the Creative Commons Attribution 4.0 License.
the Creative Commons Attribution 4.0 License.
Hydraulic characterisation of iron-oxide-coated sand and gravel based on nuclear magnetic resonance relaxation mode analyses
Christoph Weidner
Mike Müller-Petke
Georg Houben
The capability of nuclear magnetic resonance (NMR) relaxometry to characterise hydraulic properties of iron-oxide-coated sand and gravel was evaluated in a laboratory study. Past studies have shown that the presence of paramagnetic iron oxides and large pores in coarse sand and gravel disturbs the otherwise linear relationship between relaxation time and pore size. Consequently, the commonly applied empirical approaches fail when deriving hydraulic quantities from NMR parameters. Recent research demonstrates that higher relaxation modes must be taken into account to relate the size of a large pore to its NMR relaxation behaviour in the presence of significant paramagnetic impurities at its pore wall. We performed NMR relaxation experiments with water-saturated natural and reworked sands and gravels, coated with natural and synthetic ferric oxides (goethite, ferrihydrite), and show that the impact of the higher relaxation modes increases significantly with increasing iron content. Since the investigated materials exhibit narrow pore size distributions, and can thus be described by a virtual bundle of capillaries with identical apparent pore radius, recently presented inversion approaches allow for estimation of a unique solution yielding the apparent capillary radius from the NMR data. We found the NMR-based apparent radii to correspond well to the effective hydraulic radii estimated from the grain size distributions of the samples for the entire range of observed iron contents. Consequently, they can be used to estimate the hydraulic conductivity using the well-known Kozeny–Carman equation without any calibration that is otherwise necessary when predicting hydraulic conductivities from NMR data. Our future research will focus on the development of relaxation time models that consider pore size distributions. Furthermore, we plan to establish a measurement system based on borehole NMR for localising iron clogging and controlling its remediation in the gravel pack of groundwater wells.
- Article
(7152 KB) - Full-text XML
-
Supplement
(849 KB) - BibTeX
- EndNote
Iron oxides are, due to their abundance and reactive properties, amongst the most important mineral phases in the geosphere (Cornell and Schwertmann, 2003; Colombo et al., 2014). They encompass a variety of oxides, hydroxides and oxihydroxides of predominantly ferric iron but all are referred to as iron oxides in this study for the sake of brevity. They form some of the most important commercial iron ores worldwide but also play a vital role in soils and aquifers. As weathering products, iron oxides control the conditions for soil genesis and degradation (Stumm and Sulzberger, 1991; Kappler and Straub, 2005) and the mobility of nutrients, trace metals, and contaminants (Cornell and Schwertmann, 2003; Colombo et al., 2014; Cundy et al., 2014). Particularly in many tropic and subtropic soils, the building processes of iron oxide exhibit high temporal dynamics and may change the environmental conditions within a few years, which makes it necessary to further develop measurement techniques to characterise and monitor the corresponding status of soils and aquifers.
Furthermore, iron oxides play a negative role when forming in wells and drains used for the extraction of fluids from the subsurface, e.g. in drinking water production, oil wells, dewatering of mines or bogs, landfill leachate collection systems, and geothermal energy systems (Houben, 2003a; Larroque and Franceschi, 2011; Medina et al., 2013). The formation of iron oxide incrustations negatively affects the performance of these systems by blocking the entrance openings and the pore space of gravel pack and formation (Weidner et al., 2012). The removal of such deposits is expensive and time-consuming. Their spatial distribution is often inhomogeneous (Houben and Weihe, 2010; Weidner, 2016). It is therefore imperative to identify their exact location and to characterise their degree of clogging to successfully target rehabilitation measures. Ideally, this is to be done before the incrustation gained a state at which fluid movement through the pore space is significantly hindered in order to ensure maximum chance of success of the remediation activities. Although the chemical (Stumm and Lee, 1960; Pham and Waite, 2008; Geroni and Sapsford, 2011; Larese-Casanova et al., 2012) and biological processes (Tuhela et al., 1997; Cullimore, 2000; Emerson et al., 2010) involved are well investigated, accurate methods for identifying and characterising the location and degree of in situ iron mineralisation are still not available.
Geophysical field and borehole methods have the potential to comply with this demand. Methods such as electrical resistivity tomography, electromagnetics, and ground-penetrating radar are sensitive to different phases and concentrations of iron oxides in the pore space (e.g. Van Dam et al., 2002; Atekwana and Slater, 2009; Abdel Aal et al., 2009). The same is true for the method of nuclear magnetic resonance (NMR, e.g. Bryar et al., 2000; Keating and Knight, 2007, 2008, 2010). The aim of this laboratory study is to assess the potential of NMR for identifying the location and concentration of iron oxide coatings in water-saturated porous media and the assessment of their hydraulic effects.
Geophysical applications of NMR relaxometry are used in hydrocarbon exploration, hydrogeology, and environmental and soil sciences for estimating pore liquid contents, pore size distributions, and permeability. When applied in boreholes and a laboratory setting, NMR is able to identify different pore fluid components, e.g. water and oil (e.g. Bryar and Knight, 2003; Hertzog et al., 2007), to distinguish between clay-bound, capillary-bound and mobile pore water (e.g. Prammer et al., 1996; Coates et al., 1999; Dunn et al., 2002), and to provide hydraulic and soil physical parameters (e.g. Dlugosch et al., 2013; Costabel and Yaramanci, 2011, 2013; Sucre et al., 2011; Knight et al., 2016). As a non-invasive subsurface tool, it is used for investigating the subsurface distributions of water content and hydraulic conductivity and allows for the lithological categorisation of aquifers and aquitards (e.g. Legchenko et al., 2004; Costabel et al., 2017).
NMR relaxometry for hydraulic characterisation of porous media takes advantage of the paramagnetic properties of the pore surface. The NMR measurement observes the exchange of energy between stimulated proton spins of the pore fluid and the pore walls and thereby provides a proxy for pore surface-to-volume ratios, i.e. pore sizes. However, existing approaches to estimating pore sizes and permeabilities demand material-specific calibration (Kenyon, 1997; Coates et al., 1999), which is expected to be particularly difficult for materials containing a large amount of paramagnetic species (Keating and Knight, 2007). Moreover, NMR relaxation measurements are affected by additional effects such as the occurrence of additional energy losses within the pore fluid (Bryar et al., 2000; Bryar and Knight, 2002), ferromagnetism and corresponding disturbances of the magnetic fields (Keating and Knight, 2007, 2008), and the existence of pore geometries with a high level of complexity, e.g. capillaries with angular cross sections or fractal pore surfaces (Sapoval et al., 1996; Mohnke et al., 2015; Müller-Petke et al., 2015). Different iron oxide phases can produce any of these effects and can thus significantly bias the results. Foley et al. (1996) demonstrated for instance that the amount of paramagnetic iron minerals is linearly correlated with the NMR relaxation rate for materials with otherwise identical pore space. Keating and Knight (2007, 2010) found that NMR relaxation is not only influenced by the amount but also by the specific kind of iron oxide mineral. Additional complexity might occur if paramagnetic and ferromagnetic particles accumulate inhomogeneously inside the pore space (Grunewald and Knight, 2011; Keating and Knight, 2012).
In this study, we investigate the effects of paramagnetic iron oxide coatings, particularly for coarse material. For large pores in the so-called slow diffusion regime, the otherwise linear relationship between relaxation time and pore size is disturbed because higher relaxation modes become relevant (Brownstein and Tarr, 1979; Müller-Petke et al., 2015). As a significant consequence, the common interpretation schemes to estimate pore size and hydraulic conductivity are not valid anymore. Past studies dealing with iron mineral coatings reported the occurrence of slow diffusion conditions during their NMR experiments (Keating and Knight, 2010; Grunewald and Knight, 2011). Our objective is to learn how to interpret NMR data also under these conditions and how to estimate hydraulic parameters from it. Therefore, the goals of this study are as follows:
-
to investigate the NMR relaxation behaviour as a function of the content of paramagnetic iron oxide for large pores;
-
to correlate NMR relaxation parameters with hydraulically effective parameters;
-
to assess the model published by Müller-Petke et al. (2015) in the context of iron-coated sediments, which is the first NMR interpretation approach that considers higher relaxation modes.
We investigate two different sets of iron-oxide-coated samples. The first set consists of commercially available filter sand that was coated with different amounts of synthetic ferrihydrite and goethite. Using this set (Set A), we study the general impact of increasing iron concentration on the NMR relaxation behaviour and investigate how sensitive the measured NMR signature is with regard to the mineral type. The second set consists of filter sand and gravel with natural iron oxide incrustations and material taken from the clogging experiments of Weidner (2016), who investigated the influence of chemical iron-clogging on the hydraulic conductivity of gravel pack material in a sand tank model. The iron oxide content of these samples consists of different amounts of ferric oxide minerals, including ferrihydrite and goethite. Using this set (Set B), we test the general potential of NMR to provide a reliable proxy for hydraulic conductivity even with the content of individual paramagnetic iron oxides varying arbitrarily.
