the Creative Commons Attribution 4.0 License.
the Creative Commons Attribution 4.0 License.
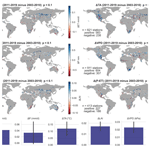
Global dryland aridity changes indicated by atmospheric, hydrological, and vegetation observations at meteorological stations
Haiyang Shi
Geping Luo
Olaf Hellwich
Xiufeng He
Alishir Kurban
Philippe De Maeyer
Tim Van de Voorde
In the context of global warming, an increase in atmospheric aridity and global dryland expansion under the future climate has been expected in previous studies. However, this conflicts with observed greening over drylands and the insignificant increase in hydrological and ecological aridity from the ecohydrology perspective. Combining climatic, hydrological, and vegetation data, this study evaluated global dryland aridity changes at meteorological stations from 2003 to 2019. A decoupling between atmospheric, hydrological, and vegetation aridity was found. Atmospheric aridity represented by the vapor pressure deficit (VPD) increased, hydrological aridity indicated by machine-learning-based precipitation minus evapotranspiration (P − ET) data did not change significantly, and ecological aridity represented by the leaf area index (LAI) decreased. P − ET showed nonsignificant changes in most of the dominant combinations of the VPD, LAI, and P − ET. This study highlights the added value of using station-scale data to assess dryland change as a complement to results based on coarse-resolution reanalysis data and land surface models.
- Article
(2531 KB) - Full-text XML
- BibTeX
- EndNote
Drylands are defined as regions with a dry climate, limited water, and scarce vegetation (Berg and McColl, 2021). In the context of global warming, the global dryland is expected to expand due to potential higher atmospheric water demand. This will severely affect the relevant ecosystem functions and livelihoods in drylands (Reynolds et al., 2007; Yao et al., 2020; Prăvălie, 2016). To date, there are still major limitations in the consensual knowledge and consistent understanding of global dryland aridity changes, such as wet–dry changes; the location, magnitude, and persistence of the potential dryland expansion; and associated mechanisms (Berg and McColl, 2021; Lian et al., 2021; Huang et al., 2016, 2017; Grünzweig et al., 2022; Pan et al., 2021). Such knowledge gaps have substantially limited effective climate adaptation and related strategy development to realize the Sustainable Development Goals in drylands, especially in the “Global South” (Li et al., 2021; Fu et al., 2021; Yao et al., 2021; Ramón Vallejo et al., 2012).
The difficulty involved with the current investigation of dryland change lies in its multifaceted nature, including the diverse characteristics of climate, hydrology, and ecosystems. Thus, the indicators and methods used to assess changes in drylands are diverse, and previous studies have obtained different findings (Lian et al., 2021) on dryland change. Typically, the aridity index (AI) (Middleton and Thomas, 1997), calculated as the multiyear average precipitation (P) divided by potential evaporation (PET), has commonly been used to measure atmospheric aridity in long-term global dryland change measuring studies (Huang et al., 2017, 2016). It uses only atmospheric inputs, focuses only on atmospheric aridity, and does not consider the effects of ecohydrological aridity nor the influence of land surface processes (Berg and McColl, 2021). AI-based studies have found global dryland expansions in the past and future (Huang et al., 2017, 2016) in a global warming context. However, such AI-based findings appear to be contrary to the global greening of dryland vegetation based on satellite remote-sensing observations (Fensholt et al., 2012; Poulter et al., 2014; Lian et al., 2021; Hickler et al., 2005; Zhu et al., 2016). This illustrates the necessity to incorporate changes in surface properties, such as vegetation, in addition to atmospheric indicators. Therefore, from an ecohydrological perspective, recent studies have employed various ecohydrological indicators and land surface property changes such as soil moisture, vegetation greenness, evapotranspiration (ET), P − ET (i.e., P minus ET as surface water availability), and runoff to assess dryland change (Berg and McColl, 2021; Lian et al., 2021; Denissen et al., 2022; Yang et al., 2018; Milly and Dunne, 2016; He et al., 2019). Such recent studies have shown that the dryland changes demonstrated by land surface changes and ecohydrological indicators did not confirm the “expansion of drylands” reported by previous atmospheric-indicator-based studies (Huang et al., 2016, 2017; Feng and Fu, 2013). In terms of the mechanistic explanation, these studies claimed that atmospheric drying and vegetation greening may occur simultaneously and that an elevated vapor pressure deficit (VPD) does not fully propagate into surface changes to exacerbate decreases in soil moisture and runoff. Under elevated atmospheric CO2, plant stomata may close and reduce transpiration and ET, thereby improving water use efficiency (WUE) (Lian et al., 2021; Berg and McColl, 2021; Roderick et al., 2015), which may compensate for the negative effects of an elevated VPD on vegetation growth. This mechanism was not accounted for in the physically based estimates of PET (e.g., the Penman–Monteith equation); thus, AI-based findings may have overestimated the aridity and contained considerable uncertainty.
However, the data used in most of the abovementioned approaches have large uncertainties, such as coarse transpiration/soil moisture data (0.5∘ × 0.5∘ resolution) from long-term climate and land surface model simulations (Berg and McColl, 2021) and coarse soil moisture/ET data (0.25∘ × 0.25∘ resolution) from the Global Land Evaporation Amsterdam Model (GLEAM) or the Global Land Data Assimilation System (GLDAS), which are not necessarily applicable to the assessment of dryland expansion at fine scales. In addition, it is difficult to validate the findings in such coarse-resolution studies with ground observations. Thus, it is essential to make better use of station-scale data, which may have the potential to measure dryland change at a finer scale, be better combined with ground observations, and provide more effective climate change adaptation suggestions for local communities.
Therefore, with the aim of reducing scale-related uncertainty and obtaining a comprehensive finding of multifaceted characteristics, this study investigated dryland change at the meteorological station scale using a combination of atmospheric, hydrological, and vegetation condition observations, including the VPD, P − ET, and the leaf area index (LAI). The VPD and P are from meteorological observations, and LAI is from MODIS imagery. ET is estimated by a random forest (RF) model trained using dryland flux stations from FLUXNET2015, and the data-driven methods can avoid uncertainties caused by physically based ET models. At the station scale, this study provides new insights into global dryland aridity change using multifaceted data with a higher proportion of observations.
