mean annual precipitation ranges from less than 600 to 1000 mm in the CZE (Küsel et al., 2016).
In the study area, thin-bedded alternations of limestones and marlstones of carbonate rock (Middle Triassic) form the bedrock overlain by a shallow Pleistocene loess layer with Cambisols and Luvisols as the dominant soil types (IUSS Working Group, 2006; Metzger et al., 2021). The median soil depth above the weathered bedrock is 37 cm, with soil depths ranging from 15 cm to a maximum depth of 87 cm (Metzger et al., 2017).
In 2019, the tree community at the research site consisted of 574 individuals of various ages (diameter at breast height ≥ 5 cm). The dominant species is European beech (Fagus sylvatica L.), which makes up 70 % of the tree community, followed by sycamore maple (Acer pseudoplatanus L.) with 21 % and European ash (Fraxinus excelsior L.) with 4 %. These dominant species are accompanied by large-leaved linden (Tilia platyphyllos Scop.), European hornbeam (Carpinus betulus L.), Norway maple (Acer platanoides L.), Scots elm (Ulmus glabra L.), and wild service tree (Sorbus torminalis (L.) Crantz). The stand has a total basal area of 40 m2 ha−1 and has been unmanaged since 1997 (Kohlhepp et al., 2017).
2.1.2 Soil moisture monitoring and soil properties
The forest site (1 ha) was equipped with a soil moisture monitoring network (SoilNet; Bogena et al., 2010) consisting of SMT100 frequency domain sensors (Treuebner GmbH, Neustadt, Germany). Metzger et al. (2017) first described the soil moisture monitoring setup. Briefly, the observation platform (Fig. 1) was divided into 100 subplots (10 m × 10 m), and 49 subplots were equipped with soil moisture sensors at two random measuring points each, for a total of 98 locations. At each measuring point, sensors were placed at two different depths, 7.5 cm (top sensors) and 27.5 cm (bottom sensors). The soil moisture network is maintained through a regular biweekly routine to avoid potential failures such as depleted sensor batteries or hardware problems.
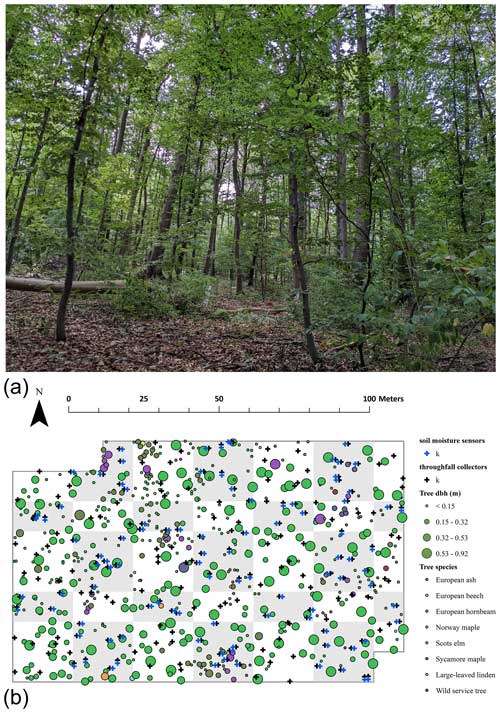
Figure 1(a) The photo of the site. (b) The field monitoring setup of stratified randomly distributed throughfall collectors and soil moisture sensors together with the trees which are sized according to the diameter at breast height (dbh) and colored according to the species. Throughfall collectors are paired with soil moisture sensors at 98 locations (n=182) in the grey-shaded subplots. White-colored subplots are equipped with only throughfall collectors.
Undisturbed soil samples were collected during the sensor installation in 2014 and 2015 to estimate bulk density and water content at field capacity. In addition, we collected additional disturbed soil samples (n=40) near sensor locations in 2019. Bulk density was determined from oven-dried (24 h, 105 °C) soil mass weight and water content at field capacity by applying 60 hPa pressure to the saturated undisturbed sample for 72 h.
Soil properties vary slightly from topsoil to subsoil at the research site. While silty loam is the dominant soil texture in both layers, the clay content is higher in the subsoil (Metzger et al., 2021). The median volumetric water content at field capacity is 44 % in the topsoil and 42 % in the subsoil. Moreover, the water content at field capacity varies from 27 % to 60 % and from 31 % to 62 % in the topsoil and subsoil, respectively. The average bulk density (dbulk) of the topsoil is 1.16 g cm−3, with a range of 0.73 to 1.5 g cm−3. In the subsoil, the average bulk density (dbulk) is slightly higher at 1.37 g cm−3 but has a similar range (0.7–1.6 g cm−3) (see the Supplement for details).
2.1.3 Gross precipitation and throughfall sampling
Five gross precipitation funnels were placed 1.5 m a.g.l. in an adjacent open grassland (ca. 250 m distance to the research site). As described in Metzger et al. (2017) and Demir et al. (2022), the precipitation funnels were made of a circular plastic funnel (12 cm in diameter) and sampling bottle (2 L in volume), and ping pong balls were placed in the funnel orifice to prevent evaporation losses.
During the early growing season of 2019, we placed throughfall collectors in soil moisture monitoring subplots at 98 locations. We paired these throughfall collectors with the soil moisture sensors by placing them within 1 m of each other. The paired collectors were placed downslope to avoid interference with soil moisture measurements. For the rest of the research site, in 51 other subplots, we adopted a separate independent stratified random design from Metzger et al. (2017). Briefly, we placed two throughfall collectors in each subplot that was not equipped with soil moisture sensors. All the throughfall collectors were placed roughly 37 cm above the ground.
We conducted weekly manual measurement of throughfall and gross precipitation during the 2019 growing season (April to August). Sampling was conducted on rain-free days only. Thus, the sampling interval ranged between 6 and 8 d.
We used the paired throughfall collectors (n=98) to identify the drivers of root water uptake patterns, as we derived root water uptake values based on soil water content measurements (see below). However, we used all the randomly placed throughfall collectors (n=200) to describe the spatiotemporal variation of throughfall within the research site.
2.2 Estimation of potential evapotranspiration
We calculated the daily potential evapotranspiration by applying the concept of thermodynamic limits of convection (Kleidon and Renner, 2013; Kleidon et al., 2014):
where Rsn is the absorbed solar radiation (W m−2), λ is the latent heat of vaporization (2.5×106 J kg−1), γ is the psychrometric constant (65 Pa K−1), and s is the slope of the saturation vapor pressure curve (Pa K−1).
Here, we acquired solar radiation, air temperature, and precipitation data for the throughfall sampling period from a nearby weather station (Reckenbuel) which is located approximately 1.4 km northeast of the research site and provides data in 10 min intervals. The site-specific albedo for the summer period was adopted from Otto et al. (2014).
We used the precipitation data measured at the weather station to define rain events and dry periods, as described below.
2.3 Data analysis
2.3.1 Quality control of soil water content data
We systematically reviewed the 6 min soil water content data for quality control in two steps: (1) identification of problems (such as jumps to extremely low and high values, duplicated time stamps of different values, long discontinuities in the measurements, and lack of temporal variation in the time series despite rain events); (2) classification and removal of detected outliers and irregularities. We visually identified and removed unrealistic measurements such as extremely low (<5 vol. %) and high values far beyond the field capacity (>75 vol. %) and long plateaus of repeated values despite rain events. We also excluded any time series that exhibited long-term discontinuities that prevented us from calculating root water uptake. During the visual inspection, we eliminated values with duplicated time stamps that violated the actual temporal trend. Next, we scanned the data using the Hampel filter function of the pracma R package (Borchers, 2021) with a customized moving window length and Pearson's rule threshold value (Pearson, 1999) to flag possible outliers.
Despite regular maintenance, many sensors failed to provide data that met the quality criteria during the growing season (March–August) in 2019. Only 56 sensor locations (out of 98) provided data from both top and bottom sensors that met the qualification criteria described above with varying date intervals throughout the growing season. Of these, only 34 sensor locations were used to estimate root water uptake as they simultaneously provided data from both top and bottom sensors within the dry periods.
2.3.2 Soil water calculation
We estimated soil water (S) at measurement locations for the monitored soil layer based on the volumetric soil water content measured by top and bottom sensors.
We similarly integrated the soil water at field capacity (SFC,i):
where zt is the depth of the soil column monitored by the top sensor, zb is the depth of the soil represented by the bottom sensor, θi,d is the volumetric soil water content at location i on date d, and θFC,i the soil water content at the field capacity.
We calculated bulk density at the sensors' locations for the monitored soil layer.
and are the bulk density of the topsoil and subsoil, respectively, at location i.
2.3.3 Descriptive statistics
We calculated the coefficient of quartile variation (CQV) and the interquartile range to describe the spatial variation of throughfall, volumetric soil water content, and root water uptake. Also, we estimated the octile skewness (OS8) of throughfall based on the first and seventh octiles.
We characterized spatial patterns of daily root water uptake (Et) by calculating the spatial deviation from the mean (, Eq. 7) (Vachaud et al., 1985).
is the daily root water uptake estimated at the i sensor location on date d, and is the spatial average of the daily root water uptake on date d.
Similarly, we calculated the spatial deviation of soil water and throughfall to identify their spatial patterns.
2.4 Root water uptake estimation
We estimated root water uptake using the multistep, multilayer regression (MSML) method, which is a water balance method and derives evapotranspiration from diurnal differences in soil water content (Guderle and Hildebrandt, 2015; Guderle et al., 2018). This approach does not require prior information on root structure but relies on high temporal and spatial resolution data on multiple soil layers. Previous studies using additional measurements such as sap flow and lysimeters demonstrated that the MSML method successfully estimates transpiration in both forest and grassland ecosystems (Guderle et al., 2018; Jackisch et al., 2020).
As described in Guderle and Hildebrandt (2015), the MSML method derives root water uptake from distinct differences in the day and night portions of soil moisture time series. The main assumption is that, in the absence of rainfall-driven rapid vertical soil water flow, evapotranspiration occurs only during the day, while soil water flow occurs both during the day and at night. As a result, soil moisture time series reflect a distinct day–night signal under dry weather conditions.
In applying this method to our study, we first excluded potential periods of fast vertical flow periods from the time series due to previous rainfall events and identified periods for estimating daily root water uptake. We considered an 8 h buffer period to include canopy dripping and 48 h for the cessation of rainfall influence on soil water. Thus, a total of 56 h was the time interval used to define the start of the water uptake estimation period. The period when the root water uptake is estimated is hereafter referred to as the dry period.
Next, we split each soil moisture time series into a day branch (transpiration-active period) and a night branch, as explained by Guderle and Hildebrandt (2015). We defined the transpiration period (starting 2 h after sunrise and ending 2 h before sunset) based on the local sunrise and sunset times. Sunrise and sunset times were obtained from the R package suncal (Thieurmel and Elmarhraoui, 2022). We fit linear models to each split branch of the time series and derived the slopes. The difference between the slope of the day branch (mtot) and the average slope of the antecedent and preceding nights () gives the rate of water uptake. Thus, we estimated the daily evapotranspiration at each soil water content location i (Eqs. 8 and 9) by accounting for soil layer thickness and slope difference.