2.1 Principle of NMR relaxometry
The measurement principle is based on the manipulation of hydrogen protons (e.g. in water molecules). They exhibit a magnetic momentum due to their proton spins. When an ensemble of proton spins is exposed to a permanent magnetic field B0, an additional (nuclear) magnetisation M is formed and aligned with B0. By electromagnetic stimulation (excitation) using an external field B1 that alternates the Larmor frequency of proton spins, M can be forced to deflect from its equilibrium position. After shutting off the excitation, the movement of M back to equilibrium is observed. This process is called NMR relaxation and the resulting signal, recorded as induced voltage in a receiver coil, is an exponential decrease (transverse or T2 relaxation) when measured perpendicular to B0. When observed parallel to B0, the signal increases correspondingly (longitudinal or T1 relaxation). Detailed information on theory and measurement techniques is found in, for example, Coates et al. (1999) and Dunn et al. (2002).
2.2 NMR relaxation in general
Because only the hydrogen proton spins of the pore water molecules contribute to the NMR signal, its amplitude is a measure for the water content of the investigated material, while the relaxation behaviour encodes relevant information on the pore environment. The NMR signal E (V) as a function of the measurement time t is described by
and
for the T1 and T2 relaxation, respectively. E0 is the initial amplitude (V), while In and (i = 1, 2) denote the relative intensity (no units) and relaxation time (s) of the nth relaxation regime.
When considering the T1 relaxation, the relaxation rate 1 is given by
where 1 and describe the relaxation rates of the pure pore water excluding the influence of the pore walls (bulk relaxation) and the interaction of the proton spins with the pore surface (surface relaxation), respectively. For the general description of the T2 relaxation, an additional term must be included:
The rates 1 and are the same as for the T1 relaxation, whereas the considers the case of an inhomogeneous B0 field. The diffusion relaxation must be taken into account, if a significant quantity of ferromagnetic minerals is present (Keating and Knight, 2007, 2008) or if the sensitive volume of the measurement includes a significant gradient in B0 (Blümich et al., 2008; Perlo et al., 2013). However, for the estimation of hydraulic properties from NMR, the surface relaxation is the most interesting phenomenon.
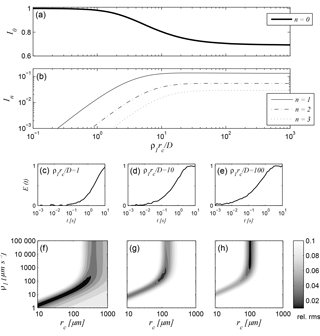
Figure 1(a, b) Intensities of the zeroth to third relaxation modes as functions of the relationship ρ1rc∕D visualising the different diffusion regimes in which NMR relaxation can take place, (c)–(e) simulated T1 relaxation data for a capillary with (c) rc = 100 µm and ρ1 = 20 µm s−1, (d) rc = 100 µm and ρ1 = 200 µm s−1, and (e) rc = 100 µm and ρ1 = 2000 µm s−1, (f)–(h) corresponding results of a parameter search regarding rc and ρ1. The NMR time series was contaminated by Gaussian-distributed random noise with an amplitude of 0.01.
Brownstein and Tarr (1979) derived the NMR relaxation behaviour in restricted environments for simple pore geometries (planar, cylindrical, and spherical). In this study, we consider the corresponding relaxation inside a cylindrical capillary with radius rc, which exhibits different relaxation modes:
D refers to the self-diffusion coefficient of water (m2 s−1) and ρi to the surface relaxivity (m s−1) for either the longitudinal (i = 1) or the transverse (i = 2) relaxation, which is a material constant describing the influence of paramagnetic minerals at the pore surface. J0 and J1 are the Bessel functions of the zeroth and first order, respectively. The quantities ξn can only be found by calculating the positive roots of the corresponding equation numerically. The intensities In are given by
According to Brownstein and Tarr (1979), the term ρirc∕D in Eq. (5) defines a controlling criterion that distinguishes between the fast (ρirc∕D ≪ 1), intermediate (1 < ρirc∕D < 10), and slow (ρirc∕D > 10) diffusion regimes. Figure 1a and b demonstrate the relative intensities In of the zeroth to third modes as functions of ρirc∕D for all diffusion regimes. Obviously, the zeroth mode I0 is the only relevant relaxation component taking place in the fast diffusion range, because the intensities of the higher modes can be neglected, i.e. the relaxation is mono-modal inside the considered pore. The phenomenological explanation for this feature is that all proton spins in the pore space diffuse fast enough to sample the entire pore surface during the NMR relaxation measurement, which is the case for small pores and low surface relaxivities. The common empirical approaches to provide hydraulic conductivity estimates (e.g. Kenyon, 1997; Coates et al., 1999; Knight et al., 2016) and pore size distributions (e.g. Hinedi et al., 1997; Costabel and Yaramanci, 2013) are only valid if this condition is satisfied: the zeroth mode in Eq. (5) simplifies to = rc∕2ρi and, given that ρi can be determined by calibration, becomes a unique proxy for a certain pore (capillary) radius.
Outside the fast diffusion regime, the intensities In for n > 1 increase (Fig. 1a and b), while I0 decreases asymptotically to about 0.7. In materials with large pores and/or high surface relaxivities, the self-diffusion of the proton spins is slow in regard to the mean distance to the pore surface and thus, the excited protons do not equally get in touch with the pore surface. Protons in the direct vicinity of the surface exchange their spin magnetisation faster than those within the pore body. The consequence is a multi-exponential (i.e. multi-modal) relaxation inside the pore. The theory of Brownstein and Tarr (1979) leads to the simplification of ξn = (n + 1∕2)2π2 in Eq. (5) describing the asymptotic behaviour in the slow diffusion regime. This is in principle a significant advantage regarding the estimation of pore radii from relaxation times, because a calibration regarding ρi is not necessary. However, natural unconsolidated sediments exhibit a large range of pore sizes, which are seldom completely in the slow diffusion regime. Thus, a close description of the problem is desired that considers all diffusion regimes at once.
2.3 Analysis of relaxation modes
The pore space of a well-sorted porous material has a narrow pore size distribution that can be described using a single effective pore radius (reff). For this case, Müller-Petke et al. (2015) showed that the consideration of relaxation modes as defined in Eqs. (5) and (6) leads to an unambiguous prediction of pore radius and surface relaxivity in the intermediate diffusion regime. In this study, we use this concept to interpret, i.e. to approximate, our NMR relaxation measurements. As demonstrated in the following section, the investigated sample material in this study allows the assumption of a single reff to describe the pore space. We accept the limitation on a single effective pore radius for the benefit of a closed model that includes the relaxation modes outside the fast diffusion regime on the one hand and that does not demand a priori information on the diffusion regime or calibration of ρi on the other.
However, depending on the actual diffusion regime of the sample, the performance of the approximation procedure as well as the general results differ significantly. To demonstrate the corresponding effects, we calculated the synthetic T1 relaxation response signals according to Eqs. (1), (5), and (6) for a cylindrical pore with a radius rc = 100 µm and surface relaxivities ρ1 = 20, 200, 2000 µm s−1. The positions of these three parameter combinations in Fig. 1a and b show that they represent one specimen for each relevant setting of the relaxation modes: the first at ρirc∕D = 1, where I0 is close to 1; the second at ρirc∕D = 10, where the corresponding I0 lays inside the decreasing range; and the third at ρirc∕D = 100, where I0 has reached the asymptote. The initial amplitudes E0 of the synthetic signals were set to 1 and the resulting synthetic signals are exposed to a Gaussian-distributed noise with an amplitude of 0.01 (Fig. 1c–e).
Figure 1f to h show the results of a parameter search for each of the three cases as surface plots (i.e. their objective functions), where the surface height demonstrates the relative root mean square (rms) value of each combination of ρ1 and rc within the search region. The black region in each figure demonstrates the area, where the resulting rms value is 0.01, i.e. where the corresponding parameter combinations lead to a reliable approximation of the original signal within its noise level. According to the findings of Müller-Petke et al. (2015), a unique solution for both parameters can only be found for the signal at ρirc∕D = 10 (Fig. 1g). The fast diffusion regime in Fig. 1f is characterised by an ambiguous region demonstrating the linear relationship of ρ1 and rc, while the solution of the third signal at ρirc∕D = 100 is independent of ρ1 (Fig. 1h). Two important facts can be deduced from Fig. 1h: first, by performing a parameter search for NMR relaxation measurements under very slow diffusion conditions, only a minimum of ρ1 can be determined, and, second, an adequate approximation algorithm based on the mode interpretation of NMR relaxation will always provide a reliable estimate of rc outside the fast diffusion region, while the corresponding ρ1 estimate becomes more and more inaccurate when passing through the slow diffusion regime.
