the Creative Commons Attribution 4.0 License.
the Creative Commons Attribution 4.0 License.
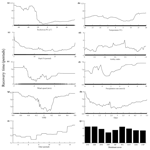
Assessing recovery time of ecosystems in China: insights into flash drought impacts on gross primary productivity
Mengge Lu
Yong Yang
Jie Xue
Hongbo Ling
Hong Zhang
Wenxin Zhang
Recovery time, referring to the duration that an ecosystem needs to return to its pre-drought condition, is a fundamental indicator of ecological resilience. Recently, flash droughts – characterised by rapid onset and development – have gained increasing attention. Nevertheless, the spatiotemporal patterns in gross primary productivity (GPP) recovery time and the factors influencing it remain largely unknown. In this study, we investigate the recovery time patterns in a terrestrial ecosystem in China based on GPP using a random forest regression model and the SHapley Additive exPlanations (SHAP) method. A random forest regression model was developed to analyse the factors influencing recovery time and establish response functions through partial correlation for typical flash drought recovery periods. The dominant driving factors of recovery time were determined using the SHAP method. The results reveal that the average recovery time across China is approximately 37.5 d, with central and southern regions experiencing the longest durations. Post-flash-drought radiation emerges as the primary environmental factor, followed by the aridity index and post-flash-drought temperature, particularly in semi-arid and sub-humid areas. Temperature exhibits a non-monotonic relationship with recovery time, where both excessively cold and hot conditions lead to longer recovery periods. Herbaceous vegetation recovers more rapidly than woody forests, with deciduous broadleaf forests demonstrating the shortest recovery time. This study provides valuable insights for comprehensive water resource and ecosystem management and contributes to large-scale drought monitoring efforts.
- Article
(2256 KB) - Full-text XML
-
Supplement
(832 KB) - BibTeX
- EndNote
Climate change has exacerbated drought, which has significant implications for the achievement the Sustainable Development Goals (SDGs) (Lindoso et al., 2018). Among the 17 SDGs outlined in the 2030 Agenda for Sustainable Development, at least five are directly linked to drought: Goal 6 “Clean water and sanitation”, Goal 11 “Sustainable cities and communities”, Goal 12 “Responsible consumption and production”, Goal 13 “Climate action”, and Goal 15 “Life on land” (Zhang et al., 2019; Nilsson et al., 2016). Flash droughts – characterised by rapid onset and intensification – have gained increasing recognition among hydrologist and the general public globally (Yuan et al., 2023). These events significantly impact terrestrial ecosystem productivity, photosynthesis, and latent heat fluxes (Zhang and Yuan, 2020; Yang et al., 2023). The effects of flash droughts are not only felt during the events but also persist in their aftermath, with legacy effects following such droughts (Liu et al., 2023a). Recovery time – defined as the duration required for an ecosystem to return to its pre-drought state – is a fundamental aspect of ecological resilience (Schwalm et al., 2017; Wu et al., 2018). Recovery time is related to ecological thresholds, as it may trigger a critical “tipping point” that leads to shifts into a new ecosystem state (Lenton et al., 2008). With the expectation of more frequent and severe flash droughts in the future (Sreeparvathy and Srinivas, 2022), exploring post-flash-drought recovery trajectories is of paramount importance (Jiao et al., 2021).
Drought recovery characteristics have been extensively observed at the ecosystem scale, typically using tree ring records, productivity or greenness measurements, and satellite data (Gazol et al., 2017; Kannenberg et al., 2019). These studies have identified varied recovery times across regions and ecosystems. Grasslands exhibit longer recovery times compared with other land covers types due to shallow-rooted plants and lower soil water retention capacity (Hao and Choi, 2023). Conversely, recovery in croplands is more influenced by human farming practices (Darnhofer et al., 2016). In forests, mixed forests tend to recover more quickly, whereas deciduous broadleaf forests have the longest recovery periods (He et al., 2018). Hydrometeorological conditions also play a role, with semi-arid and semi-humid regions experiencing longer recovery times than humid and arid regions (Zhang et al., 2021). The longer recovery time in semi-arid and semi-humid regions may be related to the specific challenges that these regions face, such as soil conditions, water availability, and climatic variability (Huxman et al., 2004; Zhang et al., 2021).
However, the contribution of driving factors to flash drought recovery remains unclear. Some studies have indicated that the background value, drought return interval, post-drought hydrometeorological conditions, and drought attributes (such as duration and intensity) are critical with respect to regulating recovery (Kannenberg et al., 2020). A lower background value may result in more severe damage, abnormal post-drought hydrometeorological conditions, and longer recovery times (Fu et al., 2017). Greater drought intensity and longer drought duration can lead to significant ecosystem losses (Godde et al., 2019). Favourable post-drought hydrometeorological conditions (e.g. increased precipitation and suitable temperature) improve the chance of complete recovery (Jiao et al., 2021). Plant physiological responses, including changes in leaf water potential and phenology, also play a crucial role in the recovery process (Miyashita et al., 2005).
While the impacts of flash droughts on ecosystems have been well documented, the recovery process remains underexplored. For instance, studies have shown that solar-induced fluorescence (SIF) and SIF yield values decline in the period following flash drought (Yao et al., 2022), and 95 % of the gross primary production (GPP) in the Indian region responded to flash droughts with an average response time of 10–19 d (Poonia et al., 2022). However, most research has focused on the immediate ecological responses to flash droughts, rather than on the recovery process (Otkin et al., 2019). Notably, a substantial contrast exists in the definition of recovery stages between flash droughts and traditional slow droughts (Wang et al., 2016). These results have led to the conclusion that recovery is a part of the former, whereas the recovery phase of the latter usually occurs at the end of the event (Qing et al., 2022). Furthermore, some studies have suggested that flash drought recovery is more reliant on changes in soil moisture or peak evapotranspiration, while traditional slow drought recovery is typically assessed using ecological or hydrological indicators (Xu et al., 2023). China experienced frequent flash drought in the period between 1980 and 2021, particularly in southwestern and central regions (Wang and Yuan, 2022). Moreover, there may be more severe and frequent flash droughts in the future (Christian et al., 2023). Research on flash drought recovery in Xiang and Wei basins found that most events were associated with a recovery time of 28 d (Wang and Yuan, 2023). However, there remains a lack of comprehensive studies on flash drought recovery and the factors influencing its spatiotemporal patterns across China.
Drought can lead to water shortages, limiting access to clean drinking water. Effective drought management is therefore crucial for achieving the SDGs. By utilising newly available datasets and hydrometeorological variables in China, this study assesses the extent of post-flash-drought impacts, documents recovery times, and analyses the factors contributing to variations in ecosystem recovery. The objectives of this study are to (1) investigate the spatial pattern of post-flash-drought recovery, (2) identify the most critical determinants of recovery, and (3) analyse the impact of various factors on flash drought recovery times. The remainder of the paper is structured as follows: Sect. 2 provides a brief description of the data and methods; Sect. 3 outlines the results, which are presented with respect to the novel methods applied; Sect. 4 entails a detailed discussion; Sect. 5 gives the conclusions; and the related Supplement provides further information.
2.1 Data
2.1.1 Soil moisture datasets
Daily root zone soil moisture (SM) data for the period from 2001 to 2018 were obtained from the Global Land Evaporation Amsterdam Model (GLEAM) (https://www.gleam.eu/, last access: 2 February 2024). GLEAM estimates root zone soil moisture using a multilayer water balance approach. The depth of the root zone varies based on the type of land cover. For tall vegetation (e.g. trees), the depth is divided into three layers (0–10, 10–100, and 100–250 cm); for low vegetation (e.g. grass), there are two layers (0–10 and 10–100 cm); and bare soil only has one layer (0–10 cm) (Martínez-Vilalta and Garcia-Forner, 2017). This model has been widely applied in the identification and impact assessment of flash drought events (Zha et al., 2023). We utilised the bilinear interpolation method to resample SM from a spatial resolution of 0.25–0.1°, aligning it with the accuracy of other datasets. This method is appropriate for continuous input values, easy to implement, and generally effective in converting coarse input data into spatially refined output (Chen et al., 2020).
