the Creative Commons Attribution 4.0 License.
the Creative Commons Attribution 4.0 License.
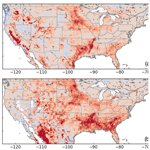
Assimilation of vegetation optical depth retrievals from passive microwave radiometry
Sujay V. Kumar
Thomas R. Holmes
Rajat Bindlish
Richard de Jeu
Christa Peters-Lidard
Vegetation optical depth (VOD) retrievals from passive microwave sensors provide analog estimates of above-ground canopy biomass. This study presents the development and analysis of assimilating VOD retrievals from X-, C-, and L-band passive microwave instruments within the Noah-MP land surface model over the Continental U.S. The results from this study demonstrate that the assimilation of VOD retrievals have a significant beneficial impact on the simulation of evapotranspiration and GPP, particularly over the agricultural areas of the U.S. The improvements in the water and carbon fluxes from the assimilation of VOD from X- and C-band sensors are found to be comparable to those obtained from the assimilation of vegetation indices from optical sensors. The study also quantifies the relative and joint impacts of assimilating surface soil moisture and VOD from the Soil Moisture Active Passive (SMAP) mission. The utility of soil moisture assimilation for improving evapotranspiration (ET) is more significant over water-limited regions, whereas VOD DA is more impactful over areas where soil moisture is not the primary controlling factor on ET. The results also indicate that the information on moisture and vegetation states from SMAP can be simultaneously exploited through the joint assimilation of surface soil moisture and VOD. Since passive microwave-based VOD retrievals are available in nearly all weather conditions, their use within data assimilation systems offers the ability to extend and improve the utility obtained from the use of optical/infrared-based vegetation retrievals.
- Article
(2550 KB) - Full-text XML
- BibTeX
- EndNote
Remote sensing estimates of vegetation are typically developed by exploiting the relationship between the stomatal stress and the spectral reflectance of leaves and canopies (Knipling, 1970). Multi-spectral and hyperspectral optical and thermal satellite sensors have been used to provide retrievals of variables such as Leaf Area Index (LAI), Normalized Difference Vegetation Index (NDVI), fraction of photosynthetically active radiation (fPAR), solar-induced fluorescence (SIF), and biomass (Myneni et al., 2002; Tucker et al., 2005; Zheng and Moskal, 2009; Myneni et al., 2011; Kumar and Mutanga, 2017). The multi-spectral vegetation indices are typically derived from atmospherically corrected bidirectional surface reflectance in the near-infrared and visible bands (Price and Bausch, 1995; Huete et al., 1997). Similarly, hyperspectral imaging is used to characterize vegetation type, health, and function (Goetz et al., 1985) at very fine (∼30 m) spatial resolution. As vegetation stress and stomatal closure influence canopy temperatures, thermal remote sensing also offers the possibility of estimating vegetation conditions. For example, Landsat (Anderson et al., 2012) and the ECOsystem Spaceborne Thermal Radiometer Experiment on Space Station (ECOSTRESS; https://ecostress.jpl.nasa.gov/, last access: 15 December 2019) provide fine-resolution (∼70–100 m) estimates of surface temperature and evapotranspiration. Houborg et al. (2015) present a summary of the major advances in the remote sensing of vegetation from these platforms.
A significant shortcoming of the optical/thermal infrared (TIR) sensors is that cloud cover can severely limit the acquisition of data, restricting the coverage to cloud-free, clear days. Gap-filling strategies, such as using the nearest clear-day observation, are often used to improve the cloud-related gaps in spatio-temporal coverage from optical/TIR instruments (Hall et al., 2010). Passive microwave measurements, on the other hand, are nearly all-weather and are not limited by cloud cover. Holmes et al. (2016), for example, used microwave estimates of land surface temperature as an alternative to TIR measurements to retrieve evapotranspiration (ET) during cloudy time periods. Microwave radiometry over land has traditionally been used for retrieving estimates of surface soil moisture by exploiting the sensitivity to low-frequency microwave radiometric measurements to changes in soil moisture (Njoku and Entekhabi, 1996). As this radiance passes through vegetation, the microwave signal is attenuated by vegetation, the level of which is described by the vegetation optical depth (VOD) parameter. Due to its sensitivity to plant water content, VOD can be used as an analog of above-ground canopy biomass (Owe et al., 2001; Y. Y. Liu et al., 2011; Konings et al., 2016, 2019; Teubner et al., 2018, 2019). The VOD retrievals from various microwave frequencies such as the K-, X-, C- and L-bands have been used for a variety of studies for examining vegetation seasonality (Jones et al., 2012), characterization of extremes such as drought (Liu et al., 2015; Konings and Gentine, 2017; Smith et al., 2020), assessment of dryland vegetation dynamics (Andela et al., 2013), and the determination of land degradation and deforestation (Liu et al., 2013; van Marle et al., 2016).
Despite the availability of vegetation measurements from various sensing platforms, the incorporation of these measurements within data assimilation systems for land surface hydrology is relatively new. Most studies to date have focused on the assimilation of LAI retrievals to improve the characterization of vegetation biomass, evapotranspiration, root zone soil moisture, and CO2 fluxes within land surface models (Sabater et al., 2008; Barbu et al., 2011, 2014; Albergel et al., 2017, 2018; Fox et al., 2018). More recently, Kumar et al. (2019b) demonstrated the beneficial impact of LAI assimilation on improving water, energy, and carbon fluxes over the Continental U.S. (CONUS). Most prominent improvements from LAI assimilation are observed over the agricultural areas, where assimilation improved the representation of vegetation seasonality impacted by cropping schedules.
As the use of all-weather VOD measurements from microwave sensors provides the opportunity to extend the spatial and temporal coverage of vegetation observations into overcast and clouded conditions, here we examine the influence of assimilating VOD retrievals from microwave radiometry. Specifically, we explore the utility of assimilating VOD retrievals from X-, C-, and L-band microwave sensors in the Noah multi-parameterization (Noah-MP) land surface model (LSM). The study uses VOD retrievals from a range of microwave frequencies, as their current and future availabilities vary significantly. For example, the L-band sensing platforms such as NASA's Soil Moisture Active Passive (SMAP; Entekhabi et al., 2010) mission are relatively new, whereas the X-band and C-band retrievals of VOD are available for significantly longer time records, with observations from multiple satellites. In addition, given the plans for sensors operating in the higher microwave frequencies (e.g., Advanced Microwave Scanning Radiometer, AMSR; the Global Precipitation Measurement Microwave Imager, GMI; Joint Polar Satellite System, JPSS-2; Copernicus Imaging Microwave Radiometer, CIMR), future observations in X- and C-band frequencies are also likely guaranteed. Quantifying the relative utility of VOD retrievals in these frequencies is, therefore, important. The model simulations are conducted over the CONUS in the North American Land Data Assimilation System phase-2 (NLDAS-2; Xia et al., 2012) configuration. As noted in prior data assimilation studies such as Kumar et al. (2019b), the NLDAS-2 configuration provides an environment with high-quality boundary conditions informed by radar- and gauge-corrected precipitation and bias-corrected shortwave radiation, which also leads to high skill in the simulated land surface conditions. Partly as a result of the high skill of the NLDAS-2 meteorology, data assimilation of variables such as soil moisture and snow has only reported marginal success in this configuration (Kumar et al., 2014). The assimilation of LAI, on the other hand, has been more impactful as it was shown to detect impacts of agricultural activity, which is not easily captured through high-quality boundary conditions alone (Kumar et al., 2019b). In general, demonstration of additional improvements through data assimilation in the NLDAS-2 configuration is indicative of the significant utility of remote sensing inputs, as such high-quality boundary conditions are not routinely available in other regions of the world.
As described in detail in Konings et al. (2017), a number of approaches have been used to retrieve VOD from microwave sensors. Here we employ VOD retrievals primarily from two approaches for data assimilation. The Land Parameter Retrieval Model (LPRM; Owe et al., 2008) uses single-frequency, polarized brightness temperature in the range of 1–20 GHz to retrieve both soil moisture and VOD. In this study, we use the C-band (6.9 GHz) and X-band (10.7 GHz) based VOD retrievals from the LPRM. The C- and X-band measurements are less sensitive to cloud water content and more sensitive to soil moisture and vegetation canopy, which are also prone to radio frequency interference (RFI). NASA's SMAP mission operates in a protected L-band over the U.S., which minimizes the impact of RFI contamination. The sensitivity of L-band to cloud water content is lower compared to the C- and X-bands. In addition, the L-band measurements provide more sensitivity to deeper soil moisture and canopy layers.
To our knowledge, this is one of the first reported studies of continental-scale assimilation of VOD retrievals within LSMs. Specifically, this article addresses the following research questions.
-
What is the impact of assimilating VOD retrievals from X-, C-, and L-band passive microwave remote sensing instruments on water and carbon states?
-
How does the utility of passive microwave VOD assimilation compare to that of assimilating vegetation (LAI) retrievals from optical instruments?
-
Does assimilating L-band VOD provide independent benefits to that from incorporating surface soil moisture retrievals? Can improved simulation of water and carbon states be developed from the simultaneous use of VOD and soil moisture?
These questions are addressed by examining the impact of assimilation with the use of a large suite of independent reference datasets of soil moisture, evapotranspiration, gross primary productivity (GPP), streamflow, and terrestrial water storage (TWS). Section 2 describes the details of the model configuration, datasets used, and assimilation configuration. The results of various data assimilation simulations are described in Sect. 3. Finally, Sect. 4 summarizes the main findings of the study.
2.1 Data
VOD, an integrated measure of the vegetation structure and water content, is typically estimated as part of the radiometric soil moisture retrieval approach based on the first-order τ-ω model (Mo et al., 1982). In this model, the L-band brightness temperature (Tb,p) estimates at the top of the atmosphere for horizontal and vertical polarizations (denoted by the subscript p) are represented as
where Ts is the surface soil temperature, Tc is the canopy temperature, rp is the rough surface reflectivity, ωp is the scattering albedo, and γ is the vegetation transmissivity. γ, which represents the attenuation of the emission due to vegetation, is a function of VOD and the measurement incidence angle θ.
VOD is determined by the canopy structure and the dielectric properties of the canopy layer. When VOD is low (∼0), the attenuation of the microwave signal is small. Soil moisture is estimated from rp using Fresnel equations that relate rp to the dielectric constant of the soil. A more detailed description of the VOD formulation is provided in Grant et al. (2016).
As mentioned earlier, the X- and C-band-based VOD datasets used in this study are based on LPRM to retrieve VOD and soil moisture from dual-polarized passive microwave observations. The LPRM uses the τ-ω model to characterize the emission and radiative transfer of low-frequency (1–20 GHz) microwave emission from the soil, vegetation, and atmosphere to the top-of-atmosphere brightness temperature recorded by the satellite. Unique to the LPRM, the method includes the analytical solution of the τ-ω model for polarized emission that describes the relationship between the microwave polarization difference ratio (MPDI) and VOD (Meesters et al., 2005). Within the framework of the τ-ω model, this allows for the retrieval of both VOD and soil moisture and has been implemented with all existing passive microwave satellites with frequencies from the L- to Ku-bands and from 1979 to present (Owe et al., 2008; Parinussa et al., 2011; der Schalie et al., 2016). The spatial resolution of this product is 0.25∘ with a global extent of the non-frozen land surface. The temporal resolution is 1–2 d for the morning overpass. In this study, we employ the VOD retrievals from LPRM version 6 (Van der Schalie et al., 2018), available from the VOD climate archive (VODCA; Moesinger et al., 2020). VODCA provides products from multiple sensors, including the Advanced Microwave Scanning Radiometer – Earth observing system (AMSR-E) aboard NASA's Aqua satellite, the AMSR2 instrument onboard the Global Change Observation Mission-Water (GCOM-W), the WindSat microwave radiometer aboard the joint DoD/Navy Coriolis platform, the Tropical Rainfall Measuring Mission's (TRMM) Microwave Imager (TMI), and the Global Precipitation Measurement (GPM) Microwave Imager (GMI). The C-band VOD retrievals rely on AMSR-E, AMSR2, and WindSat, whereas the X-band VOD retrievals include data from AMSR-E, AMSR2, WindSat, TMI, and GMI.
