the Creative Commons Attribution 4.0 License.
the Creative Commons Attribution 4.0 License.
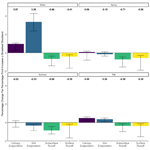
Broadleaf afforestation impacts on terrestrial hydrology insignificant compared to climate change in Great Britain
Marcus Buechel
Louise Slater
Simon Dadson
Widespread afforestation has been proposed internationally to reduce atmospheric carbon dioxide; however, the specific hydrological consequences and benefits of such large-scale afforestation (e.g. natural flood management) are poorly understood. We use a high-resolution land surface model, the Joint UK Land Environment Simulator (JULES), with realistic potential afforestation scenarios to quantify possible hydrological change across Great Britain in both present and projected climate. We assess whether proposed afforestation produces significantly different regional responses across regions; whether hydrological fluxes, stores and events are significantly altered by afforestation relative to climate; and how future hydrological processes may be altered up to 2050. Additionally, this enables determination of the relative sensitivity of land surface process representation in JULES compared to climate changes. For these three aims we run simulations using (i) past climate with proposed land cover changes and known floods and drought events; (ii) past climate with independent changes in precipitation, temperature, and CO2; and (iii) a potential future climate (2020–2050). We find the proposed scale of afforestation is unlikely to significantly alter regional hydrology; however, it can noticeably decrease low flows whilst not reducing high flows. The afforestation levels minimally impact hydrological processes compared to changes in precipitation, temperature, and CO2. Warming average temperatures (+3 °C) decreases streamflow, while rising precipitation (130 %) and CO2 (600 ppm) increase streamflow. Changes in high flow are generated because of evaporative parameterizations, whereas low flows are controlled by runoff model parameterizations. In this study, land surface parameters within a land surface model do not substantially alter hydrological processes when compared to climate.
- Article
(2495 KB) - Full-text XML
-
Supplement
(1064 KB) - BibTeX
- EndNote
Land cover (e.g. grassland and bare ground) exerts a strong control on catchment hydrology (Rogger et al., 2017; Blöschl et al., 2007; Pattison and Lane, 2012). A land use or land cover change (LULC) can obscure the impact of climate on streamflow. LULC alters streamflow by changing hydrological processes (e.g. subsurface flow) over multiple spatial and temporal scales. One example of LULC is afforestation, which can lower catchment water tables (Peskett et al., 2020; Kellner and Hubbart, 2018; Shuttleworth et al., 2019), increase precipitation downwind (Meier et al., 2021; Teuling et al., 2019; Xu et al., 2022), and alter transpiration rates over time (Hudson et al., 1997; Newson and Calder, 1989; Marc and Robinson, 2007). Many studies suggest afforestation can reduce overall streamflow; however, land management practices (e.g. artificial ditching and road cutting) may also increase peak streamflow (Beschta et al., 2000; Bathurst et al., 2018). It is therefore important to understand all the hydrological processes of afforestation (and other LULC), especially as the climate changes and the hydrological cycle intensifies (Hung et al., 2020; Kundzewicz, 2011; IPPC, 2019). Changing rainfall (Fowler et al., 2021; Gao et al., 2018), temperature (Wasko, 2021), and carbon dioxide (Betts et al., 2007; Gedney et al., 2006) could all influence how large-scale afforestation impacts hydrology.
Potential afforestation benefits include the reduction in atmospheric CO2 (Cook-Patton et al., 2020; Hawes, 2018; Palmer, 2021; Griscom et al., 2017), moderation of temperature extremes (Schwaab et al., 2020; O'Briain et al., 2020), reduction in air and noise pollution (Fenner, 2017; Oldfield et al., 2013), provision of areas of societal wellbeing (Dick et al., 2019), and reduction in flood risk as a form of natural flood management (NFM) (Dadson et al., 2017; M. Cooper et al., 2021). These potential benefits have led governments and businesses to pledge woodland acreage increases (Lewis et al., 2019; Seddon et al., 2020), but there is a need to quantify the actual (and not merely perceived) advantages of planting the right trees in the right place (Seddon et al., 2021; Grassi et al., 2017). The UK Government plans to expand woodland from 13 % to 17 % of land cover by 2050 to reach net zero (Committee on Climate Change, 2019a, b). As the UK experiences larger and more frequent floods (Griffin et al., 2019; Hannaford et al., 2021), it is important to learn how afforestation could reduce flood peaks. Therefore, additional research is required to interrogate the hydrological response to afforestation over regional to continental scales.
Determining woodland influence on hydrology is not new (Andréassian, 2004). Studies investigating afforestation impact on streamflow have used global streamflow datasets (Bradshaw et al., 2007; Do et al., 2017), paired catchments (Bosch and Hewlett, 1982; Bathurst et al., 2018; Brown et al., 2013), and modelling (Iacob et al., 2017; Li et al., 2018; Speich et al., 2018). Recent UK studies show how wet canopy evaporation can reduce high runoff (Page et al., 2020) and afforestation can increase saturated soil hydraulic conductivity in upland areas (Murphy et al., 2021). These results, and others, have been taken by some to suggest that afforestation can reduce flooding with greater water usage, higher infiltration rates, and increased floodplain hydraulic roughness (Nisbet et al., 2011; M. Cooper et al., 2021). However, it remains unclear whether hydrological afforestation effects have a detectable hydrological impact over areas larger than 50 km2 due to the small scales of existing studies of afforestation hydrology (Dadson et al., 2017; Rogger et al., 2017; Nisbet and Thomas, 2021). Further uncertainty exists between empirical studies and model results regarding peak streamflow generation following afforestation (Carrick et al., 2019; Stratford et al., 2017), making it difficult to transfer afforestation impacts on catchment hydrology over large regions.
Process-based numerical models provide one way to understand the consequences of afforestation (Gush et al., 2002; Bonan, 2008). They incorporate known processes to determine system responses to scenarios (e.g. projected climate). Many studies have applied numerical models to determine afforestation's role in reducing flood risk, although few have considered areas larger than a single catchment (Stratford et al., 2017). Land surface models (LSMs) incorporate a suite of known Earth system processes and have been used to study countrywide and continental hydrology (Blyth et al., 2021; Prudhomme et al., 2012). Inclusion of plant functional types, nutrient cycling, physiological forcing, and surface energy fluxes mean LSMs are well suited to investigate afforestation mechanistic impacts on the hydrosphere compared to simpler models. This is essential for assessing the hydrological response to a system as complex as vegetation change (Rogger et al., 2017). LSMs should therefore quantify projected hydrological changes whilst modellers determine if outputs are realistic. Previous work using an LSM has shown tree planting location has a minimal impact compared to the extent planted within catchments (Buechel et al., 2022). It is unknown whether this finding is true across all regions of Great Britain in a changing climate and given the fidelity of the model parameterizations.
In this study, the impact of increasing broadleaf afforestation on Great Britain's hydrology is analysed with a high-resolution LSM. The approach allows direct comparison of streamflow with and without afforestation on a countrywide scale whilst determining processes and catchment attributes driving modelled hydrological changes. Three central questions are investigated about realistic afforestation scenarios influencing countrywide hydrology.
-
What is the hydrological response to afforestation across Great Britain and how does it vary regionally?
-
How much of an impact would realistic afforestation scenarios have on hydrological processes compared to potential changes in climate?
-
How might realistic afforestation alter future hydrological scenarios up to 2050?
We evaluate these questions by quantifying how plausible afforestation across Great Britain could influence catchment hydrology, identifying changes across the streamflow spectrum, and testing the fitness for purpose of model process representation.
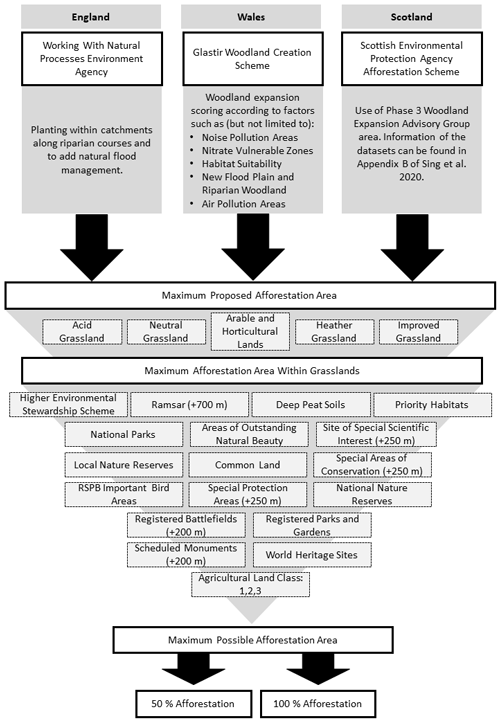
Figure 1Flow diagram explaining the creation of the two realistic afforestation scenarios. The top row indicates the three afforestation scenarios developed for England, Scotland, and Wales. The spatial extent of these scenarios is reduced by selecting the scenario areas that intersect with grasslands (as defined by the CEH Land Cover Map 2000) and several other factors as shown above (such as Areas of Outstanding Natural Beauty and Special Protection Areas). The 100 % and 50 % scenarios are created from the maximum possible afforestation area calculated.
2.1 Plausible afforestation scenarios
Several afforestation scenarios have been used to investigate the hydrological consequences of afforestation, such as the “global restoration potential” dataset (Bastin et al., 2019; Meier et al., 2021; Hoek van Dijke et al., 2022), but its realism has been questioned (Friedlingstein et al., 2019; Wilkes et al., 2020). Hydrological conclusions derived from such unlikely scenarios are therefore equally questionable. The UK Government's Net Zero Strategy includes planting 30 000 ha of trees, equivalent to sequestering 14 MtCO2e per year from 2024 onwards (Committee on Climate Change, 2018). This roughly approximates to 900 000 ha of additional woodland across the country by 2050 (30 000 ha for 30 years from 2020). Plausible afforestation scenarios for Great Britain should emphasis planting trees in areas that accomplish maximal societal benefits (Bradfer-Lawrence et al., 2014; Burke et al., 2021). This study produces credible afforestation extents with multiple purposes and minimal resistance (Fig. 1) by altering three previously developed afforestation scenarios for the countries of Great Britain: England, Wales, and Scotland. Northern Ireland is not included as model driving datasets do not cover this region. The scenario for England is the Environment Agency's Working with Natural Processes programme, which aims to reduce flood risk and restore the natural regulating function of catchments (Environment Agency, 2018). In Wales, the Glastir Woodland Creation opportunities map is utilized, which aims to plant trees for maximal benefits (Welsh Government, 2022). Finally, for Scotland, the Woodland Expansion Advisory Group's map is used that identified areas with the greatest potential for woodland expansion (Sing and Aitkenhead, 2020).
Woodland extent is spatially constrained for the chosen afforestation scenarios; however, further limits are applied to promote afforestation in low-risk locations. Principles to expand woodland into areas that minimally interfere with existing land practices are used, developed by the Scottish Woodland Expansion Advisory Group and the Forestry Commission (Sing and Aitkenhead, 2020). Afforestation takes place in acid grassland, arable and horticultural areas, heather, heather grassland, improved grassland, and neutral grassland as defined by the CEH Land Cover 2000 (Fuller et al., 2002) (Fig. 1). Woodland expansion does not encroach on urban areas, existing woodland, shrubland, bare ground, inland water, or upon biodiversity-rich grasslands (by excluding it from priority habitat areas). To note, grassland afforestation can decrease soil carbon and not sequester additional carbon over the short term (Don et al., 2009). Other spatial constraints for woodland expansion are summarized in Fig. 1. Across Great Britain, this afforestation criteria creates a geographical imbalance with largest potential afforestation areas in Scotland followed by Wales and then England (Fig. 2). This is due to more land being initially identified in Scotland and Wales for afforestation and greater areal constraints to afforestation in England. A maximal potential afforestation area of 3.53 million ha is generated in Great Britain using these afforestation constraints (approximately 700 000 ha less than the “global restoration potential”).
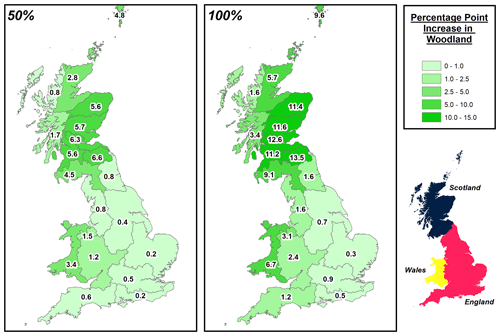
Figure 2Percentage point increase in broadleaf woodland for the two realistic afforestation scenarios generated for each of the 20 UKCP18 hydro-regions in Great Britain.
Here, 900 000 ha of broadleaf woodland is randomly “planted”, at a 25 m resolution, within the generated maximum afforestation extent (on the same projected coordinate system as the CEH Land Cover Map 2000; Fuller et al., 2002). In addition, another scenario with approximately 450 000 ha of woodland (if 15 000 ha were planted for 30 years) is made to represent afforestation at similar present rates (Forest Research, 2021a). Woodland extent across Great Britain changes from 12.3 % to 14.3 % and 16.2 % for the 50 % and 100 % afforestation scenarios respectively (using the CEH Land Cover Map 2000). These two afforestation scenarios are combined with the CEH Land Cover Map 2000 (Fuller et al., 2002) and scaled to a 1 km2 grid, similar to the CHESS-land dataset (Martinez-de la Torre et al., 2018), by calculating the fraction of eight different land cover types detailed in the following section. Arguably these scenarios are a restrictive level of afforestation, but they appear ambitious when compared to current afforestation rates of approximately 10 000 ha yr−1 (Forest Research, 2021b). The two afforestation scenarios include two static increases in broadleaf woodland and do not change the rates of afforestation over the time of the experiments. Changes in hydrological processes are compared between the base land cover and meteorology and with the two afforestation scenarios (and meteorological changes) as described below.
2.2 Modelling methodology
This study is split into three parts for each research question. This enables a coherent understanding of afforestation on potential past, present, and future hydrology within the model domain. First, the potential hydrological benefits, and drawbacks, of afforestation scenarios are considered under present climate conditions. Second, a simple factorial sensitivity analysis is undertaken to ascertain the relative importance of afforestation and climate on future hydrological changes and model process parameterization. Finally, a future climate scenario is utilized to project how plausible future afforestation may alter hydrology in Great Britain up to 2050.
2.2.1 Model description
The Joint UK Land Environment Simulator (JULES), a physically based LSM, is used and simulates water, carbon, and energy stores and fluxes (Best et al., 2011; D. B. Clark et al., 2011). JULES has been used many times previously, for example in determining the climatic impact of the Montreal protocol (Young et al., 2021), assessing trends in evapotranspiration across Great Britain since the 1960s (Blyth et al., 2019), and aiding production of high-resolution UK soil moisture datasets (Peng et al., 2021). Due to the complexity and high number of free and fixed parameters of JULES, the base validated model configuration of Buechel et al. (2022), Robinson et al. (2017b) and Martínez-de la Torre et al. (2019) is utilized. Configuration details are found in Buechel et al. (2022) and accessible as Rose suite u-ce663 from the Met Office Rose/Cylc suite control system (https://metomi.github.io/rose/doc/html/index.html, last access: 21 April 2024). The CHESS-land dataset specifies JULES' bounding conditions including soil hydraulic, thermal, vegetation, and orographic properties at a 1 km2 spatial resolution (Martinez-de la Torre et al., 2018). CHESS-met provides the meteorological information of air temperature, pressure, specific humidity, and short- and long-wave radiation for the first two parts of the study at a daily temporal resolution (Robinson et al., 2017a). In the final study section, we utilize CHESS-SCAPE (Robinson et al., 2022), a 1 km2 downscaled UKCP18 projection (Lowe et al., 2018) with all the same variables and spatial and temporal resolution of CHESS-met.
