the Creative Commons Attribution 4.0 License.
the Creative Commons Attribution 4.0 License.
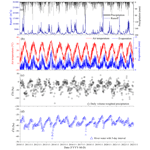
Seasonal variation and influence factors of river water isotopes in the East Asian monsoon region: a case study in the Xiangjiang River basin spanning 13 hydrological years
Xiong Xiao
Xinping Zhang
Zhuoyong Xiao
Zhiguo Rao
Xinguang He
Cicheng Zhang
Seasonal variation and influencing factors of river water isotopes were investigated in the Xiangjiang River basin located in the East Asian monsoon region. This investigation involved comprehensive sampling of daily precipitation and river water with a 5 d interval as well as observing hydrometeorological factors spanning 13 hydrological years from January 2010 to December 2022, combined with the temporal and spatial correlation analyses based on linear regression and the isotopic Atmospheric Water Balance Model. Key findings are as follows: river water δ2H (δ2HR) exhibited significant seasonal variation, with the most positive and negative values occurring in the spring flood period and summer drought period, respectively, in alignment with those observed in precipitation. The correlations of the δ2HR with corresponding hydrometeorological factors with a 5 d interval were commonly weak due to the seasonality of precipitation isotopes and mixing of various water bodies within the basin, but the changes in the runoff (ΔR) and δ2HR () between two contiguous samplings with 5 d or higher intervals showed significant responses to the corresponding accumulated precipitation and evaporation. Prolonged rainless intervals with high evaporation rates in 2013 and 2022 as well as significant precipitation events in major flood periods in 2011 and 2017 had a significant impact on the δ2HR and runoff discharge. However, the most positive δ2HR values were primarily influenced by precipitation input with the most enriched isotopes in the spring flood period, while the moderately isotope-depleted precipitation during limited wetness conditions led to the most negative δ2HR. The spatial correlation analysis between water isotopes and hydrometeorological factors at the observing site and in the surrounding regions supported the representation of the Changsha site in the Xiangjiang River basin. These results underscore the potential of as a proxy that reflects the seasonal variations in local environments, while caution is advised when interpreting extreme isotopic signals in river water. Overall, this study provides insights into the seasonal variation, extreme signal interpreting, and controlling factors of δ2HR in the study area, which was valuable for paleoclimate reconstruction and establishment of isotope hydrologic models.
- Article
(8288 KB) - Full-text XML
-
Supplement
(1530 KB) - BibTeX
- EndNote
River water is commonly recognized as a natural integrator of basin hydrological processes, gaining insights into the effects of hydrometeorological factors like air temperature, evaporation, precipitation input, and runoff discharge or water level (Yang et al., 2020; von Freyberg et al., 2022). Stable isotopes of natural water possess exceptional sensitivity and serve as remarkable recorders of environmental change; e.g., water bodies undergo phase changes throughout the water cycle, resulting in stable isotope fractionation – that is, an occurrence where light and heavy stable isotope molecules are distributed unequally between phases (Scholl et al., 2015; Xiao et al., 2022b). Generally, during water phase changes, light isotope molecules tend to evaporate more readily than heavy isotope molecules, while heavy isotope molecules preferentially condense compared to their lighter counterparts (Craig, 1961; Dansgaard, 1964). The isotope fractionation contributes to variations in stable isotopic compositions among different water bodies within the water cycle. For instance, the river water stable isotopes primarily reflect the characteristics of precipitation, as precipitation input acts as the primary water source (Sprenger et al., 2022; L. Wang et al., 2023). Moreover, due to varying degrees of evaporative enrichment and mixing processes experienced by different water bodies within a basin, the river water isotopes markedly differ from those of the precipitation input and exhibit distinct seasonality (Jiang et al., 2021; Sun et al., 2021; Das and Rai, 2022). This disparity forms the basis for employing stable isotope techniques to investigate river water generation processes in basins, while the stable isotope techniques were widely used to indicate the water cycle processes (Boral et al., 2019; Xiao et al., 2020; Wu et al., 2021) and find extensive application in hydrometeorology modeling and diagnosis (e.g., Aggarwal et al., 2016; Sinha and Chakraborty, 2019; Zhiña et al., 2022) as well as in paleoclimate reconstruction (e.g., Steinman et al., 2010; Jiménez-Iñiguez et al., 2022; Emmanouilidis et al., 2022).
Extensive efforts have been made to investigate the extent of variations in river water isotopes and to examine the relationship between stable isotopes in river water and specific environmental factors (e.g., Yang et al., 2020; Das and Rai, 2022; Ren et al., 2023). Linear regression is an effective tool to build the empirical relationships between hydrometeorological factors and river water isotopes, while numerous empirical formulas have been developed and used for paleoclimate reconstruction and interpretation based on these empirical relationships (Kendall and Coplen, 2001; Nan et al., 2019). However, in regions where new water mixes thoroughly with old water, the river water isotopes exhibit dampened signals, indicating that old water dominates the composition of stream water and that the response of river water isotopes to hydrometeorological factors is sluggish (Muñoz-Villers and McDonnell, 2013; Streletskiy et al., 2015). Moreover, extreme-precipitation and drought events have become more frequent against the background of global climate changes, as evidenced in numerous regions worldwide (Nkemelang et al., 2018; Cook et al., 2018; Grillakis, 2019; Marengo et al., 2020; Cardoso et al., 2020). Furthermore, the scale effect and spatial heterogeneity have always been a problem in hydrological model research (Seyfried and Wilcox, 1995; Blöschl, 2006; Pechlivanidis et al., 2011; Devia et al., 2015), e.g., the representation of the observations at limited sampling sites on the whole basin scale with a large area. These introduce additional complexities and uncertainties in identifying the seasonal variation and influence factors of river water isotopes on a basin scale, and thus the interpretation of the extreme isotopic signals and the variations in river water isotopes and the representation of the site observation based on the spatial correlation analysis become necessary (Uchiyama et al., 2017; Boutt et al., 2019; Saranya et al., 2020).
The East Asian monsoon region, characterized by complex water vapor sources, substantial seasonal and interannual temperature and precipitation variations, as well as frequent floods and seasonal droughts, further contributes to the hydrological complexity in this region (Huang et al., 1998; J. Zhou et al., 2019; Q. Wang et al., 2023). Hence, long-term observations of river water and precipitation isotopes and hydrometeorological factors, along with comprehensive analyses of the influencing factors, are crucial to enhancing our understanding of how climate change impacts hydrological regimes in basins within the East Asian monsoon region. Long-term observations of water isotopes are crucial as they enable the capture of extreme-precipitation and drought events, facilitating an analysis of their influences on river water isotopes while also unveiling patterns of seasonal and interannual variation (Rode et al., 2016; von Freyberg et al., 2022; Ren et al., 2023). However, the long-term observations of river water isotopes with a high sampling frequency are relatively challenging and rare due to logistical constraints (von Freyberg et al., 2017).
Therefore, in this study, the Xiangjiang River basin was selected as the study area to investigate the seasonal variation and controlling factors of river water isotopes under the influence of the monsoon. Extensive sampling of river water with a 5 d interval and daily precipitation, along with the monitoring of hydrometeorological factors, was conducted over 13 complete hydrological years from January 2010 to December 2022, while simple and multiple linear regression was used to identify the temporal correlation between the hydrometeorological factors and the river water isotopes, and the isotopic Atmospheric Water Balance Model (iAWBM) was used to simulate the spatial distribution of precipitation isotopes and hydrometeorological factors such as air temperature, evaporation, and precipitation amount. This study aims to achieve the following objectives: (1) identify the factors influencing the seasonality of river water isotopes and assess the influences of extreme drought and precipitation events; (2) interpret the environmental significance implied by the seasonality of river water isotopes; and (3) verify the representation of the observation at the Changsha site in the Xiangjiang River basin.
The study area was situated in a typical East Asian monsoon region characterized by distinct climatic variations throughout the four seasons. On average, the annual precipitation and evaporation were 1147 and 902 mm, respectively (Xiao et al., 2022a). Notably, there is a significant seasonal disparity in precipitation, with an average of 152 rainy days each year. The period from early March to mid-July experiences abundant precipitation due to the influence of the monsoon, whereas, from mid-July to September, drought conditions prevail as a result of the subtropical high-pressure system. The average annual temperature in the region is 17.4 ∘C, and the duration of the plant-growing period spans approximately 330 d.
The Xiangjiang River basin, originating in the Guangxi Zhuang Autonomous Region, encompasses a drainage area of 94 660 km2. It stretches for 856 km northward across Hunan Province, passing through cities like Hengyang, Xiangtan, Zhuzhou, and Changsha (Fig. 1). The altitudinal range of the Xiangjiang River basin varies from 1902 to 10 m, with higher elevations in the southern region characterized by multiple terraces and valley landforms, while the northern part is relatively lower. The middle and lower reaches of the Xiangjiang River basin are predominantly hilly basins surrounded by the Xuefeng Mountains and Nanling Mountains (Fig. 1).
3.1 Water sample collection and analysis
From January 2010 to December 2022, the collection of river water and precipitation samples was conducted.
River water sampling took place 929 times at the center of Orange Island, with a regular sampling interval of 5 d. Specifically, samples were obtained on the 1st, 6th, 11th, 16th, 21st, and 26th days of each month. The sampling depth of river water was relatively deep to avoid the influences of human activity; moreover, this operation can avoid the evaporation fractionation of surface water during the sampling and ensure adequate mixes of the river water.