The SMAP satellite launched in January 2015 is a mission dedicated to measuring soil moisture and freeze/thaw states, employing a passive microwave radiometer to collect measurements of vertical and horizontal polarizations of L-band brightness temperature data at an incident angle of 40∘. The retrievals from SMAP are also developed using the τ-ω model. The soil moisture retrievals are made using a single-channel algorithm using the vertical polarizations (Chan et al., 2018), whereas the VOD retrievals employ both polarized brightness temperature observations (Chaubell et al., 2020). Though the sampling resolution of the SMAP radiometer is approximately 36 km, oversampling of the antenna overpasses is used to enhance the spatial resolution to 9 km. This 9 km, level 2 SMAP dataset (SPL2SMP_E) is used in this study.
2.2 Model configuration
The model domain used in this study covers the CONUS with an extent of 25–53∘ N and 125–67∘ W at 1∕8∘ spatial resolution (Fig. 1). Hourly NLDAS-2 meteorological inputs are used to drive the Noah-MP land surface model (version 3.6), which is the next generation version of the Noah LSM. Compared to Noah, Noah-MP provides multiple options for various land surface physics computations, including multilayer snowpack, options for surface water infiltration, runoff, and groundwater, representation of an unconfined groundwater aquifer, and a dynamic vegetation model (Niu et al., 2011; Yang et al., 2011). Note that the prognostic vegetation model of Noah-MP v3.6 was used by Kumar et al. (2019b) to demonstrate the impact of assimilating LAI retrievals from the Moderate Resolution Imaging Spectroradiometer (MODIS) aboard the Terra and Aqua satellites. In addition to Noah-MP, the Hydrological Modeling and Analysis Platform (HyMAP; Getirana et al., 2012) model is used to develop estimates of routed streamflow using the gridded surface runoff and baseflow fields from Noah-MP. In this study, the impacts of regulation and reservoir operations on streamflow are not modeled within HyMAP.
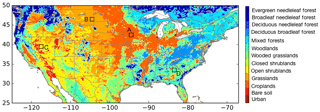
Figure 1Map of the modeling domain with the UMD landcover classification as the background. The locations A, B, C, and D denote the areas used for time series comparisons to examine the impact from VOD DA.
The model and data assimilation integrations in this study are conducted during a time period of 2000 to 2018. The initial conditions are generated through a long spinup of Noah-MP. The model is initialized with uniform conditions and is run from 1979 to 2018 twice. It is then reinitialized in 1979 with the climatological average conditions derived from the spinup. Finally, the initial conditions at the beginning of year 2000 are used for the model simulations in this article.
The NASA Land Information System (LIS; Kumar et al., 2006) is used to facilitate the model simulations presented in this article. LIS is a comprehensive land surface modeling system that includes the interoperable support for a large suite of land surface models, data assimilation algorithms, and observational data sources. As part of this study, the DA capabilities in LIS are extended to enable the assimilation of VOD retrievals, described in Sect. 2.3. The LIS framework also includes a verification system known as the Land surface Verification Toolkit (LVT; Kumar et al., 2012), enabling the systematic verification and evaluation of modeled land surface states against independent measurements and datasets. LVT-based evaluations are used in this study to assess the utility of VOD assimilation approaches.
2.3 Data assimilation configuration
Similarly to the assimilation strategy employed in Kumar et al. (2019b), a 1D ensemble Kaman filter (EnKF; Reichle et al., 2002) method is used for the assimilation of VOD retrievals. The EnKF algorithm works with an ensemble of model states, which is propagated forward in time using the LSM and updated toward the observation based on the relative uncertainty of the model states and the observation. The model state update at time k is represented by the following equation:
where and represent the model state for the ith ensemble member before and after the update, respectively. The observation vector is represented by yk, which is connected to the model states through the observation operator Hk. The relative weight given to the innovations in the analysis update is determined by the Kalman gain term (Kk). In this study, the innovation calculations employ observations interpolated to the model grid using a nearest neighbor approach.
As described in Kumar et al. (2019b), the innovations in the LAI DA configuration are specified by comparing the model prognostic LAI variable with the observations. The yk in this case is the remotely sensed LAI and is the model's LAI estimate. In the case of VOD assimilation, the computation of the innovations is tricky as Noah-MP does not directly estimate VOD within the model. To overcome this limitation, the VOD observations are rescaled into the LAI space in the data assimilation configuration. The rescaling is performed using a seasonally varying CDF matching (Kumar et al., 2015) and by using the MODIS-based LAI observations from the Global Land Cover Facility (GLCF) Global LAnd Surface Satellites (GLASS; Xiao et al., 2016) project at the University of Maryland as the LAI reference. The MODIS-based LAI retrievals from the GLASS LAI product are generated using a general regression neural network approach, enabling a spatially and temporally continuous record of LAI available at 8 d intervals on a 0.05∘ regular latitude–longitude global grid. We use GLASS data as the LAI reference, due to the improved spatio-temporal coverage as well as the high quality of the product established in intercomparison studies (Liao et al., 2012; Fang et al., 2013; Xiao et al., 2016). Monthly CDFs using multi-year information are computed for both the VOD and LAI datasets using all available data, at every model grid point. For example, the LPRM X-band and C-band CDFs are computed using datasets from 2002 to 2018, whereas SMAP CDFs are computed using the available data from 2015 to 2019. To increase the sampling density in the SMAP CDF calculations, a spatial sampling window of 2 pixels is employed. The GLASS LAI CDFs are computed using a time period of 2000 to 2018. Note that the rescaling strategy used here also relies on the fact that the systematic errors between the GLASS LAI data and the NoahMP LAI are small, as demonstrated in Kumar et al. (2019b). In this prior study when GLASS LAI retrievals were assimilated within NoahMP, the demonstrated improvements were primarily from the adjustment of vegetation/crop seasonality, rather than from the correction of systematic errors. In addition, the positive impacts from the use of this strategy shown in the following sections further confirm that this rescaling approach is reasonable.
The rescaling is performed with the assumption that there is a strong correlation between VOD and LAI. The use of VOD as an analog to existing vegetation measurements such as optical-infrared indices and fluorescence has been suggested in prior studies (Konings et al., 2017). For example, Albergel et al. (2018) demonstrated that the modeled LAI and VOD derived from the C-band backscatter measurements from the ASCAT sensor (Vreugdenhil et al., 2016, 2017) had high correlations over most of the CONUS. Figure 2 presents a similar comparison, where maps of the correlation of X-, C-, and L-band retrievals of VOD against the MODIS-based LAI retrievals from the GLASS project are shown. Based on the mutual availability of datasets, the correlation maps are generated using time periods of 2002–2018 and 2015–2018 for the LPRM and SMAP comparisons, respectively. Strong correlations are observed in the LPRM X-band VOD vs. LAI comparisons in most parts of the domain except over the arid, southwestern region of the U.S. The agreements between the LPRM X-band VOD and LAI are particularly strong over the eastern U.S., agricultural areas of the Midwest, and central California valley, which are regions of high vegetation density. The level of agreement between VOD and LAI is weaker in the C-band and L-band comparisons compared to the X-band. This is consistent with the fact that the attenuation of the lower-frequency measurements from vegetation is less compared to that for the X-band. The documented influence of RFI contamination over the CONUS (Njoku et al., 2005) is also evident in the C-band comparisons. Interestingly, the SMAP-based L-band retrievals of VOD show stronger correlations with LAI than those from the C-band, particularly in the eastern U.S. This is likely a function of data from different sensing platforms, the use of different retrieval algorithms, and different data record lengths. As documented in prior studies, the high-frequency VOD measurements are more sensitive to the top of the vegetation (Konings et al., 2017). The L-band measurements, on the other hand, are more representative of the vegetation changes in the deeper layers of the canopy. The strong relationship between VOD and LAI observed in Fig. 2 confirms that the rescaling procedure used in the DA configuration is reasonable.
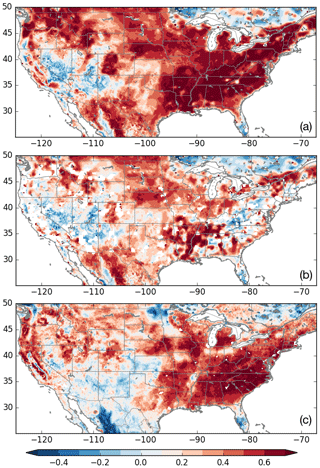
Figure 2Correlation of VOD retrievals from LPRM X-band (a), LPRM C-band (b), and SMAP L-band (c) against the MODIS-based LAI retrievals. The LPRM and SMAP comparisons employ data during the 2002–2018 and 2015–2018 time periods, respectively.
This article also compares and contrasts the impact of assimilating VOD with that from incorporating soil moisture retrievals from the L-band microwave instruments. Soil moisture in the LSMs is a model-specific quantity, an index of the moisture state (Koster et al., 2009). As a result, there are significant differences in soil moisture estimates from different LSMs, even when forced with the same meteorology and land surface parameters (Dirmeyer et al., 2006). Similarly, remote-sensing-based estimates of soil moisture are also indirect measurements generated through a retrieval model from direct measurements of the microwave emission of the land surface. Therefore, direct assimilation of soil moisture without resolving these inconsistencies is meaningless. Here we apply the commonly used strategy of CDF matching (Reichle and Koster, 2004) to address the relative differences between the remote sensing and LSM-based soil moisture by rescaling the soil moisture retrievals into the LSM climatology before assimilation. The CDFs are computed separately at each grid point on a monthly basis. Note that such a configuration only incorporates the anomaly information into the soil moisture retrievals and ignores the information inherent in the mean soil moisture signals (Kumar et al., 2015). Similarly to the strategy used in prior studies, soil moisture retrievals are excluded near water bodies, for being at the edge of the swath, when soil is frozen/covered by snow, and when the vegetation cover is thick (Kumar et al., 2019a), to account for the known limitations of passive microwave-based soil moisture retrievals. Similar flags except for thick vegetation are also applied to screen out VOD retrievals.
An ensemble size of 20 is used in the data assimilation integrations, with perturbations applied to a number of meteorological fields and the model state vector to develop representations of model uncertainty. Based on the settings used in recent DA studies in the NLDAS-2 configuration (Kumar et al., 2019a, b), the precipitation (P) and downward shortwave radiation (SW) fields are perturbed with multiplicative perturbations with a mean of 1 and standard deviations of 0.3 and 0.5, respectively. Further, additive perturbations with mean zero and standard deviation of 50 W m−2 are applied to the downward longwave radiation (LW) fields. The hourly forcing perturbations also include cross-correlations (ρ) between the forcing variables, with values of , , and . For VOD DA, additive perturbations with a standard deviation of 0.01 are applied to the model LAI fields (Kumar et al., 2019b), every 3 h. The updated LAI from DA is divided by the specific leaf area to revise the leaf biomass variable within Noah-MP. The state vector used in the soil moisture DA consists of the top soil moisture layer of Noah-MP, which is perturbed with an additive noise of 0.02 m3 m−3, applied every 3 h. The perturbations also include time series correlations employed through a first-order autoregressive (AR(1)) model with timescales of 24 and 3 h, for the forcing and model state variables, respectively. The input observation error standard deviation is set to 0.04 m3 m−3 for assimilating SMAP soil moisture retrievals, whereas the observation error standard deviation is set to 0.05 for the scaled VOD retrievals, based on settings from recent studies employing soil moisture (Kumar et al., 2019a) and LAI (Kumar et al., 2019b) retrievals. The assimilation of each dataset is performed in a sequential manner, based on their respective measurement or overpass times.