2.1.2 Hydrometeorological datasets of variables affecting recovery time
We analyse the recovery time by considering multiple influencing factors, such as meteorological variables, drought-related variables, and land cover (He et al., 2018). Meteorological data from the China Meteorological Forcing Dataset (CMFD), accessible at https://doi.org/10.6084/m9.figshare.c.4557599, is utilised for the period spanning from 2001 to 2018 (He et al., 2020). The near-surface air temperature, downward short-wave radiation, downward long-wave radiation, precipitation rate, and wind speed are used in this study. The vapour pressure deficit (VPD) is calculated based on temperature and specific humidity using Eqs. (1)–(3) (Peixoto and Oort, 1996):
where SVP and AVP are the respective saturated vapour pressure and actual vapour pressure (kPa), T is temperature (°C), qs is the specific humidity, p is the atmospheric pressure (kPa), and ε= 6.22 is the ratio of the water vapour molecular weight to the dry-air weight.
The aridity index (AI) is calculated as the ratio of precipitation to potential evapotranspiration. Typically, the multiyear average of the AI serves as an indicator of water availability and drought timing within a particular region (Huang et al., 2016). The AI is obtained from https://doi.org/10.6084/m9.figshare.7504448.v5 (Zomer et al., 2022). To analyse the distinct responses of different vegetation types, we employ the MCD12C1 Moderate Resolution Imaging Spectroradiometer (MODIS) dataset from the International Geosphere–Biosphere Programme (Fig. S1 in the Supplement; Friedl et al., 2002).
2.1.3 Gross primary productivity
Gross primary productivity (GPP) is widely used as an indicator to monitor post-drought photosynthesis dynamics (Gazol et al., 2018). The FluxSat GPP dataset (version 2), derived from MODIS, is calibrated using FLUXNET2015 and OneFlux tier-1 data and validated with independent datasets (Joiner and Yoshida, 2021).
The FluxSat GPP dataset shows strong agreement with flux data at most sites and performs reliably across the majority of global regions (Bennett et al., 2021). Additionally, it has been widely used to examine the impacts of extreme climate events on the terrestrial carbon cycle (Byrne et al., 2021). The dataset provides a spatial resolution of 0.05° and a daily temporal resolution. To match the flash drought event, daily soil moisture data were resampled to 0.1° and aggregated to pentad-mean (5 d mean) data. This study chooses the growing seasons (April–October) from 2001 to 2023 as the study period.
2.2 Method
2.2.1 The identification of flash drought events and recovery time
In this study, we identify flash drought events by analysing changes in soil moisture, taking the rapid intensification and duration of these events into account. Although evaporative demand is often used as a warning indicator for flash droughts, it may overestimate the occurrence of flash droughts (Lesinger and Tian, 2022). Therefore, in this study, we employ an alternative approach by using soil moisture data, which are aggregated into pentad-mean values to identify flash drought events. These averages are then converted into percentiles based on the climatology of each pentad period during the growing season. The identification of flash droughts should meet the following criteria: soil moisture (SM) must decrease from above the 40th percentile to below the 20th percentile within a 5 d period, with an average rate of decline per pentad of no less than the 5th percentile. A flash drought terminates if the declining SM rises back to the 20th percentile. The duration of a flash drought event must be at least four pentads (20 d) (Yuan et al., 2019; Zhang and Yuan, 2020). The speed of flash drought (Ospd) is the ratio of the difference between the 40th percentile and the lowest percentile of the onset stage to the length of onset. The frequency refers to the overall number of occurrences within a given time frame (e.g. per year or per decade). Severity is the accumulated soil moisture percentile deficits from the threshold of the 40th percentile. We employed a GPP anomaly to estimate post-flash-drought vegetation recovery times at the pixel scale. The recovery time was defined as the period between the point at which the GPP reached its maximum loss and when it returned to its pre-flash-drought level (Wang and Yuan, 2022) (Fig. 1). To ensure data consistency and minimise noise, we first applied a smoothing process to the pentad GPP data using a three-pentad forward-moving window at the pixel scale. After smoothing the data, we calculate the GPP anomaly using the following equation:
where μGPP and σGPP are the respective mean and standard deviation of the pentad time series of GPP.
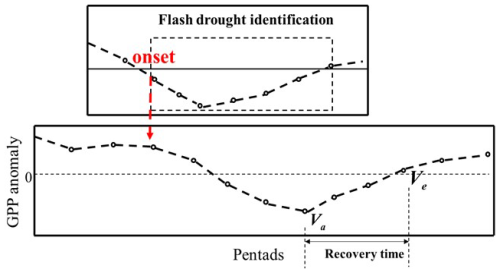
Figure 1The identification of recovery time. The GPP anomaly is a detrended vegetation production index on a time series. Zero (0) is defined as the threshold of a negative anomaly. The area below the dashed line represents that vegetation production is in a negative abnormal state. We quantify recovery time as follows: the recovery time begins when the vegetation production loss reaches the maximum and ends when the detrended vegetation production index is above 0.
The beginning of the recovery stage is identified when the post-flash-drought GPP anomaly is negative and reaches its minimum value, indicating the point of maximum GPP loss. The recovery stage concludes when the GPP anomaly returns to a positive value, signifying that productivity has reached or exceeded its pre-drought level. However, if no flash drought event occurs during the period of negative GPP anomaly, if the GPP anomaly is already negative before the onset of the flash drought event, or if negative GPP anomalies only occur for one pentad, the corresponding GPP data series is excluded from the analysis to prevent misleading results.
2.2.2 Response functions
Partial dependence plots based on the random forest algorithm are utilised to visualise response functions (Schwalm et al., 2017; Sun et al., 2016). The analysis of partial dependence focuses on evaluating the marginal impact of a covariate (or independent variable) on the response variable, while keeping other covariates constant (Breiman et al., 2002). It facilitates the exploration of insights within large datasets, particularly when random forests are primarily influenced by low-order interactions (Martin, 2014). In addition, it is a valuable tool to identify significant features, detect non-linear relationships, and gain insights into the overall behaviour of a predictive model.
2.2.3 Attribution analysis of ecosystem recovery
In order to better understand the potential factors driving terrestrial ecosystem productivity recovery after flash droughts, we conduct attribution analysis. We selected the downward radiation (the sum of downward short-wave radiation and downward short-wave radiation), temperature, wind speed, precipitation rate, VPD, flash drought speed (Ospd), flash drought severity (Osev), flash drought duration (Odur), AI, and land cover type as explanatory variables. It should be noted that these variables are considered within the recovery period. The feature importance of random forest can only indicate the extent to which the input variables influence the model's output, but it does not reveal how these input variables specifically impact the model's output (Wang et al., 2022). The SHapley Additive exPlanations (SHAP) method has emerged as a valuable tool that addresses the limitations of traditional machine learning methods (Štrumbelj and Kononenko, 2014). As a result, the SHAP method is widely utilised in the attribution analysis of variables (Wang et al., 2022; Lundberg and Lee, 2017).
where φm(v) represents the contribution of covariate m, N denotes the set of all covariates, S is a subset of N, and v(S) represents the value of that subset.