JULES runs at a numerical time step of 30 min. The land surface is divided into eight possible types, five vegetated (broadleaf, needleleaf, C3 grass, C4 grass (crops), shrubs) and three non-vegetated (urban, inland water, bare soil) as based on the CEH Land Cover Map 2000. Conversion between the CEH Land Cover Map 2000 and the eight land types mentioned can be found in the Supplement of Buechel et al. (2022). Precipitation covers a grid cell according to a constant dependent on temperature (Best et al., 2009) and is intercepted by vegetation, as a function of the leaf area index (LAI). Canopy throughfall is a function of the existing canopy water, rainfall, and the maximum amount of canopy water (related to LAI). Evapotranspiration is calculated using effective surface resistance (water stored in the canopy compared to the maximum canopy capacity), and stomatal conductance is modelled using soil moisture, atmospheric carbon dioxide, and the vapour pressure deficit (Cox et al., 1998). Water at the surface is routed as infiltration excess overland flow, at a rate controlled by the soil hydraulic conductivity, or as saturation-excess overland flow calculated by the Probability Distributed Model (PDM) (Moore, 2007; Clark and Gedney, 2008). A topography-derived parameterization of soil water storage in the PDM is used to calculate grid fraction saturation, which is used as a multiplier to convert excess water reaching the surface to saturation-excess overland flow (Martínez-de la Torre et al., 2019; Lewis and Dadson, 2021). Water flux through the four soil layers (3 m deep) is calculated by the Darcy–Richards equation, and the van Genuchten scheme calculates suction and soil conductivity (van Genuchten, 1980). Vegetation extracts excess water from the different soil layers as a function of root density and soil moisture critical and wilting points. As soil layers become progressively saturated, water is passed downwards until excess water at the base becomes subsurface runoff. The Dadson et al. (2011) river flow model (RFM) implementation of the kinematic wave equation solution routes both the surface and subsurface runoff according to a D8 flow direction grid. To clarify, river flow in this article is the water flow routed through the RFM at the river gauging station location within the model domain, whereas runoff is the specific combination of the subsurface and surface runoff for the entire catchment. The model is spun-up for 10 years in all experiments so that soil moisture content equilibrates (Martinez-de la Torre et al., 2018; Blyth et al., 2019).
Broadleaf woodland expansion is focused on for several reasons, which are further explained in Buechel et al. (2022). Firstly, the numerical implementation of broadleaf woodland is more accurate than needleleaf for hydrological processes (Broadmeadow et al., 2018). A large-scale implementation of needleleaf woodland would therefore potentially extrapolate unrealistic hydrological responses to afforestation. Secondly, more precise woodland species than just “broadleaf” are not utilized here as the full spectrum of woodland species are not implemented within JULES, and there is no clear guidance on the exact possible planting locations of where precise species would be. Furthermore, at the spatial and temporal scales at which JULES is run, the precise species' hydrological responses would not be appropriate. Unfortunately, to fully validate the experiments undertaken in this study would require comparing against a countrywide large-scale afforestation response to afforestation, which does not exist. This partly explains the need for this work to predict a potential hydrological response to the proposed scales of widespread afforestation. The relative small scale of paired-catchment studies (often <10 km2) compared to the temporal and spatial implementation of JULES means it is inappropriate to compare model output to those studies. Other processes important at smaller scales (such as forest management) would be more important in the smaller paired-catchment studies on accurately determining the hydrological response to afforestation. It should be noted however that the hydrology and biomechanics of the physical representations in our model configuration of JULES have been validated in many prior studies. Martínez-de la Torre et al. (2019) developed the rainfall–runoff mechanism utilized here and managed to achieve high accuracy in reproducing streamflow in rivers incorporated in this study. The broad structure of hydrological events and extremes have also been proven in earlier work (e.g. Prudhomme et al., 2011; Harding et al., 2014). Even more recent JULES versions have produced increasingly accurate results (e.g. Lewis and Dadson, 2021; Mathison et al., 2023). Initially, Alton et al. (2009) implemented plant hydrology within JULES that generated acceptable global evapotranspiration and runoff output. More recent studies also show that JULES can broadly obtain the correct patterns of evapotranspiration changes, although not necessarily accurately (Van den Hoof et al., 2013; Blyth et al., 2019). Harper et al. (2016, 2021) have also improved the plant functional types used within JULES so that they generate more plausible hydrological process representation in temperate regions. Although this does not provide an exhaustive list of all the studies that have validated and improved the implementation of hydrology and biomechanics in this configuration of JULES, it does illustrate JULES' ability to investigate land cover and hydrology changes.
Model output in this study (soil moisture, evaporation, and streamflow) is validated with 12 COSMOS-UK stations (H. M. Cooper et al., 2021) and the National River Flow Archive database for the investigated catchments (Vitolo et al., 2016) (Figs. S1 and S2 in the Supplement). The Kling–Gupta efficiency (KGE) measure (Gupta et al., 2009) quantifies model accuracy, where scores greater than −0.41 show greater model performance than the mean seasonal cycle (supplementary equation of Knoben et al., 2019). Several model outputs are validated to determine whether JULES provides the right result for the right reason across model parameterization domains (Lane et al., 2021; Mai et al., 2020). We find JULES performs satisfactorily: streamflow has a median KGE of 0.50 (minimum: 0.08; maximum 0.76), soil moisture 0.44 (minimum: 0.20; maximum: 0.82), and potential evaporation 0.53 (minimum: 0.22; maximum: 0.72). These are not perfect scores and so caution must be applied when considering results. KGE scores illustrate the challenges in producing a “model of everywhere” when there is so much uncertainty in model parameterizations and parameters (Beven, 2007; Blair et al., 2019). Furthermore, any changes in model results are relative to simulations and are not compared to realistic absolute values due to limitations of model process representation. Refer to Buechel et al. (2022) for further details on model validation and Supplement (Tables S1–S3). The streamflow output of JULES can also be compared to the work of Lane et al. (2019) and Lees et al. (2021) for further reference.
2.2.2 Streamflow analysis
Streamflow is the combined output of upstream hydrological processes and thus invaluable to explore afforestation effects. Fifty-one catchments are selected to assess streamflow across all regions (Figs. S1 and Table S4). Ten are from the work of Buechel et al. (2022), Crooks et al. (2014), and Martínez-de la Torre et al. (2019); 39 are from the UKBN2 (UK Benchmark Network 2) gauging station network (Harrigan et al., 2018); and 2 are from the largest catchments in the UKCP18 Dee region (as there were no rivers fitting our criteria, the dataset referred to is discussed later). UKBN2 catchments are near natural with minimal human interference; this is important for isolating changes due to LULC and climate with minimal anthropogenic interference (Villarini and Wasko, 2021), which may be unrepresented within JULES. Chosen catchments are larger than 150 km2 in size so that processes simulated are more faithful at JULES' spatial (1 km2) and temporal (30 min) resolution, apart from two rivers in Scotland which were needed so that all regions would have streamflow simulations. This is because land surface models are intended to explore processes at countrywide and continental scales. Chosen catchments cover all UKCP18 regions to enable analysis of how countrywide drought and flood conditions change with afforestation. Streamflow (or river flow) output is at a daily temporal resolution and calculated using the river flow model. Runoff is the combination of both surface and subsurface runoff.
Flow percentiles of 1 % (very high), 5 % (higher), 10 % (high), 50 % (median), 90 % (low), 95 % (lower), and 99 % (very low) are calculated to observe flow change across the whole flow spectrum with afforestation over the full hydrological year and not just individual events. The slope of the flow duration curve (FDC) is calculated to understand flow variability changes:
where Q33 % and Q66 % are the 33rd and 66th percentile of streamflow respectively. Chosen streamflow metrics enable quantification of extreme and average changes induced by afforestation to learn how flow regimes could change at present and in future.
2.3 Present hydrological response to afforestation
The period of 2000–2015 is chosen for the first study part. This is a flood-rich period, including several drought events, allowing determination of the role afforestation would have on these events across the country with our generated afforestation scenarios (Wilby and Quinn, 2013; Dadson et al., 2017). Hydrological processes are disaggregated by the 20 UKCP18 river basin boundaries (Lowe et al., 2018) (Fig. 2), which are hydrologically distinct, in order to compare afforestation influence across Great Britain. Water flux and store changes (evaporation, soil moisture, and runoff) are calculated seasonally (winter: DJF, spring: MAM, summer: JJA, autumn: SON) and for the whole period for each region. The Theil–Sen slope estimator (Theil, 1992; Sen, 1968) is employed to obtain the percentage change in the hydrological variable relative to the percentage point increase in woodland for each region. For example, a catchment where its woodland area increased from 10 % to 17 % of its overall area would represent a 7 percentage point increase. This allows the sensitivity and relative hydrological response to afforestation to be quantified regarding the spatial scales of afforestation and catchments considered. Theil–Sen, a form of nonparametric regression, is more robust than ordinary least squares regression as it is less sensitive to outliers (Helsel et al., 2020), and it is frequently used in other studies (Gudmundsson et al., 2019; Griffin et al., 2022a). The Theil–Sen slope estimators quantify both the direction and size of the response in water stores, fluxes, and metrics to afforestation. Spearman's rank correlation coefficient (ρ) is used to identify the strength of the association between afforestation and different hydrological fluxes across regions, where each data point represents an afforestation scenario of a region. The values of ρ reveals the extent to which afforestation, or factors other than afforestation (e.g. regional effects such as soil properties), influences the hydrology. For example, a weak Spearman's rank correlation (e.g. between 0.4 and −0.4) indicates that afforestation is not strongly associated with hydrological change, implying that regional effects are more important than the level of woodland planted.
2.4 Proportional influence of afforestation compared to climate
JULES' hydrological sensitivity to potential future atmospheric and afforestation changes is determined by undertaking a factorial sensitivity analysis. This allows the terrestrial hydrological response to afforestation, within the model domain, to be verified relative to atmospheric drivers. Three variables are independently altered in the base meteorological driving data (CHESS-met) for the period of 2000–2015: precipitation, temperature, and carbon dioxide. Afforestation is the fourth variable which is compared with the three meteorological variables (Fig. 3). The model is spun-up for 10 years (using 2000–2001 10 times) with the perturbed meteorological parameters. Maximal projected changes in precipitation and temperature, as stated in the UKCP18 scenarios, are the baseline for changing the meteorological data (Lowe et al., 2018). In this way, atmospheric variables are altered within a range deemed physically plausible by a validated climate model to observe maximal sensitivity within a credible realm. From the original CHESS-met data, precipitation (in mm d−1) is altered by 70 %, 100 %, and 130 %; temperature is raised by 1, 2, and 3 °C; and atmospheric carbon dioxide is enhanced from 375 to 450 and 600 ppm. For temperature and precipitation, these are the approximate maximal changes in the UKCP18 scenarios up to 2050 under the RCP8.5 scenario for the 95th percentile of the UKCP18 probabilistic projections (1981–2000 compared to 2041–2060). Current carbon dioxide levels are approximately 415 ppm, up from 375 ppm at the start of the 21st century (Global Monitoring Laboratory, 2022). Both Gedney et al. (2006) and Blyth et al. (2019) have explored the atmospheric carbon dioxide impacts on hydrology using JULES (or its predecessor) before; however, this approach goes further to see how it relates to potential future climate and LULC. An ensemble of 108 different scenarios per region is generated (Fig. 3), although this produces the full set of potential scenarios including unlikely ones. For example, an increase in carbon dioxide emissions with a decrease in both temperature and precipitation. Including the whole range not only accounts for the response to extremes in certain seasons but also provides enough data to decompose the contribution of each driver to hydrological change. This form of factorial sensitivity analysis was also undertaken due to the nonlinearity of hydrological processes.
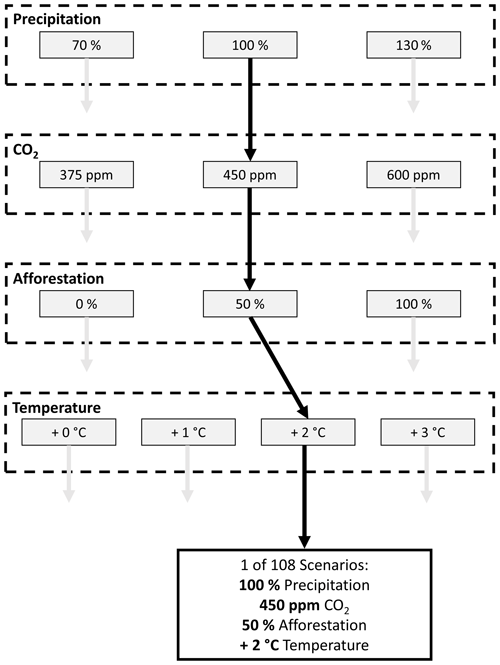
Figure 3Flowchart illustrating the potential scenarios generated with differences in precipitation, CO2, afforestation, and temperature. An indicated pathway of one of the 108 scenarios is shown with the thick black arrows. Not all the scenarios seen here are possible or likely. A scenario of 0 % afforestation, 100 % precipitation, 375 ppm, and +0 °C temperature would take us to a situation like the start of the 21st century. A scenario of 0 % afforestation, 130 % precipitation, 600 ppm, and +3 °C temperature would lead us to a situation of SSP5 (“business as usual” or extreme emissions scenario).
2.5 Hydrological response to potential future climate
The CHESS-SCAPE dataset is used to study future climate impacts (Robinson et al., 2022). CHESS-SCAPE is a 1 km resolution dataset downscaled from the 12 km UKCP18 climate projections (Met Office, 2018) used previously to investigate the influence of climate change on future UK hydrology (Kay, 2021; Griffin et al., 2022b). The 12 km simulations were generated using a perturbed parameter ensemble of the Met Office Hadley Centre Global Climate Model (HadGEM3-GA705) under the RCP8.5 scenario (Murphy et al., 2019), which is nested and forced by the wider 60 km global simulations. The CHESS-SCAPE dataset is created by selecting four model ensembles spanning the range of the original 12 model perturbed parameter ensemble of UKCP18 and then downscaled according to the CHESS-met methodology using local topography (Robinson et al., 2017a). The RCP8.5 uncorrected meteorological dataset for the period 2020–2050 forces JULES with the land cover scenarios across the 20 regions to enable identification of the tree planting effect on modulating climatic extremes produced by the worst-possible case of CO2 concentration in the atmosphere. RCP8.5 is increasingly recognized as an unlikely and extreme scenario (Hausfather and Peters, 2020); however, it is useful for detecting the climate signal from the influence of afforestation and the only scenario available in UKCP18. Vegetation is fixed with no dynamic competition between the different vegetation types in our model setup of JULES and so vegetation does not need to be recalibrated to this new climate regime. The hydrological output generated by the afforestation scenarios created in this study are then compared to the base land cover scenario. The model is spun-up for the period 2010 to 2020 so that the hydrological system is in equilibrium.