This section presents an evaluation of the impact of assimilating VOD retrievals on key terrestrial water and carbon states and fluxes. The impact of assimilating the X-band and C-band VOD retrievals is presented first, followed by the evaluation of assimilating L-band VOD retrievals from SMAP. Since soil moisture is typically considered the primary retrieval from microwave remote sensing, we also evaluate the relative benefits of assimilating both SMAP surface soil moisture and VOD retrievals. The impact of DA is quantified by comparing to a large suite of reference measurements of soil moisture, evapotranspiration, GPP, and streamflow.
3.1 Impact of assimilating X-band and C-band VOD retrievals
The impact of assimilating VOD retrievals on the simulated ET estimates is shown in Fig. 3, which shows the change in RMSE and correlation (R) of ET in the DA simulation relative to the OL. These evaluation metrics are computed using two reference data products: (1) the gridded 0.5∘, monthly FLUXNET multi-tree-ensemble (MTE) product based on tower ET measurements (Jung et al., 2009); available from 1982 to 2008) and (2) the 4 km, daily Atmosphere-Land Exchange Inverse (ALEXI; Anderson et al., 2007) model product, developed using TIR measurements, available from 2001 onwards. Strictly speaking, ALEXI is a model product with associated biases and errors of its own. Comparatively, FLUXNET MTE can be considered a close analog to a true ground-reference product, since it is derived by empirically upscaling eddy covariance measurements, though it is also affected by the sampling density and consistency of site measurements. Therefore, RMSE is used as the metric of evaluation in the FLUXNET MTE comparison, whereas R is used to assess the improvements in ET from DA relative to ALEXI. Figure 3 indicates that the assimilation of VOD generally provides beneficial impacts on ET, consistently in the comparisons against both reference datasets. In addition, most prominent improvements are obtained over the agricultural areas over the Midwest U.S., lower Mississippi basin, the central California valley, and parts of Mexico. Prior studies have documented that ALEXI is particularly skillful in detecting spatial features from agricultural management impacts (Hain et al., 2015). The fact that the spatial pattern of improvements in ET in Fig. 3 is well correlated with the crop areas provides added confirmation that VOD assimilation is helpful in improving the representation of managed vegetation (as noted in Kumar et al., 2019b).
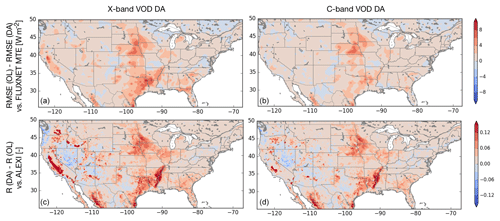
Figure 3RMSE (W m−2) or R (–) differences of evapotranspiration from X-band VOD (a, c) and C-band VOD (b, d) assimilation relative to the OL integration, using two reference datasets (FLUXNET MTE used in the top row and ALEXI in the bottom row). The time periods in the comparisons are 2000–2008 and 2000–2018, for FLUXNET MTE and ALEXI, respectively. In each plot, the warm and cool colors represent the improvement and degradation due to VOD DA, respectively.
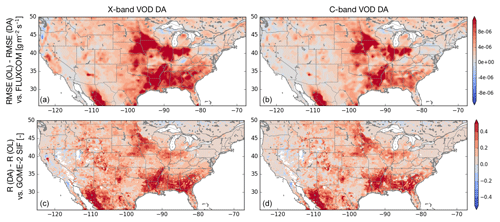
Figure 4Changes in RMSE of GPP (expressed as RMSE(OL) − RMSE (DA)) in units of g m−2s−1 using the FLUXCOM data as the reference (a, b) and R of modeled GPP with solar-induced fluorescence data from GOME-2 (c, d), expressed as R (DA) −R (OL). The warm colors represent improvements from DA and cool colors represent degradations resulting from DA.
The impact of VOD assimilation on the carbon fluxes is assessed by focusing on GPP, which represents the total carbon fixation through photosynthesis. The model-simulated GPP is compared against two datasets: (1) gridded 0.5∘ estimates of GPP from the FLUXCOM project (Tramontana et al., 2016; Jung et al., 2017) and (2) remote sensing retrievals of solar-induced fluorescence (SIF) from the Global Ozone Monitoring Experiment-2 (GOME-2) aboard the MetOp-A satellite (Joiner et al., 2014; Guanter et al., 2014). Similarly to FLUXNET MTE, the FLUXCOM estimates are produced by upscaling point measurements using machine learning approaches. SIF, which is a measure of the re-emission of light during photosynthesis, is considered an observational analog of GPP. Figure 4 provides an evaluation of GPP against these two reference datasets using two different metrics. Compared to FLUXCOM, the improvements in RMSE from the X-band and C-band VOD are shown in Fig. 4a and b. Figure 4c and d show the improvements in correlation (R) of GPP against the GOME-2 SIF measurements from VOD DA. These independent comparisons against two different products further confirm the beneficial role of VOD DA over the agricultural regions, similar to the patterns in the ET comparisons of Fig. 3. The RMSE of simulated GPP is reduced and the correlation with the SIF retrievals is improved through the assimilation of VOD.
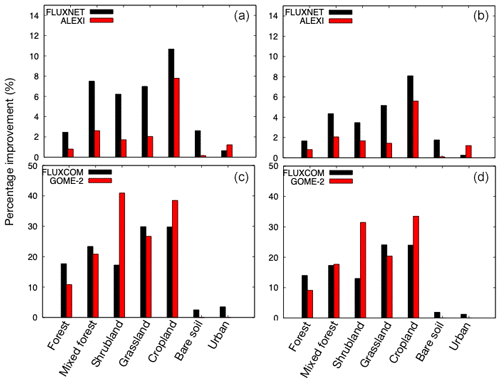
Figure 5Domain-averaged percentage improvements in ET (a, b) and GPP (c, d) stratified by vegetation type. Panels (a, c) represent the impact of DA from X-band VOD, whereas panels (b, d) represent the impact of C-band VOD DA. The percentage improvements in ET using the FLUXNET and ALEXI reference datasets are expressed for the RMSE and R metrics, respectively. Similarly, the percentage improvements in GPP using FLUXCOM and GOME-2 SIF are for the RMSE and R metrics, respectively.
Figures 3 and 4 also offer assessments of the relative utility of X-band and C-band VOD retrievals. While the spatial patterns of improvements are generally similar in both X- and C-band assimilation configurations, the assimilation of X-band VOD provides stronger improvements. This is consistent with the fact that the attenuation of the microwave signal reduces for lower-frequency measurements. As both Figs. 3 and 4 indicate the strong influence of vegetation type in the improvement maps of ET and GPP, we quantify the domain-averaged percentage improvements by vegetation type, shown in Fig. 5. For simplicity, we use a simpler vegetation classification scheme by grouping evergreen needleleaf, broadleaf needleleaf, deciduous needleleaf, and deciduous broadleaf forests into a “Forest” category, the mixed forests, woodlands, and wooded grasslands into a “Mixed forests” category, and closed shrublands and open shrublands into a “Shrublands” category. Note also that the percentage improvements shown in Fig. 5 are for different metrics. For FLUXNET and FLUXCOM comparisons, the percentage improvements are shown for RMSE, whereas for ALEXI and GOME-2, the percentage improvements in R are shown. Figure 5 confirms that the largest impact of VOD assimilation is over croplands, providing domain-averaged improvements of up to 10 % and 38 % in ET and GPP, respectively. Significant improvements are also observed over areas with moderate vegetation such as grasslands and shrublands, while over forests and mixed forests, the level of improvements reduces. Over bare soil and urban areas, the impact of VOD assimilation is very small, due to the lack of vegetation influence on ET and GPP. As seen in Fig. 5, compared to X-band VOD-DA, the level of improvements with C-band VOD-DA reduces. For ET, at a domain-averaged scale, the assimilation of C-band and X-band VOD retrievals provides 4.6 % and 6.8 % improvements in RMSE, respectively, when compared to FLUXNET MTE. Compared to ALEXI, C-band VOD DA provides 3.1 (2.0) % domain-wide improvements in RMSE (R) of ET, respectively. These percentage improvements in RMSE (R) increase to 4.0 (2.7) % for X-band VOD assimilation. Similarly, the domain-averaged percentage improvement in RMSE of GPP with C-band VOD assimilation is 17.3, and it improves to 22.3 with X-band VOD assimilation. The domain-averaged correlation of the OL-based GPP with GOME-2 SIF is 0.53, and it improves to 0.62 and 0.66 with C-band and X-band assimilation, respectively.
The impact of VOD assimilation on other land surface states such as soil moisture, terrestrial water storage, and streamflow is also evaluated using a number of reference products. The in situ measurements from the International Soil Moisture Network (ISMN; Hollinger and Isard, 1994; Jackson et al., 2010; Dorigo et al., 2011, 2013) are used for evaluating soil moisture fields. Similarly to the Kumar et al. (2019b) study, hourly data from 934 stations from nine different networks within ISMN are used for evaluating the soil moisture estimates. The surface and root zone soil moisture values are defined as the soil moisture content of the top 10 cm and 1 m of the soil column, respectively. These are computed from the layer soil moisture values as suitably weighted vertical averages based on the thickness of the soil layers. As it is well known that model-simulated soil moisture and in situ measurements are significantly biased relative to each other, the soil moisture evaluations are performed using the anomaly correlation (R) metric. The anomaly R value at each grid point is computed based on daily soil moisture anomalies (of model and in situ observations) calculated by subtracting the multi-year monthly mean values from the daily averages. The surface and root zone soil moisture anomalies are computed as the differences between the daily soil moisture and the respective monthly mean values.
Overall, VOD assimilation has marginal impacts on the simulated soil moisture estimates. The domain-averaged anomaly R values for the OL surface and root zone soil moisture are 0.54 and 0.47, respectively. With the C-band assimilation, these values marginally improve to 0.55 and 0.48, respectively. Similarly, the X-band assimilation also leads to domain-averaged anomaly R values of 0.55 for surface soil moisture and 0.49 for root zone soil moisture. Though these domain-averaged changes from assimilation are not statistically significant, there are larger regional improvements, particularly for the root zone estimates. Notably, regional improvements are observed over the Central Plains and the lower Mississippi regions (not shown), consistent with the spatial patterns seen in the ET and GPP evaluations.
The impact of VOD assimilation on streamflow is evaluated by comparing to the U.S. Geological Survey (USGS) daily gauge measurements at locations minimally impacted by reservoir operations (Kumar et al., 2014, 2019b). The impact of DA is quantified using the normalized information contribution (NIC) metric on Nash–Sutcliffe efficiency (NSE) of streamflow (Kumar et al., 2014), with positive and negative NIC values indicating benefit and degradation from assimilation, respectively. Overall, there is a small but beneficial impact from VOD assimilation on streamflow. The domain-averaged NIC improvements from X-band and C-band VOD DA are 0.03 and 0.02, respectively, with larger improvements noticed over the agricultural areas of the Midwest U.S.