We utilised a random forest model and employed these variables as predictive factors to estimate the productivity recovery time for all study grid cells. Then, we used the SHAP value to quantify the marginal contribution of each predictive variable and rank their relative importance based on the average absolute SHAP value.
3.1 Characteristics of flash droughts
Figure 2 presents the frequency, duration, severity, and speed of flash droughts over China during the 2001–2019 period. Approximately 7 % of grids did not experience a flash drought event, while the remaining 93 % of grids experienced at least one event. The middle and lower reaches of the Yangtze River exhibited a high frequency value (with above 12 events per decade), whereas other regions mainly ranged from 0 to 9 events per decade. There is a clear spatial pattern in the flash drought duration, which ranges from 0 to 20 d over China. The southwestern and the middle and lower reaches of the Yangtze River had longer durations, exceeding 90 d (Fig. S2). In addition to the higher severity of flash droughts in the southwestern region, a similar spatial pattern was observed in the severity and speed. Regarding speed, areas with a faster speed were primarily concentrated in the lower reaches of the Yangtze River. Overall, the middle and lower reaches of the Yangtze River and the southwestern region are considered hot spots, although the latter's speed is not rapid.
3.2 Spatial pattern of the ecosystem recovery time and recovery rate
Vegetation productivity showed a clear response to flash droughts, and this response typically had a certain lag (Fig. S3). Ecosystems exhibited distinct spatial differences in recovery times to flash droughts (Fig. 3). The mean recovery time for Chinese ecosystems was 37.5 d (7.5 pentads), calculated by GPP. Most regions were able to recover to their normal state within 50 d. However, certain areas, such as central China and southern China, required 90 d or more to recover. In terms of time series, there was no evident trend in the mean recovery time, with fluctuations occurring within 7.5 pentads. On average, the recovery rate of grids in China ranged from 0–2g C m−2 d−1 pentad−1 per pentad, and approximately 90 % of grids had a recovery rate of less than 1 per pentad. There is no significant trend in the recovery rate over time. To further illustrate the impact and recovery of flash droughts on different vegetation types, we calculated the recovery time and recovery rate for each type (Fig. 4). Among the different vegetation types, deciduous broadleaf forest (DBF) had a shorter recovery time and a higher recovery rate. Additionally, croplands (CRP) showed moderate recovery rates, whereas grasslands (GRS) had relatively low rates of recovery. This reflects the fact that flash droughts had a more significant impact on GRS and resulted in greater productivity losses. By employing various recovery thresholds (80 %, 90 %, 100 %, or 110 % of the original state), we confirmed that, although the recovery time of some grid pixels can vary, the overall spatial pattern of the recovery time remains consistent regardless of the threshold used (Fig. S4).
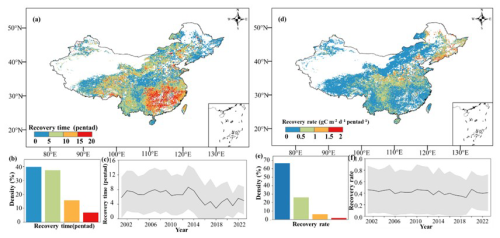
Figure 3Spatial pattern of recovery time (a–c) and recovery rate (d–f). Panels (a) and (d) represent the recovery time (pentad) and recovery rate (g C m−2 d−1 pentad−1) calculated using GPP data, respectively. Panels (b) and (e) represent the density of different recovery times and the recovery rate, respectively; the horizontal axis represents the recovery time (pentad) or recovery rate (g C m−2 d−1 pentad−1), respectively, and the vertical axis is the density. Regions with a sparse GPP or no droughts are masked using white. Publisher's remark: please note that the above figure contains disputed territories.
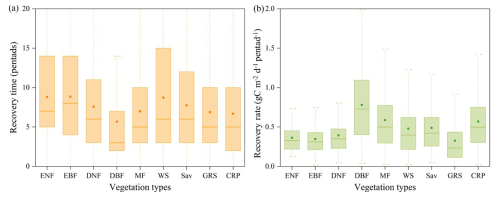
Figure 4The recovery time and recovery rate across different vegetation types. The vegetation types are as follows: ENF (evergreen coniferous forest), EBF (evergreen broadleaf forest), DNF (deciduous coniferous forest), DBF (deciduous broadleaf forest), MF (mixed forests), WS (closed shrubland, open shrubland, and woody savannas), SAV (savannas (temperate)), GRS (grasslands), and CRP (croplands).
3.3 Response functions for flash drought recovery time
The random forest regression model explained 55 % of the out-of-bag variance in recovery time (Fig. 5). Radiation emerged as the most influential factor impacting flash drought recovery time, with lower-solar-radiation conditions leading to a prolonged the recovery time (Fig. 5a). Temperature did not exhibit a monotonic response in relation to recovery time. Excessively cold or hot temperatures resulted in longer recovery times, whereas slightly higher temperatures promoted vegetation recovery (Fig. 5b). Specifically, a slight increase in temperature facilitated vegetation restoration, while higher temperatures extended the recovery time with respect to flash droughts. This suggests that the projected rise in extreme high temperatures will further lengthen the recovery time (Li et al., 2019). In terms of flash drought characteristics, the difference in recovery time was related to the discrepancy in severity and duration, albeit to a lesser extent than speed (Fig. 5c, h, i). Recovery time increased in a stepwise manner as the duration increased. Ecosystems experiencing prolonged flash drought durations typically exhibit longer recovery times. In addition, semi-arid and sub-humid areas (0.2 < AI < 0.65) have longer recovery times (Fig. 5d). The wind speed exhibited a bimodal pattern, indicating that the recovery time was shortest when it closely aligned with the multiyear average or was 3.5 times higher than the multiyear average (Fig. 5e). Adequate precipitation following a flash drought assisted in recovery, although excessively extreme precipitation could also hinder it (Fig. 5f). An extreme VPD, whether high or low, prolonged the recovery time (Fig. 5g). Among different vegetation types, herbaceous vegetation recovered more rapidly than woody forests. DBF demonstrated the shortest recovery time (Fig. 5j).
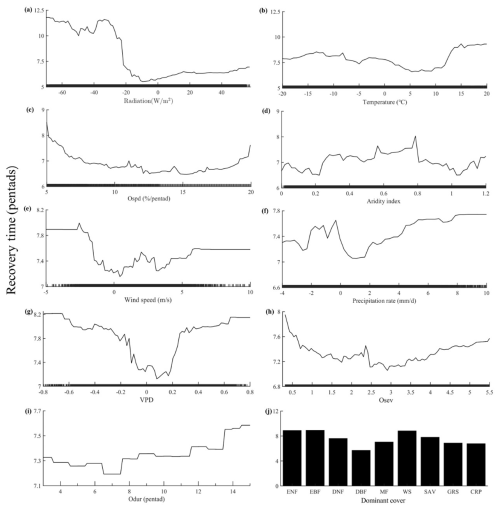
Figure 5Response functions for flash drought recovery time, reflecting the response of recovery time to a single dependent variable when others are unchanged. Note difference in the y-axis scales. The covariates in panels (a) to (j) are the deviations from the baseline. Positive (negative) indicates above (below) the average value.
3.4 Drivers of flash drought recovery time
We then performed an attribution analysis using the SHAP method to quantify the relative importance of the considered variables. The results were consistent with the results given in Sect. 3.3. In general, radiation and the aridity index were the most relevant controls on spatial variations in post-flash-drought recovery time (Fig. 6). Temperature was the third most impactful variable overall, primarily due to its high impact on predicting the recovery time (absolute mean SHAP value of 0.62). Compared with other variables, the impacts of flash drought speed and duration were relatively low. In addition, during the process of flash drought recovery, the losses caused by flash droughts can also affect productivity recovery. The relationship between recovery time and the attributes of flash drought (speed, severity, and duration) is usually negative. That is to say, faster, more severe, and longer-lasting flash droughts often have a longer recovery time. Specifically, the speed of flash droughts is one of the main controlling factors with respect to the recovery time.
