the Creative Commons Attribution 4.0 License.
the Creative Commons Attribution 4.0 License.
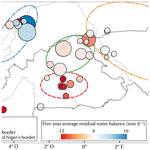
Hydrological regime of Sahelian small waterbodies from combined Sentinel-2 MSI and Sentinel-3 Synthetic Aperture Radar Altimeter data
Mathilde de Fleury
Laurent Kergoat
Manuela Grippa
In the Sahelian semi-arid region, water resources, especially small waterbodies such as ponds, small lakes, and reservoirs in rural areas are of vital importance. However, because of their high number and the scarce in situ monitoring networks, these resources and their spatiotemporal variability are not well known at the regional scale. This study investigates the hydrological regime of 37 small waterbodies, located in Mali, Niger, and Burkina Faso in central Sahel. We propose a method based on remote sensing data only, which consists of combining water height data from Sentinel-3 Synthetic Aperture Radar Altimeter (SRAL) with water area data obtained with the Sentinel-2 MultiSpectral Instrument (MSI) to create a dense water height time series. Water height variations are then compared to the evaporation estimated by the Penman–Monteith method, using ERA5 reanalysis by the European Centre for Medium-Range Weather Forecasts (ECMWF) to infer water regimes during the dry season. Three main regimes stand out, namely a net water loss, mainly resulting from anthropogenic withdrawals, a net water supply occurring after the end of the rainy season through river network or water table exchange, and a balanced behaviour, where water losses during the dry season closely correspond to evaporation rates. Spatial patterns have been identified; in central Burkina Faso, most of the reservoirs show a net dry season water loss, which is explained by frequent irrigation, while reservoirs in northern Burkina Faso, generally show little water loss, indicating that water withdrawal is not significant in this area. Lakes located in the Inner Niger Delta in Mali and connected to the Niger River network generally show an important water supply, particularly at the beginning of the dry season. Lakes in Niger tend to show a weak signal toward water inflow that could be explained by exchange processes with the groundwater. These results show that satellite data are effective at estimating hydrological regimes and the anthropogenic impact on water resources at the large scale, including resources found in small waterbodies.
- Article
(7089 KB) - Full-text XML
-
Supplement
(398 KB) - BibTeX
- EndNote
In the Sahel and more generally in West Africa, small waterbodies are critical resources for the inhabitants, who use them on a daily basis to meet vital needs, such as drinking water, livestock watering, irrigation, fishing, and bathing, among others (Cecchi et al., 2009; Frenken, 2005). These waterbodies are widespread all over the region and include numerous small reservoirs, for which dams have been built, small natural lakes and ponds, and intermediate situations, where existing lakes are more or less developed. Burkina Faso, for example, built many reservoirs, whose number increased from about 200 in 1974 to about 1650 in 2008 (Cecchi et al., 2009). The aim of such actions was to address food security issues (Douxchamps et al., 2014) after severe droughts (Sally et al., 2011). The central Sahelian region also hosts a large number of small temporary waterbodies (Haas et al., 2009; Gardelle et al., 2010; Papa et al., 2023), whose number is still not well known. There is a clear need to better survey the surface water resources in this region. Monitoring and understanding lake hydrological regimes is therefore an important step toward better management of these water resources.
Since ground-based monitoring of waterbodies in this area is usually restricted to some large lakes (mainly those supplying water to capital cities or used for electricity production), remote sensing data such as those provided by the Copernicus Sentinel missions give an interesting tool to derive useful information. Radar altimetry monitors water heights (Birkett, 1994; Morris and Gill, 1994) by calculating the return time of a radar pulse emitted by the sensor on board and reflected by the water surface. The Sentinel-3A and Sentinel-3B satellites launched in 2016 and 2018, respectively, carry a Synthetic Aperture Radar Altimeter (SRAL) on board. Their performance in measuring inland water levels has already been assessed, as in the Inner Niger Delta, resulting in an average root mean square error (RMSE) of 0.67 m (Normandin et al., 2018, Table S5). The technology offered by Sentinel-3 provides a significant step forward, with a much better-resolved footprint than previous altimeters, allowing for the observation of smaller waterbodies (Shu et al., 2020). Time series can be obtained from databases such as DAHITI (Schwatke et al., 2015), Global Reservoirs and Lakes Monitor (G-REALM; Birkett et al., 2010, 2017), and Hydroweb (Crétaux et al., 2011). Laser altimetry data from ICESat-2 have been used to derive water level changes (Cooley et al., 2021). Optical imagery is a powerful tool to detect surface water areas in cloud-free conditions, and recently, several algorithms have been developed to map waterbodies at the global scale (Pekel et al., 2016; Messager et al., 2016; DeVries et al., 2017; Cordeiro et al., 2021). However, the conditions required by these algorithms are not always met in central Sahel. This is due to the variability in the water optical reflectances of these waterbodies in time and space (e.g. Abdourhamane Touré, 2016) that is caused by the common presence of aquatic vegetation (Gardelle et al., 2010), different levels of water turbidity, including extremely turbid and bright lakes (Robert et al., 2017), and by the seasonal variability in the waterbody characteristics. The modified normalized difference water index (MNDWI) is frequently used to differentiate water from soil (Xu, 2006), usually with automatic or supervised thresholding methods. Using this index, Reis et al. (2021) found that optimal thresholds still varied over time and space in the Sahel, whereas Ji et al. (2009) showed that a fairly stable MNDWI threshold over time gives good results, even in the presence of mixed water and vegetation pixels.
Studies combining surface water areas and heights estimated by remote sensing and/or a combination of remote sensing and field measurements have been increasingly published during the last 5 to 10 years. Several works have been focused on large lakes. For example, Pham-Duc et al. (2020) developed a method based on remote sensing data to measure the surface water extent and water volume variations in Lake Chad, the fourth-largest lake in Africa. Sun et al. (2021) used remote and gauged data to estimate the water balance and water fluxes of Lake Poyang, China, over 20 years. Fewer studies focused on smaller waterbodies in Europe and America (Baup et al., 2014; Schwatke et al., 2020; Gourgouletis et al., 2022). In central Sahel, Gal et al. (2016) estimated the lake water inflow of Agoufou lake based on remote sensing data and evaporation modelling and validated the method with in situ measurements. Also in central Sahel, Fowe et al. (2015) studied the water balance of a small reservoir in southern Burkina Faso, highlighting the variations caused by anthropogenic water withdrawal. Other studies assessed lake topography through bathymetry (Arsen et al., 2013) or a digital elevation model (DEM; Avisse et al., 2017) to retrieve lake storage. The variability in the reservoirs at the global scale has been addressed by some recent works. For example, Cooley et al. (2021) showed the great seasonal variability in the reservoirs worldwide, drawing attention to the anthropogenic impacts on water resources, and Hou et al. (2022) highlighted the important role of precipitation in the observed variabilities. However, these global studies do not include a precise quantification of water fluxes over small waterbodies in the Sahel, and several questions remain unanswered. What is the hydrological regime of these small waterbodies? What are the dominant water exchanges in this region? How can their contribution be quantified? Is there a major anthropogenic impact on these water resources? This work develops a methodology based on remote sensing data to quantify the hydrological regime of small waterbodies in central Sahel and derives information about their seasonal and interannual variability. It allows us to better understand the major processes at play in this region and identify the human impact on these water resources.
