the Creative Commons Attribution 4.0 License.
the Creative Commons Attribution 4.0 License.
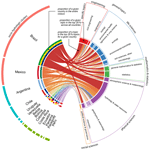
Bright and blind spots of water research in Latin America and the Caribbean
Alyssa J. DeVincentis
Hervé Guillon
Romina Díaz Gómez
Noelle K. Patterson
Francine van den Brandeler
Arthur Koehl
J. Pablo Ortiz-Partida
Laura E. Garza-Díaz
Jennifer Gamez-Rodríguez
Erfan Goharian
Samuel Sandoval Solis
Water resources management in Latin America and the Caribbean is particularly threatened by climatic, economic, and political pressures. To assess the region's ability to manage water resources, we conducted an unprecedented literature review of over 20 000 multilingual research articles using machine learning and an understanding of the socio-hydrologic landscape. Results reveal that the region's vulnerability to water-related stresses, and drivers such as climate change, is compounded by research blind spots in niche topics (reservoirs and risk assessment) and subregions (Caribbean nations), as well as by its reliance on an individual country (Brazil). A regional bright spot, Brazil, produces well-rounded water-related research, but its regional dominance suggests that funding cuts there would impede scientifically informed water management in the entire region.
- Article
(7834 KB) - Full-text XML
-
Supplement
(219 KB) - BibTeX
- EndNote
Despite being the world's most water-rich region, Latin America and the Caribbean (LAC) faces extreme weather events and a range of water-related stresses that are expected to worsen with climate change (UN-OCHA, 2020). It is critical that responses are based on sound science and contextualized. The state of water resources research in Latin America, including its bright spots and blind spots, can be thoroughly investigated using science of science: analysis of the production of science using large-scale data (Fortunato et al., 2018).
Freshwater resources face mounting pressures, brought about by human population growth and urbanization (Jenerette and Larsen, 2006; Immerzeel et al., 2020), climate change (Gosling and Arnell, 2016), economic growth and consumption patterns (Mcdonald et al., 2014; O'dorico et al., 2018), and the spread of misinformation and mistrust in science (IPCC, 2014). LAC epitomizes these water challenges with its abundant yet unequally distributed water resources (DESA, 2019), mounting pollution, and the highest income inequality in the world (Varis et al., 2019). Marked disparities exist in terms of water availability (both quality and quantity), climate change vulnerability, degree and form of urbanization, conservation habits, and scientific productivity, which each affect water resources management (Ciocca and Delgado, 2017; Lyon et al., 2019). Countries with abundant water resources, Brazil for example, experience water scarcity due to a mismatch between water-rich areas and population centers (Formiga-Johnsson and Kemper, 2005), while others, Argentina, Chile, and Bolivia for example, face flooding and melting glaciers (Barros et al., 2015; Soruco et al., 2015; Masiokas et al., 2019), yet others in Central America are increasingly devastated by hurricanes (Bárcena Ibarra et al., 2020).
LAC is among the most urbanized regions in the world where population densities in water-rich regions can be many times higher than arid regions, such as in Argentina. These high-density areas face particular vulnerability to water quality and supply reliability (Kim and Grafakos, 2019). For example, São Paulo faced severe water shortages during a 2014 drought, while Mexico City has steadily and rapidly depleted its groundwater supply (Aguilar-Barajas et al., 2015). Urban pressures on water resources are compounded by inefficient farming practices, unregulated industries, and aging infrastructure across the region. Worldwide, and particularly in LAC, these water challenges are expected to intensify with climate change as variations in precipitation, temperature, and evaporation threaten water availability for current and future water users (Dussaillant et al., 2019; Gesualdo et al., 2019; Zaninelli et al., 2019).
While uncertainty surrounds the reliability of water supplies to address water-related risks and meet future needs in LAC, water resources management is a relatively young field of study (Montanari et al., 2015) and suffers from a lack of interdisciplinary and integrative perspectives, common in the environmental sciences (Norgaard, 2008). Recent review papers are limited to a geographic area (Owusu et al., 2016; Paiva et al., 2020), individual components of the water budget such as a watershed (Dobriyal et al., 2012), particular methodology (Plummer et al., 2012), specific water user (Ran et al., 2016), or small sample of documents (Endo et al., 2017). Paiva et al. (2020), for instance, conducted a review of 250 conference papers from the 2019 Brazilian Water Resources Symposium to better understand major advances and challenges in Brazil's water science.
Given these circumstances, it is critical to understand how the breadth of past water resources research across LAC contributes to the scientific knowledge necessary for decision-making processes (Cvitanovic and Hobday, 2018). To assess the state of water research in LAC, we used a science of science approach (Fortunato et al., 2018) and performed an unprecedented, multilingual review of available peer-reviewed literature to identify bright spots of scientific inquiry: topics and locations where water research is abundant, spread out, and well connected (Uzzi et al., 2013; Astudillo, 2016; Larivière et al., 2015). Conversely, blind spots of past research are defined as topics and locations where water resources are less-thoroughly studied. This approach identifies opportunities to enhance collaboration within the research community and to ensure future research meets societal needs.
To perform the literature review, we assembled a corpus of 20 000 water resources research articles in English, Spanish, and Portuguese by querying online databases and modeled the topics of each article with latent Dirichlet allocation (Blei et al., 2003). Topics are groups of statistically co-occurring words, which we labeled based on four categories: 5 general research topics (e.g. physical sciences), 43 specific topics representing subfields of research (e.g. geochemistry), 17 water-budget topics (e.g. reservoirs), and 13 method topics (e.g. remote sensing). For each article, topic probabilities are the probabilities that the article's content corresponds to each individual topic. In addition, we read a random subset of 2000 papers from the corpus to validate results from the topic model and identify study location. We leveraged this manual reading process to identify the study location for the entire corpus using machine learning and used article metadata to generate a citation network of citing and cited references within the corpus.
To contextualize results from the literature review, we used publicly available data to statistically cluster countries into groups with similar social and hydrological systems, socio-economic metrics, and measures of water resources abundance and use. This clustering process allowed for more meaningful interpretation of subsequent results within and across countries. To further ground our results in the current research landscape, we invited 20 000 corresponding authors from our corpus to share their experiences through a survey focused on research discipline, accessibility, and connectivity. A total of 1969 respondents from 35 countries and a variety of disciplinary backgrounds completed this survey.
Bright spots and blind spots of water resources research in LAC were evaluated using three concepts: abundance, spread, and connectivity. Abundance was measured as research volume by country and by topic. Spread was estimated by topic normality across countries and articles, describing how close a topic's probability distribution is to the standard normal distribution. Connectivity was determined with a weighted citation network across countries and topics, describing the probability that a specific node (country or topic) is cited by other nodes.
The rest of the article is organized as follows. The next section details how the data underlying this study were acquired. Section 3 presents the methods we used to associate metadata (e.g. topic, location) with each document in the corpus. In that section, we also introduce the specific metrics and methods used to infer bright and blind spots. Section 4 exposes results associated with metadata generation. Section 5 presents and discusses bright spots and blind spots. The last section summarizes our findings.
In this section, we detail the specific process for corpus collection, retrieving socio-hydrologic and survey data.
2.1 Corpus collection
The process of corpus collection consisted of four steps:
- i.
querying online databases;
- ii.
retrieving documents;
- iii.
iteratively assessing quality of the corpus and correcting bias; and
- iv.
cleaning the corpus.
First, we defined the query to obtain water resources research about Latin American and Caribbean (LAC) countries. We selected a peer-reviewed literature database based on the following criteria: (1) inclusion of journals from LAC (e.g. SciELO); (2) number of query results in English, Spanish, and Portuguese; and (3) expert assessment of the results with a focus on relevancy. Web of Science and Scopus databases were chosen.
Results from the query were assembled into .ref
data files.
Second, in accordance with institutional licensing, EndNote was used to retrieve documents from the assembled reference files. This method of corpus collection showed an unequal return rate across the three languages (Table S1 in the Supplement), which was corrected for in the subsequent steps.