2.5 Linear mixed-effects model
We employed a linear mixed-effects model to investigate the driving factors for root water uptake patterns. A linear mixed-effects model is a multivariate statistical tool that describes the relationship between a dependent variable and explanatory variables (fixed effects) while controlling for dependencies in the data that may arise due to repeated sampling with certain designs (random effects). Fixed effects are informative, repeatable levels of explanatory and quantified variables that can influence the mean of the dependent variable, and they can be tested. In addition, in a linear mixed-effects model, how the relationship between the dependent variable and one predictor depends on the level of another predictor can be represented via an interaction term.
Random factors are uninformative levels of predictor variables but can explain parts of the residual of the fixed-effects model by calculating different intercepts for different category levels. They are included in mixed-effects models to account for qualitative information from repeated sampling with respect to individuals, time stamps, or treatments. Here, the sensor location and dry period, i.e., date, are taken as random effects.
For the model, we used only paired throughfall and soil moisture measurement locations where both top and bottom sensors provided data during the dry periods. All the considered explanatory drivers, which are included as fixed factors in the model, are listed in Table 1. These factors include abiotic and biotic variables that possibly influence the relative local root water uptake: they are daily spatial average soil water storage, spatial deviation of soil water from the mean, soil water at field capacity, and bulk density of the monitored soil layer.
Table 1List of fixed and random factors considered for estimating the root water uptake patterns through the linear mixed-effects model. Interaction is shown with an “x”.
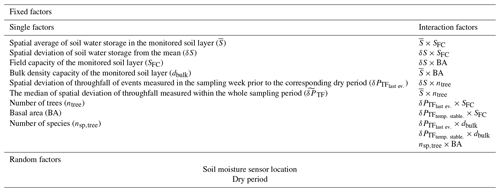
To account for spatial variability in throughfall, we calculated the spatial deviation from the mean by using Eq. (7). Here we considered this variable at two different timescales: the sampling week(s) prior to root water uptake estimation and over the entire throughfall sampling period.
Furthermore, as biotic factors, we included the number of trees, number of species within a 5 m radius of each soil moisture location, and an inverse-distance-weighted basal area (BA) within a 5 m radius of each soil moisture location, calculated as follows:
with
where i is the soil moisture sensor located at xi, R is the tree index located at xR, Atree is the individual basal area of the corresponding tree, and A is the area around the soil moisture sensor i with a 5 m radius.
Even though our research plot is a beech-dominated forest, in some spots, two to four species were present within a 5 m radius of the soil moisture sensors.
We also included interaction terms (Table 1) as fixed factors in the model to capture complex and nonlinear relationships among the biotic and abiotic factors.
We conducted all the analyses with the R statistical software (R Core Team, 2021) and used the lmer function in the lme4 package (Bates et al., 2015) for the model development. We visually checked the model assumptions using the check_model function of the performance package (Lüdecke et al., 2021).
In addition, we calculated both the conditional and marginal R2 of the model with the MuMIn package (Bartoń, 2020). While the conditional R2 includes the variance of the entire model, the marginal R2 subsumes only the fixed effects (Bartoń, 2020). Before fitting the linear mixed-effects model, we tested for co-linearity of the considered variables and scaled the data with a Z transformation by using the scale function in base R (R Core Team, 2021), which allowed us to evaluate the individual effect of fixed effects by comparing slopes and significance levels.
We developed the optimal model by applying a systematic model selection procedure based on Akaike's information criterion (AIC) comparison in combination with the examination of the factors. Model selection began with the beyond-optimal model, which included all possible fixed and random effects. We stepwise evaluated each fixed effect based on its respective significance (p-value comparison) by fitting the model with the maximum likelihood (ML) to be able to compare AIC values (Zuur et al., 2009). In each step, starting with interaction terms, we identified the least significant effect and formulated a model without it. We compared the AIC values of the model before and after removing the effect, discarding it in case the AIC was unaffected or decreased. We followed the procedure with the next equally detected effect and repeated it until only significant fixed effects remained, and the model with the lowest AIC (the optimal model) was obtained.
As a final step, the best model was refitted with a restricted maximum likelihood (REML) (Zuur et al., 2009).
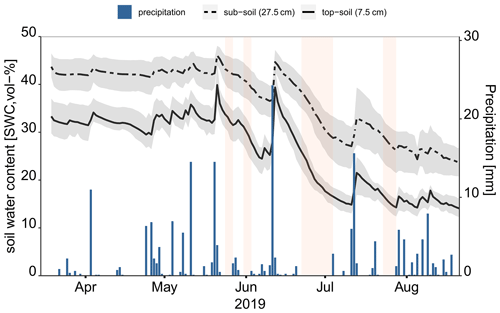
Figure 2Soil moisture temporal variation in topsoil and subsoil together with the daily precipitation measured at the nearby Reckenbühl station (approximately 1.4 km to the northeast). The solid and dashed lines are the spatial mean of the soil water content estimated based on top (7.5 cm) and bottom (27.5 cm) sensors, and grey-shaded areas show the first and third quartiles. The reddish-shaded areas show defined dry periods within the throughfall sampling when root water uptake could be estimated.
Table 2Cumulative potential evapotranspiration in millimeters (Epot,cum), gross precipitation (Pg), ratio of total precipitation to potential evapotranspiration, spatial mean of throughfall based on all the collectors (), spatial mean of throughfall-based paired collectors () in millimeters, interquartile range (IQR), coefficient of quartile variation (CQV), and octile skewness (OS8) of both all and paired throughfall collectors during the sampling week. The values are ordered according to the cumulated gross precipitation size.
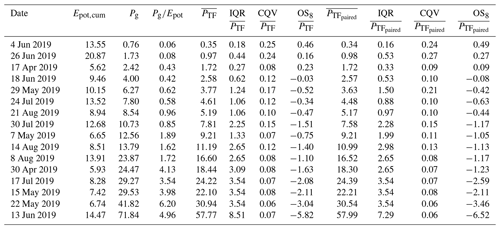
3.1 Spatiotemporal distribution of throughfall and soil water content
In 12 out of the 16 sampling weeks, the weekly gross precipitation was more than half of the total potential evapotranspiration. Table 2 shows the distribution of the throughfall sampled in 2019 (April–August) at 200 collectors and the 98 collectors that were paired with soil moisture sensors. Weekly throughfall increased with an increase in rain. The CQV of throughfall was generally lower for larger cumulative weekly rains. On average, the collectors paired with soil moisture sensors received similar amounts of throughfall to all the collectors (Table 2). The CQV of data from the paired collectors ranged from 0.27 to 0.6, which is similar to the CQV of throughfall sampled at all the collectors. The octile skew (OS8) of the paired collectors and all the collectors was also similar.
As the growing season progressed in 2019, the average soil water content decreased in both the topsoil and subsoil. In April and early May, the average volumetric soil water content in the topsoil was above 30 % and dropped to below 10 % by the end of August. In the subsoil, the volumetric soil water content similarly declined from above 40 % to below 20 % over the sampling period (Fig. 2). On average, soil water changed from 52.5 to 17.5 mm in the topsoil and from 80 to 40 mm in the subsoil.
Table 3The spatial average of the daily volumetric soil water content (, vol. %) in topsoil (0–17.5 cm) and (, vol. %) in subsoil (17.5–37.5 cm) during the defined dry periods. The IQR and CQV of the daily volumetric soil water content in both layers during the dry periods are shown.
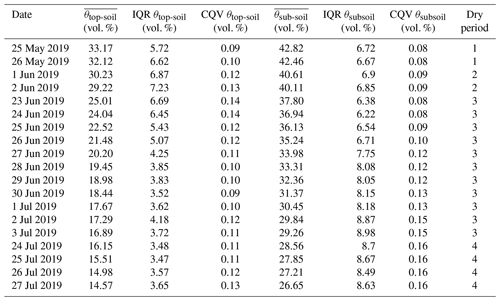
We derived the root water uptake for four periods (a total of 19 d) under different soil wetness conditions that captured the seasonal variation of soil water content, including late spring, when the soil water content was higher, and drier periods during the summer following re-wetted soil conditions with late-summer rains. As listed in Table 3 and shown in Fig. 2, the two periods were in late May and early June, and each lasted 2 d. The third period began in late June and lasted 11 d; the last was 4 d in late July. From the start of the first dry period to the end of the last one, the average soil water content declined from 33 % to 15 % in the topsoil and from 43 % to 27 % in the subsoil. Table 3 shows that, within the dry periods, the CQV of the soil water content was between 0.09 and 0.14 in the topsoil and between 0.08 and 0.16 in the subsoil, respectively. During the dry periods, the spatial heterogeneity of the soil water content in the subsoil increased systematically. In contrast, the spatial variation of the soil water content in the topsoil was not correlated with soil dryness.
3.2 Soil water storage, potential evapotranspiration, and root water uptake
The integrated field capacity of the monitored soil depth was 160 mm on average at the research site. Table 4 shows that soil water was much lower than the field capacity during the dry periods, and the mean soil water storage dropped below 42 mm in late July. In addition, Table 4 demonstrates that the average root water uptake () ranged from 0.94 to 3 mm d−1, while potential evapotranspiration (Epot) ranged from 1.75 to 3.12 mm d−1. The discrepancy between the average root water uptake and the potential evapotranspiration increased as soil water decreased, especially during the longest dry period (Table 4). Root water uptake showed greater spatial variation than water input and soil wetness. The CQV of the root water uptake ranged from 0.15 to 0.28, which was higher than the CQV of the throughfall and volumetric soil water content in both soil layers.
Table 4The daily average air temperature (Tair, °C), potential evapotranspiration (Epot, mm), mean soil water storage (, mm) in the monitored soil layer (0–37.5 cm), and spatial mean of the daily root water uptake (, mm) based on all the soil moisture sensors, together with the ratio of the root water uptake to the potential evapotranspiration and the standard deviation (SD) and CQV of the daily root water uptake during the defined dry periods.
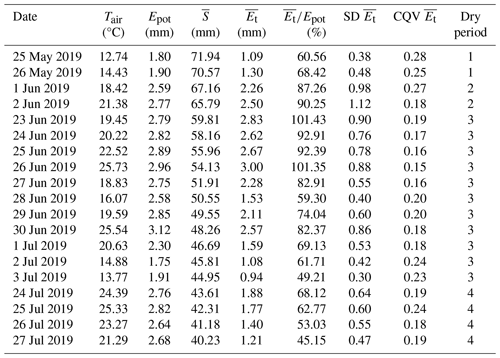
3.3 Soil water, throughfall, and root water uptake patterns
At soil moisture measurement points where the daily root water uptake was determined (n=34), we calculated the spatial deviation from the median of throughfall, soil water storage, and root water uptake to illustrate the spatial patterns. Figure 3 shows that some locations received repeatedly less (or more) throughfall than average (δPTF<0), some locations were repeatedly wetter or drier (δS<0), and some places regularly had lower or higher root water uptake (δEt) throughout the sampling period. However, these locations were not related to each other. In fact, Fig. 3 demonstrates that neither throughfall nor soil water patterns are directly correlated with the root water uptake patterns. For example, the locations with higher water uptake were not coupled with elevated throughfall input (locations colored dark) or higher soil water storage. In addition, soil water storage patterns were not correlated with throughfall patterns.