Finally, the simulated TWS anomalies are also evaluated against the Gravity Recovery and Climate Experiment (GRACE) satellite-based Tellus product (http://grace.jpl.nasa.gov/data/get-data/jpl_global_mascons/, last access: 15 December 2019), available on 1∘ horizontal resolution grids (Landerer and Swenson, 2012), during the lifespan of the mission (2003–2017). The domain-averaged anomaly R for the OL-based TWS is 0.45, and it improves to 0.48 with C-band and X-band VOD assimilation. These improvements are statistically significant. In addition, larger improvements in anomaly R (as high as ∼0.28) are observed over the agricultural areas of the Central Plains and central California (not shown).
3.2 Comparing assimilation of optical sensor-based LAI and passive microwave-based VOD
The impact of passive microwave-based VOD assimilation relative to assimilating LAI retrievals from optical instruments is presented in Table 1. The percentage improvements in various terrestrial water and carbon components against reference datasets, from the assimilation of MODIS LAI (from the Kumar et al., 2019b study) and the X- and C-band VOD retrievals, are presented in this table. Note that both Kumar et al. (2019b) and the current study use the exact same model configuration, land surface parameters, and boundary conditions. Overall, the magnitude of improvements from VOD assimilation is comparable to that of assimilating LAI. There is a marginal improvement in the aggregate soil moisture skill, where the domain-averaged anomaly R values for both surface and root zone soil moisture are improved from LAI and VOD assimilation. VOD and LAI assimilation improves ET estimates, with the percentage improvements in RMSE ranging from 3 % to 7 %, depending on the reference dataset used. Overall, comparable improvements in ET are obtained with X-band VOD DA and LAI DA, with ET estimates from C-band VOD DA being marginally less skillful than those from LAI DA. LAI and VOD assimilation provides significant improvements (with approximately 17 %–24 % domain-averaged improvements) in GPP. Similarly to the changes in soil moisture, marginal improvements in TWS and streamflow are obtained from both VOD and LAI assimilation. Note that though the magnitude of added improvements is small for certain variables, larger regional improvements are observed in these comparisons.
Table 1Comparison of the percentage improvements in domain-averaged skill metrics (relative to the model OL) for DA configurations that assimilate MODIS LAI (from Kumar et al., 2019b) and those that employ X- and C-band VOD retrievals, for different variables. SFSM – surface soil moisture, RZSM – root zone soil moisture, ET – evapotranspiration, GPP – gross primary productivity, TWS – terrestrial water storage, SF – streamflow.
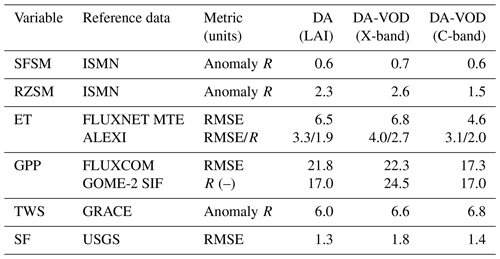
Overall, the comparison in Table 1 confirms that VOD DA is an effective option for incorporating remote sensing-based inputs of vegetation conditions. Note that the spatial resolution of passive microwave retrievals is typically coarser than those from the optical/IR sensors. In addition, passive microwave measurements are only available from low-earth orbits (LEOs) due to the antenna size requirements, so they cannot provide the diurnal view as available for optical/IR instruments from geostationary satellites. Since the results suggest that assimilation of passive microwave-based VOD retrievals provides comparable skill to that from optical sensor-based LAI, assimilation of both types of datasets will allow the sensing, coverage, and spatial resolution-based limitations of each sensor to be minimized.
3.3 Impact of assimilating L-band VOD retrievals from SMAP
In this section, the impact of assimilating L-band VOD retrievals from SMAP is evaluated and is contrasted with corresponding improvements obtained with higher-frequency VOD assimilation. As SMAP data availability is limited to April 2015–present, all evaluations in this section are limited to April 2015–December 2018. Note that not all reference datasets used in Sect. 3.1 are available during this limited time period.
Figure 6 quantifies the impact of assimilating L-band VOD retrievals from SMAP on ET and GPP. Similarly to the results seen with the X- and C-band VOD assimilation, SMAP VOD DA also provides systematic improvements in the simulated ET and GPP, comparable to those from X-band VOD assimilation. The patterns of improvements in ET in the ALEXI comparison are similar to those in Fig. 3. Strong improvements in ET and GPP over the corn and soybean areas of the Midwest and lower Mississippi are observed in the SMAP VOD DA evaluations. The ALEXI comparison indicates that the assimilation of VOD retrievals also improves the simulation of ET over the southeastern U.S., an area with thick vegetation density. Similar patterns are seen in the comparisons to GOME-2 SIF, where significant improvements in the correlation of simulated GPP with SIF observations are obtained over the southeastern U.S. and agricultural areas of the Midwest. These results suggest that the significant utility of the VOD retrievals is over the agricultural areas and locations with strong vegetation seasonality. Note that the patterns in Figs. 3 and 6 are not exactly equivalent due to the different time periods used in the evaluations.
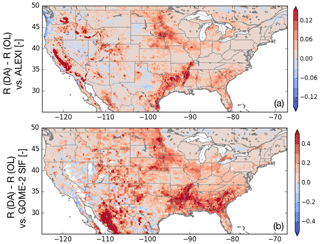
Figure 6Changes in the skill of simulated evapotranspiration and GPP as a result of assimilating L-band VOD estimates from SMAP. Panel (a) represents the changes in R of evapotranspiration (expressed as R(DA) −R (OL)), using ALEXI data as the reference. Panel (b) shows the changes in R of modeled GPP using solar-induced fluorescence data from GOME-2 as the reference, expressed as R (DA) −R (OL). The warm colors represent improvements from DA and cool colors represent degradations resulting from DA.
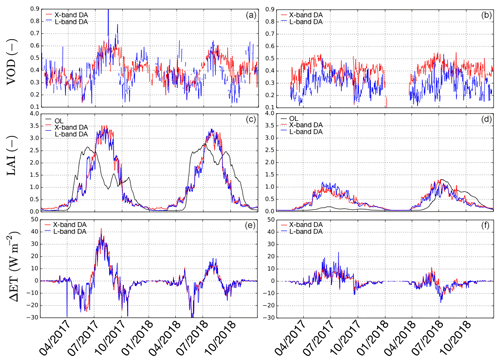
Figure 7Time series of VOD (a, b), LAI (c, d), and changes in evapotranspiration relative to the OL (e, f), for years 2017 and 2018, averaged over a cropland (location A in Fig. 1) and woodland (location B in Fig. 1) area. The left- and the right-hand side columns represent locations A and B, respectively.
To further examine the impact of VOD DA, Fig. 7 shows the time series of VOD, rescaled VOD (using CDF matching) as LAI, and the corresponding change in ET in DA simulations (relative to OL) at two locations. Location A is in Iowa with cropland as the dominant land cover and location B is in Montana with grassland as the dominant land cover (Fig. 1). The cropland location is used as an analog of an area where agricultural activity is likely present, whereas the grassland location is representative of a region where the natural variability is the dominant factor in the vegetation and ET seasonality. Note also that at location A, large improvements in ET and GPP are observed, whereas at location B, only marginal improvements are noticed in ET and GPP.
Over the cropland location A, both the L-band and X-band VOD estimates are consistent with each other, in terms of the amplitude and seasonality. The peak VOD seasonality is in the late summer and early fall, which is reflected in the rescaled LAI estimates. The model OL-based LAI, on the other hand, has an earlier peak, in the summer months. The assimilation of the rescaled VOD estimates leads to corrections in both the magnitude and phase of the LAI relative to the OL estimates. This also leads to a corresponding phase shift and increase in the peak ET estimates from DA. The changes in the ET in the DA simulations over location A range from approximately −30 to 40 W m−2 during the summer and fall months.
Compared to location A, over the grassland location B, there are small climatological differences in the VOD retrievals from the X- and L-bands. These amplitudinal differences are reduced by the CDF matching, as the rescaled X- and L-band VOD estimates are similar to each other. Overall, the changes in LAI in the assimilation runs relative to the OL are small, likely because this is an area with sparse vegetation. In year 2017, the main impact of DA is to increase the amplitude of LAI, whereas in 2018, the LAI estimates in the DA and OL are fairly consistent, except for a small phase shift. In the summer and fall months, the assimilation leads to approximately ±10 W m−2 changes in ET. The independent evaluations of ET in Fig. 3 confirm that these phase and magnitude corrections in LAI through the VOD DA (particularly at location A) are accurate. Similar but more muted impacts relative to the X-band DA are seen from the C-band DA (not shown).
3.4 Comparison of soil moisture and VOD DA
As there is a long legacy of retrieving soil moisture from microwave radiometry, the key focus of the associated missions and data assimilation studies has been on evaluating and demonstrating the utility of retrieved soil moisture measurements (Reichle et al., 2007; Q. Liu et al., 2011; Draper et al., 2012; Hain et al., 2012; Kumar et al., 2014; Lievens et al., 2017). These studies demonstrate the potential of remote sensing soil moisture retrievals to improve the simulation of moisture states. Efforts to translate the improvements in the soil moisture states to other water and energy stores, on the other hand, have only reported marginal success. Though changes in soil moisture states from DA impact the land–atmosphere fluxes at diurnal temporal scales (Santanello et al., 2016), their impacts at broader spatial and temporal scales are small. For example, studies at continental scales such as Peters-Lidard et al. (2011) and Martens et al. (2016) reported minor impacts on the simulated ET estimates from the assimilation of LPRM soil moisture retrievals. Here we compare and contrast the relative utility of assimilating the soil moisture and VOD retrievals from SMAP on various water and carbon states.
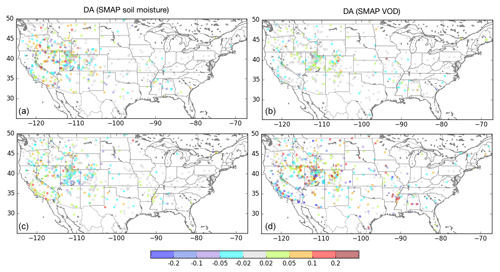
Figure 8Differences in anomaly R values for surface soil moisture (a, b) and root zone soil moisture (c, d) from the assimilation of soil moisture (a, c) and VOD (b, d), relative to the OL integration. The warm and cool colors indicate improvements and degradations from DA. The gray shading indicates locations where the anomaly R differences are not statistically significant.
Figures 8 to 10 show the impacts of separately assimilating SMAP soil moisture and VOD retrievals on various land surface water and carbon states. Using the in situ soil moisture measurements from ISMN as the reference, Fig. 8 shows the changes in anomaly R of surface and root zone soil moisture from soil moisture and VOD assimilation. Overall, soil moisture DA has a positive impact on the simulation of surface soil moisture, particularly in the western U.S. and High Plains. Approximately 2.14 % improvement in domain-averaged anomaly R is obtained from SMAP soil moisture assimilation. The impact of soil moisture DA over the eastern U.S. is small, as these regions of high vegetation density are generally excluded from soil moisture DA. Comparatively, VOD assimilation has little impact on surface soil moisture, as the changes in anomaly R are not statistically significant in most locations. Both soil moisture and VOD assimilation also impact root zone soil moisture estimates, with varying levels of improvements and degradations across the domain. The assimilation of SMAP soil moisture improves the root zone estimates over the lower Mississippi and parts of the western U.S., including California, Nevada, and Colorado. The patterns of improvements and degradations in root zone soil moisture are more mixed in the VOD assimilation results, over these same areas.