Third, we performed a quality assessment of the corpus to assess possible bias between query results and document retrieval within distinct sources or time periods (Year). We defined the within-corpus bias as the difference in relative frequencies f between the query and corpus, such that
A corpus with satisfactory quality would be one presenting minimal bias from Eqs. (1)–(2). To address the possibility that our automated approach could result in between-corpus bias in terms of return rate, we augmented the corpus by manually downloading articles to correct for biases by year and presence of digital object identifier (DOI). We defined the English corpus as well-behaved and used it as a reference to adjust the Spanish and Portuguese corpora so that each language's corpus reached similar return rates. This adjustment reduced both within- and between-corpus bias by using systematic sampling with unequal inclusion probabilities of the pi (Madow and Madow, 1949), where pi was defined using the normalized product of the bias functions and i refers to the ith non-retrieved article. This procedure was performed twice leading to a similar return rate across languages (Table S1).
Lastly, we performed a cleaning process to prepare the article texts for the topic model. Texts were converted into a standardized version in which all cases were lowered, words not found in a dictionary were removed, and special patterns for words such as emails and URLs were assigned tags. Additional cleaning removed punctuation (except for apostrophes and hyphens inside words) and single-letter words. Finally, lemmatization (the reduction of a word to a common base form) was performed using TreeTagger software by changing all nouns into singular form and all verbs into the infinite tense (Schmid, 1999). This final version of each corpus, with lemmatization, was then used as input for topic modeling.
2.2 Collecting socio-hydrologic attributes
To build a database of socio-hydrologic country descriptors, we collected 43 relevant indicators from five indexes to compare and contrast LAC countries (Table S2, Data S1). We selected the indicators from the following databases, chosen for their international recognition and global or LAC-wide breadth of data:
-
AQUASTAT, (http://www.fao.org/nr/water/aquastat/tables/index.stm, last access: May 2021);
-
Environmental Performance Index (https://epi.envirocenter.yale.edu/epi-downloads, last access: May 2021);
-
Global State of Democracy (https://www.idea.int/gsod-indices/#/indices/world-map, last access: May 2021);
-
Social Progress Index (https://www.socialprogress.org/index/global/results, last access: May 2021);
-
LAC INFORM (http://www.inform-index.org/Subnational/LAC, last access: May 2021).
AQUASTAT is a global information system produced by the Food and Agriculture Organization of the United Nations and presents a perspective on agriculture and water resources availability, infrastructure to support large-scale regional planning, and analysis. The Environmental Performance Index considers two fundamental dimensions of sustainable development: environmental health, which rises with economic growth and prosperity, and ecosystem vitality, based on 24 indicators (Wendling et al., 2018).
The Global State of Democracy indices depict democratic trends at the country, regional, and global levels across a broad range of different attributes of democracy in the period 1975–2015. Democracy is conceptualized as popular control over public decision-making and decision makers and equality of respect and voice between citizens in the exercise of that control. The index translates these principles into five main democracy attributes: representative government, fundamental rights, checks on government, impartial administration, and participatory engagement (International Institute for Democracy and Electoral Assistance, 2017).
The Social Progress Index is a comprehensive measure of quality of life, independent of economic indicators, and is designed to complement economic measures such as gross domestic product. Social progress is defined as the capacity of a society to meet the basic human needs of its citizens, establish the building blocks that allow citizens and communities to enhance and sustain the quality of their lives, and create the conditions for all individuals to reach their full potential. The index aggregates three broad dimensions of social progress: basic human needs, foundations of wellbeing, and opportunity (Fehder et al., 2018).
The LAC INFORM Risk Index simplifies risk-based information for LAC countries. A risk score is calculated for each country by combining 82 indicators that measure three dimensions: hazard and exposure, which captures potential hazardous events and the number of people that could be exposed; vulnerability, which measures the fragility of socio-economic systems and the strength of communities, households, and individuals to confront a crisis situation; and lack of coping capacity, which takes into account a country's institutional and infrastructural strength to cope with and recover from crisis (INFORM, 2018).
Although these indicators do not capture the full spectrum and complexity of factors related to water, they allow for an analysis of the topic modeling results based on country clusters with similar characteristics.
2.3 Electronic survey
Results from the literature review were ground-truthed with an electronic survey sent to corresponding authors of the articles from the corpus. The survey aimed to shed light on researchers' characteristics by including questions about their research experience, institutional affiliation, publication history, their perceptions on funding and interdisciplinarity in the field of water resources, and open-ended questions. The survey received exemption from the University of California, Davis, IRB Administration (ID 1335782-1).
The survey was designed to include 16 questions, in three languages (English, Spanish, and Portuguese), and take approximately 5 min to complete (Table S3). Respondents were asked questions about their position; institutional affiliation; years of experience; main research discipline; countries of birth, residence, and research focus; number of peer-reviewed publications; motivations for picking journals for publications; source of funding; and opinions regarding interdisciplinary research.
The survey was sent in November 2018 to corresponding authors from 22 324 papers, a subset of the final corpus, using Qualtrics survey distribution software. This included articles written in each language: 20 332 English, 1293 Spanish, and 699 Portuguese. The survey was resent to non-respondents weekly until February 2019, leading to 1969 responses. The survey response data was cleaned and prepared for analysis. The survey responses in Spanish and Portuguese were translated to English and compiled into one document.
In this section, we detail the following:
- i.
the clustering of countries from their socio-hydrologic data;
- ii.
the generation and mining of metadata; and
- iii.
the metrics to identify bright and blind spots of water research.
3.1 Clustering analysis
We clustered countries based on socio-hydrologic variables using two different methods: k-means clustering (Hartigan and Wong, 1979) and hierarchical clustering (Murtagh, 1983). We performed the clustering with Euclidean distances and following Ward's criterion. We then investigated the optimal number of clusters by evaluating the evolution with the number of clusters of the total within sums of squares and of the average silhouette width (Rousseeuw, 1987). In addition, we used the following four validation metrics to assess the stability of the clustering under the complete set of clustering variables and performed an iterative procedure where one variable is removed from the set, an approach akin to leave-one-out cross-validation:
-
The average proportion (APN) measures the proportion of observations not placed in the same cluster under both cases and evaluates how robust the clusters are under cross-validation (Datta and Datta, 2003).
-
The average distance between means (ADM) measures the variation of the cluster center and evaluates the stability of the localization of the cluster in the multidimensional clustering variable space (Datta and Datta, 2003).
-
The average distance (AD) measures the distance between observations placed in the same cluster and evaluates within-cluster stability (Datta and Datta, 2003).
-
The figure of merit (FOM) estimates the predictive power of the clustering algorithm by measuring the within-cluster variance of the removed variable (Yeung et al., 2001).
3.2 Metadata generation and mining
Each document in the corpus was augmented by generating and mining metadata. The mined metadata correspond to author keywords as well as the citing and cited literature resulting in a citation network. The generated metadata correspond to modeled topics and study location.
3.2.1 Topic model
The content of the corpus documents were modeled using latent Dirichlet allocation (LDA), a Bayesian, generative, probabilistic model conceptualizing each document in a corpus of documents as a random mixture of topics (Blei et al., 2003). Topics are a Bayesian bag of words corresponding to a distribution over the vocabulary (i.e. the words appearing within the corpus). In essence, one topic corresponds to words that have a significant probability to co-occur.
The LDA was programmed to identify 105 topics in English and 65 topics in the Spanish and Portuguese corpora based on commonly used metrics for LDA tuning. We then conducted a quality assessment of the topic models through cross-validation. For this we developed human-derived topics for the English corpus by reading a subset of 1428 papers from the corpus and manually identifying single-word tags based on keywords and main research topics. A similar percentage of documents were read for the Spanish and Portuguese corpora: 188 and 111, respectively.
As topics are statistical objects, they must be assigned a human label to make them tractable. An interdisciplinary review panel of eight water experts therefore assigned labels for each topic while simultaneously evaluating their significance. Topics were removed if multiple members of the review panel determined that the most frequently occurring words were irrelevant based on their expert knowledge of water resources science. The remaining relevant topics were tagged with five labels independently by multiple reviewers, and the collection of proposed topic labels were then harmonized to produce final topic labels. Topics were assigned labels for each of several levels: (i) specific topic name; (ii) theme, i.e., categories of scientific research as defined by the US National Science Foundation (NSF) that were either (iii) specific or (iv) broad; and (v) description, i.e., spatial scale, water budget, or methods. These labels were consolidated into four topic categories: general, specific, methods, and water budget.