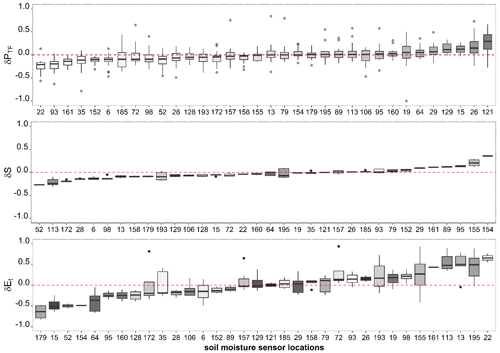
Figure 3Temporal stability of throughfall patterns which is estimated by the spatial deviation from the mean (δPTF) throughout the sampling period in 2019 (April–August), soil water (δS), and root water uptake (δEt) based on the spatial deviation from the mean during the defined dry periods. Soil moisture sensor locations are colored according to throughfall input. Soil moisture sensor locations are colored from lighter to darker throughout the figure according to throughfall input.
3.4 Fixed factors regulating root water uptake patterns
We used a linear mixed-effects model to disentangle the effects of throughfall, soil water, soil properties, and the neighboring tree characteristics on root water uptake patterns. The fixed and random effects contributed almost equally to the model. The R2 of the model was 0.77, and the contribution of the fixed effect to the R2 was 0.39 (see the Supplement for more details on the optimal model).
Figure 4 shows only the significant fixed effects for root water uptake patterns. The spatial deviation of soil water from the mean (i.e., soil water patterns) was the only single and most significant factor positively related to the spatial deviation of the root water uptake. Thus, water uptake was elevated at locations where the most water was retained in the soil at the given time, i.e., greater soil water storage.
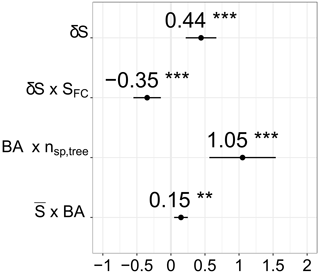
Figure 4The significant fixed factors of the best model to estimate root water uptake patterns (δEt). Values on the x axis indicate the slope of the relations. All the variables were scaled by Z transformation. Interaction is shown with an “x”. Here δS is the spatial deviation of soil water, SFC is the field capacity, nsp,tree is the number of species, BA is the basal area, and is the soil water storage. The significance codes are ≅0 and ≅0.001 (the details on the model can be found in the Supplement).
Field capacity by itself was not a significant factor affecting local root water uptake. However, it strongly influenced how local soil water controlled root water uptake as a part of the significant interaction term. Figure 5a illustrates how root water uptake was more dependent on local soil water when the field capacity was low (i.e., higher macroporosity). In contrast, soil bulk density and therefore total porosity were not part of the final model.
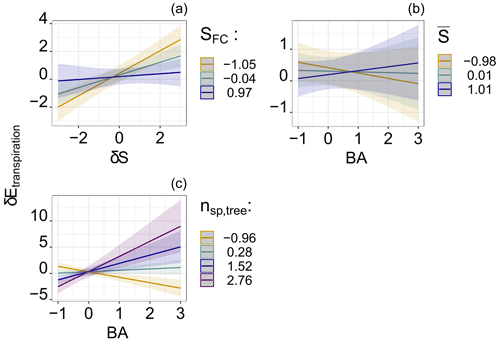
Figure 5Visualisation of the significant relations shown in Fig. 4, representing the significant drivers of root water uptake patterns during the defined dry periods. Relation to the (a) interactive relation of the spatial deviation of soil water storage and field capacity (SFC), (b) interactive relation of the BA and the spatial average of the soil water storage (), and (c) interactive relation of the number of species (nsp,tree) and the BA.
Although the spatial average of soil water storage, e.g., the state of wetness, was not an important factor in local root water uptake by itself, it moderated the impact of the BA on the spatial distribution of water uptake. We found that, as the plot dries, uptake shifts from places with a higher basal area to places with a lower basal area (Fig. 5b). Furthermore, the statistical model revealed that water uptake increased with the higher basal area at locations where multiple species co-existed (Fig. 5c). However, the number of species and the basal area were individually not significant fixed effects. Lastly, throughfall patterns were not significant predictors of local root water uptake. Only the median of the spatial deviation of throughfall, which represents temporally stable patterns within the sampling period (), marginally improved the final model.
We investigated the role of throughfall, soil water patterns, and soil and tree characteristics in the spatial variation of root water uptake. In the following sections we discuss three main findings, which are that, (1) contrary to our hypothesis, throughfall patterns do not play a role in root water uptake patterns despite the recurrence of distinctly localized greater and lesser throughfall inputs. (2) How and where water is stored in the soil, which is strongly determined by soil hydraulic properties, dominates water uptake patterns. (3) The size and species of neighboring trees regulate relative local water uptake such that locations surrounded by more diverse neighborhoods are subject to greater water uptake.
4.1 Spatial variation in throughfall does not affect root water uptake patterns
We adequately captured the spatial distribution and temporal stability of throughfall at locations where local root water uptake was derived. Consistent with previous observations in temperate forests (e.g., Whelan and Anderson, 1996; Staelens et al., 2006; Metzger et al., 2017), the amount of weekly rainfall significantly altered the spatial distribution of throughfall such that more rainfall, and thus more throughfall, resulted in less spatial variability. Previous studies repeatedly showed that throughfall patterns exhibit temporal stability in forest ecosystems (e.g., Keim et al., 2005; Staelens et al., 2006; Wullaert et al., 2009; Rodrigues et al., 2022). At our research site, using event-based sampling, Metzger et al. (2017) and Fischer-Bedtke et al. (2023) demonstrated that throughfall patterns persist over time, which was also true for our weekly sampling in 2019. With canopy cover being the key driver of throughfall (Fischer-Bedtke et al., 2023), it is not surprising that weekly cumulative events resulted in a localized high- and low-throughfall input.
Contrary to expectations (Bouten et al., 1992; Guswa and Spence, 2012; Coenders-Gerrits et al., 2013; Fischer-Bedtke et al., 2023), our results showed that throughfall hotspots do not increase or facilitate greater root water uptake. In addition, the linear mixed-effects model results confirmed that throughfall patterns do not drive the variation in root water uptake. We attributed the absence of this to two reasons: (1) decoupled soil water and throughfall patterns as well as (2) non-water-limited conditions.
Regarding (1), we confirmed that the temporally stable throughfall patterns do not correspond to the post-event soil water and root water uptake patterns. We paired the measurements of throughfall and soil water content measurements – and thus the estimates of root water uptake – within a distance of 1 m. The spatial correlation length of soil water content and throughfall is on the order of 6–10 m in natural temperate forests (Keim et al., 2005; Gerrits et al., 2010; Zehe et al., 2010). At the same study site with the spatially extended throughfall sampling, Fischer-Bedtke et al. (2023) found that the throughfall correlation length increased with decreasing event size, varying from 6.2 to 9.5 m depending on the sizes of the rain events. Thus, the paired sampling design in our study likely provided co-located throughfall and soil moisture measurements. However, variation in soil water storage was not related to throughfall patterns despite temporally persistent local high- and low-throughfall inputs.
Some studies, mostly conducted in the arid regions and coniferous forests, reported that soil wetting patterns were not or only partly linked to throughfall variation, despite recurrent throughfall patterns (Raat et al., 2002; Shachnovich et al., 2008; Zhu et al., 2021). Forest floor thickness, horizontal water flow, and soil properties were suggested as reasons for the decoupled patterns. Other modeling and field studies conducted in temperate deciduous forests found that throughfall patterns influenced soil moisture response to rain events rather than post-event soil water storage variability (Coenders-Gerrits et al., 2013; Metzger et al., 2017; Fischer et al., 2023). These studies attributed possible reasons to local processes such as preferential flow due to soil water repellency, the soil pore structure, or elevated root water uptake. Our results support it not being root water uptake but preferential flow paths that are likely to decouple the throughfall and soil water patterns. In fact, Fischer-Bedtke et al. (2023), using independent throughfall and soil water content sampling designs, demonstrated that the signature of throughfall patterns dissipated in the post-event soil water variation. However, they detected the stronger influence of throughfall patterns in the soil moisture response to rainfall in the 2015 and 2016 growing seasons. The temporal variation in soil water content in the 2019 growing season was similar to the seasonal decline in soil water content in 2015 (Metzger et al., 2017). Dry soil conditions can lead to rapid drainage due to reduced water-holding capability (Jost et al., 2004; Blume et al., 2009; Wiekenkamp et al., 2016; Demand et al., 2019; Molina et al., 2019) regardless of throughfall amount and its variation. Therefore, our findings support the localized throughfall input potentially enhancing preferential flow because of low soil retention (Fischer-Bedtke et al., 2023) rather than local root water uptake. As a result, the fast-flow processes likely dominate how water is stored and transported at our site, erasing the throughfall distribution signature in soil water and root water uptake patterns. Moreover, any short-term response of uptake to throughfall could not be captured as water uptake was calculated only after 56 h had elapsed since the last rain event, and we showed that temporally stable hotspots are not associated with elevated water uptake. Hence, our results are consistent with previous propositions stating that the spatial variation of throughfall affects drainage and subsurface flow (Keim et al., 2006; Blume et al., 2009; Guswa and Spence, 2012), while root activities such as water uptake and hydraulic redistribution do not alter canopy-attributed heterogeneity in drainage pathways (Guswa, 2012).
Reason (2) is related to water-limitation conditions. In central Europe, 2019 was the second consecutive extremely dry summer (Boergens et al., 2020), which damaged beech forests (Obladen et al., 2021). On average, however, the potential evapotranspiration demand was met at the study site despite the low soil water storage. The ratio of root water uptake to potential evapotranspiration was mostly above 65 %, which is within the expected range even in the absence of shallow groundwater storage (Nie et al., 2021). Hence, local biotic and soil-tied abiotic factors determined the spatial variation of root water uptake during the growing season rather than throughfall – water input – patterns. However, the discrepancy between daily potential evapotranspiration and root water uptake only increased as the soil in the sampled layers dried out, due to a potential shift in the water uptake depth (see below).