3.1 RQ1: changes in regional hydrology with afforestation
Broadleaf afforestation across Great Britain clearly changes modelled evaporative processes (Fig. 4) (Table 1). Canopy and soil evaporation increase on average for the entire year, which decreases canopy and soil water stores; however, the direction of change varies seasonally (Fig. 4). Averaged over the entire study period, overall canopy evaporation rises by 0.40 % (0.40 mm yr−1) per percentage point of afforestation (PPPoA), with a moderate influence of location (ρ=0.59), and is greater in winter and less in summer months for almost all regions (Fig. 4). To reiterate, weaker Spearman's rank correlation coefficients indicate that factors other than afforestation extent are causing the variation in the hydrological response to afforestation. Canopy storage decreases by 0.73 % (0.001 mm) PPPoA and is minimally affected by afforestation location (). Simulated soil evaporation, including both evaporation from the soil surface and modelled stomatal conductance, increases with afforestation by 0.26 % (0.54 mm yr−1) PPPoA and is partially dependent on geographic region (ρ=0.53). Soil evaporation is projected to increase substantially during winter and decrease in summer, particularly in Scottish regions. By contrast, modelled stomatal conductance decreases with afforestation consistently throughout the period (−0.59 % PPPoA) regardless of location (). Stomatal conductance decreases in summer and increases in winter and whilst it is sensitive to location, no systematic pattern could be discerned (, p<0.01). A notable variation is seen in the representation of stomatal conductance amongst LSMs and so this response could be particular to the configuration used here.
Table 1Changes in the average water fluxes and stores with afforestation across Great Britain for each percentage point increase in broadleaf woodland for both the present climate and potential future climate. Spearman's rank correlation coefficient (ρ) indicates the strength of association between increased afforestation and changes in the flux and stores, where each data point represents an afforestation scenario in a region. High absolute values (e.g. above 0.7) indicate planting location has a minimal influence on altering the response to afforestation. Values in bold are greater than an absolute value of 0.7. The table includes both the present and future changes to afforestation discussed in Sect. 3.1 and 3.3.
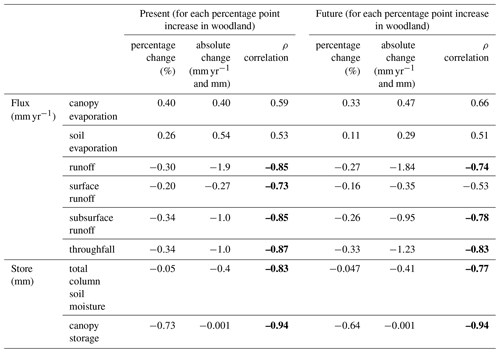
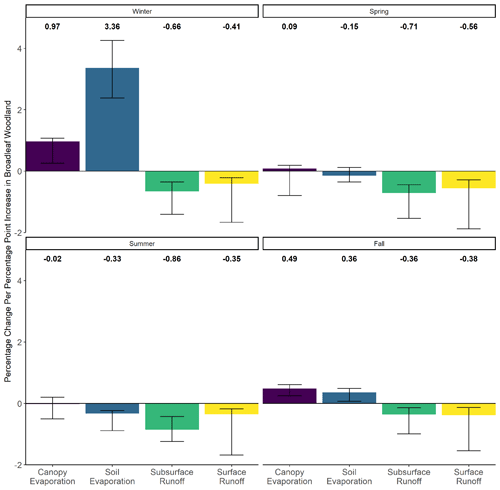
Figure 4Changes in the evaporative and runoff fluxes per percentage point of regional afforestation (PPPoA), e.g. 10 % to 11 % of a region afforested, by season with all other variables held constant. Error bars represent 1 standard deviation from the median value for the UKCP18 hydro-regions investigated.
Afforestation across Great Britain moderately reduces average river flow by 0.17 % PPPoA over the year with only a slight locational variation (). This decline in river flow is caused by decreasing surface and subsurface runoff (−0.20 %, −0.27 mm yr−1 and −0.34 %, −1.03 PPPoA respectively) (Table 1). Despite the consistent reduction in runoff components throughout the year, the response varies minimally by region (surface: , subsurface: ) (Table 1). Canopy throughfall, the simulated source of water for runoff, decreases with afforestation (−0.34 %, −1.00 mm yr−1 PPPoA) regardless of planting location () (Table 1), and its reduction is consistent throughout the year. Whilst average total soil moisture decreases with afforestation minimally (0.046 %, −0.40 mm PPPoA) and without influence of planting location () (Table 1), the moisture available to vegetation from the uppermost soil layer is noticeably influenced by planting location ().
In lower streamflow quantiles, the influence of afforestation location diminishes (Table 2). In the top 1 % of flows, there is no strong response to afforestation (−0.054 % PPPoA; , p=0.012), whereas the top 5 % of flows reduce by 0.11 % PPPoA (, p<0.01) with an even stronger median flow drop of −0.18 % PPPoA (, p<0.01) (Table 2). At the lowest flow exceedances, there are clearer patterns between streamflow reductions and afforestation with a decrease of −0.24 % PPPoA (, p<0.01) and −0.57 % PPPoA (, p<0.1) for the 90th and 99th flow percentiles accordingly (Table 2). We find an unclear picture of flow variability changes with afforestation extent, with a decrease 0.09 % PPPoA in the flow duration curve, which appears more strongly related to differences in regional factors than afforestation itself (, p=0.012).
Table 2Changes in flow metrics with afforestation across Great Britain for each percentage point increase in broadleaf woodland for both the present climate and potential future climate. Spearman's rank correlation coefficient (ρ) indicates the strength of association between increased afforestation and changes in the afforestation extent.
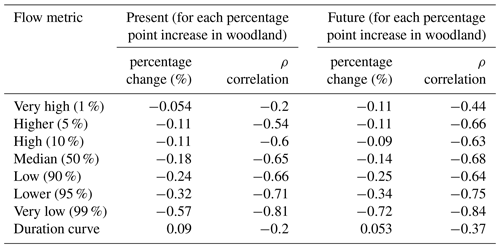
3.2 RQ2: hydrological sensitivity to climate and land cover changes
As the climate on Earth changes, land cover is also expected to change in future. Although it is expected that hydrological systems will respond significantly to climate change, it is unknown what the relative response to concurrent land cover changes will be. Hydrological processes in JULES show strong sensitivity to climate relative to LULC across the range of scenarios tested (Fig. 5). ANOVA (a statistical test – analysis of variance; Dadson, 2017) reveals significant differences in hydrological variables in all regions with proposed changes in precipitation and temperature (p<0.01). When compared to projected changes in precipitation, temperature, and CO2, the effects of LULC are almost undetectable. Only in a few isolated regions in winter are canopy storage, stomatal conductance, and soil moisture significantly altered by LULC (p<0.01) (Figs. S3 and S4). Interestingly, rising carbon dioxide only notably reduces transpiration and stomatal conductance in some regions (ANOVA, p<0.01) (Fig. S3). Although not substantial, higher CO2 suppresses soil evaporation, which increases soil moisture and therefore runoff.
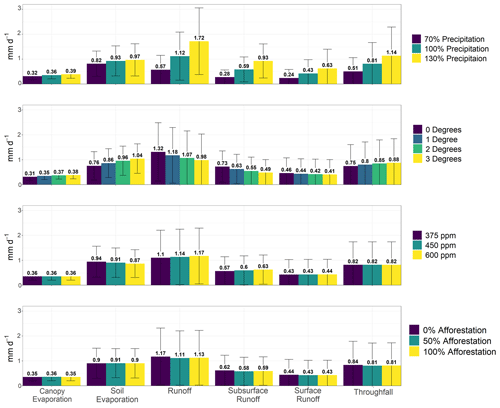
Figure 5Mean hydrological fluxes across all UKCP18 regions for each of the four variables altered relative to present climate and land cover: precipitation, temperature, CO2, and land cover. Error bars indicate 1 standard deviation. Figures S5 and S6 show how these change in summer and winter respectively.
Enhanced precipitation across Great Britain greatly increases hydrological fluxes and stores compared to all other factors altered (Fig. 5). When averaged across the whole period, precipitation does not have a significant impact on soil evaporation and transpiration, except in northwest Scotland. However, in summer, enhanced precipitation significantly increases soil evaporation across many regions (1.4 to 1.7 mm d−1 from 70 % to 130 % precipitation) (Fig. S5). Both canopy and soil moisture stores increase with precipitation, enhancing runoff (0.7 to 1.7 mm d−1 from 70 % to 130 % precipitation) (Fig. 5).
Rising temperatures appreciably alter many of JULES' hydrological processes. Soil and canopy evaporative processes rise in winter with higher temperatures (both by 0.2 to 0.4 mm d−1 with 3 °C extra). In summer, soil evaporation continues to increase (1.4 to 1.7 mm d−1 with 3 °C extra) but canopy evaporation stays approximately the same. This slightly enlarges the canopy store, although not significantly for many regions. In winter, throughfall increases (0.8 to 1.2 mm d−1 with 3 °C extra) likely due to more intense rainfall, which is parameterized by temperature. The higher throughfall further reduces canopy storage. Stomatal conductance decreases throughout the entire period with rising temperatures but is not significant for all regions, and transpiration clearly increases with temperature in winter. Soil moisture reduces in the summer with rising temperature, which minimizes subsurface runoff (0.4 to 0.2 mm d−1 with 3 °C extra). However, there are no statistically significant changes in river flow and surface runoff in winter and summer for almost all regions as temperatures rise.
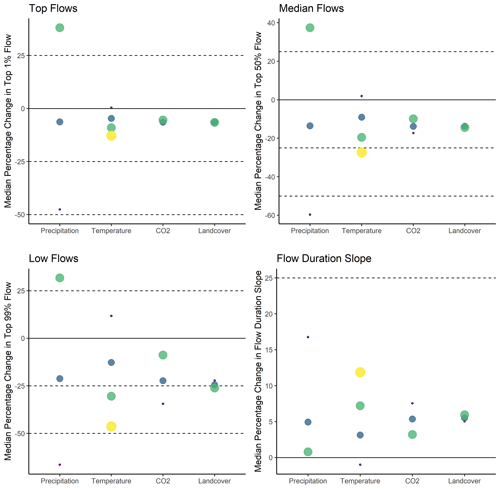
Figure 6Median percentage change in the four indicated metrics for catchments based on precipitation, temperature, CO2, and afforestation changes. Increasing dot size and lighter colour indicate larger changes in the variables explored (precipitation: 70 %, 100 %, 130 %; temperature: 0, +1, +2, +3 °C; CO2: 375, 425, 600 ppm; afforestation: 0 %, 50 %, 100 %). Colours are used to further differentiate variable quantity and are not relative to the size of the variable. The solid black horizontal line indicates a 0 % change for all variables, whereas the dashed lines indicate defined intervals of change. Top flows refers to the top 1 % of flows, median refers to the top 50 % of flows and low refers to top 99 % of flows.
Precipitation is a first-order control on flood and drought formation in JULES, as expected. Precipitation significantly (statistical Kruskal–Wallis (KW) test; Dadson, 2017, p<0.001) influences the top 1 % of flows (high flow): reducing precipitation by 30 % decreases them by 48 %, whilst increasing precipitation by 30 % enlarges them by 38 % (Fig. 6). Rising temperatures significantly (KW test, p<0.001) reduce high flows (+3 °C reduces high flows by 13 %) (Fig. 6). Enhanced CO2 insignificantly amplifies the top flows, whereas afforestation insignificantly reduces them (Fig. 6). Findings are similar at the lowest (top 99 %) flows, but the modelled flow response range is greater with climate and land cover perturbations. Increasing precipitation significantly increases low flows (KW test, p<0.001), by 32 % with a 30 % precipitation increase (−67 % with a 30 % precipitation decrease). Rising temperatures substantially reduce low flows, by 46 % with an additional 3 °C. Greater CO2 increases low streamflow across all 108 scenarios (KW test, p<0.001), from −34 % with 375 ppm of CO2 to −8.8 % with 600 ppm. Across all the proposed environmental disturbances, LULC has the smallest impact on streamflow. Afforestation only weakly decreases low flows, from −22 % (0 % afforestation) to −26 % (100 % afforestation), insignificant across all scenarios in comparison to all scenarios (KW test, p>0.1). Flow regimes became less variable with increasing precipitation (p<0.001, from 18 % to 0.60 %) and CO2 (p<0.001, reduction of 7.6 % to 3.2 %) (Fig. 6). In contrast, rising temperature increases flow variability (p<0.001) from −1.0 % to 12 % and afforestation increases flow variability by only a small amount, which is not statistically significant (p>0.1).
3.3 RQ3: potential influence of afforestation in the future
In the future, afforestation will have a similar influence on hydrology as in the present climate (Table 1). Therefore, projected climate changes are insufficient to substantially alter simulated vegetation's interaction with water fluxes. Canopy evaporation and storage are similarly influenced by land cover change location in the future (ρ=0.66; respectively) and increase by 0.33 % (0.47 mm yr−1) and decrease by 0.64 % (0.001 mm yr−1) PPPoA respectively (Table 1). However, soil evaporation increases at half the present rate at 0.11 % (0.29 mm yr−1) PPPoA and is strongly influenced by afforestation location (ρ=0.51). Transpiration robustly decreases at a rate of −0.78 % PPPoA regardless of planting location (). Regional influence on stomatal conductance is increased compared to present (ρ=0.40) and rises more rapidly at 0.14 % PPPoA.
Average simulated river flow, compared to present, drops at a slightly lower rate of −0.12 % PPPoA (). Runoff decreases with afforestation at a comparable rate to present (−0.27 %, 1.84 mm yr−1 PPPoA); however, location has a greater effect (), and surface runoff (−0.16 %, −0.35 mm yr−1) is greatly influenced by location () (Table 1). Subsurface runoff also becomes slightly more influenced by location (), with a decrease of 0.26 % (−0.95 mm yr−1) PPPoA. Both throughfall and soil moisture respond to afforestation in a similar manner to as at present regarding their trends and connection to planting location (−0.33 %, −1.23 mm yr−1 PPPoA, ; −0.047 %, −0.41 mm PPPoA, respectively) (Table 1).
In the future, planting location will have a reduced influence on streamflow when compared to increases with afforestation compared to present (Table 2). Median streamflow reduces by −0.14 % PPPoA (), low flows decrease by −0.25 % PPPoA () at the 90th percentile of flow and −0.72 % PPPoA () at the 99th percentile of flow (Table 2). The effect of afforestation is more complicated at the highest flows, with the top 1 % and 5 % of flows reducing by 0.11 % PPPoA ( and ). Flow variability does not substantially change in the future, with insignificant change (0.053 % PPPoA) and a strong regional influence ().
4.1 Afforestation influence across Great Britain
The regional hydrological response to afforestation does not significantly vary across Great Britain. Proposed countrywide afforestation is projected with JULES to have a detectable, but not substantial, impact on hydrology. However, slight nuances occur depending on afforestation location and the time of year (discussed later). LULC has a diminishing impact on streamflow further from the intervention area; however, the scale of afforestation considered here (large compared to realistic afforestation rates) means modelled large-scale hydrological processes are detectable at multiple spatial scales (Dadson et al., 2017; Blöschl et al., 2007; Pattison and Lane, 2012). The realism of the afforestation scenarios herein illustrates how large-scale hydrological changes, demonstrated by improbable widespread afforestation scenarios considered in the literature, have minor relevance to the debate of probable afforestation rate impacts (Meier et al., 2021; Denissen et al., 2022). Despite a broader modelling domain and greater hydrological diversity than in Buechel et al. (2022), we find little difference in catchment water output sensitivity to afforestation location, suggesting potential reductions in water yield can be directly estimated from the areal extent of woodland planted rather than its location. However, this finding may simply demonstrate the relative insensitivity of terrestrial processes to land cover changes modifying terrestrial processes within an LSM. No modelled hydrological change is exactly equivalent to afforestation; for example, a 1 % woodland increase does not equal a 1 % change in canopy evaporation. This is also seen in observational studies where a 1 % increase in upstream afforestation area does not detectably change streamflow (Anderson et al., 2022).