At the College of Geographic Science, Hunan Normal University, Changsha (Fig. 1, E, N), the sampling of precipitation and the measurements of precipitation amount were conducted at an altitude of 55 m, adjacent to the Yuelu Mountains, throughout the sampling period of this study (2010–2022). For precipitation sampling, a siphon rain gauge was repurposed as a collector, consisting of a 6 cm diameter funnel connected to a glass bottle via a plastic pipe. Both rainfall and snowfall were collected and measured at 08:00 and 20:00 local time on the precipitation day, and the volume-weighted value of the two samplings was used to represent the precipitation isotopic values of the day, while the precipitation amount of the day was calculated by the sum of the two samplings. Snowfall samples were carefully packed in sealed plastic bags and transferred to the glass bottle, which was later melted at room temperature because the glass bottle is sealed and the collection process is completed quickly. Thus the evaporation of precipitation samples is effectively prevented. A total of 1668 precipitation isotopic values was obtained over 1668 precipitation days. Furthermore, considering that the sampling interval for river water was set at 5 d and previous studies have indicated that it may take 3–5 d for precipitation (new water) to significantly contribute to river water (Yao et al., 2016; Xiao et al., 2022b), the precipitation isotopes were volume-weighted in the 5 d interval within this study.
To ensure proper preservation, both the river water and precipitation samples were transferred to clean, sealed, polyethylene bottles (30 mL) and stored in a refrigerator at 0 ∘C. However, few precipitation and river water samples were lost, resulting in some missing data. The isotopic composition of the samples was determined using the off-axis integrated-cavity-output spectroscopy method, specifically conducted with equipment from Los Gatos Research in the USA. The stable isotopic values are represented by the δ (per mil) value of the sample relative to Vienna Standard Mean Ocean Water (V-SMOW) as follows:
where R is the or ratio.
Comparison of the measured stable isotope values of 160 replicate samples of ultrapure water and its standard composition (known , V-SMOW) showed that the measurement precision was <1 ‰ for δ2H and <0.3 ‰ for δ18O (Lis et al., 2008).
3.2 Hydrometeorological observations
The daily air temperature and evaporation data used in this study were obtained from the National Meteorological Reference Station in Changsha (station code: 57687), specifically utilizing the large evaporator model E-601B. It is worth noting that the evaporation recorded by the E-601B evaporator closely approximates the actual evaporation experienced in small water bodies such as lakes and rivers. As such, it reliably represents the quantity and temporal variations of evaporation within the study area (Hua et al., 2019).
Daily runoff discharge data were obtained from the Xiangtan hydrological station (station code: 61102000). Following the national standard Code for liquid flow measurement in open channels GB 50179-93 (Ministry of Water Resources of the People's Republic of China, 1993), the daily discharge values are calculated by applying the weighted average of intraday instantaneous discharge. This calculation is based on water level observations and a specific stage–discharge curve. As per the guidelines outlined in GB 50179-93, the relative errors in instantaneous discharge measurements range from 2 % for high water levels, 5 % for normal water levels, and 9 % for low water levels. On average, the estimated annual discharge falls within 5 % of the actual value. To facilitate the comparison of runoff discharge with precipitation and evaporation, the daily runoff discharge data (m3 d−1) are normalized by dividing them by the basin area (m2) of the measuring cross section. Consequently, the runoff depth (m d−1 or mm d−1) and runoff discharge data (m3 d−1) are computed and utilized in the subsequent analysis.
3.3 Model analysis
Because the samplings of precipitation and river water and the observation of hydrometeorological factors were conducted at the Changsha site, it is necessary to verify the representation of the Changsha site in the Xiangjiang River basin. For the aims of supporting the foundation and reducing the uncertainty of this study, a spatial correlation analysis was conducted between the Changsha site and the surrounding regions based on data from 1979 to 2021, including precipitation isotopes, precipitation amount, evaporation, and air temperature. The analysis employed the simulated precipitation isotope data generated by the iAWBM as detailed by Zhang et al. (2015), which has a spatial resolution of , while the air temperature, evaporation, and precipitation amount data are from the ERA5 reanalysis dataset (https://cds.climate.copernicus.eu, last access: 1 June 2023) published by the European Centre for Medium-Range Weather Forecasts (ECMWF), which has a spatial resolution of (Hersbach et al., 2023). Overall, all the data employed in this spatial correlation analysis were integrated into a 5 d interval.
With the aims of building the empirical relationships between the river water isotopes and the hydrometeorological factors and identifying the controlling factor that influences the river water isotopes, multiple linear regressions (MLRs) were used to build the prediction model of the river water isotopes. The variables include the precipitation isotope and the hydrometeorological factors such as precipitation amount, air temperature, evaporation, and runoff. Linear regression and a stepwise regression method were applied in MLRs using SPSS for Windows version 22.0 (SPSS Inc., SPSS Statistics 22.0). All the variables were taken as input variables, and one or more independent variables were retained in the prediction models.
4.1 Stable isotopic characteristics of precipitation and river water
Table 1 presents the monthly average values of air temperature (∘C), precipitation (mm), evaporation (mm), and runoff discharge (108 m3), and the results illustrate the uneven distribution of these factors throughout the year. Based on the monthly patterns of these hydrometeorological factors (Table 1) and the previous findings (Qin et al., 2006; Yao et al., 2016), four distinct runoff periods have been identified: a rainless period, a spring flood period, a major flood period, and a summer drought period. The rainless period spans from October to the following February, characterized by low air temperature, minimal precipitation, evaporation, and runoff discharge. In this period, the runoff discharge exhibits slight fluctuations except for isolated peaks resulting from major rainfall events. The spring flood period, occurring in March and April, marks an increase in runoff discharge, and this can be attributed to relatively higher precipitation amounts as well as moderate air temperature and evaporation in spring. The major flood period, extending from May to mid-July, exhibits a rapid surge in runoff discharge, often reaching peak values due to intensive precipitation events. The summer drought period spans from mid-July to September, while a significant decrease in runoff discharge is always observed. This decline can be attributed to high air temperature, leading to increased evaporation in this period. Additionally, the scarcity of precipitation events and relatively low precipitation amounts contribute to the reduced runoff discharge in this period. Overall, the analysis highlights the seasonal variations in air temperature, precipitation, evaporation, and runoff discharge, leading to distinct runoff periods throughout the year.
Table 1Monthly average air temperature (∘C), precipitation (mm), evaporation (mm), and runoff discharge (×108 m3).
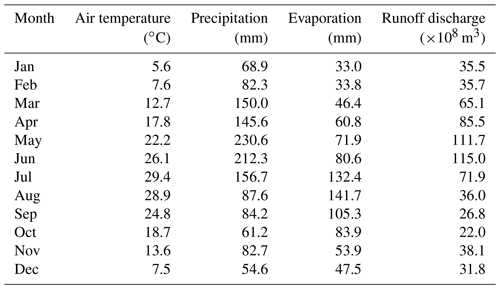
From 2010 to 2022, the 5 d volume-weighted precipitation δ2H (δ2HP) exhibited large seasonality throughout the year, as depicted in Figs. 2 and 3. Notably, the 5 d volume-weighted δ2HP ranged from −133.0 ‰ to 39.1 ‰, with a standard deviation of 27.6 ‰. The 5 d volume-weighted δ2HP showed maximum values in March, while the minimum values occurred in September (Fig. 2c). Furthermore, the 5 d volume-weighted δ2HP had the following order: spring flood period > rainless period > major flood period > summer drought period (Fig. 3b). The seasonal variations in the precipitation isotopes primarily resulted from different vapor sources, upstream effects, circulation patterns, and local meteorological factors in different seasons (H. Zhou et al., 2019; Xiao et al., 2023). The δ2H values of river water (δ2HR) ranged from −63.7 ‰ to −21.7 ‰, with a standard deviation of 6.1 ‰ (Figs. 2 and 3).
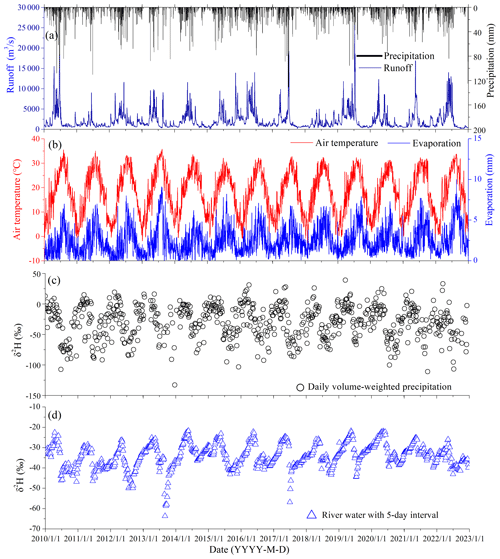
Figure 2Temporal variation of the daily runoff discharge and precipitation (a), daily air temperature and evaporation (b), daily volume-weighted precipitation δ2H (c), and river water δ2H with a 5 d interval (d).