4.2 Relative and average soil wetness shapes root water uptake patterns
We found that spatial variation in soil water storage strongly regulates local water uptake such that wetter locations enhance root water uptake. This finding is consistent with expectations as transpiration rate relies on soil water availability and distribution (Couvreur et al., 2014; Klein et al., 2014; Hildebrandt et al., 2016). Here, we provide further support for root water uptake being likely to reduce the spatial variability in soil water storage, as has been previously suggested (Hopmans and Bristow, 2002; Ivanov et al., 2010; Neumann and Cardon, 2012).
Trees take up more water in locations where water is not subject to throughfall-driven rapid drainage (see above). As a result, root water uptake patterns are determined by where water is retained longer in the soil. Our results support previous studies suggesting that tree transpiration demand is met by water with a longer residence time in the soil matrix – passive storage – while groundwater recharge is fed by rapid flow – active storage (e.g., Evaristo et al., 2019; Sprenger et al., 2019). In our statistical analyses, we investigated the soil properties of bulk density and field capacity, which are strongly dependent on other soil properties that control aggregation and soil structure. Although bulk density is strongly related to texture, porosity, and soil organic carbon content, all of which also affect water retention (Zacharias and Wessolek, 2007; Looy et al., 2017), surprisingly, soil bulk density was not retained as a predictive variable in the optimal model. In contrast, the interaction term including field capacity and local soil water storage was significant in the model with a negative relationship with relative water uptake, showing that the combination of higher field capacity (fewer macropores) and low soil water hinders water uptake because water more is more strongly bound in the soil. Differences in local soil properties regulate the matric potential at a certain soil wetness. Thus, wetter locations do not necessarily correspond to those of easier root water uptake due to differences in the soil water retention characteristics (Vereecken et al., 2007; Cai et al., 2018) for which field capacity serves as a proxy. However, soil properties alone were less important (smaller effects size of the interaction term, including field capacity) than other factors despite their control on the spatial distribution of soil moisture (Vereecken et al., 2022).
In addition, the spatial mean of soil water – a measure of the overall wetness of the stand – influenced root water uptake patterns, yet the effect depended on the basal area of neighboring trees. We found that, as the study site dries out, local water uptake increased in locations with smaller basal areas. Conversely, wetter site conditions facilitate greater water uptake at locations with higher basal areas, i.e., dense clusters of large trees. We interpret this as a sign that larger trees are likely to shift their water uptake to deeper soil layers to meet transpiration demands, beyond the monitored soil depth (37 cm), as follows.
A higher basal area is likely to increase transpiration demand and enhance water uptake as long as water is available. Moreover, locations with a higher basal area exhaust the water storage more rapidly as these locations host a larger root structure and root biomass (Le Goff and Ottorini, 2001). At the same time, larger-sized trees can shift uptake to deeper layers (Gaines et al., 2016).
Beech trees have extensive root systems at shallower depths similar to other temperate tree species, such as European ash and sycamore maple (Kreuzwieser and Gessler, 2010; Brinkmann et al., 2019). Despite their shallower root system (Leuschner, 2020) in response to declining soil water content in the topsoil, temperate tree species can tap water from the deeper soil layers (Brinkmann et al., 2019; Agee et al., 2021; Seeger and Weiler, 2021). Recently, Agee et al. (2021) used a three-dimensional water uptake model based on observations in a temperate mixed deciduous forest to show that water uptake is shifted to the deeper soil layers as soil moisture depletes, which is consistent with the field observations. Moreover, Krämer and Hölscher (2010) observed in beech and mixed deciduous stands that roots can extract water at depths down to 70 cm soil depth. Similar to our site, theirs had a shallow soil layer underlain by weathered limestone, but the soil depth varied between 50 and 120 cm. Brinkmann et al. (2019) also observed a similar depth range for beech trees in a mixed forest by tracing stable water isotopes of soil and xylem water.
Furthermore, tree age and size can affect both individual and stand level transpiration because of the different physiological characteristics and biometrics of trees associated with them (Kostner et al., 2002; Tsuruta et al., 2023). Within the same species, the larger – presumably older – trees have an advantage in accessing the deeper water storages because of their larger root biomass (Le Goff and Ottorini, 2001), and root plasticity may be able to shift the depth of water uptake, while younger trees rely on shallower soil water storages (Dawson, 1996). Our results can be interpreted as tree size, which can be attributed to tree age, affecting root water uptake patterns through differential root biomass development. Furthermore, in the Hainich range the coexisting species most likely represent a highly coherent rooting depth distribution among trees (Gebauer et al., 2012; Meinen et al., 2009) yet adopt different water uptake strategies (see below). Hence, consistent with previous studies focusing on temperate tree species, the linear mixed-effects model results indicate that trees of different sizes respond to declining soil water content by shifting water uptake depth.
4.3 Tree species richness regulates root water uptake patterns
In addition to the basal area, we included the number of species and the number of tree individuals in the linear mixed-effects analysis to further explore the biotic drivers of root water uptake patterns. While the number of trees was unimportant, the number of species and the basal area showed a significant interaction effect on the local water uptake. The result indicates that an increase in species richness leads to greater root water uptake, depending on the size and/or density of the neighboring trees: a higher basal area, combined with more species, elevates water uptake. In other words, the interactions between neighboring tree species strongly determine root water uptake patterns, and for the same basal area, more water can be taken up in a diverse neighborhood than in a less diverse location.
In temperate forests, transpiration has been observed to change with tree species richness at the stand level (Krämer and Hölscher, 2010; Gebauer et al., 2012; Kunert et al., 2012; Meißner et al., 2012; Forrester, 2014). Although some studies indicate a positive relationship between tree diversity and water uptake rate (Forrester et al., 2010; Krämer and Hölscher, 2010; Kunert et al., 2012), tree species diversity is not always positively related to water uptake. While Krämer and Hölscher (2010) observed a positive correlation between water uptake and species richness of the plots in the upper soil layers during soil drying in 2006 at the same study site, Meißner et al. (2012) found no relationship between tree diversity and root water uptake in 2009. They attributed this finding to wetter soil conditions. In contrast, Lübbe et al. (2016) observed a weak effect of diversity on transpiration under wetter soil conditions but not under drier conditions compared to previous studies (e.g., Pretzsch et al., 2013; del Río et al., 2014). Shortage of water can inflate competition mechanisms for water among tree species (González de Andrés et al., 2018; Vitali et al., 2018; Magh et al., 2020). Our results indicate that competition between neighboring tree species increases water uptake capacity in more diverse spots (Wambsganss et al., 2021).
In addition, different co-existing tree species can facilitate resource uptake or reduce competition, depending on the temporal and spatial availability of the sources, which is often defined as complementarity (Forrester and Bauhus, 2016). As reviewed and listed by Silvertown et al. (2015), several studies suggest that co-existing tree species reduce competition for subsurface water sources by adopting different vertical root water uptake strategies, referred to as hydrological niche partitioning. In addition, trees can transport water from wet to dry parts of the soil layers through their roots (Neumann and Cardon, 2012). The mechanism is called hydraulic redistribution or hydraulic lift, which can provide water availability to the shallow roots in drier layers (Burgess et al., 1998; Jonard et al., 2011; Hafner et al., 2017, 2021; Lee et al., 2018; Rodríguez-Robles et al., 2020). In an experiment with six temperate tree species, including European beech, Hafner et al. (2021) found that the neighboring tree species diversity may not be important for exploiting water uptake through hydraulic redistribution. Both hydraulic niche partitioning and redistribution have been observed vertically, whereas horizontal patterns are largely unexplored in the context of niche partitioning (Hildebrandt, 2020). Our results do not provide direct evidence for either hydraulic redistribution or horizontal niche partitioning. However, they indicate that horizontal root water uptake patterns are regulated by species richness and interactions between neighboring trees. Thus, here we emphasize the complex interplay between tree species diversity, complementary mechanisms, and water uptake patterns, which is consistent not only with the abovementioned plot-scale studies, but also with larger-scale studies. For instance, Knighton et al. (2019), using the Budyko framework across more than 100 catchments, found that transpiration losses in catchments with deep-rooted and mixed-species forests differed from those in monoculture catchments. In other words, both plot- and catchment-scale studies support our results showing that interactions among different coexisting species play a significant role in the spatiotemporal variation of root water uptake.
We investigated the factors that influence the spatial patterns of root water uptake by considering heterogeneity in throughfall and soil water. To that end, we acquired a comprehensive dataset based on throughfall measurements paired with soil moisture sensors in a mixed deciduous forest. Soil and neighboring tree characteristics were also included in the linear mixed-effects model. We found that variation in root water uptake did not correspond to throughfall, consequently rejecting our hypothesis that variation in throughfall is imprinted in water uptake patterns. Wetter soil locations, also poorly associated with higher throughfall, increased local root water uptake. In contrast, how average soil water conditions modified root water uptake depended on the neighborhood basal area. As the site dried out, large trees likely took up water in deeper layers to meet transpiration demands. Furthermore, an increase in species diversity promoted root water uptake, similarly depending on the sizes of neighboring trees and suggesting active complementarity mechanisms in the forest stand. In conclusion, our results show that soil water distribution and neighboring tree characteristics regulate root water uptake patterns more than soil properties and throughfall variation.
The datasets are available at
-
https://doi.org/10.5281/zenodo.10563567 (Demir et al., 2024a),
-
https://doi.org/10.5281/zenodo.10563472 (Demir et al., 2024b),
-
https://doi.org/10.5281/zenodo.10563871 (Demir et al., 2024c),
-
https://doi.org/10.5281/zenodo.8065170 (Metzger et al., 2023) and
-
https://doi.org/10.5281/zenodo.10564735 (Demir and Hildebrandt, 2024).
The supplement related to this article is available online at: https://doi.org/10.5194/hess-28-1441-2024-supplement.
GD and AH designed the throughfall measurement setup, and AH and JCM designed the soil moisture measurement. GD conducted the field sampling with assistance from JF and the students listed in the Acknowledgements section. GD analyzed the data, developed the linear mixed-effects model, and analyzed the results with AH and AG. GD prepared the first version of the manuscript, and all the authors contributed to discussions and the final version of the manuscript.
At least one of the (co-)authors is a member of the editorial board of Hydrology and Earth System Sciences. The peer-review process was guided by an independent editor, and the authors also have no other competing interests to declare.
Publisher's note: Copernicus Publications remains neutral with regard to jurisdictional claims made in the text, published maps, institutional affiliations, or any other geographical representation in this paper. While Copernicus Publications makes every effort to include appropriate place names, the final responsibility lies with the authors.
This study is part of the Collaborative Research Centre (CRC 1076 AquaDiva) of the Friedrich Schiller University Jena, funded by the Deutsche Forschungsgemeinschaft (DFG, German Research Foundation) – SFB 1076 – project no. 218627073. We thank the AquaDiva subproject D03 for the weather station (Reckenbuel) data and the people who contributed to the installation of soil moisture sensors at the research site: Ricardo Ontiveros-Enriques, Bernd Ruppe, Danny Schelhorn, and Josef Weckmüller. We thank the Hainich CZE site manager Robert Lehmann and Hainich National Park. We thank the bachelor and master students Carla Peter, Xiaoyu Zhao, and Stephan Bock for their contributions to throughfall sampling.