The lack of regional variability in catchment hydrological response to afforestation could be due to terrestrial hydrological similarity across the UK (Wagener et al., 2021). Alternatively, the large epistemic uncertainty within JULES means that highly sensitive hydrological parameters are not included, which would lead to diverging regional afforestation responses, such as variable vertical soil column properties (Beven and Cloke, 2012; Beven, 2018). LSMs scale physical processes from very small areas, and so the lack of sensitivity to widespread afforestation, and detected nuances related to location, could also be due to inaccurate representations of model processes, such as the use of pedotransfer functions (Beven, 1989; Clark et al., 2009). Another consideration is the uncertainty in soil products where there is large disagreement in the amount of organic material which significantly influences soil hydraulics (Feeney et al., 2022). As an offline model (i.e. the land surface is not coupled to the atmosphere) factors that enhance regional importance with land parameterizations, such as orographic rainfall, are not represented. Importantly for this work, overall soil moisture dryness may be overestimated as the rain seeding effect of afforestation is not included (Teuling et al., 2017; Xu et al., 2022). Modelling widespread afforestation with JULES has revealed further questions about the adequacy of LSM parameterizations.
The overall reduction in simulated runoff (and streamflow) by afforestation is a consequence of soil and canopy evaporation increases (Fig. 4). Although evaporative processes are more influenced by regional properties (Table 1), JULES' land surface parameterizations screen atmospheric differences, leading to diminished locational impacts on runoff compared to LULC. However, JULES systematically overestimates evaporation and so these results must be treated cautiously (Blyth et al., 2019; Van den Hoof et al., 2013). Rising evaporation rates are likely due to albedo reduction with afforestation, enhancing surface temperatures, and larger canopy stores with the higher LAI, and turbulence, compared to grasslands. Increases in canopy storage, evaporation, and interception reduce throughfall reaching the soil surface, which minimizes regional climate differences relative to afforestation (Table 1). Reduced throughfall means less soil moisture, runoff, and streamflow. The declining soil moisture with afforestation diminishes subsurface runoff and results in more water-stressed vegetation. The lower stomatal conductance, and thus transpiration, particularly in summer months, is evidence of the diminished soil moisture store. In JULES, broadleaf woodland has deeper roots than grasslands and shrublands, which leads to water being extracted lower in the soil column to maintain growth (Harper et al., 2021; Best et al., 2011). In reality, tree root depths would be much deeper than currently represented in JULES and vary according to the soil type (Vereecken et al., 2022); implementing woodland in this manner could lead to more accurate evaporation rates (Harper et al., 2021; Roebroek et al., 2020). In summer, moisture in the uppermost soil layer slightly increases, which could be a function of lower roots, compared to grasslands, and reduced stomatal conductance (Buechel et al., 2022). The slight association of afforestation with topsoil moisture increases and location could be the result of different soil types (e.g. organic) which facilitate differences in hydraulic conductivity related to afforestation. The runoff model in this setup of JULES enhances runoff during high precipitation events with increased topsoil saturation; however, the proportionally small rise in topsoil moisture with simulated afforestation would make it potentially unobservable within natural uncertainty. Several limitations and assumptions should be considered when using LSMs such as JULES. The model domain only includes known hydrological processes. Excluded, or undiscovered, processes may have important consequences on afforestation's hydrological impact, unknown to the modeller (Beven et al., 2011). Furthermore, processes within the model may inaccurately be implemented numerically or physically (Hrachowitz and Clark, 2017). Results therefore could potentially be affected by inadequate process representation and implementation.
Some regions in Great Britain exhibit slightly stronger effects of afforestation. In southeast England and Anglia, there are significantly larger hydrological variations, likely due to underlying soil properties and climate regime, similar to Buechel et al. (2022), with more sensitive catchments in drier regions. Afforestation therefore could strain water resources in regions of low water yield (Ellison et al., 2012). However, the model representation of hydrological processes in groundwater-based catchments (found in these regions) is known to be inadequate (Le Vine et al., 2016). Therefore in reality, afforestation may have a more subtle and greater influence on streamflow in these regions, with roots accessing the deeper groundwater (Roberts and Rosier, 2005). Evaporation rates are partially impacted by afforestation location, particularly in Scottish regions and parts of the west coast, which could enable flood magnitude reduction with spatially targeted broadleaf afforestation. The higher levels of wind turbulence and speed likely enable high evaporation rates to be maintained with additional woodland. Page et al. (2020) suggested canopy evaporation could reduce flood peaks in upland regions; alignment between model and observations suggests further analysis is needed to quantify evaporative processes over countrywide scales to mitigate flood risk. Regional differences in modelled stomatal conductance are due to climatic conditions, such as temperature and humidity as well as soil moisture and resulting vegetation water stress (Betts et al., 2007; Best et al., 2011). The similar connection of surface runoff with location suggests soil hydrology is an important control on stomatal conductance and runoff (Table 1). JULES' modelling paradigm for simulating stomatal conductance is not applied in all other models, and therefore other studies may find different projected stomatal conductance and thus resulting evaporation. However, JULES poses interesting questions for further exploration of the impact of widespread afforestation on regional hydrology and whether simulated changes are observable.
Afforestation across Great Britain influences the entire simulated streamflow spectrum (high to low flows) (Table 2). It is often observed significantly reducing the low-to-median flows, while high-flow changes are frequently undetectable or inconsistent (Anderson et al., 2022; Farley et al., 2005; Do et al., 2017). Afforestation's impact on streamflow is complex within JULES. Afforestation decreases the lowest flows and suggests locational factors have a minimal impact, similar to other research (Buechel et al., 2022; Bathurst et al., 2020; Birkinshaw et al., 2014). If our projections are correct, water managers need to prepare for worse hydrological droughts with proposed afforestation in conjunction with those already predicted for the future (Lowe et al., 2018; Kay et al., 2021). The mechanisms generating low-flow response to afforestation are therefore more likely to be driven by runoff and soil moisture parameterizations compared to evaporative processes. This is because both runoff and soil moisture are more influenced by afforestation extent than location (Table 1), which is then replicated in the similar Spearman's rank correlation coefficients for the lower streamflow percentiles (Table 2). To re-emphasize, larger Spearman's rank correlation coefficients indicate afforestation extent, rather than locational influences, impact the hydrological response. This highlights that the hydrological model structure within an LSM is likely to govern the model's ability to produce accurate drought predictions compared to other system parameterizations (Van Kempen et al., 2021). In comparison, at the simulated very highest flows (top 1 % of flows), afforestation both decreases and increases streamflow depending on catchment and antecedent conditions. This result is significant as simpler and conceptually based hydrological modelling often suggest afforestation reduces the highest flows (Stratford et al., 2017). The regional differences in the hydrological response of the highest flows to afforestation suggests evaporation rates are controlling the response, which is seen with the lower Spearman's rank correlation coefficients with afforestation extent and high flows, and evaporative processes. However, floods are often generated by extreme precipitation which generate “numerical daemons”, where the numerical implementation of hydrological processes generate implausibly high responses (Clark et al., 2021; La Follette et al., 2021). Therefore, floods could be more sensitive to terrestrial model parameters, which could also lead to strong regional influences on afforestation impact. Increases in small and large floods suggest similar generation mechanisms. Top flows usually decrease in catchments that are predominantly grass and pasture, where the chosen woodland planting criteria allow for larger areas to be afforested. Greater afforestation reduces simulated soil moisture, reduces throughfall, and increases interception and canopy evaporation. When over 5 % of a catchment's area is afforested, total soil and canopy evaporation rise, enhancing the catchment capacity to store and remove precipitation. During high-magnitude precipitation events, initial woodland planting reduces the overall effectiveness of the catchment to reduce flood peaks. Preliminary afforestation reduces the overall simulated maximal catchment water storage capacity in winter, due to decreased LAI, thus reducing water usage and evaporative fluxes.
These results have three major outcomes for future work. Firstly, models that encapsulate more known earth surface dynamic processes (e.g. dynamic vegetation coupled with soil hydraulics and river runoff routines) produce a more nuanced understanding of how afforestation could impact hydrology (M. Cooper et al., 2021). Although not perfect, particularly at hydrological extremes (Cuntz et al., 2016; Brunner et al., 2021), LSMs allow us to propose new hypotheses related to the influence of afforestation (and LULC) on hydrological processes as a form of multiple working hypotheses (M. P. Clark et al., 2011). Secondly, analysis of median hydrological fluxes across hydro regions reveals reductions in overall runoff and streamflow over an entire year, which would lead to the incorrect assertion that afforestation could effectively act to mitigate peak flows over extensive areas as higher flows are less influenced. Targeted afforestation locations within large catchments (>150 km2) may be ineffective for NFM downstream unless extensive or coupled with other flood mitigation measures. Finally, model parameterizations have a significant bearing on our derived conclusions, with hydrological model structure being more significant than other model parameters in determining afforestation impacts on streamflow. Evaporative processes strongly influence simulated floods, whereas runoff model implementations are more important for calculating droughts with LULC change. Future work should therefore continue to investigate the role of hydrological model structure within LSMs to assess its impact on quantifying the hydrological response to LULC change (Clark et al., 2021).
4.2 Sensitivity to climate and afforestation changes
Compared to the most extreme proposed atmospheric changes, the impact of afforestation on Great Britain's hydrology is relatively limited, with precipitation being the greatest driver of hydrological change compared to other variables studied (Fig. 5). All water fluxes and stores rise in JULES with enhanced precipitation; in a projection of greatly increased rainfall, flooding will likely increase, regardless of plausible land cover and other climate changes. For example, with heavier rainfall, hotter temperatures, and more CO2 in winter (such as under the high-emissions Shared Socioeconomic Pathway 5 (SSP5) – “Taking the Highway”; Riahi et al., 2017), countrywide afforestation would not reduce flood magnitude. Conversely, in summer, an overall decrease in precipitation (with increased temperatures and CO2) could greatly reduce runoff, which is only slightly further diminished by afforestation. In the UK, more intense rainfall (convective) is predicted in the summer (Fosser et al., 2020; Kay et al., 2021; Kendon et al., 2023) and our results indicate realistic afforestation is likely to be ineffective for flood management during these events. The use in the present study of JULES without atmospheric coupling, and no vegetation competition in this model configuration, means there is no large-scale moisture recycling or vegetation mortality that would further modulate the model precipitation response (e.g. Cui et al., 2022). For example, reduced rainfall could result in large-scale vegetation dieback, amplifying the effect of high precipitation with reduced interception and less infiltration. Precipitation decreases demonstrate the nonlinear parameterizations of JULES' hydrology with larger reductions at smaller streamflow percentiles (Fig. 6) (further justifying the factorial sensitivity analysis). Increased precipitation saturates the canopy and topsoil which quickly routes excess water to rivers. There are two consequences of JULES' hydrological implementation. Firstly, uncertainty in precipitation products measurably alters conclusions derived using JULES. Any small differences in precipitation used to drive JULES will lead to larger differences in the modelled hydrological outputs. As a result, secondly, slight precipitation product differences could negate the impact of LULC using JULES (or other similar LSMs) when comparing studies that utilize different precipitation datasets. This is important as it suggests that works using LSMs to determine countrywide changes in hydrology over periods where there have been relatively small land cover changes can justify not using evolving land cover as it would likely minimally reduce uncertainty (e.g. Blyth et al., 2019). Further attention is therefore required to minimize uncertainty in meteorological datasets to predict floods and droughts as terrestrial processes within LSMs will have a comparatively minor influence.
Previous work has illustrated the minor influence land cover has on hydrological processes compared to other atmospheric processes, both in models and in observational studies (Gedney et al., 2014; Oudin et al., 2008), and our results confirm that afforestation has the smallest impact on modelled streamflow compared to climate changes (Fig. 5). Therefore, to detect afforestation influence upon streamflow, one must be aware of climate changes over the same period and be able to accurately remove any climatic effect which could obscure the LULC signal (Milly et al., 2008; Slater et al., 2021b). This may explain why observational studies have found an insignificant impact of afforestation on streamflow (e.g. Anderson et al., 2022), particularly with the large number of dependent interacting processes associated with woodland hydrology. Hydrological conclusions using JULES over long time periods therefore determine that LULC is of minor relevance when compared to climate (e.g. Blyth et al., 2019). With climate change, afforestation is likely to be insufficient to reduce the largest pluvial flood risks. However, some research suggests smaller-magnitude floods are becoming more frequent, and so plausible afforestation may mitigate the risk they pose (Wasko et al., 2021; Griffin et al., 2019). Our results emphasize LSMs pushing to be “models of everywhere” are relatively insensitive to terrestrial process parameterizations in relation to climate drivers (Blair et al., 2019). By applying atmospheric changes across the whole country, variations in land cover, topography, and soil type are insufficient to substantially alter the hydrological response. It is possible that in JULES, overparameterization could lead to high complexity of interacting processes, muting terrestrial parameter impact, or large epistemic uncertainty might be responsible for the minimal response compared to climate (Hrachowitz and Clark, 2017; Beven and Lane, 2022). Nonetheless, the JULES community model is continually being improved, and further work should test whether terrestrial properties, including LULC, are adequately represented.
Simulations of future climate suggest raised atmospheric CO2 could negate the influence of increasing afforestation on streamflow (Fig. 6). With increases in CO2, simulated streamflow rises across the flow spectrum because of reduced vegetation water usage. Amplified CO2 decreases vegetation growth as the CO2 pressure gradient between the stomata and atmosphere diminishes (Blyth et al., 2019; Prudhomme et al., 2014; Gedney et al., 2006), which reduces soil water usage, increasing soil moisture and overall runoff. JULES has exhibited strong sensitivity, be it correct or not, to CO2 previously (Prudhomme et al., 2014). If these results are accurate, afforestation in a changing climate may not be the silver bullet for mitigating flood risk and reducing atmospheric carbon policy makers envisage, particularly as vegetation becomes less effective at absorbing the additional CO2 with increased atmospheric CO2 (IPPC, 2019; Leung et al., 2019; Cook-Patton et al., 2020). A simpler hydrological model, and not an LSM, is unlikely to show the effect of CO2 seen here. Atmospheric CO2 has a strong control on low flows, which is important to consider in the context of future droughts and illustrates its strong influence on JULES' runoff mechanisms (Fig. 6). CO2 fertilization is not currently included within the version of JULES (vn5.6) used here, which would influence the effectiveness of vegetation in interacting with water fluxes and potentially minimize the CO2 impact (Bonan, 2008; Ritchie et al., 2019; Zhang et al., 2022). Therefore, afforestation may have an equivalent or larger impact on streamflow than CO2, and we encourage further research to test these results.
Temperature is a second-order control on hydrological sensitivity within JULES and is more important than afforestation and CO2 changes (Fig. 5). Streamflow is significantly reduced across the whole flow regime by increasing temperatures due to increased evaporation and water usage by vegetation. Again, looking under the high-emissions SSP5 scenario, afforestation may enhance drought formation (both magnitude and duration) due to warmer temperatures. Increased flow variability with higher temperatures also likely makes it more difficult to adequately manage water resources. In JULES, precipitation is converted to convective rainfall at a certain temperature (the same amount of rainfall occurs in a smaller fraction of the grid box) (Best et al., 2011). Temperature rises therefore trigger more “convective” rainfall events, but interestingly, even with increased precipitation, top flows do not grow, even with larger throughfall. This suggests that in JULES, greater temperatures reduce antecedent soil moisture, decreasing runoff and minimizing the impact of the more intense rainfall. However, even the largest temperature increases cannot mitigate the impact of greater precipitation on increasing flood magnitude and frequency. Furthermore, this uncoupled model does not include realistic changes in rainfall intensity and magnitude, which might be expected with increases in temperature, and could change land surface responses to floods and droughts (Lee et al., 2022; Wasko et al., 2019).
