the Creative Commons Attribution 4.0 License.
the Creative Commons Attribution 4.0 License.
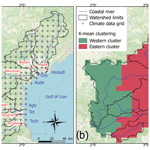
Declining water resources in response to global warming and changes in atmospheric circulation patterns over southern Mediterranean France
Camille Labrousse
Wolfgang Ludwig
Sébastien Pinel
Mahrez Sadaoui
Andrea Toreti
Guillaume Lacquement
Warming trends are responsible for an observed decrease of water discharge in southern France (northwestern Mediterranean). Ongoing climate change and the likely increase of water demand threaten the availability of water resources over the coming decades. Drought indices like the Reconnaissance Drought Index (RDI) are increasingly used in climate characterization studies, but little is known about the relationships between these indices, water resources, and the overall atmospheric circulation patterns. In this study, we investigate the relationships between the RDI, water discharge, and four atmospheric teleconnection patterns (TPs) for six coastal river basins in southern France, both for the historical period of the last 60 years and for a worst-case climatic scenario (RCP8.5) reaching the year 2100. We combine global and regional climate model (CGM and RCM, respectively) outputs with a set of observed climatic and hydrological data in order to investigate the past relationships between the RDI, water discharge, and TPs and to project their potential evolution in space and time. Results indicate that annual water discharge can be reduced by −49 % to −88 % by the end of the century under the extreme climate scenario conditions. Due to unequal links with TPs, the hydroclimatic evolution is unevenly distributed within the study area. Indeed a clustering analysis performed with the RDI time series detects two major climate clusters, separating the eastern and western part of the study region. The former indicates stronger relationships with the Atlantic TPs (e.g. the North Atlantic Oscillation (NAO) and the Scandinavian Oscillation (Scand) patterns), whereas the latter is more closely related to the Mediterranean TPs (Mediterranean Oscillation (MO) and Western Mediterranean Oscillation (WeMO)). The future climate simulations predict an antagonistic evolution in both clusters which are likely driven by decreasing trends of Scand and WeMO. The former provokes a general tendency of lower P in both clusters during spring, summer, and autumn, whereas the latter might partly compensate for this evolution by enhanced precipitation in the eastern cluster during autumn and winter. However, compared to observations, representation of the Mediterranean TPs WeMO and MO in the considered climate models is less satisfactory compared to the Atlantic TPs NAO and Scand, and further improvement of the model simulations therefore requires better representations of the Mediterranean TPs.
- Article
(8746 KB) - Full-text XML
-
Supplement
(1379 KB) - BibTeX
- EndNote
The Mediterranean area was identified as a prominent hot spot for future climate change (Giorgi, 2006). In many areas of its drainage basin, climate models predict decreasing total precipitation (P) together with increasing temperatures (T) over the 21st century and in turn a severe threat for surface water resources and river discharges (Q) (Arnell et al., 2011; Pascual et al., 2015). Water stress may be further exacerbated by growing population (Cramer et al., 2018), and dealing with the increasing water demand may become a serious challenge.
Atmospheric teleconnection patterns (TPs) explain part of the variability in P, Q, and T in the Mediterranean area (e.g. López-Moreno et al., 2011; Vicente-Serrano et al., 2009; Lopez-Bustins et al., 2008). In its northwestern part, climate conditions are influenced by both large-scale TPs – such as the North Atlantic Oscillation (NAO), the Scandinavian Oscillation (Scand) and the Mediterranean Oscillation (MO) – and smaller-scale TPs, such as the Western Mediterranean Oscillation (WeMO). Reliable predictions of their future evolution and associated impacts on hydroclimatic key parameters are hence crucial for the assessment of water cycle changes in response to climate change. These predictions are however complicated by the marked relief which characterizes large parts of the Mediterranean hinterlands. Mountain areas are important contributors to the annual water discharge of rivers (Weingartner et al., 2007), but they also form barriers and morphological corridors for the advection of air masses from remote humidity sources of Atlantic or Mediterranean origins (Molinié et al., 2012). Climatic characteristics at subregional and local scales can therefore be variable, which requires the identification of uniform areas in terms of their hydroclimatic functioning in order to better understand the potential forcing of TPs on past and future climate evolution.
In southwestern Mediterranean France, previous studies already demonstrated that recent climate change had a strong negative impact on water resources in coastal river basins (Lespinas et al., 2010, 2014; Labrousse et al., 2020). These studies however also demonstrated that these catchments have different climatic regimes, and the detected hydroclimatic trends were not uniform. The major objective of this study was therefore to examine whether these trends can be related to modifications of atmospheric TPs and whether identification of regional climatic subunits in the study region can help in the understanding of these relationships. We further also tested whether coupled global and regional climate models (GCMs and RCMs, respectively) were able to reproduce the detected patterns both in terms of the evolution of TPs and the resulting hydroclimatic trends. On the one hand, this is crucial if one intends to use this model in order to produce realistic future climate scenarios and their potential impacts on water resources. On the other hand, identification of potential mismatch between observations and modelled climatologies may also supply useful information for the improvement of coupled GCM–RCM modelling approaches. We finally also projected the hydroclimatic evolution in the study region until the end of the 21th century on the basis of the considered GCMs and RCMs which were forced by the RCP8.5 climate scenario. Changes in P and T were converted into changes of annual water discharge following a set of empirical relationships which have been validated previously (Labrousse et al., 2020). We deliberately selected a worst-case scenario for this exercise as it is expected to produce the strongest trends. Rather than producing the most realistic budgets of future water resources, which are naturally strongly constrained by the realism of the selected future climate scenario, we mainly followed the question of whether the detected spatial differences in the past evolution might be exacerbated by future climate change.
