the Creative Commons Attribution 4.0 License.
the Creative Commons Attribution 4.0 License.
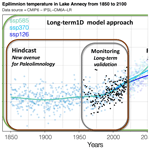
Past and future climate change effects on the thermal regime and oxygen solubility of four peri-alpine lakes
Olivia Desgué-Itier
Laura Melo Vieira Soares
Orlane Anneville
Damien Bouffard
Vincent Chanudet
Pierre Alain Danis
Isabelle Domaizon
Jean Guillard
Théo Mazure
Najwa Sharaf
Frédéric Soulignac
Viet Tran-Khac
Brigitte Vinçon-Leite
Jean-Philippe Jenny
Long-term effects of climate change on lakes globally will include a substantial modification in the thermal regime and the oxygen solubility of lakes, resulting in the alteration of ecosystem processes, habitats, and concentrations of critical substances. Recent efforts have led to the development of long-term model projections of climate change effects on lake thermal regimes and oxygen solubility. However, such projections are hardly ever confronted with observations extending over multiple decades. Furthermore, global-scale forcing parameters in lake models present several limitations, such as the need of significant downscaling. In this study, the effects of climate change on thermal regime and oxygen solubility were analyzed in the four largest French peri-alpine lakes over 1850–2100. We tested several one-dimensional (1D) lake models' robustness for long-term variations based on up to 63 years of limnological data collected by the French Observatory of LAkes (OLA). Here, we evaluate the possibility of forcing mechanistic models by following the long-term evolution of shortwave radiation and air temperature while providing realistic seasonal trends for the other variables for which local-scale downscaling often lacks accuracy. Based on this approach, MyLake, forced by air temperatures and shortwave radiations, predicted accurately the variations in the lake thermal regime over the last 4 to 6 decades, with RMSE < 1.95 ∘C. Over the previous 3 decades, water temperatures have increased by 0.46 ∘C per decade (±0.02 ∘C) in the epilimnion and 0.33 ∘C per decade (±0.06 ∘C) in the hypolimnion. Concomitantly and due to thermal change, O2 solubility has decreased by −0.104 mg L−1 per decade (±0.005 mg L−1) and −0.096 mg L−1 per decade (±0.011 mg L−1) in the epilimnion and hypolimnion, respectively. Based on the shared socio-economic pathway SSP370 of the Intergovernmental Panel on Climate Change (IPCC), peri-alpine lakes could face an increase of 3.80 ∘C (±0.20 ∘C) in the next 70 years, accompanied by a decline of 1.0 mg L−1 (±0.1 mg L−1) of O2 solubility. Together, these results highlight a critical alteration in lake thermal and oxygen conditions in the coming decades, and a need for a better integration of long-term lake observatories data and lake models to anticipate climate effects on lake thermal regimes and habitats.
Please read the corrigendum first before continuing.
-
Notice on corrigendum
The requested paper has a corresponding corrigendum published. Please read the corrigendum first before downloading the article.
-
Article
(7155 KB)
- Corrigendum
-
Supplement
(951 KB)
-
The requested paper has a corresponding corrigendum published. Please read the corrigendum first before downloading the article.
- Article
(7155 KB) - Full-text XML
- Corrigendum
-
Supplement
(951 KB) - BibTeX
- EndNote
Lakes are critical resources providing humanity with key ecosystem services such as hydropower and drinking water production (Jenny et al., 2020) and are considered sentinels of climate change (Williamson et al., 2009). These ecosystems are increasingly altered by anthropogenic pressures and ongoing global warming, which requires continuous water quality monitoring. Water temperature is a critical indicator for long-term lake ecosystems monitoring, which is also needed for adapting management practices (Daufresne et al., 2009). It is an important parameter impacting lake ecosystems' metabolism, composition, and functioning, and reflects their response to climate change. Water temperature directly affects the growth rate and reproductive success of many aquatic organisms (Angilletta and Dunham, 2003; Mari et al., 2016) and their phenology (Parmesan, 2006; Walther et al., 2002). Furthermore, the water temperature can also indirectly affect organisms, by altering the mixing regime of lakes and gas solubility with potential impacts on oxygenation, one of the most fundamental parameters of life in lakes (Roberts et al., 2009a; Wetzel, 2001).
Recent studies from lakes around the world have already shown that increasing air temperature significantly affects the intensification and the duration of their stratification (Woolway and Merchant, 2019; Piccioni et al., 2021; Woolway et al., 2021). In deep temperate lakes, vertical mixing of the water column has experienced a decrease in intensity, frequency, and duration (Råman Vinnå et al., 2021; Danis et al., 2004), thereby increasing the vertical temperature gradient between the surface and deep layers (Livingstone, 2003). However, both retrospective and prospective studies of lake thermal regimes over decadal to centennial timescales are still limited, precluding our understanding of the evolution of lake physicochemical conditions and habitats and the response mechanisms to the forcings involved over such timescales.
Mechanistic lake models have been widely implemented over the last years (Bruce et al., 2018; Snortheim et al., 2017; Shatwell et al., 2019), and are considered as essential tools for understanding, analyzing, testing different scenarios, and predicting the state of an ecosystem under external constraints over different timescales (Trolle et al., 2012). More specifically, using one-dimensional (1D) models for global-scale studies has become the standard in lake research. These models are suitable to simulate complex ecosystems, as they require minimum configuration parameters (Hamilton and Schladow, 1997; Vinçon-Leite et al., 2014), and to perform reliable predictions of lake responses to global warming (Balsamo et al., 2012). However, such an approach requires global-scale forcing parameters that have several limitations that are barely discussed. Among these limitations, the influence of the rivers and watersheds is not systematically assessed, even though it can have a strong effect on the thermal structure of lakes with a short residence time (e.g., <1–3 years) (Råman Vinnå et al., 2017, 2018; Perga et al., 2018). Another limitation relies on the quality of the forcing. Although some studies showed very similar model performances between different kinds of measured or re-analyzed meteorological databases (Sadeghian et al., 2021), lakes are often smaller than the meteorological grid size used in studies. Some parameters such as wind can strongly vary at local scales, especially in alpine regions. This issue is at times tackled by using meteorological forcing downscaled to local weather stations (Råman Vinnå et al., 2021), or by trying to simplify the heat budget (e.g., Piccolroaz et al., 2013). The quality of the input data also limits the possibility of estimating changes in the thermal structure over very long trends (>100 years). Models are most of the time calibrated against very few years of limnological records so far (Soares and Calijuri, 2021), potentially limiting the robustness of hindcast/forecast exercises on long-term timescales such as pluri-decadal or pluri-centennial.
To address this limitation, the first aim of our study was to adapt an existing modeling approach for long-term studies constrained by the Intergovernmental Panel on Climate Change (IPCC) scenarios, and to calibrate and validate the model against up to 63 years of limnological records from four peri-alpine lakes monitored by the Observatory of LAkes (OLA) (Rimet et al., 2020): Geneva, Annecy, Bourget, and Aiguebelette. Here we evaluate the possibility of forcing mechanistic models by following the long-term evolution of only shortwave radiation (W m−2) and air temperature (∘C) while providing realistic seasonal trends for the other variables, such as wind speed, for which local-scale downscaling often lacks accuracy and present more uncertainties in the long term. This was supported by a previous study showing that ∼60 % of the observed warming of spring and summer lake surface temperatures was caused by increased air temperature and ∼40 % by increased solar radiation (Schmid and Köster, 2016). We use unique long observation datasets to insure the robustness of our findings.
The second objective of this study was to investigate the evolution of the thermal regime and the solubility of oxygen, as proxies for the species' habitats and their capacity to cope with temperature change (Kraemer et al., 2021), in the lakes over 1850–2100 to assess their different responses and sensitivity to global warming.
We begin as follows: (1) by describing our approach for long-term forecast/hindcast based on a reduced number of meteorological forcing variables; (2) by presenting the MyLake model calibration and validation over a relatively short period of 10 years, using both complete and reduced meteorological inputs; then (3) we test the method over longer timescales, i.e., against 37 to 63 years of monitoring data; and finally (4) we explore trends in lake thermal regimes through 15 physical indices, and we estimate effects of climate change on the dissolved oxygen solubility in the four lakes over the 1850–2100 period using an ensemble of climate projections based on the shared socio-economic pathways (SSP126, SSP370, and SSP585) (Riahi et al., 2017).
2.1 Study sites
We consider four lakes in France's peri-alpine region: Lake Geneva, Lake Annecy, Lake Bourget, and Lake Aiguebelette (Fig. 1). They are situated in a continental mountain climate, and less than 150 km separates the four lakes from each other. The four lakes are mono or meromictic – they mix only once per year – and are deep and of glacial origin. Lake Geneva and Lake Aiguebelette are mesotrophic lakes, whereas Lake Annecy and Lake Bourget are oligotrophic and oligo-mesotrophic, respectively (Table 1).
2.2 Lake data
The Observatory of LAkes (OLA) managed by the CARRTEL (https://si-ola.inra.fr/si_lacs/login.jsf, last access: 17 July 2021) has regularly monitored the physical and chemical conditions of peri-alpine lakes. Limnological data derived from the OLA observatory databases provide several decades (from 37 to 63 years) (Table 5) of monitoring of lake thermal conditions, i.e., water temperatures along the water column in the pelagic zone. The study sites were monitored for up to 6 decades by CARRTEL. Measurements were recorded at the deepest point of each lake, generally twice a month from March to November, and once a month during the rest of the year, except for Lake Aiguebelette, which is monitored six times a year.
2.3 Hydrodynamic modeling
A modeling approach was performed to run five one-dimensional hydrodynamic lake models simultaneously (FLake, GLM, GOTM, Simstrat, and MyLake) to simulate the vertical water temperature profile in the four peri-alpine lakes. The same configuration and driver data were used for each lake to account for different sources of uncertainties in the model predictions. We used the R package LakeEnsemblR version 1.0.0. (Moore et al., 2021). The models were calibrated and validated against OLA limnological data, and further used to assess long-term trends in the thermal regime of each lake. The MyLake model, identified as the most performant for the four peri-alpine lakes based on five performance metrics calculated, was selected to develop and test our approach for long-term reconstruction that extends the simulation period beyond the instrumental one, as this model is well adapted to northern and alpine regions (Couture et al., 2018; Kobler and Schmid, 2019; Saloranta, 2006). MyLake was developed by the Norwegian Institute for Water Research (NIVA), the University of Helsinki (Finland), and Université Laval (Canada). It simulates daily vertical water temperature profiles, density stratification, seasonal ice and snow cover, sediment–water dynamics, and phosphorus–phytoplankton interactions (Saloranta and Andersen, 2007).
2.4 Climate warming scenarios
The model was forced with statistically bias-adjusted (Lange, 2019a) and downscaled climate projections (Cucchi et al., 2020; Lange, 2019b) (ISIMIP3BASD method) from phase 3b of the Inter-Sectoral Impact Model Intercomparison Project (ISIMIP3b). This was based on the output of phase 6 of the Coupled Model Intercomparison Project (CMIP6; Eyring et al., 2016). The better-performing models, providing daily data for all variables during the period of interest (1850–2100), were selected (Lange, 2019a) (GFDLESM4, IPSL-CM6-LR, UKESM1-0-LL, MPI-ESM1-2-HR, and MRI-ESM2-0; Tables S1 and S2 in the Supplement) and compared to meteorological station data (Fig. S1 in the Supplement).