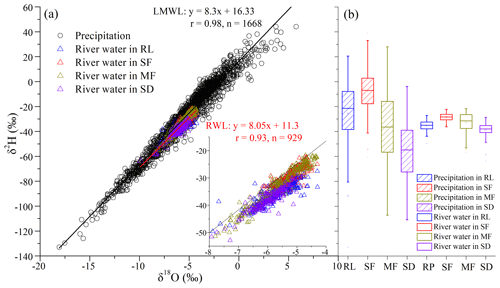
Figure 3Stable isotope composition (δ2H and δ18O) of daily volume-weighted precipitation and river water samples (a) and box plots of precipitation and river water δ2H (b) in different periods (rainless period, spring flood period, major flood period, and summer drought period). LMWL (black solid line) and RWL (red solid line) represent the local meteoric water line and river water line based on all the precipitation and river water isotopic data from January 2010 to December 2022, respectively. The letters (RL, SF, MF, and SD) on the x axis represent the rainless period, spring flood period, major flood period, and summer drought period, respectively.
The magnitude of δ2HR was ranked as follows: spring flood period, major flood period, rainless period, and summer drought period (Fig. 3b). The most depleted and enriched isotopic values in river water in the sampling period occurred on 1 September 2013 and 21 May 2014, respectively (Fig. 2). The seasonal variations in river water isotopes generally aligned with those observed in precipitation, indicating that river water directly derives from the precipitation input and is influenced by its isotopic composition. Moreover, the local meteoric water line (LMWL) and the river water line (RWL) have relatively close slopes of 8.3 and 8.05, respectively (Fig. 3a). However, the δ2HR exhibited a relatively smaller range of variation and a more attenuated temporal pattern compared to the δ2HP. This is likely due to the processes that precipitation undergoes before recharging river water, such as evaporation and mixing with older waters in the subsurface, which significantly reduce the variability in δ2HR (Xiao et al., 2022b, 2023).
4.2 Relationship between river water isotopes and various hydrometeorological factors
The relationships between the δ2HR and the corresponding 5 d accumulated precipitation and evaporation and average runoff discharge and air temperature in different periods are illustrated in Fig. 4. Based on the relationship between the 5 d volume-weighted δ2HP and the corresponding accumulated precipitation in different runoff periods (Fig. S1 in the Supplement; left panel), it is evident that an “amount effect” is observed in the precipitation isotopes across various runoff periods. Specifically, the increased precipitation amounts consistently result in more isotope-depleted precipitation. Furthermore, the δ2HR exhibits a positive correlation versus the corresponding 5 d volume-weighted δ2HP, with a correlation coefficient of 0.55 and p<0.001 (Fig. S2 in the Supplement). This suggests that precipitation input is the primary factor influencing δ2HR. However, as shown in Fig. 4, the δ2HR exhibited relatively weak correlations with the corresponding 5 d accumulated precipitations in the rainless period, major flood period, and summer drought period, as indicated by low correlation coefficient values and p>0.1 (Fig. 4a, i, and m). Although a positive correlation existed between δ2HR and the corresponding 5 d accumulated precipitations in the spring flood period, their correlation coefficients were not exceptionally high (Fig. 4e). The weak correlation between the δ2HR and the precipitation amount or runoff discharge can be attributed to the expansive area and water reserves in the Xiangjiang River basin – that is, after precipitation falls in the basin and the new input precipitation within 5 d may influence the δ2HR to some extent. However, it tends to mix with old waters, such as groundwater, soil water, and river water consisting of a high proportion of older water components in the subsurface flow paths (Xiao et al., 2023), thereby attenuating the impact of precipitation input and predominantly shaping the river water isotopes.
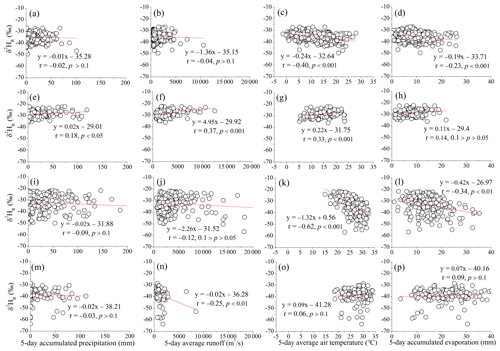
Figure 4Relationships between the river water δ2H and the corresponding 5 d accumulated precipitation (the first column), 5 d average runoff discharge (the second column), 5 d average air temperature (the third column), and 5 d accumulated evaporation (the fourth column) in different periods, and the first row (a, b, c, and d)), second row ((e, f, g, and h)), third row (i, j, k, and l), and fourth raw (m, n, o, and p) represent the rainless period, spring flood period, major flood period, and summer drought period, respectively.
The δ2HR exhibits a consistent relationship with the corresponding 5 d average air temperature and accumulated evaporation within each respective runoff period. However, positive or negative correlations may be observed in these relationships across the four runoff periods. Specifically, in the spring flood period and summer drought period, the δ2HR demonstrates either a highly significant (p<0.001) or non-significant ( or p>0.1) positive correlation with the corresponding 5 d average air temperature and accumulated evaporation (Fig. 4g, h and o, p). Conversely, in the rainless period and major flood period, a highly significant (p<0.001 or p<0.01) negative correlation is observed (Fig. 4c, d and k, i). The negative relationship between the δ2HR and the corresponding 5 d average air temperature or accumulated evaporation may seem counter-intuitive, as river water isotopes typically become enriched with increasing air temperature and progressing evaporation (Gibson et al., 2016; Jiang et al., 2021). This discrepancy can be explained by considering the seasonality of the relationship between precipitation isotopes and air temperature in different runoff periods (Fig. S1; right panel). For instance, the 5 d volume-weighted δ2HP gradually decreases as the corresponding average air temperature increases in the major flood period, but it increases as the corresponding average air temperature decreases in the rainless period (Figs. 2 and 3). This would lead to the negative relationship between the 5 d volume-weighted δ2HP and the corresponding average air temperature in the rainless period and major flood period (Fig. S1b and f), subsequently resulting in the negative relationship between the δ2HR and the corresponding 5 d average air temperature in these two periods (Fig. 4c, d and k, i). Moreover, as there is strong consistency between evaporation and air temperature (Allen et al., 2005), this alignment causes evaporation to also exhibit a negative correlation with δ2HR in these two periods (Fig. 4). Therefore, in the major flood period and rainless period, the influences of air temperature and evaporation on river water isotopes are somewhat masked by the seasonality of precipitation isotopes.
In the spring flood period and summer drought period, the river water isotopes exhibited an enrichment trend with the increasing corresponding average air temperature and accumulated evaporation (Fig. 4g, h and o, p). However, these relationships may be somewhat misleading due to the positive correlation observed between the 5 d volume-weighted δ2HP and the corresponding average air temperature in the spring flood period (p<0.05) and summer drought period (p>0.1) (Fig. S1d and h). This suggests that the positive correlation between river water isotopes and air temperature or evaporation may also be influenced by the seasonality of precipitation isotopes. Moreover, in the spring flood period, the increases in precipitation amount and runoff discharge lead to more isotope-enriched river water, as indicated in Fig. 4e and f. This phenomenon can be attributed to the most enriched precipitation isotopes occurring in the spring flood period (Figs. 2c and 3b), as the precipitation amount and runoff discharge gradually increase in this period (Fig. 2a). This contributes to the positive relationship observed between the δ2HR and the corresponding accumulated precipitation or average runoff discharge. Consequently, based on the findings of this section, when interpreting the relationships between the river water isotopes and hydrometeorological factors, it is important to consider the uncertainty arising from the seasonality of precipitation isotopes.
4.3 Relationship between the changes in river water isotopes and the precipitation and evaporation
As shown in Fig. 4, the relationship between the δ2HR and various factors may not be significant or may contradict common sense – that is, precipitation input and evaporation are likely the major driving factors that change the isotopic compositions of river water. For instance, the river water isotopes tend to be more depleted, and the slope of RWL tends to be high in heavy-precipitation events, while the river water generally exhibits gradually enriched isotopes and a lower evaporation line slope on rainless days with high air temperature and evaporation (Gibson et al., 2016; Yang et al., 2020; Jiang et al., 2021). Therefore, under the river water sample collection at a 5 d interval, it is essential to analyze the influences of the individual hydrometeorological factors on the changes in the δ2HR between two contiguous samplings (), which were calculated using the δ2HR differences between the δ2HR of the following and preceding samplings (i.e., ).
The 5 d runoff depth changes (i.e., ) and exhibited significant increases and decreases, respectively (p<0.001), in response to the 5 d accumulated precipitation (Fig. 5a and b). These findings indicate that precipitation input is the primary factor influencing the variations in runoff discharge and river water isotopes. Furthermore, based on the intersections between the linear fitting line and the x axis, the thresholds for precipitation amounts influencing the and ΔR at a 5 d interval were determined to be 19.0 and 19.4 mm, respectively. This suggests that heavier precipitation events are more likely to alter the river water isotopes. The weak correlation (p>0.1) between the and corresponding 5 d accumulated precipitation below the threshold value of 19.0 mm supports this observation (Fig. 5c). Conversely, when the 5 d accumulated precipitation exceeded 19.0 mm, a significant (p<0.001) negative correlation was observed between the and the corresponding 5 d accumulated precipitation (Fig. 5d). In other words, greater 5 d accumulated precipitation led to more negative δ2H values in the subsequent river water sample, with a correlation coefficient of −0.34 and p<0.001. Therefore, it can be concluded that the variation in river water isotopes reflects the isotopic signal of the input precipitation, and the isotopic composition of river water exhibits a significant amount effect by the precipitation input.