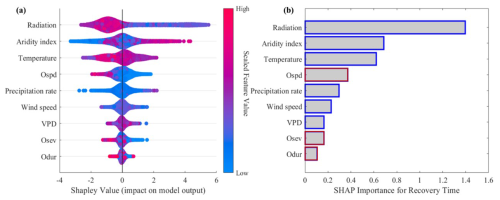
Figure 6Identifying the drivers of patterns of post-flash-drought recovery time. (a) The summary plot of SHAP values in random forest machine learning. (b) The SHAP importance (averaged absolute SHAP values) for recovery time. Considered drivers include flash drought characteristics (in red) and post-flash-drought hydrometeorological conditions (in blue).
4.1 Assessment of flash drought recovery time based on vegetation productivity
Given the prevalence of drought in widespread regions over the past few decades, it is a major natural disaster worldwide (Douris and Kim, 2021). In addition, regions' exposure, vulnerability, and risk are expected to further increase due to future climate and socio-economic changes (Tabari and Willems, 2018; Cook et al., 2020). Flash drought is widely recognised as a sub-seasonal phenomenon that develops rapidly (Tyagi et al., 2022). Flash droughts have varying degrees of impact on the photosynthesis, productivity, and respiration of ecosystems (Mohammadi et al., 2022). Reducing drought risks and strengthening social drought resistance are also important tasks in order to achieve the SDGs by 2030 (Tabari and Willems, 2023). Flash droughts interact with ecological droughts: ecological droughts potentially make ecosystems more vulnerable to flash droughts, while flash droughts can exacerbate the effects of persistent ecological droughts (Cravens et al., 2021; Xi et al., 2024). The interplay between these two types of droughts can intensify the pressure on ecosystems, complicating and prolonging the recovery process. The SIF response frequency to flash droughts in the Pearl River Basin of China exceeds 80 %, with 96.85 % of the regional response occurring within 16 d (Yang et al., 2023). Previous studies have calculated that the recovery time with respect to flash drought based on changes in soil moisture ranges from 8 to 40 d (Otkin et al., 2019). Additionally, the recovery time is generally longer in humid areas compared with arid areas. However, not all flash drought events result in a decrease in ecosystem productivity (Liu et al., 2019). For instance, a study conducted by Zhang et al. (2020) revealed that 81 % of flash droughts in China between 2003 and 2018 displayed negative normalised GPP anomalies, while the remaining 19 % of events did not exhibit such negative anomalies. Therefore, GPP serves as a more appropriate indicator for monitoring post-drought photosynthesis-related dynamics and evaluating ecosystem recovery time (Yu et al., 2017). Based on GPP, most flash drought events in the Xiang and Weihe basins recovered within 2 to 8 d. Moreover, the recovery time in the Xiang Basin, which is located in a humid area, tended to be longer (Wang and Yuan, 2022). It should be noted that this study only investigated the aforementioned two watersheds and did not include semi-humid/semi-arid areas. Our study revealed that the average recovery time with respect to flash droughts in China is approximately 37.5 d (7.5 pentads) (Fig. 3).
4.2 Factors that affect drought recovery time
Solar radiation and the aridity index were the primary factors that influenced the recovery time (Figs. 5, 6, S5). The recovery time was regulated by a combination of drought characteristics (drought return interval, severity, and duration), post-drought hydrometeorological conditions, and vegetation physiological characteristics (Fathi-Taperasht et al., 2022; Liu et al., 2019). Physiological responses, such as the rate of productivity decline upon exposure to flash drought, also influenced the recovery time. Notably, there is a significant negative correlation between the rate of decline and the recovery rate (Lu et al., 2024). In the case of flash droughts, which are characterised by rapid development, the speed is one of the most important factors controlling the recovery time (Fig. 6). The Yangtze River Basin experienced one of the most severe flash droughts on record during the summer of 2022, primarily driven by abnormally high temperatures and abrupt changes in precipitation (Liu et al., 2023b). The high temperatures accelerated the onset of the drought (Wang and Yuan, 2022). As a result, the total GPP loss from July to October 2022 was 26.12 ± 16.09 Tg C, representing a decrease of approximately 6.08 % compared with the 2001–2021 average (Li et al., 2024). Ecological drought is characterised by prolonged conditions lasting from months to years and resulting in long-term changes in ecosystem function and structure (Sadiqi et al., 2022). In contrast, flash droughts develop rapidly, within days to weeks, due to extreme weather, leading to immediate reductions in soil moisture and plant health (Yuan et al., 2023). The long-term nature of ecological drought can cause profound impacts, such as reduced plant populations, increased soil erosion, and decreased biodiversity, necessitating a longer recovery period (Cravens et al., 2021). In contrast, flash droughts, while shorter in duration, cause rapid plant wilting, reduced crop yields, and soil cracking, with significant long-term consequences for ecosystem recovery (Xi et al., 2024). These two types of droughts can interact: ecological droughts can potentially make ecosystems more susceptible to flash droughts, while flash droughts can exacerbate the impacts of ongoing ecological droughts (Hacke et al., 2001; Schwalm et al., 2017). The combined effects of both types of drought can intensify stress on ecosystems, complicating and prolonging the recovery process. Previous studies have shown that the spatial patterns of flash drought recovery were similar to those of precipitation, temperature, and radiation (Wang and Yuan, 2022). Increased radiation energy and precipitation following a drought can promote vegetation photosynthesis (Zhang et al., 2021). Additionally, there are regional variations in the time required for drought recovery. Generally, semi-arid and semi-humid areas took longer to recover to their pre-drought state (Fig. 5). Ecosystems in these areas exhibited higher overall sensitivity to drought (Vicente-Serrano et al., 2013; Yang et al., 2016). Vegetation in arid areas adapted to long-term water deficit through various physiological, anatomical, and functional mechanisms, resulting in high drought resistance (Craine et al., 2013). In humid areas, sufficient water storage helped resist drought (Liu et al., 2018; Sun et al., 2023). Vegetation also played a crucial role in regulating the recovery trajectory. The drought resistance of plants was determined using various traits, such as stomatal conductance, hydraulic conductivity, and cell turgor pressure (Bartlett et al., 2016; Martínez-Vilalta et al., 2017). Grasslands and shrublands could quickly recover from drought, whereas forest systems required longer periods of time (Gessler et al., 2017). This may be because grasslands and shrublands have relatively simple vegetation structures, shorter life cycles, and faster growth rates (Ru et al., 2023), whereas forest systems have more complex vegetation structures and ecological processes (Tuinenburg et al., 2022), which can lead to differing responses to environmental changes. Deep roots enhance tree tolerance to drought (McDowell et al., 2008; Nardini et al., 2016). Compared with shallow roots, deep roots have larger conduit diameters and vessel cells, resulting in higher hydraulic conductivity. During droughts, deep roots may play a critical role in water absorption, as increased root growth with soil depth could represent an adaptation to drought conditions (Germon et al., 2020), enabling rapid access to substantial water reserves stored in deeper soils (Christina et al., 2017).