All five climate models were downscaled at 0.5∘ (55 km) resolution. These models are good representatives of the entire CMIP6 ensemble, as they are characterized with low (GFDL-ESM4, MPI-ESM1-2-HR, MRI-ESM2-0) and high climate sensitivity (IPSL-CM6A-LR and UKESM1-0-LL). The aim of the study being reducing of model input variables to only those with the highest level of confidence in the long term, such as air temperature and downwelling shortwave radiation, these two variables from each model were compared to available observation data from a meteorological station located at Thonon-les-Bains (1987–2019 and 1971–2019 for air temperature and shortwave radiation, respectively) (monitoring data from the INRAE CLIMATIK platform, https://agroclim.inrae.fr/climatik/, last access: 16 June 2021, in French, managed by the AgroClim laboratory of Avignon, France). The model data were collected from the historical (1850–2014) and the intermediate (SSP370: 2015–2100) scenarios, in order to cover the entire period with available observation data. Only years with more than 350 d of data available were considered. Annual means and daily means during the monitoring periods were calculated and compared between modeled and observed data. Three error assessment metrics (root mean square error – RMSE, normalized mean absolute error – NMAE, and Pearson correlation coefficient – r) were calculated to evaluate the performance of climatic models. The most performant of the five models (IPSL-CM6A-LR) was selected and used as input data for the study.
CMIP6 experiments used were historical climates from 1850 to 2014, and scenarios SSP126 (SSP1-RCP2.6 climate), SSP370 (SSP3-RCP7 climate), and SSP585 (SSP5-RCP8.5 climate) from 2015 to 2100. The SSPs consider how societal choices will affect greenhouse emissions, SSP126 being the most sustainable scenario and SSP585 the worst one (Riahi et al., 2017). The Representative Concentration Pathways (RCPs) correspond to the range of the year 2100's radiative forcings values, from 2.6 to 8.5 W m−2 (van Vuuren et al., 2011).
2.5 Meteorological forcing data
The meteorological forcing variables required in the LakeEnsemblR package are air temperature (∘C), downwelling shortwave radiation (W m−2), 10 m elevation wind speed (m s−1), cloud cover fraction, relative humidity (%), rain (mm d−1), and surface-level barometric pressure (Pa), at a daily time step.
From the chosen climate model (IPSL-CM6-LR), all forcing variables were extracted for the grid cells containing the four lakes, lakes Bourget and Aiguebelette being situated in the same grid. A sensitivity test was carried out on all seven climate variables to validate our hypothesis that forcing variables can be reduced into the hydrodynamic models to only air temperature and shortwave radiations to identify long-term trends accurately (Table S3). Each climate forcing variable (air temperature, downwelling shortwave radiation, wind speed, cloud cover, relative humidity, rain, and surface pressure) was tested from MyLake water temperature simulations over a 10-year period for the four peri-alpine lakes. A percentage of ±20 % was added to each climate variable daily values, and then the performance metrics (RMSE and R2) were calculated from model outputs and observation data. The difference between model performance with raw data and after applying the 20 % coefficient to the input values was calculated. Variables with the most significant variation of its performance metrics were identified as variables with the most important effect on the MyLake model performance.
Then simulations with the MyLake model for the four lakes were computed with four different climatic configurations: (i) with only air temperature and shortwave radiation from ISIMIP3b while all other variables were extracted from meteorological observations from which daily means were calculated and replicated every year from 1850 to 2100 (Fig. S2); (ii) with all input meteorological forcing variables extracted from ISIMIP3b. The period of meteorological observations extends from 2000 to 2011 for Lake Geneva (MétéoSuisse Data Warehouse) and from 1959 to 2020 for the other lakes (Table 4) (SAFRAN climatic data are provided by Météo-France and were downloaded via the SICLIMA platform developed by AgroClim-INRAE). Decoupling meteorological parameters is a strong assumption, which is addressed in the Discussion section. The cloud cover was available only for Lake Geneva, and values were adopted as the same for the other lakes. Surface pressure was considered constant in this study. Configurations (iii) and (iv) are based on configurations (i) and (ii), respectively, with a correction factor for both air temperature and shortwave radiation (Table 2). These two variables were compared to available data from the closest meteorological stations (from INRAE and Meteo France networks – CLIMATIK and SAFRAN database), encompassing 32 to 61 years of meteorological time series data (Fig. 1) (Table 3). Correction factors were calculated from the difference between raw climatic model data and observed data from local stations, at the daily resolution, to fit better to meteorological data and correct the altitude bias (Figs. S3 and S4). Further, daily corrected meteorological data were used between 1850 and 2100.
Table 3Meteorological data sources used for the meteorological patterns calculated from daily means of cloud cover (Cl), relative humidity (RH), wind speed (Ws), and rain (R) variables, for the four study sites.
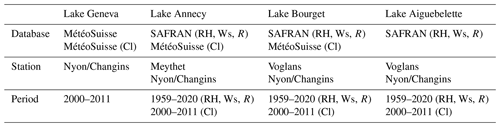
Daily meteorological data from the SAFRAN analysis system were extracted from the SICLIMA database (Bertuzzi and Clastre, 2022), collected by Meteo France since 1 January 1959. Data have been recalculated from daily local observations at smaller grid cells (8 km × 8 km) by Meteo France.
Table 4Meteorological forcing configuration and performance metrics for MyLake (ISIMIP) calculated between daily simulations and observations. Outputs comparison between four configurations: (i) only air temperature (T, ∘C) and shortwave radiation (Sw) extracted from ISIMIP3B without a correction factor, and others from meteorological observations; (ii) all ISIMIP3b forcing data with no correction factor; (iii) only air T, ∘C, and Sw extracted from ISIMIP3B with a correction factor; (iv) all ISIMIP3b forcing data with a correction factor applied to air T, ∘C, and Sw, over 10 years. Bold: best performance metrics for each lake within the four configurations (Lower RMSE, bias and MAE; Higher Pearson r and NSE).
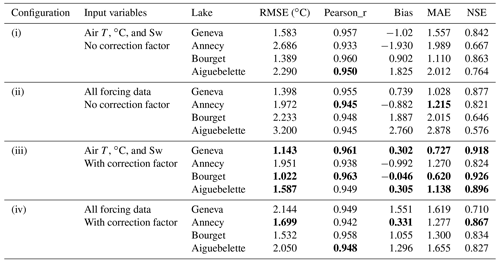
2.6 Model setup, calibration, and validation
MyLake was run through the LakeEnsemblR package. The model was calibrated considering the most sensitive parameters identified in previous studies (Saloranta, 2006) (Table S4): scaling factors for wind speed and shortwave radiation and physical C_shelter parameter. The Latin hypercube calibration (LHC) method was used for calibration. The LHC method uses upper and lower bounds for all parameters considered, and then samples evenly within the parameter space given by these bounds. Then MyLake was run and evaluated for 100 parameter sets. The model's performance was assessed through six statistical metrics: RMSE, Nash–Sutcliffe efficiency (NSE), r, bias, MAE, and NMAE.
The optimal values of model parameters were determined based on the performance metrics. First, calibration and validation were performed over 10 years, depending on each lake's density of observation data (Table S5). This period corresponds to the temporal scale generally covered by modeling studies conducted between 2015 to 2020 (Soares and Calijuri, 2021). Further, the robustness of the model to perform long-term simulations was assessed by computing the performance metrics over the entire period with field data availability, covering 63, 54, 37, and 46 years for lakes Geneva, Annecy, Bourget, Aiguebelette, respectively (Table 2).
For the long-term simulations according to the three scenarios (from 1850 to 2100), the initial water temperature remained unknown. To address this limitation, a method was developed to identify the year with available observation data the closest from climatic conditions estimated on 31 January 1850. Air temperature from 1 to 31 January 1850 was compared to air temperature of the instrumental period (from the OLA database) to identify years with similar climate conditions. The water temperature profile of the year with the lowest RMSE between winter air temperatures (1992 for lakes Bourget and Geneva, 2000 for lakes Annecy and Aiguebelette) was used as the initial profile for 31 January 1850.
2.7 Assessment of lake response to warming
The model fit was assessed for 15 thermal indices: lake temperature (complete profile); surface temperature (Ts at 5 m); bottom temperature (Tb at 60, 60, 299, and 140 m for lakes Annecy, Aiguebelette, Geneva, and Bourget, respectively); mean temperature along the water column (Tm); metalimnion depth (top and bottom) defined as the water stratum with the steepest thermal gradient, demarcated by the bottom of the epilimnion and top of the hypolimnion (Wetzel, 2001); epilimnion temperature; hypolimnion temperature; Schmidt stability (Idso, 1973); buoyancy frequency (Brunt–Vaisala frequency); thermocline depth; and stratification characteristics (start, end, duration, maximum intensity, and day of the year) (see Supplement). The R package rLakeAnalyzer (Winslow et al., 2019) was used to calculate epilimnion extent and temperature, hypolimnion extent and temperature, metalimnion upper and lower depths, Schmidt stability, buoyancy frequency, and thermocline depth. The stratification onsets/breakups dates were defined as the day when the surface-to-bottom temperature differences were greater or less than 2 ∘C (Robertson and Ragotzkie, 1990) for at least 5 consecutive days. As defined above, the stratification duration was calculated as the period between the day of onset and breakup. The maximum stratification intensity was the most significant difference between Ts and Tb. Water volumes above certain thresholds of ecological interest (7, 9, and 12 ∘C) (Pourriot and Meybeck, 1995) above which the reproduction, growth, and survival of certain fish species may be affected (Réalis-Doyelle, 2016) were calculated from bathymetry files. Finally, potential oxygen solubility was calculated as a function of water temperature following a Winkler table.
2.8 Statistical analysis
The slope of the significant trends was evaluated by least squares linear regression and t tests when followed a normal distribution. Otherwise, the non-parametric Mann–Kendall test and the Theil–Sen method were used to estimate if the slope was significant and provide the slope's value. The Shapiro–Wilk and Fisher's F tests evaluated the distribution normality and variance homoscedasticity, respectively. Average values of each thermal metric in the present (1990–2020) and future (2070–2100) were compared with either Student's t mean difference test in case of residuals normal distribution and homoscedasticity or the Welsh t mean difference test when variances were different. When the normal distribution of residuals was not followed, the Mann–Whitney U test (equal variances) or the Kolmogorov–Smirnov test (other variances) were used instead. A p value of 0.05 was used to represent the significance of the statistical tests. Kernel densities were calculated to compare averaged daily data distribution over 2000–2010 and 2090–2100. The coefficient of overlap of the two distributions was calculated (Ridout and Linkie, 2009). The analyses were carried out with the R software (version 4.1.2, R Core Team, 2021).
3.1 Model performance
The MyLake model, forced by air temperature and shortwave radiation, reproduced well the observed temperature along the water column in the four deep alpine lakes (Fig. 2). Model performances were compared across a 10-year validation and more extended periods (Table 6), depending on the availability of observations for each lake. During both validation periods (i.e., 10-year and 37 to 63-year periods), the model predicted water temperature with good precision, as RMSE values are generally less than 2 and 1.22 ∘C for the two deepest lakes (Geneva and Bourget). The model reproduced well the interannual temperature variability of the four lakes (Fig. 3) with Pearson correlation coefficient values (r) > 0.9 over the 10-year calibration and validation periods. The model robustness has been maintained over the long term as r values remained >0.9. Depending on the lake considered, the model either slightly underestimated the water temperature (bias was −0.03 to 0.05 ∘C and −0.91 to 0.99 ∘C for Lake Bourget and Annecy, respectively) or overestimated it (+0.31–+0.69 ∘C and +0.3 ∘C for lakes Aiguebelette and Geneva, respectively). These results showed that this is not a systematic tendency to over- or underestimate the water temperature, depending on the lake's characteristics.
Table 6MyLake performance indicators of water temperature simulations (root mean square error, RMSE, and Pearson correlation coefficient, r) for the calibration and validation periods (val: 10 years, lt-val: long term); nobs data: number of limnological observation data for comparison with simulated data.