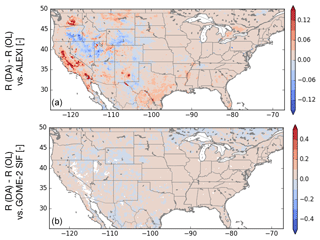
Figure 9Differences in R values for ET (a) and GPP (b) from the assimilation of SMAP soil moisture and VOD relative to the OL integration, using ALEXI ET and GOME-2 SIF datasets. The warm and cool colors indicate improvements and degradations from DA.
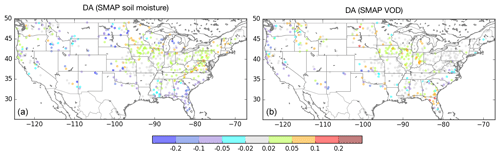
Figure 10Improvements in streamflow NSE shown as NIC using the USGS daily streamflow observations as the reference for SMAP soil moisture DA (a) and SMAP VOD DA (b).
Figure 9 shows the impact of soil moisture assimilation on ET and GPP. Consistent with prior studies, the impact of soil moisture assimilation on ET and GPP is small over most of the domain. Compared to ALEXI, SMAP soil moisture assimilation marginally improves the correlation of simulated ET over parts of central California, Washington, Montana, Texas, and lower Mississippi, with small degradations over several western states. The SMAP soil moisture assimilation has little impact on the simulation of GPP, as the change map of R against the GOME-2 SIF measurements shows no distinct spatial patterns of improvements or degradations. Comparatively, VOD assimilation has a strong and mostly beneficial impact on the simulation of ET and GPP, as shown in Fig. 6. In the comparisons against ALEXI and GOME-2, strong patterns of improvements are observed over the agricultural areas of the U.S. such as the Central Plains, lower Mississippi basin, and central California, from VOD DA.
Similarly to the description in Sect. 3.1, the normalized NSE improvements are represented using the NIC metric. Soil moisture assimilation has a beneficial impact on the streamflow simulation, with improvements over the Midwest and eastern U.S. and degradations over the southeast and parts of the Missouri basin and western locations. The impact of VOD assimilation on streamflow is marginal and is mostly restricted to the Midwest areas, which also are correlated with the corn-growing areas. Note that though there are some regional patterns of improvements and degradations in streamflow from soil moisture or VOD DA, these changes are small (most of the NIC changes are in the range of ±0.05 to 0.02).
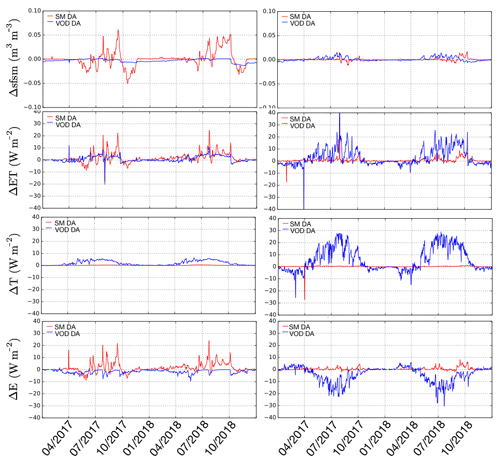
Figure 11Time series of changes (relative to the OL) in ET, surface soil moisture, transpiration, and bare soil evaporation for years 2017 and 2018, at locations C (western U.S.) and D (eastern U.S.). The left- and right-hand columns represent locations C and D, respectively.
To further investigate the relative utility of VOD and soil moisture DA, we compare the time series of changes in surface soil moisture, ET, transpiration, and bare soil evaporation at two locations (C and D) in the domain, in Fig. 11. Location C is in the arid western U.S. with moderate vegetation, whereas location D is in the eastern U.S., representing a wet region with thick vegetation. In the arid location C, soil moisture DA leads to changes in surface soil moisture primarily in the summer months, with differences as large as 0.05 m3 m−3 relative to the OL. The changes in soil moisture subsequently drive the changes in ET estimates. The comparison of the time series of transpiration and bare soil evaporation indicates that the changes in ET at location C are more directly connected to the changes in bare soil evaporation. There is essentially no change in transpiration from soil moisture DA at this location, but larger changes in bare soil evaporation occur as a result of changing soil moisture. Comparatively, at location C, VOD DA has little impact on soil moisture and ET. The changes in LAI introduced by VOD DA lead to a small increase in transpiration and a minor reduction in bare soil evaporation. These changes in the evaporative fluxes are not driven by the soil moisture changes, but rather by the small change to the vegetation coverage.
In contrast, over location D, there are little changes in soil moisture and ET from soil moisture DA, because not many observations are assimilated over this area with thick vegetation. The time series of transpiration and bare soil evaporation confirms that soil moisture DA has little impact on the evaporation regime. VOD DA, on the other hand, leads to large changes in ET as a result of the changes in LAI. The increased LAI leads to increased transpiration and root uptake of soil moisture. The reduction in root zone soil moisture also leads to reduced bare soil evaporation. Overall, VOD DA leads to increased ET in the summer months at this location because of these changes. These comparisons indicate that there is information in both soil moisture and VOD retrievals of SMAP that is useful in improving estimates of ET. Soil moisture information is more impactful over water-limited regions, where moisture conditions on the land are the primary controls on the evaporative fluxes. Over areas with high vegetation and little water limitation, vegetation growth and stomatal control, more than surface moisture conditions, influence the ET evolution. Since passive microwave retrievals of soil moisture are unreliable over such areas, the use of VOD provides an effective alternative. The above cases show a direct impact on the relative importance of transpiration vs. bare soil evaporation in the ET generation. Accurate estimation of this ET partitioning is important for a proper connection to the carbon cycle (Kumar et al., 2018).
The small improvements in hydrological budget terms such as ET and streamflow from soil moisture DA are also partly due to the mechanisms used in soil moisture DA configurations. As noted earlier, because of the use of rescaled retrievals (using CDF matching) in soil moisture DA, the analysis updates only reflect the corrections in the anomalies of soil moisture, rather than large changes in mean soil moisture estimates. The transformed VOD retrievals, on the other hand, are ingested directly as LAI within the LSM, essentially allowing the incorporation of the information inherent in the mean VOD/LAI signals. The limited use of the information in the soil moisture DA configuration is partly the reason for the limited impact on water budget states such as ET.
3.5 Joint assimilation of soil moisture and VOD retrievals
As the results in the previous section indicate that assimilation of soil moisture and VOD can provide mutually exclusive information, an assimilation configuration that employs these retrievals simultaneously is developed. Note that in this joint configuration, rather than augmenting the observation vector to encompass both VOD and soil moisture retrievals, we simply combine the two separate sequential univariate assimilation instances within a single integration. Similarly to the univariate configurations, in this multivariate configuration, soil moisture retrievals are used to update the surface soil moisture state, whereas VOD retrievals are used to update the prognostic LAI variable within the LSM.
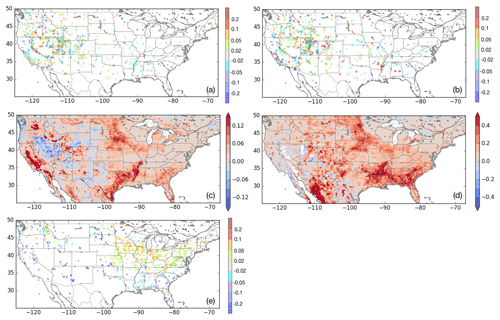
Figure 12Impact of jointly assimilating SMAP surface soil moisture and VOD retrievals on surface soil moisture (a), root zone soil moisture (b), ET (c), GPP (d), and streamflow (e). Panels (a) and (b) show differences in anomaly R values using ISMN data as the reference; panel (c) shows the differences in R values for ET using ALEXI as the reference dataset; panel (d) shows the difference in R values for GPP with GOME-2 SIF retrievals as the reference; panel (e) shows the NIC in streamflow using USGS daily streamflow observations as the reference. In each panel, the differences in the metric of evaluation are computed relative to the OL.
Figure 12 summarizes the impact on key water budget terms as a result of the joint assimilation of soil moisture and VOD. Overall, the joint assimilation consolidates the beneficial impact from the univariate assimilation configurations. For example, the multivariate DA configuration provides improved skills in both surface and root zone soil moisture, whereas the univariate VOD DA has little impact on surface soil moisture. Similarly, the univariate soil moisture DA configuration has little influence on the ET skill, whereas the ET improvement maps from the joint assimilation mirror the patterns of changes obtained with univariate VOD DA. The spatial influence of the individual assimilation configurations is also evident in these comparisons. For example, the ET improvement map (with ALEXI as the reference) from the joint DA shows strong patterns of improvements in the eastern U.S. similar to the result from the VOD DA configuration. The improvement in ET is accompanied by even higher percentage improvements in GPP. It is interesting to note the strong improvements centered on the Mississippi, as in where partitioning contributes to ET uncertainty (Kumar et al., 2018). In the western U.S., there are some patterns of degradation in ET, similar to what is observed when assimilating soil moisture alone. Similarly, in the streamflow comparisons, the joint assimilation shows strong patterns of improvements in areas east of Mississippi, whereas the impact of assimilation is mostly disadvantageous in the western parts of the domain. As noted earlier, these patterns reflect the larger impact of soil moisture and VOD in the water-limited (western U.S.) and water-sufficient (eastern U.S.) domains.
Vegetation conditions have a significant influence on the terrestrial water, energy, and carbon exchanges and feedbacks. Through stomatal control, plants influence transpiration, root uptake of soil moisture, and evaporative fluxes. The presence of vegetation also impacts the evolution of snow by influencing surface albedo and the amount of net radiation on the land surface. In addition to the changes in vegetation phenology driven by natural variability, anthropogenic activities such as agriculture and vegetation disturbances also significantly alter the vegetation characteristics on the land surface. Data assimilation of remotely sensed estimates of vegetation conditions within land surface models enables the refinement of modeled estimates, enhancement of the spatio-temporal coverage of remote sensing measurements, and the extension of the remote sensing vegetation information to water, energy, and carbon states and fluxes.
Remote-sensing-based estimates of vegetation conditions are typically developed from multi-spectral and hyperspectral optical and thermal satellite sensors. Though passive microwave sensors are often used for retrieving soil moisture estimates, they also enable the estimation of vegetation optical depth, an analog of above-ground canopy biomass. As microwave measurements are not influenced by clouds, they can be made in virtually all weather conditions. This article examines the utility of VOD retrievals from passive microwave sensors by assimilating them within the dynamic phenology model of the Noah-MP LSM.
The study is conducted in the NLDAS-2 configuration over the Continental U.S. A suite of publicly available VOD retrievals from X-, C-, and L-band instruments is assimilated in Noah-MP using a 1-D ensemble Kalman filter algorithm. The X- and C-band retrievals are from the Land Parameter Retrieval Model, whereas the L-band retrievals of VOD are from SMAP. Since Noah-MP does not include a prognostic representation of VOD, the assimilation is conducted by transforming the VOD retrievals into LAI estimates, using the MODIS-based GLASS LAI product. The impact of assimilating VOD on key water and carbon budget terms is evaluated by comparing against a large suite of reference datasets.