This research has been supported by the Deutsche Forschungsgemeinschaft (grant no. SFB 1076 – Projektnummer 218627073).
This paper was edited by Lixin Wang and reviewed by Jaivime Evaristo and one anonymous referee.
Agee, E., He, L., Bisht, G., Couvreur, V., Shahbaz, P., Meunier, F., Gough, C. M., Matheny, A. M., Bohrer, G., and Ivanov, V.: Root lateral interactions drive water uptake patterns under water limitation, Adv. Water Resour., 151, 103896, https://doi.org/10.1016/j.advwatres.2021.103896, 2021.
Bachmair, S., Weiler, M., and Troch, P. A.: Intercomparing hillslope hydrological dynamics: Spatio-temporal variability and vegetation cover effects, Water Resour. Res., 48, W05537, https://doi.org/10.1029/2011WR011196, 2012.
Baroni, G., Ortuani, B., Facchi, A., and Gandolfi, C.: The role of vegetation and soil properties on the spatio-temporal variability of the surface soil moisture in a maize-cropped field, J. Hydrol., 489, 148–159, https://doi.org/10.1016/j.jhydrol.2013.03.007, 2013.
Bartoń, K.: MuMIn: Multi-Model Inference, https://CRAN.Rproject.org/package=MuMIn (last access: 5 July 2021), 2020.
Bates, D., Mächler, M., Bolker, B., and Walker, S.: Fitting Linear Mixed-Effects Models Using lme4, J. Stat. Softw., 67, 1–48, https://doi.org/10.18637/jss.v067.i01, 2015.
Blume, T., Zehe, E., and Bronstert, A.: Use of soil moisture dynamics and patterns at different spatio-temporal scales for the investigation of subsurface flow processes, Hydrol. Earth Syst. Sci., 13, 1215–1233, https://doi.org/10.5194/hess-13-1215-2009, 2009.
Boergens, E., Güntner, A., Dobslaw, H., and Dahle, C.: Quantifying the Central European Droughts in 2018 and 2019 With GRACE Follow-On, Geophys. Res. Lett., 47, e2020GL087285, https://doi.org/10.1029/2020GL087285, 2020.
Bogena, H. R., Herbst, M., Huisman, J. A., Rosenbaum, U., Weuthen, A., and Vereecken, H.: Potential of Wireless Sensor Networks for Measuring Soil Water Content Variability, Vadose Zone J., 9, 1002–1013, https://doi.org/10.2136/vzj2009.0173, 2010.
Borchers, H. W.: pracma: Practical Numerical Math Functions, R package version 2.4.2, https://CRAN.R-project.org/package=pracma (last access: 11 May 2022), 2021.
Bouten, W., Heimovaara, T. J., and Tiktak, A.: Spatial patterns of throughfall and soil water dynamics in a Douglas fir stand, Water Resour. Res., 28, 3227–3233, https://doi.org/10.1029/92WR01764, 1992.
Brinkmann, N., Eugster, W., Buchmann, N., and Kahmen, A.: Species-specific differences in water uptake depth of mature temperate trees vary with water availability in the soil, Plant Biol., 21, 71–81, https://doi.org/10.1111/plb.12907, 2019.
Brum, M., Vadeboncoeur, M. A., Ivanov, V., Asbjornsen, H., Saleska, S., Alves, L. F., Penha, D., Dias, J. D., Aragão, L. E. O. C., Barros, F., Bittencourt, P., Pereira, L., and Oliveira, R. S.: Hydrological niche segregation defines forest structure and drought tolerance strategies in a seasonal Amazon forest, J. Ecol., 107, 318–333, https://doi.org/10.1111/1365-2745.13022, 2019.
Burgess, S. S. O., Adams, M. A., Turner, N. C., and Ong, C. K.: The redistribution of soil water by tree root systems, Oecologia, 115, 306–311, https://doi.org/10.1007/s004420050521, 1998.
Cai, G., Vanderborght, J., Langensiepen, M., Schnepf, A., Hüging, H., and Vereecken, H.: Root growth, water uptake, and sap flow of winter wheat in response to different soil water conditions, Hydrol. Earth Syst. Sci., 22, 2449–2470, https://doi.org/10.5194/hess-22-2449-2018, 2018.
Cardon, G. E. and Letey, J.: Plant Water Uptake Terms Evaluated for Soil Water and Solute Movement Models, Soil Sci. Soc. Am. J., 56, 1876–1880, https://doi.org/10.2136/sssaj1992.03615995005600060038x, 1992.
Carlyle-Moses, D. E., Lishman, C. E., and McKee, A. J.: A preliminary evaluation of throughfall sampling techniques in a mature coniferous forest, J. Forest. Res., 25, 407–413, https://doi.org/10.1007/s11676-014-0468-8, 2014.
Coenders-Gerrits, A. M. J., Hopp, L., Savenije, H. H. G., and Pfister, L.: The effect of spatial throughfall patterns on soil moisture patterns at the hillslope scale, Hydrol. Earth Syst. Sci., 17, 1749–1763, https://doi.org/10.5194/hess-17-1749-2013, 2013.
Cosh, M. H., Jackson, T. J., Moran, S., and Bindlish, R.: Temporal persistence and stability of surface soil moisture in a semi-arid watershed, Remote Sens. Environ., 112, 304–313, https://doi.org/10.1016/j.rse.2007.07.001, 2008.
Couvreur, V., Vanderborght, J., Beff, L., and Javaux, M.: Horizontal soil water potential heterogeneity: simplifying approaches for crop water dynamics models, Hydrol. Earth Syst. Sci., 18, 1723–1743, https://doi.org/10.5194/hess-18-1723-2014, 2014.
Crockford, R. H. and Richardson, D. P.: Partitioning of rainfall into throughfall, stemflow and interception: effect of forest type, ground cover and climate, Hydrol. Process., 14, 2903–2920, 2000.
Dawson, T. E.: Determining water use by trees and forests from isotopic, energy balance and transpiration analyses: the roles of tree size and hydraulic lift, Tree Physiol., 16, 263–272, https://doi.org/10.1093/treephys/16.1-2.263, 1996.
del Río, M., Schütze, G., and Pretzsch, H.: Temporal variation of competition and facilitation in mixed species forests in Central Europe, Plant Biol., 16, 166–176, https://doi.org/10.1111/plb.12029, 2014.
Demand, D., Blume, T., and Weiler, M.: Spatio-temporal relevance and controls of preferential flow at the landscape scale, Hydrol. Earth Syst. Sci., 23, 4869–4889, https://doi.org/10.5194/hess-23-4869-2019, 2019.
Demir, G. and Hildebrandt, A.: Root water uptake, May–July 2019, Hainich, Germany, project AquaDiva, Zenodo [data set], https://doi.org/10.5281/zenodo.10564735, 2024.
Demir, G., Michalzik, B., Filipzik, J., Metzger, J., and Hildebrandt, A.: Spatial variation of grassland canopy affects soil wetting patterns and preferential flow, AUTHOREA, https://doi.org/10.22541/au.164970545.54927607/v1, 2022.
Demir, G., Hildebrandt, A., and Filipzik, J.: Weekly cumulated throughfall and rain data, April–August 2019, Hainich, Germany, project AquaDiva, Zenodo [data set], https://doi.org/10.5281/zenodo.10563567, 2024a.
Demir, G., Metzger, J. C., and Hildebrandt, A.: High-resolution throughfall measurement design since 2019, Hainich, Germany, project AquaDiva, Zenodo [data set], https://doi.org/10.5281/zenodo.10563472, 2024b.
Demir, G., Hildebrandt, A., and Filipzik, J.: High-resolution soil water content, March–August 2019, Hainich, Germany, project AquaDiva, Zenodo [data set], https://doi.org/10.5281/zenodo.10563871, 2024c.
Dunkerley, D.: Stemflow on the woody parts of plants: dependence on rainfall intensity and event profile from laboratory simulations, Hydrol. Process., 28, 5469–5482, https://doi.org/10.1002/hyp.10050, 2014.
Emerman, S. H. and Dawson, T. E.: Hydraulic Lift and Its Influence on the Water Content of the Rhizosphere: An Example from Sugar Maple, Acer saccharum, Oecologia, 108, 273–278, 1996.
Evaristo, J., Kim, M., van Haren, J., Pangle, L. A., Harman, C. J., Troch, P. A., and McDonnell, J. J.: Characterizing the Fluxes and Age Distribution of Soil Water, Plant Water, and Deep Percolation in a Model Tropical Ecosystem, Water Resour. Res., 55, 3307–3327, https://doi.org/10.1029/2018WR023265, 2019.
Fan, J., Oestergaard, K. T., Guyot, A., Jensen, D. G., and Lockington, D. A.: Spatial variability of throughfall and stemflow in an exotic pine plantation of subtropical coastal Australia, Hydrol. Process., 29, 793–804, https://doi.org/10.1002/hyp.10193, 2015.
Fischer-Bedtke, C., Metzger, J. C., Demir, G., Wutzler, T., and Hildebrandt, A.: Throughfall spatial patterns translate into spatial patterns of soil moisture dynamics – empirical evidence, Hydrol. Earth Syst. Sci., 27, 2899–2918, https://doi.org/10.5194/hess-27-2899-2023, 2023.
Forrester, D. I.: The spatial and temporal dynamics of species interactions in mixed-species forests: From pattern to process, Forest Ecol. Manage., 312, 282–292, https://doi.org/10.1016/j.foreco.2013.10.003, 2014.
Forrester, D. I. and Bauhus, J.: A Review of Processes Behind Diversity – Productivity Relationships in Forests, Curr. Forest. Rep., 2, 45–61, https://doi.org/10.1007/s40725-016-0031-2, 2016.
Forrester, D. I., Theiveyanathan, S., Collopy, J. J., and Marcar, N. E.: Enhanced water use efficiency in a mixed Eucalyptus globulus and Acacia mearnsii plantation, Forest Ecol. Manage., 259, 1761–1770, https://doi.org/10.1016/j.foreco.2009.07.036, 2010.
Gaines, K. P., Stanley, J. W., Meinzer, F. C., McCulloh, K. A., Woodruff, D. R., Chen, W., Adams, T. S., Lin, H., and Eissenstat, D. M.: Reliance on shallow soil water in a mixed-hardwood forest in central Pennsylvania, Tree Physiol., 36, 444–458, https://doi.org/10.1093/treephys/tpv113, 2016.
Gebauer, T., Horna, V., and Leuschner, C.: Canopy transpiration of pure and mixed forest stands with variable abundance of European beech, J. Hydrol., 442–443, 2–14, https://doi.org/10.1016/j.jhydrol.2012.03.009, 2012.
Gerrits, A. M. J., Pfister, L., and Savenije, H. H. G.: Spatial and temporal variability of canopy and forest floor interception in a beech forest, Hydrol. Process., 24, 3011–3025, https://doi.org/10.1002/hyp.7712, 2010.