2.1 Study area
The study area consists of six coastal watersheds located in southern France which drain to the Gulf of Lion. From north to south, these are the Herault, Orb, Aude, Agly, Tet, and Tech watersheds (Fig. 1). Their areas range from 729 km2 (Tech) to 4838 km2 (Aude). In terms of climatology, they are characterized by a Mediterranean climate type with hot and dry summers and cool and humid winters. However, not all watersheds are uniformly exposed to Mediterranean climate conditions (Lespinas et al., 2010). Only the Orb and Herault watersheds in the north entirely fulfil the Köppen criteria for Mediterranean climate types (Köppen, 1936), whereas the watersheds further in the south depict reduced seasonal rainfall variability in their hinterlands because of their strong altitudinal gradients and associated connections with air masses from Atlantic origins. Previous studies demonstrated that these watersheds were already affected by decreasing trends in water discharge over the last decades, which could be attributed to recent climatic change (Labrousse et al., 2020; Lespinas et al., 2014, 2010). In terms of morphology, the area is bordered by several mountain ranges, which are the Pyrenees in the south and the Haut-Languedoc heights in the north. These mountains play an important role in the climatology of the watersheds. During autumn and winter, Mediterranean cyclonic systems can bring humid air masses from the sea to the hinterlands. Their confrontation with the colder air masses on the mainland is likely to enhance the convection process, which can lead to heavy rainfall (Vautard et al., 2015) with hundreds of millimetres of precipitation per day. In terms of land use, the study area is densely populated in the coastal and lowland areas and counts four major cities with more than 45 000 inhabitants. The main economic sector is agriculture, which strongly relies on the availability of water resources and on appropriate climatic conditions. All these characteristics make the study area particularly suitable for examination of the evolution of climatic conditions in the future and their potential impacts on the environment and human activities.
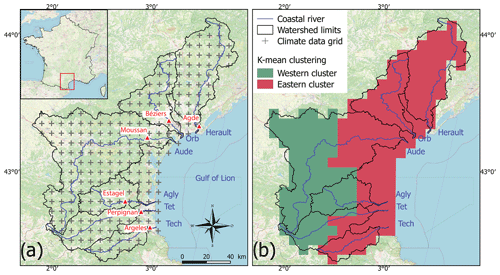
Figure 1Location and characteristics of the study area. (a) Watershed limits, rivers, and Safran grid with daily temperature and precipitation. Red triangles are the gauging stations used for historical evolution of the annual water discharge for each river. (b) Cluster distribution obtained by the k-means method applied to RDI-03 data over the period 1959–2018. Map data: © OpenStreetMap contributors 2021. Distributed under the Open Data Commons Open Database License (ODbL) v1.0.
2.2 Hydroclimatic data
In our study, we use the gridded daily T and P data provided by Safran on a regular projected grid of 8 km × 8 km for the period 1959–2018 (Fig. 1). Safran is a mesoscale atmospheric system developed by the French meteorological agency Meteo-France that uses observation data as well as model outputs for the production of reanalysis data (Durand et al., 1993; Quintana-Seguí et al., 2008). We computed pixel-wise monthly and seasonal averages of each variable. Watershed and cluster averages (see Sect. 2.4) were computed by calculating the mean of all grid points falling within each spatial entity. Boundaries for each watershed were provided by the Carthage database (BD Carthage Métropole, 2021). Although potential evapotranspiration (PET) can be directly extracted from the combination of Safran–Isba, the land surface model which uses the output data from Safran to compute water and surface energy budgets (Soubeyroux et al., 2008; Habets et al., 2008), we reconstructed series of this parameter from temperature data alone according to the formula proposed by Folton and Lavabre (2007). This formula was validated in our area by previous studies (Labrousse et al., 2020; Lespinas et al., 2014). The reason for this is that PET is not available in simulations of future climate conditions (see Sect. 2.7), and we intended to use a uniform approach for this parameter both for past and future climate conditions.
Data of water discharge for each river were taken from the HYDRO database hosted at the French Ministry of Ecology, Sustainable Development and Energy (hydroweb, 2020), which provides daily records for the most downstream gauging stations in the studied river basins. We used the same data series as in Labrousse et al. (2020). Contrarily to our P and T observations, water discharge series do not cover the entire 1959–2018 period, as monitoring of the water gauging stations only started around 1970, and some of the time series are affected by monitoring gaps. Moreover, water discharge for each resulting cluster (see Sect. 3.1.1) is the sum of the water discharge for each river whose delineations of the watershed fall dominantly within the limits of a cluster. Data are available in cubic metres per second (m3 s−1), which we convert per unit area and per year, thus being millimetres per year (mm yr−1).