In contrast to ferromagnetic impurities that mainly affect the diffusion relaxation by small-scaled disturbances of the magnetic fields involved, the appearance of purely paramagnetic iron mineral coatings is expected to cause an increase in ρi and thus a faster relaxation (e.g. Foley et al., 1996; Keating and Knight, 2007). However, iron oxides are known to have large surface areas (e.g. Houben and Kaufhold, 2011) and will consequently affect the NMR relaxation also by an increasing pore surface-to-volume ratio, S∕V (Foley et al., 1996; Müller-Petke et al., 2015). It is generally impossible to relate an observed increase in NMR relaxation unambiguously to either an increase in ρi or to an increase in S∕V without additional information. Along with the general behaviour of relaxation modes, numerical modelling of Müller-Petke et al. (2015) demonstrated that an increasing roughness of the surface inside a capillary with otherwise low and constant ρi leads to a similar relaxation as an increasing surface relaxivity, while keeping the radius unchanged. They introduced and defined the apparent surface relaxivity ρi,app in combination with an apparent pore radius to explain NMR relaxation of porous media with narrow pore size distribution. Following their suggestion, we define ρi,app to include both the effect of an increasing ρi and the corresponding increase in pore surface roughness due to iron oxide coating, while is considered to be the mean radius of the corresponding capillary. The hypothesis demands the assumption that the coating and the corresponding distribution of ρi,app is homogeneously distributed. This is a crucial point, because a perfect homogeneous distribution of iron precipitation on the pore scale due to natural chemical or microbiological processes or even synthetic chemical treatment is questionable. However, regarding the slow NMR relaxation in coarse sediments it is expected that, during the NMR measurement, the diffusing spins statistically sample possible inhomogeneities in the distribution of ρi or ρi,app inside the pore space uniformly enough to allow the assumption of a mean surface relaxivity (Kenyon, 1997; Grunewald and Knight, 2011; Keating and Knight, 2012). An important objective of this study is the comparison of with the effective hydraulic pore radius reff.
3.1 Samples with controlled synthetic ferrihydrite and goethite coating
In the first experimental step, the focus was set on a simplified binary system consisting of (a) a relatively uniform carrier phase, quartz in the form of commercially available filter gravel, and (b) synthetically produced iron oxides. For the latter, ferrihydrite and goethite mineral phases were studied separately, both of which are common constituents in soils and aquifers but also in incrustations. Synthetic iron oxides were used because of their controlled crystallite size and composition (Schwertmann and Cornell, 2000). Ferrihydrite is a poorly crystalline mineral that usually precipitates as the first stable oxidation product when dissolved ferrous iron comes into contact with oxygen. Since ferrihydrite is thermodynamically meta-stable, it will convert over time into the more stable goethite (e.g., Houben and Kaufhold, 2011). This process is strongly accelerated at higher temperatures (> 50 ∘C) and involves a significant reduction of specific surface area and therefore water content, density, and chemical reactivity. Thus, this study does not only encompass two of the most important iron oxides but, at the same time, two different stages of crystallinity, age, and reactivity.
Two series of artificially coated filter sand samples (Set A) were prepared by precipitating the Fe(III)-minerals ferrihydrite and goethite onto quartz following Schwertmann and Cornell (2000). Therefore, iron nitrate nonahydrate (Fe(NO3)3 ⋅ 9H2O; CAS: 7782-61-8, technical purity, BDH Prolabo) was dissolved in twice de-ionised water to attain a 1 mol L−1 solution. A 5 mol L−1 potassium hydroxide solution (KOH, CAS: 1310-58-3, Bernd Kraft) was used to trigger precipitation of ferrihydrite (Fe5HO8 ⋅ 4H2O). The desired contents of iron in the filter sands were realised by varying the amounts of the two solutions, added to a fixed amount of filter sand. After precipitation the residual solution was carefully exchanged by washing with de-ionised water. For transformation of ferrihydrite to goethite (α-FeOOH), a second batch of ferrihydrite was held in a closed glass bottle at 70 ∘C for 60 h. The applied recipes for ferrihydrite and goethite are based on the collection of standard synthesis procedures compiled in the reference book by Schwertmann and Cornell (2000). They have been successfully applied in numerous studies (e.g. Janney et al., 2000; Houben, 2003b; Houben and Kaufhold, 2011).
After preparation, the sample material was filled into circular petri dishes with a diameter of 50 mm and a height of 15 mm to perform the initial NMR measurements. Most of the iron particles settled to the bottom and formed a gradient in iron concentration inside the dishes, which could visually be observed for most of the samples due to an obvious increase in reddish colour from top to bottom. Initial NMR measurements were performed to qualitatively analyse the vertical distribution of the iron content. Therefore, measurements at different heights of the sample holders were conducted. However, for the quantitative analysis of NMR parameters, the samples were homogenised before the final NMR measurements, because it was not possible to determine the amount of iron as a function of height inside the sample holders by chemical analyses. To homogenise the iron content inside the petri dishes, the material was exposed to the atmosphere for 1 day, where it evaporated to a certain state of partial saturation (resulting saturation: 0.2 to 0.5), mixed, and filled into dishes with a diameter of 50 mm and a height of 10 mm. Afterwards, samples were dried completely to ensure a proper coating of the pore walls with the iron particles. To maintain a homogeneous iron distribution throughout the sample and a better adhesion to the quartz surface, the material was moistened (de-ionised water) and dried out again. This procedure was repeated 4 times for each sample. Finally, the samples were completely saturated with de-ionised water prior to the NMR measurements.
After the final NMR measurements, the samples were air-dried again to determine their porosity Φ by weight. Afterwards they were subdivided for the controlling analysis. The iron content of each sample was analysed chemically to identify whether and to what extent the precipitation had led to the desired results. This was done by analysing the amount of dithionite-soluble iron, following the method of Mehra and Jackson (1960). The oxidic iron coatings that are expected to affect the NMR results are re-dissolved with dithionite solution and quantified by measuring the iron concentration in the solution. The total iron content was investigated by X-ray fluorescence analysis (XRF, using a PANalytical Axios and a PW2400 spectrometer) for verification. The latter method is expected to yield slightly higher iron contents, because XRF also captures the iron content bound in silicates of the filter sand or gravel grains. The difference for the samples of Set A indicates an amount of siliceous iron in the range of 0.5 to 0.7 g kg−1. The further analysis is thus based on the actual measured contents of dithionite-soluble iron. The grain size distributions were determined using a CAMSIZER (Retsch GmbH). The specifications of the samples are summarised in Table 1. The comparison of the desired with the actually achieved Fe contents indicates that, during the exchange of the remaining synthesis solutions (Fe(NO3)3 and KOH) with H2Odest, some of the fine precipitates have been washed out. A part of each sample was also prepared for the determination of the specific surface area using the BET method (Brunauer et al., 1938). However, the corresponding results fell below the accuracy limit of the device and are not reliable. Obviously, the contents of iron oxide in the investigated samples are too small and the surface area is still dominated by the quartz grains.
Table 1List of samples with synthetic ferrihydrite (F) and goethite (G) coating (Set A).
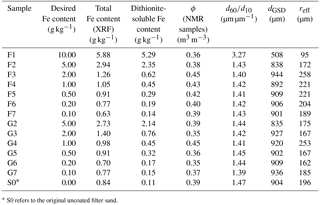
* S0 refers to the original uncoated filter sand.
Table 2List of samples with artificial and natural iron clogging (Set B).
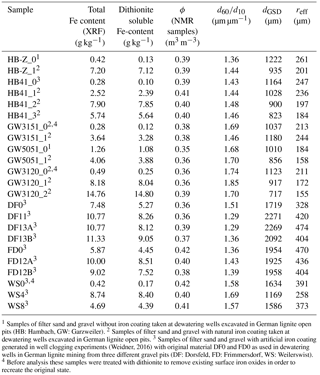
1 Samples of filter sand and gravel without iron coating taken at dewatering wells excavated in German lignite open pits (HB: Hambach, GW: Garzweiler). 2 Samples of filter sand and gravel with natural iron coating taken at dewatering wells excavated in German lignite open pits. 3 Samples of filter sand and gravel with artificial iron coating generated in well clogging experiments (Weidner, 2016) with original material DF0 and FD0 as used in dewatering wells in German lignite mining from three different gravel pits (DF: Dorsfeld, FD: Frimmersdorf, WS: Weilerswist). 4 Before analysis these samples were treated with dithionite to remove existing surface iron oxides in order to recreate the original state.