González de Andrés, E., Camarero, J. J., Blanco, J. A., Imbert, J. B., Lo, Y.-H., Sangüesa-Barreda, G., and Castillo, F. J.: Tree-to-tree competition in mixed European beech–Scots pine forests has different impacts on growth and water-use efficiency depending on site conditions, J. Ecol., 106, 59–75, https://doi.org/10.1111/1365-2745.12813, 2018.
Grayson, R. B., Western, A. W., Chiew, F. H. S., and Blöschl, G.: Preferred states in spatial soil moisture patterns: Local and nonlocal controls, Water Resour. Res., 33, 2897–2908, https://doi.org/10.1029/97WR02174, 1997.
Guderle, M. and Hildebrandt, A.: Using measured soil water contents to estimate evapotranspiration and root water uptake profiles – a comparative study, Hydrol. Earth Syst. Sci., 19, 409–425, https://doi.org/10.5194/hess-19-409-2015, 2015.
Guderle, M., Bachmann, D., Milcu, A., Gockele, A., Bechmann, M., Fischer, C., Roscher, C., Landais, D., Ravel, O., Devidal, S., Roy, J., Gessler, A., Buchmann, N., Weigelt, A., and Hildebrandt, A.: Dynamic niche partitioning in root water uptake facilitates efficient water use in more diverse grassland plant communities, Funct. Ecol., 32, 214–227, https://doi.org/10.1111/1365-2435.12948, 2018.
Guo, J. S., Hungate, B. A., Kolb, T. E., and Koch, G. W.: Water source niche overlap increases with site moisture availability in woody perennials, Plant Ecol., 219, 719–735, https://doi.org/10.1007/s11258-018-0829-z, 2018.
Guswa, A. J.: Canopy vs. Roots: Production and Destruction of Variability in Soil Moisture and Hydrologic Fluxes, Vadose Zone J., 11, vzj2011.0159, https://doi.org/10.2136/vzj2011.0159, 2012.
Guswa, A. J. and Spence, C. M.: Effect of throughfall variability on recharge: application to hemlock and deciduous forests in western Massachusetts, Ecohydrology, 5, 563–574, https://doi.org/10.1002/eco.28, 2012.
Hafner, B. D., Tomasella, M., Häberle, K.-H., Goebel, M., Matyssek, R., and Grams, T. E. E.: Hydraulic redistribution under moderate drought among English oak, European beech and Norway spruce determined by deuterium isotope labeling in a split-root experiment, Tree Physiol., 37, 950–960, https://doi.org/10.1093/treephys/tpx050, 2017.
Hafner, B. D., Hesse, B. D., and Grams, T. E. E.: Friendly neighbours: Hydraulic redistribution accounts for one quarter of water used by neighbouring drought stressed tree saplings, Plant Cell Environ., 44, 1243–1256, https://doi.org/10.1111/pce.13852, 2021.
Hildebrandt, A.: Root-Water Relations and Interactions in Mixed Forest Settings, in: Forest-Water Interactions, edited by: Levia, D. F., Carlyle-Moses, D. E., Iida, S., Michalzik, B., Nanko, K., and Tischer, A., Springer International Publishing, Cham, 319–348, https://doi.org/10.1007/978-3-030-26086-6_14, 2020.
Hildebrandt, A., Kleidon, A., and Bechmann, M.: A thermodynamic formulation of root water uptake, Hydrol. Earth Syst. Sci., 20, 3441–3454, https://doi.org/10.5194/hess-20-3441-2016, 2016.
Hopmans, J. W. and Bristow, K. L.: Current Capabilities and Future Needs of Root Water and Nutrient Uptake Modeling, Adv. Agron., 77, 103–183, https://doi.org/10.1016/S0065-2113(02)77014-4, 2002.
Hupet, F. and Vanclooster, M.: Micro-variability of hydrological processes at the maize row scale: implications for soil water content measurements and evapotranspiration estimates, J. Hydrol., 303, 247–270, https://doi.org/10.1016/j.jhydrol.2004.07.017, 2005.
Hupet, F., Lambot, S., Javaux, M., and Vanclooster, M.: On the identification of macroscopic root water uptake parameters from soil water content observations, Water Resour. Res., 38, 36-1–36-14, https://doi.org/10.1029/2002WR001556, 2002.
IUSS Working Group: World reference base for soil resources 2006, A framework for international classification, correlation and communication, World Soil Resources Reports, 103 pp., 2006.
Ivanov, V. Y., Fatichi, S., Jenerette, G. D., Espeleta, J. F., Troch, P. A., and Huxman, T. E.: Hysteresis of soil moisture spatial heterogeneity and the “homogenizing” effect of vegetation, Water Resour. Res., 46, W09521, https://doi.org/10.1029/2009WR008611, 2010.
Jackisch, C., Knoblauch, S., Blume, T., Zehe, E., and Hassler, S. K.: Estimates of tree root water uptake from soil moisture profile dynamics, Biogeosciences, 17, 5787–5808, https://doi.org/10.5194/bg-17-5787-2020, 2020.
Jarecke, K. M., Bladon, K. D., and Wondzell, S. M.: The Influence of Local and Nonlocal Factors on Soil Water Content in a Steep Forested Catchment, Water Resour. Res., 57, e2020WR028343, https://doi.org/10.1029/2020WR028343, 2021.
Jonard, F., André, F., Ponette, Q., Vincke, C., and Jonard, M.: Sap flux density and stomatal conductance of European beech and common oak trees in pure and mixed stands during the summer drought of 2003, J. Hydrol., 409, 371–381, https://doi.org/10.1016/j.jhydrol.2011.08.032, 2011.
Jost, G., Schume, H., and Hager, H.: Factors controlling soil water-recharge in a mixed European beech (Fagus sylvatica L.) – Norway spruce [Picea abies (L.) Karst.] stand, Eur. J. Forest Res., 123, 93–104, https://doi.org/10.1007/s10342-004-0033-7, 2004.
Katul, G. G. and Siqueira, M. B.: Biotic and abiotic factors act in coordination to amplify hydraulic redistribution and lift, New Phytol., 187, 3–6, 2010.
Keim, R. F., Skaugset, A. E., and Weiler, M.: Temporal persistence of spatial patterns in throughfall, J. Hydrol., 314, 263–274, https://doi.org/10.1016/j.jhydrol.2005.03.021, 2005.
Keim, R. F., Skaugset, A. E., and Weiler, M.: Storage of water on vegetation under simulated rainfall of varying intensity, Adv. Water Resour., 29, 974–986, https://doi.org/10.1016/j.advwatres.2005.07.017, 2006.
Kirchen, G., Calvaruso, C., Granier, A., Redon, P.-O., Van der Heijden, G., Bréda, N., and Turpault, M.-P.: Local soil type variability controls the water budget and stand productivity in a beech forest, Forest Ecol. Manage., 390, 89–103, https://doi.org/10.1016/j.foreco.2016.12.024, 2017.
Kleidon, A. and Renner, M.: Thermodynamic limits of hydrologic cycling within the Earth system: concepts, estimates and implications, Hydrol. Earth Syst. Sci., 17, 2873–2892, https://doi.org/10.5194/hess-17-2873-2013, 2013.
Kleidon, A., Renner, M., and Porada, P.: Estimates of the climatological land surface energy and water balance derived from maximum convective power, Hydrol. Earth Syst. Sci., 18, 2201–2218, https://doi.org/10.5194/hess-18-2201-2014, 2014.
Klein, T., Rotenberg, E., Cohen-Hilaleh, E., Raz-Yaseef, N., Tatarinov, F., Preisler, Y., Ogée, J., Cohen, S., and Yakir, D.: Quantifying transpirable soil water and its relations to tree water use dynamics in a water-limited pine forest, Ecohydrology, 7, 409–419, https://doi.org/10.1002/eco.1360, 2014.
Knighton, J., Singh, K., and Evaristo, J.: Understanding Catchment-Scale Forest Root Water Uptake Strategies Across the Continental United States Through Inverse Ecohydrological Modeling, Geophys. Res. Lett., 47, e2019GL085937, https://doi.org/10.1029/2019GL085937, 2019.
Kohlhepp, B., Lehmann, R., Seeber, P., Küsel, K., Trumbore, S. E., and Totsche, K. U.: Aquifer configuration and geostructural links control the groundwater quality in thin-bedded carbonate–siliciclastic alternations of the Hainich CZE, central Germany, Hydrol. Earth Syst. Sci., 21, 6091–6116, https://doi.org/10.5194/hess-21-6091-2017, 2017.
Kostner, B., Falge, E., and Tenhunen, J. D.: Age-related effects on leaf area/sapwood area relationships, canopy transpiration and carbon gain of Norway spruce stands (Picea abies) in the Fichtelgebirge, Germany, Tree Physiol., 22, 567–574, https://doi.org/10.1093/treephys/22.8.567, 2002.
Krämer, I. and Hölscher, D.: Soil water dynamics along a tree diversity gradient in a deciduous forest in Central Germany, Ecohydrology, 3, 262–271, https://doi.org/10.1002/eco.103, 2010.
Kreuzwieser, J. and Gessler, A.: Global climate change and tree nutrition: influence of water availability, Tree Physiol., 30, 1221–1234, https://doi.org/10.1093/treephys/tpq055, 2010.
Kühnhammer, K., Kübert, A., Brüggemann, N., Deseano Diaz, P., van Dusschoten, D., Javaux, M., Merz, S., Vereecken, H., Dubbert, M., and Rothfuss, Y.: Investigating the root plasticity response of Centaurea jacea to soil water availability changes from isotopic analysis, New Phytol., 226, 98–110, https://doi.org/10.1111/nph.16352, 2020.
Kunert, N., Schwendenmann, L., Potvin, C., and Hölscher, D.: Tree diversity enhances tree transpiration in a Panamanian forest plantation, J. Appl. Ecol., 49, 135–144, https://doi.org/10.1111/j.1365-2664.2011.02065.x, 2012.
Küsel, K., Totsche, K. U., Trumbore, S. E., Lehmann, R., Steinhäuser, C., and Herrmann, M.: How Deep Can Surface Signals Be Traced in the Critical Zone? Merging Biodiversity with Biogeochemistry Research in a Central German Muschelkalk Landscape, Front. Earth Sci., 4, 32, https://doi.org/10.3389/feart.2016.00032, 2016.
Lee, E., Kumar, P., Barron-Gafford, G. A., Hendryx, S. M., Sanchez-Cañete, E. P., Minor, R. L., Colella, T., and Scott, R. L.: Impact of Hydraulic Redistribution on Multispecies Vegetation Water Use in a Semiarid Savanna Ecosystem: An Experimental and Modeling Synthesis, Water Resour. Res., 54, 4009–4027, https://doi.org/10.1029/2017WR021006, 2018.