2.1 Study site and lake selection
The study area is located in central Sahel and includes waterbodies in Mali, Burkina Faso, and Niger. It covers arid, semi-arid (Sahelian), and sub-humid (Soudanian) areas, according to the classification in Andam-Akorful et al. (2017), with well-defined rainy and dry seasons enforced by a tropical monsoon system. The rainy season starts in June and ends in October, with variations due to latitude (Frappart et al., 2009; Panthou et al., 2018). The north's rainy season is shorter, and annual rainfall ranges from around 200 to 900 mm yr−1 from the north to south area. Four regions of interest can be defined based on different geomorphology and development policies, namely the Inner Niger Delta, the centre of Burkina Faso around the capital Ouagadougou, which is densely populated and has a large number of reservoirs, the western area of Niger, and Burkina Faso's northern borders with Mali and Niger. Numerous waterbodies are located in the study area, but the Sentinel-3 satellite orbits constrain the selection of waterbodies. The intertrack distance of 104 km for one satellite and 52 km for a combination of the two (Fig. 1), with a footprint of 300 m below the track, reduces the observable surface. Due to a potential track shifting of ±1 km at maximum (Crétaux et al., 2018), the lakes located between 0 and 0.3 km from the nominated altimeter track have been included in the potential lakes to be studied. Using the maximum water extent of the Global Surface Water dataset (Pekel et al., 2016), 150 lakes were detected below the tracks. Among them, 42 had suitable altimeter data to provide a long and consistent time series for analysis. This amounts to 26.2 % of the lakes initially detected (Fig. 1 and Table 1), and 21 are located in Burkina Faso, including 19 reservoirs, 12 in Mali, and 9 in Niger, including 1 reservoir.
Table 1Information and key results on the study lakes. Lakes highlighted in bold are also shown in Sect. 4. Country abbreviations are BF for Burkina Faso, M for Mali, and N for Niger. The “nan” values indicate that conditions for the 5-year average residual water balance calculation have not been met.
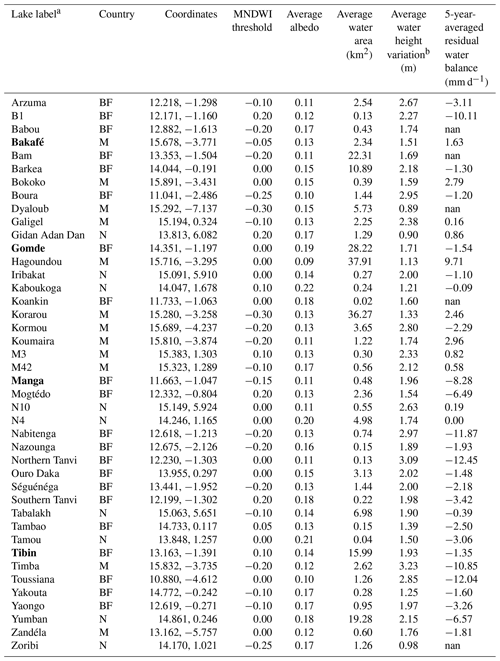
2.2 Data
Water areas and water albedos are derived from the freely available Sentinel-2 (S2) MultiSpectral instrument (MSI) images using the top-of-atmosphere reflectance products from the dataset Sentinel-2 MSI: MultiSpectral Instrument, Level-1C, provided by Google Earth Engine (Gorelick et al., 2017). Measurements are made in 13 optical bands from VNIR (visible near infrared) to SWIR (shortwave infrared) at a resolution of 10, 20, or 60 m, depending on the band. In this study, we used the blue band (B2) at 0.490 µm, the green band (B3) at 0.560 µm, the red band (B4) at 0.665 µm, all at 10 m resolution, and two SWIR bands (B11 and B12) at 1.610 and 2.190 µm with a 20 m resolution. Images are acquired from 2015 to the present, with a revisit frequency of 10 d before the launch of Sentinel-2B in 2017 and 5 d afterwards, which allows good temporal monitoring, except when it is cloudy. Water heights are obtained from Sentinel-3 SRAL (Ku band at 20 Hz) data (S3), provided by the Centre de Topographie des Océans et de l'Hydrosphère or Centre of Topography of the Oceans and the Hydrosphere (CTOH; Frappart et al., 2021) and referenced with an EGM2008 geoid model. The temporal frequency of measurements is 27 d, with a spatial resolution of 300 m (along-track) × 1.64 km (across-track) from 2016 to the present. Precipitation is estimated by the Integrated Multi-satEllite Retrievals algorithm of the international satellite mission Global Precipitation Measurement (IMERG-GPM; Huffman et al., 2019). The data are provided by Google Earth Engine, through the Global Precipitation Measurement (GPM) v6 collection, with a spatial resolution of and a temporal resolution of 30 min. Other meteorological data are provided by the database of ERA5 reanalysis hourly data on single levels from 1959 to the present (Hersbach et al., 2018), which has been produced by the European Centre for Medium-Range Weather Forecasts (ECMWF) within the Copernicus Climate Change Service (C3S). The data are provided at the resolution of . Evaporation rate data, provided by the global lake evaporation volume (GLEV) dataset (Zhao et al., 2022) and Colorado pan evaporation data over a small reservoir (Boura) from Fowe et al. (2015), are used for validation over the April 2012–April 2014 period.
3.1 Lake water balance estimation
The water balance approach defines the different fluxes controlling a waterbody regime (Winter, 1995). In this paper, we adapt the equation developed by Fowe et al. (2015), who propose a water balance equation expressed as the variations in the water volumes applied to a small reservoir in Burkina Faso (Boura). All terms can be also expressed in water height, which fits our data better. The water height variation between two dates t0 and t1 can be written as the sum of precipitation and evaporation over the same period minus a residual term and is referred to as the residual water balance (R). This term is the net result of different hydrological fluxes, including water inflow from the watershed into the lake, groundwater inflow, water losses due to overflow, infiltration losses, and withdrawals due to anthropogenic uses, and can be expressed in millimetres per day.
where Pi (mm) and Ei (mm), respectively, indicate the daily precipitation over the lake and the daily evaporation from the lake. During the dry season, precipitation (Pi) is null. Evaporation (Ei) is estimated using the available meteorological data. Water height variation () is estimated using altimetric data. As the altimeter offers data with a temporal resolution of 27 d, the time series are completed by other water height estimations derived from water areas through an area–height relationship, called the A-H curve.
3.2 Lake water height estimation
Water height time series are extracted through the Altimetric Time Series Software (AlTiS, version 2.0; Frappart et al., 2021), which is an open-source software developed by CTOH. The process of extracting the time series is partially manual and has to be done for each lake. Among the Geophysical Data Record (GDR) variables proposed, the backscatter coefficient, having a very high value for surface water, is appropriate to distinguish water from soil (Taburet et al., 2020). The backscatter coefficient is extracted for data within the lake polygon. Samples that do not correspond to water are removed by thresholding. Following an empirical analysis, a threshold of 40 dB is retained. This is in line with Kittel et al. (2021) and Taburet et al. (2020), who propose thresholds of 30 and 45 dB, respectively. Kittel et al. (2021) also state that changes in data processed from 2020 onwards result in a drop in the backscatter coefficient of 18 dB; the threshold has therefore been set to 22 dB for acquisition dates after 2020. To reduce the influence of the remaining outliers on the resulting time series, the median values are extracted (Fig. 2), as suggested by Frappart et al. (2021), and a threshold of 0.25 m is applied on the associated median absolute deviations (MADs). Water-like echoes created by wet sand that may appear when the waterbody is empty may also lead to outliers. To best prevent the use of such erroneous height values, data corresponding to periods when the water area is zero are removed.
3.3 Surface water area estimation
Sentinel-2 water optical reflectance (ρ) is firstly preprocessed to mask clouds using the Sentinel-2 QA (quality) band at 60 m (QA60) and an additional blue band threshold so that only values with ρB2<0.2 are retained. Water detection (Fig. 2) is performed by applying a thresholding on the modified normalized difference water index (MNDWI; Xu, 2006):
The MNDWI threshold (Table 1) is chosen ad hoc for each lake and kept constant over the study period. This method ensures that water pixels are not detected when waterbodies dry up. Highly negative thresholds are mostly used for lakes with vegetation.