We produced ET data for global dryland meteorological stations using an RF model trained from FLUXNET2015 dryland flux station (AI < 0.65) data. We selected daily ET observations (i.e., latent heat observations) from the FLUXNET2015 dataset for stations in drylands as the target variable. The selected predictor variables included downward shortwave radiation (RSDN), air temperature (TA), daily variance (half-hourly daily maximum temperature minus daily minimum temperature, TArange), VPD, wind speed (WS), and the LAI from remote sensing (Table 1).
The RF model was constructed using the RandomForestRegressor function from the scikit-learn package of Python. The “n_estimators” parameter was set to 500, and default parameter values were used for the other parameters (Zhao et al., 2019). For the evaluation of model performance, we used a leave-one-station-out cross-validation approach, as employed in previous studies of ET predictions (Tramontana et al., 2016; Zhang et al., 2021; Shi et al., 2022). This is a type of cross-validation approach in which each station's observation is considered to be the validation set and the remaining stations' observations are considered to be the training set. It can help us understand the potential adaptability of the model to new data in the prediction set. Feature importance (IMP) was used to measure the contributions of predictors, and we adopted the permutation importance indices to represent IMP due to their reliability (Díaz-Uriarte and Alvarez de Andrés, 2006; Strobl et al., 2008; Grömping, 2009; Zhang et al., 2021) in RF models.
Finally, the constructed RF model was applied to the global dryland meteorological stations in the Global Surface Summary of the Day (GSOD) dataset. In this way, daily-scale ET time series data were predicted for each meteorological station. For each station, when the number of predicted daily ET records for a given year exceeded 100, the annual ET mean was calculated using the arithmetic mean of the daily ET values. Given the absence of data such as the LAI during the winter snowpack at a small number of arid-zone stations, this approach allows for an effective, dense sampling of growing season days to represent annual ET and distinguish between high and low annual ET values across years. In the subsequent formal dryland change analysis, cropland meteorological stations were removed due to a potential considerable irrigation influence.
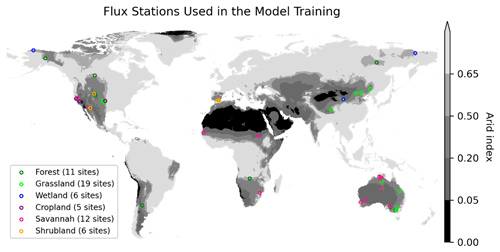
Figure 1The 59 flux stations in drylands (AI < 0.65) in FLUXNET2015 used in the RF model construction. The AI level classification is as follows (Middleton and Thomas, 1997): hyperarid (0 < AI < 0.05), arid (0.05 < AI < 0.2), semiarid (0.2 < AI < 0.5), and dry subhumid (0.5 < AI < 0.65).
3.1 ET estimation evaluation
We evaluated the performance of the RF model at each flux station using leave-one-station-out cross-validation, and most stations showed high accuracy (Fig. 2) with respect to both the Pearson correlation coefficients (Rcorr) of observed and predicted daily ET values and the root-mean-square error (RMSE). This indicated the feasibility of accurate daily ET simulations at most dryland flux stations. Furthermore, among the predictors, the LAI had the highest IMP (Fig. 2d), followed by the RSDN, TA, WS, VPD, and TArange. This demonstrated the importance of surface vegetation conditions in ET simulations at dryland stations.
3.2 Climatic, hydrological, and vegetation changes over drylands
The pattern of change in each climate and vegetation variable between the 2003–2010 and 2011–2019 periods showed considerable variations (Fig. 3). The number of stations with significant increases in the TA, LAI, and VPD was considerably greater than the number of stations with significant decreases. The number of stations with significant increases in P, ET, and P − ET was also greater than the number of stations with significant decreases. For P − ET, the ratio between the number of increasing and decreasing sites is the lowest. This shows the spatial variability in the trends indicated by the different indicators: the increase in the TA and VPD in the context of global warming is widespread and their spatial pattern is also similarity high. The increasing trend in the LAI is also dominant. The spatial pattern of ET changes is highly similar to that of the LAI. Both ET and the LAI show significant regional increases in the high latitudes of North America and in central Eurasia as well as decreases in the middle and low latitudes of North America. The spatial pattern of changes in P − ET is more similar to that of P, but the increase in P is not completely propagated to P − ET and may be partially offset by the trend in ET.
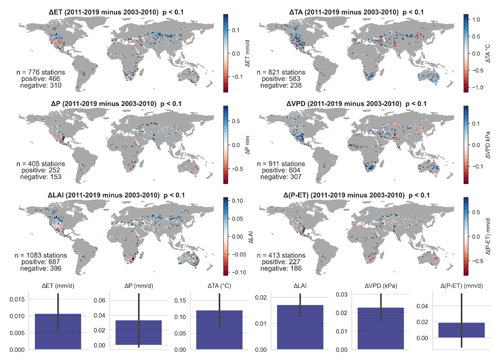
Figure 3Significant changes (p < 0.1) in ET, TA, P, VPD, LAI, and P − ET for dryland meteorological stations (from 2003–2010 to 2011–2019).
We compared the relationship between ΔVPD, which represents changes in atmospheric aridity, ΔP − ET, which represents changes in hydrological aridity, and ΔLAI, which represents changes in vegetation growth. ΔVPD showed a negative correlation with ΔP − ET (R= −0.19, p < 0.001), indicating that an elevated VPD in drylands did lead to a decrease in surface water availability. However, the negative correlation between ΔVPD and ΔLAI was not strong (R = −0.13, p < 0.001), indicating that atmospheric drying was not a dominant determinant of vegetation greening nor browning. The positive correlation between ΔP − ET and ΔLAI was not significant (p > 0.1), indicating a decoupling between the greening of dryland vegetation and changes in surface water availability.