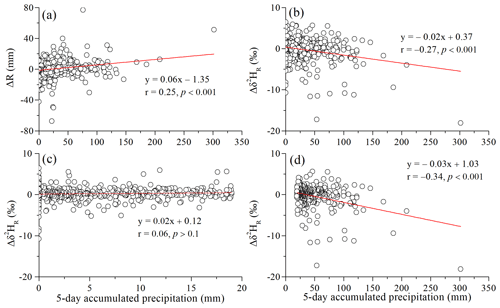
Figure 5Relationship between the 5 d accumulated precipitation and the changes in 5 d runoff discharge (ΔR) (a) and river water δ2H () (b), and the relationships between the and the corresponding 5 d accumulated precipitation <19.0 and >19.0 mm were shown in subplot (c) and (d), respectively.
Because of the confluence processes of the river water from upstream to downstream and the mixing processes between the new and old waters in the subsurface, river water may consist of a certain proportion of old water with a relatively long residence time (Xiao et al., 2022b, 2023), and thus we analyze the relationship between the river water isotopes and the hydrometeorological factors at a longer time interval. The relationships between the and the corresponding accumulated evaporation at various time intervals (5, 10, 15, 20, 25, and 30 d) are shown in Fig. 6. As there is a strong consistency between evaporation and air temperature (Allen et al., 2005), the relationship between the and air temperature was not analyzed in this paper. The results revealed a weak and statistically non-significant correlation (p>0.1) between the and corresponding accumulated evaporation at the 5, 10, 15, and 20 d intervals (Fig. 6a–d). However, a significant (p<0.05) negative correlation with relatively low correlation coefficients was observed at the 25 and 30 d intervals (Fig. 6e and f). The negative relationship between the and the corresponding accumulated evaporation (Fig. 6c–f) also contradicts the common sense that the river water generally becomes more enriched under the influences of evaporation. This may be due to the negative relationship between the and the corresponding average air temperature and accumulated evaporation in the rainless period and major flood period (Figs. 2 and 3) as discussed earlier. In addition, the effect of dilution precipitation input on river water isotopes (Figs. 5 and S1) and the relatively low air temperature and high relative humidity in the heavy-precipitation events may be greater than the enrichment effect of evaporation (e.g., similar to the effect demonstrated in Xiao et al., 2022a).
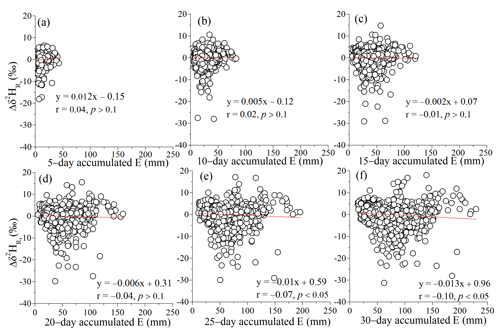
Figure 6Relationship between the changes in river water δ2H () and the corresponding accumulated evaporation (E) at 5 (a), 10 (b), 15 (c), 20 (d), 25 (e), and 30 d (f) time intervals.
To highlight the influences of evaporation on the river water isotopes and to eliminate the influence of precipitation input, we analyzed the relationship between the and the corresponding accumulated evaporation at different intervals (5, 10, 15, 20, 25, and 30 d) without precipitation input (Fig. 7). The findings revealed a significant (p<0.01 or p<0.001) positive correlation between these variables at different time intervals. In other words, as the accumulated evaporation increased, the also increased, with the correlation coefficient generally increasing with longer rainless intervals. Notably, the influence of greater evaporation on the was particularly pronounced at the 30 d interval, exhibiting a high correlation coefficient of 0.35 and a p value of less than 0.001, while these intervals occurred exclusively in 2013 and 2022, which were characterized as very dry years with high evaporation and multiple 30 d intervals devoid of precipitation (Figs. 2 and S3 in the Supplement).
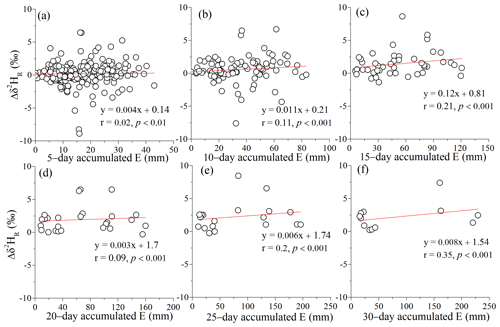
Figure 7Relationship between the changes in river water δ2H () and the corresponding accumulated evaporation (E) at 5 (a), 10 (b), 15 (c), 20 (d), 25 (e), and 30 (f) rainless time intervals.
The decrease in runoff discharge or water level in the basin can be attributed to evaporation within the basin, as evaporation increases the outflow component in the river water balance, leading to a reduction in the amount of water flowing out of the basin. Additionally, as runoff discharge can increase due to precipitation input, we examined the relationship between the and the corresponding changes in the runoff (ΔR) at different intervals (5, 10, 15, 20, 25, and 30 d) without precipitation input (Fig. 8). The results indicated a negative correlation between the and the corresponding ΔR at the 10, 15, 20, and 25 d intervals, demonstrating relatively high correlation coefficients around −0.4 and p values of less than 0.001. Considering the higher correlation coefficients observed between the and the corresponding ΔR (Fig. 8) compared to those between the and the corresponding accumulated evaporation (Fig. 7), it can be inferred that the ΔR serves as a suitable proxy to represent the effects of evaporation on river water isotopes. However, the correlation between the and the corresponding ΔR was relatively weak at the 5 d intervals, with correlation coefficients of −0.11 (Fig. 8a). Thus, this could be attributed to the short time interval and the limited impact of accumulated evaporation.
4.4 Influences of extreme drought and precipitation events on the river water isotopes
Analysis of the annual accumulated evaporation, average air temperature, accumulated precipitation, and average runoff discharge in the major flood period and summer drought period reveals that 2013 and 2022 experienced severe summer drought conditions (Fig. S3). These periods were characterized by exceptionally high temperatures and evaporation and low precipitation levels compared to the 13-year observations, particularly in 2022. The summer drought period of 2022 recorded only 16.3 mm of precipitation, the lowest among the 13-year observations and significantly lower than the highest precipitation recorded in the summer drought period of 2010 (i.e., 300.5 mm). Accumulated evaporation and average air temperature in the summer drought period of 2022 reached 385.1 mm and 28.8 ∘C, second only to the 393.7 mm and 29.8 ∘C recorded in the summer drought period of 2013 (Fig. 3). Furthermore, the extreme drought events in 2013 and 2022 have been extensively reported, indicating widespread meteorological, hydrological, and soil droughts that pose a significant threat to water resources for domestic, agricultural, ecological, and human needs (Ma et al., 2022; Bonaldo et al., 2023). Therefore, this section primarily focuses on these two extreme drought processes in 2013 and 2022.
As depicted in Fig. 9, the period from late June to mid-August 2013 witnessed rare precipitation, high evaporation rates, and elevated air temperatures. Consequently, the runoff discharge gradually decreased from 2699 m3 s−1 on 30 June to 415 m3 s−1 on 16 August. Simultaneously, the δ2HR progressively increased from −42.0 % to −34.8 % (Fig. 9e). Similarly, from mid-July to early November 2022, only 72.6 mm of precipitation was recorded, resulting in a gradual decline in runoff discharge until early September. In this process, the δ2HR increased from −53.1 % on 16 July to −37.9 % on 6 September (Fig. 9f). Subsequently, the Xiangjiang River maintained low runoff discharge and raised δ2HR levels until the end of December, and the δ2HR increased by up to 20.2 ‰ from −53.1 % on 16 July to −32.9 ‰ on 26 November 2022 (Fig. 9f). These findings align with the results obtained in the previous section, indicating that decreases in runoff discharge and higher evaporation rates on long rainless days contribute to the gradual enrichment of river water isotopes. However, it is noteworthy that the δ2HR range (−63.7 ‰ to −21.7 ‰) mentioned earlier includes the most positive δ2HR values influenced by the extreme drought events in 2013 and 2022 (Fig. 2d), while the most isotope-enriched river water occurred on 21 May 2014 (Fig. 2d) and the most isotope-enriched precipitation occurred in the spring flood period (Fig. 3b). This indicates that the input of relatively enriched spring precipitation isotopes plays a crucial role in controlling the isotopic enrichment of river water.
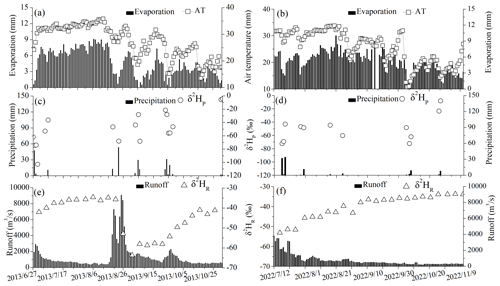
Figure 9Temporal variations of evaporation and air temperature (a, b), daily precipitation amount and precipitation δ2H (δ2HP) (c, d), and runoff discharge and river water δ2H (δ2HR) (e, f) in the extreme drought processes in 2013 (left panel) and 2017 (right panel).