3.2 Samples with natural iron coating
A second set of samples with natural iron coatings was also studied (Set B, Table 2). This set consists of gravel samples from laboratory well clogging experiments (Weidner, 2016), but also encrusted filter sand and gravel samples taken from excavated wells. The analyses were the same as for Set A.
3.3 Estimation of effective pore radius and hydraulic conductivity from grain size distribution
To obtain consistent reference values for comparison with the NMR results, we estimated the effective pore radius from the effective grain diameter dGSD as defined by Carrier (2003), who suggested the use of the equations of Kozeny (1927) and Carman (1939) to estimate the hydraulic conductivity from grain size distribution (GSD) data:
where fi refers to the ith weight fraction of grains within the respective sieve size limits Dli and Dui with = 1.
To estimate the effective pore radius reff from dGSD, we determine the ratio of the wetted surface to the pore volume (= specific surface) for both the capillary geometry of our pore model and the spherical geometry assumed for the effective grain diameter:
with ϕ being the porosity. The effective pore radius is then given by the following:
The Kozeny–Carman equation, when considering a cylindrical capillary with effective radius reff, is defined as follows (e.g. Pape et al., 2006):
The parameter τ refers to the tortuosity (no units), g to the gravity acceleration (9.81 m s−2), and ϱ and η to the density (1000 kg m−3) and dynamic viscosity (1 g m−1 s−1) of the pore water, respectively. The tortuosity is set to 1.5 in this study, which is a reliable estimate for coarse sand and gravel (e.g. Pape et al., 2006; Dlugosch et al., 2013).
An alternative to the semi-empirical Kozeny–Carman equation is the well-known empirical formula of Hazen (1892). The effective measure in this approach is assumed to be the grain diameter corresponding to the 10 wt % percentile of the cumulative GSD (d10). The corresponding estimates of hydraulic conductivity KHz were used as an additional set of reference values.
3.4 NMR measurements
As described above in Sect. 3.1, the stimulated precipitation yielded an obvious vertical gradient in iron oxide content. To identify the corresponding level of heterogeneity and to control and verify the homogeneity of the iron oxide distribution after the final mixing, an NMR device with vertical sensitivity, i.e. the ability to apply distinct measurements at different heights of the sample holder had to be applied. Using a common NMR core analyser, the entire specimen is measured at once, which can lead to a misinterpretation if different relaxation regimes overlap. Therefore, the experiments in this study were realised using a single-sided NMR apparatus (NMR Mouse, Magritek) with strong sensitivity to vertical changes inside the sample (Fig. 2). Four permanent magnets for the B0 and the measurement coil for the B1 field are arranged in a way that the sensitive volume is as a slice with a thickness of 200 µm and a footprint of about 40 by 40 mm (Kolz et al., 2007; Blümich et al., 2008). The operating frequency is 13.05 MHz. The sample is placed on a table, while the sensor is mounted on a platform adjustable in height, i.e. to move the sensitive volume over the sample (along the z axis) with an accuracy of a few micrometres.
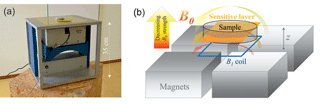
Figure 2(a) Measurement device and (b) schematic showing the configuration of the permanent B0 magnets, B1 coil, and the resulting sensitive layer.
Although homogeneous in the plane parallel to the B1 coil, the B0 field strength decreases with increasing distance to the magnets, which yields a strong B0 gradient in the z direction (mean gradient according to user's manual: 273 kHz mm−1) inside the sensitive slide. Consequently, the T2 measurements (CPMG sequence, for details please see Coates et al., 1999 and Dunn et al., 2002) are dominated by the diffusion relaxation rate. In principle, this effect can be corrected to identify the proportion of surface relaxation in the data (Keating and Knight, 2008). However, testing and discussion of the quality and potential of the additional measurements and calculations necessary for this correction are beyond the scope of this paper. Thus, we use the T2 measurements only for determining the NMR porosity ΦNMR from the initial amplitude of the corresponding exponential decay. Due to the linearity between NMR signal and water content inside the sensitive volume of the measurement (e.g. Costabel and Yaramanci, 2011; Behroozmand et al., 2014), ΦNMR can simply be determined by the ratio of the initial amplitude of the investigated sample and that of pure water in a sample holder with exactly the same dimensions. The CPMG measurements were conducted with an echo time of 66 µs, while the total number of echoes was varied individually between 3000 and 9000. The corresponding measurement times vary in a range of about 0.2 to 0.6 s.
For investigating the impact of the iron oxide coating, we use the T1 relaxation, which is unaffected by gradients in B0. These measurements are realised as saturation recovery (SR) measurements (details see Coates et al., 1999 and Dunn et al., 2002). Each record consists of 50 single recovery times, which are logarithmically spaced along the measurement time axis. The exact positioning of the recovery times was adjusted for each sample to realise a similar distribution of time samples from zero to equilibrium nuclear magnetisation, which was estimated beforehand by screening SR measurements with a reduced number of time samples (15) and stacks. The maximum observation time for the final SR measurements was set 5 times higher than the prior T1 estimates. For each sample, SR measurements at different heights were conducted using 1 mm steps in the range of z = 3 to 15 mm before and z = 3 to 10 mm after homogenisation. In this way, the vertical distribution of iron inside the samples before homogenisation and the natural scattering of the NMR parameters after homogenisation were taken into account. For the latter, mean values and double standard deviations (95 % confidence interval) were calculated from the measurements at different heights. After the T1 measurements, a small sample of pore water (a few tenths of a millilitre) was extracted from the samples using a pipette in order to measure Tbulk. In some cases the extracted amount of pore water was not high enough to achieve a sufficient signal-to-noise ratio for an accurate NMR measurement. However, the Tbulk values of the successful measurements did not vary significantly among the samples. Consequently, for the analysis of the relaxation behaviour (Eq. 3) we use a mean Tbulk (2.46 ms ± 0.07 ms) for all samples.
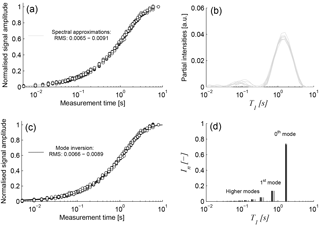
Figure 3Panels (a) and (c): normalised T1 measurements at different heights of sample F4 after homogenisation and corresponding approximations using (b) multi-exponential spectrum and (d) relaxation modes.
Because the NMR porosity was determined from the T2 measurements, it was not necessary to take the initial amplitude of the T1 measurements into account. Thus, each SR time series was normalised to 1 prior to the final signal approximation. Although the main focus of our interpretation is on the approximation using the relaxation modes, we also fitted the data using the commonly used multi-exponential spectral inversion for comparison. As an example, Fig. 3a shows all T1 measurements of the homogenised sample F4, i.e. all repetitions at different heights, and their approximations using the spectral approach. The corresponding spectra, depicted in Fig. 3b, demonstrate that the probability functions of all repeated T1 data are in good agreement. They show a dominating peak with a maximum at about 1.3 s and a smaller peak around 0.1 s.
3.5 Testing for NMR diffusion regimes
The analysis of relaxation modes is useful only outside the fast diffusion regime. Thus, the question arises as to how the diffusion regime can be tested in practice. According to Kenyon (1997), the diffusion condition inside a pore is defined by the ratio of the time for a proton spin to diffuse across the pore (= ) and the surface relaxation time:
Using the logarithmic mean of the measured relaxation spectra T1,lm, the self-diffusion coefficient of water, and accepting reff as a reliable estimate of rc, we combine Eq. (11) with Eq. (3) to determine a measure that can be used for practical testing of the diffusion regime:
3.6 Inversion of NMR relaxation modes
The uniformity coefficient is defined by the ratio of the grain diameters corresponding to the 60 and 10 wt % percentiles of the cumulative GSD. For all samples investigated in this study it is very low (i.e. < 5, see Tables 1 and 2), which indicates a narrow grain size and consequently narrow pore size distribution (see also Fig. S1 in the Supplement). Thus, the precondition to use the approach of Müller-Petke et al. (2015) (see Sect. 2.3) to fit and interpret the NMR data is fulfilled. The approximation algorithm, i.e. the data inversion yielding the relaxation modes,
-
starts using an initial model with given ρ1,app and ;
-
calculates the corresponding multi-exponential NMR response by solving Eqs. (3), (5), and (6);
-
compares the result with the measured NMR signal by means of least squares;
-
modifies the parameters ρ1,app and if necessary, that is if the modelled response and the measurement do not coincide; and
-
repeats the procedure until an optimal parameter set ρ1,app and is found that explains the data.