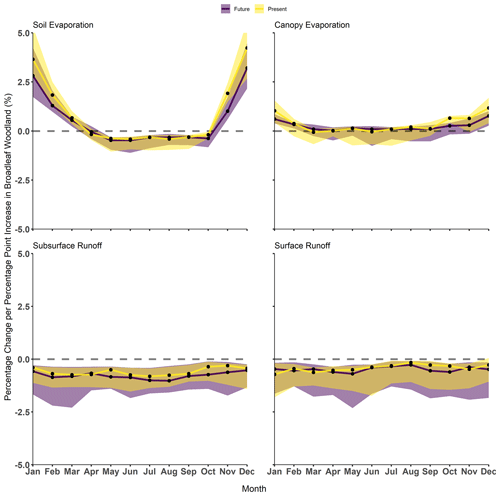
Figure 7Hydrological flux changes with PPPoA between the present of 2000–2015 (yellow) and future of 2020–2050 (purple). Use of an unpaired two-samples Wilcoxon test reveals no significant difference in the above hydrological fluxes between the future and present (p>0.1). Shaded regions represent the 10th and 90th percentile of hydrological flux changes across all regions.
4.3 Afforestation impact with climate change
In an extreme potential future climate scenario (RCP8.5/SSP5), we find afforestation is unlikely to alter hydrological processes differently than under our present climate (Fig. 7). There are no statistically significant differences (KW test, p>0.5) between the hydrological impacts for the same amount of afforestation in the present climate and the future (Fig. 7). Although future precipitation, temperature, and CO2 could have altered the woodland hydrological response, as seen in the previous part of the study, the average climate changes are insufficient to induce significant changes. Afforestation is therefore unlikely to provide any greater protection from projected increases in hydrological extremes (Lane and Kay, 2021; Griffin et al., 2022b). This finding suggests that current estimates of the impact of afforestation on hydrology can also be used for the future; catchments where afforestation has reduced the largest floods are likely to continue to experience some protection from future events of similar magnitude. This is important as other work using extreme land cover and climate scenarios suggest significantly different hydrological systems in the future. Projected climate changes are unlikely to be large enough to generate differing responses from land surface parameterizations in JULES compared to the simple sensitivity analysis undertaken. Future studies should justify and utilize plausible land cover scenarios for policy recommendations to more credibly determine the future effect of changing climate and land cover on extremes when using numerical methods.
Some future impacts of afforestation on hydrological fluxes in JULES depart from present estimates. Transpiration will decrease in the future, driven by lower summer precipitation, which mitigates the impact of growing broadleaf woodland relative to grasslands. Future rising temperatures, in conjunction with reduced albedo from more woodland, may therefore reduce stomatal conductance, due to the increased vapour pressure deficit. Decreases in both average river flow and runoff appear to be more influenced by regional effects with a reduced correlation between amount of afforestation and percentage reduction in runoff across the studied regions. This is likely due to greater differences in precipitation and temperatures (previously shown as the main differentiator of streamflow response) leading to changes in evaporation and runoff. It might also suggest that evaporative processes have a stronger effect on runoff generation, the former having been shown to be driven by regional controls (Table 1). However, a stronger correlation between the top 1 % of flows and afforestation extent in the future (Table 2) suggests climate is likely to alter the modulating role of land cover during extreme events. Current implementations of land cover into LSMs need to ensure land cover parameterizations are accurate to ensure modelled responses to climate are faithful. If they are not, we are projecting further uncertainty into future scenarios.
Modelling “realistic” countrywide afforestation in line with UK Government ambitions shows only small changes in hydrological processes and streamflow. Afforestation could generate unintended reductions in low flows in some locations, both at present and in the future. Although there are not significantly divergent regional responses to afforestation, catchment attributes and climate do produce nuanced hydrological responses (such as soil moisture). Evaporative processes govern high-flow generation, while runoff parameterization controls lower streamflow generation. Our sensitivity analysis shows that large-scale plausible afforestation only has a minimal impact on hydrology compared to possible climate changes. Precipitation changes have the largest impact on the modelled streamflow regime, whereas temperature and CO2 only have a discernible impact on the lowest flows. Furthermore, this study illustrates the epistemic uncertainties within the JULES model and potentially under-sensitive land surface parameters and parameterizations. Finally, the effects of afforestation on land surface hydrology and the terrestrial hydrosphere are similar in the present and future. Climate changes (e.g. precipitation and temperature) do not alter woodland regulation of hydrological extremes and only slightly alter regional differences in the hydrological response to afforestation. Future research could use fully coupled land surface–atmosphere LSMs to assess how afforestation influences hydrology over larger spatial scales than the catchments studied to elucidate the strength and spatial extent of water cycling from increased canopy evaporation.
JULES' configuration details are found in Buechel et al. (2022) and accessible as Rose suite u-ce663 from the Met Office Rose/Cylc suite control system (https://metomi.github.io/rose/doc/html/index.html, ROSE, 2024). The source code for JULES can be found at: https://github.com/jules-lsm/jules-lsm.github.io, jules-lsm, 2024).
NetCDF afforestation scenarios used to run experiments can be found at: https://doi.org/10.5281/zenodo.7957084 (Buechel, 2023). The CHESS datasets used to run model experiments can be found at: https://catalogue.ceh.ac.uk/documents/7de9790e-66a2-44b5-988e-283d764ef52f (UKCEH, 2024). CHESS-SCAPE can be found at: https://doi.org/10.5285/8194b416cbee482b89e0dfbe17c5786c (Robinson et al., 2022). Additional data can be provided from the primary author on request.
The supplement related to this article is available online at: https://doi.org/10.5194/hess-28-2081-2024-supplement.
MB ran the model simulations, analysed the results, and wrote the paper. LS and SD provided guidance and avenues of investigation and edited the paper.
At least one of the (co-)authors is a member of the editorial board of Hydrology and Earth System Sciences. The peer-review process was guided by an independent editor, and the authors also have no other competing interests to declare.
Publisher's note: Copernicus Publications remains neutral with regard to jurisdictional claims made in the text, published maps, institutional affiliations, or any other geographical representation in this paper. While Copernicus Publications makes every effort to include appropriate place names, the final responsibility lies with the authors.
We thank Toby Marthews and Emma Robinson for assistance with the original configuration of the JULES model. The JASMIN CEDA service provided access to facilities and resources.
Marcus Buechel is funded by NERC (NE/L002612/1). Louise Slater is supported by UKRI (MR/V022008/1) and NERC (NE/S015728/1). Simon Dadson is funded by NERC (NE/S017380/1).
This paper was edited by Yi He and reviewed by Steve Birkinshaw and one anonymous referee.
Alton, P., Fisher, R., Los, S., and Williams, M.: Simulations of global evapotranspiration using semiempirical and mechanistic schemes of plant hydrology, Global Biogeochem. Cy., 23, 1–12, https://doi.org/10.1029/2009GB003540, 2009.
Anderson, B. J., Slater, L. J., Dadson, S. J., Blum, A. G., and Prosdocimi, I.: Statistical Attribution of the Influence of Urban and Tree Cover Change on Streamflow: A Comparison of Large Sample Statistical Approaches, Water Resour. Res., 58, 1–20, https://doi.org/10.1029/2021wr030742, 2022.
Andréassian, V.: Waters and forests: From historical controversy to scientific debate, J. Hydrol., 291, 1–27, https://doi.org/10.1016/j.jhydrol.2003.12.015, 2004.
Bastin, J.-F., Finegold, Y., Garcia, C., Mollicone, D., Rezende, M., Routh, D., Zohner, C. M., and Crowther, T. W.: The global tree restoration potential, Science, 365, 76–79, https://doi.org/10.1126/science.aax0848, 2019.
Bathurst, J., Birkinshaw, S., Johnson, H., Kenny, A., Napier, A., Raven, S., Robinson, J., and Stroud, R.: Runoff, flood peaks and proportional response in a combined nested and paired forest plantation/peat grassland catchment, J. Hydrol., 564, 916–927, https://doi.org/10.1016/j.jhydrol.2018.07.039, 2018.
Bathurst, J. C., Fahey, B., Iroumé, A., and Jones, J.: Forests and floods: Using field evidence to reconcile analysis methods, Hydrol. Process., 34, 3295–3310, https://doi.org/10.1002/hyp.13802, 2020.
Beschta, R. L., Pyles, M. R., Skaugset, A. E., and Surfleet, C. G.: Peakflow responses to forest practices in the western cascades of Oregon, USA, J. Hydrol., 233, 102–120, https://doi.org/10.1016/S0022-1694(00)00231-6, 2000.
Best, M., Essery, R., and Cox, P.: JULES Technical Documentation MOSES 2.2 Technical Documentation, 36 pp., https://jules.jchmr.org/sites/default/files/2023-06/JULES-Technical-documentation-2.pdf (last access: 21 April 2024), 2009.
Best, M. J., Pryor, M., Clark, D. B., Rooney, G. G., Essery, R. L. H., Ménard, C. B., Edwards, J. M., Hendry, M. A., Porson, A., Gedney, N., Mercado, L. M., Sitch, S., Blyth, E., Boucher, O., Cox, P. M., Grimmond, C. S. B., and Harding, R. J.: The Joint UK Land Environment Simulator (JULES), model description – Part 1: Energy and water fluxes, Geosci. Model Dev., 4, 677–699, https://doi.org/10.5194/gmd-4-677-2011, 2011.
Betts, R. A., Boucher, O., Collins, M., Cox, P. M., Falloon, P. D., Gedney, N., Hemming, D. L., Huntingford, C., Jones, C. D., Sexton, D. M. H., and Webb, M. J.: Projected increase in continental runoff due to plant responses to increasing carbon dioxide, Nature, 448, 1037–1041, https://doi.org/10.1038/nature06045, 2007.
Beven, K.: Changing ideas in hydrology – The case of physically-based models, J. Hydrol., 105, 157–172, https://doi.org/10.1016/0022-1694(89)90101-7, 1989.
Beven, K.: Towards integrated environmental models of everywhere: Uncertainty, data and modelling as a learning process, Hydrol. Earth Syst. Sci., 11, 460–467, https://doi.org/10.5194/hess-11-460-2007, 2007.
Beven, K. and Lane, S.: On (in)validating environmental models. 1. Principles for formulating a Turing-like Test for determining when a model is fit-for purpose, Hydrol. Process., 36, 1–32, https://doi.org/10.1002/hyp.14704, 2022.
Beven, K., Smith, P. J., and Wood, A.: On the colour and spin of epistemic error (and what we might do about it), Hydrol. Earth Syst. Sci., 15, 3123–3133, https://doi.org/10.5194/hess-15-3123-2011, 2011.
Beven, K. J.: On hypothesis testing in hydrology: Why falsification of models is still a really good idea, Wiley Interdisciplin. Rev. Water, 5, e1278, https://doi.org/10.1002/wat2.1278, 2018.
Beven, K. J. and Cloke, H. L.: Comment on “Hyperresolution global land surface modeling: Meeting a grand challenge for monitoring Earth's terrestrial water” by Eric F. Wood et al., Water Resour. Res., 48, 2–4, https://doi.org/10.1029/2011wr010982, 2012.
Birkinshaw, S. J., Bathurst, J. C., and Robinson, M.: 45 years of non-stationary hydrology over a forest plantation growth cycle, Coalburn catchment, Northern England, J. Hydrol., 519, 559–573, https://doi.org/10.1016/j.jhydrol.2014.07.050, 2014.
Blair, G. S., Beven, K., Lamb, R., Bassett, R., Cauwenberghs, K., Hankin, B., Dean, G., Hunter, N., Edwards, L., Nundloll, V., Samreen, F., Simm, W., and Towe, R.: Models of everywhere revisited: A technological perspective, Environ. Model. Softw., 122, 104521, https://doi.org/10.1016/j.envsoft.2019.104521, 2019.
Blöschl, G., Ardoin-Bardin, S., Bonell, M., Dorninger, M., Goodrich, D., Gutknecht, D., Matamoros, D., Merz, B., Shand, P., and Szolgay, J.: At what scales do climate variability and land cover change impact on flooding and low flows?, Hydrol. Process., 21, 1241–1247, https://doi.org/10.1002/hyp.6669, 2007.
Blyth, E. M., Martínez-de la Torre, A., and Robinson, E. L.: Trends in evapotranspiration and its drivers in Great Britain: 1961 to 2015, Prog. Phys. Geogr., 43, 666–693, https://doi.org/10.1177/0309133319841891, 2019.
Blyth, E. M., Arora, V. K., Clark, D. B., Dadson, S. J., De Kauwe, M. G., Lawrence, D. M., Melton, J. R., Pongratz, J., Turton, R. H., Yoshimura, K., and Yuan, H.: Advances in Land Surface Modelling, Curr. Clim. Change Rep., 7, 45–71, https://doi.org/10.1007/s40641-021-00171-5, 2021.
Bonan, G. B.: Forests and Climate Change: Forcings, Feedbacks, and the Climate Benefits of Forests, Science, 320, 1444–1449, https://doi.org/10.1126/science.1155121, 2008.
Bosch, J. M. and Hewlett, J. D.: A review of catchment experiments to determine the effect of vegetation changes on water yield and evapotranspiration, J. Hydrol., 55, 3–23, https://doi.org/10.1016/0022-1694(82)90117-2, 1982.
Bradfer-Lawrence, T., Finch, T., Bradbury, R. B., Buchanan, G. M., and Field, R. H.: Mapping potential areas for woodland creation in the UK, 1–13, https://datastorre.stir.ac.uk/bitstream/11667/179/2/Mapping potential areas for woodland creation.pdf (last access: 30 April 2024), 2014.
Bradshaw, C. J. A., Sodhi, N. S., Peh, K. S.-H., and Brook, B. W.: Global evidence that deforestation amplifies flood risk and severity in the developing world, Glob. Change Biol., 13, 2379–2395, https://doi.org/10.1111/j.1365-2486.2007.01446.x, 2007.
Broadmeadow, S., Thomas, H., Nisbet, T., Valatin, G., Nisbet, T., and Valatin, G.: Valuing flood regulation services of existing forest cover to inform natural capital accounts, The Research Agency of the Forestry Commission, 28 pp., https://cdn.forestresearch.gov.uk/2019/02/final_report_valuing_flood_regulation_services_051218.pdf (last access: 30 April 2024), 2018.
Brown, A. E., Western, A. W., McMahon, T. A., and Zhang, L.: Impact of forest cover changes on annual streamflow and flow duration curves, J. Hydrol., 483, 39–50, https://doi.org/10.1016/j.jhydrol.2012.12.031, 2013.
Brunner, M. I., Slater, L., Tallaksen, L. M., and Clark, M.: Challenges in modeling and predicting floods and droughts: A review, WIREs Water, 8, 1–32, https://doi.org/10.1002/wat2.1520, 2021.
Buechel, M.: Realistic afforestation scenarios in Great Britain at a 1 km scale to run with the land surface model JULES, Zenodo [data set], https://doi.org/10.5281/zenodo.7957084, 2023.
Buechel, M., Slater, L., and Dadson, S.: Hydrological impact of widespread afforestation in Great Britain using a large ensemble of modelled scenarios, Commun. Earth Environ., 3, 1–10, https://doi.org/10.1038/s43247-021-00334-0, 2022.
Burke, T., Rowland, C., Whyatt, J. D., Blackburn, G. A., and Abbatt, J.: Achieving national scale targets for carbon sequestration through afforestation: Geospatial assessment of feasibility and policy implications, Environ. Sci. Policy, 124, 279–292, https://doi.org/10.1016/j.envsci.2021.06.023, 2021.