3.3 Combined atmospheric, hydrological, and vegetation perspectives
We also analyzed the combinations of the VPD, LAI, and P − ET changes, and the distribution patterns of the different combinations across the globe represented different mechanisms of dryland changes (Fig. 5). In the dry subhumid, semiarid, and arid regions, three of the top four combinations exhibited significant increases in the LAI, while the VPD exhibited increases, no significant change, increases, and decreases, respectively. In the top four combinations, the combination with an increase in the VPD accompanied by an LAI decrease only ranked third or fourth. This suggests that the effect of vegetation browning caused by an increasing VPD may not be dominant and that the increasing atmospheric water demand did not considerably decrease vegetation growth. In the dry subhumid region, compared with the semiarid and arid regions, the “VPD↓ & LAI↑ & P − ET (−)” and “VPD↓ & LAI↑ & P − ET↑” combinations ranked higher. This indicates that the possibility of the combination of a VPD decrease accompanied by an LAI increase is higher in the dry subhumid region. In the arid region, the combination of “VPD↑ & LAI↑ & P − ET (−)” dropped from first to second in the ranking compared with the dry sub-humid and semiarid regions, indicating that the mechanism represented by the combination of the simultaneous increase in the VPD and LAI is less likely to occur when AI is lower. Surprisingly, of the seven combinations of the VPD, LAI, and P − ET in the top ranking, P − ET showed no significant change. This suggests a smaller contribution from changes in surface water availability in explaining the variation in combinations of mechanisms for dryland change, although the changes in P − ET and the VPD in the lower-ranked combinations showed opposite trends. The aridity increase represented by surface water obtained in this study is smaller than that indicated by soil moisture and runoff reported previously (Lian et al., 2021).
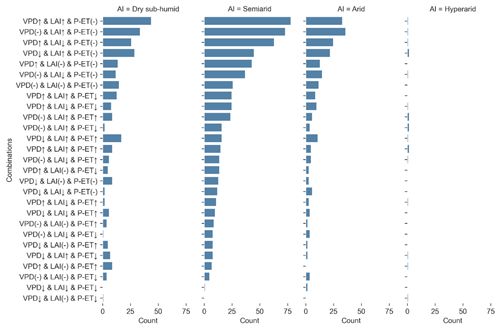
Figure 5Combinations of the VPD, LAI, and P − ET changes across various AI areas from 2003–2010 to 2011–2019. The “↑” symbol represents a significant increase (p < 0.1) in the VPD, LAI, or P − ET; “↓” represents a significant decrease (p < 0.1); and “(−)” represents insignificant change.
The distribution of these combinations is also highly heterogeneous spatially, indicating the high regional heterogeneity in global dryland change (Feng et al., 2022; Lian et al., 2021). Given that this study is at the station scale, the impacts of heterogeneous underlying surface conditions can be higher. Combinations with nonsignificant changes in P − ET are widely distributed globally (Fig. 6a, b, c, d, e, f, g), including in the western part of North America, Australia, and southern Europe, where there are more dense stations. Although the combinations of VPD and LAI changes appear to be spatially variable, some regional patterns were still found. For example, “VPD↑ & LAI↑ & P − ET (−)” is the dominant combination in Mongolian grasslands (Fig. 6a). An increase in the LAI due to increased P − ET was also observed in northwestern China and northern Central Asia (Fig. 6i, k), suggesting that the recent trend of wetting and greening in this region is more likely to be caused by increased surface water availability (Shi et al., 2007). The results of previous coarse regional patterns of dryland change may not necessarily be applicable at the station scale; however, this requires more station-scale evaluation and validation.
4.1 Implications and perspective
This study investigated the characteristics of dryland change at global dryland meteorological stations using a combination of atmospheric, hydrological, and vegetation indicators. A decoupling between atmospheric, hydrological, and ecological aridity was found in this work – specifically, atmospheric aridity represented by the VPD increased, hydrological aridity indicated by P − ET did not change significantly, and ecological aridity represented by the LAI decreased. This is consistent with the decoupling found in previous studies based on reanalysis data and coarse-resolution land surface model simulations (Lian et al., 2021) which considered the impacts of elevated CO2 concentration. This study also found that P − ET showed nonsignificant changes in most of the dominant combinations of the VPD, LAI, and P − ET. This is slightly different from the reported slight increase in hydrological aridity in previous studies based on soil moisture and runoff data (Lian et al., 2021), although the time span from 2003 to 2019 in the present study was smaller than these studies (usually more than 50 years).
The aim of this study is to revisit the dryland change issue at the station scale. The key to this is the use of a machine learning approach to estimate daily-scale ET data from meteorological stations and to combine the measured P and, thus, calculate P − ET. Machine-learning-based ET simulations (Jung et al., 2010, 2019) may effectively avoid the setting of various hypothetical mechanisms in physics-based ET models (Martens et al., 2017; Zhang et al., 2010; Mu et al., 2011), mine the relationship between dryland ET and various environmental factors such as climate and vegetation from measured data, and achieve a high estimation accuracy. Therefore, the estimation of P − ET at the station-scale effectively measured the status of surface water change, as soil moisture and runoff data are difficult to obtain at the meteorological station scale. Station-scale studies of dryland change may be a new direction for the future, given the limitation in the coarse resolution of current reanalysis data, land surface models, etc., and the difficulty with respect to validating their results in the field via ground in situ data. Combined use of climate, hydrological, and vegetation condition variables at the station scale may have the potential to provide an interface for dryland change studies to be more connected to ground observations and associated field experiments. The current satellite remote-sensing data still cannot fully capture the physiological and hydraulic characteristics (Zeng et al., 2022) of dryland plants in the context of climate change and extreme weather conditions. This illustrates that station-scale studies will be of ongoing importance in the future.
4.2 Limitations and uncertainties
4.2.1 Uncertainties in the ET estimation
In the past, data for P − ET have rarely been produced at the meteorological station scale, with most being produced at the coarse-resolution grid scale (Jung et al., 2019; Martens et al., 2017; Zhang et al., 2010); thus, this study combined machine-learning-based estimates of daily ET with actual measurements of P to produce P − ET data for dryland meteorological stations. ET simulations exhibit high accuracy at most stations, but accuracy is limited at a few stations, possibly due to the inefficiency of the selected predictor variables in the explanation of the station-specific ET variations (Shi et al., 2022). In future studies, it would be effective to incorporate station-specific plant hydraulic characteristics as well as vegetation-trait-related predictor variables (Anderegg, 2015; Anderegg et al., 2018; Zhao et al., 2022; Shi et al., 2023). In addition, combining data-driven machine learning methods with physical process-based ET estimation models would be promising (Zhao et al., 2019), with the potential to further improve ET simulation accuracy. Moreover, it may be beneficial to combine transpiration observations such as SAPFLUXNET (Poyatos et al., 2021) to provide estimates of transpiration. Compared with ET, transpiration can be more precisely correlated with plant physiological and hydraulic characteristics, thereby providing more detailed mechanism interpretations in dryland aridity change.