We use the nonlinear solver lsqnonlin of the MATLAB® optimisation toolbox (MATLAB®, 2016) for this processing step.
Figure 3c shows the same data as Fig. 3a, but together with the approximations resulting from the relaxation mode inversion that obviously lead to identical fits compared to the spectral inversion. Figure 3d shows the corresponding results in the In − T1 domain, that is, the first 10 modes for each measurement as separate spectral lines. The accuracy of the approximations using the relaxation modes represented by the corresponding rms values are similar to the ones of the spectral inversion.
4.1 NMR-based porosity measurements
As mentioned above, to determine ΦNMR of a sample, an additional NMR measurement using pure water is necessary. Figure 4a shows the T2 data of sample F4 (synthetic ferrihydrite on quartz) and pure water. Due to the diffusion relaxation, the latter exhibits a relaxation time of less than 0.2 s, which is much shorter than that usually measured for water (2–3 s) in a homogeneous B0. Because the initial signal amplitudes are not affected by the B0 gradient, ΦNMR can nevertheless be estimated from the T2 data. Figure 4b shows the NMR-based porosities of all samples after homogenisation compared to those measured by weight. The NMR porosities coincide with the reference values within their uncertainties, which are determined as doubled standard deviations (95 % confidence interval) of the measurement repetitions at different sample heights. However, the uncertainties of the ΦNMR estimates measured using the single-sided NMR device in this study are larger than those of past studies, where conventional laboratory NMR techniques are applied (e.g. Costabel and Yaramanci, 2011; Behroozmand et al., 2014). The reason for this is the relatively thin sensitive slice of 200 µm in combination with the investigated coarse material exhibiting mean reff values of 95 to 474 µm (see Tables 1 and 2). The inaccuracy of the porosity estimates must be accepted as a natural consequence of the fact that some of the observed pores exceed the z dimension of the probed reference volume (e.g. Costanza-Robinson et al., 2011).
4.2 The logarithmic mean of relaxation as qualitative measure for iron content at the pore walls
A photograph of sample F4 after the ferrihydrite precipitation is shown in Fig. 5a. The reddish section indicates that most ferrihydrite particles settled at the bottom of the petri dish. The same phenomenon was optically observed for almost all samples of Set A. Even though this separation was not visibly apparent in samples F1, F2, and G2 with the highest iron contents, we still expected a gradient in the iron content with z direction for these samples as well. Although not quantifiable to date, it is expected that the mean NMR relaxation time depends on the amount of paramagnetic iron oxides in the pore space (Keating and Knight, 2007). Thus, we performed initial NMR measurements (T1 and T2) to qualitatively analyse the level of inhomogeneity in the vertical ferrihydrite and goethite distributions by comparing the NMR parameters at different heights over the sample holders. Figure 5b and c depict the NMR data of sample F4 and those of the pure uncoated filter sand (sample S0), that is, the corresponding porosity determined from the E0 amplitude of the CPMG data and the distributions of the logarithmic mean relaxation times (T1,lm and T2,lm), respectively. Apart from a decrease at the top, the porosity distributions of both samples are homogeneous. It is likely that the decrease at the top is caused by evaporation caused by an imperfect sealing of the sample. The same feature was observed for all samples of Set A to varying extent. Figures S2–S16 show the photographs of all samples compared to the corresponding distributions of porosity and mean relaxation times. Some of the samples also show a significant decrease in porosity at the bottom of the sample holder, which is caused by small iron oxide particles accumulating in the voids between the quartz grains.
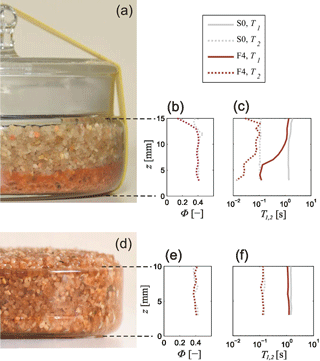
Figure 5(a) Sample F4 after chemical treatment and precipitation of ferrihydrite particles at the bottom of the sample holder. Panels (b) and (c): vertical distributions of corresponding porosities Φ and mean relaxation times T1 and T2, compared to those of untreated sand S0. Panels (d)–(f): sample F4 after homogenisation and corresponding distributions of Φ and T1,2.
Whereas both the T1,lm and T2,lm distributions of the uncoated sample S0 appear to be homogeneous throughout the z axis, the general trend in the distributions of sample F4 is a gradual decrease from top to bottom (Fig. 5c), indicating the increase in surface relaxation with increasing ferrihydrite content. The difference between T1 and T2 is about 1 order of magnitude, which is caused by the high diffusion relaxation rate in the inhomogeneous B0 field of the single-sided NMR apparatus, as expected (see Sect. 3.4). When comparing the T1,lm and T2,lm curves of F4 with S0, it seems that no ferrihydrite remains at the top, because here the curves of both samples are almost in agreement. Although we cannot quantify the ferrihydrite content as a function of z by chemical analyses, we note that the logarithmic means of both T1 and T2 are qualified proxies for the corresponding iron content distributions.
Table 3Estimates of κ according to Eq. (12) for the samples with artificial ferrihydrite and goethite coatings (Set A).
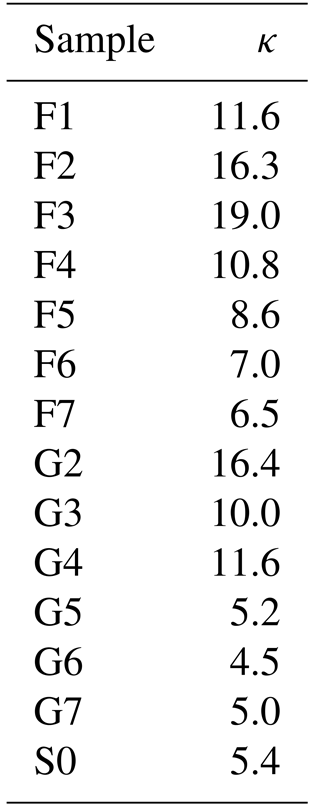
To relate the measured NMR parameters with the iron content, the samples had to be homogenised (see Sect. 3.1). Obviously, both the T1,lm and T2,lm distribution of the homogenised F4 sample are almost constant with z (Fig. 5d–f). The T1,lm values of F4 are generally smaller than the ones of S0. In contrast, the T2,lm distributions of F4 and S0 are almost identical, which is due to the influence of the high diffusion relaxation that masks the impact of the ferrihydrite content on the surface relaxation. As for the inhomogeneous sample, the porosity distributions of F4 and S0 are almost identical, i.e. an obvious impact of the increased content of ferrihydrite on the porosity is not observed. The process of homogenisation was applied and controlled for each sample of Set A. Figures S17–S31 show the corresponding distributions of porosity and mean relaxation times as functions of sample height for all samples. The remaining scattering of the z-dependent NMR parameters is considered as uncertainty intervals depicted by error bars (95 % confidence intervals) in the following analysis.
In Fig. 6, we show the relaxation time spectra of all samples of Set A and their corresponding mean values as a function of iron content. The principle trend is the same for both minerals. For iron contents smaller than approximately 0.7 g kg−1, the main peak (between approximately 0.5 and 4 s) does not change significantly, whereas the logarithmic mean slightly decreases with increasing iron content in the same range. This increase is caused by an increase in the smaller peak (between approximately 0.05 and 0.2 s). If the iron content increases further to values of 1 g kg−1 and higher, the main peak shifts towards shorter time periods, while the increase in the smaller peak continues. Considering the classical interpretation of NMR relaxation spectra, it is not clear at this point whether the described changes of the spectra with increasing iron content are caused by an increasing amount of small pores (possibly within the iron minerals at the pore walls), by enhanced surface relaxivity (due to the increasing amount of paramagnetic coating), or by a combination of both. However, because all samples, including the initial iron-free sand, are outside the fast diffusion regime (see Table 3), we must also consider that the increase in the smaller peak might be due to the increasing occurrence of the higher relaxation modes. Since it is not possible to distinguish between the existence of relaxation modes and different pore sizes when considering the spectral approximation approach, we analyse the relaxation modes in the next section by considering a bundle of capillaries with identical pore radius (= apparent pore radius ; see details in Sec. 2.3). This assumption is acceptable because the grain size distribution and consequently also the pore size distribution is narrow for the well-sorted materials studied here, which is proven by their small uniformity coefficient d60∕d10 (see Tables 1 and 2).