Carrick, J., Abdul Rahim, M. S. A. Bin, Adjei, C., Ashraa Kalee, H. H. H., Banks, S. J., Bolam, F. C., Campos Luna, I. M., Clark, B., Cowton, J., Domingos, I. F. N., Golicha, D. D., Gupta, G., Grainger, M., Hasanaliyeva, G., Hodgson, D. J., Lopez-Capel, E., Magistrali, A. J., Merrell, I. G., Oikeh, I., Othman, M. S., Ranathunga Mudiyanselage, T. K. R., Samuel, C. W. C., Sufar, E. K. H., Watson, P. A., Zakaria, N. N. A. B., and Stewart, G.: Is planting trees the solution to reducing flood risks?, J. Flood Risk Manage., 12, 1–10, https://doi.org/10.1111/jfr3.12484, 2019.
Clark, D. B. and Gedney, N.: Representing the effects of subgrid variability of soil moisture on runoff generation in a land surface model, J. Geophys. Res., 113, D10111, https://doi.org/10.1029/2007JD008940, 2008.
Clark, D. B., Mercado, L. M., Sitch, S., Jones, C. D., Gedney, N., Best, M. J., Pryor, M., Rooney, G. G., Essery, R. L. H., Blyth, E., Boucher, O., Harding, R. J., Huntingford, C., and Cox, P. M.: The Joint UK Land Environment Simulator (JULES), model description – Part 2: Carbon fluxes and vegetation dynamics, Geosci. Model Dev., 4, 701–722, https://doi.org/10.5194/gmd-4-701-2011, 2011.
Clark, M. P., Rupp, D. E., Woods, R. A., Tromp-van Meerveld, H. J., Peters, N. E., and Freer, J. E.: Consistency between hydrological models and field observations: linking processes at the hillslope scale to hydrological responses at the watershed scale, Hydrol. Process., 23, 311–319, 2009.
Clark, M. P., Kavetski, D., and Fenicia, F.: Pursuing the method of multiple working hypotheses for hydrological modeling, Water Resour. Res., 47, W09301, https://doi.org/10.1029/2010WR009827, 2011.
Clark, M. P., Zolfaghari, R., Green, K. R., Trim, S., Knoben, W. J. M., Bennett, A., Nijssen, B., Ireson, A., and Spiteri, R. J.: The numerical implementation of land models: Problem formulation and laugh tests, J. Hydrometeorol., 22, 1627–1648, https://doi.org/10.1175/JHM-D-20-0175.1, 2021.
Committee on Climate Change: Land use: Reducing emissions and preparing for climate change, 100, 2018.
Committee on Climate Change: Net Zero: The UK's contribution to stopping global warming, Comm. Clim. Change, 277 pp., https://www.theccc.org.uk/wp-content/uploads/2019/05/Net-Zero-The-UKs-contribution-to-stopping-global-warming.pdf (last access: 30 April 2024), 2019a.
Committee on Climate Change: Net Zero Technical Report, 269 pp., https://www.theccc.org.uk/wp-content/uploads/2019/05/Net-Zero-Technical-report-CCC.pdf (last access: 30 April 2024), 2019b.
Cook-Patton, S. C., Leavitt, S. M., Gibbs, D., Harris, N. L., Lister, K., Anderson-Teixeira, K. J., Briggs, R. D., Chazdon, R. L., Crowther, T. W., Ellis, P. W., Griscom, H. P., Herrmann, V., Holl, K. D., Houghton, R. A., Larrosa, C., Lomax, G., Lucas, R., Madsen, P., Malhi, Y., Paquette, A., Parker, J. D., Paul, K., Routh, D., Roxburgh, S., Saatchi, S., van den Hoogen, J., Walker, W. S., Wheeler, C. E., Wood, S. A., Xu, L., and Griscom, B. W.: Mapping carbon accumulation potential from global natural forest regrowth, Nature, 585, 545–550, https://doi.org/10.1038/s41586-020-2686-x, 2020.
Cooper, H. M., Bennett, E., Blake, J., Blyth, E., Boorman, D., Cooper, E., Evans, J., Fry, M., Jenkins, A., Morrison, R., Rylett, D., Stanley, S., Szczykulska, M., Trill, E., Antoniou, V., Askquith-Ellis, A., Ball, L., Brooks, M., Clarke, M. A., Cowan, N., Cumming, A., Farrand, P., Hitt, O., Lord, W., Scarlett, P., Swain, O., Thornton, J., Warwick, A., and Winterbourn, B.: COSMOS-UK: National soil moisture and hydrometeorology data for environmental science research, Earth Syst. Sci. Data, 13, 1737–1757, https://doi.org/10.5194/essd-13-1737-2021, 2021a.
Cooper, M., Patil, S. D., Nisbet, T. R., Thomas, H., Smith, A. R., and McDonald, M. A.: Role of forested land for natural flood management in the UK: A review, WIREs Water, 8, 1–16, https://doi.org/10.1002/wat2.1541, 2021b.
Cox, P. M., Huntingford, C., and Harding, R. J.: A canopy conductance and photosynthesis model for use in a GCM land surface scheme, J. Hydrol., 212–213, 79–94, https://doi.org/10.1016/S0022-1694(98)00203-0, 1998.
Crooks, S. M., Kay, A. L., Davies, H. N., and Bell, V. A.: From catchment to national scale rainfall-runoff modelling: Demonstration of a hydrological modelling framework, Hydrology, 1, 63–88, https://doi.org/10.3390/hydrology1010063, 2014.
Cui, J., Lian, X., Huntingford, C., Gimeno, L., Wang, T., Ding, J., He, M., Xu, H., Chen, A., Gentine, P., and Piao, S.: Global water availability boosted by vegetation-driven changes in atmospheric moisture transport, Nat. Geosci., 15, 982–988, https://doi.org/10.1038/s41561-022-01061-7, 2022.
Cuntz, M., Mai, J., Samaniego, L., Clark, M., Wulfmeyer, V., Branch, O., Attinger, S., and Thober, S.: The impact of standard and hard-coded parameters on the hydrologic fluxes in the Noah-MP land surface model, J. Geophys. Res.-Atmos., 121, 10676–10700, https://doi.org/10.1002/2016JD025097, 2016.
Dadson, S. J.: Statistical Analysis of Geographical Data, John Wiley & Sons, 252 pp., ISBN 0470977043, 2017.
Dadson, S. J., Bell, V. A., and Jones, R. G.: Evaluation of a grid-based river flow model configured for use in a regional climate model, J. Hydrol., 411, 238–250, 2011.
Dadson, S. J., Hall, J. W., Murgatroyd, A., Acreman, M., Bates, P., Beven, K., Heathwaite, L., Holden, J., Holman, I. P., Lane, S. N., O'Connell, E., Penning-Rowsell, E., Reynard, N., Sear, D., Thorne, C., and Wilby, R.: A restatement of the natural science evidence concerning catchment-based `natural' flood management in the UK, Proc. Roy. Soc. A, 473, 20160706, https://doi.org/10.1098/rspa.2016.0706, 2017.
Davies, H., Rameshwaran, P., and V, B.: Gridded (1 km) physical river characteristics for the UK, UK CEH, https://doi.org/10.5285/6da95899-f3b8-4089-b621-560818aa78ba, 2022.
Denissen, J. M. C., Teuling, A. J., Pitman, A. J., Koirala, S., Migliavacca, M., Li, W., Reichstein, M., Winkler, A. J., Zhan, C., and Orth, R.: Widespread shift from ecosystem energy to water limitation with climate change, Nat. Clim. Change, 12, 677–684, https://doi.org/10.1038/s41558-022-01403-8, 2022.
Dick, J., Miller, J. D., Carruthers-Jones, J., Dobel, A. J., Carver, S., Garbutt, A., Hester, A., Hails, R., Magreehan, V., and Quinn, M.: How are nature based solutions contributing to priority societal challenges surrounding human well-being in the United Kingdom: A systematic map protocol, Environ. Evid., 8, 37, https://doi.org/10.1186/s13750-019-0180-4, 2019.
Do, H. X., Westra, S., and Leonard, M.: A global-scale investigation of trends in annual maximum streamflow, J. Hydrol., 552, 28–43, https://doi.org/10.1016/j.jhydrol.2017.06.015, 2017.
Don, A., Rebmann, C., Kolle, O., Scherer-Lorenzen, M., and Schulze, E. D.: Impact of afforestation-associated management changes on the carbon balance of grassland, Global Change Biol., 15, 1990–2002, https://doi.org/10.1111/j.1365-2486.2009.01873.x, 2009.
Ellison, D., Futter, M. N., and Bishop, K.: On the forest cover-water yield debate: From demand- to supply-side thinking, Global Change Biol., 18, 806–820, https://doi.org/10.1111/j.1365-2486.2011.02589.x, 2012.
Environment Agency: Mapping the potential for Working with Natural Processes-technical report Mapping the potential for Working with Natural Processes-technical report SC150005, 85 pp., https://assets.publishing.service.gov.uk/media/6036c659d3bf7f0ab2f070c1/Working_with_natural_processes_mapping_technical_report.pdf (last access: 30 April 2024), 2018.
Farley, K. A., Jobbágy, E. G., and Jackson, R. B.: Effects of afforestation on water yield: A global synthesis with implications for policy, Global Change Biol., 11, 1565–1576, https://doi.org/10.1111/j.1365-2486.2005.01011.x, 2005.
Feeney, C. J., Cosby, B. J., Robinson, D. A., Thomas, A., Emmett, B. A., and Henrys, P.: Multiple soil map comparison highlights challenges for predicting topsoil organic carbon concentration at national scale, Sci. Rep., 12, 1–13, https://doi.org/10.1038/s41598-022-05476-5, 2022.
Fenner, R.: Spatial evaluation of multiple benefits to encourage multi-functional design of sustainable drainage in Blue-Green cities, Water, 9, 16, https://doi.org/10.3390/w9120953, 2017.
Forest Research: Forestry Statistics 2021 – Chapter 1: Woodland Area and Planting, 60 pp., https://www.forestresearch.gov.uk/tools-and-resources/statistics/forestry-statistics/forestry-statistics-2020/1-woodland-area-and-planting/ (last access: 30 April 2024), 2021a.
Forest Research: Provisional Woodland Statistics, 2021 Edition, 1–33, https://www.forestresearch.gov.uk/tools-and-resources/statistics/statistics-by-topic/woodland-statistics/ (last access: 30 April 2024), 2021b.
Fosser, G., Kendon, E., Chan, S., Lock, A., Roberts, N., and Bush, M.: Optimal configuration and resolution for the first convection-permitting ensemble of climate projections over the United Kingdom, Int. J. Climatol., 40, 3585–3606, https://doi.org/10.1002/joc.6415, 2020.
Fowler, H. J., Lenderink, G., Prein, A. F., Westra, S., Allan, R. P., Ban, N., Barbero, R., Berg, P., Blenkinsop, S., Do, H. X., Guerreiro, S., Haerter, J. O., Kendon, E. J., Lewis, E., Schaer, C., Sharma, A., Villarini, G., Wasko, C., and Zhang, X.: Anthropogenic intensification of short-duration rainfall extremes, Nat. Rev. Earth Environ., 2, 107–122, https://doi.org/10.1038/s43017-020-00128-6, 2021.
Friedlingstein, P., Allen, M., Canadell, J. G., Peters, G. P., and Seneviratne, S. I.: Comment on “The global tree restoration potential,” Science, 366, 76–79, https://doi.org/10.1126/science.aay8060, 2019.
Fuller, R., Smith, G., Sanderson, J., Hill, R. A., Thomson, A. G., Cox, R., Brown, N. J., Clarke, R. T., Rothery, P., and Gerard, F. F.: Land cover map 2000 – final report, UK CEH, https://doi.org/10.5285/f802edfc-86b7-4ab9-b8fa-87e9135237c9, 2002.
Gao, J., Kirkby, M., and Holden, J.: The effect of interactions between rainfall patterns and land-cover change on flood peaks in upland peatlands, J. Hydrol., 567, 546–559, https://doi.org/10.1016/j.jhydrol.2018.10.039, 2018.
Gedney, N., Cox, P. M., Betts, R. A., Boucher, O., Huntingford, C., and Stott, P. A.: Detection of a direct carbon dioxide effect in continental river runoff records, Nature, 439, 835–838, https://doi.org/10.1038/nature04504, 2006.
Gedney, N., Huntingford, C., Weedon, G. P., Bellouin, N., Boucher, O., and Cox, P. M.: Detection of solar dimming and brightening effects on Northern Hemisphere river flow, Nat. Geosci., 7, 796–800, https://doi.org/10.1038/ngeo2263, 2014.
Grassi, G., House, J., Dentener, F., Federici, S., Den Elzen, M., and Penman, J.: The key role of forests in meeting climate targets requires science for credible mitigation, Nat. Clim. Change, 7, 220–226, https://doi.org/10.1038/nclimate3227, 2017.
Griffin, A., Vesuviano, G., and Stewart, E.: Have trends changed over time? A study of UK peak flow data and sensitivity to observation period, Nat. Hazards Earth Syst. Sci., 19, 2157–2167, https://doi.org/10.5194/nhess-19-2157-2019, 2019.
Griffin, A., Kay, A., Stewart, L., and Spencer, P.: Climate change allowances, non-stationarity and flood frequency analyses, J. Flood Risk Manage., 15, e12783, https://doi.org/10.1111/jfr3.12783, 2022a.
Griffin, A., Kay, A., Sayers, P., Bell, V., Stewart, E., and Carr, S.: Widespread flooding dynamics changing under climate change: characterising floods using UKCP18, Hydrol. Earth Syst. Sci. Discuss. [preprint], https://doi.org/10.5194/hess-2022-243, in review, 2022b.
Griscom, B. W., Adams, J., Ellis, P. W., Houghton, R. A., Lomax, G., Miteva, D. A., Schlesinger, W. H., Shoch, D., Siikamäki, J. V., Smith, P., Woodbury, P., Zganjar, C., Blackman, A., Campari, J., Conant, R. T., Delgado, C., Elias, P., Gopalakrishna, T., Hamsik, M. R., Herrero, M., Kiesecker, J., Landis, E., Laestadius, L., Leavitt, S. M., Minnemeyer, S., Polasky, S., Potapov, P., Putz, F. E., Sanderman, J., Silvius, M., Wollenberg, E., and Fargione, J.: Natural climate solutions, P. Natl. Acad. Sci. USA, 114, 11645–11650, https://doi.org/10.1073/pnas.1710465114, 2017.
Gudmundsson, L., Leonard, M., Do, H. X., Westra, S., and Seneviratne, S. I.: Observed Trends in Global Indicators of Mean and Extreme Streamflow, Geophys. Res. Lett., 46, 756–766, https://doi.org/10.1029/2018GL079725, 2019.
Gupta, H. V, Kling, H., Yilmaz, K. K., and Martinez, G. F.: Decomposition of the mean squared error and NSE performance criteria: Implications for improving hydrological modelling, J. Hydrol., 377, 80–91, https://doi.org/10.1016/j.jhydrol.2009.08.003, 2009.
Gush, M. B., Scott, D. F., Jewitt, G. P. W., Schulze, R. E., Hallowes, L. A., and Görgens, A. H. M.: A new approach to modelling streamflow reductions resulting from commercial afforestation in South Africa, S. Afr. Forest. J., 196, 27–36, https://doi.org/10.1080/20702620.2002.10434615, 2002.
Hannaford, J., Mastrantonas, N., Vesuviano, G., and Turner, S.: An updated national-scale assessment of trends in UK peak river flow data: How robust are observed increases in flooding?, Hydrol. Res., 52, 699–718, https://doi.org/10.2166/nh.2021.156, 2021.
Harding, R. J., Weedon, G. P., van Lanen, H. A. J., and Clark, D. B.: The future for global water assessment, J. Hydrol., 518, 186–193, https://doi.org/10.1016/j.jhydrol.2014.05.014, 2014.