2.3 Future simulation of water discharge
For the prediction of future water discharge series, we applied a statistical multi-parameter hydrological model based on two single climatic indices: RDI-12 and Qpike. The Reconnaissance Drought Index (RDI) corresponds to the drought index of Tsakiris et al. (2007), which is derived from the combination of P and T data. It can be calculated annually (RDI-12) or seasonally (RDI-03). Qpike is based on the combination of annual PET and P data (Pike, 1964) and has been proven to give a realistic estimate of average annual water discharge in Mediterranean rivers (Sadaoui et al., 2018; Ludwig et al., 2009). This hydrological empirical model has been developed in Labrousse et al. (2020) over the same watersheds investigated in this current work. Labrousse et al. (2020) demonstrated that in all of the six study catchments, the model was able to explain 78 %–88 % of the variability of annual water discharge during the study period 1959–2018. Therefore, for future simulations of annual water discharge we followed the methodology used in this former work.
2.4 K-means clustering
Our study approach is driven by the hypothesis that the climatic and hydrological behaviour of the study area is not uniform, given the differences in morphology and possible connections to air masses of different origins. Following the results from the studies of Labrousse et al. (2020) and Lespinas et al. (2014, 2010), we expect two zones with different connections to the atmospheric circulation which are one zone encompassing the watersheds of the Herault, Orb, and Tech rivers and a second one including the other watersheds. The former would be more associated with the Mediterranean functioning (showing higher precipitation seasonality and being morphologically oriented towards the Mediterranean), and the latter would be more influenced by air masses coming from the Atlantic (given a weaker seasonality and an open corridor that leads to the west). K-means clustering (Lloyd, 1982; Cam and Neyman, 1967) is a technique that can be used for the detection of climatic regions which behave uniformly and which can consequently be used to test whether different climatic subunits in our study area exist (Fovell and Fovell, 1993; DeGaetano and Shulman, 1990). K-means clustering, as most methods, suffers from an a priori selection of the number of clusters and from high dependence on initial conditions. Here we used an elbow heuristic method: the selection of the initial number of clusters is given by a bend in the value of the total within-cluster sum of square (see Bholowalia and Kumar, 2014). Results and final selection of the number of clusters were done using the function raster.kmeans() from the ecbtools package in the R program (Williamson, 2016). Given the a priori assumption that two zones are climatically different, the number of clusters expect after running the test is two.
2.5 Teleconnection patterns
Monthly historical values for NAO and Scand were taken from the Climate and Prediction Center of the National Oceanic and Atmospheric Administration of USA (NOAA, 2021), while monthly historical values for MO and WeMO were made available by the Climatic Research Unit of the University of East Anglia (MOI data, 2021). For the calculation of future TP projections, we followed the method proposed by Compo et al. (2011) at the Physical Science Laboratory of the NOAA (NOAA Physical Sciences Laboratory, 2021). NAO is consequently defined as the difference in the standardized monthly sea-level pressure anomalies at Lisbon, being the high-pressure pole (HP), and Reykjavik, being the low-pressure pole (LP). Similarly, and for their positive phase, the Scand pattern has its HP pole over northern Scandinavia (which we defined as the location of the city of Kautokeino, Norway) and has two LP poles located over the southeastern Atlantic (which we defined as the city of Porto, Portugal) and eastern Russia. The MO pattern has its HP over Gibraltar and its LP over Tel Aviv (Israel), and WeMO has a HP pole over San Fernando (Spain) and a LP pole over Padua (Italy). For each standardized series, we computed the mean sea-level pressure that surrounds the exact location of each pole (corresponding generally to 4 pixels of each gridded product). Location of the selected poles for each TP and their computation are given in Table 1.
2.6 Wavelet analysis
Wavelet analysis is a powerful method of time series analysis compared with more traditional methods and has been widely used for hydrologic or atmospheric variables since the 1990s (Holman et al., 2011; Liesch and Wunsch, 2019; Holman et al., 2011; Kang and Lin, 2007; Grinsted et al., 2004; Torrence and Compo, 1998). A wavelet is characterized by its localization in both time and frequency, and wavelet analysis is therefore an adequate method to examine multiscale phenomena of a climatic series. A review of the detailed applications and objectives of wavelet analysis was made available by Sang (2013). In addition, the cross-wavelet transform provides a correlation between two signals in the time–frequency space, named the common power, as well as their relative phase, named the continuous wavelet coherence. Cross-wavelet analysis is thus an appropriate method for tracking the relationships between two climatic time series, and further work for its application over multivariate climate series has been recently carried out (Polanco-Martínez et al., 2020). As performed in Liesch and Wunsch (2019), we computed here the wavelet analyses with a Morlet wavelet and based on the monthly data of historical TPs, Q, and RDI-03.