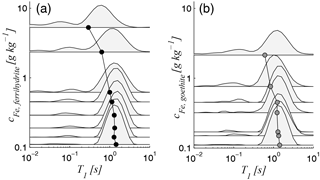
Figure 6Relaxation time spectra as functions of Fe content for (a) ferrihydrite and (b) goethite samples (Set A); the circles mark the logarithmic mean for each spectrum.
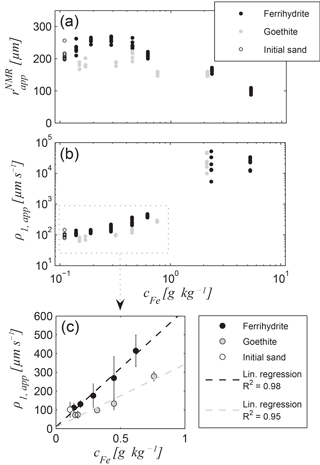
Figure 7Results of relaxation mode inversion for the ferrihydrite and goethite data sets (Set A): (a) apparent pore radius and (b) apparent surface relaxivity ρ1,app as functions of iron content, (c) the mean values and 95 % confidence intervals as error bars for Fe contents smaller than 1 g kg−1 and corresponding linear regression lines; regression coefficient for the ferrihydrite series: 646 µm s−1 ppm−1 (offset: 8.7 µm s−1) and for goethite series: 349 µm s−1 ppm−1 (offset: 9.5 µm s−1).
4.3 The relaxation modes as quantitative measure for iron content at the pore walls
The relaxation mode inversion was performed for all T1 data of Set A and B samples. When considering the relaxation modes (see Sect. 2.3), the underlying model consists of the apparent pore radius of a virtual capillary with a circular cross section and a rough surface, the NMR sink rate of which is described by the apparent surface relaxivity ρ1,app (Müller-Petke et al., 2015). The corresponding and ρ1,app results for Set A are presented in Fig. 7a and b, respectively. All results of the individual measurements for each sample (= measurement at different heights) are depicted in order to avoid error bars in the logarithmic plot. We note that generally tends to smaller values for increasing iron content. However, the trend is only obvious for the iron contents higher than 0.5 g kg−1. At least for the ferrihydrite series, the values even increase slightly for small iron contents, whereas the of the goethite series remains more or less constant. The reason for this variation is likely due to the repacking of the samples after iron oxide precipitation. Considering an initially homogeneous porosity before iron precipitation, one would expect a decrease in porosity with an increasing amount of iron oxide. However, due to the repacking, each sample exhibits an individual porosity. Consequently, the apparent radius, no matter whether it was estimated by NMR or from GSD, also reflects the porosity variations, which covers the dependence on the iron content to some extent. Thus, the expected increase in becomes visible only for the higher iron contents. Interestingly, the estimates of ρ1,app seem to be independent from the individual porosities. Figure 7b shows a monotonous increase in ρ1,app with iron content, at least for the samples with iron contents of < 1 g kg−1. For the higher iron contents, ρ1,app exhibits large uncertainties, because these reach the range where correct ρ1,app estimates cannot reliably be provided anymore (see Fig. 1 and corresponding discussion).
It is expected that a linear dependence between the surface relaxivity and the content of paramagnetic impurities at the pore walls exists (Foley et al., 1996). To test this expectation for the apparent surface relaxivity, Fig. 7c provides a focus on the data with accurate ρ1,app estimates, i.e. the data of samples with iron contents < 1 g kg−1. The linear regression can be verified with R2 values of 0.98 and 0.95 for the ferrihydrite and the goethite series, respectively. We note that the ρ1,app estimates for the goethite series are smaller than those for the ferrihydrite series by a factor of 1.85. We assume that this is an effect of the specific surface area of goethite being about up to 5 times smaller than that of ferrihydrite (goethite ≈ 20–80 m2 g−1 vs. ferrihydrite ≈ 180–300m2 g−1; Cornell and Schwertmann, 2003; Houben and Kaufhold, 2011). The larger specific surface of ferrihydrite leads to a higher surface roughness of the pore wall coating. As explained in Sect. 2.3, the apparent surface relaxivity does not distinguish between the increase in the surface roughness and increase in the actual surface relaxivity due to paramagnetic impurities at the pore wall. Because both are naturally linked to each other in an iron mineral by its individual surface area, we also expect an indirect sensitivity of ρ1,app on the type of iron mineral, i.e. on the composition of the iron oxide assemblage, if considering natural samples. However, to verify this assumption more iron oxides and their influence on the NMR relaxation modes must be studied in the future. Moreover, an accurate inspection of Fig. 7c leads to the assumption that a slight systematic discrepancy from linearity exists for both data sets. We hypothesise that this phenomenon is also caused by the influence of the surface roughness. We have found quadratic relationships yielding regression coefficients of 1 for both data sets. However, each of our data sets consists of just five points, which is not sufficient to validate this finding. Further research is necessary to quantify the influence of the surface roughness on the apparent surface relaxivity for natural iron coatings.
4.4 Comparison of NMR-effective pore radius and hydraulic parameters
Whether the NMR-based estimates of can be considered to be reliable estimates of the effective hydraulic radius reff is examined in the cross plot in Fig. 8. The linear correlation between the two is verified with an R2 of 0.58 when considering a constant offset (regression coefficient: 0.79) and 0.53 when enforcing the point [0, 0] in the fitting algorithm. The regression coefficient of the latter is very close to identity with 1.02.
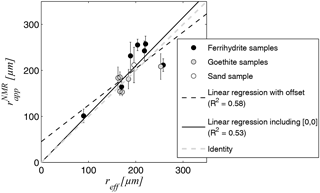
Figure 8Correlation of effective radius estimates from grain size distribution reff and the apparent radius estimates from NMR , the regression coefficient for fitting with constant offset is 0.79 and for fitting without offset, i.e. including the point [0, 0], is 1.02.
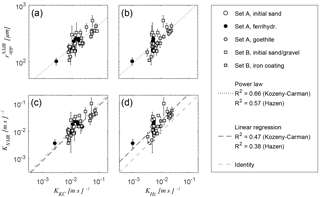
Figure 9Correlation of NMR-based estimates of apparent radius (a, b) and hydraulic conductivity KNMR (b, c) with reference values for hydraulic conductivity, which are estimated from grain size distribution according to (a, c) Kozeny (1927) and Carman (1939) and (b, d) to Hazen (1892).
Figure 9 correlates and the corresponding estimates of hydraulic conductivity KNMR with the reference values of hydraulic conductivity K for both Sets A and B. The KNMR values were estimated according to Eq. (10) using the porosities determined from the T2 measurements discussed with regard to Fig. 4. Because measurements of K are only available for eight samples of Set B, we use the K estimates derived from the GSD (Sect. 3.3) as reference values for all investigated samples, i.e. KKC according to Eq. (10) in Fig. 9a and KHz according to Hazen (1892) in Fig. 9c. For both approaches, the correlation between and K is verified with an R2 of 0.66 and 0.57, when considering a power law to describe the relation mathematically (Fig. 9a and b). The assumption of a power law is suggested by the Kozeny–Carman equation (Eq. 10), where the exponent of the pore radius should be 2. The actual exponent for our data set reaches slightly higher values of 2.41 (KKC) and 2.20 (KHz). The linear regression between KNMR with KKC and KHz (Fig. 9c and d) is verified with an R2 of 0.47 and 0.38, while the corresponding regression factors are 0.85 and 2.45, respectively.
4.5 Discussion on field applicability
The relaxation analysis in this study is limited to T1 data, the measurement of which, in boreholes and on the surface, is time-consuming and therefore often inefficient to date. Besides improving the performance of T1 measurements, future research activities in the given context will also focus on T2 relaxation measurements, which are often the preferred choice in practical applications. Considering the NMR relaxation theory, the findings of this study regarding the influence of the iron-coated pore surface on T1 are expected to be valid for T2 as well. However, the exact analysis of T2 data regarding higher relaxation modes is crucial if measured in inhomogeneous B0, because the diffusion relaxation will mask the effect of the modes to some extent. This is expected to be the case for the measurement device used in this study but is also for borehole NMR (e.g. Sucre et al., 2011; Perlo et al., 2013). Moreover, the data quality of field and borehole measurements is lowered compared to laboratory data by environmental electromagnetic noise. Future research in the framework of iron-coated soils and sediments will therefore focus on potential approaches to correct the influence of the diffusion relaxation rate caused by external field gradients and to identify and characterise the occurrence of relaxation modes in T2 data under field conditions. However, this study demonstrates that the NMR method is principally applicable to locate and hydraulically characterise zones with iron oxide accumulation in the pore space. In addition, NMR can provide indications for a beginning iron coating by changes in the apparent surface relaxivity, even before the effective hydraulic radius decreases, i.e. before a serious hydraulic clogging takes place.