The assimilation of VOD from the passive microwave sensors is found to have a significant beneficial impact on improving the simulation of ET and GPP, particularly over the agricultural areas of the U.S. The assimilation of X-band-based VOD retrievals is found to provide larger improvements in ET, relative to the assimilation of C-band VOD retrievals. The impacts on soil moisture, terrestrial water storage, and streamflow from VOD DA are found to be marginal. Regionally, the largest impacts on these variables are also observed over the agricultural areas. Though the time period of available data is limited, the assimilation of L-band VOD retrievals from SMAP is also found to have significant beneficial impacts on the simulation of ET and GPP, similar to that from the X-band VOD DA. It must be stressed that as the retrieval algorithms used to develop these VOD products are different, this particular study is not structured to assess the relative merits of each algorithm.
Though passive microwave-based measurements are available in nearly all-weather conditions, their spatial resolution and temporal frequency are coarser than the optical/IR-based vegetation estimates. This study compared the impact of VOD assimilation to that of assimilating optical sensor-based LAI from a prior study. Overall, the magnitude of improvements from VOD DA is comparable to that from assimilating MODIS LAI. These findings confirm that assimilation of VOD retrievals can provide an effective augmentation or alternative to assimilating data from optical sensors, enabling the mitigation of sensing, coverage, and spatial-resolution-based limitations of each type of sensor.
The relative and joint utilities of assimilating soil moisture and VOD retrievals from SMAP are also examined in this study. Overall, the assimilation of soil moisture retrievals has a positive impact on the simulation of surface soil moisture and little impact on evaporative fluxes. In contrast, VOD DA has significant impacts on the simulation of vegetation conditions, root zone soil moisture, and evapotranspiration. Over water-limited domains with sparse vegetation where soil moisture is the primary control on ET, the assimilation of surface soil moisture is more beneficial than VOD DA. Over regions with dense vegetation and where water availability is not limiting, transpiration has a significant influence on evapotranspiration. The assimilation of VOD is more beneficial in developing improvements in ET over such locations. In addition, when vegetation coverage is dense, the soil moisture retrievals have large uncertainty and are unreliable. In those areas, the use of VOD provides an alternate way to develop improved estimates of terrestrial hydrologic responses informed by remote sensing. The results in the paper also confirm that the soil moisture and VOD retrievals provide information that can be jointly exploited through their simultaneous assimilation.
As noted in the description of the data assimilation methodology, the VOD retrievals are assimilated by rescaling them to the GLASS MODIS LAI climatology. This approach was employed as the prior study Kumar et al. (2019b) demonstrated significant positive impacts from the assimilation of the GLASS LAI data. Such an approach is needed also because the LSM does not have a prognostic representation of VOD. Though the beneficial impacts observed in the results indicate that this is a reasonable strategy, the rescaling essentially ignores the information on vertical heterogeneity in the canopy from these sensors. For example, the X-band data are documented to be more sensitive to the vegetation, whereas the L-band data are more representative of the lower canopy. A more direct use of the VOD data is likely to help in resolving these sensitivities within modeling. Extensions to this study that either use a prognostic representation of VOD or a forward model that simulates VOD will enable such approaches. The current study serves as a useful benchmark for such future efforts.
Finally, as noted earlier, the NLDAS-2 configuration is a conservative environment to evaluate the utility of data assimilation configurations due to availability of high-quality boundary condition data. The significant utility of VOD DA demonstrated in this paper suggests that larger benefits from VOD DA are likely over areas with lower-quality meteorological boundary conditions.
The underlying data can be obtained by contacting the lead author of this manuscript. The data will then be served through NASA's open source data distribution mechanisms, which provide temporary storage for large datasets for a limited time period. Note that the total data size for all the model runs and analysis presented in this paper amounts to 966 GB, which is too large for commonly available open source public data repositories.
The source code used for the model and data assimilation integrations used in this article can be obtained at Kumar (2020).
SVK led the formulation of the idea, development of the model runs and the article. TRH assisted with the concept development and article review. RB provided interpretation of the SMAP soil moisture and VOD datasets. RdJ provided assistance with the LPRM VOD data. CPL contributed to the manuscript development. All the authors contributed to the synthesis of results and key conclusions.
The authors declare that they have no conflict of interest.
Funding for this work was provided by the NOAA's climate program office (MAPP program). Computing was supported by the resources at the NASA Center for Climate Simulation. The NLDAS-2 forcing data used in this effort were acquired as part of the activities of NASA's Science Mission Directorate and are archived and distributed by the Goddard Earth Sciences (GES) Data and Information Services Center (DISC).
This research has been supported by the NOAA climate program office (grant no. GC17-701A).
This paper was edited by Pierre Gentine and reviewed by three anonymous referees.
Albergel, C., Munier, S., Leroux, D. J., Dewaele, H., Fairbairn, D., Barbu, A. L., Gelati, E., Dorigo, W., Faroux, S., Meurey, C., Le Moigne, P., Decharme, B., Mahfouf, J.-F., and Calvet, J.-C.: Sequential assimilation of satellite-derived vegetation and soil moisture products using SURFEX_v8.0: LDAS-Monde assessment over the Euro-Mediterranean area, Geosci. Model Dev., 10, 3889–3912, https://doi.org/10.5194/gmd-10-3889-2017, 2017. a
Albergel, C., Munier, S., Bocher, A., Bonan, B., Zheng, Y., Draper, C., Leroux, D., and Calvet, J.-C.: LDAS-Monde sequential assimilation of satellite derived observations applied to the contiguous US: An ERA-5 driven reanalysis of the land surface variables, Remote Sens., 10, 1627, https://doi.org/10.3390/rs10101627, 2018. a, b
Andela, N., Liu, Y. Y., van Dijk, A. I. J. M., de Jeu, R. A. M., and McVicar, T. R.: Global changes in dryland vegetation dynamics (1988–2008) assessed by satellite remote sensing: comparing a new passive microwave vegetation density record with reflective greenness data, Biogeosciences, 10, 6657–6676, https://doi.org/10.5194/bg-10-6657-2013, 2013. a
Anderson, M., Norman, J., Mecikalski, J., Otkin, J., and Kustas, W.: A climatological study of evapotranspiration and moisture stress across the continental U.S. based on thermal remote sensing: I. Model formulation, J. Geophys. Res., 112, D11112, https://doi.org/10.1029/2006JD007506, 2007. a
Anderson, M. C., Allen, R. G., Morse, A., and Kustas, W. P.: Use of Landsat thermal imagery in monitoring evapotranspiration and managing water resources, Remote Sens. Environ., 122, 50–65, https://doi.org/10.1016/j.rse.2011.08.025, 2012. a
Barbu, A. L., Calvet, J.-C., Mahfouf, J.-F., Albergel, C., and Lafont, S.: Assimilation of Soil Wetness Index and Leaf Area Index into the ISBA-A-gs land surface model: grassland case study, Biogeosciences, 8, 1971–1986, https://doi.org/10.5194/bg-8-1971-2011, 2011. a
Barbu, A. L., Calvet, J.-C., Mahfouf, J.-F., and Lafont, S.: Integrating ASCAT surface soil moisture and GEOV1 leaf area index into the SURFEX modelling platform: a land data assimilation application over France, Hydrol. Earth Syst. Sci., 18, 173–192, https://doi.org/10.5194/hess-18-173-2014, 2014. a
Chan, S., Bindlish, R., O'Neill, P., Jackson, T., Njoku, E., Dunbar, S., Chaubell, J., Piepmeier, J., Yueh, S., Entekhabi, D., Colliander, A., Chen, F., Cosh, M., Caldwell, T., Walker, J., Berg, A., McNairn, H., Thibeault, M., Martínez-Fernández, J., Uldall, F., Seyfried, M., Bosch, D., Starks, P., Collins, C. H., Prueger, J., van der Velde, R., Asanuma, J., Palecki, M., Small, E., Zreda, M., Calvet, J., Crow, W., and Kerr, Y.: Development and assessment of the SMAP enhanced passive soil moisture product, Remote Sens. Environ., 204, 931–941, https://doi.org/10.1016/j.rse.2017.08.025, 2018. a
Chaubell, M. J., Yueh, S. H., Dunbar, R. S., Colliander, A., Chen, F., Chan, S. K., Entekhabi, D., Bindlish, R., O'Neill, P. E., Asanuma, J., Berg, A. A., Bosch, D. D., Caldwell, T., Cosh, M. H., Collins, C. H., Martínez-Fernández, J., Seyfried, M., Starks, P. J., Su, Z., Thibeault, M., and Walker, J.: Improved SMAP Dual-Channel Algorithm for the Retrieval of Soil Moisture, IEEE T. Geosci. Remote, 1–12, 2020. a
der Schalie, R., Kerr, Y., Wigneron, J., Rodríguez-Fernández, N., Al-Yaari, A., and Jeu, R.: Global SMOS Soil Moisture Retrievals from The Land Parameter Retrieval Model, Int. J. Appl. Earth Obs., 45, 125–134, https://doi.org/10.1016/j.jag.2015.08.005 2016. a
Dirmeyer, P., Gao, X., Zhao, M., Guo, Z., Oki, T., and Hanasaki, N.: GSWP-2: Multimodel analysis and implications for our perception of the land surface, B. Am. Meteorol. Soc., 87, 1381–1397, https://doi.org/10.1175/BAMS-87-10-1381, 2006. a
Dorigo, W. A., Wagner, W., Hohensinn, R., Hahn, S., Paulik, C., Xaver, A., Gruber, A., Drusch, M., Mecklenburg, S., van Oevelen, P., Robock, A., and Jackson, T.: The International Soil Moisture Network: a data hosting facility for global in situ soil moisture measurements, Hydrol. Earth Syst. Sci., 15, 1675–1698, https://doi.org/10.5194/hess-15-1675-2011, 2011. a