2.7 Climate projections
Projected climate data (T and P) under a RCP8.5 scenario were taken from six RCMs which were forced by four different GCMs at their boundary conditions. Climate data received a correction, through the ADAMONTv1.0 method (Verfaillie et al., 2017), which uses Safran as a forcing function. Sea-level pressure data were taken from the same four GCMs. The data are available in the Copernicus database CMIP5 (Copernicus, 2021), and the characteristics of each GCM and RCM are shown in Table 2. RCMs provide daily T and P values which cover the period 1950–2018. By definition, historical simulations span the period 1959–2005 and can consequently be used to validate the models by comparing the seasonal means of each parameter and their linear trends with the observed data of Safran. Future projections under a RCP8.5 scenario span the period 2006–2100. Also here, we computed the annual mean for each parameter and performed linear trends to explore their evolution through the projected period. We focus in our study exclusively on the RCP8.5, which was released in the fifth assessment report of the IPCC in 2014 (IPCC, 2014). It should be considered a worst-case scenario, which assumes the greatest fossil fuel use and results in an additional 8.5 W m−2 of radiative forcing by 2100. Its realism is therefore debated today (see, for example, Burgess et al., 2020; Hausfather and Peters, 2020; Schwalm et al., 2020), and the predicted climate evolution should naturally be considered with caution. The main interest of using this “no-climate policy scenario” is that extreme conditions are more suitable for detection of the general trends related to global warming, even if the magnitude of the predicted changes can be exaggerated.
2.8 Statistics
Single-correlation and multiple-regression analyses were performed on the basis of the squared Pearson correlation coefficient (Pearson, 1931), which allows for quantification of the strength of linear relationships. Linear trend analyses of hydroclimatic variables were performed using Mann–Kendall and Sen slope tests (Mann, 1945; Kendall, 1975; Sen, 1968). For the validation of simulated TPs compared to observations, we applied a Tukey's honest significance difference (HSD) test (Tukey, 1949). Tukey's HSD test is a single-step multiple-comparison post hoc test that is commonly used to assess the significant differences between pairs of group means.
3.1 Evolution of the climatic conditions and teleconnection patterns over the historical period
3.1.1 K-mean clustering
When testing the k-means clustering algorithm for our study region on the basis of different climatic parameters and different k values (we tested k= 2 and k= 3), we obtained the most satisfactory results by fixing k to a value of 2 and using the parameter RDI-03, which is based both on T and P data. This results in two clusters which basically separate the study region into an eastern and a western climate cluster (Fig. 1). The eastern cluster is the larger one and dominantly includes the Herault, Orb, and Tech basins. The western cluster, on the other hand, covers large parts of the upper Aude, Agly, and Tet basins. This feature corroborates with the finding of Labrousse et al. (2020), who reported a generally more elevated warming trend in the former basins during the 1959–2018 period compared to the latter ones, whereas the latter basins depicted a stronger tendency towards decreasing precipitation (although statistically only weakly significant). This indicates that both clusters could behave differently from a climatic point of view. Moreover, it also fits with the findings of Lespinas et al. (2010), who analysed the seasonality of precipitation in the study region by dividing the six river basins into a series of 15 sub-basins. They reported on the basis of the 1965–2004 averages that the strong contrast between high winter and low summer precipitation, as this is required for the Mediterranean climate definition according to Köppen (1936), only matches in the entire Herault and Orb basins as well as in the lower parts of the other basins. The upper parts of the Tech, Tet, Agly, and Aude basins have less contrasted seasonality. It is therefore likely that the eastern cluster we identified is more under the influence of local air masses from Mediterranean origin compared to the western cluster, which might be more strongly influenced by air masses from remote origin. Notice that especially the Aude basin in the central part of our study region is morphologically connected to the Garonne River basin, which drains to the Atlantic. For cluster-specific statistical analysis of observed water discharge, we consider in the following that the sum of water discharge of the Herault, Orb, and Tech rivers corresponds to the eastern cluster, whereas the sum of water discharge of the Aude, Agly, and Tet rivers corresponds to the western cluster.
3.1.2 Wavelet analysis
Univariate wavelet analyses allow for an overview of significant periodicities in time series. Figure 2 shows the resulting power spectra for all TPs and selected hydroclimatic variables (RDI-03, Q) within the two clusters. The local maxima of the power spectra are given in Table S1 in the Supplement. The represented time series are rather homogeneous and do not depict major break points, which indicates that the hydroclimatic regime did not fundamentally change over the study period. RDI-03 shows generally the strongest yearly cycle, with an average power of 15.2 and 16.9 for the eastern and western cluster, respectively. Such high values reflect the fact that this parameter is based on a combination of T and P data and therefore perfectly represents the contrasting seasonal conditions which characterize the Mediterranean climate type. Also, water discharge, MO, and WeMO depict significant annual cycles but with lower power values. They decrease respectively in the sense in which the parameters are listed. With respect to the clusters, one can notice that power values are generally greater in the western cluster than in the eastern cluster, which means that in the former one there is more regular periodicity than in the latter one.
Also, cycles other than annual can be detected. Water discharge in the western cluster further depicts periodicities of 4.3 and 14 years and in the eastern cluster of 3.0, 8.4, and 9.3 years. Periodicities are hence generally longer in the western cluster than in the eastern one. The large-scale TPs NAO and Scand might show both half-year cycles as well as a decade-like cycles ranging from 11 to 16 years (NAO) and from 8 to 10 years (Scand), but those are however not very evident and have been pointed out as such in the study of Chiodo et al. (2019). Finally, also for WeMO a long-term cycle of 10 to 20 years (local maximum of 18 years) can be detected, which is, however, as for NAO and Scand, less significant.