4.3 Limitations and perspectives
We have emphasised that the post-flash-drought recovery trajectory of an ecosystem is influenced by several factors, including post-flash-drought hydrological conditions, flash drought characteristics, and the physiological characteristics of vegetation. However, we should note that the same percentile threshold (20 % or 40 %) was used in this study to identify flash drought events based on empirical values from previous research findings. Further investigation should examine how to determine region-specific thresholds and explore the sensitivity of these thresholds to flash drought recognition (Gou et al., 2022). Furthermore, it is important to consider that plant strategies for coping with flash drought can vary due to species-specific differences (Gupta et al., 2020). There is still a need for improved understanding of the physiological and ecological mechanisms involved in flash drought recovery. To gain a more comprehensive understanding, future research should explore the mechanism of ecosystem restoration from multiple perspectives, such as evaluating greenness and photosynthesis. Although flash droughts can lead to significant short-term disruptions, there remains a need to more comprehensively explore their long-term effects. Future research should prioritise understanding (1) how these intense, short-term drought events might evolve into more conventional droughts and (2) the persistence of their impacts over time (Liu et al., 2023a). Understanding these dynamics will be crucial for predicting and managing the carbon balance and the resilience of ecosystems under changing climate conditions.
Effectively reduction of drought risk and drought exposure is crucial for achieving the SDGs related to health and food security. This study applied a random forest regression model to analyse the factors influencing recovery time, and the response functions were established using partial correlation for typical flash drought recovery time. The most important environmental factor affecting recovery time is post-flash-drought radiation, followed by the aridity index and post-flash-drought temperature. The recovery time is prolonged under lower-solar-radiation conditions. Moreover, semi-arid and sub-humid areas have a longer recovery time. Temperature does not exhibit a monotonic response in relation to recovery time: excessively cold or hot temperatures lead to longer recovery times. Finally, herbaceous vegetation recovers more rapidly than woody forests, with deciduous broadleaf forests demonstrating the shortest recovery time.
Our study assessed the recovery time of ecosystems to flash droughts based on a GPP dataset and identified the dominant factors affecting the recovery time. Results show that 78 % of ecosystems could recover within 0 to 50 d. However, certain areas, such as central China and southern China, required 90 d or more to recover. An analysis of the response functions showed that radiation emerged as the most influential factor impacting flash drought recovery time, with lower-solar-radiation conditions leading to a prolonged recovery time. Additionally, temperature did not exhibit a monotonic response in relation to recovery time. In terms of flash drought characteristics, the difference in recovery time is more associated with speed than with severity and duration.
Although this study provides a good basis for further investigation of flash drought characteristics, it is important to note that the further extension of this study may lead to a more in-depth understanding of flash drought for hydrological applications or worldwide practices. It is important to determine region-specific thresholds and examine the sensitivity of these thresholds to flash drought recognition. Furthermore, plant strategies for coping with flash drought can vary due to species-specific differences. To gain a more comprehensive understanding of flash drought recovery, future research should also explore the mechanism of ecosystem restoration from multiple perspectives, such as evaluating greenness and photosynthesis.
Global Land Evaporation Amsterdam Model (GLEAM) soil moisture data are available from https://www.gleam.eu/#downloads (GLEAM, 2024). The China Meteorological Forcing Dataset (CMFD) is available from Figshare (https://doi.org/10.6084/m9.figshare.c.4557599.v1, He et al., 2020). The FluxSat GPP dataset (version 2) is available from https://doi.org/10.3334/ORNLDAAC/1835 (Joiner and Yoshida, 2021). The MODIS MCD12C1 land cover dataset is available from https://doi.org/10.5067/MODIS/MCD12C1.006 (Friedl and Sulla-Menashe, 2015).
The supplement related to this article is available online at: https://doi.org/10.5194/hess-29-613-2025-supplement.
ML: conceptualisation, methodology, data curation, formal analysis, and writing – original draft; HS: conceptualisation, project administration, supervision, and writing – review and editing; YY, JX, HL, HZ, and WZ: writing – review and editing.
The contact author has declared that none of the authors has any competing interests.
Publisher's note: Copernicus Publications remains neutral with regard to jurisdictional claims made in the text, published maps, institutional affiliations, or any other geographical representation in this paper. While Copernicus Publications makes every effort to include appropriate place names, the final responsibility lies with the authors.
This study was funded by the Third Xinjiang Scientific Expedition Program (grant no. 2022xjkk0105 to Huaiwei Sun). The authors also acknowledge funding from the NSFC (grant nos. 51879110, 52079055, and 52011530128). In addition, Huaiwei Sun acknowledges funding from an NSFC-STINT project (grant no. 202100-3211). Finally, Mengge Lu acknowledges support from the China Scholarship Council (grant no. 202306160083).
This study was funded by the Third Xinjiang Scientific Expedition Program (grant no. 2022xjkk0105 to Huaiwei Sun). The authors also received funding from the NSFC (grant nos. 51879110, 52079055, and 52011530128). In addition, Huaiwei Sun received funding from an NSFC-STINT project (grant no. 202100-3211). Finally, Mengge Lu received support from the China Scholarship Council (grant no. 202306160083).
This paper was edited by Xing Yuan and reviewed by two anonymous referees.
Bartlett, M. K., Klein, T., Jansen, S., Choat, B., and Sack, L.: The correlations and sequence of plant stomatal, hydraulic, and wilting responses to drought, P. Natl. Acad. Sci. USA, 113, 13098–13103, https://doi.org/10.1073/pnas.1604088113, 2016.
Bennett, B. F., Joiner, J., and Yoshida, Y.: Validating satellite based FluxSat v2.0 Gross Primary Production (GPP) trends with FluxNet 2015 eddy covariance observations, in: AGU Fall Meeting 2021: AGU, https://ui.adsabs.harvard.edu/abs/2021AGUFM.B55F1272B (last access: 2 February 2024), 2021.
Breiman, L., Cutler, A., Liaw, A., and Wiener, M.: RandomForest: Breiman and Cutlers Random Forests for Classification and Regression, CRAN: Contributed Packages, The R Foundation, https://doi.org/10.32614/cran.package.randomforest, 2002.
Byrne, B., Liu, J., Lee, M., Yin, Y., Bowman, K. W., Miyazaki, K., Norton, A. J., Joiner, J., Pollard, D. F., Griffith, D. W. T., Velazco, V. A., Deutscher, N. M., Jones, N. B., and Paton-Walsh, C.: The carbon cycle of southeast Australia during 2019–2020: Drought, fires, and subsequent recovery, AGU Advances, 2, e2021AV000469, https://doi.org/10.1029/2021av000469, 2021.
Chen, S., Xiong, L., Ma, Q., Kim, J. S., Chen, J., and Xu, C. Y.: Improving daily spatial precipitation estimates by merging gauge observation with multiple satellite-based precipitation products based on the geographically weighted ridge regression method, J. Hydrol., 589, 125156, https://doi.org/10.1016/j.jhydrol.2020.125156, 2020.
Christina, M., Nouvellon, Y., Laclau, J. P., Stape, J. L., Bouillet, J., Lambais, G. R., and Maire, G.: Importance of deep water uptake in tropical eucalypt forest, Funct. Ecol., 31, 509–519, 2017.
Christian, J. I., Martin, E. R., Basara, J. B., Furtado, J. C., Otkin, J. A., Lowman, L. L., Hunt, E. D., Mishra, V., and Xiao, X. M.: Global projections of flash drought show increased risk in a warming climate, Commun. Earth. Environ., 4, 165, https://doi.org/10.1038/s43247-023-00826-1, 2023.
Craine, J. M., Ocheltree, T. W., Nippert, J. B., Gene Towne, E., Skibbe, A. M., Kembel, S. W., and Fargione, J. E.: Global diversity of drought tolerance and grassland climate-change resilience, Nat. Clim. Change, 3, 63–67, 2013.
Cravens, A. E., McEvoy, J., Zoanni, D., Crausbay, S., Ramirez, A., and Cooper, A. E.: Integrating Ecological Impacts: Perspectives on Drought in the Upper Missouri Headwaters, Montana, United States, Weather Clim. Soc., 13, 363–376, 2021.