3.4 Area–height curve estimation and water height time series densification
To estimate the A-H curve (Fig. 3), water heights and water areas are combined. We select quasi-simultaneous data in a ±3 d interval, which follows the work of Gao et al. (2012) with MODIS and altimetry data. Based on Crétaux et al. (2016), a 2∘ polynomial curve is fitted to the data. Data outside the 95 % prediction interval (area within the dotted lines in Fig. 3) are considered outliers and are removed to obtain the final A-H curve, in line with Busker et al. (2019). The root mean squared error (RMSE) and the R2 values are calculated to evaluate the accuracy of the regression.
Water heights are then estimated from water areas via the A-H curve, within the limits of the polynomial regression, i.e. without extrapolating the A-H curve. A filter is further applied to remove data with inconsistent variation (significant and rapid variations in the dry season, for example) caused mainly by the occasional mismatch between areas and heights. We have carried out validations of our A-H curves with in situ data (see the Supplement) over two lakes (Séguénéga and Bam; Table 1). We obtained RMSEs of 0.073 and 0.015 m and biases of −0.070 and 0.006. The final water height time series (Fig. 4) is composed of water heights derived from Sentinel-3 and water heights estimated from Sentinel-2 through the A-H curve. All altimetry-derived water heights are considered, even those not used to build the A-H curve.
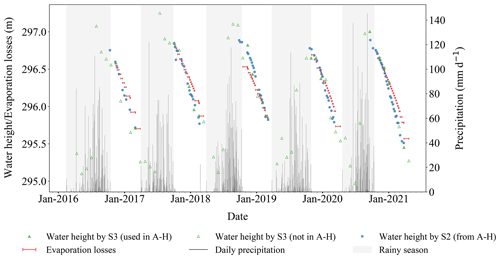
Figure 4Water height time series from Sentinel-3 (S3) and Sentinel-2 (S2) data, along with the cumulated evaporation losses, daily rainfall and rainy season for Lake Tibin. The starting point to calculate evaporation losses is fixed to the water height at the first date in the dry season. The length of the red lines in the x axis corresponds to the time between two successive water height samples.
3.5 Evaporation estimation
Gal et al. (2016) estimated the evaporation of a shallow lake (Agoufou) in Mali with the Penman equation (Penman and Keen, 1948). As the context of this study is similar in terms of the climate, environment, and type of lakes, the same approach is used to estimate evaporation using the Penman–Monteith equations and the methods by McMahon et al. (2013, Sect. S11). It requires the following meteorological data, which we extracted from the ERA5 archive: downward surface solar radiation (J m−2), downward surface thermal radiation (J m−2), daily air temperature (K), daily dew point temperature (K), 10 m u component and 10 m v component of wind (m s−1), and altitude of wind speed measurement (m). It also requires the altitude (m) derived from the Shuttle Radar Topography Mission (SRTM) DEM and the average albedo of the lake surface (α), which is derived following Naegeli et al. (2017),
from the Sentinel-2 water reflectance (ρ). To calculate evaporation over the dry season period only, we estimate the start and end dates from the rainfall data. The end of the dry season is taken as the date of the first day with rainfall exceeding 5 mm and followed by at least another rainfall exceeding 5 mm in the following 30 d. The start of the dry season is taken as the first day with rainfall below 5 mm and followed by 60 consecutive dry days.
Over the whole study period, including the dry and the rainy season, the average water areas of the 42 lakes vary from 0.02 km2 (Koankin) to 37.91 km2 (Hagoundou) and average to 5.28 km2. We have found that 69 % of the lakes turned out to be temporary lakes. Height seasonal variations vary from 4.86 m for the Boura reservoir in Burkina Faso in 2018 to 0.28 m for Lake Hagoundou in Mali in 2017 and average to 1.94 m. Evaporation losses in the dry season show some spatial variability which follows a latitude gradient, with higher values in the north (with 7.04 mm d−1) than in the south (with 4.12 mm d−1) and average to 5.66 mm d−1 over the study period. The average albedo observed is 0.14, with a minimum value of 0.09 and a maximum value of 0.22.
4.1 The 5-year-averaged residuals water balance during the dry season
Of the 42 lakes studied, 37 have complete time series between 2016 and 2021 for which a 5-year-averaged residual water balance (Eq. 1) is estimated (Fig. 5 and Table 1). The 5-year-averaged residual water balance shows contrasting situations, with values ranging from gains of 9.71 mm d−1 to losses of −12.45 mm d−1. In total, 24 lakes, of which 75.0 % are located in Burkina Faso, have a residual water balance below −1 mm d−1. The central Burkina Faso (red zone in Fig. 5) contains only lakes with a negative residual water balance, of which 88.9 % have a highly negative residual water balance below −3 mm d−1. In northern Burkina Faso and near the western Niger border (green zone in Fig. 5), 87.5 % of the lakes have a weak negative residual water balance, such as the Tibin reservoir illustrated previously (Fig. 4). Five lakes, all located in the Inner Niger Delta (blue zone in Fig. 5), have a positive residual water balance greater than 1 mm d−1. Finally, eight lakes display a residual water balance close to zero. They are located near the Niger River in the Tillabéri region of Niger, in southern Mali on the border with Niger, and in the eastern part of the study area (orange zone in Fig. 5).
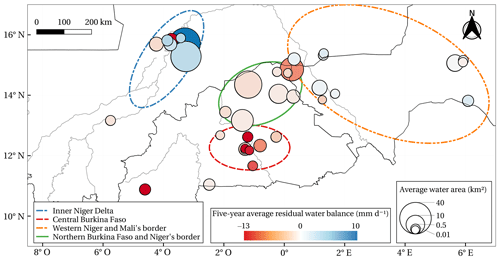
Figure 5The 5-year-averaged residual water balance of each lake studied in central Sahel from 2016 to 2021. The circles representing the studied lakes have an area proportional to the lake average water area. Lakes with water supply and water loss behaviours appear in blue and red, respectively.
An example of water losses is given by a reservoir located in southern Burkina Faso (Manga in Table 1), close to the large lake of Bagré. The 2017–2018 dry season (Fig. 6) starts in late October 2017, when the lake water height is at 264.19 m, and ends in February, when the water height is 262.60 m (i.e. a variation of 1.58 m). The evaporation losses are about twice as small as the height decrease, meaning that a significant part of the water losses is not due to evaporation. The residual water balance for 2017–2018 is −8.54 mm d−1 and averages −8.28 mm d−1 over 5 years. False colour images during the dry season show that the lake is surrounded by irrigated fields, which occupy an area similar to the lake area. This suggests that most water losses are due to irrigation in this case (Fig. 7a and b).
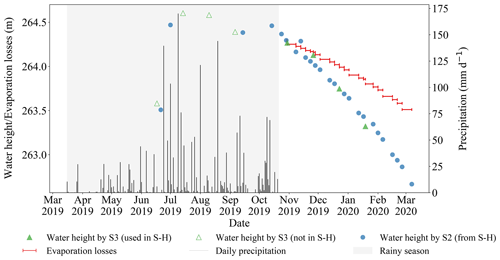
Figure 6Water height time series (in blue and green) for a lake with water loss behaviour (Manga), along with the cumulated evaporation losses (in red), daily rainfall (in black), and rainy season (in grey).
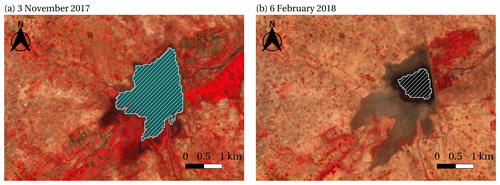
Figure 7Sentinel-2 false colour images (NIR, red, and green) of the Manga reservoir surroundings, with the lake contours (in white) obtained by thresholding of the MNDWI on (a) 3 November 2017 and (b) 6 February 2018. Active vegetation appears in red.
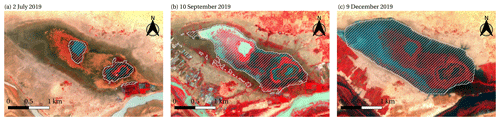
Figure 8Sentinel-2 false colour images (NIR, red, and green) of lake Bakafé surroundings, with the lake contours (in white) obtained by thresholding on the MNDWI at (a) 2 July 2019, (b) 10 September 2019, and (c) 9 December 2019.