We furthermore performed cross-wavelet analyses between TPs, RDI03, and water discharge for each cluster. The corresponding plots and statistics are presented in the Supplement (Fig. S1; Table S2). Also here, water discharge of the western cluster generally shows longer cycles of cross-wavelet coherence with TPs than in the eastern cluster. Mediterranean TPs (MO, WeMO) and their coherence with water discharge are more complex compared to Atlantic TPs (NAO and Scand) in the sense that the Mediterranean overall functioning is more irregular than the Atlantic one.
3.1.3 Correlation analysis
Correlation analysis between TPs and selected hydroclimatic parameters (RDI-03, water discharge, T, and P) at the seasonal scales is shown in Fig. 3. For all parameters, highly significant correlation (or anti-correlation) is detected. The strength and sign of these correlations however strongly depend on the considered season, which again confirm the complex hydroclimatic regime of our analysed region driven by an interplay of air mass fluxes from different origins. For all parameters and all seasons, it can nevertheless be noticed that the eastern cluster has generally higher values of correlation and greater significance levels with TPs than the western cluster. This cluster is obviously more closely connected to the Mediterranean Sea, which is an important reservoir for the atmospheric transfer of water and heat fluxes in our region.
During most of the year, temperature is anti-correlated with Scand and WeMO, which means that during positive phases, colder air masses of northern (Scand) and northwestern (WeMO) origin trigger lower temperatures in the study region. This is valid for both clusters. The influence of Scand is especially dominant during the first part of the year (spring and summer), while the influence of WeMO increases during autumn. Only in winter does the influence of both TPs cease, and T is mainly controlled by warm air masses of southwestern origin, as indicated by the positive correlation with NAO and MO.
Humidity fluxes are revealed by the correlation between TPs and P and Q. The patterns associated with both parameters are closely connected, which is also the case for RDI-03 (for which variability in P is obviously more important than variability of T). P is positively correlated in both clusters with Scand throughout the year, except for winter. The colder air masses of northern origin are consequently a source of humidity in the study region. Nonetheless, it should be borne in mind that both the overall formation and maintenance mechanisms of such atmospheric patterns are complex processes (e.g. Wang and Tan, 2020). Only in winter does anti-correlation with NAO become dominant for P in the eastern cluster. This anti-correlation of P with NAO is often cited in the literature as one of the major drivers of inter-annual variability of P in the northwestern Mediterranean area (see, for example, López-Moreno et al., 2011; Vicente-Serrano et al., 2009; López-Moreno and Vicente-Serrano, 2007). Furthermore, an important modulating influence exists between the Scand and the NAO, which in turn partly affects the climate variability over Europe (Comas-Bru and McDermott, 2014). But here, and for this period analysed, our data indicate that during most of the year, the influence of Scand is more important.
Besides correlation with the large-scale TPs Scand and NAO, P, Q, and RDI-03 are also significantly connected to the Mediterranean TPs MO and WeMO. These relationships are particularly interesting because, here, the western and eastern cluster depict large differences, which can at least partly explain why climate in both clusters is different. P in the eastern cluster is significantly anti-correlated with MO in spring, autumn, and winter and with WeMO in autumn and winter. This reflects the arrival of air masses from southern and eastern origins, which could be enriched in humidity when crossing the Mediterranean Sea. Especially the relationship between P and WeMO is worth being highlighted here. Correlations between P and WeMO switch from positive to negative between the first and the second half of the year. During spring and summer, it is positive (although only significant in summer) for both clusters. This reflects the arrival of humid Atlantic air masses from the northwest. During autumn and winter, however, correlation coefficients switch to highly significant negative values, but this only holds for the eastern cluster. Negative WeMO index indicates the arrival of Mediterranean air masses from the east, which can be enriched in humidity when passing over the Mediterranean. As during this period of the year, land surfaces cool more rapidly than sea surfaces, their arrival is often associated with the formation of violent precipitation and associated flash floods which are one of the hydrological characteristics in this area (Chazette et al., 2016; Ricard et al., 2012; Nuissier et al., 2011).
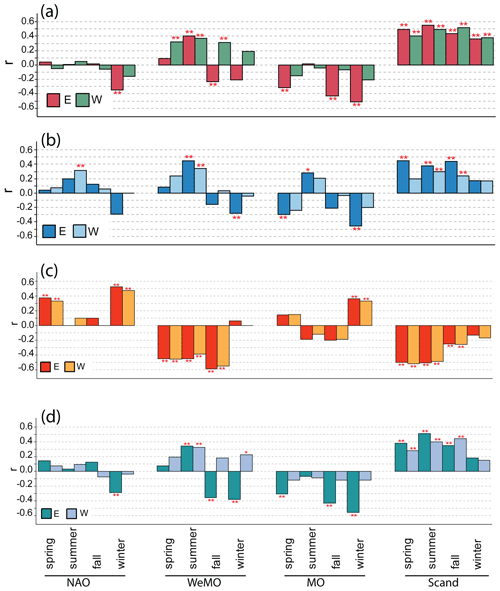
Figure 3Estimated correlations between the teleconnection patterns and (a) RDI03, (b) water discharge, (c) temperature, and (d) precipitation. For (a), (c), and and (d) the period considered is 1959–2018, whereas in (b) the period considered is 1976–2018. Water discharge for each cluster is the sum of each river's water discharge whose watershed belongs to either one of the cluster. Thus, western discharge is the sum of the Aude, Agly, and Tet water discharge, and eastern discharge the sum of Herault, Orb, and Tech water discharge.