Cook, B. I., Mankin, J. S., Marvel, K., Williams, A. P., Smerdon, J. E., and Anchukaitis, K. J.: Twenty-First Century Drought Projections in the CMIP6 Forcing Scenarios, Earth Future, 8, e2019EF001461, https://doi.org/10.1029/2019EF001461, 2020.
Darnhofer, I., Lamine, C., Strauss, A., and Navarrete, M.: The resilience of family farms: Towards a relational approach, J. Rural Stud., 44, 111–122, 2016.
Douris, J. and Kim, G.: The Atlas of Mortality and Economic Losses from Weather, Climate and Water Extremes, Climate Change and Law Collection, https://coilink.org/20.500.12592/b3d1n6 (last access: 2 February 2024), 2021.
Fathi-Taperasht, A., Shafizadeh-Moghadam, H., Minaei, M., and Xu, T.: Influence of drought duration and severity on drought recovery period for different land cover types: evaluation using MODIS-based indices, Ecol. Indic., 141, 109146, https://doi.org/10.1016/j.ecolind.2022.109146, 2022.
Friedl, M. and Sulla-Menashe, D.: MCD12C1 MODIS/Terra+Aqua Land Cover Type Yearly L3 Global 0.05Deg CMG V006, NASA EOSDIS Land Processes distributed Active Archive Center, USGS [data set], https://doi.org/10.5067/MODIS/MCD12C1.006, 2015.
Friedl, M. A., McIver, D. K., Hodges, J. C., Zhang, X. Y., Muchoney, D., Strahler, A. H., Woodcock, C. E., Gopal, S., Schneider, A., and Cooper, A.: Global land cover mapping from MODIS: algorithms and early results, Remote Sens. Environ., 83, 287–302, 2002.
Fu, Z., Li, D., Hararuk, O., Schwalm, C., Luo, Y., Yan, L., and Niu, S.: Recovery time and state change of terrestrial carbon cycle after disturbance, Environ. Res. Lett., 12, 104004, https://doi.org/10.1088/1748-9326/aa8a5c, 2017.
Gazol, A., Camarero, J. J., Anderegg, W. R. L., and Vicente-Serrano, S. M.: Impacts of droughts on the growth resilience of Northern Hemisphere forests, Glob. Ecol. Biogeogr., 26, 166–176, https://doi.org/10.1111/geb.12526, 2017.
Gazol, A., Camarero, J. J., Vicente-Serrano, S. M., Sánchez-Salguero, R., Gutiérrez, E., de Luis, M., Sangüesa-Barreda, G., Novak, K., Rozas, V., Tíscar, P. A., Linares, J. C., Martín-Hernández, N., Martínez del Castillo, E., Ribas, M., García-González, I., Silla, F., Camisón, A., Génova, M., Olano, J. M., Longares, L. A., Hevia, A., Tomás-Burguera, M., and Galván, J. D.: Forest resilience to drought varies across biomes, Glob. Change Biol., 24, 2143–2158, 2018.
Germon, A., Laclau, J.-P., Robin, A., and Jourdan, C.: Tamm Review: Deep fine roots in forest ecosystems: Why dig deeper?, Forest Ecol. Manag., 466, 118135, https://doi.org/10.1016/j.foreco.2020.118135, 2020.
Gessler, A., Schaub, M., and McDowell, N. G.: The role of nutrients in drought-induced tree mortality and recovery, New Phytol., 214, 513–520, 2017.
GLEAM: Method GLEAM, Global Land Evaporation Amsterdam Model, GLEAM [data set], https://www.gleam.eu/#downloads, last access: 2 February 2024.
Godde, C., Dizyee, K., Ash, A., Thornton, P., Sloat, L., Roura, E., Henderson, B., and Herrero, M.: Climate change and variability impacts on grazing herds: Insights from a system dynamics approach for semi-arid Australian rangelands, Glob. Change Biol., 25, 3091–3109, 2019.
Gou, Q., Zhu, Y., Lü, H., Horton, R., Yu, X., Zhang, H., Wang, X., Su, J., Liu, E., and Ding, Z.: Application of an improved spatio-temporal identification method of flash droughts, J. Hydrol., 604, 127224, https://doi.org/10.1016/j.jhydrol.2021.127224, 2022.
Gupta, A., Rico-Medina, A., and Caño-Delgado, A. I.: The physiology of plant responses to drought, Science, 368, 266–269, https://doi.org/10.1126/science.aaz7614, 2020.
Hacke, U. G., Stiller, V., Sperry, J. S., Pittermann, J., and McCulloh, K. A.: Cavitation Fatigue. Embolism and Refilling Cycles Can Weaken the Cavitation Resistance of Xylem1, Plant Physiol., 125, 779–786, https://doi.org/10.1104/pp.125.2.779, 2001.
Hao, Y. and Choi, M.: Recovery of ecosystem carbon and water fluxes after drought in China, J. Hydrol., 622, 129766, https://doi.org/10.1016/j.jhydrol.2023.129766, 2023.
He, B., Liu, J., Guo, L., Wu, X., Xie, X., Zhang, Y., Chen, C., Zhong, Z., and Chen, Z.: Recovery of Ecosystem Carbon and Energy Fluxes From the 2003 Drought in Europe and the 2012 Drought in the United States, Geophys. Res. Lett., 45, 4879–4888, 2018.
He, J., Yang, K., Tang, W., Lu, H., Qin, J., Chen, Y., and Li, X.: The first high-resolution meteorological forcing dataset for land process studies over China, Sci. Data, 7, 25, https://doi.org/10.1038/s41597-020-0369-y, 2020 (data available at: https://doi.org/10.6084/m9.figshare.c.4557599.v1).
Huang, J., Yu, H., Guan, X., Wang, G., and Guo, R.: Accelerated dryland expansion under climate change, Nat. Clim. Change, 6, 166–171, 2016.
Huxman, T. E., Smith, M. D., Fay, P. A., Knapp, A. K., Shaw, M. R., Loik, M. E., Smith, S. D., Tissue, D. T., Zak, J. C., and Weltzin, J. F.: Convergence across biomes to a common rain-use efficiency, Nature, 429, 651–654, 2004.
Jiao, T., Williams, C. A., De Kauwe, M. G., Schwalm, C. R., and Medlyn, B. E.: Patterns of post-drought recovery are strongly influenced by drought duration, frequency, post-drought wetness, and bioclimatic setting, Glob. Change Biol., 27, 4630–4643, 2021.
Joiner, J. and Yoshida, Y.: Global MODIS and FLUXNET-derived Daily Gross Primary Production, V2, ORNL DAAC, Oak Ridge, Tennessee, USA, Earth Data [data set], https://doi.org/10.3334/ORNLDAAC/1835, 2021.
Kannenberg, S. A., Novick, K. A., Alexander, M. R., Maxwell, J. T., Moore, D. J. P., Phillips, R. P., and Anderegg, W. R. L.: Linking drought legacy effects across scales: From leaves to tree rings to ecosystems, Glob. Change Biol., 25, 2978–2992, https://doi.org/10.1111/gcb.14710, 2019.
Kannenberg, S. A., Schwalm, C. R., and Anderegg, W. R. L.: Ghosts of the past: how drought legacy effects shape forest functioning and carbon cycling, Ecol. Lett., 23, 891–901, 2020.
Lenton, T. M., Held, H., Kriegler, E., Hall, J. W., Lucht, W., Rahmstorf, S., and Schellnhuber, H. J.: Tipping elements in the Earth's climate system, P. Natl. Acad. Sci. USA, 105, 1786–1793, https://doi.org/10.1073/pnas.0705414105, 2008.