Furthermore, mismatches between the flux footprints of flux stations and remote-sensing data pixels may also cause uncertainty, especially if the flux footprints include considerable spatial heterogeneity (Chu et al., 2021). The 500 m scale of data extraction in this study may have reduced this effect partially, but it may still exist due to the variability in flux footprints across stations. Previous studies have shown that the representativeness of the flux footprint area's land cover types can be considerably decreased when data are extracted at scales larger than 500 m (Chu et al., 2021). The use of a fixed target area extent for data extraction may bias model–data integration in multi-station-level studies. In the future, to reduce the related bias, we should pay more attention to the heterogeneity within the flux footprints of specific flux stations, especially in remote-sensing data extraction and processing (Walther et al., 2022).
The low performance of some flux stations (e.g., shrubland stations) may be related to inadequate modeling of the influence of belowground hydrologic processes. Belowground hydrogeologic properties and groundwater dynamics are difficult to quantify directly via remote sensing or meteorological data. Thus, it is difficult to capture the effects of subterranean ventilation (López-Ballesteros et al., 2017) and the dynamic relationship between the plant root zone and groundwater. Previous studies have shown that the root zone storage capacity (Gao et al., 2014; Wang-Erlandsson et al., 2016; Singh et al., 2020) is important in hydrological processes in drylands and during drought events. Researchers have attempted to estimate the root depth and root zone storage capacity (Wang-Erlandsson et al., 2016; Stocker et al., 2023) or to couple drylands' deep-root distribution modules into Earth system models (Zhang et al., 2013; Li et al., 2015) and improve the hydrological and ecological prediction (Gao et al., 2014). However, in these approaches, partial limitations remain, such as the dependency on satellite-based ET data (Wang-Erlandsson et al., 2016) containing uncertainty. On the other hand, accurately modeling groundwater dynamics remains limited (Gleeson et al., 2016, 2021). Uncertainties in station-scale groundwater dynamics also affect our understanding of the root–groundwater relationship and groundwater's contribution to ET. Combining drought indices at different timescales (e.g., the Standardized Precipitation Evapotranspiration Index, SPEI) at the regional scale (Secci et al., 2021) and the Gravity Recovery and Climate Experiment (GRACE)-based anomalies in terrestrial water storage (Li et al., 2019) can be promising to indirectly represent the groundwater dynamics, but mismatches in spatial scales may still cause errors. In addition, our accuracy evaluation was based on the leave-one-station-out cross-validation (Zhang et al., 2021). Thus, the validation accuracy may be relatively low when there are no stations with similar environmental conditions in the training set. The RF model that we finally applied to the weather stations included all stations (i.e., no flux station was left out); thus, the accuracy can be improved a little, especially at weather stations with similar environmental conditions (e.g., shrubland stations) to the previously omitted flux station in the leave-one-station-out cross-validation.
4.2.2 Spatial and temporal representativeness of meteorological stations on dryland change
Although meteorological stations can provide more accurate climate, hydrology, and vegetation data at fine scales to support studies associated with dryland change, they may still have limitations with respect to spatial and temporal representativeness. First, the temporal representativeness of meteorological stations is highly variable across different regions of the globe. Inconsistencies in the length of station observation records, etc., may lead to imbalance when intercomparing regions. Second, meteorological stations are sparsely located in hyperarid areas, and the representativeness of hyperarid regions can be low. In other dryland types (i.e., dry subhumid, semiarid, and arid), the representativeness of meteorological stations may also be affected by other factors, such as human activities. In this study, it was considered that irrigation of dryland cropland could greatly affect the assessment of P − ET and the VPD; therefore, stations in croplands were removed. However, other disturbances from human activities may still exist, such as possible grazing (Huang et al., 2018) within the 500 m surrounding extent of the station. In contrast, climate adaptation management in surrounding regions of local meteorological stations may not require much attention due to the lack of spatial and temporal representativeness. The combined use of station-scale VPD, LAI, and P − ET data would be valuable for the development of associated adaptation policies in local agriculture management and ecological conservation.
Compared with previous dryland change studies spanning decades, the period in this study is only 2003–2019 due to the constraint of using MODIS-derived data. We split 2003–2019 into two periods with similar time spans, 2003–2010 and 2011–2019. In this way, it is possible to reduce the effect of extreme years when comparing the differences between the two periods. However, the time spans in this study are not very long compared with studies with longer time series (Lian et al., 2021; Huang et al., 2016); thus, the associated findings should be treated with more caution.
By combining climatic, hydrological, and vegetation data, this study assesses global dryland change at meteorological stations from 2003 to 2019. It shows that global drylands' atmospheric, hydrological and ecological aridity changes are inconsistent. Specifically, atmospheric aridity increased and ecological aridity decreased. Changes in hydrologic aridity were not significant in most of the dominant combinations of the VPD, LAI, and P − ET. This study highlights the significance of the investigation of dryland aridity changes using weather station-scale data, which can complement previous findings based on coarse-resolution climate reanalysis. It also has the promise of being combined with more station-scale data to provide support for local community's climate change adaptation.
The codes that were used for all analyses are available from the first author (haiyang.shi@hhu.edu.cn).
The data used in this study are available from the first author (haiyang.shi@hhu.edu.cn).
HS and GL initiated this research and were responsible for the integrity of the work as a whole. HS performed formal analysis and calculations and drafted the manuscript. HS was responsible for the data collection and analysis. GL, PDM, TVdV, OH, XH, and AK contributed resources and financial support.
The contact author has declared that none of the authors has any competing interests.
Publisher’s note: Copernicus Publications remains neutral with regard to jurisdictional claims made in the text, published maps, institutional affiliations, or any other geographical representation in this paper. While Copernicus Publications makes every effort to include appropriate place names, the final responsibility lies with the authors.