3.1.4 Variability of TPs in reanalysis and GCM simulations
During the previous section, we demonstrated that TPs exert a significant control on the hydroclimatic regime of our study region. Before assessing future climate conditions within the RCP8.5 scenario, we therefore tested first whether there is a reasonable fit between modelled and the reanalysis values of TPs. Figure 4 illustrates that for the historical period 1950–2005, the four selected GCMs generally succeed in reproducing the average TP values for NAO, Scand, and MO. For NAO, the observed variability is also reproduced in a realistic manner, whereas for Scand, and even more extremely for MO, the simulated variabilities are larger than the reanalysis ones (Fig. 4a). For WeMO, the opposite holds, which means that the models fail in reproducing the average value and simulate inter-annual variabilities which are lower than the reanalysed ones. With respect to the long-term trends (Fig. 4b), it should be noticed that the models and reanalysis generally follow similar trends for NAO, Scand, and MO but not for WeMO. Reanalysis for the latter TP depicts a strong and significant trend towards lower values during the period 1950–2005, whereas the models produce a rather stationary evolution.
Modelled TPs therefore fit well with reanalysed TPs for NAO and still reasonably well for Scand. But the fits are less satisfactory for MO and not satisfactory at all for WeMO. Part of these discrepancies may be explained by the fact that WeMO is rather a local-scale TP which consequently could suffer from the coarse spatial resolution of GCMs, generally ranging from 100 to 200 km. Notice that the distance between the two poles of WeMO is about 1700 km, compared to 3500–4000 km for the other TPs. Another reason may be related to the fact that both WeMO and MO stretch over the (western) Mediterranean Sea, and atmospheric circulation should also be significantly influenced by the heat and energy content of the Mediterranean water masses. In other words, unless GCMs and RCMs are coupled to detailed oceanic circulation models for the Mediterranean Sea, realistic simulations of WeMO and MO might be difficult.
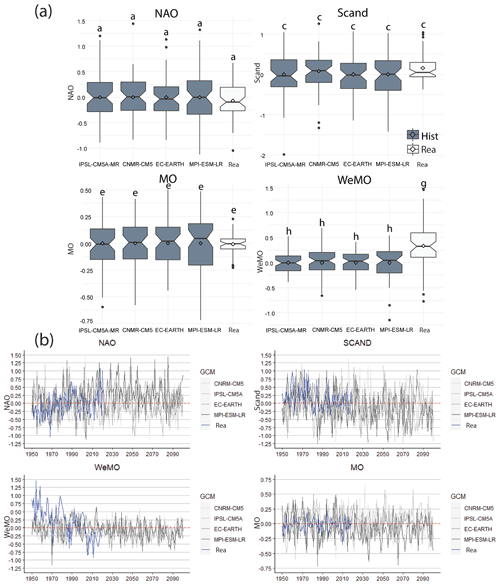
Figure 4Evolution of the annual teleconnection pattern. (a) Variability of teleconnection patterns provided by the reanalysis for the period 1950–2005 (Rea) and simulated by the four GCMs in the historical period 1950–2005 (Hist). Letters indicate whether box plots are significantly equal (same letter as box plot “Rea”) or not. (b) Annual future projections under a RCP8.5 scenario. The blue line represents the annual reanalysis data and is referred to as “Rea” in the legend.
3.2 Comparison of historical RCM simulations with Safran
For simulation of the long-term climatic evolution during the period of 1959–2100, we extracted the monthly T and P values from our RCMs and calculated the multi-model averages. For a control of the ability of our RCMs to reproduce the historical conditions, we further compare the seasonal averages and linear trends with the observed data extracted from Safran. Figure 5 presents the results of this comparison, as well as corresponding values for the projected period of 2006–2100 (see Sect. 3.3.2). The figure demonstrates that the historical values (1959–2005 averages) almost perfectly fit with the data from Safran for the same period. This is not really surprising since the RCM data were corrected using the ADAMONT v1.0 method (Verfaillie et al., 2017), which uses Safran as a forcing function. Both datasets also agree on the seasonal patterns of each cluster, which remain preserved in the future simulations. For all seasons, the eastern cluster depicts slightly higher temperatures than the western cluster, in agreement with its vicinity to the Mediterranean Sea and greater coverage of lowland terrains. For precipitation, however, the relative importance of each cluster is variable according to the season. The western cluster always shows higher precipitation values during spring and summer compared to the eastern one, whereas the opposite is the case during autumn.