Harper, A. B., Cox, P. M., Friedlingstein, P., Wiltshire, A. J., Jones, C. D., Sitch, S., Mercado, L. M., Groenendijk, M., Robertson, E., Kattge, J., Bönisch, G., Atkin, O. K., Bahn, M., Cornelissen, J., Niinemets, Ü., Onipchenko, V., Peñuelas, J., Poorter, L., Reich, P. B., Soudzilovskaia, N. A., and Van Bodegom, P.: Improved representation of plant functional types and physiology in the Joint UK Land Environment Simulator (JULES v4.2) using plant trait information, Geosci. Model Dev., 9, 2415–2440, https://doi.org/10.5194/gmd-9-2415-2016, 2016.
Harper, A. B., Williams, K. E., Mcguire, P. C., Duran Rojas, M. C., Hemming, D., Verhoef, A., Huntingford, C., Rowland, L., Marthews, T., Breder Eller, C., Mathison, C., Nobrega, R. L. B., Gedney, N., Vidale, P. L., Otu-Larbi, F., Pandey, D., Garrigues, S., Wright, A., Slevin, D., De Kauwe, M. G., Blyth, E., Ardö;, J., Black, A., Bonal, D., Buchmann, N., Burban, B., Fuchs, K., De Grandcourt, A., Mammarella, I., Merbold, L., Montagnani, L., Nouvellon, Y., Restrepo-Coupe, N., and Wohlfahrt, G.: Improvement of modeling plant responses to low soil moisture in JULESvn4.9 and evaluation against flux tower measurements, Geosci. Model Dev., 14, 3269–3294, https://doi.org/10.5194/gmd-14-3269-2021, 2021.
Harrigan, S., Hannaford, J., Muchan, K., and Marsh, T. J.: Designation and trend analysis of the updated UK Benchmark Network of river flow stations: The UKBN2 dataset, Hydrol. Res., 49, 552–567, https://doi.org/10.2166/nh.2017.058, 2018.
Hausfather, Z. and Peters, G. P.: Emissions – the `business as usual' story is misleading, Nature, 577, 618–620, https://doi.org/10.1038/d41586-020-00177-3, 2020.
Hawes, M.: Planting carbon storage, Nat. Clim. Change, 8, 556–558, https://doi.org/10.1038/s41558-018-0214-x, 2018.
Helsel, D. R., Hirsch, R. M., Ryberg, K. R., Archfield, S. A., and Gilroy, E. J.: Statistical methods in water resources: US Geological Survey Techniques and Methods, USGS, 458 pp., https://doi.org/10.3133/tm4A3, 2020.
Hoek van Dijke, A. J., Herold, M., Mallick, K., Benedict, I., Machwitz, M., Schlerf, M., Pranindita, A., Theeuwen, J. J. E., Bastin, J. F., and Teuling, A. J.: Shifts in regional water availability due to global tree restoration, Nat. Geosci., 15, 363–368, https://doi.org/10.1038/s41561-022-00935-0, 2022.
Hrachowitz, M. and Clark, M. P.: HESS Opinions: The complementary merits of competing modelling philosophies in hydrology, Hydrol. Earth Syst. Sci., 21, 3953–3973, https://doi.org/10.5194/hess-21-3953-2017, 2017.
Hudson, J. A., Crane, S. B., and Robinson, M.: The impact of the growth of new plantation forestry on evaporation and streamflow in the Llanbrynmair catchments, Hydrol. Earth Syst. Sci., 1, 463–475, https://doi.org/10.5194/hess-1-463-1997, 1997.
Hung, C.-L. J., James, L. A., Carbone, G. J., and Williams, J. M.: Impacts of combined land-use and climate change on streamflow in two nested catchments in the Southeastern United States, Ecol. Eng., 143, 105665, https://doi.org/10.1016/j.ecoleng.2019.105665, 2020.
Iacob, O., Brown, I., and Rowan, J.: Natural flood management, land use and climate change trade-offs: the case of Tarland catchment, Scotland, Hydrolog. Sci. J., 62, 1931–1948, https://doi.org/10.1080/02626667.2017.1366657, 2017.
IPPC: Climate Change and Land, An IPCC Special Report on climate change, desertification, land degradation, sustainable land management, food security, and greenhouse gas fluxes in terrestrial ecosystems, 1542 pp., https://doi.org/10.4337/9781784710644, 2019.
Jules-Lsm: Jules-Lsm.github.io, GitHub [code], https://github.com/jules-lsm/jules-lsm.github.io (last access: 21 April 2024), 2024.
Kay, A. L.: Simulation of river flow in Britain under climate change: Baseline performance and future seasonal changes, Hydrol. Process., 35, 1–10, https://doi.org/10.1002/hyp.14137, 2021.
Kay, A. L., Griffin, A., Rudd, A. C., Chapman, R. M., Bell, V. A., and Arnell, N. W.: Climate change effects on indicators of high and low river flow across Great Britain, Adv. Water Resour., 151, 103909, https://doi.org/10.1016/j.advwatres.2021.103909, 2021.
Kellner, E. and Hubbart, J. A.: Land use impacts on floodplain water table response to precipitation events, Ecohydrology, 11, e1913, https://doi.org/10.1002/eco.1913, 2018.
Kendon, E. J., Fischer, E. M., and Short, C. J.: Variability conceals emerging trend in 100 yr projections of UK local hourly rainfall extremes, Nat. Commun., 14, 1133, https://doi.org/10.1038/s41467-023-36499-9, 2023.
Knoben, W. J. M., Freer, J. E., and Woods, R. A.: Technical note: Inherent benchmark or not? Comparing Nash-Sutcliffe and Kling–Gupta efficiency scores, Hydrol. Earth Syst. Sci., 23, 4323–4331, https://doi.org/10.5194/hess-23-4323-2019, 2019.
Kundzewicz, Z. W.: Nonstationarity in water resources – Central European perspective, J. Am. Water Resour. Assoc., 47, 550–562, https://doi.org/10.1111/j.1752-1688.2011.00549.x, 2011.
La Follette, P. T., Teuling, A. J., Addor, N., Clark, M., Jansen, K., and Melsen, L. A.: Numerical daemons of hydrological models are summoned by extreme precipitation, Hydrol. Earth Syst. Sci., 25, 5425–5446, https://doi.org/10.5194/hess-25-5425-2021, 2021.
Lane, R. A., Coxon, G., Freer, J. E., Wagener, T., Johnes, P. J., Bloomfield, J. P., Greene, S., Macleod, C. J. A., and Reaney, S. M.: Benchmarking the predictive capability of hydrological models for river flow and flood peak predictions across over 1000 catchments in Great Britain, Hydrol. Earth Syst. Sci., 23, 4011–4032, https://doi.org/10.5194/hess-23-4011-2019, 2019.
Lane, R. A. and Kay, A. L.: Climate Change Impact on the Magnitude and Timing of Hydrological Extremes Across Great Britain, Front. Water, 3, 1–14, https://doi.org/10.3389/frwa.2021.684982, 2021.
Lane, R. A., Freer, J. E., Coxon, G., and Wagener, T.: Incorporating Uncertainty Into Multiscale Parameter Regionalization to Evaluate the Performance of Nationally Consistent Parameter Fields for a Hydrological Model, Water Resour. Res., 57, 1–19, https://doi.org/10.1029/2020WR028393, 2021.
Lee, D., Min, S. K., Park, I. H., Ahn, J. B., Cha, D. H., Chang, E. C., and Byun, Y. H.: Enhanced Role of Convection in Future Hourly Rainfall Extremes Over South Korea, Geophys. Res. Lett., 49, 1–11, https://doi.org/10.1029/2022GL099727, 2022.
Lees, T., Buechel, M., Anderson, B., Slater, L., Reece, S., Coxon, G., and Dadson, S. J.: Benchmarking data-driven rainfall-runoff models in Great Britain: A comparison of long short-term memory (LSTM)-based models with four lumped conceptual models, Hydrol. Earth Syst. Sci., 25, 5517–5534, https://doi.org/10.5194/hess-25-5517-2021, 2021.
Leung, J. Y. S., Russell, B. D., and Connell, S. D.: Global Warming of 1.5 °C – Summary for Policymakers, Intergov. Panel Clim. Change, 1, 374–381, 2019.
Le Vine, N., Butler, A., McIntyre, N., and Jackson, C.: Diagnosing hydrological limitations of a land surface model: Application of JULES to a deep-groundwater chalk basin, Hydrol. Earth Syst. Sci., 20, 143–159, https://doi.org/10.5194/hess-20-143-2016, 2016.
Lewis, H. W. and Dadson, S. J.: A regional coupled approach to water cycle prediction during winter 2013/14 in the United Kingdom, Hydrol. Process., 35, 1–24, https://doi.org/10.1002/hyp.14438, 2021.
Lewis, S. L., Wheeler, C. E., Mitchard, E. T. A., and Koch, A.: Regenerate natural forests to store carbon, Nature, 568, 25–28, 2019.
Li, Y., Piao, S., Li, L. Z. X., Chen, A., Wang, X., Ciais, P., Huang, L., Lian, X., Peng, S., Zeng, Z., Wang, K., and Zhou, L.: Divergent hydrological response to large-scale afforestation and vegetation greening in China, Sci. Adv., 4, 1–10, https://doi.org/10.1126/sciadv.aar4182, 2018.
Lowe, J. A., Bernie, D., Bett, P., Bricheno, L., Brown, S., Calvert, D., Clark, R., Eagle, K., Edwards, T., Fosser, G., Fung, F., Gohar, L., Good, P., Gregory, J., Harris, G., Howard, T., Kaye, N., Kendon, E., Krijnen, J., Maisey, P., McDonald, R., McInnes, R., McSweeney, C., Mitchell, J. F. B., Murphy, J., Palmer, M., Roberts, C., Rostron, J., Sexton, D., Thornton, H., Tinker, J., Tucker, S., Yamazaki, K., and Belcher, S.: UKCP18 science overview report, Met Office Hadley Centre, Exeter, UK, 1–73, https://www.metoffice.gov.uk/pub/data/weather/uk/ukcp18/science-reports/UKCP18-Overview-report.pdf (last access: 30 April 2024), 2018.
Mai, J., Craig, J. R., and Tolson, B. A.: Simultaneously determining global sensitivities of model parameters and model structure, Hydrol. Earth Syst. Sci., 24, 5835–5858, https://doi.org/10.5194/hess-24-5835-2020, 2020.
Marc, V. and Robinson, M.: The long-term water balance (1972–2004) of upland forestry and grassland at Plynlimon, mid-Wales, Hydrol. Earth Syst. Sci., 11, 44–60, https://doi.org/10.5194/hess-11-44-2007, 2007.
Martínez-de la Torre, A., Blyth, E. M., and Robinson, E. L.: Water, carbon and energy fluxes simulation for Great Britain using the JULES Land Surface Model and the Climate Hydrology and Ecology research Support System meteorology dataset (1961–2015) [CHESS-land], UK CEH, https://doi.org/10.5285/c76096d6-45d4-4a69-a310-4c67f8dcf096, 2018.
Martínez-de la Torre, A., Blyth, E. M., and Weedon, G. P.: Using observed river flow data to improve the hydrological functioning of the JULES land surface model (vn4.3) used for regional coupled modelling in Great Britain (UKC2), Geosci. Model Dev., 12, 765–784, https://doi.org/10.5194/gmd-12-765-2019, 2019.
Mathison, C., Burke, E., Hartley, A. J., Kelley, D. I., Burton, C., Robertson, E., Gedney, N., Williams, K., Wiltshire, A., Ellis, R. J., Sellar, A. A., and Jones, C. D.: Description and evaluation of the JULES-ES set-up for ISIMIP2b, Geosci. Model Dev., 16, 4249–4264, https://doi.org/10.5194/gmd-16-4249-2023, 2023.
Meier, R., Schwaab, J., Seneviratne, S. I., Sprenger, M., Lewis, E., and Davin, E. L.: Empirical estimate of forestation-induced precipitation changes in Europe, Nat. Geosci., 14, 473–478, https://doi.org/10.1038/s41561-021-00773-6, 2021.
Met Office: UKCP18 Regional Projections on a 12 km grid over the UK for 1980–2080, https://catalogue.ceda.ac.uk/uuid/589211abeb844070a95d061c8cc7f604 (last access: 30 April 2024), 2018.
Milly, P. C. D., Betancourt, J., Falkenmark, M., Hirsch, R. M., Kundzewicz, Z. W., Lettenmaier, D. P., and Stouffer, R. J.: Climate change: Stationarity is dead: Whither water management?, Science, 319, 573–574, https://doi.org/10.1126/science.1151915, 2008.
Moore, R. J.: The PDM rainfall-runoff model, Hydrol. Earth Syst. Sci., 11, 483–499, https://doi.org/10.5194/hess-11-483-2007, 2007.
Murphy, J., Harris, G., Sexton, D., Kendon, E., Bett, P., Clark, R., Eagle, K., Fosser, G., Fung, F., Lowe, J., McDonald, R., McInnes, R., McSweeney, C., Mitchell, J., Rostron, J., Thornton, H., Tucker, S., and Yamazaki, K.: UKCP18 Land report, UKCP18 L. Proj. Sci. Rep. 2018, Met Office, https://www.metoffice.gov.uk/pub/data/weather/uk/ukcp18/science-reports/UKCP18-Land-report.pdf (last access: 30 April 2024), 2019.
Murphy, T. R., Hanley, M. E., Ellis, J. S., and Lunt, P. H.: Native woodland establishment improves soil hydrological functioning in UK upland pastoral catchments, Land Degrad. Dev., 32, 1034–1045, https://doi.org/10.1002/ldr.3762, 2021.
Newson, M. D. and Calder, I. R.: Forests and water resources: problems of prediction on a regional scale, Philos. T. Roy. Soc. Lond. B, 324, 283–298, https://doi.org/10.1098/rstb.1989.0049, 1989.
Nisbet, T. and Thomas, H.: Trees, woodlands and flooding, Q. J. Forest., 115, 55–63, 2021.
Nisbet, T., Silgram, M., Shah, N., Morrow, K., and Broadmeadow, S.: Woodland for Water: Woodland measures for meeting Water Framework Directive objectives, Forest Research, 156 pp., https://cdn.forestresearch.gov.uk/2022/02/frmg004_woodland4water-2.pdf (last access: 30 April 2024), 2011.
NOAA: Global Monitoring Laboratory – Carbon Cycle Greenhouse Gases: Trends in CO2, https://gml.noaa.gov/ccgg/trends/mlo.html (last access: 31 October 2022), 2022.
O'Briain, R., Shephard, S., Matson, R., Gordon, P., and Kelly, F. L.: The efficacy of riparian tree cover as a climate change adaptation tool is affected by hydromorphological alterations, Hydrol. Process., 34, 2433–2449, https://doi.org/10.1002/hyp.13739, 2020.
Oldfield, E. E., Warren, R. J., Felson, A. J., and Bradford, M. A.: FORUM: Challenges and future directions in urban afforestation, J. Appl. Ecol., 50, 1169–1177, https://doi.org/10.1111/1365-2664.12124, 2013.
Oudin, L., Andréassian, V., Lerat, J., and Michel, C.: Has land cover a significant impact on mean annual streamflow? An international assessment using 1508 catchments, J. Hydrol., 357, 303–316, https://doi.org/10.1016/j.jhydrol.2008.05.021, 2008.
Page, T., Chappell, N. A., Beven, K. J., Hankin, B., and Kretzschmar, A.: Assessing the significance of wet-canopy evaporation from forests during extreme rainfall events for flood mitigation in mountainous regions of the United Kingdom, Hydrol. Process., 34, 4740–4754, https://doi.org/10.1002/hyp.13895, 2020.