NMR relaxation data of water-saturated sand and gravel are very sensitive to the amount of paramagnetic iron oxides. Here, this is confirmed using samples with synthetic ferrihydrite and goethite coatings as well as filter sand and gravel pack samples with varying contents of different natural iron oxides. We showed that the mean relaxation time can serve as a robust qualitative measure for the inhomogeneous distribution of iron content inside a sample. When focusing on the quantification of NMR parameters as a function of the iron content, the inversion of NMR data considering higher relaxation modes (Brownstein and Tarr, 1979; Müller-Petke et al., 2015) turns out to be a powerful tool, as long as the NMR relaxation takes place outside the fast diffusion regime, which is true for all samples investigated in this study. First, the inherent estimates of apparent surface relaxivity represent a qualified measure that linearly depends on the iron content, at least for values < 1 g kg−1 for our data, above which the surface relaxivity cannot be estimated precisely. However, a further increase in iron content above that limit is nevertheless indicated by a decrease in the NMR-based estimate of apparent pore radius. Second, the corresponding NMR-based apparent pore radius is shown to be a reliable proxy for the effective hydraulic radius, which was verified in this study by comparison with reference estimates from grain size distributions. An important consequence of this finding is that estimates of hydraulic conductivity can be provided from NMR outside the fast diffusion regime without any calibration.
The need for future research must be noted. Besides the limitation on intermediate and slow diffusion regimes, relaxation mode inversion as suggested in this paper is only reliable for well-sorted material with narrow pore size distributions. Otherwise the assumption of a single effective radius might not be true. Future studies will consider the existence of both different characteristic pore sizes and higher relaxation modes. In contrast to the experimental design used here, these studies must combine NMR and direct hydraulic measurements, because broad distributions of grains can systematically bias the results of simple hydraulic models based on texture (e.g. Boadu, 2000). Corresponding reference analysis regarding the pore size distribution might consist of imaging analysis or pressure-based water retention measurement.
The findings of this study are promising and interesting within the framework of hydraulic characterisation of aquifers or soils with significant content of paramagnetic iron oxides. The NMR method can complement other geophysical methods in the detection of natural iron oxide accumulations, such as bog iron, laterites, iron-rich palaeo-soils, and hardpan, provided that they are water-saturated. Moreover, a new potential application field for borehole NMR can be established: the identification and localisation of beginning iron incrustation in wells and/or the efficiency control of rehabilitation measures. Our future research activities will focus on the development of a corresponding methodology.
The NMR data at every state of processing as well as the reference data can be made available upon request. Please contact the corresponding author.
The supplement related to this article is available online at: https://doi.org/10.5194/hess-22-1713-2018-supplement.
GH initiated and motivated the study and organised the hydrochemical treatment and reference analyses. MMP developed the software for the NMR mode inversion. CW developed and conducted the experiments for the iron oxide precipitation and organised and characterised the sample material. SC developed and performed the NMR experiments and prepared the paper with contributions of all authors.
The authors declare that they have no conflict of interest.
We thank the Institute of Hydrogeology and the Institute of Hydraulic
Engineering and Water Resources Management of the RWTH Aachen University and
the RWE Power AG for providing us with sample material, Stephan Kaufhold and
Jens Gröger-Trampe for their advice and support on the geochemical
analysis, and Raphael Dlugosch for fruitful discussions on the
interpretation of the NMR data.
Edited by: Christine Stumpp
Reviewed by: Chi Zhang and one anonymous referee
Abdel Aal, G., Atekwana, E., Radzikowski, S., and Rossbach, S.: Effect of bacterial adsorption on low frequency electrical properties of clean quartz sands and iron-oxide coated sands, Geophys. Res. Lett. 36, L04403, https://doi.org/10.1029/2008GL036196, 2009.
Atekwana, E. A. and Slater, L. D.: Biogeophysics: A new frontier in Earth science research, Rev. Geophys., 47, RG4004, https://doi.org/10.1029/2009RG000285, 2009.
Behroozmand, A. A., Keating, K., and Auken, E.: A Review of the principles and applications of the NMR technique for near-surface characterization, Surv. Geophys., 36, 27–85, https://doi.org/10.1007/s10712-014-9304-0, 2014.
Blümich, B., Perlo, J., and Casanova, F.: Mobile single-sided NMR, Progr. Nucl. Magnet. Reson. Spectrosc., 52, 197–269, 2008.
Boadu, F. K.: Hydraulic Conductivity of Soils from Grain-Size Distributions: New Models, J. Geotech. Geoenviron. Eng., 126, 739–746, 2000.
Brownstein, K. R. and Tarr, C. E.: Importance of classical diffusion in NMR studies of water in biological cells, Phys. Rev. A, 19, 2446–2453, 1979.
Brunauer, S., Emmett, P. H., and Teller, E.: Adsorption of Gases in Multimolecular Layers, J. Am. Chem. Soc., 60, 309–319, https://doi.org/10.1021/ja01269a023, 1938.
Bryar, T. R. and Knight, R. J.: Sensitivity of nuclear magnetic resonance relaxation measurements to changing soil redox conditions, Geophys. Res. Lett., 29, 2197, https://doi.org/10.1029/2002GL016043, 2002.
Bryar, T. R. and Knight, R. J.: Laboratory studies of the detection of sorbed oil with proton nuclear magnetic resonance, Geophysics, 68, 942–948, 2003.
Bryar, T. R., Daughney, C. J., and Knight, R. J.: Paramagnetic effects of iron(III) species on nuclear magnetic relaxation of fluid protons in porous media, J. Magnet. Reson., 142, 74–85, 2000.
Carman, P. C.: Permeability of saturated sands, soils and clays, J. Agr. Sci., 29, 262–273, 1939.
Carrier, W. D.: Goodbye, Hazen; Hello, Kozeny–Carman, J. Geotech. Geoenviron. Eng., 129, 1054–1056, 2003.
Coates, G., Xiao, L., and Prammer, M.: NMR Logging Principles and Application, Halliburton Energy Services, Houston, 1999.
Colombo, C., Palumbo, G., He, J.-Z., Pinton, R., and Cesco, S.: Review on iron availability in soil: interaction of Fe minerals, plants, and microbes, J. Soils Sediments, 14, 538–548, https://doi.org/10.1007/s11368-013-0814-z, 2014.
Cornell, R. M. and Schwertmann, U.: The iron oxides: structure, properties, reactions, occurences and uses, Wiley-VCH, Weinheim, 703 pp., 2003.
Costabel, S. and Yaramanci, U.: Relative hydraulic conductivity and effective saturation from Earth's field nuclear magnetic resonance – a method for assessing the vadose zone, Near Surf. Geophys., 9, 155–167, https://doi.org/10.3997/1873-0604.2010055, 2011.
Costabel, S. and Yaramanci, U.: Estimation of water retention parameters from nuclear magnetic resonance relaxation time distributions, Water Resour. Res., 49, 2068–2079, https://doi.org/10.1002/wrcr.20207, 2013.
Costabel, S., Siemon, B., Houben, G., and Günther, T.: Geophysical investigation of a freshwater lens on the island of Langeoog, Germany – Insights from combined HEM, TEM and MRS data, J. Appl. Geophys., 136, 231–245, https://doi.org/10.1016/j.jappgeo.2016.11.007, 2017.
Costanza-Robinson, M. S., Estabrook, B. D. and Fouhey, D. F.: Representative elementary volume estimation for porosity, moisture saturation, and air-water interfacial areas in unsaturated porous media: Data quality implications, Water Resour. Res., 47, W07513, https://doi.org/10.1029/2010WR009655, 2011.
Cullimore, D. R.: Microbiology of well biofouling, CRC Press, Boca Raton, FL, 456 pp., 2000.
Cundy, A. B., Hopkinson, L., and Whitby, R. L. D.: Use of iron-based technologies in contaminated land and groundwater remediation: A review, Sci. Total Environ., 400, 42–51, 2014.
Dlugosch, R., Günther, T., Müller-Petke, M., and Yaramanci, U.: Improved prediction of hydraulic conductivity for coarse-grained, unconsolidated material from nuclear magnetic resonance, Geophysics, 78, EN55–EN64, 2013.
Dunn, K., Bergman, D. J., and LaTorraca, G. A.: Nuclear Magnetic Resonance – Petrophysical and Logging Applications, in: Handbook of Geophysical Exploration: Seismic Exploration, Vol. 32, Elsevier Science, Oxford, 312 pp., 2002.
Emerson, D., Fleming, E. J., and McBeth, J. M.: Iron-Oxidizing Bacteria: An Environmental and Genomic Perspective, Annu. Rev. Microbiol., 64, 561–583, 2010.