3.2.2 Metadata mining and citation network
Article metadata containing the citing literature and author-defined keywords for articles from the English corpus were extracted using Elsevier API and each article's DOI. We used this citing literature to build a citation network. Keywords were used to supplement the country location labels assigned during human-reading by looking for regular expressions of the name of the target countries. Of the 31 countries in LAC, only 23 countries had a sufficient occurrence (i.e., at least 30 articles) within the human-read subset of the English corpus and were included in the citation network.
The citation network was extracted from article metadata, which stores identifiers for the citing article (i.e. the article to which the metadata are attached to), and the cited articles. A total of 29 900 citations were found between 4603 unique articles of the English corpus. The resulting bibliographic network is defined by its 4603×4603 adjacency matrix B and was filtered to remove edges between nodes external to the collected corpus. The citation network between countries, BC, was obtained by
where C is a 4603×23 matrix containing the output of the machine learning probabilistic predictions of the location of study between 23 possible countries of study (see below). In consequence, BC is a weighted directed adjacency matrix. Similar reasoning leads to the citation network between specific research, method, and water budget topics by changing the matrix C in the previous equation for the LDA probabilistic predictions of topics.
3.2.3 Location prediction
We used machine learning to predict the location of the country of study of each paper in the English corpus. The training labels were provided by human-reading randomly chosen articles from the corpus (1428 human-derived labels) and from text mining the article metadata (2663 text-mined labels). Interestingly, the human-reading provided 563 observations of irrelevant country locations (i.e. outside LAC) or irrelevant subjects of study (i.e. not water resources related). This occurred in some cases when our queries returned articles containing accurate keywords but different meanings than intended; for example, the search work “Mexico” returned irrelevant locations including regions of the United States around the Gulf of Mexico and irrelevant topics such as signal processing analyses employing the “Mexican hat” wavelet.
The human-derived labels were first used for constructing a relevance filter based on simple binary classification between “Relevant” and “Irrelevant” documents (n=1386 after quality control). The predictors consist of the text document term matrix derived from the cleaned corpus text using tokens related to country names and of the topic membership output by the topic model (p=138). A benchmark of the six following models was conducted: featureless (baseline), random forest, support vector machine, naive Bayes, multinomial regression, and extreme gradient boosting. The hyper-parameters were initially set at standard default value. The resampling scheme was 10 repetitions of 10-fold cross-validation. Best performing models were selected for further tuning using a nested cross-validation (Bischl et al., 2012) with a simple hold-out inner loop and 10 repeats of 10-fold cross-validation as an outer loop. The size of the tuning grid was set to 16 between standard values for each hyper-parameter.
The prediction of the location of study for each document was performed using both human-derived and text-mined labels (n=3494 after quality control). The predictors consisted of the text document-term matrix derived from the cleaned corpus text using tokens related to country names (p=33). Models and resampling schemes were similar to the ones used for the relevance filter. The hyper-parameters were initially set at standard default value.
3.3 Bright spots and blind spots
This section details assessments of the following:
- i.
ground truth from analyzing survey data;
- ii.
research volume;
- iii.
research spread using topic normality; and
- iv.
research connectivity from network analysis.
3.3.1 Survey analysis
Closed-ended responses of the electronic survey were analyzed by tallying aggregate data by country and discipline of study. This allowed for a number of inferences such as the most commonly represented research disciplines and the countries of study and of origin of respondents. Research collaborations were analyzed based on the three main countries of study and the three main countries of research collaborations for every respondent.
Open-ended responses were coded for content and analyzed in ATLAS.ti, resulting in dozens of codes used to group responses of similar content (Table S7). Comments irrelevant to the study were removed. We also identified relationships between codes based on connections in the data. If respondents mentioned political issues that hamper funding availability, we coded the two elements (e.g. “political issues” and “funding difficulties”, and we then linked them with the qualifier “is the cause of”). In addition, through the “word cruncher” tool, we generated a word frequency from the survey comments resulting in a word cloud visualization where words' sizes are proportional to their use frequency.
3.3.2 Research volume over time
A timeline was created with the number of new research articles published per year representing countries in the three socio-hydrologic clusters, to visualize growth in research output over time. The articles were sourced from the English corpus of water resources research, because these articles were labeled with the country of research and could therefore be associated with a socio-hydrologic cluster. To better understand trends observed in each socio-hydrologic cluster, a residual analysis was performed. The data were transformed with a logarithmic transformation to obtain a roughly linear relationship between time and research output, and then a linear regression was calculated. The residuals for each year were plotted and displayed starting from 2000 to 2017. Year 2000 was chosen as the starting point, because it marks the time by which research output had increased enough to reach at least 30 new articles in each socio-hydrologic cluster per year. The residuals were then plotted along with brackets of the standard deviation (for both positive and negative values) to provide a reference of significance.
3.3.3 Topic normality
The normality of research topics was estimated for general and specific topics, as well as for the method and water budget topics. Documents in each subset were sourced from the English corpus. Each subset was filtered for documents that were labeled with a country of research and then for countries where the sum of documents per country was greater than 30. A statistical distance from standard, normal distribution was calculated to describe the normality of topic probabilities from two perspectives: across documents and across countries. As a statistical distance, we chose the Jensen–Shannon distance because of its link with entropy (Lin, 1991) and its interpretation in terms of a proper distance (Endres and Schindelin, 2003). The Jensen–Shannon distance, dJS, is calculated between two probability density functions N and P, and dJS=0 if P=N (see Appendix A for full derivation). Here, we chose N as the standard normal distribution, 𝒩(0,1). P is the standardized probability distribution of topic probability distribution across either documents or countries. To better meet the intuition of normality, we define it as 1−dJS so that normality is closer to 1 when the P is closer to the standard normal distribution.
3.3.4 Citation network analysis
The citation network was analyzed using Gephi 0.9.2 (Bastian et al., 2009). Descriptive parameters and geometry metrics were calculated. We conducted this network analysis at each LAC country level for general and specific topics, as well as water budget and methods topics. Probability adjacency matrices were extracted from predictive model results and normalized to highlight the relationship between topics or countries regardless of sample size in our corpus.
A force-directed graph algorithm, Fruchterman–Reignold (FR), was selected to produce the networks' visualizations. It simulates the graph as a system of mass particles. The nodes are the mass particles and the edges are springs between the particles. The algorithm tries to minimize the energy of this physical system. FR is most suitable for small networks and a better performance (Fruchterman and Reingold, 1991; Jacomy et al., 2014). The force-directed citation networks show the degree of connectivity by the nodes' size and edge thickness, but there is no distinction between positive or negative citations (Bruggeman et al., 2012).
For each network, the following geometric descriptions were calculated: number of nodes (countries or research topics), number of edges (citation between countries or research topics), and thickness of the edges (connectivity proportion). We also calculated network density and degree. Network density is a measure of the connectedness of a graph, defined as the number of connections divided by the number of possible connections, with all possible edges and density = 1 (Tonin et al., 2019). Degree is the number of connections each node (country or topic) has with another node (country or topic). Degree has generally been extended to the sum of weights when analyzing weighted networks and labeled node strength, so the weighted degree and the weighted in- and out-degree were calculated (Newman, 2001; Barrat et al., 2004; Opsahl et al., 2010). In all visualizations, edge thickness represents weighted degree, and network node size represents research volume defined as the sum of probabilities for the given node in the corpus.
4.1 Clustering results
LAC countries were clustered based on socio-hydrological characteristics using hierarchical and k-mean clustering. Both clustering methods yielded similar results. The total within sums of squares evolved after two clusters were chosen. Similarly, the average silhouette width strongly exhibited a peak for two clusters. Further inspection of clustering in principal component dimensions indicated that the cluster with Mexico and Brazil was significantly distinct from all other countries, explaining the observation of a sharp peak in average silhouette width. However, validation metrics exhibited optimal null values of average proportion (AP) and average distance between means (ADM) for two or three clusters. In addition, average distance (AD) and figure of merit (FOM) were lower for three clusters than for two. Based on these results, we chose three clusters to describe the grouping of countries based on their socio-hydrologic variables.