The water supply behaviour (positive residual water balance) is illustrated by a lake located in the Inner Niger Delta, where the Niger river splits into multiple reaches (lake Bakafé in Table 1; Fig. 8a–c). The 2019–2020 dry season illustrates this case well (Fig. 9). Between October and May, water heights change from 262.14 to 260.92 m, resulting in a 1.22 m decrease. However, the maximum height is reached about 2 months after the start of the dry season, indicating that precipitation is not the main cause of lake filling. Visual analysis of the Sentinel-2 images shows a connection between the lake and the river network, which is flooded from late October onwards (as in Fig. 8c). Once the peak of the water height is reached, the lake empties approximately at the same rate as the estimated evaporation losses, since the two curves are parallel. The residual water balance for 2019–2020 is 2.03 mm d−1 and averages 1.63 mm d−1 over the 5 years, which means that there is a regular dry season water inflow.
4.2 Interannual variability
The residual water balance can vary from year to year (Fig. 10), as a result of variability in anthropogenic management of resources, rainfall, length of the dry season, changes in inflow or outflow, etc. Overall, the lakes do not show any trend in the residual water balance throughout the study period. About half of the reservoirs in central Burkina Faso show greater losses in 2020–2021 than the other years. The standard deviation (SD) over 5 years has a minimum value of 0.27 mm d−1 (Lake N4) and a maximum value of 5.00 mm d−1 (Lake Yumban) and is equal to 1.86 mm d−1 on average over all lakes. In total, 29 waterbodies have a SD greater than 1 mm d−1, and six lakes show a regime change switching between positive and negative values. The evaporation rate is quite constant over the 5 years for all lakes, and its maximum standard deviation is equal to 0.42 mm d−1. The interannual water balance variability is sometimes caused by changes in dam functioning, like for the Gomde, a reservoir located in northern Burkina Faso. This reservoir was built to supply the water needed by a gold mine, which is located to the southeast of the reservoir (Fig. 11), similar to the Tibin reservoir, created in 2012 for the Bissa Gold Mine (Newall, 2012; Ba, 2012). The standard deviation of the residual water balance over the 5 years is equal to 2.10 mm d−1, but the first 2 years show a residual water balance close to 0, while the last 3 years show important losses, with an average of −3.48 mm d−1; this is a drastic change which is seen also in the water height time series (Fig. 12). The maximum water height variation is obtained in 2020–2021 with 2.49 m, and the minimum is in 2016–2017 with 0.84 m.
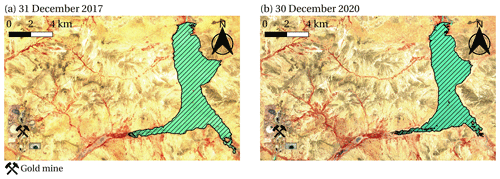
Figure 11Sentinel-2 false colour images (NIR, red, and green) of the Gomde reservoir surroundings, with the lake contours obtained by thresholding of the MNDWI on (a) 31 December 2017 and (b) 30 December 2020.
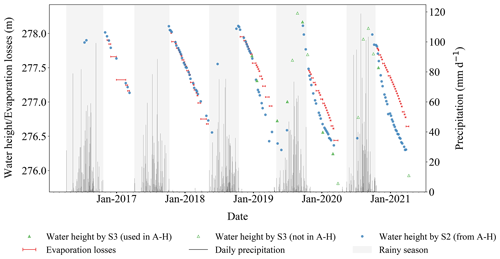
Figure 12Water height time series from Sentinel-3 (S3) and Sentinel-2 (S2) data, along with the cumulative evaporation losses, daily rainfall, and rainy season for Gomde lake. The starting point for calculating evaporation losses is fixed to the water height at the first date in the dry season. The length of the red lines in the x axis corresponds to the time between two successive water height samples.
The combination of altimetry, the time series of optical images, and evaporation modelling reveals a large variety of situations and different hydrological regimes. The studied lakes are located in diverse environments. For instance, they can be surrounded by bare or vegetated areas (rainfed crops, irrigated crops, and natural vegetation) and by soils with different hydraulic characteristics (sandy, loamy, and rocky soils). Lakes also differ in terms of their characteristics, such as whether they are open water or harbouring dense aquatic vegetation, if there are trees growing in the flooded areas, or if the water is clear or extremely turbid, which is associated with very high water albedo. This complexity results in uncertainties in water detection by MNDWI thresholding calculation (Eq. 2), which impact the water regime calculation (Eq. 1). First, aquatic vegetation leads to radiometric variability in the water pixels, which makes it difficult to use automatic algorithms for water detection. Moreover, lakes drying up may also affect classification. These water detection difficulties were recently pointed out by Reis et al. (2021), and in the same vein, Ogilvie et al. (2018) showed that, in central Tunisia, the Global Surface Water dataset (Pekel et al., 2016) had an omission error rate of 41 % on shallow lakes, mostly due to pixels with vegetation or algae. Moreover, the albedo values (Table 1) highlight the diversity of water colour, with values ranging from 0.09 for dark clear surface water to 0.22 for bright turbid surface water, which is higher than the albedo values generally found in lake studies. McMahon et al. (2013), for instance, suggest a default value of albedo of 0.08 to compute the evaporation of open water.
This variability challenges the determination of an optimum MNDWI threshold. In this work, the threshold is chosen ad hoc for each lake (Sect. 3.3), as recommended by Reis et al. (2021), and a constant threshold throughout the study period proved to be efficient for our study. However, the method developed here is not very sensitive to systematic errors in waterbody surface area detection. Given that water areas are only used to estimate water heights via the A-H curve, systematic errors in water area detection will not affect the final height estimation. For example, systematically missing a part of the lake in the water area detection (truncation) will modify the absolute water area values in the A-H curve but will not change the water height values. This situation is encountered for some lakes without a well identified connection to a river or with another lake or lakes that overflow, for example, downstream of a dam. Despite this, water area classification remains a source of error in the calculation of the residual water balance, occasionally creating outliers in the water area time series. A close inspection of these cases points to the misclassification of aquatic vegetation for some images. As a result, 19 lakes under the altimeter tracks could not be included in the study because non-systematic detection problems in water areas made the time series too noisy.
In addition, several other lakes were discarded because of noisy or inaccurate water height time series. This is caused by the radar altimeter resolution along-track of 300 m that only allows the detection of lakes larger than or similar to its resolution. Sometimes, we do not have enough data to construct the A-H curve, even for lakes below the track. For some cases, this is due to the fact that certain lakes are dry for the most part of the time span. For other few cases, the retracking algorithm, which was designed for ice surfaces (Crétaux et al., 2018), does not provide consistent water heights. Moreover, other water-like sources in the altimeter footprint sometimes contaminate the signal (Jiang et al., 2020), which is possible due to the across-track resolution of 1.64 km and shifting of the track up to 1 km. The different filtering processes allow for the elimination of remaining outliers. All the different filtering processes allowed us to reduce the errors in the final water height time series. The RMSE value obtained by comparing to in situ data are considerably lower than the value of 0.67 m reported by Normandin et al. (2018, Table S5).
The seasonal amplitude, averaged over the period 2015–2021 for all lakes, ranges between 0.89 and 3.23 m, with a median value of 2.06 m. This is higher than the values reported by Cooley et al. (2021), who estimated water height amplitudes over 127 lakes in the Niger basin to range between 1.49 and 1.71 m (median 1.60 m), using 2 years of ICESat-2 data. The temporal resolution of the ICESat-2 time series of about 91 d may miss the maximum and minimum heights of most lakes, even if the larger lakes may cross several ICESat-2 tracks, pointing to the importance of having a finer temporal resolution. In addition, our study samples a larger variety of hydrological behaviours and analyses lakes that are not found in the global database employed by Cooley et al. (2021) and other global studies. This differences in the medians could also lie in the margin of error for both satellites.