The authors would like to thank Pierre Gentine for his insightful suggestions on ET modeling. We are also grateful to the editor and reviewers for their insightful comments and suggestions.
This research was supported by the Tianshan Talent Cultivation (grant no. 2022TSYCLJ0001), the Key projects of the Natural Science Foundation of Xinjiang Autonomous Region (grant no. 2022D01D01), the Strategic Priority Research Program of the Chinese Academy of Sciences (grant no. XDA20060302), and the High-End Foreign Experts Project.
This open-access publication was funded by Technische Universität Berlin.
This paper was edited by Hongkai Gao and reviewed by Wenbin Liu and one anonymous referee.
Anderegg, W. R.: Spatial and temporal variation in plant hydraulic traits and their relevance for climate change impacts on vegetation, New Phytol., 205, 1008–1014, 2015.
Anderegg, W. R., Konings, A. G., Trugman, A. T., Yu, K., Bowling, D. R., Gabbitas, R., Karp, D. S., Pacala, S., Sperry, J. S., and Sulman, B. N.: Hydraulic diversity of forests regulates ecosystem resilience during drought, Nature, 561, 538–541, 2018.
Berg, A. and McColl, K. A.: No projected global drylands expansion under greenhouse warming, Nat. Clim. Change, 11, 331–337, https://doi.org/10.1038/s41558-021-01007-8, 2021.
Chu, H., Luo, X., Ouyang, Z., Chan, W. S., Dengel, S., Biraud, S. C., Torn, M. S., Metzger, S., Kumar, J., Arain, M. A., Arkebauer, T. J., Baldocchi, D., Bernacchi, C., Billesbach, D., Black, T. A., Blanken, P. D., Bohrer, G., Bracho, R., Brown, S., Brunsell, N. A., Chen, J., Chen, X., Clark, K., Desai, A. R., Duman, T., Durden, D., Fares, S., Forbrich, I., Gamon, J. A., Gough, C. M., Griffis, T., Helbig, M., Hollinger, D., Humphreys, E., Ikawa, H., Iwata, H., Ju, Y., Knowles, J. F., Knox, S. H., Kobayashi, H., Kolb, T., Law, B., Lee, X., Litvak, M., Liu, H., Munger, J. W., Noormets, A., Novick, K., Oberbauer, S. F., Oechel, W., Oikawa, P., Papuga, S. A., Pendall, E., Prajapati, P., Prueger, J., Quinton, W. L., Richardson, A. D., Russell, E. S., Scott, R. L., Starr, G., Staebler, R., Stoy, P. C., Stuart-Haëntjens, E., Sonnentag, O., Sullivan, R. C., Suyker, A., Ueyama, M., Vargas, R., Wood, J. D., and Zona, D.: Representativeness of Eddy-Covariance flux footprints for areas surrounding AmeriFlux sites, Agr. Forest Meteorol., 301–302, 108350, https://doi.org/10.1016/j.agrformet.2021.108350, 2021.
Denissen, J. M., Teuling, A. J., Pitman, A. J., Koirala, S., Migliavacca, M., Li, W., Reichstein, M., Winkler, A. J., Zhan, C., and Orth, R.: Widespread shift from ecosystem energy to water limitation with climate change, Nat. Clim. Change, 12, 677–684, 2022.
Díaz-Uriarte, R. and Alvarez de Andrés, S.: Gene selection and classification of microarray data using random forest, BMC Bioinformatics, 7, 1–13, 2006.
Feng, S. and Fu, Q.: Expansion of global drylands under a warming climate, Atmos. Chem. Phys., 13, 10081–10094, https://doi.org/10.5194/acp-13-10081-2013, 2013.
Feng, S., Gu, X., Luo, S., Liu, R., Gulakhmadov, A., Slater, L. J., Li, J., Zhang, X., and Kong, D.: Greenhouse gas emissions drive global dryland expansion but not spatial patterns of change in aridification, J. Climate, 35, 2901–2917, 2022.
Fensholt, R., Langanke, T., Rasmussen, K., Reenberg, A., Prince, S. D., Tucker, C., Scholes, R. J., Le, Q. B., Bondeau, A., and Eastman, R.: Greenness in semi-arid areas across the globe 1981–2007 – an Earth Observing Satellite based analysis of trends and drivers, Remote Sens. Environ., 121, 144–158, 2012.
Fu, B., Stafford-Smith, M., Wang, Y., Wu, B., Yu, X., Lv, N., Ojima, D. S., Lv, Y., Fu, C., Liu, Y., Niu, S., Zhang, Y., Zeng, H., Liu, Y., Liu, Y., Feng, X., Zhang, L., Wei, Y., Xu, Z., Li, F., Cui, X., Diop, S., and Chen, X.: The Global-DEP conceptual framework – research on dryland ecosystems to promote sustainability, Curr. Opin. Env. Sust., 48, 17–28, https://doi.org/10.1016/j.cosust.2020.08.009, 2021.
Gao, H., Hrachowitz, M., Schymanski, S., Fenicia, F., Sriwongsitanon, N., and Savenije, H.: Climate controls how ecosystems size the root zone storage capacity at catchment scale, Geophys. Res. Lett., 41, 7916–7923, 2014.
Gleeson, T., Befus, K. M., Jasechko, S., Luijendijk, E., and Cardenas, M. B.: The global volume and distribution of modern groundwater, Nat. Geosci., 9, 161–167, 2016.
Gleeson, T., Wagener, T., Döll, P., Zipper, S. C., West, C., Wada, Y., Taylor, R., Scanlon, B., Rosolem, R., Rahman, S., Oshinlaja, N., Maxwell, R., Lo, M.-H., Kim, H., Hill, M., Hartmann, A., Fogg, G., Famiglietti, J. S., Ducharne, A., de Graaf, I., Cuthbert, M., Condon, L., Bresciani, E., and Bierkens, M. F. P.: GMD perspective: The quest to improve the evaluation of groundwater representation in continental- to global-scale models, Geosci. Model Dev., 14, 7545–7571, https://doi.org/10.5194/gmd-14-7545-2021, 2021.
Grömping, U.: Variable importance assessment in regression: linear regression versus random forest, The American Statistician, 63, 308–319, 2009.