4.2 Metadata generation
4.2.1 Topic model
We determined if the LDA successfully identified a relevant topic based on the top 10 occurring words which showed a 86 % agreement between expert-identified topics and LDA-derived topics. We judged the performance of the LDA by comparing the topic model output from the English corpus to the output from the Spanish and Portuguese corpora. The specific topic label for each topic was used for comparison. The number of topics with each specific label were grouped by language and tallied (Fig. 1, Tables S4–S6).
Our findings are based on the output of a topic model of articles written in English and are predicated on the assumption that the English language corpus accurately represents the breadth of regional research published in English, Spanish, and Portuguese given that non-English corpora were small fractions of the English corpus (4 % and 2 % for Spanish and Portuguese, respectively). Comparing the topic model performance of the three corpora (Fig. 1) supports this assumption. One-third of the specific topics present in the English corpus are present in all languages. Importantly, this subset of topics includes the majority (10 of 16) of the top 25 % of research topics from the English corpus. Another third of the topics are present in two of the three languages, and the last third is only present in English. Because of the small size of the Spanish and Portuguese corpora, it is likely that their topics do not cover the entire scope of peer-reviewed water resources research published in these languages. While access to more articles would have resulted in even closer alignment with the English corpus, the existing alignment between topics present across all three corpora is sufficient to support our decision to base topic model conclusions on the English corpus alone.
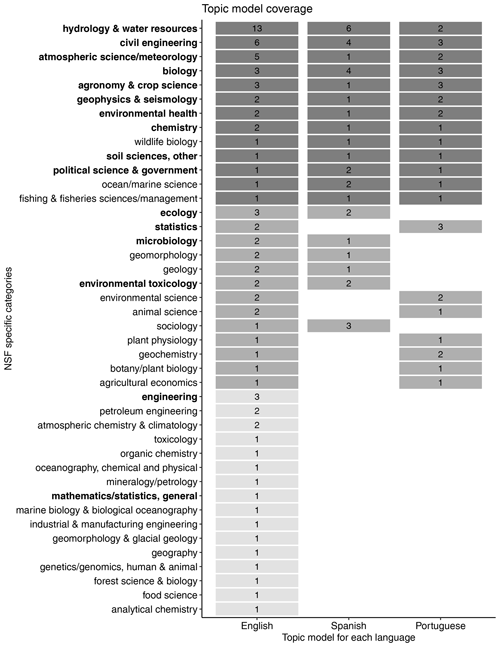
Figure 1Topic model performance assessed by the total number of specific topics present in each language. Bolded topics represent the top 12.5 % of research (named topics in Fig. 6). Numbers in each box correspond to the number of topics from the topic model that were manually grouped together under a common label.
4.2.2 Relevance filter and location prediction
For the relevance filter, the random forest, multinomial, and support vector machine models were the best-performing models and showed no statistical difference in the distribution of their performance measured by area under curve (AUC, Fig. 2a). Random forest and multinomial were the best-performing tuned models and showed no statistical difference in the distribution of their performance measured by AUC (Fig. 2b). The distribution of the hyper-parameter resulting from the nested cross-validation showed a better constraint for the multinomial model which was selected for predictions. There were 12 616 articles predicted as relevant to our study. The performance of this multinomial model corresponds to a mean AUC of 0.82, a mean accuracy of 77 %, and a true positive rate of 86 %.
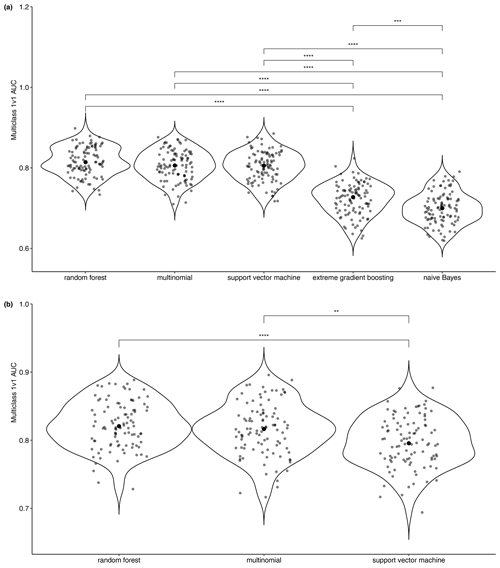
Figure 2Machine learning performance for relevance prediction based on simple binary classification between “Relevant” and “Irrelevant” documents (n=1386). (a) Performance of untuned models; (b) performance of selected tuned models. Brackets highlight statistical differences between distributions.
For predicting location of the country of study of each paper, random forest outperformed every other model with a mean multiclass AUC of 0.99 and a mean accuracy of 96 % (Fig. 3). No further tuning was performed, and the random forest with default hyper-parameters was used for predictions. More complex approaches were investigated: using additional geographical tokens (e.g. rivers and mountain range names), multi-label classifications (e.g. binary relevance, label powerset), or deep learning models of natural language processing (e.g. Google's BERT; Devlin et al., 2018) both on full texts and abstracts. These more complex methods yielded similar results than our simpler initial approach and were therefore not pursued further for this study.
4.3 Survey results
The most common categories of answers in the comment section were summarized (Table S7). Difficulties related to funding stood out as the main challenge for respondents (Fig. 4). These were often linked to political or economic trends in their countries. Respondents further explained that available funds are not only distributed unequally across countries but also within countries and across research areas. Collaboration, or the lack thereof, was another important theme. Many respondents claimed that they collaborated more with North America, Europe, and Australia than with other LAC countries, in large part due to funding opportunities. Many indicated an interest in collaborating with researchers in other LAC countries.
5.1 Volume: growth through time
First, we look at research volume by country and topic. The cumulative research growth showed an exponential growth trend over the last 4 decades, although the trend was more evident in cluster 1 (Brazil and Mexico) than in clusters 2 or 3 (Fig. 5). These results were used to interpret periods of time in which the scientific production of water research was increasing or decreasing on a region-wide scale, in reference to the growth trajectories of each socio-hydrologic cluster. Reasons for this growth could relate to a wide array of changes in LAC over the last 40 years beyond an increasing interest in addressing water-related knowledge gaps: increased access to the internet and online resources, availability of existing research in online repositories, and expansion of opportunities in higher education (Delgado-Troncoso and Fischman, 2014; Baskaran, 2017).
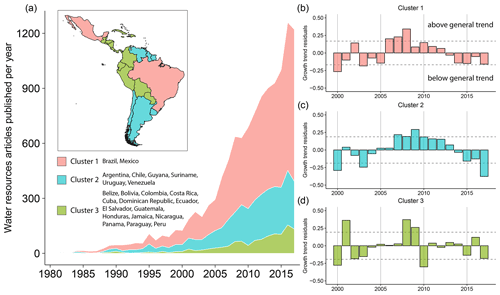
Figure 5Growth of water resources research. (a) New articles per year for each socio-hydrologic cluster (inset). (b–d) Residual analysis estimating the deviation from the exponential trend for each socio-hydrologic cluster.
A residual analysis identifies three distinct periods since 2000, the first year that each socio-hydrologic cluster was represented by over 30 research papers in each language. Annual output was lower than each cluster's general trend for the first few years, followed by a period of relatively higher output from 2007–2012, ending with a trend of decreasing growth from 2013–2017, although some residuals are below a single standard deviation within these periods. It is possible that uniform anomalies below or above general growth trends correspond to region-wide events, although a several-year lag could be possible between causal events and effects in research output. For example, a connection may exist between Brazil's economic crisis starting in 2012 and the subsequent drop in research output from 2013–2017.
5.2 Where and what is being studied?
Combining research topics with predicted study location describes the composition of water research in LAC with a chord diagram (Fig. 6). This chord diagram was obtained from the weighted bipartite network between countries and topics (Fig. 6). For legibility, the network was filtered to remove the edges with weight lower than the 75th percentile of the edges of a given country. In other words, for each country, only the top 25 % links are displayed.