Evaporation is an important term for the lake water balance. We have compared the results obtained with the Penman–Monteith method with the evaporation derived from pan observations available for the Boura reservoir and with the GLEV method. Multi-annual averages, from 2012 to 2014, were equal to 5.33 mm d−1 by our estimation, 5.40 mm d−1 by derived evaporation (Fowe et al., 2015), and 5.38 mm d−1 by GLEV (Zhao et al., 2022). Evaporation differences by these three methods are lower than an evaporation uncertainty of ±1 mm d−1 considered by Gal et al. (2016) for the Penman method.
Finally, the calculation of the dry season annual residual water balance is impacted by the first and last data at the beginning and end of the dry season. A correct estimation of these values is therefore important, otherwise some fluxes may be overseen, such as early dry season filling by rivers. Overall, we consider that the residual water balance variations above 1 mm d−1 are unlikely to be caused by errors but rather indicate water inflows in the dry season, whereas a residual water balance below −1 mm d−1 would point towards water losses.
The 5-year-averaged residual water balance shows consistent spatial patterns. In the Inner Niger Delta, waterbodies predominantly show a water supply behaviour. Indeed, in this area, lakes are connected to the river network, and they are initially filled by rainfall and then to a greater extent by river waters coming from the Upper Niger watershed, causing dry season flooding of the delta (Olivry, 1995). Sometimes two processes successively dominate a lake water regime, such as water supply at the beginning of the dry season and water loss afterwards. Lakes in the eastern part of the study region (from about 0∘ E) show a weak positive residual water balance. Even if these low values are below the uncertainty that we estimate, limited water supply during the dry season would be in line with the water supplied by groundwater in this region (Favreau et al., 2009). In Burkina Faso, and more importantly in the centre, a water loss signal prevails. These observations are in line with Fowe et al. (2015) and Venot and Krishnan (2011), who show that the variations in the small reservoirs in this region are due to water withdrawn for small-scale irrigation, which is usually detected by the growth of surrounding crops during the dry season. Exchanges with groundwater (Sophocleous, 2002) could also lead to losses due to infiltration through the lake bottom. This is more likely to occur at the beginning of the dry season, when lakes area have expanded and banks are flooded, whereas later on water losses are less significant because lake bottoms are usually silted. Further north in Burkina Faso, near the border with Mali and Niger, waterbodies show little or no residual water balance loss, which is consistent with limited anthropogenic actions over these reservoirs. The fact that several reservoirs in this area show residual water balance close to zero moderates the conclusions of Cooley et al. (2021) on the substantial influence exerted by humans on surface water storage variability. Our results show very different regimes within the same catchment, so it is complicated to apply variations at a catchment scale to lakes within that catchment.
The reservoirs in our study area are sometimes reported to have low performance (Venot and Cecchi, 2011), mainly due to the limited funding and human resources put into reservoir management (Frenken, 2005). Some of these reservoirs were built for gold mining, so the absence of anthropogenic withdrawals may seem inconsistent. However, this area suffers from serious security issues. Since 2015, the number of armed conflicts has been increasing, and these conflicts are now spreading to the whole region. Discussions with colleagues in Burkina Faso (Jean-Marie Dipama, personal communication, 2021) and headlines in the local press lead to the hypothesis that part of the population living near these reservoirs has moved to avoid conflicts, leaving reservoirs with fewer personnel and limited irrigation projects. Another example of the possible impact of conflicts in this area is the Gomde reservoir, which shows a significant change in the residual water balance after 2018 (close to 0 before 2018 and significantly negative afterwards). In this case, attacks by armed groups interested in the gold mines (Assanvo et al., 2019) are the probable cause of damages to the dikes, leading to dam leakage since 2019.
This study illustrates the potential of recent remote sensing sensors to explore the hydrological behaviour of lakes in semi-arid areas. Although it is not yet possible to identify and quantify all fluxes, the residual water balance approach provides very valuable information on surface water resources at the regional scale. The spatiotemporal resolution of current satellites allows monitoring of small waterbodies. The methodology developed here, based on freely available data and tools, is easily transposable to other regions with similar climate. Currently, the water balance estimation is restricted to lakes below the altimeter tracks, which may be around 1 % or less of the total number of lakes in this region. For example, of the 1650 reservoirs analysed by Cecchi et al. (2009) in Burkina Faso, only 21 are surveyed in this study. Only one among the lakes studied is found in the DAHITI database and none in the Hydroweb and G-REALM databases, and all three are usually employed in global studies. A common approach to address waterbodies for which water level estimations are not available is to assume that, in similar geological situations, their shapes are not very different (Cooley et al., 2021; Hou et al., 2022). For the method developed here, it is, however, essential to derive A-H curves for each lake, since applying a general one for all waterbodies in our study region would result in misleading quantification of water fluxes. With the arrival of the Surface Water and Ocean Topography (SWOT) mission, which was launched at the end of 2022, the number of lakes that can be monitored to assess water height changes will greatly increase (Grippa et al., 2019).
In this study, a method to estimate the hydrological regime of 37 small waterbodies from 0.04 to 37.91 km2 in central Sahel was proposed, based on remote sensing data from 2016 to 2021. The method combines Sentinel-3 and Sentinel-2 for the water height and water area, respectively, with meteorological variables from ERA5 and ancillary data from multiple sensors. A dry season water balance is estimated over 5 years for each lake by comparing evaporation and water height changes which characterize the lake hydrological regime. This method allows for a large-scale study of many ungauged waterbodies, including small ones and lakes with aquatic vegetation cover that are frequently overlooked in large-scale studies. Lakes showing dry season water losses (where water depletion is greater than evaporation) were mainly found in central Burkina Faso. This behaviour also concerns lakes in the north of the country but to a lesser extent. In the Inner Niger Delta, lakes mostly show dry season water supply, caused by water inflow from multiple river networks during flooding of the delta, and filling of the lakes generally at the start of the dry season. Other lakes display a balanced behaviour, where water height closely follows the evaporation rate. The limited water supply observed for lakes in Niger may be caused by exchanges with groundwater, which has been observed in this region. Interannual variations in the lake hydrological regimes have been observed, with some significant changes attributed to changes in the anthropogenic use of water resources.
The Sentinel-2 MultiSpectral Instrument (MSI) data are available from Google Earth Engine (GEE; Gorelick et al., 2017) through the Sentinel-2 MSI: MultiSpectral Instrument, Level-1C collection (https://developers.google.com/earth-engine/datasets/catalog/COPERNICUS_S2, European Union, ESA, and Copernicus, 2023). The Sentinel-3 Synthetic Aperture Radar Altimeter (SRAL) data and the Altimetric Time Series Software (AlTiS; Frappart et al., 2021) are obtained from the Centre de Topographie des Océans et de l'Hydrosphère (CTOH; http://ctoh.legos.obs-mip.fr/, CTOH, 2021). Meteorological data are obtained from the European Centre for Medium-Range Weather Forecasts (ECMWF) of the Copernicus Climate Change Service (C3S) through the ERA5 reanalysis hourly data on single levels from 1959 to present database (Hersbach et al., 2018). The results contain modified Copernicus Sentinel and C3S information (2022). Precipitation is available on GEE through the Global Precipitation Measurement (GPM)v6 collection (Huffman et al., 2019, https://doi.org/10.5067/GPM/IMERG/3B-HH/06). Evaporation rate data are retrieved from the Global Lake Evaporation Volume dataset (GLEV; Zhao et al., 2022; https://doi.org/10.5281/zenodo.4646621; Zhao, 2021) and from Fowe et al. (2015).
The supplement related to this article is available online at: https://doi.org/10.5194/hess-27-2189-2023-supplement.
The conceptualization, formal analysis, investigation, methodology, validation, visualization, and writing of this study was conducted by all authors (MdF, LK, and MG). MdF was also in charge of programming, implementation, and testing of the methods. Funding and resources were acquired by MG and LK. This study was conducted under the supervision of MG and LK. All authors reviewed and edited the paper.