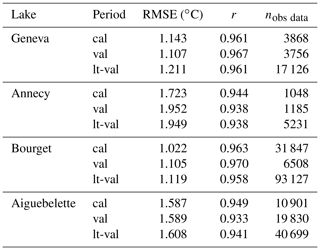
The ability of the model to predict the evolution of specific thermal indices has been assessed (Table 7). The model performance in predicting epilimnion temperatures was the best for the two deepest lakes (Geneva and Bourget), with RMSEs ranging between 1.92 and 2.08 ∘C. For Lake Annecy, the discrepancies obtained for the epilimnion simulations were similar to those of the simulated temperature profiles over the 10-year validation period (RMSE was 2.02 and 1.56 ∘C, respectively). Still, it was less effective over the long-term validation (RMSE is 3.69 ∘C). Similarly, the model was more performant in predicting the epilimnion temperature of Lake Aiguebelette during the 10-year validation period (RMSE was 2.61 ∘C) compared to the long-term validation (RMSE was 4.6 ∘C). The epilimnion interannual variability of the four lakes was well reproduced, with R2>0.89 and R2>0.65 for the 10-year and long-term validations, respectively.
Table 7Comparison of model validation metrics for eight thermal indices over 10 years and a long-term validation period (37 to 63 years) for the four lakes (see extended Table S6); bold: RMSE < 2 ∘C and R2>0.7; italics: RMSE<2.7 ∘C and R2>0.5.
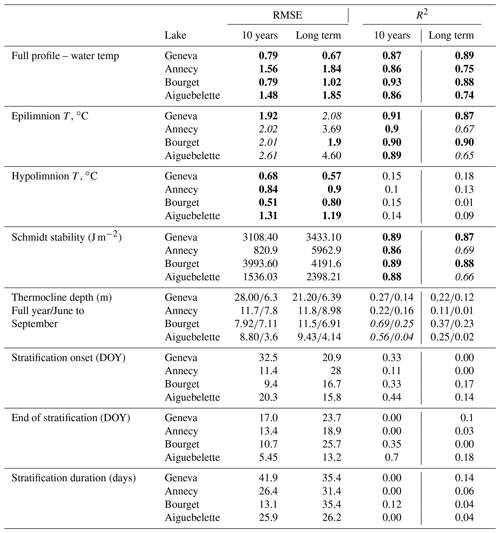
A clear difference in amplitude has been identified between Schmidt stability calculated from simulations and observed water temperature profiles (mean RMSE: 3270.7, 4092.6, 3391.9, and 1967.1 for Geneva, Bourget, Annecy, and Aiguebelette, respectively). However, general seasonal patterns across the four lakes were well simulated by the model (R2>0.86 for the 10-year and R2>0.66 for the long-term validation periods). The average model performance between the two validation periods after comparing calculations of observed and simulated thermocline depth is better for lakes Bourget and Aiguebelette (with RMSE: 9.7, 9.1, 11.7, and 24.6 m for lakes Bourget, Aiguebelette, Annecy, and Geneva, respectively). When considering only the stratified summer period, from June to September, the MyLake model was more performant to predict the thermocline depth, especially in lakes Geneva and Aiguebelette (with average RMSE: 6.4, 3.9, 7, and 8.4 m for lakes Geneva, Aiguebelette, Bourget, and Annecy, respectively). The RMSE associated with the prediction of the onset stratification was lower for lakes Bourget and Aiguebelette over the long-term validation period (RMSE: 12.9 and 15.8 d, respectively) and less accurate for lakes Annecy and Geneva (RMSE: 28.0 and 20.9 d, respectively). The estimation of the end-of-stratification date was reasonably close for lakes Bourget and Annecy (10.7 < RMSE < 13.4 d and 15.2 < RMSE < 18.9 d for 10-year and long-term validation periods, respectively). The model predicted better the end of stratification for Lake Aiguebelette (RMSE: d) compared to Lake Geneva (RMSE: d). Moreover, the stratification duration was represented more accurately for Lake Bourget (RMSE: 13.09 and 17.7 d for the 10-year and long-term validation periods, respectively) and ranged between 1 month and 1.5 months for the other lakes (25.9 < RMSE < 41.9 d).
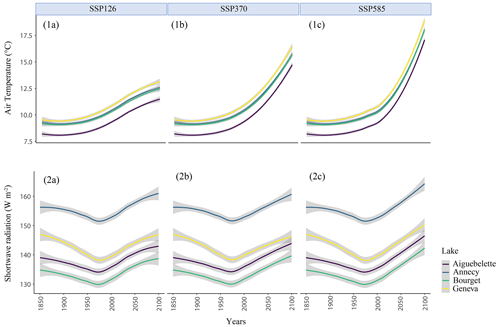
Figure 4Selected meteorological forcing variables over the studied period of 1850–2100. Projected evolution of air temperature (1) and shortwave radiation (2) from IPSL-CM6A-LR (ISIMIP3b) under SSP126 (a), SSP370 (b), and SSP585 (c) for the four lakes (Geneva, Annecy, Bourget, Aiguebelette) (loess statistical method used).
3.2 Meteorological trends
Based on the different scenarios adopted in the present work, mean annual air temperature has increased by +0.39 ∘C (Lake Bourget) and +0.5 ∘C (Lake Geneva) per decade over the past 30 years (from 1990 to 2020) (Fig. 4). At the Horizon 2100 (from 2070 to 2100), on the one hand, the mean annual air temperature with the SSP126 scenario decreased on average by −0.008 ∘C per decade across all lakes. On the other hand, with the SSP370 and SSP585 scenarios, an average increase of +0.9 and +1.13 ∘ C per decade were predicted, respectively. Comparing the average annual air temperature between the two periods, increases of +1.69, +4.04, and +5.81 ∘C are predicted according to SSP126, SSP370, and SSP585 scenarios, respectively. Mean annual shortwave radiations have increased on average by +1.58 W m−2 per decade from 1990 to 2020, and are expected to decrease by −1.5 W m−2 per decade according to the most optimistic scenario (SSP126). According to the most optimistic scenario (SSP126), an increase of +6.21 W m−2 is expected at the Horizon 2100, unlike the most pessimistic scenario (SSP585) which predicted an increase of +7.66 W m−2.
3.3 Lakes' response to the meteorological scenarios
3.3.1 Water temperature
The epilimnion temperature increased by around 0.44 ∘C per decade (Lake Aiguebelette) to 0.48 ∘C per decade (Lake Geneva) over the past 30 years (1990–2020). In the future projections (2070–2100), according to the most optimistic scenario (SSP126), a decrease of −0.07 to −0.08 ∘C per decade could be expected (Fig. 5). The intermediate scenario (SSP370) predicted a significant increase of +0.77 ∘C per decade (Lake Annecy) to +0.89 ∘C per decade (Lake Geneva). In the worst-case scenario, the epilimnion temperature in the four lakes could increase by +1.03 ∘C per decade (Lake Annecy) to +1.13 ∘C per decade (Lake Geneva). In all cases, the model predicted a significant change in epilimnion temperature over the two periods.
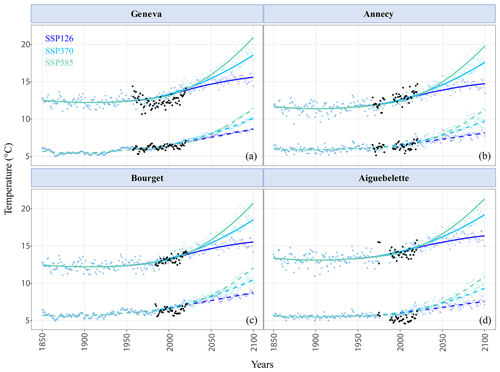
Figure 5Annual averages of epilimnion (line) and hypolimnion (dashed lines) temperatures from MyLake daily water temperature simulations over the period 1850–2100, for three different climate scenarios (SSP126, SSP370, SSP585) in lakes Geneva (a), Annecy (b), Bourget (c), and Aiguebelette (d). Black dots represent annual averages of observation data.
Over the last 30 years, hypolimnion temperature increased by +0.29, +0.31, +0.32, and +0.39 ∘C per decade in lakes Aiguebelette, Geneva, Annecy, and Bourget, respectively. The rate of increase was higher for surface layers than for deep hypolimnetic layers. The difference between the epilimnion and hypolimnion warming rates was more prominent for Lake Geneva. A less significant increase in hypolimnion temperature could be expected at the Horizon 2100 according to the SSP126 scenario, with +0.19 ∘C per decade (Lake Geneva) to +0.3 ∘C per decade (Lake Bourget). Unlike the epilimnion, even the most optimistic scenario predicted an increase in deep layers. In the four lakes, a significant rise in +0.55 ∘C per decade (Lake Annecy) to +0.73 ∘C per decade (Lake Bourget) was expected in the case of the intermediate scenario. The SSP585 scenario predicted an increase by +0.67 ∘C per decade (Lake Annecy) to +0.82 ∘C per decade (Lake Geneva).
The water temperature change was quantified as the non-overlapped area of the two daily averaged temperature distributions in the present (2000–2010) and the future (2090–2100) as a percentage of the combined area of those distributions for the intermediate scenario (SSP370) (Fig. 6). The greatest thermal change occurred in Lake Geneva and Bourget with 90 % and 86 % non-overlap, respectively, between the two periods. In Lake Annecy and Aiguebelette, 77 % and 76 % thermal non-overlap were predicted, respectively.
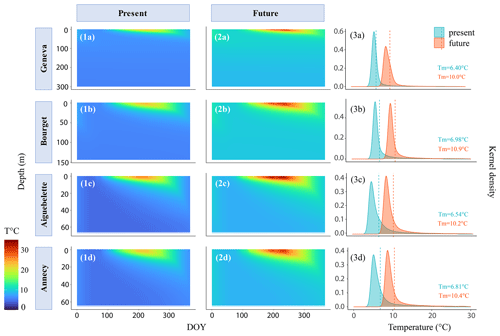
Figure 6MyLake water temperature simulations from intermediate climate scenario (SSP370). Daily averaged water temperature over the periods 2000–2010 (1) and 2090–2100 (2) in Lake Geneva (a), Bourget (b), Aiguebelette (c), and Annecy (d). Estimated frequency of daily average temperature data (3) over the present (2000–2010) and the future (2090–2100) using kernel density. The dashed lines represent average annual temperatures over the entire periods.
The annual average temperature was expected to increase between the two periods on average by +3.59, +3.60, +3.66, and +3.92 ∘C in Lake Annecy, Geneva, Aiguebelette, and Bourget, respectively.
3.3.2 Stratification characteristics
Schmidt stability, describing the stability of the water column and its resistance to mixing, has significantly increased over the past 30 years by an annual average of +174.6, +522.97, +654.57, and +1753.5 J m−2 per decade for Lake Annecy, Aiguebelette, Bourget, and Geneva, respectively (Fig. 7). No significant trend was predicted by the model at the Horizon 2100 for the SSP126 scenario in Lake Geneva and Bourget. For Lake Annecy and Aiguebelette, a significant decrease of −100.5 and −281.3 J m−2 per decade could be expected according to that optimistic scenario, respectively. The evolutions predicted by the model in the case of the intermediate scenario varied between the four lakes, with an increase of +285.5 J m−2 per decade in Lake Annecy, +927.8 J m−2 per decade in Aiguebelette, +1088.7 J m−2 per decade in Bourget, and +3435 J m−2 per decade in Lake Geneva. In the worst-case scenario (SSP585), an increase of +445 J m−2 per decade (Lake Annecy) to +4695 J m−2 per decade (Lake Geneva) could be expected. For the four lakes, in any scenario, a significant evolution was predicted by the model between the last 30 years and the future (2070–2100), however with different annual averages from one lake to another (Schmidt stability is 2646 and 23 042 J m−2 for Lake Annecy and Geneva, respectively) (Table S7).