By examining the 13-year observations and ranking the 5 d accumulated precipitation, we found that the maximum accumulated precipitation, totaling 301.6 mm, occurred from 27 June to 1 July 2017 (Fig. 2). Notably, on 30 June and 1 July 2017, daily precipitation reached 146.4 and 130.3 mm, respectively. Additionally, between 21 and 26 June 2017, the precipitation amount reached 185.5 mm. Another significant precipitation event took place in June 2011, when the total precipitation for the month reached 340.3 mm, with a single-day rainfall of 110.4 mm on 28 June. To analyze the impact of extreme precipitation on river water isotopes in the major flood period, the temperature, evaporation, precipitation, runoff discharge, δ2HP, and δ2HR in these two extreme-precipitation processes are presented in Fig. 10.
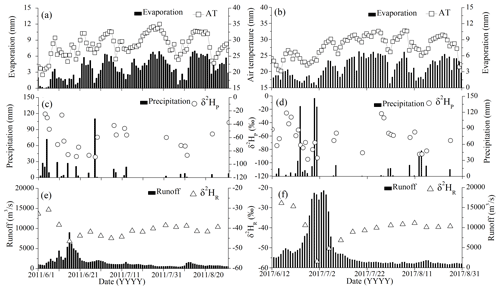
Figure 10Temporal variations of the evaporation and air temperature (a, b), precipitation amount and precipitation δ2H (c, d), and runoff discharge and river water δ2H (e, f) in the extreme-precipitation processes and the following summer drought period in 2011 (left panel) and 2017 (right panel).
In the heavy-precipitation period from 5 to 16 June 2011, the runoff discharge increased from 1410 to 9010 m3 s−1, while the δ2HR decreased from −30.8 ‰ to −46.5 ‰ (Fig. 10e). Similarly, from 21 June to 1 July 2017, the runoff discharge and river water isotopes exhibited significant fluctuations and depletion under the influence of precipitation input (Fig. 10f). For instance, the runoff discharge increased from 3961 to 18 237 m3 s−1, while the δ2HR decreased from −29.3 ‰ to −56.8 ‰, which represents the third-lowest δ2HR value among the 13-year observations. In both extreme-precipitation processes, the precipitation isotopes were relatively depleted (Fig. 10c and d). Specifically, the volume-weighted δ2HP was −50.9 ‰ from 5 to 16 June 2011, and the value was −76.7 ‰ from 21 June to 1 July 2017. This indicates that the input of extreme precipitation leads to rapid decreases in δ2HR. For instance, in the extreme-precipitation process of June 2011, the δ2HR was reduced by −15.7 ‰ under a precipitation input of 144.7 mm from 5 to 16 June, while the δ2HR decreased by −27.5 ‰ with a precipitation input of 487.1 mm in the extreme-precipitation process from 21 June to 1 July 2017. Subsequently, in the summer drought periods of 2011 and 2017, the river water isotope was gradually enriched to approximately −38 % (Fig. 10e and f).
River water isotopes serve as a valuable record not only for detecting the isotopic depletion signal of extreme-precipitation input in the major flood period (Fig. 10), but also for capturing the influence of moderate precipitation in the summer drought period. In the latter case, the river water isotopes gradually become enriched with the decrease in the runoff discharge in prolonged periods of drought. When the basin is relatively dry, i.e., the reserves of soil water, groundwater, and river water are limited, the river water may be influenced by a medium precipitation amount resulting in a highly depleted river water isotope signal observed in this extended river water sample series. For instance, following a precipitation input of 53.5 mm with a δ2HP of −68.1 ‰ on 23 August 2013 (Fig. 9c), the river water isotopes exhibited a significant depletion (Fig. 9e). Notably, the δ2HR rapidly declined from −35.7 ‰ on 21 August to −63.7 ‰ on 1 September, representing the most negative δ2HR value observed over the 13-year observations. This δ2HR signal differs significantly from rapid decreases in δ2HR caused by extreme-precipitation input in the river water (Fig. 10), highlighting the importance of careful consideration when reconstructing precipitation based on isotopic signals derived from river water.
5.1 Factors that influence the seasonality in river water isotopes
By examining the relationship between the and the corresponding accumulated evaporation, it becomes evident that a stronger correlation between the two variables emerges at time intervals exceeding 10 d (Fig. 6). This suggests that the influence of evaporation on river water isotopes manifests over relatively long time intervals, spanning from tens of days to even several months, particularly in long dry periods without precipitation input (Fig. 9). The influences of evaporation on river water isotopes occur gradually, making it challenging to capture using short-term analyses. Conversely, as indicated by Xiao et al. (2022b, 2023), the relatively weak influence of evaporation on river water isotopes observed at short intervals (Figs. 6 and 7) can be attributed to the significant influx of precipitation input (i.e., new water) rapidly flowing into the river network, which experiences limited evaporation effects in the relatively short residence time. Furthermore, the variation in runoff discharge exhibits a notable relationship with the (Fig. 8); however, it is important to note that the decline in runoff discharge may not be solely due to evaporation but could also be influenced by water transport from the Xiangjiang River to Dongting Lake due to rapid downstream drainage (Zhan et al., 2015). This introduces uncertainties in the analysis based on the changes in runoff discharge and river water isotopes between different time intervals.
The independent variables, including volume-weighted precipitation isotopes (δ2HP), accumulated precipitation (P), accumulated evaporation (E), changes in runoff depth (ΔR), average air temperature (Tave), maximum air temperature (Tmax), and minimum air temperature (Tave), were used to build the prediction model of the dependent variable (i.e., the river water isotopes). According to the correlations between the river water isotopes and the hydrometeorological factors (Figs. 4–8), the changes in the δ2HR () and a 15 d time interval were used as the dependent variable and the time step, respectively, of all the variables in the MLRs. As indicated by the regression equations in the different runoff periods (Eqs. 2–5), the river water isotopes showed strong seasonality and were controlled by different factors. For instance, in the major flood period and summer drought period, the δ2HR usually reflected heavy-precipitation inputs with depleted isotopes, as supported by the negative correlation between the and ΔR or accumulated precipitation (Eqs. 2 and 3), indicating the amount effect by the precipitation input. The amount effect of water stable isotopes was widely observed around the world, especially in the regions with flash input of depleted precipitation (e.g., Dansgaard, 1964; H. Zhou et al., 2019). Specifically, the precipitation isotopes were relatively depleted in these two periods (Fig. 3b), while the river water isotopes captured the precipitation input signal, particularly when the accumulated precipitation exceeded the threshold precipitation amount (Fig. 5). Additionally, the extreme-precipitation events mainly occurred in the major flood period, resulting in relatively isotope-depleted precipitation that was reflected as negative records in the δ2HR (Figs. 2 and 10, Eq. 2).
In the rainless period, the δ2HR values were more positive compared to the summer drought period (Fig. 3b), possibly influenced by the input of more enriched precipitation and evaporation enrichment along with the decreases in ΔR (Eq. 4). In addition, the δ2HR reached its highest positive values in the spring flood period, influenced by the precipitation input with relatively depleted isotopes (Figs. 2 and 3), while an “inverse amount effect” and an “inverse temperature effect” were found in this period, as indicated by the positive correlation between the and accumulated precipitation and the negative correlation between the and air temperature (Tmax) (Eq. 5), and the reasons can be attributed to the seasonality of precipitation isotopes and air temperature as discussed in Sect. 4.3. Overall, the river water isotopes in the Xiangjiang River basin are controlled by various complex factors in different runoff periods, while such findings in the controlling factors that influence river water isotopes may be beneficial in paleoclimate reconstruction and establishment of isotope hydrologic models.
5.2 Environmental significance implied by the seasonality of river water isotopes
The Xiangjiang River, serving as a significant inflow water source, exerts an influence on the hydrologic and isotope mass balance of Dongting Lake, the second-largest freshwater lake in China (Zhan et al., 2015; H. Zhou et al., 2019). The isotopic composition of lake water primarily reflects the input waters, including lake surface precipitation and inflowing river water (Steinman and Abbott, 2013; Gibson et al., 2016; Xiao et al., 2022a), while the isotopic information in lake water can also influence the stable isotopic signatures preserved in lake sediment. Consequently, proxy indicators recorded in lake sediments can be utilized for paleoclimate reconstruction, benefiting from the relationships between the input water isotopes and the local environments.
Through the analysis of river water isotopes and various hydrometeorological factors on a seasonal scale, it becomes evident that the can reflect the corresponding accumulated evaporation and precipitation input (Figs. 5–7) and the decline in runoff discharge (Fig. 8) at the observed time intervals. Moreover, river water isotopes entering the lakes can record signals of extreme precipitation (Fig. 10) or exhibit gradual isotopic enrichment under the influence of evaporation in relatively dry periods spanning tens of days or even several months without precipitation (Fig. 9). In addition, the isotopic characteristics of precipitation are governed by large-scale factors such as moisture sources, upstream effects, and circulation patterns and are less influenced by local meteorological factors (Aggarwal et al., 2016; H. Zhou et al., 2019), and thus the river water isotopes are better suited to reflecting local environments. Consequently, in comparison to the isotopic characteristics of precipitation, the river water isotopes may provide valuable insights into the relationship between the proxy indicators and the local environments.