Grünzweig, J. M., De Boeck, H. J., Rey, A., Santos, M. J., Adam, O., Bahn, M., Belnap, J., Deckmyn, G., Dekker, S. C., Flores, O., Gliksman, D., Helman, D., Hultine, K. R., Liu, L., Meron, E., Michael, Y., Sheffer, E., Throop, H. L., Tzuk, O., and Yakir, D.: Dryland mechanisms could widely control ecosystem functioning in a drier and warmer world, Nat. Ecol. Evol., 6, 1064–1076, https://doi.org/10.1038/s41559-022-01779-y, 2022.
He, B., Wang, S., Guo, L., and Wu, X.: Aridity change and its correlation with greening over drylands, Agr. Forest Meteorol., 278, 107663, https://doi.org/10.1016/j.agrformet.2019.107663, 2019.
Hickler, T., Eklundh, L., Seaquist, J. W., Smith, B., Ardö, J., Olsson, L., Sykes, M. T., and Sjöström, M.: Precipitation controls Sahel greening trend, Geophys. Res. Lett., 32, L21415, https://doi.org/10.1029/2005GL024370, 2005.
Howell, T. A. and Dusek, D. A.: Comparison of Vapor-Pressure-Deficit Calculation Methods—Southern High Plains, J. Irrig. Drain. E., 121, 191–198, https://doi.org/10.1061/(ASCE)0733-9437(1995)121:2(191), 1995.
Huang, J., Yu, H., Guan, X., Wang, G., and Guo, R.: Accelerated dryland expansion under climate change, Nat. Clim. Change, 6, 166–171, https://doi.org/10.1038/nclimate2837, 2016.
Huang, J., Li, Y., Fu, C., Chen, F., Fu, Q., Dai, A., Shinoda, M., Ma, Z., Guo, W., Li, Z., Zhang, L., Liu, Y., Yu, H., He, Y., Xie, Y., Guan, X., Ji, M., Lin, L., Wang, S., Yan, H., and Wang, G.: Dryland climate change: Recent progress and challenges, Rev. Geophys., 55, 719–778, https://doi.org/10.1002/2016RG000550, 2017.
Huang, X., Luo, G., Ye, F., and Han, Q.: Effects of grazing on net primary productivity, evapotranspiration and water use efficiency in the grasslands of Xinjiang, China, J. Arid Land, 10, 588–600, 2018.
Jung, M., Reichstein, M., Ciais, P., Seneviratne, S. I., Sheffield, J., Goulden, M. L., Bonan, G., Cescatti, A., Chen, J., de Jeu, R., Dolman, A. J., Eugster, W., Gerten, D., Gianelle, D., Gobron, N., Heinke, J., Kimball, J., Law, B. E., Montagnani, L., Mu, Q., Mueller, B., Oleson, K., Papale, D., Richardson, A. D., Roupsard, O., Running, S., Tomelleri, E., Viovy, N., Weber, U., Williams, C., Wood, E., Zaehle, S., and Zhang, K.: Recent decline in the global land evapotranspiration trend due to limited moisture supply, Nature, 467, 951–954, https://doi.org/10.1038/nature09396, 2010.
Jung, M., Koirala, S., Weber, U., Ichii, K., Gans, F., Camps-Valls, G., Papale, D., Schwalm, C., Tramontana, G., and Reichstein, M.: The FLUXCOM ensemble of global land-atmosphere energy fluxes, Sci. Data, 6, 74, https://doi.org/10.1038/s41597-019-0076-8, 2019.
Li, B., Rodell, M., Kumar, S., Beaudoing, H. K., Getirana, A., Zaitchik, B. F., de Goncalves, L. G., Cossetin, C., Bhanja, S., and Mukherjee, A.: Global GRACE data assimilation for groundwater and drought monitoring: Advances and challenges, Water Resour. Res., 55, 7564–7586, 2019.
Li, C., Zhang, C., Luo, G., Chen, X., Maisupova, B., Madaminov, A. A., Han, Q., and Djenbaev, B. M.: Carbon stock and its responses to climate change in C entral A sia, Glob. Change Biol., 21, 1951–1967, 2015.
Li, C., Fu, B., Wang, S., Stringer, L. C., Wang, Y., Li, Z., Liu, Y., and Zhou, W.: Drivers and impacts of changes in China's drylands, Nat. Rev. Earth Environ., 2, 858–873, https://doi.org/10.1038/s43017-021-00226-z, 2021.
Lian, X., Piao, S., Chen, A., Huntingford, C., Fu, B., Li, L. Z. X., Huang, J., Sheffield, J., Berg, A. M., Keenan, T. F., McVicar, T. R., Wada, Y., Wang, X., Wang, T., Yang, Y., and Roderick, M. L.: Multifaceted characteristics of dryland aridity changes in a warming world, Nat. Rev. Earth Environ., 2, 232–250, https://doi.org/10.1038/s43017-021-00144-0, 2021.
López-Ballesteros, A., Serrano-Ortiz, P., Kowalski, A. S., Sánchez-Cañete, E. P., Scott, R. L., and Domingo, F.: Subterranean ventilation of allochthonous CO2 governs net CO2 exchange in a semiarid Mediterranean grassland, Agr. Forest Meteorol., 234–235, 115–126, https://doi.org/10.1016/j.agrformet.2016.12.021, 2017.
Martens, B., Miralles, D. G., Lievens, H., van der Schalie, R., de Jeu, R. A. M., Fernández-Prieto, D., Beck, H. E., Dorigo, W. A., and Verhoest, N. E. C.: GLEAM v3: satellite-based land evaporation and root-zone soil moisture, Geosci. Model Dev., 10, 1903–1925, https://doi.org/10.5194/gmd-10-1903-2017, 2017.
Middleton, N. and Thomas, D.: World Atlas of Desertification, Arnold, London, 1997.
Milly, P. C. D. and Dunne, K. A.: Potential evapotranspiration and continental drying, Nat. Clim. Change, 6, 946–949, https://doi.org/10.1038/nclimate3046, 2016.
Mu, Q., Zhao, M., and Running, S. W.: Improvements to a MODIS global terrestrial evapotranspiration algorithm, Remote Sens. Environ., 115, 1781–1800, https://doi.org/10.1016/j.rse.2011.02.019, 2011.