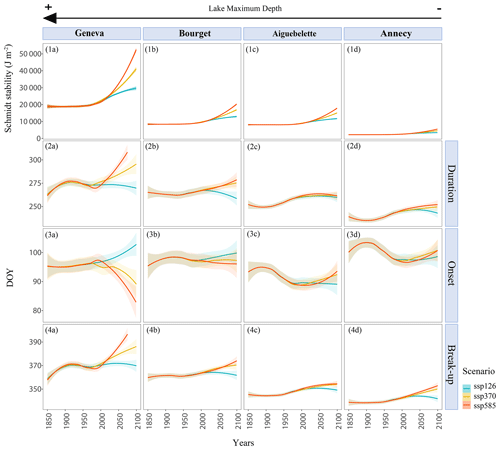
Figure 7Stratification trend characteristics for the four lakes – Geneva (a), Bourget (b), Aiguebelette (c), and Annecy (d). Estimations of Schmidt stability (1) from June to September, stratification duration (2), onset (3), and breakup (4) over the period 1850–2100 for the three scenarios (SSP126, SSP370, and SSP585), calculated from MyLake water temperature simulations.
No significant trend was identified for the day of onset of stratification (DOY) in the present and the future, except for Lake Annecy according to SSP126 scenario (DOY: +1.6 and +4.3 d per decade for present and future periods, respectively) (Fig. 7). For Lake Geneva, the onset of stratification occurred significantly earlier in the case of SSP585 by 11 d on average (DOY: 96 and 85 in the present and the future, respectively), contrary to the SSP126 scenario, which predicted a significant delay of 5 d later (DOY: 96 to 101 for present and future periods, respectively). No significant change in stratification onset has been identified for Lake Annecy, Bourget, and Aiguebelette. When comparing the average days of stratification onset between the last 30 years and at the Horizon 2100, a significant difference appeared only for Lake Geneva according to SSP126 and SSP585 scenarios. Indeed, a 6 d delay was predicted by the model in the most optimistic scenario against an 11 d advance in the most pessimistic scenario.
Regarding the break up of stratification, a significant trend was only predicted for Lake Geneva in the present (1990–2020) with an average of 5 d per decade later. Except for Lake Annecy and Aiguebelette SSP126 and Lake Bourget SSP370, the end of stratification appeared significantly later in the future than in the last 30 years. In the SSP126 scenario, the stratification could end on average 6 and 5 d earlier for Lake Geneva and Bourget, respectively. According to the SSP370 scenario, it was predicted to end on average 5.7, 7.4, and 3 d later in Lake Annecy, Geneva, and Aiguebelette, respectively. In the worst-case scenario, with a delay of 7.7, 14.7, 6.8, and 3.6 d in Lake Annecy, Geneva, Bourget, and Aiguebelette, respectively.
As a result, a significant increase in the average stratification duration was predicted in Lake Geneva – SSP585 of +21.2 d (DOYpresent=278.3 and DOYfuture=299.5). In the most optimistic scenario (SSP126), a significant decrease could be expected in Lake Geneva and Bourget (−8.8 and −9.45 d, respectively) between the two periods. The model for Lake Annecy and Aiguebelette predicted no significant difference. The average duration of stratification within the four lakes was from 246 d (Lake Annecy) to 279 d (Lake Geneva) for the present period. In the worst-case scenario, the duration could last from 251 d (Lake Annecy) to 300 d (Lake Geneva).
3.3.3 Water volumes: habitat
Changes in thermal habitat between the present (2000–2010) and the future (2090–2100) were assessed based on the lake volume fraction that exceeded specific temperature thresholds (>7, >9, and >12 ∘C) (Fig. 8), above which the reproduction, growth, and survival of certain fish species may be affected (Réalis-Doyelle, 2016). These lake volume fractions were calculated from the daily average water temperature simulated by the MyLake model and the bathymetry file. Annual averages have been calculated from these daily data, and were then quantified as the non-overlapped area of the two lake volume fraction distributions (present and future based on the three different scenarios). Kernel density allowed to compare both lake volume fractions and the number of associated days. In lakes Geneva and Bourget, the temperature was predicted to exceed 7 ∘C in all case scenarios, with a lake volume fraction non-overlap of 100 % between the present (2000–2010) and the future (2090–2100). In Lake Annecy, 77.8 % of the water column could reach a temperature greater than 7 ∘C in the SSP126 case scenario and extend to the entire water column in the two other scenarios, with a 100 % non-overlap between present and future projections. In Lake Aiguebelette, the lake volume fraction with the temperature above 7 ∘C has increased from 15 % (present) to 87 % (SSP370 and SSP585), involving a lake volume fraction non-overlap of 49 % (SSP126) and 100 % (SSP370 and SSP585). In all lakes, a significant increase of water volume above 7 ∘C was predicted by the model for the three scenarios (Figs. S5 and S6).
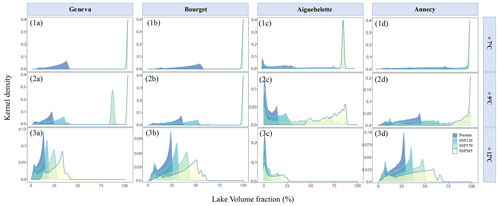
Figure 8Lake volume fraction with temperature exceeding three characteristics' thresholds – 7 ∘C (1), 9 ∘C (2), and 12 ∘C (3) – calculated from simulated water temperature profile and bathymetry in Lake Geneva (a), Bourget (b), Aiguebelette (c), and Annecy (d). Kernel density estimates of daily average lake volume fraction over the present (2000–2010) and the future (2090–2100) predicted by the three scenarios (SSP126, SSP370, and SSP585).
In Lake Annecy, Geneva, and Bourget, temperature could exceed 9 ∘C according to the intermediate scenario (annual average lake volume fraction is 70 %, 84 %, and 100 %, respectively). According to the SSP585 scenario, temperatures of almost the entire lakes could reach 9 ∘C (annual average lake volume fraction is 96 %–100 %). The specific bathymetry of Lake Aiguebelette caused a progressive increase of lake volume fraction with the temperature above 9 ∘C, yet never reached the total volume (72.5 % of the lake for SSP585). According to the SSP126 scenario, lake volume fraction with the temperature above 9 ∘C non-overlap was 35 %, 47 %, 61 %, and 62 % for lakes Aiguebelette, Annecy, Bourget, and Geneva, respectively. The increase was more important for the two larger and deeper lakes. The model predicted a significant change between the present and the three future scenarios. The thermal overlaps between the present and the future, according to SSP585 scenarios were predicted to reach 100 % for all four lakes. This means that the average daily lake volume fraction with the temperature above 9 ∘C was expected to be completely different between the two periods, with higher values in the future. A 100 % increase was also predicted in the SSP370 case scenario for Lake Geneva and Bourget, against 69 % and 75 % for lakes Aiguebelette and Annecy, respectively.
Water volumes above 12 ∘C have increased on average by +45 % (SSP126) for lakes Annecy, Geneva, and Bourget, with a slightly smaller increase in Lake Aiguebelette (+30 % of lake volume fraction non-overlapped). The model predicted the same increase in the SSP370 case scenario for the two deepest lakes (Geneva and Bourget) with on average +70 % of lake volume fraction non-overlap. In Lake Aiguebelette and Annecy, +53 % and +34 % could be expected, respectively. Finally, in the worst-case scenario (SSP585), a higher increase in water volume above 12 ∘C was predicted for Lake Geneva (+91 % of non-overlap), followed by Lake Bourget (+82 % of non-overlap), Lake Annecy (+70 % of non-overlap), and Lake Aiguebelette (+64 % of thermal non-overlap).
3.3.4 Oxygen solubility
Oxygen solubility was calculated from the Winkler tables, as a function of temperature. In all scenarios, the model for the four lakes predicted significant trends in potential oxygen solubility. Over the last 30 years (1990–2020), an average annual decrease of −0.07, −0.09, −0.12, and −0.13 mg L−1 per decade was computed for Lake Geneva, Aiguebelette, Annecy, and Bourget, respectively (Fig. 9). At the Horizon 2100 (2070–2100), according to the SSP126 scenario, a decrease of −0.05 mg L−1 per decade (lakes Geneva and Aiguebelette) to −0.09 mg L−1 per decade (Lake Bourget) could be expected. A significant decrease of −0.16 mg L−1 per decade (Lake Geneva) to −0.21 mg L−1 per decade (lakes Bourget and Aiguebelette) was predicted in the intermediate scenario and from −0.17 mg L−1 per decade (Lake Annecy) to −0.23 mg L−1 per decade (Lake Bourget) in the SSP585 scenario. In all cases, a significant difference has been forecast between present and future periods within the four lakes. An annual average of 12.15, 12.21, 12.22, and 12.3 mg L−1 was calculated for the current period from the model simulations in lakes Bourget, Annecy, Geneva, and Aiguebelette, respectively. In the future, predicted by the intermediate scenario, annual averages from 11.28 mg L−1 (Lake Bourget) to 11.49 mg L−1 (Lake Aiguebelette) could be expected.
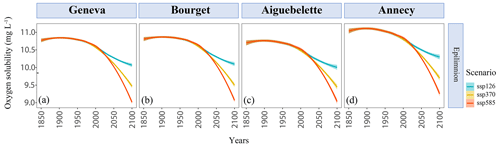
Figure 9Annual averages of potential oxygen solubility over the period 1850–2100, in the epilimnion calculated from MyLake daily water temperature simulations for three different climate scenarios (SSP126, SSP370, SSP585) in lakes Geneva (a), Bourget (b), Aiguebelette (c), and Annecy (d).
Furthermore, the model predicted a faster decrease in oxygen solubility in the epilimnion compared to the hypolimnion in the four lakes over the last 30 years, with an average of −0.104 and −0.096 mg L−1 per decade in the epilimnion and the hypolimnion, respectively. No significant trend was forecast for oxygen solubility in the epilimnion for all lakes according to the SSP126 scenario, resulting in the stabilization of oxygen solubility. In the SSP370 case scenario, the model predicted a decrease in oxygen solubility in the epilimnion of −0.16, −0.17, and −0.18 mg L−1 per decade in Lake Annecy, Aiguebelette, and both Geneva and Bourget, respectively. According to the worst scenario (SSP585), oxygen solubility in epilimnion was expected to decrease by an average of −0.2 mg L−1 per decade (±0.01 mg L−1 per decade). Oxygen solubility in deep layers is expected to be closely related to its evolution into the epilimnion, depending on the intensity and depth of the water column mixing.
Potential change in oxygen solubility was also quantified as the non-overlapped area of the two daily averaged oxygen solubility distributions in the present (2000–2010) and the future (2090–2100) as a percentage of the combined area of those distributions for the intermediate scenario (SSP370) (Fig. 10). As for the thermal regime, the highest changes in oxygen solubility were in lakes Geneva and Bourget with 60 % of non-overlap between the present and the future. In lakes Aiguebelette and Annecy, the non-overlap rate has been reduced to 55 % and 54 %.
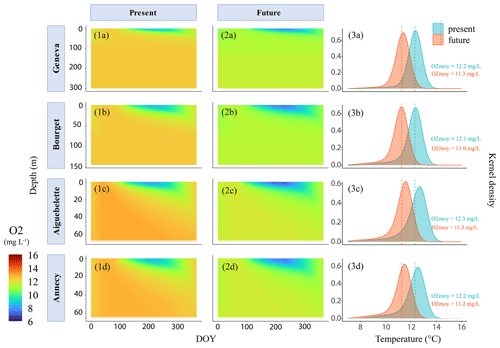
Figure 10Potential oxygen solubility in lake waters of the four lakes, calculated from MyLake water temperature simulations for the intermediate climate scenario (SSP370). Daily averaged oxygen solubility over the present (1) and future (2) in lakes Geneva (a), Bourget (b), Aiguebelette (c), and Annecy (d). Estimated frequency of daily average oxygen solubility (3) over the present (2000–2010) and future (2090–2100) using kernel density. The dashed lines represent the annual average oxygen solubility over the entire period.
Annual potential average oxygen solubility was predicted to decrease by −0.9 mg L−1 (Lake Geneva) to −1.1 mg L−1 (Lake Bourget) in the future.