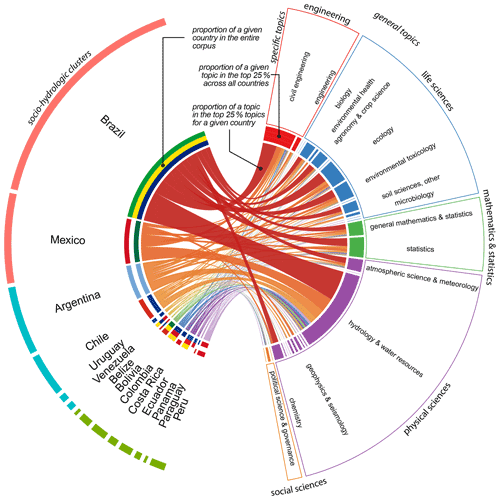
Figure 6Composition of water research in LAC according to study location and top 25 % of studied research topics. On the left, countries are identified individually and by their associated socio-hydrologic cluster. On the right, research topics are grouped by their general category. The top 50 % of specific topics are listed within each general category.
While research about Brazil, Mexico, Argentina, and Chile are bright spots that dominate the research landscape, the absence of countries in the Caribbean and most of Central America indicates a shortage of research in these regions. A country's socio-hydrologic cluster correlates to its representation in overall research, with cluster 1 (Brazil and Mexico) receiving the most research, followed by cluster 2, then cluster 3, which includes most of Central America and the Caribbean. Although population size likely affects each country's representation in the overall research output, it does not precisely correlate with research volume. We therefore expect that other factors used to define the country clusters (e.g. a country's water and economic resources, geography, and history) influence the likelihood that researchers study that country.
Mexico and Argentina alternate for second highest representation, depending on the topic, after Brazil. These findings are confirmed by survey respondents, of which 35 % study Brazil, followed by Mexico with 15 %, and Argentina with 9 % (across all research topics). Only 12 % of survey respondents focused most of their research on countries in the Caribbean or Central America.
Water research is not distributed equally among disciplines and is primarily conducted in the physical and life sciences, representing together 80 % of topic probabilities. Survey responses also confirm these findings, as 80 % of respondents identified as life and physical scientists. The lack of water research in social sciences may reflect a combination of low publication rates (compared to physical sciences), disciplinary preference for publishing in books rather than peer-reviewed articles, a historical framing of water management as a purely technical discipline (Callaghan et al., 2020), and financial resources from governments biased towards physical sciences and engineering.
5.3 Spread: research on reservoirs and risk assessment is siloed
Next, we look at the spread of water research in LAC, described by topic normality for general, specific, water budget, and method topics. Importantly, Caribbean nations and most of Central America did not have enough research to be included in these analyses and represent blind spots. For general topics (Fig. 7a), physical and life sciences represent bright spots, with topics spread normally across both countries and documents. Conversely, underlining findings from research volume (Fig. 7), the limited spread of social sciences across documents represents a blind spot. For specific topics (Fig. 7b), hydrology, atmospheric sciences, statistics, and mathematics are bright spots across countries and documents, while marine biology and forest science are likely blind spots. The majority of specific topics have high normality across countries (>0.8) but low normality across documents (<0.4). These topics are either infrequently mentioned or, if mentioned, are the sole subject of a paper, lacking integration in interdisciplinary research. For water budget topics (Fig. 8a), precipitation, which must be monitored and understood to manage water resources, has a distribution closest to normal, representing a bright spot, while glaciers is far from having a normal distribution, likely because few countries have glaciers to study (i.e. Argentina, Bolivia, Chile), and they are relatively less relevant for other countries' water budgets. For method topics (Fig. 8b), statistics, quantitative methods, and water sampling are identified as bright spots of water research methods across the region.
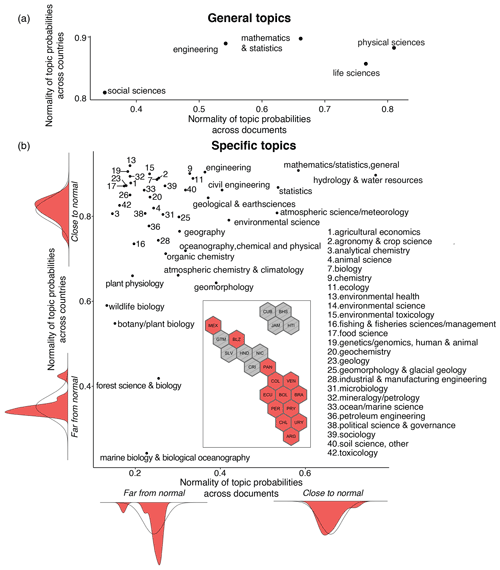
Figure 7Normality of research topics for (a) general topics and (b) specific topics. Inset shows the countries excluded from the analysis (gray).
The least normality is seen in two topics of great importance for water management: reservoirs and risk assessment. Both topics have normality values far below 1 across both countries and documents, suggesting poor representation of these topics on a broad scale (Fig. 8). These are alarming blind spots given the importance of reservoirs for water supply reliability and their impacts on local communities and ecosystems. Furthermore, a lack of research in reservoirs and risk assessment has troubling future consequences as climate change increasingly acts as a risk multiplier, including for reservoir operation. Although it is possible that information on reservoirs and risk assessments exists in gray literature, such as government reports and university publications, this information is not as accessible as scientific publications and limits regional knowledge sharing. These findings confirm similar results in a country-specific analysis of water research opportunities in Brazil (Paiva et al., 2020).
5.4 Connectivity: Brasil é número um
We complete our description of LAC's water research portfolio by estimating degrees of connectivity. Four network graphs were produced. The network graphs showed a density value of 1; as expected, all topics and countries are connected. The country citation network identified 23 nodes and 529 edges. The network degree of connectivity has a maximum degree of <50 %, medium degree of <30 %, and minimum degree of less than <10 %, where each node corresponds to a LAC country and where colors represent socio-hydrological clusters. The general topics network identified 5 nodes and 25 edges, with a degree of connectivity from a maximum of 45 % to a minimum of 27 %. The specific topics network has 43 nodes and 1849 edges, with a maximum degree of connectivity of 18 %, a medium degree of 10 % and a minimum of 5 %. The water budget and topics network has 27 nodes and 729 edges, and a maximum degree of connectivity of 5 %.
The high volume of research about Brazil (45 % of the labeled English corpus) motivated further investigation to see if this large scientific output is proportionally more influential than the research about other countries. Brazil is a central bright spot of the citation network (Fig. 9), as citations by publications from all countries, including Brazil itself, are primarily directed toward research about Brazil (49 %). Furthermore, the geographic location or socio-hydrologic cluster of a country do not appear to indicate the extent of citations of researchers working in that country. For example, although Argentina and Chile share a particularly long border and a rich history of political and economic ties, the citation network reveals only a medium degree (14 %) of connectivity between the two countries. Similarly, countries in the Caribbean and Central American regions that share many similarities, including water management challenges, show a low degree of connectivity (less than 5 %).
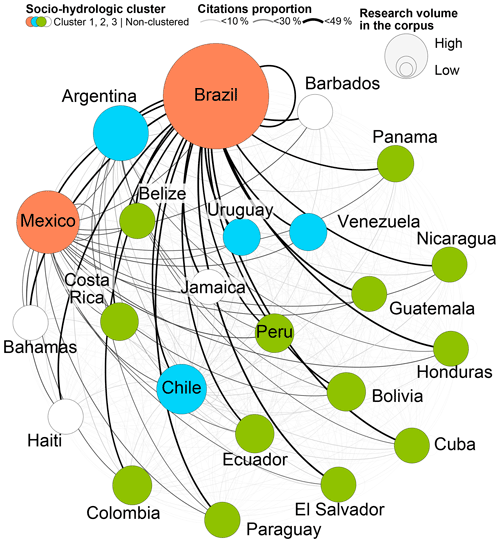
Figure 9Connectivity between LAC countries, measured by directional citations between articles' country of study. The direction of each edge is represented by drawing it clockwise from an earlier node to a later node.
Results from our survey complement findings from the citation network. Over half of participating researchers collaborated with researchers outside of the country(ies) they study. Despite being the only Portuguese-speaking country, Brazil was the country most often listed in collaborations within LAC (17 %). Brazil's prominence may be partially explained by the legacy of relationships formed during graduate-level training when Brazilian researchers study abroad and when graduate students from other South American countries study in Brazil. Despite its greater connectivity, the review of 250 water science papers presented at the 2019 Brazilian Water Resources Symposium still found a lack of a common scientific agenda within the country and a need for more interdisciplinary research and collaboration with international communities, “especially with other Latin American countries with shared water issues” (Paiva et al., 2020).