Pan, N., Wang, S., Liu, Y., Li, Y., Xue, F., Wei, F., Yu, H., and Fu, B.: Rapid increase of potential evapotranspiration weakens the effect of precipitation on aridity in global drylands, J. Arid Environ., 186, 104414, https://doi.org/10.1016/j.jaridenv.2020.104414, 2021.
Poulter, B., Frank, D., Ciais, P., Myneni, R. B., Andela, N., Bi, J., Broquet, G., Canadell, J. G., Chevallier, F., and Liu, Y. Y.: Contribution of semi-arid ecosystems to interannual variability of the global carbon cycle, Nature, 509, 600–603, 2014.
Poyatos, R., Granda, V., Flo, V., Adams, M. A., Adorján, B., Aguadé, D., Aidar, M. P. M., Allen, S., Alvarado-Barrientos, M. S., Anderson-Teixeira, K. J., Aparecido, L. M., Arain, M. A., Aranda, I., Asbjornsen, H., Baxter, R., Beamesderfer, E., Berry, Z. C., Berveiller, D., Blakely, B., Boggs, J., Bohrer, G., Bolstad, P. V., Bonal, D., Bracho, R., Brito, P., Brodeur, J., Casanoves, F., Chave, J., Chen, H., Cisneros, C., Clark, K., Cremonese, E., Dang, H., David, J. S., David, T. S., Delpierre, N., Desai, A. R., Do, F. C., Dohnal, M., Domec, J.-C., Dzikiti, S., Edgar, C., Eichstaedt, R., El-Madany, T. S., Elbers, J., Eller, C. B., Euskirchen, E. S., Ewers, B., Fonti, P., Forner, A., Forrester, D. I., Freitas, H. C., Galvagno, M., Garcia-Tejera, O., Ghimire, C. P., Gimeno, T. E., Grace, J., Granier, A., Griebel, A., Guangyu, Y., Gush, M. B., Hanson, P. J., Hasselquist, N. J., Heinrich, I., Hernandez-Santana, V., Herrmann, V., Hölttä, T., Holwerda, F., Irvine, J., Isarangkool Na Ayutthaya, S., Jarvis, P. G., Jochheim, H., Joly, C. A., Kaplick, J., Kim, H. S., Klemedtsson, L., Kropp, H., Lagergren, F., Lane, P., Lang, P., Lapenas, A., Lechuga, V., Lee, M., Leuschner, C., Limousin, J.-M., Linares, J. C., Linderson, M.-L., Lindroth, A., Llorens, P., López-Bernal, Á., Loranty, M. M., Lüttschwager, D., Macinnis-Ng, C., Maréchaux, I., Martin, T. A., Matheny, A., McDowell, N., McMahon, S., Meir, P., Mészáros, I., Migliavacca, M., Mitchell, P., Mölder, M., Montagnani, L., Moore, G. W., Nakada, R., Niu, F., Nolan, R. H., Norby, R., Novick, K., Oberhuber, W., Obojes, N., Oishi, A. C., Oliveira, R. S., Oren, R., Ourcival, J.-M., Paljakka, T., Perez-Priego, O., Peri, P. L., Peters, R. L., Pfautsch, S., Pockman, W. T., Preisler, Y., Rascher, K., Robinson, G., Rocha, H., Rocheteau, A., Röll, A., Rosado, B. H. P., Rowland, L., Rubtsov, A. V., Sabaté, S., Salmon, Y., Salomón, R. L., Sánchez-Costa, E., Schäfer, K. V. R., Schuldt, B., Shashkin, A., Stahl, C., Stojanović, M., Suárez, J. C., Sun, G., Szatniewska, J., Tatarinov, F., Tesař, M., Thomas, F. M., Tor-ngern, P., Urban, J., Valladares, F., van der Tol, C., van Meerveld, I., Varlagin, A., Voigt, H., Warren, J., Werner, C., Werner, W., Wieser, G., Wingate, L., Wullschleger, S., Yi, K., Zweifel, R., Steppe, K., Mencuccini, M., and Martínez-Vilalta, J.: Global transpiration data from sap flow measurements: the SAPFLUXNET database, Earth Syst. Sci. Data, 13, 2607–2649, https://doi.org/10.5194/essd-13-2607-2021, 2021.
Prăvălie, R.: Drylands extent and environmental issues. A global approach, Earth-Sci. Rev., 161, 259–278, https://doi.org/10.1016/j.earscirev.2016.08.003, 2016.
Ramón Vallejo, V., Smanis, A., Chirino, E., Fuentes, D., Valdecantos, A., and Vilagrosa, A.: Perspectives in dryland restoration: approaches for climate change adaptation, New Forests, 43, 561–579, 2012.
Reynolds, J. F., Smith, D. M. S., Lambin, E. F., Turner, B. L., Mortimore, M., Batterbury, S. P. J., Downing, T. E., Dowlatabadi, H., Fernández, R. J., Herrick, J. E., Huber-Sannwald, E., Jiang, H., Leemans, R., Lynam, T., Maestre, F. T., Ayarza, M., and Walker, B.: Global Desertification: Building a Science for Dryland Development, Science, 316, 847–851, https://doi.org/10.1126/science.1131634, 2007.
Roderick, M. L., Greve, P., and Farquhar, G. D.: On the assessment of aridity with changes in atmospheric CO2, Water Resour. Res., 51, 5450–5463, 2015.
Ryu, Y., Jiang, C., Kobayashi, H., and Detto, M.: MODIS-derived global land products of shortwave radiation and diffuse and total photosynthetically active radiation at 5 km resolution from 2000, Remote Sens. Environ., 204, 812–825, https://doi.org/10.1016/j.rse.2017.09.021, 2018.
Secci, D., Tanda, M. G., D'Oria, M., Todaro, V., and Fagandini, C.: Impacts of climate change on groundwater droughts by means of standardized indices and regional climate models, J. Hydrol., 603, 127154, https://doi.org/10.1016/j.jhydrol.2021.127154, 2021.
Shi, H., Luo, G., Hellwich, O., Xie, M., Zhang, C., Zhang, Y., Wang, Y., Yuan, X., Ma, X., Zhang, W., Kurban, A., De Maeyer, P., and Van de Voorde, T.: Evaluation of water flux predictive models developed using eddy-covariance observations and machine learning: a meta-analysis, Hydrol. Earth Syst. Sci., 26, 4603–4618, https://doi.org/10.5194/hess-26-4603-2022, 2022.