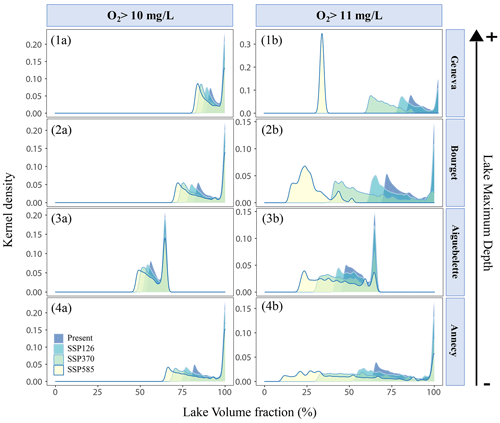
Figure 11Lake volume fraction with oxygen solubility exceeding two thresholds – 10 mg L−1 (a) and 11 mg L−1 (b) – calculated from simulated water temperature profiles and bathymetry in Lake Geneva (1), Bourget (2), Aiguebelette (3) and Annecy (4). Kernel density estimates of daily average lake volume fraction over the present (2000–2010) and the future (2090–2100) predicted by the three scenarios (SSP126, SSP370, and SSP585).
As for the thermal habitat, water volumes with sufficient dissolved oxygen levels to support fish survival were assessed based on lake volume fraction that exceeded certain thresholds (10 and 11 mg L−1) (Fig. 11). The differences between the present (2000–2010) and the future (2090–2100) according to the three scenarios were then quantified as the non-overlapped area of the two lake volume fraction distributions. Kernel density allowed us to compare the evolution of potential oxygen solubility over a whole year between the present and the future. The non-overlap areas quantification between these two periods was a good way to represent the difference in potential oxygen solubility and the number of days associated during 1 year. At present, an average of 95.6 % (±1.6 %) of the total lake volume had a potential oxygen solubility > 10 mg L−1 in lakes Annecy, Geneva, and Bourget, unlike Lake Aiguebelette with only 61.2 % of the lake volume. Averages of 25.8 % (±3.2 %), 40.5 % (±3.5 %), and 48.3 % (±5.3 %) of lake volume fraction non-overlap were predicted in SSP126, SSP370, and SSP585 scenarios, respectively. In the worst-case scenario, lake volume fractions were expected to be reduced to 85.9 %, 92 %, 86.8 %, and 57.7 % for lakes Annecy, Geneva, Bourget, and Aiguebelette, respectively.
In the two deepest lakes (Geneva and Bourget), the lake volume fraction with potential oxygen solubility above 11 mg L−1 has decreased gradually according to the three scenarios, and reached 100 % of non-overlap between the present and the future (SSP585). The decrease was less sharp for lakes Annecy and Aiguebelette, with 72 % and 68 % of non-overlap in the SSP585 scenario, respectively. However, in the intermediate scenario, 68.7 % (Geneva), 67.7 % (Annecy), 61.6 % (Bourget), and 48.6 % (Aiguebelette) of the total lake volume could have potential dissolved oxygen levels greater than 11 mg L−1. In the worst-case scenario, these lake volume fractions could be reduced to 52.3 %, 41.3 %, 33.2 %, and 26.8 % in lakes Annecy, Aiguebelette, Geneva, and Bourget.
4.1 Large peri-alpine lakes' warming
Most lakes in the world tend to warm due to climate change, with a mean increase of +0.34 ∘C per decade in their surface water temperatures during summer between 1985 and 2009 (O'Reilly et al., 2015). Our study shows that large peri-alpine lakes tend to warm at fast rates, with a mean epilimnetic temperature increase of +0.46 ∘C per decade in the case of lakes Geneva, Bourget, Aiguebelette, and Annecy. These rates of change in surface water temperature are congruent with those found for other peri-alpine lakes over the period 1975–2015 (Ficker et al., 2017). Moreover, it is consistent with the average increase in air temperature (from 0.39 to 0.5 ∘C per decade), indicating a direct response in lake temperature trends, as expected. The epilimnion of our studied lakes experienced higher warming rates than in the hypolimnion. This observation is in accordance with the expected increasing density gradients, leading to a deeper stratification that isolates deep layers from wind energy, reduces vertical mixing, and prevents hypolimnetic water temperatures from increasing (Fernández Castro et al., 2021). Further, deep lakes' dynamic of deep-water temperature is linked with the extent and frequency of total winter mixing (Livingstone and Dokulil, 2001) and inter-total mixing periods, characterized by a regular increase in deep water temperature.
In the future, simulated water temperatures will show different responses to global warming under different projections. In the most optimistic scenario, where strong environmental and political measures would be implemented, surface water temperatures are expected to decrease, whereas hypolimnetic water is expected to warm. The mechanistic effect of surface cooling in the most optimistic scenario over 1970–2100 is explained by the future development of both air temperature and solar radiation predicted to decrease. The recent past surface warming trend is not expected to persist in other large deep lakes in Central Europe (Schmid and Köster, 2016). However, hypolimnion warming could be explained by the thermal inertia and heat accumulation in deep waters (Crossman et al., 2016). In the intermediate (SSP370) and most pessimistic (SSP585) scenarios, both epilimnion and hypolimnion temperatures are increasing, driven by higher rates of air temperature warming in comparison to the recent past warming, with higher increases in the two deepest lakes (Geneva and Bourget) and faster warmings of the surface layers than deep layers. Similarly, the entire water column of the two shallowest lakes (Annecy and Aiguebelette) could warm up less quickly than the two deepest ones. This might be explained by less frequent complete mixing in deeper lakes, leading to longer inter-mixing periods during which water temperature increases. However, by 2100, there is a high probability that the hydrological regime in the Rhône River upstream Lake Geneva will change because of earlier snow melting leading to an earlier, and maybe shorter, input of cold water into the lake. The effects of hydrological changes on the thermal regime of large deep lakes are expected to be relatively modest, such as observed in preliminary sensitivity tests (results not shown here). However, these interactions would require further investigation, especially in lakes supplied by an upstream snow and glacier area.
4.2 Sensitivity of peri-alpine lakes to climate change
The four peri-alpine lakes share the same climate region and, due to their large size, they also share the same topographical properties to varying extents. Despite similarities, the four studied lakes tend to respond differently to climate change. For instance, the highest increase of Schmidt stability was predicted in Lake Geneva according to SSP370 and SSP585 scenarios, Lake Annecy being the least sensitive. These results were consistent, as changes in stability vary by lake archetype, with larger increases in deeper and more turbid lakes (Butcher et al., 2015). Regardless of the climate scenario, an increase in the stratification intensity and duration with depth is expected in the four lakes. Accordingly, Lake Geneva was predicted to experience the highest changes in the start, end, and duration of stratification, with a significant advance in stratification onset, later end of stratification, and longer stratification duration. This is consistent with its greater resistance to mixing. Still, it also has the particularity to mix over the whole water column in particular years under specific meteorological conditions (last complete mixing of its water column was in 2012). Thus, Lake Geneva must be sensitive to the frequency of the complete water column mixing, which should be investigated in more detail in upcoming studies. Conversely, the shallowest lake (Annecy) was predicted to stratify the latest with the shortest duration in all scenarios. This analysis showed that Lake Geneva will be more vulnerable to global warming in future projections than Lake Annecy, with a depth-related vulnerability gradient. These results are coherent with the differential warming of the surface and deep waters, which could influence the strength and duration of a lake's stratification period (Råman Vinnå et al., 2021). Finally, in the four lakes, the stratification duration should last longer with an early spring and a gradual delay of cold temperatures in autumn. The changes in the beginning, end, and period of stratification could also impact oxygenation conditions in deep waters (Roberts et al., 2009b), in addition to the oxygen solubility decreasing when water temperature increases. But all these results should be considered with caution, as the wind exposure is very different within the four lakes (with greater exposure for Lake Geneva) and could counteract the increase in thermal stratification (Butcher et al., 2015).
In all three scenarios, the entire water column in the four lakes would exceed 7 ∘C, even in the deepest lakes such as Geneva. Likewise, the four lakes could reach a temperature above 9 ∘C according to the intermediate and most pessimistic scenarios. This exceeding of temperature thresholds can impair the development and reproduction of some iconic fish species, leading to important issues for lake managers and fishers. Previous studies have demonstrated that Arctic charr (Salvelinus alpinus), an emblematic fish of peri-alpine lakes (Caudron et al., 2014), has a limited thermal tolerance range compared with other salmonids (Baroudy and Elliott, 1994; Elliott and Elliott, 2010), and hatch survival decreased significantly as the water temperature at spawning increased from 5 to 8.5 ∘C (Mari et al., 2016). In autumn, the water temperature must fall below 7 ∘C for Arctic charr's endocrine phenomena to occur and trigger ovulation and spermiation (Gillet et al., 2011). Similarly, female ovulation and male spermiation are completely blocked when the water temperature exceeds 10 ∘C, and embryonic development is impacted up to total embryo mortality when the temperature exceeds 12 ∘C (Guillard et al., 1992). Similar deleterious effects have been observed for whitefish (Coregonus sp.), which require a water temperature drop below 7 ∘C to stimulate the onset of spawning (Anneville et al., 2013). Furthermore, trout survival has been shown to decrease with an increase of +4 ∘C in the water temperature (Réalis-Doyelle, 2016), and the whitefish population, the main targeted fish in peri-alpine lakes (Anneville et al., 2017), will suffer from increased temperature (Gerdeaux, 2004; Eckmann, 2013).
Once again, the two deepest lakes (Geneva and Bourget) were the most sensitive to climate change, with the most important changes in water volumes exceeding the temperature thresholds considered, especially in the worst-case scenario (SSP585). However, when focusing on the lake volume fraction exceeding these characteristic temperature thresholds, without considering the associated duration, Lake Annecy appeared to be the most sensitive, with almost 75 % of the lake above 12 ∘C in the most pessimistic scenario.
4.3 Implications on oxy-thermal habitats
Dissolved oxygen (DO) is one of the most fundamental variables in lake systems (Wetzel, 2001). Dissolved oxygen depends on several variables, such as oxygen solubility and hydrometeorological conditions (Rajwa-Kuligiewicz et al., 2014), but also the intensity of biological processes such as photosynthesis, respiration, and decomposition of organic matter.
Jane et al. (2021) found that a decline in dissolved oxygen is widespread in surface water habitats, primarily associated with reduced solubility under warmer water temperatures. Here we investigate changes in oxygen solubility, i.e., the oxygen concentration in solution in equilibrium with the oxygen pressure in a gas phase, as a function of temperature.
In the four peri-alpine lakes, oxygen solubility as a function of water temperature has decreased in both surface and deep waters over the last 30 years, with differences in amplitude. Water warming explained average rates of changes of −0.1 mg L−1 per decade in oxygen solubility over that period, which are similar to those observed in temperate lakes (Jane et al., 2021). Lake Geneva and Bourget would face the most significant decrease in oxygen solubility, consistent with previous observations on thermal changes. Moreover, a faster decrease in the epilimnion has been identified, which was correlated to a higher increase in surface temperature, and did not consider the negative effect of shorter and less deep mixing periods on DO in hypolimnion.
Despite the decrease in oxygen solubility being a direct inference of the increase in water temperature, our approach allows us to quantitatively assess oxy-thermal habitat changes in a global warming context relative to the long-term evolution of the water temperature. Our analysis provides a broader context for climate change impacts on lake physics that goes further than water warming, but also has implications in terms of oxy-thermal habitat changes that may increase the likelihood of community disruptions due to the disappearance of habitat over their suitable thermal ranges (Kraemer et al., 2021). The oxy-thermal stress substantially impacts the fish population's renewal, as it can substantially increase the yellow perch extirpation (Magee et al., 2018). However, they do not integrate oxygen consumption and production by photosynthesis or lateral flow paths and production in littoral zones which can be important for large and deep lake ecosystems. It would need to be adjusted with studies dealing with ecosystem oxygen production and consumption in the pelagic zone.