Lesinger, K. and Tian, D.: Trends, Variability, and Drivers of Flash Droughts in the Contiguous United States, Water Resour. Res., 58, e2022WR032186, https://doi.org/10.1029/2022WR032186, 2022.
Li, L., Yao, N., Li, Y., Li Liu, D., Wang, B., and Ayantobo, O. O.: Future projections of extreme temperature events in different sub-regions of China, Atmos. Res., 217, 150–164, 2019.
Li, T., Wang, S., Chen, B., Wang, Y., Chen, S., Chen, J., Xiao, Y., Xia, Y., Zhao, Z., and Chen, X.: Widespread reduction in gross primary productivity caused by the compound heat and drought in Yangtze River Basin in 2022, Environ. Res. Lett., 19, 034048, https://doi.org/10.1088/1748-9326/ad2cac, 2024.
Lindoso, D. P., Eiró, F., Bursztyn, M., Rodrigues-Filho, S., and Nasuti, S.: Harvesting water for living with drought: Insights from the Brazilian human coexistence with semi-aridity approach towards achieving the sustainable development goals, Sustainability, 10, 622, https://doi.org/10.3390/su10030622, 2018.
Liu, L., Gudmundsson, L., Hauser, M., Qin, D., Li, S., and Seneviratne, S. I.: Revisiting assessments of ecosystem drought recovery, Environ. Res. Lett., 14, 114028, https://doi.org/10.1088/1748-9326/ab4c61, 2019.
Liu, Y., Zhu, Y., Ren, L., Singh, V. P., and Yuan, S.: Flash drought fades away under the effect of accumulated water deficits: the persistence and transition to conventional drought, Environ. Res. Lett., 18, 114035, https://doi.org/10.1088/1748-9326/acfccb, 2023a.
Liu, Y., Yuan, S., Zhu, Y., Ren, L., Chen, R., Zhu, X., and Xia, R.: The patterns, magnitude, and drivers of unprecedented 2022 mega-drought in the Yangtze River Basin, China, Environ. Res. Lett., 18, 114006, https://doi.org/10.1088/1748-9326/acfe21, 2023b.
Liu, Y. Y., van Dijk, A. I., Miralles, D. G., McCabe, M. F., Evans, J. P., de Jeu, R. A., Gentine, P., Huete, A., Parinussa, R. M., and Wang, L.: Enhanced canopy growth precedes senescence in 2005 and 2010 Amazonian droughts, Remote Sens. Environ., 211, 26–37, 2018.
Lu, M., Sun, H., Cheng, L., Li, S., Qin, H., Yi, S., Zhang, H., and Zhang, W.: Heterogeneity in vegetation recovery rates post-flash droughts across different ecosystems, Environ. Res. Lett., 19, 074028, https://doi.org/10.1088/1748-9326/ad5570, 2024.
Lundberg, S. M. and Lee, S.-I.: A unified approach to interpreting model predictions, in: Proceedings of the 31st International Conference on Neural Information Processing Systems, Long Beach, California, USA, 4–9 December 2017, 4768-477, https://doi.org/10.48550/arXiv.1705.07874, 2017.
Martin, D. P.: Partial dependence plots, http://dpmartin42.github.io/posts/r/partial-dependence (last access: 2 February 2024), 2014.
Martínez-Vilalta, J. and Garcia-Forner, N.: Water potential regulation, stomatal behaviour and hydraulic transport under drought: deconstructing the iso/anisohydric concept, Plant Cell Environ., 40, 962–976, https://doi.org/10.1111/pce.12846, 2017.
McDowell, N., Pockman, W. T., Allen, C. D., Breshears, D. D., Cobb, N., Kolb, T., Plaut, J., Sperry, J., West, A., Williams, D. G., and Yepez, E. A.: Mechanisms of plant survival and mortality during drought: why do some plants survive while others succumb to drought?, New Phytol., 178, 719–739, https://doi.org/10.1111/j.1469-8137.2008.02436.x, 2008.
Miyashita, K., Tanakamaru, S., Maitani, T., and Kimura, K.: Recovery responses of photosynthesis, transpiration, and stomatal conductance in kidney bean following drought stress, Environ. Exp. Bot., 53, 205–214, 2005.
Mohammadi, K., Jiang, Y., and Wang, G.: Flash drought early warning based on the trajectory of solar-induced chlorophyll fluorescence, P. Natl. Acad. Sci. USA, 119, e2202767119, https://doi.org/10.1073/pnas.2202767119, 2022.
Nardini, A., Casolo, V., Dal Borgo, A., Savi, T., Stenni, B., Bertoncin, P., Zini, L., and McDowell, N. G.: Rooting depth, water relations and non-structural carbohydrate dynamics in three woody angiosperms differentially affected by an extreme summer drought, Plant Cell Environ., 39, 618–627, https://doi.org/10.1111/pce.12646, 2016.
Nilsson, M., Griggs, D., and Visbeck, M.: Policy: map the interactions between Sustainable Development Goals, Nature, 534, 320–322, 2016.
Otkin, J. A., Zhong, Y., Hunt, E. D., Basara, J., Svoboda, M., Anderson, M. C., and Hain, C.: Assessing the evolution of soil moisture and vegetation conditions during a flash drought–flash recovery sequence over the South-Central United States, J. Hydrometeorol., 20, 549–562, 2019.
Peixoto, J. and Oort, A. H.: The Climatology of Relative Humidity in the Atmosphere, J. Climate, 9, 3443–3463, 1996.
Poonia, V., Goyal, M. K., Jha, S., and Dubey, S.: Terrestrial ecosystem response to flash droughts over India, J. Hydrol., 605, 127402, https://doi.org/10.1016/j.jhydrol.2021.127402, 2022.
Qing, Y., Wang, S., Ancell, B. C., and Yang, Z.-L.: Accelerating flash droughts induced by the joint influence of soil moisture depletion and atmospheric aridity, Nat. Commun., 13, 1139, https://doi.org/10.1038/s41467-022-28752-4, 2022.
Rigden, A., Mueller, N. v., Holbrook, N., Pillai, N., and Huybers, P.: Combined influence of soil moisture and atmospheric evaporative demand is important for accurately predicting US maize yields, Nat. Food., 1, 127–133, 2020.
Ru, J., Wan, S., Hui, D., and Song, J.: Overcompensation of ecosystem productivity following sustained extreme drought in a semiarid grassland, Ecology, 104, e3997, https://doi.org/10.1002/ecy.3997, 2023.
Sadiqi, S. S. J., Hong, E.-M., Nam, W.-H., and Kim, T.: An integrated framework for understanding ecological drought and drought resistance, Sci. Total Environ., 846, 157477, https://doi.org/10.1016/j.scitotenv.2022.157477, 2022.
Schwalm, C. R., Anderegg, W. R., Michalak, A. M., Fisher, J. B., Biondi, F., Koch, G., Litvak, M., Ogle, K., Shaw, J. D., Wolf, A., Huntzinger, D. N., Schaefer, K., Cook, R., Wei, Y. X., Fang, Y. Y., Hayes, D., Huang, M. Y., Jain, A., and Tian, H.: Global patterns of drought recovery, Nature, 548, 202–205, https://doi.org/10.1038/nature23021, 2017.
Sreeparvathy, V. and Srinivas, V.: Meteorological flash droughts risk projections based on CMIP6 climate change scenarios, npj Clim. Atmos. Sci., 5, 77, https://doi.org/10.1038/s41612-022-00302-1, 2022.
Štrumbelj, E. and Kononenko, I.: Explaining prediction models and individual predictions with feature contributions, Knowl. Inf. Syst., 41, 647–665, 2014.