For trend analyses, the fits between the model simulations and Safran are less satisfactory. During the historical period 1959–2005, Safran only depicts significant trends for temperature but not for precipitation. Temperature has strongly increased in summer (especially in the eastern cluster) followed by spring and by winter. Increase of autumn temperature is the weakest and statistically not significant. This pattern is in good agreement with the results of Lespinas et al. (2010), who reconstructed the trends on manual extrapolation of station data for about the same period. However, in the corresponding RCM simulations, the warming patterns are different. T increased most strongly in autumn and in summer, followed by winter and by spring, the season with the weakest T increase (which is only significant in the eastern cluster). Here, precipitation also follows significant negative trends, especially in summer and winter in the western cluster.
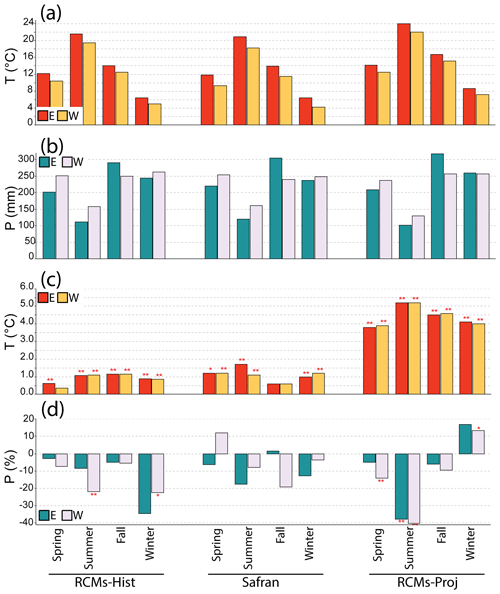
Figure 5Comparison between Safran and RCMs precipitation and temperature for the historical period 1959–2005 and for the projected period 2006–2100. RCMs-Hist stands for the historical period of RCMs, whereas RCMs-Proj is for the projected period 2006–2100 (a, b) mean annual temperatures and precipitation, respectively, and (c, d) Mann–Kendall linear trend of temperatures and precipitation, respectively.
3.3 Projection of future hydroclimatic conditions under a RCP8.5 scenario
3.3.1 Future evolution of TPs
Future evolution of the TPs as produced by the GCM simulations is shown in Fig. 4 and Table 3. When averaging the outputs of all models, we find a stationary evolution for NAO and MO, whereas WeMO and Scand follow a significant trend towards lower values. Although the models did not catch the strong decrease of WeMO in the past, they nevertheless predict that the general evolution towards lower values of this TP will persist in the future.
3.3.2 Future climatic conditions
For the predicted future changes, model simulations predict an important temperature increase, which is strongest in summer (+5.2 ∘C) and weakest in spring (+3.9 ∘C) (Fig. 5). These increases are almost identical in both clusters. The evolution of precipitation is characterized by decreasing trends, especially in summer (−37 % to −43 %) where the trends are significant for both clusters and in spring (−5 % to −14 %) where the trends are however only significant in the western cluster. Surprisingly, the models predict even a slight precipitation increase in winter (13 % to 17 %), which is however only significant in the western cluster.
At the watershed scale, and considering the mean ensemble of the six RCMs, the linear evolution of climatic conditions from 2006 to 2100 shows a homogeneous warming for all the watersheds and a decrease of precipitation generally enhanced in the watersheds that belong to the western cluster (Fig. 6). This decrease ranges from −10 % (Agly) to −14 % (Tet and Aude) and is highly significant, whereas in the watersheds of the Herault and Orb only a decrease of −3 % that is non-significant is seen. Surprisingly, the Tech lies closer to the Agly, Aude, and Tet watersheds' behaviour with a decrease of −10 %. Cluster-wise, and on an annual basis, this can translate into a highly significant temperature increase of about +4.5 and +4.4 ∘C for the western and eastern clusters respectively (Fig. S4). Changes in precipitation are only significant in the western cluster, with −12 % by the end of the century.
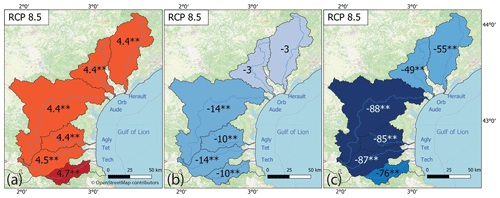
Figure 6Linear trends for projected annual hydroclimatic variables under a RCP8.5 scenario and for the period 2006–2100. Results are shown for the mean ensemble of the six RCMs used in this study. (a) Temperature (in ∘C), (b) precipitation (in %), and (c) water discharge (in %). Map data: © OpenStreetMap contributors 2021. Distributed under the Open Data Commons Open Database License (ODbL) v1.0.
3.3.3 Evolution of water discharge
Future series of annual water discharge for each river were computed following the methodology presented in Labrousse et al. (2020), which was tested and validated over the same watersheds as in this current study. For a RCP8.5 scenario, linear trend analysis depict a strong decrease in the annual water discharge for all the watersheds (Fig. 6). Trends are also highly significant (pv < 0.05) for every watershed. One interesting observation in those results is the stronger decrease occurring in the watersheds belonging to the western cluster (Fig. S4). Evolution shows there a decrease of about −85 % (Agly) to −88 % (Aude) against a decrease of −49 % (Orb), −50 % (Herault), and −76 % (Tech) for those belonging to the eastern cluster and linked more closely – according to the correlation and wavelet analysis – to the Mediterranean Sea. The overall change for each cluster is thus −86.5 % for the western cluster and −58.4 % for the eastern cluster (Fig. S4). We note that the higher rate of reduction detected for the Tech watershed might be linked to the reduction of precipitation (Sect. 3.3.2).