Finally, the effect of decreased oxygen solubility on fish habitats was assessed through some potential thresholds. The model predicted faster decreases of the lake volume fraction with oxygen solubility > 11 mg L−1 in the two deepest lakes (Geneva and Bourget), according to the three scenarios. For instance, the decrease in oxygen solubility can reduce the habitat for cold water fish, which could face warmer water temperatures and lower dissolved oxygen concentrations (Mohseni et al., 2003). Further research using fish habitat simulation models, such as TDO3 (Jiang et al., 2012), would be necessary to investigate whether the oxy-thermal conditions predicted by the three different scenarios would allow fish to survive in the four studied lakes.
4.4 Model reliability and limitations of the approach for the long term
The approach developed in this study, which consisted of reducing the number of forcing variables to only air temperature and shortwave radiation, was defined based on the variables with the highest confidence level of the long-term predictions. This assumption is in line with the importance of both variables in warming trends in a peri-alpine lake, which were the main driving variables responding with 60 % and 40 %, respectively, of the increasing temperature trend, while all other meteorological variables showed small to negligible effects, which was assumed as representative for the majority of large deep lakes in Central Europe following a dimictic or monomictic regime (Schmid and Köster, 2016). Furthermore, it seems well adapted to long-term simulation approaches, with potential implications for paleolimnological studies, long-term forecast studies, or studies focused on the effects of climate change on lake ecological dynamics. The model errors for the long term were relatively small for the four study sites, with RMSE < 2 ∘C during 10-year calibration and validation periods, with even better performance for the two deepest lakes (Geneva and Bourget). This could be explained by a more stable and stronger stratification in the deepest lakes with greater density gradients that would increase the model accuracy. This seems consistent, as it has been shown that in deeper lakes (>40 m) the General Lake Model (GLM) predicts hypolimnion temperature with greater accuracy when surface mixing dynamics have less influence on the deep layers' temperature (Bruce et al., 2018). Further, hypolimnion temperature was simulated with better precision in lakes Geneva and Bourget compared to the two shallower lakes, just like the epilimnion temperature and Schmidt stability. Nevertheless, the interannual variabilities were not well captured by the model. As for the thermocline depth, it was more difficult to predict with the model in lakes Geneva, Annecy, and Aiguebelette, yet good results were observed for Lake Bourget. These uncertainties could be partly caused by the presence of internal seiches, which increased the variability and made it difficult to reproduce by 1D models (Ayala et al., 2020).
Thus, applying only air temperature and downwelling shortwave radiation from climatic projections provided a well-adapted model to the study of the four peri-alpine lakes in the long term, even with considering only the seasonal trends in wind speed, cloud cover, air relative humidity, and rainfall. In this sense, the approach seems adequate for long-term simulation approaches. No systematic error has been identified, as the model slightly underestimated (Lake Bourget) or overestimated (Lake Geneva) the water temperature, depending on the lake morphology. Positive biases could be attributed to the measurements carried out around midday and in good weather, whereas model outputs were averaged daily (Vinçon-Leite et al., 2014). Similarly, biases of estimations of the start, end, and duration of stratification may be attributed to the low frequency (i.e., bi-monthly) of limnological measurements, which do not allow precise estimations on the stratification periods. Besides this limitation, good performances for the Schmidt stability index suggest that the model could be used to assess the long-term variations in the stratification regime, while the duration still needs to be carefully interpreted.
This method allowed overcoming certain limitations such as the quality of the input files related to the climate variables scaling. For instance, the wind can vary within the same 0.5∘ grid, depending on the surrounding terrain. The influence of rivers and watersheds may also be difficult to simulate in the long term. Reducing the number of forcing variables to only air temperature and shortwave radiations is an efficient approach to analyzing thermal regime and oxygen solubility trends in the long term, as more and more studies are dedicated to forecast and exploring future scenarios. However, there is a need to assess changes in the past that 1D modeling approaches could address, including hindcast and paleolimnological studies. Indeed, several applications to link paleoenvironmental data and models have been identified, such as model validation, synthesis of research findings, and support of paleoecological data interpretation (Anderson et al., 2006). There is a need for models to produce physical and biogeochemical scenarios over the following years, decades, and centuries (Anderson et al., 2006). Paleoenvironmental data usually represent the only means for driving and testing simulation models in lakes where instrumental data are available only for short timescales (annual or pluri-annual).
A further limitation of this method is related to the correlation between the different climate variables, such as relative humidity and air temperature dependency. As the meteorological patterns replicated the seasonal fluctuations of each variable, this error is limited. Another possible option would have been to use a weather generator to simulate climate variables' evolutions, implemented to integrate these correlations.
Finally, the GLM model, integrating the energy balance for surface mixing and the diffusive transport below thermocline (Hipsey et al., 2019), simulated the water temperature in lakes Geneva and Bourget with quite good precision, but was less performant for lakes Annecy and Aiguebelette. Simstrat has well reproduced the temperature along the water column in Lake Geneva and quite well in Lake Bourget, but was much less efficient at simulating water temperature in the two shallowest lakes, Annecy and Aiguebelette.
In this study, an approach to simulate long-term trends in lake thermal regime and oxygen solubility has been tested and validated against 63 years of limnological data from the OLA lakes observatory. The approach shows that 1D thermal lake models perform well when run only with air temperatures and shortwave radiations as forcing variables, hence allowing to overcome certain limitations related to the quality of climate input data for the long term. Future application of the 1D model approach for long-term variations can be anticipated in paleolimnology, but also to assess past and future effects of climate change on the ecological dynamics and lake habitats.
Simulations show that over the last 30 years, epilimnion temperature has increased on average by +0.46 ∘C per decade in the four lakes. At the Horizon 2100, simulations anticipate a minimum increase of +0.77 and +0.56 ∘C per decade in epi- and hypolimnion, respectively (Lake Annecy – SSP370) to a maximum of +1.13 ∘C per decade (epilimnion) and +0.82 ∘C per decade (hypolimnion) (Lake Geneva – SSP585). The response to climate change varies between lakes, with the deepest lakes (Bourget and Geneva) experiencing the fastest warming, with an average of +3.92 and +3.6 ∘C at the Horizon 2100.
Regarding oxygen condition, a decrease in oxygen solubility occurred over the last 30 years, at least in lakes Annecy and Bourget, with −0.12 mg L−1 and −0.13 mg L−1 per decade, respectively. At the Horizon 2100, simulations indicate that lakes Bourget and Geneva will face the most significant decrease of oxygen solubility, with −0.21 mg L−1 per decade according to the intermediate scenario, which may alter the chemical and ecological functioning of the lakes. Simulations of the duration and intensity of thermal stratification suggest that the decrease in lake oxygen conditions will be more pronounced in the case of Lake Geneva and that Lake Annecy would be the least sensitive to climate change.
Code and data used in this paper are available from the corresponding author upon a reasonable request.
The supplement related to this article is available online at: https://doi.org/10.5194/hess-27-837-2023-supplement.
JPJ, OD, DB, PAD, and BVL conceived and designed the study. OD and LS collected the data. OD performed the analyses and calculations. All authors actively took part in the interpretation of the results. OD wrote the original draft of the paper, and all the authors reviewed it. JPJ acquired the funding and guided the research.
The contact author has declared that none of the authors has any competing interests.
Publisher's note: Copernicus Publications remains neutral with regard to jurisdictional claims in published maps and institutional affiliations.
We thank the HESS editor, the reviewers, and the participants of the lively discussion in HESSD for their comments that helped to improve the paper. We gratefully acknowledge the financial support from the ANR C-ARCHIVES and the Pole ECLA (French Research Organization in Lake Ecology). We would like to thank the French Observatory of LAkes of the platform Analyses et Expérimentations pour les Ecosystèmes (OLA-ANAEE) for providing the long-term limnological data, and Victor Frossard from CARRTEL for thorough advances on the paper conception. We also thank Jean-Christophe Clément from CARRTEL for his proofreading.
This research has been supported by the French National Agency ANR C-ARCHIVES (grant no. 5283) and the Pole ECLA Ecosystèmes lacustres of the Office Français de la Biodiversité (OFB).
This paper was edited by Matthew Hipsey and reviewed by two anonymous referees.
Anderson, N. J., Bugmann, H., Dearing, J. A., and Gaillard, M.-J.: Linking palaeoenvironmental data and models to understand the past and to predict the future, Trends Ecol. Evol., 21, 696–704, https://doi.org/10.1016/j.tree.2006.09.005, 2006.
Angilletta, M. J. and Dunham, A. E.: The temperature-size rule in ectotherms: simple evolutionary explanations may not be general, Am. Nat., 162, 332–342, https://doi.org/10.1086/377187, 2003.
Anneville, O., Beniston, M., Gallina, N., Gillet, C., Jacquet, S., and Lazzarotto, J.: L'empreinte du changement climatique sur le Léman, Arch. Sci., 16, 157–172, 2013.
Ayala, A. I., Moras, S., and Pierson, D. C.: Simulations of future changes in thermal structure of Lake Erken: proof of concept for ISIMIP2b lake sector local simulation strategy, Hydrol. Earth Syst. Sci., 24, 3311–3330, https://doi.org/10.5194/hess-24-3311-2020, 2020.
Balsamo, G., Salgado, R., Dutra, E., Boussetta, S., Stockdale, T., and Potes, M.: On the contribution of lakes in predicting near-surface temperature in a global weather forecasting model, Tellus A, 64, 15829, https://doi.org/10.3402/tellusa.v64i0.15829, 2012.
Baroudy, E. and Elliott, J. M.: Racial differences in eggs and juveniles of Windermere charr, Salvelinus alpinus, J. Fish Biol., 45, 407–415, https://doi.org/10.1111/j.1095-8649.1994.tb01323.x, 1994.
Bertuzzi, P. and Clastre, P.: Information sur les mailles SAFRAN, Recherche Data Gouv, V2, https://doi.org/10.57745/1PDFNL, 2022.
Bruce, L. C., Frassl, M. A., Arhonditsis, G. B., Gal, G., Hamilton, D. P., Hanson, P. C., Hetherington, A. L., Melack, J. M., Read, J. S., Rinke, K., Rigosi, A., Trolle, D., Winslow, L., Adrian, R., Ayala, A. I., Bocaniov, S. A., Boehrer, B., Boon, C., Brookes, J. D., Bueche, T., Busch, B. D., Copetti, D., Cortés, A., de Eyto, E., Elliott, J. A., Gallina, N., Gilboa, Y., Guyennon, N., Huang, L., Kerimoglu, O., Lenters, J. D., MacIntyre, S., Makler-Pick, V., McBride, C. G., Moreira, S., Özkundakci, D., Pilotti, M., Rueda, F. J., Rusak, J. A., Samal, N. R., Schmid, M., Shatwell, T., Snorthheim, C., Soulignac, F., Valerio, G., van der Linden, L., Vetter, M., Vinçon-Leite, B., Wang, J., Weber, M., Wickramaratne, C., Woolway, R. I., Yao, H., and Hipsey, M. R.: A multi-lake comparative analysis of the General Lake Model (GLM): Stress-testing across a global observatory network, Environ. Model. Softw., 102, 274–291, https://doi.org/10.1016/j.envsoft.2017.11.016, 2018.
Butcher, J. B., Nover, D., Johnson, T. E., and Clark, C. M.: Sensitivity of lake thermal and mixing dynamics to climate change, Climatic Change, 129, 295–305, https://doi.org/10.1007/s10584-015-1326-1, 2015.
Caudron, A., Lasne, E., Gillet, C., Guillard, J., and Champigneulle, A.: Thirty years of reoligotrophication do not contribute to restore self-sustaining fisheries of Arctic charr, Salvelinus alpinus, in Lake Geneva, Fish. Res., 154, 165–171, https://doi.org/10.1016/j.fishres.2014.01.023, 2014.