Dorigo, W. A., Xaver, A., Vreugdenhil, M., Gruber, A., Hegyiova, A., Sanchis-Dufau, A., Zamojski, D., Cordes, C., Wagner, W., and Drusch, M.: Global Automated Quality Control of In Situ Soil Moisture Data from the International Soil Moisture Network, Vadose Zone Journal, 12, 1–21, https://doi.org/10.2136/vzj2012.0097, 2013. a
Draper, C., Reichle, R., De Lannoy, G., and Liu, Q.: Assimilation of passive and active microwave soil moisture retrievals, Geophys. Res. Lett., 39, L04401, https://doi.org/10.1029/2011GL050655, 2012. a
Entekhabi, D., Njoku, E., O'Neill, P., Kellogg, K., Crow, W. T., Edelstein, W., Entin, J., Goodman, S., Jackson, T., Johnson, J., Kimball, J., Piepmeier, J., Koster, R., Martin, N., McDonald, K., Moghaddam, M., Moran, S., Reichle, R., Shi, J., Spencer, M., Thurman, S., Tsang, L., and Van Zyl, J.: The Soil Moisture Active Passive (SMAP) Mission, P. IEEE, 98, 704–716, https://doi.org/10.1109/jproc.2010.2043918, 2010. a
Fang, H., Jiang, C., Li, W., Wei, S., Baret, F., Chen, J. M., Garcia-Haro, J., Liang, S., Liu, R., Myneni, R. B., Pinty, B., Xiao, Z., and Zhu, Z.: Characterization and intercomparison of global moderate resolution leaf area index (LAI) products: Analysis of climatologies and theoretical uncertainties, J. Geophys. Res.-Biogeo., 118, 529–548, https://doi.org/10.1002/jgrg.20051, 2013. a
Fox, A. M., Hoar, T. J., Anderson, J. L., Arellano, A. F., Smith, W. K., Litvak, M. E., MacBean, N., Schimel, D. S., and Moore, D. J. P.: Evaluation of a Data Assimilation System for Land Surface Models Using CLM4.5, J. Adv. Model. Earth Syst., 10, 2471–2494, https://doi.org/10.1029/2018MS001362, 2018. a
Getirana, A., Boone, A., Yamazaki, D., Decharme, B., Papa, F., and Mognard, N.: The Hydrological Modeling and Analysis Platform (HyMAP): Evaluation in the Amazon Basin, J. Hydrometeor., 13, 1641–1665, https://doi.org/10.1175/JHM-D-12-021.1, 2012. a
Goetz, A., Vane, G., Solomon, J., and Rock, B.: Imaging specdtrometry for earth remote sensing, Science, 228, 1147–1153, 1985. a
Grant, J., Wigneron, J.-P., Jeu, R. D., Lawrence, H., Mialon, A., Richaume, P., Bitar, A. A., Drusch, M., van Marle, M., and Kerr, Y.: Comparison of SMOS and AMSR-E vegetation optical depth to four MODIS-based vegetation indices, Remote Sens. Environ., 172, 87–100, https://doi.org/10.1016/j.rse.2015.10.021, 2016. a
Guanter, L., Zhang, Y., Jung, M., Joiner, J., Voigt, M., Berry, J. A., Frankenberg, C., Huete, A. R., Zarco-Tejada, P., Lee, J.-E., Moran, M. S., Ponce-Campos, G., Beer, C., Camps-Valls, G., Buchmann, N., Gianelle, D., Klumpp, K., Cescatti, A., Baker, J. M., and Griffis, T. J.: Global and time-resolved monitoring of crop photosynthesis with chlorophyll fluorescence, P. Natl. Acad. Sci. USA, 111, E1327–E1333, https://doi.org/10.1073/pnas.1320008111, 2014. a
Hain, C., Crow, W., Anderson, M., and Mecikalski, J.: An ensemble Kalman filter dual assimilation of thermal infrared and microwave satellite observations of soil moisture into the Noah land surface model, Water Resour. Res., 48, W11517, https://doi.org/10.1029/2011WR011268, 2012. a
Hain, C. R., Crow, W. T., Anderson, M. C., and Yilmaz, M. T.: Diagnosing Neglected Soil Moisture Source-Sink Processes via a Thermal Infrared-Based Two-Source Energy Balance Model, J. Hydrometeorol., 16, 1070–1086, https://doi.org/10.1175/JHM-D-14-0017.1, 2015. a
Hall, D. K., Riggs, G. A., Foster, J. L., and Kumar, S. V.: Development and evaluation of a cloud-gap-filled MODIS daily snow-cover product, Remote Sens. Environ., 114, 496–503, https://doi.org/10.1016/j.rse.2009.10.007, 2010. a
Hollinger, S. E. and Isard, S. A.: A Soil Moisture Climatology of Illinois, J. Climate, 7, 822–833, https://doi.org/10.1175/1520-0442(1994)007<0822:ASMCOI>2.0.CO;2, 1994. a
Holmes, T. R. H., Hain, C. R., Anderson, M. C., and Crow, W. T.: Cloud tolerance of remote-sensing technologies to measure land surface temperature, Hydrol. Earth Syst. Sci., 20, 3263–3275, https://doi.org/10.5194/hess-20-3263-2016, 2016. a
Houborg, R., Fisher, J. B., and Skidmore, A. K.: Advances in remote sensing of vegetation function and traits, Int. J. Appl. Earth Obs. Geoinformation, 43, 1–6, https://doi.org/10.1016/j.jag.2015.06.001, 2015. a
Huete, A., Liu, H., Batchily, K., and Van Leeuwen, W.: A comparison of vegetation indices over a global set of TM images for EOS-MODIS, Remote Sens. Environ., 59, 440–451, 1997. a
Jackson, T. J., Cosh, M. H., Bindlish, R., Starks, P. J., Bosch, D. D., Seyfried, M., Goodrich, D. C., Moran, M. S., and Du, J.: Validation of Advanced Microwave Scanning Radiometer Soil Moisture Products, IEEE T. Geosci. Remote, 48, 4256–4272, 2010. a
Joiner, J., Yoshida, Y., Vasilkov, A., Schaefer, K., Jung, M., Guanter, L., Zhang, Y., Garrity, S., Middleton, E., Huemmrich, K., Gu, L., and Marchesini, L. B.: The seasonal cycle of satellite chlorophyll fluorescence observations and its relationship to vegetation phenology and ecosystem atmosphere carbon exchange, Remote Sens. Environ., 152, 375–391, https://doi.org/10.1016/j.rse.2014.06.022, 2014. a
Jones, M. O., Kimball, J. S., Jones, L. A., and McDonald, K. C.: Satellite passive microwave detection of North America start of season, Remote Sens. Environ., 123, 324–333, 2012. a
Jung, M., Reichstein, M., and Bondeau, A.: Towards global empirical upscaling of FLUXNET eddy covariance observations: validation of a model tree ensemble approach using a biosphere model, Biogeosciences, 6, 2001–2013, https://doi.org/10.5194/bg-6-2001-2009, 2009. a
Jung, M., Reichstein, M., Schwalm, C., Huntingford, C., Sitch, S., Ahlström, A., Arneth, A., Camps-Valls, G., Ciais, P., Friedlingstein, P., Gans, F., Ichii, K., Jain, A., Kato, E., Papale, D., Poulter, B., Raduly, B., Rödenbeck, C., Tramontana, G., Viovy, N., Wang, Y.-P., Weber, U., Zaehle, S., and Zeng, N.: Compensatory water effects link yearly global land CO2 sink changes to temperature, Nature, 541, 516–520, https://doi.org/10.1038/nature20780, 2017. a
Knipling, E. B.: Physical and physiological basis for the reflectance of visible and near-infrared radiation from vegetation, Remote Sens. Environ., 1, 155–159, https://doi.org/10.1016/S0034-4257(70)80021-9, 1970. a
Konings, A. G. and Gentine, P.: Global variations in ecosystem-scale isohydricity, Glob. Change Biol., 23, 891–905, 2017. a
Konings, A. G., Piles, M., Rotzer, K., McColl, K. A., Chan, S. K., and Entekhabi, D.: Vegetation optical depth and scattering albedo retrieval using time series of dual-polarized L-band radiometer observations, Remote Sens. Environ., 172, 178–189, https://doi.org/10.1016/j.rse.2015.11.009, 2016. a
Konings, A. G., Piles, M., Das, N., and Entekhabi, D.: L-band vegetation optical depth and effective scattering albedo estimation from SMAP, Remote Sens. Environ., 198, 460–470, https://doi.org/10.1016/j.rse.2017.06.037, 2017. a, b, c
Konings, A. G., Rao, K., and Steele-Dunne, S. C.: Macro to micro: microwave remote sensing of plant water content for physiology and ecology, New Phytol., 223, 1166–1172, https://doi.org/10.1111/nph.15808, 2019. a
Koster, R., Guo, Z., Dirmeyer, P., Yang, R., Mitchell, K., and Puma, M.: On the nature of soil moisture in land surface models, J. Climate, 22, 4322–4335, https://doi.org/10.1175/2009JCLI2832.1, 2009. a
Kumar, L. and Mutanga, O.: Remote sensing of above-ground biomass, Remote Sensing, 9, 935, https://doi.org/10.3390/rs9090935, 2017. a
Kumar, S. V.: Land information system, Zenodo, https://doi.org/10.5281/zenodo.3883907, 2020. a
Kumar, S. V., Peters-Lidard, C., Tian, T., Houser, P., Geiger, J., Olden, S., Lighty, L., Eastman, J., Doty, B., Dirmeyer, P., Adams, J., Mitchell, K., Wood, E., and Sheffield, J.: Land Information System: An interoperable framework for high resolution land surface modeling, Environ. Model. Softw., 21, 1402–1415, 2006. a
Kumar, S. V., Peters-Lidard, C. D., Santanello, J., Harrison, K., Liu, Y., and Shaw, M.: Land surface Verification Toolkit (LVT) – a generalized framework for land surface model evaluation, Geosci. Model Dev., 5, 869–886, https://doi.org/10.5194/gmd-5-869-2012, 2012. a
Kumar, S. V., Peters-Lidard, C. D., Mocko, D., Reichle, R., Liu, Y., Arsenault, K. R., Xia, Y., Ek, M., Riggs, G., Livneh, B., and Cosh, M.: Assimilation of Remotely Sensed Soil Moisture and Snow Depth Retrievals for Drought Estimation, J. Hydrometeorol., 15, 2446–2469, https://doi.org/10.1175/JHM-D-13-0132.1, 2014. a, b, c, d
Kumar, S. V., Peters-Lidard, C. D., Santanello, J. A., Reichle, R. H., Draper, C. S., Koster, R. D., Nearing, G., and Jasinski, M. F.: Evaluating the utility of satellite soil moisture retrievals over irrigated areas and the ability of land data assimilation methods to correct for unmodeled processes, Hydrol. Earth Syst. Sci., 19, 4463–4478, https://doi.org/10.5194/hess-19-4463-2015, 2015. a, b
Kumar, S. V., Holmes, T., Mocko, D. M., Wang, S., and Peters-Lidard, C.: Attribution of Flux Partitioning Variations between Land Surface Models over the Continental U.S., Remote Sensing, 10, 751, https://doi.org/10.3390/rs10050751, 2018. a, b
Kumar, S. V., Jasinski, M., Mocko, D. M., Rodell, M., Borak, J., Li, B., Beaudoing, H. K., and Peters-Lidard, C. D.: NCA-LDAS Land Analysis: Development and Performance of a Multisensor, Multivariate Land Data Assimilation System for the National Climate Assessment, J. Hydrometeorol., 20, 1571–1593, https://doi.org/10.1175/JHM-D-17-0125.1, 2019a. a, b, c
Kumar, S. V., Mocko, D. M., Wang, S., Peters-Lidard, C. D., and Borak, J.: Assimilation of remotely sensed Leaf Area Index into the Noah-MP land surface model: Impacts on water and carbon fluxes and states over the Continental U.S., J. Hydrometeorol., 20, 1359–1377, https://doi.org/10.1175/JHM-D-18-0237.1, 2019b. a, b, c, d, e, f, g, h, i, j, k, l, m, n, o, p, q
Landerer, F. and Swenson, S.: Accuracy of scaled GRACE terrestrial water storage estimates, Water Resour. Res., 48, W04531, https://doi.org/10.1029/2011WR011453, 2012. a
Liao, Y., Gai, Y., Fan, W., Xu, X., Yan, B., and Liu, Y.: Validation methods of LAI products based on scaling effect, in: 2012 IEEE International Geoscience and Remote Sensing Symposium, 22–27 July 2012, Munich, Germany, 1692–1694, https://doi.org/10.1109/IGARSS.2012.6351200, 2012. a