Sun, H., Gui, D., Yan, B., Liu, Y., Liao, W., Zhu, Y., Lu, C., and Zhao, N.: Assessing the potential of random forest method for estimating solar radiation using air pollution index, Energy Conv. Manag., 119, 121–129, 2016.
Sun, H., Lu, M., Yang, Y., Chen, J., Wang, J., Yan, D., Xue, J., and Zhang, W.: Revisiting the role of transpiration in the variation of ecosystem water use efficiency in China, Agr. Forest Meteorol., 332, 109344, https://doi.org/10.1016/j.agrformet.2023.109344, 2023.
Tabari, H. and Willems, P.: More prolonged droughts by the end of the century in the Middle East, Environ. Res. Lett., 13, 104005, https://doi.org/10.1088/1748-9326/aae09c, 2018.
Tabari, H. and Willems, P.: Sustainable development substantially reduces the risk of future drought impacts, Commun. Earth Environ., 4, 180, https://doi.org/10.1038/s43247-023-00840-3, 2023.
Tuinenburg, O. A., Bosmans, J. H., and Staal, A.: The global potential of forest restoration for drought mitigation, Environ. Res. Lett., 17, 034045, https://doi.org/10.1088/1748-9326/ac55b8, 2022.
Tyagi, S., Zhang, X., Saraswat, D., Sahany, S., Mishra, S. K., and Niyogi, D.: Flash Drought: Review of Concept, Prediction and the Potential for Machine Learning, Deep Learning Methods, Earth Future., 10, e2022EF002723, https://doi.org/10.1029/2022EF002723, 2022.
Vicente-Serrano, S. M., Gouveia, C., Camarero, J. J., Beguería, S., Trigo, R., López-Moreno, J. I., Azorín-Molina, C., Pasho, E., Lorenzo-Lacruz, J., Revuelto, J., Morán-Tejeda, E., and Sanchez-Lorenzo, A.: Response of vegetation to drought time-scales across global land biomes, P. Natl. Acad. Sci. USA, 110, 52–57, https://doi.org/10.1073/pnas.1207068110, 2013.
Wang, H., Zhu, Q., Wang, Y., and Zhang, H.: Spatio-temporal characteristics and driving factors of flash drought recovery: From the perspective of soil moisture and GPP changes, Weather Climate Extremes, 42, 100605, https://doi.org/10.1016/j.wace.2023.100605, 2023.
Wang, L., Yuan, X., Xie, Z., Wu, P., and Li, Y.: Increasing flash droughts over China during the recent global warming hiatus, Sci. Rep., 6, 30571, https://doi.org/10.1038/srep30571, 2016.
Wang, S., Peng, H., and Liang, S.: Prediction of estuarine water quality using interpretable machine learning approach, J. Hydrol., 605, 127320, https://doi.org/10.1016/j.jhydrol.2021.127320, 2022.
Wang, Y. and Yuan, X.: Land-atmosphere coupling speeds up flash drought onset, Sci. Total Environ., 851, 158109, https://doi.org/10.1016/j.scitotenv.2022.158109, 2022.
Wang, Y. and Yuan, X.: High Temperature Accelerates Onset Speed of the 2022 Unprecedented Flash Drought Over the Yangtze River Basin, Geophys. Res. Lett., 50, e2023GL105375, https://doi.org/10.1029/2023GL105375, 2023.
Wu, X., Liu, H., Li, X., Ciais, P., Babst, F., Guo, W., Zhang, C., Magliulo, V., Pavelka, M., Liu, S., Huang, Y., Wang, P., Shi, C., and Ma, Y.: Differentiating drought legacy effects on vegetation growth over the temperate Northern Hemisphere, Glob. Change Biol., 24, 504–516, https://doi.org/10.1111/gcb.13920, 2018.
Xi, X., Liang, M., and Yuan, X.: Increased atmospheric water stress on gross primary productivity during flash droughts over China from 1961 to 2022, Weather Climate Extremes, 44, 100667, https://doi.org/10.1016/j.wace.2024.100667, 2024.
Xu, S., Wang, Y., Liu, Y., Li, J., Qian, K., Yang, X., and Ma, X.: Evaluating the cumulative and time-lag effects of vegetation response to drought in Central Asia under changing environments, J. Hydrol., 627, 130455, https://doi.org/10.1016/j.jhydrol.2023.130455, 2023.
Yang, L., Wang, W., and Wei, J.: Assessing the response of vegetation photosynthesis to flash drought events based on a new identification framework, Agr. Forest Meteorol., 339, 109545, https://doi.org/10.1016/j.agrformet.2023.109545, 2023.
Yang, Y., Guan, H., Batelaan, O., McVicar, T. R., Long, D., Piao, S., Liang, W., Liu, B., Jin, Z., and Simmons, C. T.: Contrasting responses of water use efficiency to drought across global terrestrial ecosystems, Sci. Rep., 6, 23284, https://doi.org/10.1038/srep23284, 2016.
Yao, T., Liu, S., Hu, S., and Mo, X.: Response of vegetation ecosystems to flash drought with solar-induced chlorophyll fluorescence over the Hai River Basin, China during 2001–2019, J. Environ. Manage., 313, 114947, https://doi.org/10.1016/j.jenvman.2022.114947, 2022.
Yu, Z., Wang, J., Liu, S., Rentch, J. S., Sun, P., and Lu, C.: Global gross primary productivity and water use efficiency changes under drought stress, Environ. Res. Lett., 12, 014016, https://doi.org/10.1088/1748-9326/aa5258, 2017.
Yuan, X., Wang, L., Wu, P., Ji, P., Sheffield, J., and Zhang, M.: Anthropogenic shift towards higher risk of flash drought over China, Nat. Commun., 10, 4661, https://doi.org/10.1038/s41467-019-12692-7, 2019.
Yuan, X., Wang, Y., Ji, P., Wu, P., Sheffield, J., and Otkin, J. A.: A global transition to flash droughts under climate change, Science, 380, 187–191, https://doi.org/10.1126/science.abn6301, 2023.
Zha, X., Xiong, L., Liu, C., Shu, P., and Xiong, B.: Identification and evaluation of soil moisture flash drought by a nonstationary framework considering climate and land cover changes, Sci. Total Environ., 856, 158953, https://doi.org/10.1016/j.scitotenv.2022.158953, 2023.
Zhang, M. and Yuan, X.: Rapid reduction in ecosystem productivity caused by flash droughts based on decade-long FLUXNET observations, Hydrol. Earth Syst. Sci., 24, 5579–5593, https://doi.org/10.5194/hess-24-5579-2020, 2020.
Zhang, M., Yuan, X., and Otkin, J. A.: Remote sensing of the impact of flash drought events on terrestrial carbon dynamics over China, Carbon Balanc. Manag., 15, 20, https://doi.org/10.1186/s13021-020-00156-1, 2020.
Zhang, S., Yang, Y., Wu, X., Li, X., and Shi, F.: Postdrought recovery time across global terrestrial ecosystems, J. Geophys. Res.-Biogeo., 126, e2020JG005699, https://doi.org/10.1029/2020jg005699, 2021.
Zhang, X., Chen, N., Sheng, H., Ip, C., Yang, L., Chen, Y., Sang, Z., Tadesse, T., Lim, T. P. Y., and Rajabifard, A.: Urban drought challenge to 2030 sustainable development goals, Sci. Total Environ., 693, 133536, https://doi.org/10.1016/j.scitotenv.2019.07.342, 2019.
Zomer, R. J., Xu, J., and Trabucco, A.: Version 3 of the global aridity index and potential evapotranspiration database, Sci. Data., 9, 409, https://doi.org/10.1038/s41597-022-01493-1, 2022.