In previous studies, due to limited data availability, the inflow water isotopes of the lake were often represented by the volume-weighted precipitation isotopes in hydrologic and isotope-mass-balance models (e.g., Steinman and Abbott, 2013; Skrzypek et al., 2015; Jones et al., 2016). However, based on the 13-year observations conducted in this study, the annual volume-weighted δ2HR and δ2HP were found to closely match only in the period of 2012–2015, with differences within 2 ‰ (Table 2). In other years, the annual volume-weighted δ2HP was either more negative or more positive compared to the annual volume-weighted δ2HR. These differences in the annual δ2HR and δ2HP were mainly influenced by the seasonality of δ2HP and precipitation amount. For instance, significant variations were observed between the volume-weighted δ2HP and δ2HR in the different runoff periods (Table 2). Therefore, representing the inflow water of the lake solely by the annual volume-weighted precipitation isotopes can only serve as a rough estimation. To accurately depict the detailed variations in the lake hydrologic and isotope mass balance, more comprehensive observations of the inflowing river water are required.
Table 2Annual volume-weighted precipitation δ2H and river water δ2H (δ2HR and δ2HP) and volume-weighted average values in different runoff periods. The letters (RL, SF, MF, and SD) represent the rainless period, spring flood period, major flood period, and summer drought period, respectively.
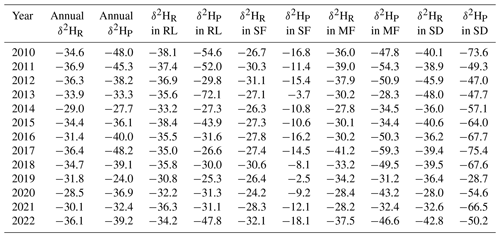
Nevertheless, according to the analysis on an annual scale based on the 13-year observations, the volume-weighted δ2HR values in the different runoff periods exhibited non-significant correlations ( or p>0.1) with the corresponding total precipitation, average runoff discharge, average air temperature, and total evaporation (Fig. S4 in the Supplement). Although this study encompasses 13-year observations with a sampling interval of 5 d, there is a need for longer and systematic observations of various water types and hydrometeorological factors, spanning decadal or longer timescales, to better elucidate the relationships between river water isotopes and local environments on the annual scale.
5.3 The representation of the observation at the Changsha site in the Xiangjiang River basin
The increases in runoff discharge and water level in the Changsha section of the Xiangjiang River are primarily attributed to the precipitation recharge in the middle and upper reaches, while the influences of evaporation on river water isotopes mainly occur within the basin. It should be noted that the sampling and observation sites for precipitation, air temperature, and evaporation in this study, located at Hunan Normal University and the National Meteorological Reference Station in Changsha, respectively, only represent the local conditions in Changsha. Therefore, the extent of their influence on the runoff discharge and water stable isotopes of the entire Xiangjiang River may be limited. To assess whether the sampling and observations in Changsha can adequately represent the Xiangjiang River basin, the spatial correlation analysis based on the iAWBM was conducted, and the results of air temperature (Fig. 11a), evaporation (Fig. 11b), precipitation amount (Fig. 11c), and precipitation isotopes (Fig. 11d), respectively, were shown.
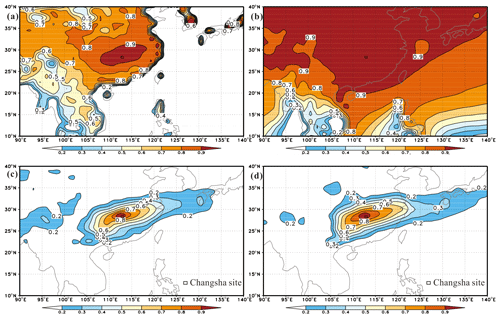
Figure 11Spatial correlation analysis of the air temperature (a), evaporation (b), precipitation amount (c), and precipitation isotope (d) between the Changsha (CS) site and the surrounding regions at a 5 d interval. This analysis employed the simulated precipitation isotope data generated by the isotopic Atmospheric Water Balance Model (iAWBM) (Zhang et al., 2015) and the air temperature, evaporation, and precipitation amount data from the ERA5 reanalysis dataset.
The results of spatial correlation analysis revealed a high correlation between the reanalysis data of air temperature and evaporation at the Changsha site and those in the surrounding regions, with correlation coefficients above 0.8 and p<0.001 for the grid points in the Xiangjiang River basin (Fig. 11a and b). Furthermore, while the relationship between the reanalysis data of precipitation amount and simulated precipitation isotopes at the Changsha site and in the surrounding regions is not as strong as that for air temperature and evaporation, the correlation is still high (Fig. 11c and d). For instance, the correlation coefficients between the Changsha site and the grid points in the Xiangjiang River basin exceed 0.7 with p<0.001 for both the reanalysis data of the precipitation amount and the simulated precipitation isotopes (Fig. 11c and d).
Overall, the high correlation coefficients for these factors support the time consistency between the Changsha site and the surrounding regions, while the high spatial consistency is characterized by the large area of the high correlation coefficients, which covers the whole Xiangjiang River basin. These may be related to the fact that the Xiangjiang River basin as a whole is located in the subtropical zone of southern China, which makes the meteorological elements (precipitation, evaporation, temperature) of the basin have a certain consistency. Moreover, the precipitation isotope of the basin also has a high correlation, which can be attributed to the uniform water vapor source, precipitation form, and atmospheric circulation within the Xiangjiang River basin (H. Zhou et al., 2019; Liu et al., 2023). These spatial correlation analyses provide support for the study basis of using the observations at the Changsha site as representative of the Xiangjiang River basin.
The main findings of this study are as follows. (1) Both the δ2HP and δ2HR displayed significant seasonal variation throughout the year. The temporal pattern of the δ2HR was similar but attenuated compared to the δ2HP, indicating the influences of direct precipitation input, evaporation, and mixing with older waters. (2) The δ2HR showed weak correlations with the corresponding hydrometeorological factors, which can be attributed to the mixing between the new input precipitation and the old waters within the basin and the seasonality of the precipitation isotopes and air temperature. (3) The ΔR and exhibited significant responses to the corresponding accumulated precipitation, with heavier precipitation events more likely to alter runoff discharge and river water isotopes. However, the input of moderate precipitation leads to the most negative δ2HR in the summer drought period, when wetness conditions are limited. (4) The showed a notably positive correlation with the corresponding accumulated evaporation and average ΔR, particularly at longer time intervals without precipitation input, but the spring flood period shows the most positive δ2HR due to the input of enriched precipitation isotopes. (5) In the major flood period, the δ2HR may rapidly decrease with a maximum range of −27.5 ‰ due to the input of extreme precipitation with relatively depleted isotopes, while the river water isotopes were gradually enriched in the subsequent summer drought period. In the summer drought periods of 2013 and 2022, the runoff discharge and δ2HR showed a gradual decrease and increase, respectively, with the δ2HR potentially increasing by up to 20.2 ‰ and influenced by the extreme drought.
The main contributions and scientific values of these findings can be summarized as follows. (1) Better than δ2HR, the can serve as a proxy that was influenced by the local environment. (2) Caution is advised when interpreting extreme isotopic signals in river water due to complex influences of hydrometeorological factors. (3) River water isotopes are controlled by various complex factors, which may show an “amount effect” and an “inverse amount effect” in different runoff periods. (4) The spatial correlation analysis based on the iAWBM confirms that the site observations are representative of the Xiangjiang River basin. These implications may be valuable for water stable isotopes applied in future paleoclimate reconstruction and for establishment of the isotope hydrologic models. To enhance the reliability of the observations and to ensure their representativeness for the entire Xiangjiang River basin, further spatial measurements of hydrometeorological factors and sampling of precipitation samples are needed within the basin itself. Furthermore, the more extensive sampling of river water (e.g., daily sampling) should be conducted in future fieldwork with the aim of a more detailed depiction and interpretation of the seasonal variation and influencing factors of river water isotopes.
The data and code that support the findings of this study are available from the corresponding author upon reasonable request.
The supplement related to this article is available online at: https://doi.org/10.5194/hess-27-3783-2023-supplement.
XX: data curation, methodology, software, writing – original draft, and editing. XZ: methodology, writing – original draft, and editing. ZX: data curation. ZR, XH, and CZ: methodology.
The contact author has declared that none of the authors has any competing interests.
Publisher's note: Copernicus Publications remains neutral with regard to jurisdictional claims made in the text, published maps, institutional affiliations, or any other geographical representation in this paper. While Copernicus Publications makes every effort to include appropriate place names, the final responsibility lies with the authors.
This study was supported by the Natural Science Foundation of Hunan Province, China (grant no. 2023JJ40445), and the National Natural Science Foundation of China (grant no. 42101130). We are grateful to the graduate students who laboriously sampled water samples without interruption and tested water stable isotopes in the 13 hydrological years.
This research was supported by the Natural Science Foundation of Hunan Province (grant no. 2023JJ40445) and the National Natural Science Foundation of China (grant no. 42101130).
This paper was edited by Natalie Orlowski and reviewed by two anonymous referees.
Aggarwal, P. K., Romatschke, U., Araguas-Araguas, L., Belachew, D., Longstaffe, F. J., Berg, P., Schumacher, C., and Funk, A.: Proportions of convective and stratiform precipitation revealed in water isotope ratios, Nat. Geosci., 9, 624–629, https://doi.org/10.1038/ngeo2739, 2016.