Couture, R.-M., Moe, S. J., Lin, Y., Kaste, Ø., Haande, S., and Lyche Solheim, A.: Simulating water quality and ecological status of Lake Vansjø, Norway, under land-use and climate change by linking process-oriented models with a Bayesian network, Sci. Total Environ., 621, 713–724, https://doi.org/10.1016/j.scitotenv.2017.11.303, 2018.
Crossman, J., Eimers, M. C., Kerr, J. G., and Yao, H.: Sensitivity of physical lake processes to climate change within a large Precambrian Shield catchment, Hydrol. Process., 30, 4353–4366, https://doi.org/10.1002/hyp.10915, 2016.
Cucchi, M., Weedon, G. P., Amici, A., Bellouin, N., Lange, S., Müller Schmied, H., Hersbach, H., and Buontempo, C.: WFDE5: bias-adjusted ERA5 reanalysis data for impact studies, Earth Syst. Sci. Data, 12, 2097–2120, https://doi.org/10.5194/essd-12-2097-2020, 2020.
Danis, P.-A., Von Grafenstein, U., Masson-Delmotte, V., Planton, S., Gerdeaux, D., and Moisselin, J.-M.: Vulnerability of two European lakes in response to future climatic changes, Geophys. Res. Lett., 31, L21507, https://doi.org/10.1029/2004GL020833, 2004.
Daufresne, M., Lengfellner, K., and Sommer, U.: Global warming benefits the small in aquatic ecosystems, P. Natl. Acad. Sci. USA, 106, 12788–12793, https://doi.org/10.1073/pnas.0902080106, 2009.
Eckmann, R.: A review of the population dynamics of coregonids in European alpine lakes, Adv. Limnol., 64, 3–24, https://doi.org/10.1127/1612-166X/2013/0064-0002, 2013.
Elliott, J. M. and Elliott, J. A.: Temperature requirements of Atlantic salmon Salmo salar, brown trout Salmo trutta and Arctic charr Salvelinus alpinus: predicting the effects of climate change, J. Fish Biol., 77, 1793–1817, https://doi.org/10.1111/j.1095-8649.2010.02762.x, 2010.
Eyring, V., Bony, S., Meehl, G. A., Senior, C. A., Stevens, B., Stouffer, R. J., and Taylor, K. E.: Overview of the Coupled Model Intercomparison Project Phase 6 (CMIP6) experimental design and organization, Geosci. Model Dev., 9, 1937–1958, https://doi.org/10.5194/gmd-9-1937-2016, 2016.
Fernández Castro, B., Bouffard, D., Troy, C., Ulloa, H. N., Piccolroaz, S., Sepúlveda Steiner, O., Chmiel, H. E., Serra Moncadas, L., Lavanchy, S., and Wüest, A.: Seasonality modulates wind-driven mixing pathways in a large lake, Commun. Earth Environ., 2, 1–11, https://doi.org/10.1038/s43247-021-00288-3, 2021.
Ficker, H., Luger, M., and Gassner, H.: From dimictic to monomictic: Empirical evidence of thermal regime transitions in three deep alpine lakes in Austria induced by climate change, Freshwater Biol., 62, 1335–1345, https://doi.org/10.1111/fwb.12946, 2017.
Gerdeaux, D.: The recent restoration of the whitefish fisheries in Lake Geneva: The roles of stocking, reoligotrophication, and climate change, Ann. Zool. Fenn., 41, 181–189, 2004.
Gillet, C., Breton, B., Mikolajczyk, T., Bodinier, P., and Fostier, A.: Disruption of the secretion and action of 17,20β-dihydroxy-4-pregnen-3-one in response to a rise in temperature in the Arctic charr, Salvelinus alpinus. Consequences on oocyte maturation and ovulation, Gen. Comp. Endocrinol., 172, 392–399, https://doi.org/10.1016/j.ygcen.2011.04.002, 2011.
Guillard, J., Gillet, C., and Champigneulle, A.: Revue bibliographique – Principales caractéristiques de l'élevage de l'omble chevalier (Salvelinus alpinus L.) en eau douce, Bull. Fr. Pêche Piscic., 325, 47–68, https://doi.org/10.1051/kmae:1992014, 1992.
Hamilton, D. P. and Schladow, S. G.: Prediction of water quality in lakes and reservoirs. Part I – Model description, Ecol. Model., 96, 91–110, https://doi.org/10.1016/S0304-3800(96)00062-2, 1997.
Hipsey, M. R., Bruce, L. C., Boon, C., Busch, B., Carey, C. C., Hamilton, D. P., Hanson, P. C., Read, J. S., de Sousa, E., Weber, M., and Winslow, L. A.: A General Lake Model (GLM 3.0) for linking with high-frequency sensor data from the Global Lake Ecological Observatory Network (GLEON), Geosci. Model Dev., 12, 473–523, https://doi.org/10.5194/gmd-12-473-2019, 2019.
Idso, S. B.: On the concept of lake stability1, Limnol. Oceanogr., 18, 681–683, https://doi.org/10.4319/lo.1973.18.4.0681, 1973.
Jane, S. F., Hansen, G. J. A., Kraemer, B. M., Leavitt, P. R., Mincer, J. L., North, R. L., Pilla, R. M., Stetler, J. T., Williamson, C. E., Woolway, R. I., Arvola, L., Chandra, S., DeGasperi, C. L., Diemer, L., Dunalska, J., Erina, O., Flaim, G., Grossart, H.-P., Hambright, K. D., Hein, C., Hejzlar, J., Janus, L. L., Jenny, J.-P., Jones, J. R., Knoll, L. B., Leoni, B., Mackay, E., Matsuzaki, S.-I. S., McBride, C., Müller-Navarra, D. C., Paterson, A. M., Pierson, D., Rogora, M., Rusak, J. A., Sadro, S., Saulnier-Talbot, E., Schmid, M., Sommaruga, R., Thiery, W., Verburg, P., Weathers, K. C., Weyhenmeyer, G. A., Yokota, K., and Rose, K. C.: Widespread deoxygenation of temperate lakes, Nature, 594, 66–70, https://doi.org/10.1038/s41586-021-03550-y, 2021.
Jenny, J.-P., Anneville, O., Arnaud, F., Baulaz, Y., Bouffard, D., Domaizon, I., Bocaniov, S. A., Chèvre, N., Dittrich, M., Dorioz, J.-M., Dunlop, E. S., Dur, G., Guillard, J., Guinaldo, T., Jacquet, S., Jamoneau, A., Jawed, Z., Jeppesen, E., Krantzberg, G., Lenters, J., Leoni, B., Meybeck, M., Nava, V., Nõges, T., Nõges, P., Patelli, M., Pebbles, V., Perga, M.-E., Rasconi, S., Ruetz, C. R., Rudstam, L., Salmaso, N., Sapna, S., Straile, D., Tammeorg, O., Twiss, M. R., Uzarski, D. G., Ventelä, A.-M., Vincent, W. F., Wilhelm, S. W., Wängberg, S.-Å., and Weyhenmeyer, G. A.: Scientists' Warning to Humanity: Rapid degradation of the world's large lakes, J. Gt. Lakes Res., 46, 686–702, https://doi.org/10.1016/j.jglr.2020.05.006, 2020.
Jiang, L., Fang, X., Stefan, H. G., Jacobson, P. C., and Pereira, D. L.: Oxythermal habitat parameters and identifying cisco refuge lakes in Minnesota under future climate scenarios using variable benchmark periods, Ecol. Model., 232, 14–27, https://doi.org/10.1016/j.ecolmodel.2012.02.014, 2012.
Kobler, U. G. and Schmid, M.: Ensemble modelling of ice cover for a reservoir affected by pumped-storage operation and climate change, Hydrol. Process., 33, 2676–2690, https://doi.org/10.1002/hyp.13519, 2019.
Kraemer, B. M., Pilla, R. M., Woolway, R. I., Anneville, O., Ban, S., Colom-Montero, W., Devlin, S. P., Dokulil, M. T., Gaiser, E. E., Hambright, K. D., Hessen, D. O., Higgins, S. N., Jöhnk, K. D., Keller, W., Knoll, L. B., Leavitt, P. R., Lepori, F., Luger, M. S., Maberly, S. C., Müller-Navarra, D. C., Paterson, A. M., Pierson, D. C., Richardson, D. C., Rogora, M., Rusak, J. A., Sadro, S., Salmaso, N., Schmid, M., Silow, E. A., Sommaruga, R., Stelzer, J. A. A., Straile, D., Thiery, W., Timofeyev, M. A., Verburg, P., Weyhenmeyer, G. A., and Adrian, R.: Climate change drives widespread shifts in lake thermal habitat, Nat. Clim. Change, 11, 521–529, https://doi.org/10.1038/s41558-021-01060-3, 2021.
Lange, S.: Trend-preserving bias adjustment and statistical downscaling with ISIMIP3BASD (v1.0), Geosci. Model Dev., 12, 3055–3070, https://doi.org/10.5194/gmd-12-3055-2019, 2019a.
Lange, S.: WFDE5 over land merged with ERA5 over the ocean (W5E5) (1.0), PIK – Potsdam Institute For Climate Impact Reseach, https://doi.org/10.5880/PIK.2019.023, 2019b.
Livingstone, D. M.: Impact of Secular Climate Change on the Thermal Structure of a Large Temperate Central European Lake, Climatic Change, 57, 205–225, https://doi.org/10.1023/A:1022119503144, 2003.
Livingstone, D. M. and Dokulil, M. T.: Eighty years of spatially coherent Austrian lake surface temperatures and their relationship to regional air temperature and the North Atlantic Oscillation, Limnol. Oceanogr., 46, 1220–1227, https://doi.org/10.4319/lo.2001.46.5.1220, 2001.
Magee, M. R., McIntyre, P. B., and Wu, C. H.: Modeling oxythermal stress for cool-water fishes in lakes using a cumulative dosage approach, Can. J. Fish. Aquat. Sci., 75, 1303–1312, https://doi.org/10.1139/cjfas-2017-0260, 2018.
Mari, L., Garaud, L., Evanno, G., and Lasne, E.: Higher temperature exacerbates the impact of sediments on embryo performances in a salmonid, Biol. Lett., 12, 20160745, https://doi.org/10.1098/rsbl.2016.0745, 2016.
Mohseni, O., Stefan, H. G., and Eaton, J. G.: Global Warming and Potential Changes in Fish Habitat in U.S. Streams, Climatic Change, 59, 389–409, https://doi.org/10.1023/A:1024847723344, 2003.
Moore, T. N., Mesman, J. P., Ladwig, R., Feldbauer, J., Olsson, F., Pilla, R. M., Shatwell, T., Venkiteswaran, J. J., Delany, A. D., Dugan, H., Rose, K. C., and Read, J. S.: LakeEnsemblR: An R package that facilitates ensemble modelling of lakes, Environ. Model. Softw., 143, 105101, https://doi.org/10.1016/j.envsoft.2021.105101, 2021.
O'Reilly, C. M., Sharma, S., Gray, D. K., Hampton, S. E., Read, J. S., Rowley, R. J., Schneider, P., Lenters, J. D., McIntyre, P. B., Kraemer, B. M., Weyhenmeyer, G. A., Straile, D., Dong, B., Adrian, R., Allan, M. G., Anneville, O., Arvola, L., Austin, J., Bailey, J. L., Baron, J. S., Brookes, J. D., de Eyto, E., Dokulil, M. T., Hamilton, D. P., Havens, K., Hetherington, A. L., Higgins, S. N., Hook, S., Izmest'eva, L. R., Joehnk, K. D., Kangur, K., Kasprzak, P., Kumagai, M., Kuusisto, E., Leshkevich, G., Livingstone, D. M., MacIntyre, S., May, L., Melack, J. M., Mueller-Navarra, D. C., Naumenko, M., Noges, P., Noges, T., North, R. P., Plisnier, P.-D., Rigosi, A., Rimmer, A., Rogora, M., Rudstam, L. G., Rusak, J. A., Salmaso, N., Samal, N. R., Schindler, D. E., Schladow, S. G., Schmid, M., Schmidt, S. R., Silow, E., Soylu, M. E., Teubner, K., Verburg, P., Voutilainen, A., Watkinson, A., Williamson, C. E., and Zhang, G.: Rapid and highly variable warming of lake surface waters around the globe, Geophys. Res. Lett., 42, 10773–10781, https://doi.org/10.1002/2015GL066235, 2015.