Shi, H., Luo, G., Hellwich, O., Kurban, A., De Maeyer, P., and Van de Voorde, T.: Revisiting and attributing the global controls over terrestrial ecosystem functions of climate and plant traits at FLUXNET sites via causal graphical models, Biogeosciences, 20, 2727–2741, https://doi.org/10.5194/bg-20-2727-2023, 2023.
Shi, Y., Shen, Y., Kang, E., Li, D., Ding, Y., Zhang, G., and Hu, R.: Recent and future climate change in northwest China, Clim. Change, 80, 379–393, 2007.
Singh, C., Wang-Erlandsson, L., Fetzer, I., Rockström, J., and Van Der Ent, R.: Rootzone storage capacity reveals drought coping strategies along rainforest-savanna transitions, Environ. Res. Lett., 15, 124021, https://doi.org/10.1088/1748-9326/abc377, 2020.
Stocker, B. D., Tumber-Dávila, S. J., Konings, A. G., Anderson, M. C., Hain, C., and Jackson, R. B.: Global patterns of water storage in the rooting zones of vegetation, Nat. Geosci., 16, 250–256, https://doi.org/10.1038/s41561-023-01125-2, 2023.
Strobl, C., Boulesteix, A.-L., Kneib, T., Augustin, T., and Zeileis, A.: Conditional variable importance for random forests, BMC Bioinformatics, 9, 307, https://doi.org/10.1186/1471-2105-9-307, 2008.
Tramontana, G., Jung, M., Schwalm, C. R., Ichii, K., Camps-Valls, G., Ráduly, B., Reichstein, M., Arain, M. A., Cescatti, A., Kiely, G., Merbold, L., Serrano-Ortiz, P., Sickert, S., Wolf, S., and Papale, D.: Predicting carbon dioxide and energy fluxes across global FLUXNET sites with regression algorithms, Biogeosciences, 13, 4291–4313, https://doi.org/10.5194/bg-13-4291-2016, 2016.
Walther, S., Besnard, S., Nelson, J. A., El-Madany, T. S., Migliavacca, M., Weber, U., Carvalhais, N., Ermida, S. L., Brümmer, C., Schrader, F., Prokushkin, A. S., Panov, A. V., and Jung, M.: Technical note: A view from space on global flux towers by MODIS and Landsat: the FluxnetEO data set, Biogeosciences, 19, 2805–2840, https://doi.org/10.5194/bg-19-2805-2022, 2022.
Wang-Erlandsson, L., Bastiaanssen, W. G. M., Gao, H., Jägermeyr, J., Senay, G. B., van Dijk, A. I. J. M., Guerschman, J. P., Keys, P. W., Gordon, L. J., and Savenije, H. H. G.: Global root zone storage capacity from satellite-based evaporation, Hydrol. Earth Syst. Sci., 20, 1459–1481, https://doi.org/10.5194/hess-20-1459-2016, 2016.
Yang, Y., Zhang, S., McVicar, T. R., Beck, H. E., Zhang, Y., and Liu, B.: Disconnection Between Trends of Atmospheric Drying and Continental Runoff, Water Resour. Res., 54, 4700–4713, https://doi.org/10.1029/2018WR022593, 2018.
Yao, J., Liu, H., Huang, J., Gao, Z., Wang, G., Li, D., Yu, H., and Chen, X.: Accelerated dryland expansion regulates future variability in dryland gross primary production, Nat. Commun., 11, 1665, https://doi.org/10.1038/s41467-020-15515-2, 2020.
Yao, Y., Liu, Y., Wang, Y., and Fu, B.: Greater increases in China's dryland ecosystem vulnerability in drier conditions than in wetter conditions, J. Environ. Manage., 291, 112689, https://doi.org/10.1016/j.jenvman.2021.112689, 2021.
Zeng, Y., Hao, D., Huete, A., Dechant, B., Berry, J., Chen, J. M., Joiner, J., Frankenberg, C., Bond-Lamberty, B., Ryu, Y., Xiao, J., Asrar, G. R., and Chen, M.: Optical vegetation indices for monitoring terrestrial ecosystems globally, Nat. Rev. Earth Environ., 3, 477–493, https://doi.org/10.1038/s43017-022-00298-5, 2022.
Zhang, C., Li, C., Luo, G., and Chen, X.: Modeling plant structure and its impacts on carbon and water cycles of the Central Asian arid ecosystem in the context of climate change, Ecol. Model., 267, 158–179, https://doi.org/10.1016/j.ecolmodel.2013.06.008, 2013.
Zhang, C., Luo, G., Hellwich, O., Chen, C., Zhang, W., Xie, M., He, H., Shi, H., and Wang, Y.: A framework for estimating actual evapotranspiration at weather stations without flux observations by combining data from MODIS and flux towers through a machine learning approach, J. Hydrol., 603, 127047, https://doi.org/10.1016/j.jhydrol.2021.127047, 2021.
Zhang, K., Kimball, J. S., Nemani, R. R., and Running, S. W.: A continuous satellite-derived global record of land surface evapotranspiration from 1983 to 2006, Water Resour. Res., 46, W09522, https://doi.org/10.1029/2009WR008800, 2010.
Zhao, M., A, G., Liu, Y., and Konings, A. G.: Evapotranspiration frequently increases during droughts, Nat. Clim. Change, 12, 1024–1030, https://doi.org/10.1038/s41558-022-01505-3, 2022.
Zhao, W. L., Gentine, P., Reichstein, M., Zhang, Y., Zhou, S., Wen, Y., Lin, C., Li, X., and Qiu, G. Y.: Physics-Constrained Machine Learning of Evapotranspiration, Geophys. Res. Lett., 46, 14496–14507, https://doi.org/10.1029/2019GL085291, 2019.
Zhu, Z., Piao, S., Myneni, R. B., Huang, M., Zeng, Z., Canadell, J. G., Ciais, P., Sitch, S., Friedlingstein, P., and Arneth, A.: Greening of the Earth and its drivers, Nat. Clim. Change, 6, 791–795, 2016.