Allen, R. G., Pereira, L. S., Smith, M., Raes, D., and Wright, J. L.: FAO-56 dual crop coefficient method for estimating evaporation from soil and application extensions, J. Irrig. Drain. Eng., 131, 2–13, https://doi.org/10.1061/(ASCE)0733-9437(2005)131:1(2), 2005.
Blöschl, G.: Hydrologic synthesis: Across processes, places, and scales, Water Resour. Res., 42, 1–3, https://doi.org/10.1029/2005WR004319, 2006.
Bonaldo, D., Bellafiore, D., Ferrarin, C., Ferretti, R., Ricchi, A., Sangelantoni, L., and Vitelletti, M. L.: The summer 2022 drought: a taste of future climate for the Po valley (Italy), Reg. Environ. Change, 23, 1–6, https://doi.org/10.1007/s10113-022-02004-z, 2023.
Boral, S., Sen, I. S., Ghosal, D., Peucker-Ehrenbrink, B., and Hemingway, J. D.: Stable water isotope modeling reveals spatio-temporal variability of glacier meltwater contributions to Ganges River headwaters, J. Hydrol., 577, 123983, https://doi.org/10.1016/j.jhydrol.2019.123983, 2019.
Boutt, D. F., Mabee, S. B., and Yu, Q.: Multiyear increase in the stable isotopic composition of stream water from groundwater recharge due to extreme precipitation, Geophys. Res. Lett., 46, 5323–5330, https://doi.org/10.1029/2019GL082828, 2019.
Cardoso Pereira, S., Marta-Almeida, M., Carvalho, A. C., and Rocha, A.: Extreme precipitation events under climate change in the Iberian Peninsula, Int. J. Climatol., 40, 1255–1278, https://doi.org/10.1002/joc.6269, 2020.
Cook, B. I., Mankin, J. S., and Anchukaitis, K. J.: Climate change and drought: From past to future, Curr. Clim. Change Rep., 4, 164–179, https://doi.org/10.1007/s40641-018-0093-2, 2018.
Craig, H.: Standard for reporting concentrations of deuterium and oxygen-18 in natural waters, Science, 133, 1833–1834, https://doi.org/10.1126/science.133.3465.1702, 1961.
Dansgaard, W.: Stable isotopes in precipitation, Tellus, 16, 436–468, https://doi.org/10.3402/tellusa.v16i4.8993, 1964.
Das, S. and Rai, S. K.: Stable isotopic variations (δD and δ18O) in a mountainous river with rapidly changing altitude: Insight into the hydrological processes and rainout in the basin, Hydrol. Process., 36, e14547, https://doi.org/10.1002/hyp.14547, 2022.
Devia, G. K., Ganasri, B. P., and Dwarakish, G. S.: A review on hydrological models, Aquat. Pr., 4, 1001–1007, https://doi.org/10.1016/j.aqpro.2015.02.126, 2015.
Emmanouilidis, A., Katrantsiotis, C., Dotsika, E., Kokkalas, S., Unkel, I., and Avramidis, P.: Holocene paleoclimate variability in the eastern Mediterranean, inferred from the multi-proxy record of Lake Vouliagmeni, Greece, Palaeogeogr. Palaeocl., 595, 110964, https://doi.org/10.1016/j.palaeo.2022.110964, 2022.
Gibson, J. J., Birks, S. J., and Yi, Y.: Stable isotope mass balance of lakes: a contemporary perspective, Quaternary Sci. Rev., 131, 316–328, https://doi.org/10.1016/j.quascirev.2015.04.013, 2016.
Grillakis, M. G.: Increase in severe and extreme soil moisture droughts for Europe under climate change, Sci. Total Environ., 660, 1245–1255, https://doi.org/10.1016/j.scitotenv.2019.01.001, 2019.
Hersbach, H., Bell, B., Berrisford, P., Biavati, G., Horányi, A., Muñoz Sabater, J., Nicolas, J., Peubey, C., Radu, R., Rozum, I., Schepers, D., Simmons, A., Soci, C., Dee, D., and Thépaut, J.-N.: ERA5 hourly data on single levels from 1940 to present, Copernicus Climate Change Service (C3S) Climate Data Store (CDS) [data set], https://doi.org/10.24381/cds.adbb2d47, 2023.
Hua, M., Zhang, X., Yao, T., Luo, Z., Zhou, H., Rao, Z., and He, X.: Dual effects of precipitation and evaporation on lake water stable isotope composition in the monsoon region, Hydrol. Process., 33, 2192–2205, https://doi.org/10.1002/hyp.13462, 2019.
Huang, R., Xu, L., Yuan, X., Lu, R., Sung-Euii, M., and Ung-Jun, K.: Seasonal prediction experiments of the summer droughts and floods during the early 1990's in East Asia with numerical models, Adv. Atmos. Sci., 15, 433–446, https://doi.org/10.1007/s00376-998-0025-5, 1998.
Jiang, D., Li, Z., Luo, Y., and Xia, Y.: River damming and drought affect water cycle dynamics in an ephemeral river based on stable isotopes: The Dagu River of North China, Sci. Total Environ., 758, 143682, https://doi.org/10.1016/j.scitotenv.2020.143682, 2021.
Jiménez-Iñiguez, A., Ampuero, A., Valencia, B. G., Mayta, V. C., Cruz, F. W., Vuille, M., Novello, V. F., Misailidis, S. N., Aranda, N., and Conicelli, B.: Stable isotope variability of precipitation and cave drip-water at Jumandy cave, western Amazon River basin (Ecuador), J. Hydrol., 610, 127848, https://doi.org/10.1016/j.jhydrol.2022.127848, 2022.
Jones, M. D., Cuthbert, M. O., Leng, M. J., McGowan, S., Mariethoz, G., Arrowsmith, C., Sloane, H. J., Humphrey, K. K., and Cross, I.: Comparisons of observed and modelled lake δ18O variability, Quaternary Sci. Rev., 131, 329–340, https://doi.org/10.1016/j.quascirev.2015.09.012, 2016.
Kendall, C. and Coplen, T. B.: Distribution of oxygen-18 and deuterium in river waters across the United States, Hydrol. Process., 15, 1363–1393, https://doi.org/10.1002/hyp.217, 2001.
Lis, G., Wassenaar, L. I., and Hendry, M. J.: High-precision laser spectroscopy and measurements of microliter natural water samples, Anal. Chem., 80, 287–293, https://doi.org/10.1021/ac701716q, 2008.
Liu, Z., Zhang, X., Xiao, Z., He, X., Rao, Z., and Guan, H.: The relations between summer droughts/floods and oxygen isotope composition of precipitation in the Dongting Lake basin, Int. J. Climatol., 43, 3590–3604, https://doi.org/10.1002/joc.8047, 2023.
Ma, M., Qu, Y., Lyu, J., Zhang, X., Su, Z., Gao, H., Yang, X., Chen, X., Jiang, T., Zhang, J., Shen, M., and Wang, Z.: The 2022 extreme drought in the Yangtze River Basin: Characteristics, causes and response strategies, River, 1, 162–171, https://doi.org/10.1002/rvr2.23, 2022.
Marengo, J. A., Alves, L. M., Ambrizzi, T., Young, A., Barreto, N. J., and Ramos, A. M.: Trends in extreme rainfall and hydrogeometeorological disasters in the Metropolitan Area of São Paulo: a review, Ann. N. Y. Acad. Sci., 1472, 5–20, https://doi.org/10.1111/nyas.14307, 2020.
Ministry of Water Resources of the People's Republic of China: Code for liquid flow measurement in open channels GB 50179-93, China Planning Press, Beijing, ISBN 9788005887000, 1993 (in Chinese).
Muñoz-Villers, L. E. and McDonnell, J. J.: Land use change effects on runoff generation in a humid tropical montane cloud forest region, Hydrol. Earth Syst. Sci., 17, 3543–3560, https://doi.org/10.5194/hess-17-3543-2013, 2013.
Nan, Y., Tian, F., Hu, H., Wang, L., and Zhao, S.: Stable isotope composition of river waters across the world, Water, 11, 1760, https://doi.org/10.3390/w11091760, 2019.
Nkemelang, T., New, M., and Zaroug, M.: Temperature and precipitation extremes under current, 1.5 ∘C and 2.0 ∘C global warming above pre-industrial levels over Botswana, and implications for climate change vulnerability, Environ. Res. Lett., 13, 065016, https://doi.org/10.1088/1748-9326/aac2f8, 2018.
Pechlivanidis, I. G., Jackson, B. M., Mcintyre, N. R., and Wheater, H. S.: Catchment scale hydrological modelling: A review of model types, calibration approaches and uncertainty analysis methods in the context of recent developments in technology and applications, Global NEST J., 13, 193–214, https://doi.org/10.1007/s10393-012-0741-2, 2011.
Qin, S. H., Li, X. D., and Hu, D. S.: Hydrogeology of Hunan Province, Hunan hydrology and water resources Survey Bureau, China Water and Power Press, Beijing, 42–50, ISBN 9787508440637, 2006 (in Chinese).
Ren, W., Tian, L., and Shao, L.: Temperature and precipitation control the seasonal patterns of discharge and water isotopic signals of the Nyang River on the southeastern Tibetan Plateau, J. Hydrol., 617, 129064, https://doi.org/10.1016/j.jhydrol.2023.129064, 2023.