Le Goff, N. and Ottorini, J.-M.: Root biomass and biomass increment in a beech (Fagus sylvatica L.) stand in North-East France, Ann. Forest Sci., 58, 1–13, https://doi.org/10.1051/forest:2001104, 2001.
Leuschner, C.: Drought response of European beech (Fagus sylvatica L.) – A review, Perspect. Plant Ecol. Evol. Syst., 47, 125576, https://doi.org/10.1016/j.ppees.2020.125576, 2020.
Levia, D. F. and Frost, E. E.: A review and evaluation of stemflow literature in the hydrologic and biogeochemical cycles of forested and agricultural ecosystems, J. Hydrol., 274, 1–29, https://doi.org/10.1016/S0022-1694(02)00399-2, 2003.
Levia, D. F. and Frost, E. E.: Variability of throughfall volume and solute inputs in wooded ecosystems, Prog. Phys. Geogr., 30, 605–632, https://doi.org/10.1177/0309133306071145, 2006.
Levia, D. F., Keim, R. F., Carlyle-Moses, D. E., and Frost, E. E.: Throughfall and Stemflow in Wooded Ecosystems, in: Forest Hydrology and Biogeochemistry: Synthesis of Past Research and Future Directions, edited by: Levia, D. F., Carlyle-Moses, D., and Tanaka, T., Springer Netherlands, Dordrecht, 425–443, https://doi.org/10.1007/978-94-007-1363-5_21, 2011.
Levia, D. F., Hudson, S. A., Llorens, P., and Nanko, K.: Throughfall drop size distributions: a review and prospectus for future research: Throughfall drop size distributions, WIREs Water, 4, e1225, https://doi.org/10.1002/wat2.1225, 2017.
Lhomme, J.-P.: Formulation of root water uptake in a multi-layer soil-plant model: does van den Honert's equation hold?, Hydrol. Earth Syst. Sci., 2, 31–39, https://doi.org/10.5194/hess-2-31-1998, 1998.
Looy, K. V., Bouma, J., Herbst, M., Koestel, J., Minasny, B., Mishra, U., Montzka, C., Nemes, A., Pachepsky, Y. A., Padarian, J., Schaap, M. G., Tóth, B., Verhoef, A., Vanderborght, J., van der Ploeg, M. J., Weihermüller, L., Zacharias, S., Zhang, Y., and Vereecken, H.: Pedotransfer Functions in Earth System Science: Challenges and Perspectives, Rev. Geophys., 55, 1199–1256, https://doi.org/10.1002/2017RG000581, 2017.
Lübbe, T., Schuldt, B., Coners, H., and Leuschner, C.: Species diversity and identity effects on the water consumption of tree sapling assemblages under ample and limited water supply, Oikos, 125, 86–97, https://doi.org/10.1111/oik.02367, 2016.
Lüdecke, D., Ben-Shachar, M., Patil, I., Waggoner, P., and Makowski, D.: performance: An R Package for Assessment, Comparison and Testing of Statistical Models, J. Open Sour. Softw., 6, 3139, https://doi.org/10.21105/joss.03139, 2021.
Magh, R.-K., Eiferle, C., Burzlaff, T., Dannenmann, M., Rennenberg, H., and Dubbert, M.: Competition for water rather than facilitation in mixed beech-fir forests after drying-wetting cycle, J. Hydrol., 587, 124944, https://doi.org/10.1016/j.jhydrol.2020.124944, 2020.
Magliano, P. N., Whitworth-Hulse, J. I., Florio, E. L., Aguirre, E. C., and Blanco, L. J.: Interception loss, throughfall and stemflow by Larrea divaricata: The role of rainfall characteristics and plant morphological attributes, Ecol. Res., 34, 753–764, https://doi.org/10.1111/1440-1703.12036, 2019.
Martínez García, G., Pachepsky, Y. A., and Vereecken, H.: Effect of soil hydraulic properties on the relationship between the spatial mean and variability of soil moisture, J. Hydrol., 516, 154–160, https://doi.org/10.1016/j.jhydrol.2014.01.069, 2014.
Meinen, C., Leuschner, C., Ryan, N. T., and Hertel, D.: No evidence of spatial root system segregation and elevated fine root biomass in multi-species temperate broad-leaved forests, Trees, 23, 941–950, https://doi.org/10.1007/s00468-009-0336-x, 2009.
Meißner, M., Köhler, M., Schwendenmann, L., and Hölscher, D.: Partitioning of soil water among canopy trees during a soil desiccation period in a temperate mixed forest, Biogeosciences, 9, 3465–3474, https://doi.org/10.5194/bg-9-3465-2012, 2012.
Metzger, J. C., Wutzler, T., Valle, N. D., Filipzik, J., Grauer, C., Lehmann, R., Roggenbuck, M., Schelhorn, D., Weckmüller, J., Küsel, K., Totsche, K. U., Trumbore, S., and Hildebrandt, A.: Vegetation impacts soil water content patterns by shaping canopy water fluxes and soil properties, Hydrol. Process., 31, 3783–3795, https://doi.org/10.1002/hyp.11274, 2017.
Metzger, J. C., Filipzik, J., Michalzik, B., and Hildebrandt, A.: Stemflow Infiltration Hotspots Create Soil Microsites Near Tree Stems in an Unmanaged Mixed Beech Forest, Front. Forest. Glob. Change, 4, 701293, https://doi.org/10.3389/ffgc.2021.701293, 2021.
Metzger, J. C., Hildebrandt, A., and Filipzik, J.: Soil moisture sensor network, design, location attributes and soil properties, Hainich, Germany, project AquaDiva, Zenodo [data set], https://doi.org/10.5281/zenodo.8065170, 2023.
Molina, A. J., Llorens, P., Garcia-Estringana, P., Moreno de las Heras, M., Cayuela, C., Gallart, F., and Latron, J.: Contributions of throughfall, forest and soil characteristics to near-surface soil water-content variability at the plot scale in a mountainous Mediterranean area, Sci. Total Environ., 647, 1421–1432, https://doi.org/10.1016/j.scitotenv.2018.08.020, 2019.
Nadezhdina, N., Cermak, J., Meiresonne, L., and Ceulemans, R.: Transpiration of Scots pine in Flanders growing on soil with irregular substratum, Forest Ecol. Manage., 9, 1–9, https://doi.org/10.1016/j.foreco.2007.01.089, 2007.
Neumann, R. B. and Cardon, Z. G.: The magnitude of hydraulic redistribution by plant roots: a review and synthesis of empirical and modeling studies, New Phytol., 194, 337–352, https://doi.org/10.1111/j.1469-8137.2012.04088.x, 2012.
Nie, C., Huang, Y., Zhang, S., Yang, Y., Zhou, S., Lin, C., and Wang, G.: Effects of soil water content on forest ecosystem water use efficiency through changes in transpiration/evapotranspiration ratio, Agr. Forest Meteorol., 308–309, 108605, https://doi.org/10.1016/j.agrformet.2021.108605, 2021.
Obladen, N., Dechering, P., Skiadaresis, G., Tegel, W., Keßler, J., Höllerl, S., Kaps, S., Hertel, M., Dulamsuren, C., Seifert, T., Hirsch, M., and Seim, A.: Tree mortality of European beech and Norway spruce induced by 2018–2019 hot droughts in central Germany, Agr. Forest Meteorol., 307, 108482, https://doi.org/10.1016/j.agrformet.2021.108482, 2021.
Otto, J., Berveiller, D., Bréon, F.-M., Delpierre, N., Geppert, G., Granier, A., Jans, W., Knohl, A., Kuusk, A., Longdoz, B., Moors, E., Mund, M., Pinty, B., Schelhaas, M.-J., and Luyssaert, S.: Forest summer albedo is sensitive to species and thinning: how should we account for this in Earth system models?, Biogeosciences, 11, 2411–2427, https://doi.org/10.5194/bg-11-2411-2014, 2014.
Pearson, R. K.: Data cleaning for dynamic modeling and control, in: 1999 European Control Conference (ECC), 31 August–3 September 1999, Karlsruhe, Germany, 2584–2589, ISBN 978-3-9524173-5-5, https://doi.org/10.23919/ECC.1999.7099714, 1999.
Pretzsch, H., Schütze, G., and Uhl, E.: Resistance of European tree species to drought stress in mixed versus pure forests: evidence of stress release by inter-specific facilitation, Plant Biol., 15, 483–495, https://doi.org/10.1111/j.1438-8677.2012.00670.x, 2013.
Priyadarshini, K. V. R., Prins, H. H. T., de Bie, S., Heitkönig, I. M. A., Woodborne, S., Gort, G., Kirkman, K., Ludwig, F., Dawson, T. E., and de Kroon, H.: Seasonality of hydraulic redistribution by trees to grasses and changes in their water-source use that change tree-grass interactions: Hydraulic Redistribution By Trees To Grasses And Changes In Their Water Sources, Ecohydrology, 9, 218–228, https://doi.org/10.1002/eco.1624, 2016.
Pypker, T. G., Levia, D. F., Staelens, J., and Van Stan, J. T.: Canopy Structure in Relation to Hydrological and Biogeochemical Fluxes, in: Forest Hydrology and Biogeochemistry: Synthesis of Past Research and Future Directions, edited by: Levia, D. F., Carlyle-Moses, D., and Tanaka, T., Springer Netherlands, Dordrecht, 371–388, https://doi.org/10.1007/978-94-007-1363-5_18, 2011.
Raat, K. J., Draaijers, G. P. J., Schaap, M. G., Tietema, A., and Verstraten, J. M.: Spatial variability of throughfall water and chemistry and forest floor water content in a Douglas fir forest stand, Hydrol. Earth Syst. Sci., 6, 363–374, https://doi.org/10.5194/hess-6-363-2002, 2002.
R Core Team: R: The R Project for Statistical Computing, R Foundation for Statistical Computing, Vienna, Austria, https://www.R-project.org/ (last access: 8 March 2024), 2021.
Rodrigues, A. F., Terra, M. C. N. S., Mantovani, V. A., Cordeiro, N. G., Ribeiro, J. P. C., Guo, L., Nehren, U., Mello, J. M., and Mello, C. R.: Throughfall spatial variability in a neotropical forest: Have we correctly accounted for time stability?, J. Hydrol., 608, 127632, https://doi.org/10.1016/j.jhydrol.2022.127632, 2022.
Rodríguez-Robles, U., Arredondo, J. T., Huber-Sannwald, E., Yépez, E. A., and Ramos-Leal, J. A.: Coupled plant traits adapted to wetting/drying cycles of substrates co-define niche multidimensionality, Plant Cell Environ., 43, 2394–2408, https://doi.org/10.1111/pce.13837, 2020.
Rosenbaum, U., Bogena, H. R., Herbst, M., Huisman, J. A., Peterson, T. J., Weuthen, A., Western, A. W., and Vereecken, H.: Seasonal and event dynamics of spatial soil moisture patterns at the small catchment scale: Dynamics Of Catchment-Scale Soil Moisture Patterns, Water Resour. Res., 48, W10544, https://doi.org/10.1029/2011WR011518, 2012.