The contact author has declared that none of the authors has any competing interests.
Neither the European Commission nor ECMWF is responsible for any use that may be made of the Copernicus information or data it contains.
Publisher's note: Copernicus Publications remains neutral with regard to jurisdictional claims in published maps and institutional affiliations.
We acknowledge Fréderic Frappart and Fabien Blarel for their involvement in the processing of data through the AlTiS software, Jean-François Crétaux for advice on the area–height curve derivation, and Félix Girard for discussion of our water detection and altimetry methods. We thank Hedwige Nikiema and Jean-Marie Dipama for their information on the region and lakes and Yasmin Fitts for her valuable proofreading of the paper. We also thank the Direction Générale des Ressources en Eau (DGRE), Burkina Faso, Tazen Fowe, and Roland O. Yonaba for making their data available for validation. Finally, we acknowledge the referees of this paper for their detailed and attentive review and their valuable comments and suggestions.
This research has been supported by the Centre National d'Etudes Spatiales (APR SPLASH).
This paper was edited by Pieter van der Zaag and reviewed by two anonymous referees.
Abdourhamane Touré, A., Tidjani, A., Guillon, R., Rajot, J. L., Petit, C., Garba, Z., and Sebag, D.: Teneur en matières en suspension des lacs sahéliens en liaison avec les variations piézométrique et pluviométrique: cas des lacs Bangou Kirey et Bangou Bi, Sud-Ouest Niger, Afrique Science, 12, 384–392, 2016. a
Andam-Akorful, S. A., Ferreira, V. G., Ndehedehe, C. E., and Quaye-Ballard, J. A.: An investigation into the freshwater variability in West Africa during 1979–2010, Int. J. Climatol., 37, 333–349, https://doi.org/10.1002/joc.5006, 2017. a
Arsen, A., Crétaux, J.-F., Berge-Nguyen, M., and Del Rio, R. A.: Remote Sensing-Derived Bathymetry of Lake Poopó, Remote Sens., 6, 407–420, https://doi.org/10.3390/rs6010407, 2013. a
Assanvo, W., Dakono, B., Théroux-Bénoni, L. A., and Maïga I.: Extrémisme violent, criminalité organisée et conflits locaux dans le Liptako-Gourma, Report, Institut d'Etudes de Sécurité, 28 pp., https://issafrica.s3.amazonaws.com/site/uploads/war-26-fr.pdf (last access: 22 September 2022), 2019. a
Avisse, N., Tilmant, A., Müller, M. F., and Zhang, H.: Monitoring small reservoirs’ storage with satellite remote sensing in inaccessible areas, Hydrol. Earth Syst. Sci., 21, 6445–6459, https://doi.org/10.5194/hess-21-6445-2017, 2017. a
Ba, M.: Diagnostic environnemental d'un site minier en construction : cas de la mine d'or Bissa Gold, MS thesis, International Institute for Water and Environmental Engineering, 59 pp., http://documentation.2ie-edu.org/cdi2ie/opac_css/doc_num.php?explnum_id=177 (last access: 22 September 2022), 2012. a
Baup, F., Frappart, F., and Maubant, J.: Combining high-resolution satellite images and altimetry to estimate the volume of small lakes, Hydrol. Earth Syst. Sci., 18, 2007–2020, https://doi.org/10.5194/hess-18-2007-2014, 2014. a
Birkett, C. M.: Radar altimetry: A new concept in monitoring lake level changes, Eos Trans. Am. Geophys. Union, 75, 273–275, https://doi.org/10.1029/94EO00944, 1994. a
Birkett, C. M., Reynolds, C., Beckley, B. D., and Doorn, B.: From Research to Operations: The USDA Global Reservoir and Lake Monitor, in: Coastal Altimetry, Chap. 2, edited by: Vignudelli, S., Kostianoy, A., Cipollini, P., Benveniste, J., Springer, Berlin, Heidelberg, https://doi.org/10.1007/978-3-642-12796-0_2, 2010. a
Birkett, C. M., Ricko, M., Beckley, B. D., Yang, X., and Tetrault, R. L.: G-REALM: A lake/reservoir monitoring tool for drought monitoring and water resources management, in: American Geophysical Union Fall Meeting, 12 December 2017, New-Orleans, Louisiana, USA, https://agu.confex.com/agu/fm17/meetingapp.cgi/Paper/209563 (last access: 24 September 2022), 2017. a
Busker, T., de Roo, A., Gelati, E., Schwatke, C., Adamovic, M., Bisselink, B., Pekel, J.-F., and Cottam, A.: A global lake and reservoir volume analysis using a surface water dataset and satellite altimetry, Hydrol. Earth Syst. Sc., 23, 669–690, https://doi.org/10.5194/hess-23-669-2019, 2019. a
Cecchi, P.: Qualité des eaux et risques sanitaires associés aux lacs et réservoirs du Burkina Faso: opération FasoTour 2014, Mission Report, IRD, 35 pp., https://horizon.documentation.ird.fr/exl-doc/pleins_textes/divers16-01/010065652.pdf (last access: 22 September 2022), 2014. a
Cecchi, P., Meunier-Nikiema, A., Moiroux, N., and Sanou, B.: Towards an Atlas of Lakes and Reservoirs in Burkina Faso, Small reservoirs toolkit, IWMI, Colombo, Sri Lanka, https://horizon.documentation.ird.fr/exl-doc/pleins_textes/divers16-05/010046819.pdf (last access: 22 September 2022), 2009. a, b, c
Cooley, S. W., Ryan, J. C., and Smith, L. C.: Human alteration of global surface water storage variability, Nature, 591, 78–81, https://doi.org/10.1038/s41586-021-03262-3, 2021. a, b, c, d, e, f
Cordeiro, M. C. R., Martinez, J.-M., and Peña-Luque, S.: Automatic water detection from multidimensional hierarchical clustering for Sentinel-2 images and a comparison with Level 2A processors, Remote Sens. Environ., 253, 112209, https://doi.org/10.1016/j.rse.2020.112209, 2021. a
Crétaux, J.-F., Arsen, A., Calmant, S., Kouraev, A., Vuglinski, V., Bergé-Nguyen, M., Gennero, M.-C., Nino, F., Abarca Del Rio, R., Cazenave, A., and Maisongrande, P.: SOLS: A lake database to monitor in the Near Real Time water level and storage variations from remote sensing data, Adv. Space Res., 47, 1497–1507, https://doi.org/10.1016/j.asr.2011.01.004, 2011. a
Crétaux, J.-F., Abarca-del-Río, R., Bergé-Nguyen, M., Arsen, A., Drolon, V., Clos, G., and Maisongrande, P.: Lake Volume Monitoring from Space, Surv. Geophys., 37, 269–305, https://doi.org/10.1007/s10712-016-9362-6, 2016. a
Crétaux, J.-F., Bergé-Nguyen, M., Calmant, S., Jamangulova, N., Satylkanov, R., Lyard, F., Perosanz, F., Verron, J., Samine Montazem, A., Le Guilcher, G., Leroux, D., Barrie, J., Maisongrande, P., and Bonnefond, P.: Absolute Calibration or Validation of the Altimeters on the Sentinel-3A and the Jason-3 over Lake Issykkul (Kyrgyzstan), Remote Sens., 10, 1679, https://doi.org/10.3390/rs10111679, 2018. a, b
CTOH – Center of Topographic studies of the Ocean and Hydrosphere: http://ctoh.legos.obs-mip.fr/ (last access: 19 November 2021), 2021. a, b