Parmesan, C.: Ecological and evolutionary responses to recent climate change, Annu. Rev. Ecol. Evol. Syst., 37, 637–669, https://doi.org/10.1146/annurev.ecolsys.37.091305.110100, 2006.
Perga, M.-E., Bruel, R., Rodriguez, L., Guénand, Y., and Bouffard, D.: Storm impacts on alpine lakes: Antecedent weather conditions matter more than the event intensity, Global Change Biol., 24, 5004–5016, https://doi.org/10.1111/gcb.14384, 2018.
Piccioni, F., Casenave, C., Lemaire, B. J., Le Moigne, P., Dubois, P., and Vinçon-Leite, B.: The thermal response of small and shallow lakes to climate change: new insights from 3D hindcast modelling, Earth Syst. Dynam., 12, 439–456, https://doi.org/10.5194/esd-12-439-2021, 2021.
Piccolroaz, S., Toffolon, M., and Majone, B.: A simple lumped model to convert air temperature into surface water temperature in lakes, Hydrol. Earth Syst. Sci., 17, 3323–3338, https://doi.org/10.5194/hess-17-3323-2013, 2013.
Pourriot, R. and Meybeck, M.: Limnologie générale, in: Collection d'écologie 25, Masson, Paris, p. 956, ISBN 2-225-84687-1, 1995.
Rajwa-Kuligiewicz, A., Rowiński, P., Bialik, R., and Karpiński, M.: Stream diurnal profiles of dissolved oxygen – case studies, in: Proc. 3rd IAHR Europe Congress, Porto, Portugal, ISBN 978-989-96479-2-3, 2014.
Råman Vinnå, L., Wüest, A., and Bouffard, D.: Physical effects of thermal pollution in lakes, Water Resour. Res., 53, 3968–3987, https://doi.org/10.1002/2016WR019686, 2017.
Råman Vinnå, L., Wüest, A., Zappa, M., Fink, G., and Bouffard, D.: Tributaries affect the thermal response of lakes to climate change, Hydrol. Earth Syst. Sci., 22, 31–51, https://doi.org/10.5194/hess-22-31-2018, 2018.
Råman Vinnå, L., Medhaug, I., Schmid, M., and Bouffard, D.: The vulnerability of lakes to climate change along an altitudinal gradient, Commun. Earth Environ., 2, 35, https://doi.org/10.1038/s43247-021-00106-w, 2021.
R Core Team: R: A language and environment for statistical computing, R Foundation for Statistical Computing, https://www.R-project.org/ (last access: 14 February 2023), 2021.
Réalis-Doyelle, E.: Influence de la température sur les premiers stades de vie de trois espèces de poissons dulcicoles: étude de la survie et de la plasticité phénotypique, Phd thesis, Université de Lorraine, https://theses.hal.science/tel-01540861/document (last access: 13 February 2023), 2016.
Riahi, K., van Vuuren, D. P., Kriegler, E., Edmonds, J., O'Neill, B. C., Fujimori, S., Bauer, N., Calvin, K., Dellink, R., Fricko, O., Lutz, W., Popp, A., Cuaresma, J. C., Kc, S., Leimbach, M., Jiang, L., Kram, T., Rao, S., Emmerling, J., Ebi, K., Hasegawa, T., Havlik, P., Humpenöder, F., Da Silva, L. A., Smith, S., Stehfest, E., Bosetti, V., Eom, J., Gernaat, D., Masui, T., Rogelj, J., Strefler, J., Drouet, L., Krey, V., Luderer, G., Harmsen, M., Takahashi, K., Baumstark, L., Doelman, J. C., Kainuma, M., Klimont, Z., Marangoni, G., Lotze-Campen, H., Obersteiner, M., Tabeau, A., and Tavoni, M.: The Shared Socioeconomic Pathways and their energy, land use, and greenhouse gas emissions implications: An overview, Global Environ. Change, 42, 153–168, https://doi.org/10.1016/j.gloenvcha.2016.05.009, 2017.
Ridout, M. S. and Linkie, M.: Estimating overlap of daily activity patterns from camera trap data, J. Agric. Biol. Environ. Stat., 14, 322–337, https://doi.org/10.1198/jabes.2009.08038, 2009.
Rimet, F., Anneville, O., Barbet, D., Chardon, C., Crépin, L., Domaizon, I., Dorioz, J.-M., Espinat, L., Frossard, V., Guillard, J., Goulon, C., Hamelet, V., Hustache, J.-C., Jacquet, S., Lainé, L., Montuelle, B., Perney, P., Quetin, P., Rasconi, S., and Monet, G.: The Observatory on LAkes (OLA) database: Sixty years of environmental data accessible to the public, J. Limnol., 79, 164–178, https://doi.org/10.4081/jlimnol.2020.1944, 2020.
Roberts, J. J., Höök, T. O., Ludsin, S. A., Pothoven, S. A., Vanderploeg, H. A., and Brandt, S. B.: Effects of hypolimnetic hypoxia on foraging and distributions of Lake Erie yellow perch, J. Exp. Mar. Biol. Ecol., 381, S132–S142, 2009a.
Roberts, J. J., Höök, T. O., Ludsin, S. A., Pothoven, S. A., Vanderploeg, H. A., and Brandt, S. B.: Effects of hypolimnetic hypoxia on foraging and distributions of Lake Erie yellow perch, J. Exp. Mar. Biol. Ecol., 381, S132–S142, https://doi.org/10.1016/j.jembe.2009.07.017, 2009b.
Robertson, D. and Ragotzkie, R.: Changes in the thermal structure of moderate to large sized lakes in response to changes in air temperature, Aquat. Sci., 52, 360–380, https://doi.org/10.1007/BF00879763, 1990.
Sadeghian, A., Hudson, J., and Lindenschmidt, K.-E.: Effects of quality controlled measured and re-analysed meteorological data on the performance of water temperature simulations, Hydrolog. Sci. J., 67, 21–39, https://doi.org/10.1080/02626667.2021.1994975, 2021.
Saloranta, T. M.: Highlighting the model code selection and application process in policy-relevant water quality modelling, Ecol. Model., 194, 316–327, https://doi.org/10.1016/j.ecolmodel.2005.10.031, 2006.
Saloranta, T. M. and Andersen, T.: MyLake – A multi-year lake simulation model code suitable for uncertainty and sensitivity analysis simulations, Ecol. Model., 207, 45–60, https://doi.org/10.1016/j.ecolmodel.2007.03.018, 2007.
Schmid, M. and Köster, O.: Excess warming of a Central European lake driven by solar brightening, Water Resour. Res., 52, 8103–8116, https://doi.org/10.1002/2016WR018651, 2016.
Shatwell, T., Thiery, W., and Kirillin, G.: Future projections of temperature and mixing regime of European temperate lakes, Hydrol. Earth Syst. Sci., 23, 1533–1551, https://doi.org/10.5194/hess-23-1533-2019, 2019.
Snortheim, C. A., Hanson, P. C., McMahon, K. D., Read, J. S., Carey, C. C., and Dugan, H. A.: Meteorological drivers of hypolimnetic anoxia in a eutrophic, north temperate lake, Ecol. Model., 343, 39–53, https://doi.org/10.1016/j.ecolmodel.2016.10.014, 2017.
Soares, L. M. V. and Calijuri, M. do C.: Deterministic modelling of freshwater lakes and reservoirs: Current trends and recent progress, Environ. Model. Softw., 144, 105143, https://doi.org/10.1016/j.envsoft.2021.105143, 2021.
Trolle, D., Hamilton, D. P., Hipsey, M. R., Bolding, K., Bruggeman, J., Mooij, W. M., Janse, J. H., Nielsen, A., Jeppesen, E., Elliott, J. A., Makler-Pick, V., Petzoldt, T., Rinke, K., Flindt, M. R., Arhonditsis, G. B., Gal, G., Bjerring, R., Tominaga, K., Hoen, J., Downing, A. S., Marques, D. M., Fragoso, C. R., Søndergaard, M., and Hanson, P. C.: A community-based framework for aquatic ecosystem models, Hydrobiologia, 683, 25–34, https://doi.org/10.1007/s10750-011-0957-0, 2012.
van Vuuren, D. P., Edmonds, J., Kainuma, M., Riahi, K., Thomson, A., Hibbard, K., Hurtt, G. C., Kram, T., Krey, V., Lamarque, J.-F., Masui, T., Meinshausen, M., Nakicenovic, N., Smith, S. J., and Rose, S. K.: The representative concentration pathways: an overview, Climatic Change, 109, 5–31, https://doi.org/10.1007/s10584-011-0148-z, 2011.
Vinçon-Leite, B., Lemaire, B. J., Khac, V. T., and Tassin, B.: Long-term temperature evolution in a deep sub-alpine lake, Lake Bourget, France: how a one-dimensional model improves its trend assessment, Hydrobiologia, 731, 49–64, https://doi.org/10.1007/s10750-014-1818-4, 2014.
Walther, G. R., Post, E., Convey, P., Menzel, A., Parmesan, C., Beebee, T. J. C., Fromentin, J. M., Hoegh-Guldberg, O., and Bairlein, F.: Ecological responses to recent climate change, Nature, 416, 389–395, https://doi.org/10.1038/416389a, 2002.
Wetzel, R. G.: Limnology: Lake and River Ecosystems, Academic Press, 1024 pp., eBook ISBN 9780080574394, Hardcover ISBN 9780127447605, 2001.
Williamson, C. E., Saros, J. E., Vincent, W. F., and Smol, J. P.: Lakes and reservoirs as sentinels, integrators, and regulators of climate change, Limnol. Oceanogr., 54, 2273–2282, https://doi.org/10.4319/lo.2009.54.6_part_2.2273, 2009.
Winslow, L., Read, J., Woolway, R., Brentrup, J., Leach, T., Zwart, J., Albers, S., and Collinge, D.: rLakeAnalyzer: Lake Physics Tools, https://cran.r-project.org/web/packages/rLakeAnalyzer/rLakeAnalyzer.pdf (last access: 13 February 2023), 2019.
Woolway, R. I. and Merchant, C. J.: Worldwide alteration of lake mixing regimes in response to climate change, Nat. Geosci., 12, 271–276, https://doi.org/10.1038/s41561-019-0322-x, 2019.
Woolway, R. I., Sharma, S., Weyhenmeyer, G. A., Debolskiy, A., Golub, M., Mercado-Bettín, D., Perroud, M., Stepanenko, V., Tan, Z., Grant, L., Ladwig, R., Mesman, J., Moore, T. N., Shatwell, T., Vanderkelen, I., Austin, J. A., DeGasperi, C. L., Dokulil, M., La Fuente, S., Mackay, E. B., Schladow, S. G., Watanabe, S., Marcé, R., Pierson, D. C., Thiery, W., and Jennings, E.: Phenological shifts in lake stratification under climate change, Nat. Commun., 12, 2318, https://doi.org/10.1038/s41467-021-22657-4, 2021.
The requested paper has a corresponding corrigendum published. Please read the corrigendum first before downloading the article.
- Article
(7155 KB) - Full-text XML
- Corrigendum
-
Supplement
(951 KB) - BibTeX
- EndNote