A quarter of research collaborations involve non-LAC researchers, mostly in the United States (14 %). This may reflect differences in access to funds and highlights how more affluent countries can influence the scope of research conducted in LAC. Respondents indicated that insufficient and precarious funding arrangements are their main challenge. 89 % of respondents said that the government is their main source of funding, which may explain that a country's political and economic context were mentioned as further aggravating funding availability. Funding difficulties were also associated with a lack of value given to water research and to the long timeframes associated with research that are misaligned with decision-makers' timelines.
Furthermore, being physically close or part of the same socio-hydrologic clusters did not increase the likelihood of cross-country collaboration between countries. For instance, 22 % of researchers in the neighboring countries of Argentina or Chile either study both countries or collaborate with one another, while 24 % of those researchers report collaboration with Brazil. Researchers from Mexico and Brazil, who share a socio-hydrologic cluster, collaborate even less, with only 14 % reporting to work or collaborate in both countries, despite a high level of connectivity from Mexico to Brazil in the citation network. Conversely, more than 80 % of researchers in the Caribbean reported collaborating with researchers from other Caribbean nations and few collaborated with Brazil. This is in opposition to the findings from the citation network, showing few citations within the Caribbean and more frequent citations of publications on Brazil, but this could partially be due to the limited number of articles studying the Caribbean region included in our corpus.
5.5 Opportunities exist for building research communities
We assessed the connectivity of water research throughout the region by aggregating research from all countries by topic (Fig. 10). Separate networks are presented to illustrate connectivity between the five general topics (Fig. 10a) and specific topics (Fig. 10b). A reciprocal relationship between the physical and life sciences dominates the citation network, while research within social sciences, engineering, and mathematics is cited less often (Fig. 10a). Research connectivity from all subdisciplines is heavily polarized towards research on hydrology and water resources; however, certain topics display a comparable degree of self-citation (Fig. 10b). This may indicate that water resources research communities in political science and governance, civil engineering, ecology, geophysics, and toxicology are siloed or more connected to non-water resources research communities.
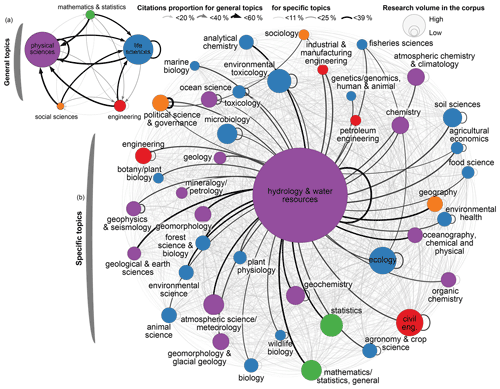
Figure 10Connectivity between topics of research, measured by directional citations between articles' research topic for (a) general topics and (b) specific topics. The direction of each edge is represented by drawing it clockwise from an earlier node to a later node.
Interestingly, behind these few silos and the central node, a vast network of connectivity exists. While this level of connectivity is low (less than 10 %), it supports the characterization of water resources as a scientific discipline where research topics are already integrated (Rosbjerg and Rodda, 2019), albeit with room for strengthened interdisciplinarity. Survey respondents confirm this low level of background connectivity, reporting dissatisfaction with the interdisciplinarity of their research. Nonetheless, an opportunity exists to build off these existing connections to form communities of researchers and strengthen research impact through knowledge sharing and collaboration across disciplines (Uzzi et al., 2013; Astudillo, 2016; Larivière et al., 2015).
5.6 Limitations and future research opportunities
The wide scope of this study, intended to capture the breadth of the state of water resources research across LAC, required inevitable compromises in the depth of information and the subsequent ability to thoroughly interpret our results. Notably, much scientific literature in Spanish and Portuguese was not readily available or accessible online, and this resulted in the need to rely on English publications as a proxy of research across LAC. A targeted method to collect gray literature would increase the size of the Spanish and Portuguese corpora. Of the literature we found, very little focused on Caribbean countries, and this lack of information limited subsequent analysis. A targeted method of corpus augmentation and human-read validation towards less-represented countries and topics will likely increase the model's predictive capabilities and may improve the representation of Caribbean countries.
In addition, while the presented citation network included all LAC countries, the exclusion of countries outside of LAC prevented a more comprehensive analysis of LAC countries' reliance on non-regional research. Survey responses suggested that reliance on non-LAC research was high, as researchers stated they were more likely to collaborate with scientists outside of LAC than within LAC. Inclusion of non-LAC countries in the analysis of scientific interactions could present many opportunities for expanding our findings in future research. Finally, our study indicated where bright and blind spots appear across research in LAC, but did not aim to examine causal relations for these patterns, a common shortcoming in Science of Science (Fortunato et al., 2018). Survey results indicate that funding for research is an important driver influencing where bright and blind spots occur.
However, a more comprehensive answer would require exploring historical, political, economic, and social dynamics influencing the allocation of research resources. Overall, this work displays the value of our novel method to interpret results from machine learning, points to the need for a deeper and wider understanding of existing water resources research in water vulnerable regions, and warrants expanding our methods to include gray literature and coverage across the Global South.
This unprecedented multilingual literature review provides insights into bright and blind spots of water research throughout LAC. Our results reveal that the region's vulnerability to water-related stresses, and drivers such as climate change, is compounded by research blind spots in certain topics (e.g. reservoirs and risk assessment) and in entire subregions (e.g. Caribbean nations). Although certain topics and countries are under-studied in relation to the rest of the corpus, research on most components of the water budget (e.g. precipitation) represents a bright spot and suggests that most countries can make science-informed decisions regarding their water management. Research on water resources in Brazil dominates the research landscape, representing another bright spot. However, Brazil's dominance also highlights a regional vulnerability: while research on Brazil is vast, well-rounded, and highly influential across LAC, funding cuts and policy shifts that affect the country's scientific output can halt progress and impede scientifically informed water management throughout the region. Supporting societal and ecological needs while addressing challenges linked with future water-related risks will depend on countries' abilities to improve the accessibility of existing research (in English, Spanish, and Portuguese), expand research in under-studied topics (particularly in the social sciences), and harness existing opportunities for knowledge sharing.
The Kullback–Leibler divergence measures the expected information for discriminating between discrete probability distributions P and Q when only observing P (Kullback and Leibler, 1951):
The Kullback–Leibler divergence is asymmetric and, in nontrivial cases, . The Jensen–Shannon divergence is a symmetric measure of discrimination between the two probability distribution functions P and Q. It is directly related to the Kullback–Leibler divergence (Lin, 1991; Topsoe, 2000):
with the midpoint probability distribution.
Finally, the Jensen–Shannon distance, , retains the advantageous symmetric property of the Jensen–Shannon divergence but also satisfies the triangular inequality making it a proper distance metric (Endres and Schindelin, 2003). This property allows for the construction of distance matrices, which is a common tool in data analysis (e.g. correlation matrix).
Code and data are available as the R
package wateReview v0.1
archived at https://doi.org/10.5281/zenodo.4552771 (Guillon et al., 2021). Long-form documentation is also available there.
The supplement related to this article is available online at: https://doi.org/10.5194/hess-25-4631-2021-supplement.
AJD and HG contributed to this paper's conceptualization, methodology, investigation, data management, analysis, visualization, project administration, funding acquisition, and writing, including the original draft, revisions, and editing. RDG, NPK, and FvdB contributed to this paper's conceptualization, methodology, investigation, data management, analysis, visualization, and writing, including the original draft, revisions, and editing. AK contributed to this paper's methodology, investigation, data management, and writing, including revisions and editing. JPOP contributed to this paper's conceptualization, investigation, analysis, visualization, and writing, including revisions and editing. LEGD contributed to this paper's conceptualization, investigation, and writing, including the original draft, revisions, and editing. JGR contributed to this paper's investigation. EG contribution to this paper's investigation and writing, including revisions and editing. SSS contributed to this paper's conceptualization, methodology, investigation, project administration, and writing, including revisions and editing.