DeVries, B., Huang, C., Lang, M. W., Jones, J. W., Huang, W., Creed, I. F., and Carroll, M. L.: Automated Quantification of Surface Water Inundation in Wetlands Using Optical Satellite Imagery, Remote Sens., 9, 807, https://doi.org/10.3390/rs9080807, 2017. a
Douxchamps, S., Ayantunde, A., and Barron, J.: Taking stock of forty years of agricultural water management interventions in smallholder systems of Burkina Faso, Water Resour. Rural Dev., 3, 1–13, https://doi.org/10.1016/j.wrr.2013.12.001, 2014. a
European Union, ESA, and Copernicus: Sentinel-2 MSI: MultiSpectral Instrument, Level-1C, European Union, ESA, and Copernicus [data set], https://developers.google.com/earth-engine/datasets/catalog/COPERNICUS_S2, (last access: 22 September 2022), 2015. a
Favreau, G., Cappelaere, B., Massuel, S., Leblanc, M., Boucher, M., Boulain, N., and Leduc, C.: Land clearing, climate variability, and water resources increase in semiarid southwest Niger: A review, Water Resour. Res., 45, W00A16, https://doi.org/10.1029/2007WR006785, 2009. a
Fowe, T., Karambiri, H., Paturel, J.-E., Poussin, J.-C., and Cecchi, P.: Water balance of small reservoirs in the Volta basin: A case study of Boura reservoir in Burkina Faso, Agr. Water Manage., 152, 99–109, https://doi.org/10.1016/j.agwat.2015.01.006, 2015. a, b, c, d, e, f
Frappart, F., Hiernaux, P., Guichard, F., Mougin, E., Kergoat, L., Arjounin, M., Lavenu, F., Koité, M., Paturel, J.-E., and Lebel, T.: Rainfall regime across the Sahel band in the Gourma region, Mali, J. Hydrol., 375, 128–142, https://doi.org/10.1016/j.jhydrol.2009.03.007, 2009. a
Frappart, F., Blarel, F., Fayad, I., Bergé-Nguyen, M., Crétaux, J.-F., Shu, S., Schregenberger, J., and Baghdadi, N.: Evaluation of the Performances of Radar and Lidar Altimetry Missions for Water Level Retrievals in Mountainous Environment: The Case of the Swiss Lakes, Remote Sens., 13, 2196, https://doi.org/10.3390/rs13112196, 2021. a, b, c, d
Frenken, K.: Irrigation in Africa in figures, AQUASTAT survey – 2005, Food and Agriculture Organization of the United Nations Water Reports, 29, 649 pp., https://www.researchgate.net/profile/Karen-Frenken/publication/235704388_Irrigation_in_Africa_in_figures_AQUASTAT_survey_2005/links/554f6bb708ae956a5d245b31/Irrigation-in-Africa-in-figures-AQUASTAT-survey-2005.pdf (last access: 22 September 2022), 2005. a, b
Gal, L., Grippa, M., Hiernaux, P., Peugeot, C., Mougin, E., and Kergoat, L.: Changes in lakes water volume and runoff over ungauged Sahelian watersheds, J. Hydrol., 540, 1176–1188, https://doi.org/10.1016/j.jhydrol.2016.07.035, 2016. a, b, c
Gao, H., Birkett, C. M., and Lettenmaier, D. P.: Global monitoring of large reservoir storage from satellite remote sensing, Water Resour. Res., 48, W09504, https://doi.org/10.1029/2012WR012063, 2012. a
Gardelle, J., Hiernaux, P., Kergoat, L., and Grippa, M.: Less rain, more water in ponds: a remote sensing study of the dynamics of surface waters from 1950 to present in pastoral Sahel (Gourma region, Mali), Hydrol. Earth Syst. Sci., 14, 309–324, https://doi.org/10.5194/hess-14-309-2010, 2010. a, b
Gorelick, N., Hancher, M., Dixon, M., Ilyushchenko, S., Thau, D., and Moore, R.: Google Earth Engine: Planetary-scale geospatial analysis for everyone, Remote Sens. Environ., 202, 18–27, https://doi.org/10.1016/j.rse.2017.06.031, 2017. a, b
Gourgouletis, N., Bariamis, G., Anagnostou, M. N., and Baltas, E.: Estimating Reservoir Storage Variations by Combining Sentinel-2 and 3 Measurements in the Yliki Reservoir, Greece, Remote Sens., 14, 1860, https://doi.org/10.3390/rs14081860, 2022. a
G-REALM – Global Reservoir And Lakes Monitor: https://ipad.fas.usda.gov/cropexplorer/global_reservoir/Default.aspx (last access: 24 October 2022), 2022. a
Grippa, M., Rouzies, C., Biancamaria, S., Blumstein, D., Cretaux, J.-F., Gal, L., Robert, E., Gosset, M., and Kergoat, L.: Potential of SWOT for Monitoring Water Volumes in Sahelian Ponds and Lakes, IEEE J. Select. Top. Appl., 12, 2541–2549, https://doi.org/10.1109/JSTARS.2019.2901434, 2019. a
Haas, E. M., Bartholomé, E., and Combal, B.: Time series analysis of optical remote sensing data for the mapping of temporary surface water bodies in sub-Saharan western Africa, J. Hydrol., 370, 52–63, https://doi.org/10.1016/j.jhydrol.2009.02.052, 2009. a
Hersbach, H., Bell, B., Berrisford, P., Biavati, G., Horányi, A., Muñoz Sabater, J., Nicolas, J., Peubey, C., Radu, R., Rozum, I., Schepers, D., Simmons, A., Soci, C., Dee, D., and Thépaut, J.-N.: ERA5 hourly data on single levels from 1959 to present, Copernicus Climate Change Service (C3S) Climate Data Store (CDS) [data set], https://doi.org/10.24381/cds.adbb2d47, 2018. a, b
Hou, J., van Dijk, A. I. J. M., Beck, H. E., Renzullo, L. J., and Wada, Y.: Remotely sensed reservoir water storage dynamics (1984–2015) and the influence of climate variability and management at a global scale, Hydrol. Earth Syst. Sci., 26, 3785–3803, https://doi.org/10.5194/hess-26-3785-2022, 2022. a, b
Huffman, G. J., Stocker, E. F., Bolvin, D. T., Nelkin, E. J., and Tan, J.: Global Precipitation Measurement, GPM IMERG Final Precipitation L3 Half Hourly 0.1 degree × 0.1 degree V06, Greenbelt, MD, Goddard Earth Sciences Data and Information Services Center (GES DISC) [data set], https://doi.org/10.5067/GPM/IMERG/3B-HH/06, 2019. a, b
Ji, L., Zhang, L., and Wylie, B.: Analysis of Dynamic Thresholds for the Normalized Difference Water Index, Photogram. Eng. Rem. Sens., 75, 1307–1317, https://doi.org/10.14358/PERS.75.11.1307, 2009. a
Jiang, L., Nielsen, K., Dinardo, S., Andersen, O. B., and Bauer-Gottwein, P.: Evaluation of Sentinel-3 SRAL SAR altimetry over Chinese rivers, Remote Sens. Environ., 237, 111546, https://doi.org/10.1016/j.rse.2019.111546, 2020. a
Kittel, C. M. M., Jiang, L., Tøttrup, C., and Bauer-Gottwein, P.: Sentinel-3 radar altimetry for river monitoring – a catchment-scale evaluation of satellite water surface elevation from Sentinel-3A and Sentinel-3B, Hydrol. Earth Syst. Sci., 25, 333–357, https://doi.org/10.5194/hess-25-333-2021, 2021. a, b
Messager, M. L., Lehner, B., Grill, G., Nedeva, I., and Schmitt, O.: Estimating the volume and age of water stored in global lakes using a geo-statistical approach, Nat. Commun., 7, 13603, https://doi.org/10.1038/ncomms13603, 2016. a
McMahon, T. A., Peel, M. C., Lowe, L., Srikanthan, R., and McVicar, T. R.: Estimating actual, potential, reference crop and pan evaporation using standard meteorological data: a pragmatic synthesis, Hydrol. Earth Syst. Sci., 17, 1331–1363, https://doi.org/10.5194/hess-17-1331-2013, 2013. a, b