Palmer, L.: How trees and forests reduce risks from climate change, Nat. Clim. Change, 11, 374–377, https://doi.org/10.1038/s41558-021-01041-6, 2021.
Pattison, I. and Lane, S. N.: The link between land-use management and fluvial flood risk: A chaotic conception?, Prog. Phys. Geogr., 36, 72–92, https://doi.org/10.1177/0309133311425398, 2012.
Peng, J., Tanguy, M., Robinson, E. L., Pinnington, E., Evans, J., Ellis, R., Cooper, E., Hannaford, J., Blyth, E., and Dadson, S.: Estimation and evaluation of high-resolution soil moisture from merged model and Earth observation data in the Great Britain, Remote Sens. Environ., 264, 112610, https://doi.org/10.1016/j.rse.2021.112610, 2021.
Peskett, L., MacDonald, A., Heal, K., McDonnell, J., Chambers, J., Uhlemann, S., Upton, K., and Black, A.: The impact of across-slope forest strips on hillslope subsurface hydrological dynamics, J. Hydrol., 581, 124427, https://doi.org/10.1016/j.jhydrol.2019.124427, 2020.
Prudhomme, C., Parry, S., Hannaford, J., Clark, D. B., Hagemann, S., and Voss, F.: How well do large-scale models reproduce regional hydrological extremes: In Europe?, J. Hydrometeorol., 12, 1181–1204, https://doi.org/10.1175/2011JHM1387.1, 2011.
Prudhomme, C., Dadson, S., Morris, D., Williamson, J., Goodsell, G., Crooks, S., Boelee, L., Davies, H., Buys, G., Lafon, T., and Watts, G.: Future flows climate: An ensemble of 1-km climate change projections for hydrological application in Great Britain, Earth Syst. Sci. Data, 4, 143–148, https://doi.org/10.5194/essd-4-143-2012, 2012.
Prudhomme, C., Giuntoli, I., Robinson, E. L., Clark, D. B., Arnell, N. W., Dankers, R., Fekete, B. M., Franssen, W., Gerten, D., Gosling, S. N., Hagemann, S., Hannah, D. M., Kim, H., Masaki, Y., Satoh, Y., Stacke, T., Wada, Y., and Wisser, D.: Hydrological droughts in the 21st century, hotspots and uncertainties from a global multimodel ensemble experiment, P. Natl. Acad. Sci. USA, 111, 3262–3267, https://doi.org/10.1073/pnas.1222473110, 2014.
Riahi, K., van Vuuren, D. P., Kriegler, E., Edmonds, J., O'Neill, B. C., Fujimori, S., Bauer, N., Calvin, K., Dellink, R., Fricko, O., Lutz, W., Popp, A., Cuaresma, J. C., KC, S., Leimbach, M., Jiang, L., Kram, T., Rao, S., Emmerling, J., Ebi, K., Hasegawa, T., Havlik, P., Humpenöder, F., Da Silva, L. A., Smith, S., Stehfest, E., Bosetti, V., Eom, J., Gernaat, D., Masui, T., Rogelj, J., Strefler, J., Drouet, L., Krey, V., Luderer, G., Harmsen, M., Takahashi, K., Baumstark, L., Doelman, J. C., Kainuma, M., Klimont, Z., Marangoni, G., Lotze-Campen, H., Obersteiner, M., Tabeau, A., and Tavoni, M.: The Shared Socioeconomic Pathways and their energy, land use, and greenhouse gas emissions implications: An overview, Global Environ. Change, 42, 153–168, https://doi.org/10.1016/j.gloenvcha.2016.05.009, 2017.
Ritchie, P. D. L., Harper, A. B., Smith, G. S., Kahana, R., Kendon, E. J., Lewis, H., Fezzi, C., Halleck-Vega, S., Boulton, C. A., Bateman, I. J., and Lenton, T. M.: Large changes in Great Britain's vegetation and agricultural land-use predicted under unmitigated climate change, Environ. Res. Lett., 14, 114012, https://doi.org/10.1088/1748-9326/ab492b, 2019.
Roberts, J. and Rosier, P.: The impact of broadleaved woodland on water resources in lowland UK: I. Soil water changes below beech woodland and grass on chalk sites in Hampshire, Hydrol. Earth Syst. Sci., 9, 596–606, https://doi.org/10.5194/hess-9-596-2005, 2005.
Robinson, E. L., Blyth, E. M., Clark, D. B., Comyn-Platt, E., Finch, J., and Rudd, A. C.: Climate hydrology and ecology research support system meteorology dataset for Great Britain (1961–2015) [CHESS-met] v1.2, NERC Environmental Information Data Centre [data set], https://doi.org/10.5285/b745e7b1-626c-4ccc-ac27-56582e77b900, 2017a.
Robinson, E. L., Blyth, E. M., Clark, D. B., Finch, J., and Rudd, A. C.: Trends in atmospheric evaporative demand in Great Britain using high-resolution meteorological data, Hydrol. Earth Syst. Sci., 21, 1189–1224, https://doi.org/10.5194/hess-21-1189-2017, 2017b.
Robinson, E. L., Huntingford, C., Semeena, V. S., and Bullock, J. M.: CHESS-SCAPE: Future projections of meteorological variables at 1 km resolution for the United Kingdom 1980–2080 derived from UK Climate Projections 2018, NERC EDS Centre for Environmental Data Analysis [data set], https://doi.org/10.5285/8194b416cbee482b89e0dfbe17c5786c, 2022.
Roebroek, C. T. J., Melsen, L. A., Hoek van Dijke, A. J., Fan, Y., and Teuling, A. J.: Global distribution of hydrologic controls on forest growth, Hydrol. Earth Syst. Sci., 24, 4625–4639, https://doi.org/10.5194/hess-24-4625-2020, 2020.
Rogger, M., Agnoletti, M., Alaoui, A., Bathurst, J. C., Bodner, G., Borga, M., Chaplot, V., Gallart, F., Glatzel, G., Hall, J., Holden, J., Holko, L., Horn, R., Kiss, A., Kohnová, S., Leitinger, G., Lennartz, B., Parajka, J., Perdigão, R., Peth, S., Plavcová, L., Quinton, J. N., Robinson, M., Salinas, J. L., Santoro, A., Szolgay, J., Tron, S., van den Akker, J. J. H., Viglione, A., and Blöschl, G.: Land use change impacts on floods at the catchment scale: Challenges and opportunities for future research, Water Resour. Res., 53, 5209–5219, https://doi.org/10.1002/2017WR020723, 2017.
ROSE: Rose Documentation, GitHub, https://metomi.github.io/rose/doc/html/index.html (last access: 21 April 2024), 2024.
Schwaab, J., Davin, E. L., Bebi, P., Duguay-Tetzlaff, A., Waser, L. T., Haeni, M., and Meier, R.: Increasing the broad-leaved tree fraction in European forests mitigates hot temperature extremes, Sci. Rep., 10, 1–9, https://doi.org/10.1038/s41598-020-71055-1, 2020.
Seddon, N., Chausson, A., Berry, P., Girardin, C. A. J. J., Smith, A., and Turner, B.: Understanding the value and limits of nature-based solutions to climate change and other global challenges, Philos. T. Roy. Soc. B, 375, 20190120, https://doi.org/10.1098/rstb.2019.0120, 2020.
Seddon, N., Smith, A., Smith, P., Key, I., Chausson, A., Girardin, C., House, J., Srivastava, S., and Turner, B.: Getting the message right on nature-based solutions to climate change, Global Change Biol., 27, 1518–1546, https://doi.org/10.1111/gcb.15513, 2021.
Sen, P. K.: Estimates of the Regression Coefficient Based on Kendall's Tau, J. Am. Stat. Assoc., 63, 1379–1389, https://doi.org/10.1080/01621459.1968.10480934, 1968.
Shuttleworth, E. L., Evans, M. G., Pilkington, M., Spencer, T., Walker, J., Milledge, D., and Allott, T. E. H.: Restoration of blanket peat moorland delays stormflow from hillslopes and reduces peak discharge, J. Hydrol. X, 2, 100006, https://doi.org/10.1016/j.hydroa.2018.100006, 2019.
Sing, L. and Aitkenhead, M.: Analysis of land suitability for woodland expansion in Scotland: update 2020, Edinburgh Research Archive, https://doi.org/10.7488/era/494, 2020.
Slater, L. J., Anderson, B., Buechel, M., Dadson, S., Han, S., Harrigan, S., Kelder, T., Kowal, K., Lees, T., Matthews, T., Murphy, C., and Wilby, R. L.: Nonstationary weather and water extremes: a review of methods for their detection, attribution, and management, Hydrol. Earth Syst. Sci., 25, 3897–3935, https://doi.org/10.5194/hess-25-3897-2021, 2021b.
Speich, M. J. R., Zappa, M., and Lischke, H.: Sensitivity of forest water balance and physiological drought predictions to soil and vegetation parameters – A model-based study, Environ. Model. Softw., 102, 213–232, https://doi.org/10.1016/j.envsoft.2018.01.016, 2018.
Stratford, C., Miller, J., House, A., Old, G., Acreman, M., Dueñas-Lopez, M. A., Nisbet, T., Newman, J., Burgess-Gamble, L., Chappell, N., Clarke, S., Leeson, L., Monbiot, G., Paterson, J., Robinson, M., Rogers, M., and Tickner, D.: Do Trees in the UK-Relevant River Catchments Influence Fluvial Flood Peaks?, 46 pp., https://core.ac.uk/download/pdf/96704761.pdf (last access: 30 April 2024), 2017.
Teuling, A. J., Taylor, C. M., Meirink, J. F., Melsen, L. A., Miralles, D. G., van Heerwaarden, C. C., Vautard, R., Stegehuis, A. I., Nabuurs, G.-J., and de Arellano, J. V.-G.: Observational evidence for cloud cover enhancement over western European forests, Nat. Commun., 8, 14065, https://doi.org/10.1038/ncomms14065, 2017.
Teuling, A. J., de Badts, E. A. G., Jansen, F. A., Fuchs, R., Buitink, J., Hoek van Dijke, A. J., and Sterling, S. M.: Climate change, reforestation/afforestation, and urbanization impacts on evapotranspiration and streamflow in Europe, Hydrol. Earth Syst. Sci., 23, 3631–3652, https://doi.org/10.5194/hess-23-3631-2019, 2019.
Theil, H.: A Rank-Invariant Method of Linear and Polynomial Regression Analysis, in: Indagationes mathematicae, vol. 12, Springer, 345–381, https://doi.org/10.1007/978-94-011-2546-8_20, 1992.
UKCEH: Climate hydrology and ecology research support system [CHESS], https://catalogue.ceh.ac.uk/documents/7de9790e-66a2-44b5-988e-283d764ef52f (last access: 21 April 2024), 2024.
Van den Hoof, C., Vidale, P. L., Verhoef, A., and Vincke, C.: Improved evaporative flux partitioning and carbon flux in the land surface model JULES: Impact on the simulation of land surface processes in temperate Europe, Agr. Forest Meteorol., 181, 108–124, https://doi.org/10.1016/j.agrformet.2013.07.011, 2013.
van Genuchten, M. T.: A Closed-form Equation for Predicting the Hydraulic Conductivity of Unsaturated Soils, Soil Sci. Soc. Am. J., 44, 892–898, https://doi.org/10.2136/sssaj1980.03615995004400050002x, 1980.
van Kempen, G., van der Wiel, K., and Melsen, L. A.: The impact of hydrological model structure on the simulation of extreme runoff events, Nat. Hazards Earth Syst. Sci., 21, 961–976, https://doi.org/10.5194/nhess-21-961-2021, 2021.
Vereecken, H., Amelung, W., Bauke, S. L., Bogena, H., Brüggemann, N., Montzka, C., Vanderborght, J., Bechtold, M., Blöschl, G., Carminati, A., Javaux, M., Konings, A. G., Kusche, J., Neuweiler, I., Or, D., Steele-Dunne, S., Verhoef, A., Young, M., and Zhang, Y.: Soil hydrology in the Earth system, Nat. Rev. Earth Environ., 3, 573–587, https://doi.org/10.1038/s43017-022-00324-6, 2022.
Villarini, G. and Wasko, C.: Humans, climate and streamflow, Nat. Clim. Change, 11, 725–726, https://doi.org/10.1038/s41558-021-01137-z, 2021.
Vitolo, C., Fry, M., and Buytaert, W.: Rnrfa: An r package to retrieve, filter and visualize data from the uk national river flow archive, R J., 8, 102–116, https://doi.org/10.32614/rj-2016-036, 2016.
Wagener, T., Gleeson, T., Coxon, G., Hartmann, A., Howden, N., Pianosi, F., Rahman, M., Rosolem, R., Stein, L., and Woods, R.: On doing hydrology with dragons: Realizing the value of perceptual models and knowledge accumulation, Wiley Interdisciplin. Rev. Water, 8, 1–17, https://doi.org/10.1002/wat2.1550, 2021.
Wasko, C.: Review: Can temperature be used to inform changes to flood extremes with global warming?, Philos. T. Roy. Soc. A, 379, 20190551, https://doi.org/10.1098/rsta.2019.0551, 2021.
Wasko, C., Sharma, A., and Lettenmaier, D. P.: Increases in temperature do not translate to increased flooding, Nat. Commun., 10, 4–6, https://doi.org/10.1038/s41467-019-13612-5, 2019.
Wasko, C., Nathan, R., Stein, L., and O'Shea, D.: Evidence of shorter more extreme rainfalls and increased flood variability under climate change, J. Hydrol., 603, 126994, https://doi.org/10.1016/j.jhydrol.2021.126994, 2021.
Welsh Government: Woodland Opportunity Map 2021|DataMapWales, https://datamap.gov.wales/maps/woodland-opportunity-map-2021/ (last access: 4 August 2022), 2022.
Wilby, R. L. and Quinn, N. W.: Reconstructing multi-decadal variations in fluvial flood risk using atmospheric circulation patterns, J. Hydrol., 487, 109–121, https://doi.org/10.1016/j.jhydrol.2013.02.038, 2013.
Wilkes, M. A., Bennett, J., Burbi, S., Charlesworth, S., Dehnen-Schmutz, K., Rayns, F., Schmutz, U., Smith, B., Tilzey, M., Trenchard, L., and van de Wiel, M.: Making way for trees? Changes in land-use, habitats and protected areas in Great Britain under “Global tree restoration potential”, Sustainability, 12, 5845, https://doi.org/10.3390/su12145845, 2020.
Xu, R., Li, Y., Teuling, A. J., Zhao, L., Spracklen, D. V., Garcia-Carreras, L., Meier, R., Chen, L., Zheng, Y., Lin, H., and Fu, B.: Contrasting impacts of forests on cloud cover based on satellite observations, Nat. Commun., 13, 670, https://doi.org/10.1038/s41467-022-28161-7, 2022.
Young, P. J., Harper, A. B., Huntingford, C., Paul, N. D., Morgenstern, O., Newman, P. A., Oman, L. D., Madronich, S., and Garcia, R. R.: The Montreal Protocol protects the terrestrial carbon sink, Nature, 596, 384–388, https://doi.org/10.1038/s41586-021-03737-3, 2021.
Zhang, X., Jin, J., Zeng, X., Hawkins, C. P., Neto, A. A. M., and Niu, G.: The Compensatory CO2 Fertilization and Stomatal Closure Effects on Runoff Projection From 2016–2099 in the Western United States, Water Resour. Res., 58, e2021WR030046, https://doi.org/10.1029/2021wr030046, 2022.