The authors declare that they have no conflict of interest.
Publisher's note: Copernicus Publications remains neutral with regard to jurisdictional claims in published maps and institutional affiliations.
We are indebted to Shanti Sandosham, Miranda Romero, Lilly Mccaffrey Pecher (interns at the University of California, Davis (UCD)), and Carly M. Lawyer (intern at the University of South Carolina). Pamela Reynolds and Carl Stahmer at the DataLab (UCD) provided invaluable insights. This study was supported by funds from Jastro Research Fellowship (UCD).
This research has been supported by the Jastro Research Fellowship of UC Davis.
This paper was edited by Graham Jewitt and reviewed by José Luis Arumí and one anonymous referee.
Aguilar-Barajas, I., Mahlknecht, J., Kaledin, J., Kjellén, M., and Mejía-Betancourt, A.: Water and cities in Latin America: challenges for sustainable development, Routledge, New York, 2015. a
Astudillo, P.: Manifesto for Science, Editorial: CATALONIA-Fundación Science & Life Collection ón: Science & Life, Editorial Catalonia, available at: https://books.google.com/books?hl=en&lr=&id=3SRLDwAAQBAJ&oi=fnd&pg=PT4&dq=Manifesto+by+the+science+of+Pablo+Astudillo&ots=xHbG0U69Y-&sig=ldKyg-bxJc_ZQgtCY3TOzM8FV3s#v=onepage&q=Manifesto by the science of Pablo Astudillo&f=false (last access: May 2021), 2016. a, b
Bárcena Ibarra, A., Samaniego, J., Peres, W., and Alatorre, J.: The Climate Emergency in Latin America and the Caribbean: The Path Ahead–Resignation or Action?, Economic Commission for Latin America and the Caribbean, Santiago, 2020. a
Barrat, A., Barthelemy, M., Pastor-Satorras, R., and Vespignani, A.: The architecture of complex weighted networks, P. Natl. Acad. Sci. USA, 101, 3747–3752, 2004. a
Barros, V. R., Boninsegna, J. A., Camilloni, I. A., Chidiak, M., Magrín, G. O., and Rusticucci, M.: Climate change in Argentina: trends, projections, impacts and adaptation, Wiley Interdisciplin. Rev.: Clim. Change, 6, 151–169, 2015. a
Baskaran, A.: UNESCO science report: Towards 2030, Institutions and Economies, UNESCO Publishing, Paris, 125–127, 2017. a
Bastian, M., Heymann, S., and Jacomy, M.: Gephi: an open source software for exploring and manipulating networks, in: Third international AAAI conference on weblogs and social media, 17–20 May 2009, San Jose, 2009. a
Bischl, B., Mersmann, O., Trautmann, H., and Weihs, C.: Resampling Methods for Meta-Model Validation with Recommendations for Evolutionary Computation, Evolut. Comput., 20, 249–275, https://doi.org/10.1162/evco_a_00069, 2012. a
Blei, D. M., Ng, A. Y., and Jordan, M. I.: Latent dirichlet allocation, J. Mach. Learn. Res., 3, 993–1022, 2003. a, b
Bruggeman, J., Traag, V., and Uitermark, J.: Detecting communities through network data, Am. Sociolog. Rev., 77, 1050–1063, 2012. a
Callaghan, M. W., Minx, J. C., and Forster, P. M.: A topography of climate change research, Nat. Clim. Change, 10, 118–123, 2020. a
Ciocca, D. R. and Delgado, G.: The reality of scientific research in Latin America; an insider's perspective, Cell Stress Chaperon., 22, 847–852, https://doi.org/10.1007/s12192-017-0815-8, 2017. a
Cvitanovic, C. and Hobday, A. J.: Building optimism at the environmental science-policy-practice interface through the study of bright spots, Nat. Commun., 9, 3466, https://doi.org/10.1038/s41467-018-05977-w, 2018. a
Datta, S. and Datta, S.: Comparisons and validation of statistical clustering techniques for microarray gene expression data, Bioinformatics, 19, 459–466, 2003. a, b, c
Delgado-Troncoso, J. E. and Fischman, G. E.: The future of Latin American academic journals, in: The future of the academic journal, Elsevier, Oxford, 379–400, 2014. a
DESA: United Nations, Department of Economic and Social Affairs, Population Division, World Population Prospects 2019: Highlights, United Nations, New York, 2019. a
Devlin, J., Chang, M.-W., Lee, K., and Toutanova, K.: Bert: Pre-training of deep bidirectional transformers for language understanding, arXiv: preprint, arXiv:1810.04805, 2018. a
Dobriyal, P., Qureshi, A., Badola, R., and Hussain, S. A.: A review of the methods available for estimating soil moisture and its implications for water resource management, J. Hydrol., 458, 110–117, 2012. a
Dussaillant, I., Berthier, E., Brun, F., Masiokas, M., Hugonnet, R., Favier, V., Rabatel, A., Pitte, P., and Ruiz, L.: Two decades of glacier mass loss along the Andes, Nat. Geosci., 12, 802–808, 2019. a
Endo, A., Tsurita, I., Burnett, K., and Orencio, P. M.: A review of the current state of research on the water, energy, and food nexus, J. Hydrol.: Reg. Stud., 11, 20–30, https://doi.org/10.1016/j.ejrh.2015.11.010, 2017. a
Endres, D. M. and Schindelin, J. E.: A new metric for probability distributions, IEEE T. Inform. Theor., 49, 1858–1860, https://doi.org/10.1109/TIT.2003.813506, 2003. a, b
Fehder, D., Porter, M., and Stern, S.: The empirics of social progress: The interplay between subjective well-being and societal performance, in: vol. 108, AEA Papers and Proceedings, 108, 477–482, https://doi.org/10.1257/pandp.20181036, 2018. a
Formiga-Johnsson, R. and Kemper, K.: Institutional and policy analysis of river basin management in the Alto-Tiete river basin, Sao Paulo, Brazil, Policy Research Working Paper, The World Bank, Sao Paulo, p. 2649, 2005. a
Fortunato, S., Bergstrom, C. T., Börner, K., Evans, J. A., Helbing, D., Milojević, S., Petersen, A. M., Radicchi, F., Sinatra, R., Uzzi, B., and Vespignani, A.: Science of science, Science, 359, eaao0185, https://doi.org/10.1126/science.aao0185, 2018. a, b, c
Fruchterman, T. M. and Reingold, E. M.: Graph drawing by force-directed placement, Softw.: Pract. Exper., 21, 1129–1164, 1991. a
Gesualdo, G. C., Oliveira, P. T., Rodrigues, D. B. B., and Gupta, H. V.: Assessing water security in the São Paulo metropolitan region under projected climate change, Hydrol. Earth Syst. Sci., 23, 4955–4968, https://doi.org/10.5194/hess-23-4955-2019, 2019. a
Gosling, S. N. and Arnell, N. W.: A global assessment of the impact of climate change on water scarcity, Climatic Change, 134, 371–385, 2016. a
Guillon, H., DeVincentis, A. J., Patterson, N. K., Díaz Gómez, R., and Koehl, A.: hrvg/wateReview v0.1 (v0.1), Zenodo [code and data set], https://doi.org/10.5281/zenodo.4552771, 2021. a
Hartigan, J. A. and Wong, M. A.: A K-means Clustering Algorithm, J. Roy. Stat. Soc. Ser. C, 28, 100–108, 1979. a
Immerzeel, W. W., Lutz, A. F., Andrade, M., Bahl, A., Biemans, H., Bolch, T., Hyde, S., Brumby, S., Davies, B. J., Elmore, A. C., Emmer, A., Feng, M., Fernández, A., Haritashya, U., Kargel, J. S., Koppes, M., Kraaijenbrink, P. D. A., Kulkarni, A. V., Mayewski, P. A., Pacheco, P., Painter, T. H., Pellicciotti, F., Rajaram, H., Rupper, S., Sinisalo, A., Shrestha, A. B., Viviroli, D., Wada, Y., Xiao, C., Yao, T., and Baillie, J. E. M.: Importance and vulnerability of the world's water towers, Nature, 577, 364–369, https://doi.org/10.1038/s41586-019-1822-y, 2020. a