Rothfuss, Y. and Javaux, M.: Reviews and syntheses: Isotopic approaches to quantify root water uptake: a review and comparison of methods, Biogeosciences, 14, 2199–2224, https://doi.org/10.5194/bg-14-2199-2017, 2017.
Sadeghi, S. M. M., Gordon, D. A., and Van Stan II, J. T.: A Global Synthesis of Throughfall and Stemflow Hydrometeorology, in: Precipitation Partitioning by Vegetation: A Global Synthesis, edited by: Van Stan, I., John, T., Gutmann, E., and Friesen, J., Springer International Publishing, Cham, 49–70, https://doi.org/10.1007/978-3-030-29702-2_4, 2020.
Schume, H., Jost, G., and Hager, H.: Soil water depletion and recharge patterns in mixed and pure forest stands of European beech and Norway spruce, J. Hydrol., 289, 258–274, https://doi.org/10.1016/j.jhydrol.2003.11.036, 2004.
Schwärzel, K., Menzer, A., Clausnitzer, F., Spank, U., Häntzschel, J., Grünwald, T., Köstner, B., Bernhofer, C., and Feger, K.-H.: Soil water content measurements deliver reliable estimates of water fluxes: A comparative study in a beech and a spruce stand in the Tharandt forest (Saxony, Germany), Agr. Forest Meteorol., 149, 1994–2006, https://doi.org/10.1016/j.agrformet.2009.07.006, 2009.
Seeger, S. and Weiler, M.: Temporal dynamics of tree xylem water isotopes: in situ monitoring and modeling, Biogeosciences, 18, 4603–4627, https://doi.org/10.5194/bg-18-4603-2021, 2021.
Shachnovich, Y., Berliner, P. R., and Bar, P.: Rainfall interception and spatial distribution of throughfall in a pine forest planted in an arid zone, J. Hydrol., 349, 168–177, https://doi.org/10.1016/j.jhydrol.2007.10.051, 2008.
Shani, U. and Dudley, L. M.: Modeling water uptake by roots under waterand salt stress: Soil-based and crop response root sink terms, in: PlantRoots: The Hidden Half, 2nd Edn., edited by: Waisel, Y., Eshel, A., and Kafkafi, U., Marcel Dekker, New York, 635–641, ISBN 0-8247-9685-3, 1996.
Silvertown, J., Araya, Y., and Gowing, D.: Hydrological niches in terrestrial plant communities: a review, J. Ecol., 103, 93–108, https://doi.org/10.1111/1365-2745.12332, 2015.
Spanner, G. C., Gimenez, B. O., Wright, C. L., Menezes, V. S., Newman, B. D., Collins, A. D., Jardine, K. J., Negrón-Juárez, R. I., Lima, A. J. N., Rodrigues, J. R., Chambers, J. Q., Higuchi, N., and Warren, J. M.: Dry Season Transpiration and Soil Water Dynamics in the Central Amazon, Front. Plant Sci., 13, 825097, https://doi.org/10.3389/fpls.2022.825097, 2022.
Sprenger, M., Llorens, P., Cayuela, C., Gallart, F., and Latron, J.: Mechanisms of consistently disjunct soil water pools over (pore) space and time, Hydrol. Earth Syst. Sci., 23, 2751–2762, https://doi.org/10.5194/hess-23-2751-2019, 2019.
Staelens, J., De Schrijver, A., Verheyen, K., and Verhoest, N. E. C.: Spatial variability and temporal stability of throughfall water under a dominant beech (Fagus sylvatica L.) tree in relationship to canopy cover, J. Hydrol., 330, 651–662, https://doi.org/10.1016/j.jhydrol.2006.04.032, 2006.
Staelens, J., De Schrijver, A., Verheyen, K., and Verhoest, N. E. C.: Rainfall partitioning into throughfall, stemflow, and interception within a single beech (Fagus sylvatica L.) canopy: influence of foliation, rain event characteristics, and meteorology, Hydrol. Process., 22, 33–45, https://doi.org/10.1002/hyp.6610, 2008.
Teuling, A. J. and Troch, P. A.: Improved understanding of soil moisture variability dynamics, Geophys. Res. Lett., 32, L05404, https://doi.org/10.1029/2004GL021935, 2005.
Thieurmel, B. and Elmarhraoui, A.: suncalc: Compute Sun Position, Sunlight Phases, Moon Position and Lunar Phase, R package version 0.5.1, https://CRAN.R-project.org/package=suncalc (last access: 8 March 2024), 2022.
Tromp-van Meerveld, H. J. and McDonnell, J. J.: On the interrelations between topography, soil depth, soil moisture, transpiration rates and species distribution at the hillslope scale, Adv. Water Resour., 29, 293–310, https://doi.org/10.1016/j.advwatres.2005.02.016, 2006.
Tsuruta, K., Kwon, H., Law, B. E., and Kume, T.: Relationship between stem diameter and whole-tree transpiration across young, mature and old-growth ponderosa pine forests under wet and dry soil conditions, Ecohydrology, 16, e2572, https://doi.org/10.1002/eco.2572, 2023.
Vachaud, G., Passerat De Silans, A., Balabanis, P., and Vauclin, M.: Temporal Stability of Spatially Measured Soil Water Probability Density Function, Soil Sci. Soc. Am. J., 49, 822–828, https://doi.org/10.2136/sssaj1985.03615995004900040006x, 1985.
Van Stan, J. T., Siegert, C. M., Levia, D. F., and Scheick, C. E.: Effects of wind-driven rainfall on stemflow generation between codominant tree species with differing crown characteristics, Agr. Forest Meteorol., 151, 1277–1286, https://doi.org/10.1016/j.agrformet.2011.05.008, 2011.
Van Stan, J. T., Hildebrandt, A., Friesen, J., Metzger, J. C., and Yankine, S. A.: Spatial Variability and Temporal Stability of Local Net Precipitation Patterns, in: Precipitation Partitioning by Vegetation: A Global Synthesis, edited by: Van Stan, I., John, T., Gutmann, E., and Friesen, J., Springer International Publishing, Cham, 89–104, https://doi.org/10.1007/978-3-030-29702-2_6, 2020.
Vereecken, H., Kamai, T., Harter, T., Kasteel, R., Hopmans, J., and Vanderborght, J.: Explaining soil moisture variability as a function of mean soil moisture: A stochastic unsaturated flow perspective, Geophys. Res. Lett., 34, L22402, https://doi.org/10.1029/2007GL031813, 2007.
Vereecken, H., Amelung, W., Bauke, S. L., Bogena, H., Brüggemann, N., Montzka, C., Vanderborght, J., Bechtold, M., Blöschl, G., Carminati, A., Javaux, M., Konings, A. G., Kusche, J., Neuweiler, I., Or, D., Steele-Dunne, S., Verhoef, A., Young, M., and Zhang, Y.: Soil hydrology in the Earth system, Nat. Rev. Earth Environ., 3, 573–587, https://doi.org/10.1038/s43017-022-00324-6, 2022.
Vitali, V., Forrester, D. I., and Bauhus, J.: Know Your Neighbours: Drought Response of Norway Spruce, Silver Fir and Douglas Fir in Mixed Forests Depends on Species Identity and Diversity of Tree Neighbourhoods, Ecosystems, 21, 1215–1229, https://doi.org/10.1007/s10021-017-0214-0, 2018.
Volkmann, T. H. M., Haberer, K., Gessler, A., and Weiler, M.: High-resolution isotope measurements resolve rapid ecohydrological dynamics at the soil–plant interface, New Phytol., 210, 839–849, https://doi.org/10.1111/nph.13868, 2016.
Wambsganss, J., Beyer, F., Freschet, G. T., Scherer-Lorenzen, M., and Bauhus, J.: Tree species mixing reduces biomass but increases length of absorptive fine roots in European forests, J. Ecol., 109, 2678–2691, https://doi.org/10.1111/1365-2745.13675, 2021.
Whelan, M. J. and Anderson, J. M.: Modelling spatial patterns of throughfall and interception loss in a Norway spruce (Picea abies) plantation at the plot scale, J. Hydrol., 186, 335–354, 1996.
Wiekenkamp, I., Huisman, J. A., Bogena, H. R., Lin, H. S., and Vereecken, H.: Spatial and temporal occurrence of preferential flow in a forested headwater catchment, J. Hydrol., 534, 139–149, https://doi.org/10.1016/j.jhydrol.2015.12.050, 2016.
Wullaert, H., Pohlert, T., Boy, J., Valarezo, C., and Wilcke, W.: Spatial throughfall heterogeneity in a montane rain forest in Ecuador: Extent, temporal stability and drivers, J. Hydrol., 377, 71–79, https://doi.org/10.1016/j.jhydrol.2009.08.001, 2009.
Yu, K. and D'Odorico, P.: Hydraulic lift as a determinant of tree–grass coexistence on savannas, New Phytol., 207, 1038–1051, https://doi.org/10.1111/nph.13431, 2015.
Zacharias, S. and Wessolek, G.: Excluding Organic Matter Content from Pedotransfer Predictors of Soil Water Retention, Soil Sci. Soc. Am. J., 71, 43–50, https://doi.org/10.2136/sssaj2006.0098, 2007.
Zarebanadkouki, M., Kim, Y. X., and Carminati, A.: Where do roots take up water? Neutron radiography of water flow into the roots of transpiring plants growing in soil, New Phytol., 199, 1034–1044, https://doi.org/10.1111/nph.12330, 2013.
Zehe, E., Graeff, T., Morgner, M., Bauer, A., and Bronstert, A.: Plot and field scale soil moisture dynamics and subsurface wetness control on runoff generation in a headwater in the Ore Mountains, Hydrol. Earth Syst. Sci., 14, 873–889, https://doi.org/10.5194/hess-14-873-2010, 2010.
Zhang, Y., Wang, X., Hu, R., and Pan, Y.: Throughfall and its spatial variability beneath xerophytic shrub canopies within water-limited arid desert ecosystems, J. Hydrol., 539, 406–416, https://doi.org/10.1016/j.jhydrol.2016.05.051, 2016.
Zhu, X., He, Z., Du, J., Chen, L., Lin, P., and Tian, Q.: Spatial heterogeneity of throughfall and its contributions to the variability in near-surface soil water-content in semiarid mountains of China, Forest Ecol. Manage., 488, 119008, https://doi.org/10.1016/j.foreco.2021.119008, 2021.
Zimmermann, A., Zimmermann, B., and Elsenbeer, H.: Rainfall redistribution in a tropical forest: Spatial and temporal patterns, Water Resour. Res., 45, W11413, https://doi.org/10.1029/2008WR007470, 2009.
Zuur, A. F., Ieno, E. N., Walker, N., Saveliev, A. A., and Smith, G. M.: Mixed effects models and extensions in ecology with R, Springer, New York, NY, https://doi.org/10.1007/978-0-387-87458-6, 2009.