Lievens, H., Reichle, R. H., Liu, Q., De Lannoy, G. J. M., Dunbar, R. S., Kim, S. B., Das, N. N., Cosh, M., Walker, J. P., and Wagner, W.: Joint Sentinel-1 and SMAP data assimilation to improve soil moisture estimates, Geophys. Res. Lett., 44, 6145–6153, https://doi.org/10.1002/2017GL073904, 2017. a
Liu, Q., Reichle, R., Bindlish, R., Cosh, M., Crow, W., de Jeu, R., De Lannoy, G. J. M., Huffman, G., and Jackson, T.: The contributions of precipitation and soil moisture observations to the skill of soil moisture estimates in a land data assimilation system, J. Hydrometeorol., 12, 750–765, https://doi.org/10.1175/JHM-D-10-05000, 2011. a
Liu, Y. Y., de Jeu, R. A., McCabe, M. F., Evans, J. P., and van Dijk, A. I.: Global long-term passive microwave satellite-based retrievals of vegetation optical depth, Geophys. Res. Lett., 38, L18402, https://doi.org/10.1029/2011GL048684, 2011. a
Liu, Y. Y., Evans, J., McCabe, M., de Jeu, R., van Dijk, A., Dolman, A., and Saizen, I.: Changing climate and overgrazing are decimating Mongolian steppes, PloS one, 8, e57599, https://doi.org/10.1371/journal.pone.0057599, 2013. a
Liu, Y. Y., Van Dijk, A. I., De Jeu, R. A., Canadell, J. G., McCabe, M. F., Evans, J. P., and Wang, G.: Recent reversal in loss of global terrestrial biomass, Nature Climate Change, 5, 470–474, https://doi.org/10.1038/nclimate2581, 2015. a
Martens, B., Miralles, D., Lievens, H., Fernández-Prieto, D., and Verhoest, N.: Improving terrestrial evaporation estimates over continental Australia through assimilation of SMOS soil moisture, Int. J. Appl. Earth Obs., 48, 146–162, https://doi.org/10.1016/j.jag.2015.09.012, 2016. a
Meesters, A. G. C. A., De Jeu, R. A. M., and Owe, M.: Analytical derivation of the vegetation optical depth from the microwave polarization difference index, IEEE Geosci. Remote S., 2, 121–123, https://doi.org/10.1109/LGRS.2005.843983, 2005. a
Mo, T., Choudhury, B. J., Schmugge, T. J., Wang, J. R., and Jackson, T. J.: A model for microwave emission from vegetation-covered fields, J. Geophys. Res.-Oceans, 87, 11229–11237, https://doi.org/10.1029/JC087iC13p11229, 1982. a
Moesinger, L., Dorigo, W., de Jeu, R., van der Schalie, R., Scanlon, T., Teubner, I., and Forkel, M.: The global long-term microwave Vegetation Optical Depth Climate Archive (VODCA), Earth Syst. Sci. Data, 12, 177–196, https://doi.org/10.5194/essd-12-177-2020, 2020. a
Myneni, R., Hoffman, S., Knyazikhin, Y., Privette, J., Glassy, J., Tian, Y., Wang, Y., Song, X., Zhang, Y., Smith, G., Lotsch, A., Friedl, M., Morisette, J., Votava, P., Nemani, R., and Running, S.: Global products of vegetation leaf area and fraction absorbed PAR from year one of MODIS data, Remote Sens. Environ., 83, 214–231, https://doi.org/10.1016/S0034-4257(02)00074-3, 2002. a
Myneni, R., Knyazikhin, Y., and Shabanov, N.: Leaf area index and fraction of absorbed PAR products from Terra and Aqua MODIS sensors: Analysis, Validation and Refinement, in: Land remote sensing and global environmental change, vol. 11, 603–633, Springer, New York, NY, USA, 2011. a
Niu, G.-Y., Yang, Z.-L., Mitchell, K., Chen, F., Ek, M., Barlage, M., Kumar, A., Manning, K., Niyogi, D., Rosero, E., Tewari, M., and Xia, Y.: The community Noah land surface model with multiparameterization options (Noah-MP): 1. Model description and evaluation with local-scale measurements, J. Geophys. Res.-Atmos., 116, D12109, https://doi.org/10.1029/2010JD015139, 2011. a
Njoku, E. G. and Entekhabi, D.: Passive microwave remote sensing of soil moisture, J. Hydrol., 184, 101–129, https://doi.org/10.1016/0022-1694(95)02970-2, 1996. a
Njoku, E. G., Ashcroft, P., Chan, T. K., and Li, L.: Global survey and statistics of radio-frequency interference in AMSR-E land observations, IEEE T. Geosci. Remote, 43, 938–947, https://doi.org/10.1109/TGRS.2004.837507, 2005. a
Owe, M., de Jeu, R., and Walker, J.: A methodology for surface soil moisture and vegetation optical depth retrieval using the microwave polarization difference index, IEEE T. Geosci. Remote, 39, 1643–1654, https://doi.org/10.1109/36.942542, 2001. a
Owe, M., de Jeu, R., and Holmes, T. : Multi-sensor historical climatology of satellite-derived global land surface moisture, J. Geophys. Res., 13, F01002, https://doi.org/10.1029/2007JF000769, 2008. a, b
Parinussa, R. M., Holmes, T. R. H., Yilmaz, M. T., and Crow, W. T.: The impact of land surface temperature on soil moisture anomaly detection from passive microwave observations, Hydrol. Earth Syst. Sci., 15, 3135–3151, https://doi.org/10.5194/hess-15-3135-2011, 2011. a
Peters-Lidard, C. D., Kumar, S. V., Mocko, D. M., and Tian, Y.: Estimating evapotranspiration with land data assimilation systems, Hydrol. Process., 25, 3979–3992, https://doi.org/10.1002/hyp.8387, 2011. a
Price, J. C. and Bausch, W. C.: Leaf area index estimation from visible and near-infrared reflectance data, Remote Sens. Environ., 52, 55–65, 1995. a
Reichle, R. and Koster, R.: Bias reduction in short records of satellite soil moisture, Geophys. Res. Lett., 31, L19501, https://doi.org/10.1029/2004GL020938, 2004. a
Reichle, R., McLaughlin, D., and Entekhabi, D.: Hydrologic data assimilation with the ensemble Kalman Filter, Mon. Weather Rev., 130, 103–114, 2002. a
Reichle, R., Koster, R., Liu, P. and. Mahanama, S., Njoku, E., and Owe, M.: Comparison and assimilation of global soil moisture retrievals from the Advanced Microwave Scanning Radiometer for the Earth Observing System (AMSR-E) and the Scanning Multichannel Microwave Radiometer (SMMR), J. Geophys. Res.-Atmos., 112, D09108, https://doi.org/10.1029/2006JD008033, 2007. a
Sabater, J., Rudiger, C., Calvet, J.-C., Fritz, N., Jarlan, L., and Kerr, Y.: Joint assimilation of surface soil moisture and LAI observations into a land surface model, Agr. Forest Meteorol., 148, 1362–1373, https://doi.org/10.1016/j.agrformet.2008.04.003, 2008. a
Santanello, J. A., Kumar, S. V., Peters-Lidard, C. D., and Lawston, P. M.: Impact of Soil Moisture Assimilation on Land Surface Model Spinup and Coupled Land–Atmosphere Prediction, J. Hydrometeorol. 17, 517–540, https://doi.org/10.1175/JHM-D-15-0072.1, 2016. a
Smith, W. K., Fox, A. M., MacBean, N., Moore, D. J. P., and Parazoo, N. C.: Constraining estimates of terrestrial carbon uptake: new opportunities using long-term satellite observations and data assimilation, New Phytol., 225, 105–112, https://doi.org/10.1111/nph.16055, 2020. a
Teubner, I. E., Forkel, M., Jung, M., Liu, Y. Y., Miralles, D. G., Parinussa, R., van der Schalie, R., Vreugdenhil, M., Schwalm, C. R., Tramontana, G., Camps-Valls, G., and Dorigo, W. A.: Assessing the relationship between microwave vegetation optical depth and gross primary production, Int. J. Appl. Earth Obs., 65, 79–91, https://doi.org/10.1016/j.jag.2017.10.006, 2018. a
Teubner, I. E., Forkel, M., Camps-Valls, G., Jung, M., Miralles, D. G., Tramontana, G., van der Schalie, R., Vreugdenhil, M., Mösinger, L., and Dorigo, W. A.: A carbon sink-driven approach to estimate gross primary production from microwave satellite observations, Remote Sens. Environ., 229, 100–113, https://doi.org/10.1016/j.rse.2019.04.022, 2019. a
Tramontana, G., Jung, M., Schwalm, C. R., Ichii, K., Camps-Valls, G., Ráduly, B., Reichstein, M., Arain, M. A., Cescatti, A., Kiely, G., Merbold, L., Serrano-Ortiz, P., Sickert, S., Wolf, S., and Papale, D.: Predicting carbon dioxide and energy fluxes across global FLUXNET sites with regression algorithms, Biogeosciences, 13, 4291–4313, https://doi.org/10.5194/bg-13-4291-2016, 2016. a
Tucker, C. J., Pinzon, J. E., Brown, M. E., Slayback, D. A., Pak, E. W., Mahoney, R., Vermote, E. F., and Saleous, N. E.: An extended AVHRR 8-km NDVI dataset compatible with MODIS and SPOT vegetation NDVI data, Int. J. Remote Sens., 26, 4485–4498, https://doi.org/10.1080/01431160500168686, 2005. a
Van der Schalie, R., De Jeu, R., Parinussa, R., Rodríguez-Fernández, N., Kerr, Y., Al-Yaari, A., Wigneron, J.-P., and Drusch, M.: The Effect of Three Different Data Fusion Approaches on the Quality of Soil Moisture Retrievals from Multiple Passive Microwave Sensors, Remote Sensing, 10, 107, https://doi.org/10.3390/rs10010107, 2018. a
van Marle, M. J. E., van der Werf, G. R., de Jeu, R. A. M., and Liu, Y. Y.: Annual South American forest loss estimates based on passive microwave remote sensing (1990–2010), Biogeosciences, 13, 609–624, https://doi.org/10.5194/bg-13-609-2016, 2016. a
Vreugdenhil, M., Dorigo, W. A., Wagner, W., de Jeu, R. A. M., Hahn, S., and van Marle, M. J. E.: Analyzing the Vegetation Parameterization in the TU-Wien ASCAT Soil Moisture Retrieval, IEEE T. Geosci. Remote, 54, 3513–3531, 2016. a
Vreugdenhil, M., Hahn, S., Melzer, T., Bauer-Marschallinger, B., Reimer, C., Dorigo, W. A., and Wagner, W.: Assessing Vegetation Dynamics Over Mainland Australia With Metop ASCAT, IEEE J. Sel. Top. Appl., 10, 2240–2248, 2017. a
Xia, Y., Mitchell, K., Ek, M., Sheffield, J., Cosgrove, B., Wood, E., Luo, L., Alonge, C., Wei, H., Meng, J., Livneh, B., Lettenmaier, D.and Koren, V., Duan, Q., K., M., Fan, Y., and Mocko, D.: Continental-scale water and energy flux analysis and validation for the North American Land Data Assimilation System Project Phase 2 (NLDAS-2), Part 1: Comparison Analysis and Application of Model Products, J. Geophys. Res.-Atmos., 117, D03109, https://doi.org/10.1029/2011JD016048, 2012. a
Xiao, Z., Liang, S., Wang, J., Xiang, Y., Zhao, X., and Song, J.: Long-Time-Series Global Land Surface Satellite Leaf Area Index Product Derived From MODIS and AVHRR Surface Reflectance, IEEE T. Geosci. Remote, 54, 5301–5318, https://doi.org/10.1109/TGRS.2016.2560522, 2016. a, b
Yang, Z.-L., Niu, G.-Y., Mitchell, K., Chen, F., Ek, M., Barlage, M., Longuevergne, L., Manning, K., Niyogi, D., Tewari, M., and Xia, Y.: The community Noah land surface model with multiparameterization options (Noah-MP): 2. Evaluation over global river basins, J. Geophys. Res.-Atmos., 116, D12110, https://doi.org/10.1029/2010JD015140, 2011. a
Zheng, G. and Moskal, L.: Retrieving Leaf Area Index (LAI) Using Remote Sensing: Theories, Methods and Sensors, Sensors, 9, 2719–2745, https://doi.org/10.3390/s90402719, 2009. a