INFORM: Latin America and Caribbean INFORM Risk Index, Tech. rep., INFORM, available at: https://drmkc.jrc.ec.europa.eu/inform-index/INFORM-Subnational-Risk/Latin-America-and-Caribbean/moduleId/1800/id/368/controller/Admin/action/Results (last access: May 2021), 2018. a
International Institute for Democracy and Electoral Assistance: The Global State of Democracy: Exploring Democracy's Resilience, International IDEA, Stromsberg, 2017. a
IPCC: Climate Change 2014: Synthesis Report, in: Contribution of Working Groups I, II, and III to the Fifth Assessment Report of the Intergovernmental Panel on Climate Change, Tech. rep., Geneva, Switzerland, 2014. a
Jacomy, M., Venturini, T., Heymann, S., and Bastian, M.: ForceAtlas2, a continuous graph layout algorithm for handy network visualization designed for the Gephi software, PloS One, 9, e98679, https://doi.org/10.1371/journal.pone.0098679, 2014. a
Jenerette, G. D. and Larsen, L.: A global perspective on changing sustainable urban water supplies, Global Planet. Change, 50, 202–211, 2006. a
Kim, H. and Grafakos, S.: Which are the factors influencing the integration of mitigation and adaptation in climate change plans in Latin American cities?, Environ. Res. Lett., 14, 105008, https://doi.org/10.1088/1748-9326/ab2f4c, 2019. a
Kullback, S. and Leibler, R. A.: On information and sufficiency, Ann. Math. Stat., 22, 79–86, https://doi.org/10.1214/aoms/1177729694, 1951. a
Larivière, V., Haustein, S., and Börner, K.: Long-distance interdisciplinarity leads to higher scientific impact, Plos One, 10, e0122565, https://doi.org/10.1371/journal.pone.0122565, 2015. a, b
Lin, J.: Divergence measures based on the Shannon entropy, IEEE T. Inform. Theor., 37, 145–151, https://doi.org/10.1109/18.61115, 1991. a, b
Lyon, S. W., Goethals, P., Schneider, P., Dominguez-Granda, L., Hampel, H., Lam, N., Nolivos, I., Reinstorf, F., Tejeda, R. C. R., Vázquez, R. F., and Ho, L.: Improving Water Management Education across the Latin America and Caribbean Region, Water, 11, 2318, https://doi.org/10.3390/w11112318, 2019. a
Madow, W. G. and Madow, L. H.: On the theory of systematic sampling, II, Ann. Math. Stat., 20, 333–354, 1949. a
Masiokas, M., Cara, L., Villalba, R., Pitte, P., Luckman, B., Toum, E., Christie, D., Le Quesne, C., and Mauget, S.: Streamflow variations across the Andes (18–55∘ S) during the instrumental era, Scient. Rep., 9, 1–13, 2019. a
Mcdonald, R. I., Weber, K., Padowski, J., Flo, M., Schneider, C., Green, P. A., Gleeson, T., Eckman, S., Montgomery, M., Lehner, B., Balk, D., and Boucher, T.: Water on an urban planet: Urbanization and the reach of urban water infrastructure, Global Environ. Change, 27, 96–105, https://doi.org/10.1016/j.gloenvcha.2014.04.022, 2014. a
Montanari, A., Bahr, J., Blöschl, G., Cai, X., Mackay, D. S., Michalak, A. M., Rajaram, H., and Sander, G.: Fifty years of Water Resources Research: Legacy and perspectives for the science of hydrology, Water Resour. Res., 51, 6797–6803, 2015. a
Murtagh, F.: A survey of recent advances in hierarchical clustering algorithms, Comput. J., 26, 354–359, 1983. a
Newman, M. E.: Scientific collaboration networks. II. Shortest paths, weighted networks, and centrality, Phys. Rev. E, 64, 016132, https://doi.org/10.1103/PhysRevE.64.016132, 2001. a
Norgaard, R. B.: Finding hope in the millennium ecosystem assessment, Conserv. Biol., 22, 862–869, 2008. a
O'dorico, P. D., Davis, K. F., Rosa, L., Carr, J. A., Chiarelli, D., Angelo, J. D., Gephart, J., Macdonald, G. K., Seekell, D. A., Suweis, S., and Rulli, M. C.: The Global Food-Energy-Water Nexus, Rev. Geophys., 56, 456–531, https://doi.org/10.1029/2017RG000591, 2018. a
Opsahl, T., Agneessens, F., and Skvoretz, J.: Node centrality in weighted networks: Generalizing degree and shortest paths, Social Netw., 32, 245–251, 2010. a
Owusu, P. A., Asumadu-Sarkodie, S., and Ameyo, P.: A review of Ghana's water resource management and the future prospect, Cogent Eng., 3, 1164275, https://doi.org/10.1080/23311916.2016.1164275, 2016. a
Paiva, R. C. D. D., Chaffe, P. L. B., Anache, J. A. A., Fontes, A. S., Araujo, L. M. N. D., Araujo, A. N. D., Bartiko, D., Bleninger, T., Amorim, P. B. D., Buarque, D. C., and Carlotto, T.: Advances and challenges in the water sciences in Brazil: a community synthesis of the XXIII Brazilian Water Resources Symposium, RBRH, 25, 27 November 2019, Foz do Iguaçu, 2020. a, b, c, d
Plummer, R., de Loë, R., and Armitage, D.: A systematic review of water vulnerability assessment tools, Water Resour. Manage., 26, 4327–4346, 2012. a
Ran, Y., Lannerstad, M., Herrero, M., Van Middelaar, C., and De Boer, I. J.: Assessing water resource use in livestock production: A review of methods, Livestock Sci., 187, 68–79, 2016. a
Rosbjerg, D. and Rodda, J.: IAHS: a brief history of hydrology, Hist. Geo-Space Sci., 10, 109–118, 2019. a
Rousseeuw, P. J.: Silhouettes: a graphical aid to the interpretation and validation of cluster analysis, J. Comput. Appl. Math., 20, 53–65, 1987. a
Schmid, H.: Improvements in part-of-speech tagging with an application to German, in: Natural language processing using very large corpora, Springer, Dordrecht, 13–25, 1999. a
Soruco, A., Vincent, C., Rabatel, A., Francou, B., Thibert, E., Sicart, J. E., and Condom, T.: Contribution of glacier runoff to water resources of La Paz city, Bolivia (16∘ S), Ann. Glaciol., 56, 147–154, 2015. a
Tonin, F. S., Borba, H. H., Mendes, A. M., Wiens, A., Fernandez-Llimos, F., and Pontarolo, R.: Description of network meta-analysis geometry: a metrics design study, PloS One, 14, e0212650, https://doi.org/10.1371/journal.pone.0212650, 2019. a
Topsoe, F.: Some inequalities for information divergence and related measures of discrimination, IEEE T. Inform. Theor., 46, 1602–1609, https://doi.org/10.1109/18.850703, 2000. a
UN-OCHA: Latin America and the Caribbean: Natural Disasters 2000–2019, UN Office for the Coordination of Humanitarian Affairs, available at: https://reliefweb.int/report/world/natural-disasters-latin-america-and-caribbean-2000-2019 (last access: May 2021), 2020. a
Uzzi, B., Mukherjee, S., Stringer, M., and Jones, B.: Atypical combinations and scientific impact, Science, 342, 468–472, 2013. a, b
Varis, O., Taka, M., and Kummu, M.: The Planet's Stressed River Basins: Too Much Pressure or too Little Adaptive Capacity?, Earth's Future, 7, 1118–1135, 2019. a
Wendling, Z. A., Emerson, J. W., Esty, D. C., Levy, M. A., and De Sherbinin, A.: Environmental Performance Index, Yale Center for Environmental Law & Policy, New Haven, CT, 2018. a
Yeung, K. Y., Haynor, D. R., and Ruzzo, W. L.: Validating clustering for gene expression data, Bioinformatics, 17, 309–318, 2001. a
Zaninelli, P. G., Menéndez, C. G., Falco, M., López-Franca, N., and Carril, A. F.: Future hydroclimatological changes in South America based on an ensemble of regional climate models, Clim. Dynam., 52, 819–830, https://doi.org/10.1007/s00382-018-4225-0, 2019. a