Morris, C. S. and Gill, S. K.: Evaluation of the TOPEX/POSEIDON altimeter system over the Great Lakes, J. Geophys. Res.-Oceans, 99, 24527–24539, https://doi.org/10.1029/94JC01642, 1994. a
Naegeli, K., Damm, A., Huss, M., Wulf, H., Schaepman, M., and Hoelzle, M.: Cross-Comparison of Albedo Products for Glacier Surfaces Derived from Airborne and Satellite (Sentinel-2 and Landsat 8) Optical Data, Remote Sens., 9, 110, https://doi.org/10.3390/rs9020110, 2017. a
Newall, P.: High River Gold Mines LTD, The Bissa Asset, Tech. Report, Wardell Armstrong, Burkina Faso, 200 pp., https://www.miningdataonline.com/reports/Bissa_2012_TR.pdf (last access: 22 September 2022), 2012. a
Normandin, C., Frappart, F., Diepkilé, A. T., Marieu, V., Mougin, E., Blarel, F., Lubac, B., Braquet, N., and Ba, A.: Evolution of the Performances of Radar Altimetry Missions from ERS-2 to Sentinel-3A over the Inner Niger Delta, Remote Sens., 10, 833, https://doi.org/10.3390/rs10060833, 2018. a, b
Ogilvie, A., Belaud, G., Massuel, S., Mulligan, M., Le Goulven, P., and Calvez, R.: Surface water monitoring in small water bodies: potential and limits of multi-sensor Landsat time series, Hydrol. Earth Syst. Sci., 22, 4349–4380, https://doi.org/10.5194/hess-22-4349-2018, 2018. a
Olivry, J.-C.: Fonctionnement hydrologique de la Cuvette Lacustre du Niger et essai de modélisation de l'inondation du Delta intérieur, in: Grands bassins fluviaux périatlantiques: Congo, Niger, Amazone (Colloques et Séminaires), edited by: Olivry J.-C. and Boulègue J., ORSTOM Editions, Paris, France, 267–280, https://horizon.documentation.ird.fr/exl-doc/pleins_textes/pleins_textes_6/colloques2/42682.pdf (last access: 22 September 2022), 1995. a
Panthou, G., Lebel, T., Vischel, T., Quantin, G., Sane, Y., Ba, A., Ndiaye, O., Diongue-Niang, A., and Diopkane, M.: Rainfall intensification in tropical semi-arid regions: the Sahelian case, Environ. Res. Lett., 13, 064013, https://doi.org/10.1088/1748-9326/aac334, 2018. a
Papa, F., Crétaux, J.-F., Grippa, M., Robert, E., Trigg, M., Tshimanga, R. M., Kitambo, B., Paris, A., Carr, A., Fleischmann, A. S., de Fleury, M., Gbetkom, P. G., Calmettes, B., and Calmant, S.: Water Resources in Africa under Global Change: Monitoring Surface Waters from Space, Surv. Geophys., 44, 43–93, https://doi.org/10.1007/s10712-022-09700-9, 2023. a
Pekel, J.-F., Cottam, A., Gorelick, N., and Belward, A. S.: High-resolution mapping of global surface water and its long-term changes, Nature, 540, 418–422, https://doi.org/10.1038/nature20584, 2016. a, b, c
Penman, H. L. and Keen, B. A.: Natural evaporation from open water, bare soil and grass, P. Roy. Soc. Lond. A, 193, 120–145, https://doi.org/10.1098/rspa.1948.0037, 1948. a
Pham-Duc, B., Sylvestre, F., Papa, F., Frappart, F., Bouchez, C., and Crétaux, J.-F.: The Lake Chad hydrology under current climate change, Sci. Rep.-UK, 10, 5498, https://doi.org/10.1038/s41598-020-62417-w, 2020. a
Reis, L. G. de M., Souza, W. de O., Ribeiro Neto, A., Fragoso, C. R., Ruiz-Armenteros, A. M., Cabral, J. J. da S. P., and Montenegro, S. M. G. L.: Uncertainties Involved in the Use of Thresholds for the Detection of Water Bodies in Multitemporal Analysis from Landsat-8 and Sentinel-2 Images, Sensors, 21, 7494, https://doi.org/10.3390/s21227494, 2021. a, b, c
Robert, E., Kergoat, L., Soumaguel, N., Merlet, S., Martinez, J.-M., Diawara, M., and Grippa, M.: Analysis of Suspended Particulate Matter and Its Drivers in Sahelian Ponds and Lakes by Remote Sensing (Landsat and MODIS): Gourma Region, Mali, Remote Sens., 9, 1272, https://doi.org/10.3390/rs9121272, 2017. a
Sally, H., Lévite, H., and Cour, J.: Local Water Management of Small Reservoirs: Lessons from Two Case Studies in Burkina Faso, Water Altern., 4, 365–382, 2011. a
Schwatke, C., Dettmering, D., Bosch, W., and Seitz, F.: DAHITI – an innovative approach for estimating water level time series over inland waters using multi-mission satellite altimetry, Hydrol. Earth Syst. Sci., 19, 4345–4364, https://doi.org/10.5194/hess-19-4345-2015, 2015. a
Schwatke, C., Dettmering, D., and Seitz, F.: Volume Variations of Small Inland Water Bodies from a Combination of Satellite Altimetry and Optical Imagery, Remote Sens., 12, 1606, https://doi.org/10.3390/rs12101606, 2020. a
Shu, S., Liu, H., Beck, R. A., Frappart, F., Korhonen, J., Xu, M., Yang, B., Hinkel, K. M., Huang, Y., and Yu, B.: Analysis of Sentinel-3 SAR altimetry waveform retracking algorithms for deriving temporally consistent water levels over ice-covered lakes, Remote Sens. Environ., 239, 111643, https://doi.org/10.1016/j.rse.2020.111643, 2020. a
Sophocleous, M.: Interactions between groundwater and surface water: the state of the science, Hydrogeol. J., 10, 52–67, https://doi.org/10.1007/s10040-001-0170-8, 2002. a
Sun, F., Ma, R., Liu, C., and He, B.: Comparison of the Hydrological Dynamics of Poyang Lake in the Wet and Dry Seasons, Remote Sens., 13, 985, https://doi.org/10.3390/rs13050985, 2021. a
Taburet, N., Zawadzki, L., Vayre, M., Blumstein, D., Le Gac, S., Boy, F., Raynal, M., Labroue, S., Crétaux, J.-F., and Femenias, P.: S3MPC: Improvement on Inland Water Tracking and Water Level Monitoring from the OLTC Onboard Sentinel-3 Altimeters, Remote Sens., 12, 3055, https://doi.org/10.3390/rs12183055, 2020. a, b
Venot, J.-P. and Cecchi, P.: Valeurs d'usage ou performance techniques: comment apprécier le rôle des petits barrages en Afrique subsaharienne?, Cah. Agric., 20, 112–117, https://doi.org/10.1684/agr.2010.0457, 2011. a
Venot, J.-P. and Krishnan, J.: Discursive Framing: Debates over Small Reservoirs in the Rural South, Water Altern., 4, 316–324, 2011. a
Winter, T. C.: Hydrological Processes and the Water Budget of Lakes, in: Physics and Chemistry of Lakes, edited by: Lerman, A., Imboden, D. M., and Gat, J. R., Springer, Berlin, Heidelberg, New York, 37–62, https://doi.org/10.1007/978-3-642-85132-2_2, 1995. a
Xu, H.: Modification of normalised difference water index (NDWI) to enhance open water features in remotely sensed imagery, Int. J. Remote Sens., 27, 3025–3033, https://doi.org/10.1080/01431160600589179, 2006. a, b
Zhao, G.: Global lake evaporation volume (GLEV) dataset, Zenodo [data set], https://doi.org/10.5281/zenodo.4646621, 2021. a
Zhao, G., Li, Y., Zhou, L., and Gao, H.: Evaporative water loss of 1.42 million global lakes, Nat. Commun., 13, 3686, https://doi.org/10.1038/s41467-022-31125-6, 2022. a, b, c