Foley, I., Farooqui, S. A., and Kleinberg, R. L.: Effect of paramagnetic ions on NMR relaxation of fluids at solid surfaces, J. Magnet. Reson. Ser. A, 123, 95–104, 1996.
Geroni, J. N. and Sapsford, D. J.: Kinetics of iron (II) oxidation determined in the field, Appl. Geochem., 26, 1452–1457, 2011.
Grunewald, E. and Knight, R.: A laboratory study of NMR relaxation times in unconsolidated heterogeneous sediments, Geophysics, 76, G73–G83, 2011.
Hazen, A.: Some physical properties of sands and gravels, with special reference to their use in filtration, 24th Annual Rep., Pub. Doc. No. 34, Massachusetts State Board of Health, Massachusetts, 539–556, 1892.
Hertzog, R. C., White, T. A., and Straley, C.: Using NMR decay-time measurements to monitor and characterize DNAPL and moisture in subsurface porous media, J. Environ. Eng. Geophys., 12, 293–306, 2007.
Hinedi, Z. R., Chang, A. C., and Anderson, M. A.: Quantification of microporosity by nuclear magnetic resonance relaxation of water imbibed in porous media, Water Resour. Res., 33, 2697–2704, 1997.
Houben, G. J.: Iron oxide incrustations in wells. Part 1: Genesis, mineralogy and geochemistry, Appl. Geochem., 18, 927–939, 2003a.
Houben, G. J.: Iron oxide incrustations in wells. Part 2: Chemical dissolution and modeling, Appl. Geochem., 18, 941–954, 2003b.
Houben, G. J. and Kaufhold, S.: Multi-Method characterization of the ferrihydrite to goethite transformation, Clay Minerals, 46, 387–395, 2011.
Houben, G. J. and Weihe, U.: Spatial distribution of incrustations around a water well after 38 years of use, Ground Water, 48, 53–58, 2010.
Janney, D. E., Cowley, J. M., and Buseck, P. R.: Transmission Electron Microscopy of Synthetic 2- and 6-line Ferrihydrite, Clays Clay Miner., 48, 111–119, 2000.
Kappler, A. and Straub, K. L.: Geomicrobiological Cycling of Iron, Rev. Mineral. Geochem., 59, 85–108, 2005.
Keating, K. and Knight, R.: A laboratory study to determine the effects of iron oxides on proton NMR measurements, Geophysics, 72, E27–E32, 2007.
Keating, K. and Knight, R.: A laboratory study of the effect of magnetite on NMR relaxation rates, J. Appl. Geophys., 66, 188–196, 2008.
Keating, K. and Knight, R.: A laboratory study of the effect of Fe(II)-bearing minerals on nuclear magnetic resonance (NMR) relaxation measurements, Geophysics, 75, F71–F82, 2010.
Keating, K. and Knight, R.: The effect of spatial variation in surface relaxivity on nuclear magnetic resonance relaxation rates, Geophysics, 77, E365–E377, 2012.
Kenyon, W. E.: Petrophysical Principles of Applications of NMR Logging, Log. Analyst., 38, 21–43, 1997.
Knight, R., Walsh, D. O., Butler Jr., J. J., Grunewald, E., Liu, G., Parsekian, A. D., Reboulet, E. C., Knobbe, S., and Barrows, M.: NMR logging to estimate hydraulic conductivity in unconsolidated aquifers, Groundwater, 54, 104–114, https://doi.org/10.1111/gwat.12324, 2016.
Kolz, J., Goga, N., Casanova, F., Mang, T., and Blümich, B.: Spatial localization with single-sided NMR sensors, Appl. Magn. Reson., 32, 171–184, 2007.
Kozeny, J.: Uber kapillare Leitung des Wassers im Boden: Sitzungsberichte, in: Mathematisch-Naturwissenschaftliche Klasse Abteilung IIa, Akademie der Wissenschaften, Wien, 136, 271–306, 1927.
Larese-Casanova, P., Kappler, A., and Haderlein, S. B.: Heterogeneous oxidation of Fe(II) on iron oxides in aqueous systems: Identification and controls of Fe(III) product formation, Geochim. Cosmochim. Ac., 91, 171–186, 2012.
Larroque, F. and Franceschi, M.: Impact of chemical clogging on de-watering well productivity: numerical assessment, Environ. Earth Sci., 64, 119–131, 2011.
Legchenko, A., Baltassat, J.-M., Bobachev, A., Martin, C., Henri, R., and Vouillamoz, J.-M.: Magnetic resonance sounding applied to aquifer characterization, Groundwater, 42, 363–373, 2004.
MATLAB®: 9.0.0.341360 (R2016a), Optimization Toolbox TM User's Guide, The MathWorks, Inc., Natick, Massachusetts, 2016.
Medina, D. A. B., van den Berg, G. A., van Breukelen, B. M., Juhasz-Holterman, M., and Stuyfzand, P. J.: Iron-hydroxide clogging of public supply wells receiving artificial recharge: near-well and in-well hydrological and hydrochemical observations, Hydrogeol. J., 21, 1393–1412, 2013.
Mehra, O. P. and Jackson, M. L.: Iron oxide removal from soils and clays by a dithionite-citrate system buffered with sodium bicarbonate, Clays Clay Miner., 5, 317–327, 1960.
Mohnke, O., Jorand, R., Nordlund, C., and Klitzsch, N.: Understanding NMR relaxometry of partially water-saturated rocks, Hydrol. Earth Syst. Sci., 19, 2763–2773, https://doi.org/10.5194/hess-19-2763-2015, 2015.
Müller-Petke, M., Dlugosch, R., Lehmann-Horn, J., and Ronczka, M.: Nuclear magnetic resonance average pore-size estimations outside the fast-diffusion regime, Geophysics, 80, D195–D206, 2015.
Pape, H., Tillich, J. E., and Holz, M.: Pore geometry of sandstone derived from pulsed field gradient NMR, J. Appl. Geophys., 58, 232–252, 2006.
Perlo, J., Danieli, E., Perlo, J., Blümich, B., and Casanova, F.: Optimized slim-line logging NMR tool to measure soil moisture in situ, J. Magnet. Reson., 233, 74–79, 2013.
Pham, A. N. and Waite, T. D.: Oxygenation of Fe(II) in natural waters revisited: Kinetic modeling approaches, rate constant estimation and the importance of various reaction pathways, Geochim. Cosmochim. Ac., 72, 3616–3630, 2008.
Prammer, M. G., Drack, E. D., Bouton, J. C., and Gardner, J. S.: Measurements of clay-bound water and total porosity by magnetic resonance logging, SPE paper 36522, Society of Petroleum Engineers, 6–9 October 1996, Denver, Colorado, 311–320, https://doi.org/10.2118/36522-MS, 1996.
Sapoval, B., Russ, S., Petit, D., and Korb, J. P.: Fractal geometry impact on nuclear relaxation in irregular pores, Magnet. Reson. Imag., 14, 863–867, 1996.
Schwertmann, U. and Cornell, R. M.: Iron Oxides in the Laboratory – Preparation and Characterization, Wiley-VCH, Weinheim, 188 pp., 2000.
Stumm, W. and Lee, G. F.: The chemistry of aqueous iron, Schweiz. Z. Hydrol., 22, 295–319, 1960.
Stumm, W. and Sulzberger, B.: Cycling of iron in natural environments: Considerations based on laboratory studies of heterogeneous redox processes, Geochim. Cosmochim. Ac., 56, 3233–3257, 1991.
Sucre, O., Pohlmeier, A., Miniere, A., and Blümich, B.: Low-field NMR logging sensor for measuring hydraulic parameters of model soils, J. Hydrol., 406, 30–38, https://doi.org/10.1016/j.jhydrol.2011.05.045, 2011.
Tuhela, L., Carlson, L., and Tuovinen, O. H.: Biogeochemical transformations of Fe and Mn in oxic groundwater and well water environments, J. Environ. Sci. Health Pt. A, 32, 407–426, 1997.
Van Dam, R. L., Schlager, W., Dekkers, M. J., and Huisman, J. A.: Iron oxides as a cause of GPR reflections, Geophysics, 67, 536–545, 2002.
Weidner, C.: Experimental Modelling and Prevention of Chemical Fe-Clogging in Deep Vertical Wells for Open-Pit Dewatering, PhD Thesis, RWTH Aachen University, Aachen, 219 pp., 2016.
Weidner, C., Henkel, S., Lorke, S., Rüde, T. R., Schüttrumpf, H., and Klauder, W.: Experimental modelling of chemical clogging processes in dewatering wells, Mine Water Environ., 31, 242–251, 2012.