Rode, M., Wade, A. J., Cohen, M. J., Hensley, R. T., Bowes, M. J., Kirchner, J. W., Arhonditsis, G. B., Jordan, P., Kronvang, B., Halliday, S. J., Skeffington, R. A., Rozemeijer, J. C., Aubert, A. H., Rinke, K., and Jomaa, S.: Sensors in the stream: the high-frequency wave of the present, Environ. Sci. Technol., 50, 10297–10307, https://doi.org/10.1021/acs.est.6b02155, 2016.
Saranya, P., Krishnakumar, A., Kumar, S., and Krishnan, K. A.: Isotopic study on the effect of reservoirs and drought on water cycle dynamics in the tropical Periyar basin draining the slopes of Western Ghats, J. Hydrol., 581, 124421, https://doi.org/10.1016/j.jhydrol.2019.124421, 2020.
Scholl, M., Shanley, J., Murphy, S., Willenbring, J., Occhi, M., and González, G.: Stable-isotope and solute-chemistry approaches to flow characterization in a forested tropical watershed, Luquillo Mountains, Puerto Rico, Appl. Geochem., 63, 484–497, https://doi.org/10.1016/j.apgeochem.2015.03.008, 2015.
Seyfried, M. S. and Wilcox, B. P.: Scale and the nature of spatial variability: Field examples having implications for hydrologic modeling, Water Resour. Res., 31, 173–184, https://doi.org/10.1029/94WR02025, 1995.
Sinha, N. and Chakraborty, S.: Isotopic interaction and source moisture control on the isotopic composition of rainfall over the Bay of Bengal, Atmos. Res., 235, 104760, https://doi.org/10.1016/j.atmosres.2019.104760, 2020.
Skrzypek, G., Mydłowski, A., Dogramaci, S., Hedley, P., Gibson, J. J., and Grierson, P. F.: Estimation of evaporative loss based on the stable isotope composition of water using Hydrocalculator, J. Hydrol., 523, 781–789, https://doi.org/10.1016/j.jhydrol.2015.02.010, 2015.
Sprenger, M., Llorens, P., Gallart, F., Benettin, P., Allen, S. T., and Latron, J.: Precipitation fate and transport in a Mediterranean catchment through models calibrated on plant and stream water isotope data, Hydrol. Earth Syst. Sci., 26, 4093–4107, https://doi.org/10.5194/hess-26-4093-2022, 2022.
Steinman, B. A. and Abbott, M. B.: Isotopic and hydrologic responses of small, closed lakes to climate variability: Hydroclimate reconstructions from lake sediment oxygen isotope records and mass balance models, Geochim. Cosmochim. Ac., 105, 342–359, https://doi.org/10.1016/j.gca.2012.11.027, 2013.
Steinman, B. A., Rosenmeier, M. F., Abbott, M. B., and Bain, D. J.: The isotopic and hydrologic response of small, closed-basin lakes to climate forcing from predictive models: Application to paleoclimate studies in the upper Columbia River basin, Limnol. Oceanogr., 55, 2231–2245, https://doi.org/10.4319/lo.2010.55.6.2231, 2010.
Streletskiy, D. A., Tananaev, N. I., Opel, T., Shiklomanov, N. I., Nyland, K. E., Streletskaya, I. D., and Shiklomanov, A. I.: Permafrost hydrology in changing climatic conditions: seasonal variability of stable isotope composition in rivers in discontinuous permafrost, Environ. Res. Lett., 10, 095003, https://doi.org/10.1088/1748-9326/10/9/095003, 2015.
Sun, Z., Zhu, G., Zhang, Z., Xu, Y., Yong, L., Wan, Q., Ma, H., Sang, L., and Liu, Y.: Identifying surface water evaporation loss of inland river basin based on evaporation enrichment model, Hydrol. Process., 35, e14093, https://doi.org/10.1002/hyp.14093, 2021.
Uchiyama, R., Okochi, H., Ogata, H., Katsumi, N., Asai, D., and Nakano, T.: H and O isotopic differences in typhon and urban-induced heavy rain in Tokyo, Environ. Chem. Lett., 15, 739–745, https://doi.org/10.1007/s10311-017-0652-0, 2017.
von Freyberg, J., Studer, B., and Kirchner, J. W.: A lab in the field: high-frequency analysis of water quality and stable isotopes in stream water and precipitation, Hydrol. Earth Syst. Sci., 21, 1721–1739, https://doi.org/10.5194/hess-21-1721-2017, 2017.
von Freyberg, J., Rücker, A., Zappa, M., Schlumpf, A., Studer, B., and Kirchner, J. W.: Four years of daily stable water isotope data in stream water and precipitation from three Swiss catchments, Sci. Data, 9, 46, https://doi.org/10.1038/s41597-022-01148-1, 2022.
Wang, L., Dong, Y., Xie, Y., and Chen, M.: Hydrological processes and water quality in arid regions of Central Asia: insights from stable isotopes and hydrochemistry of precipitation, river water, and groundwater, Hydrogeol. J., 23, 1–17, https://doi.org/10.1007/s10040-023-02654-1, 2023.
Wang, Q., Huang, G., Wang, L., Piao, J., Ma, T., Hu, P., Chotamonsak, C., and Limsakul, A.: Mechanism of the summer rainfall variation in Transitional Climate Zone in East Asia from the perspective of moisture supply during 1979–2010 based on the Lagrangian method, Clim. Dynam., 60, 1225–1238, https://doi.org/10.1007/s00382-022-06344-8, 2023.
Wu, H., Huang, Q., Fu, C., Song, F., Liu, J., and Li, J.: Stable isotope signatures of river and lake water from Poyang Lake, China: Implications for river–lake interactions, J. Hydrol., 592, 125619, https://doi.org/10.1016/j.jhydrol.2020.125619, 2021.
Xiao, X., Zhang, F., Che, T., Shi, X., Zeng, C., and Wang, G.: Changes in plot-scale runoff generation processes from the spring–summer transition period to the summer months in a permafrost-dominated catchment, J. Hydrol., 587, 124966, https://doi.org/10.1016/j.jhydrol.2020.124966, 2020.
Xiao, X., Zhang, C., He, X., and Zhang, X.: Simulating the water δ18O of a small open lake in the East Asian monsoon region based on hydrologic and isotope mass-balance models, J. Hydrol., 612, 128223, https://doi.org/10.1016/j.jhydrol.2022.128223, 2022a.
Xiao, X., Zhang, X., Wu, H., Zhang, C., and Han, L.: Stable isotopes of surface water and groundwater in a typical subtropical basin in south-central China: Insights into the young water fraction and its seasonal origin, Hydrol. Process., 36, e14574, https://doi.org/10.1002/hyp.14574, 2022b.
Xiao, Z., Zhang, X., Xiao, X., Chang, X., He, X., and Zhang, C.: Comparisons of precipitation isotopic effects on daily, monthly and annual time scales – a case study in the subtropical monsoon region of eastern China, Water, 15, 438, https://doi.org/10.3390/w15030438, 2023.
Yang, J., Dudley, B. D., Montgomery, K., and Hodgetts, W.: Characterizing spatial and temporal variation in 18O and 2H content of New Zealand river water for better understanding of hydrologic processes, Hydrol. Process., 34, 5474–5488, https://doi.org/10.1002/hyp.13962, 2020.
Yao, T., Zhang, X., Li, G., Huang, H., Wu, H., Huang, Y., and Zhang, W.: Characteristics of the stable isotopes in different water bodies and their relationships in surrounding areas of Yuelu Mountain in the Xiangjiang River basin, J. Nat. Resour., 31, 1198, https://doi.org/10.11849/zrzyxb.20150810, 2016 (in Chinese).
Zhan, L., Chen, J., Zhang, S., Huang, D., and Li, L.: Relationship between Dongting Lake and surrounding rivers under the operation of the Three Gorges Reservoir, China, Isot. Environ. Healt. S., 51, 255–270, https://doi.org/10.1080/10256016.2015.1020306, 2015.
Zhang, X. P., Guan, H. D., Zhang, X. Z., Wu, H. W., Li, G., and Huang, Y. M.: Simulation of stable water isotopic composition in the atmosphere using an isotopic Atmospheric Water Balance Model, Int. J. Climatol., 35, 846–859, https://doi.org/10.1002/joc.4019, 2015.
Zhiña, D. X., Mosquera, G. M., Esquivel-Hernández, G., Córdova, M., Sánchez-Murillo, R., Orellana-Alvear, J., and Crespo, P.: Hydrometeorological factors controlling the stable isotopic composition of precipitation in the highlands of south Ecuador, J. Hydrometeorol., 23, 1059–1074, https://doi.org/10.1175/JHM-D-21-0180.1, 2022.
Zhou, H., Zhang, X., Yao, T., Hua, M., Wang, X., Rao, Z., and He, X.: Variation of δ18O in precipitation and its response to upstream atmospheric convection and rainout: A case study of Changsha station, south-central China, Sci. Total Environ., 659, 1199–1208, https://doi.org/10.1016/j.scitotenv.2018.12.396, 2019.
Zhou, J., Wan, R. R., Li, B., and Dai, X.: Assessing the impact of climate change and human activities on runoff in the Dongting Lake basin of China, Appl. Ecol. Env. Res., 17, 1–16, https://doi.org/10.15666/aeer/1703_57975812, 2019.