It has been widely established that atmospheric teleconnection patterns like NAO, Scand, Mo, and WeMO drive seasonal variability of T, P, and Q in the northwestern Mediterranean (see, for example, Mathbout et al., 2020; Ulbrich et al., 2012; Toreti et al., 2010) and likely will play a significant role in the control their future evolution (Beranová and Kyselý, 2016). Our data confirm this for our study region. But there is a complex interplay between the dominance of each TP, which strongly depends on the considered season and the morphological peculiarities. Understanding of the hydroclimatic regime in this area is hence complicated.
However, when clustering the area according to the statistical k-means technique, this understanding can be largely improved. We identified two major clusters which represent respectively the eastern terrains, which are more closely connected to air masses of Mediterranean origins, as well as the western terrains, which correspond dominantly to the more elevated hinterlands and which are more closely connected to air masses of remote origins. The parameter we used for the cluster separation is the drought index parameter RDI-03. Other studies also demonstrated that drought indices are suitable for clustering climate subunits in the Mediterranean area (Manzano et al., 2019). In general, correlations between TPs and hydroclimatic parameters are stronger for the eastern than for the western cluster, especially with regard to the water-flux parameters P and Q. In mountainous areas, local air convection is important for triggering precipitation (Giorgi et al., 2016; Smith, 1979), which consequently can outweigh the influence of large-scale air mass exchanges. Also, wavelet analysis confirms that both clusters are different. Short- and long-term periodicity is generally more regular in the western cluster, as revealed by greater power values. Periodicity in the eastern cluster should also be affected by variability of the heat and water mass fluxes of the Mediterranean Sea, which might explain the lower power values in this cluster.
Among the different TPs we tested, Scand and WeMO obviously have the strongest impact on heat and water fluxes in the study region. In its positive phase, the Scand is associated with an arrival of air masses from northern Europe, which then reach the French Mediterranean coast via a southeasterly flow over the Mediterranean (Kalimeris and Kolios, 2019; Krichak et al., 2014; Bueh and Nakamura, 2007; Lionello et al., 2006). Positive phases of WeMO correspond to a low-pressure centre located over central and eastern Europe and to an anticyclone in the southwest of Spain (Martin-Vide and Lopez-Bustins, 2006), which favours the arrival of air masses of Atlantic origin in our study region via a northeasterly flow. For precipitation, these remote air masses are generally associated with values greater than average. This holds however only for Scand in the indicated seasons and clusters, whereas in the case of WeMO, the situation is more differentiated. Greater P values in association with positive phases are restricted to the first half of the year (especially summer), but then it switches to a significant negative correlation between both parameters in the eastern cluster. This translates the additional control of this TP into the arrival of humid air masses of Mediterranean origin, which generate most of the P during autumn and winter. WeMO is therefore a significant driver both for humidity sources from Atlantic and Mediterranean origins in our study region.
Interestingly, Scand and WeMO are the two TPs which show significant negative trends in their long-term evolution of the future climate conditions we constructed on the basis of the extreme RCP8.5 scenario. The mean ensemble model simulation produces a decrease of Scand of about −0.186 between 2006 and 2100 (Table 4). For WeMO this is −0.149. Consequently, for all of our six study catchments, the future scenarios predict moderate decreases of P (which are however only significant in the southern Aude, Agly, Tet, and Tech catchments) and, in combination with the important warming trends, a strong decrease of Q (Fig. 6). Both P and Q decreases are stronger in the catchments belonging to the western cluster than in those belonging to the eastern cluster. The latter are also influenced by WeMO during autumn and winter, and, as WeMO is anti-correlated with P here, the long-term evolution of WeMO might be associated with relatively more precipitation during these seasons, which can counteract with the general P decrease in relation to Scand.
It should be kept in mind that the values which are depicted in Fig. 6 correspond to a worst-case scenario, and the 55 %–88 % reduction of annual water discharge that we found for the individual rivers should naturally be considered with caution. Moreover, the statistical models we used for the prediction of annual discharge series were calibrated on a narrower range of temperature increase, and it is not clear whether they can be extrapolated to such extreme warming conditions. It should nevertheless be noticed that also Lespinas et al. (2014), who used the hydrological model GR2M for a simulation of water discharge in the study region under similar future climate conditions, reported that decreases of > 80 % could be expected. There is hence little doubt that future warming should have severe consequences on the availability of surface water resources.
One of the main interests of our future simulation is that it can be directly compared to the evolution during the recent past in order to test whether there are evolution patterns that are consistent with each other. Both the past (see also Labrousse et al., 2020) and future evolution patterns agree in the sense that they depict a general tendency towards lower precipitation in the southern catchments compared to northern ones, i.e. in the catchments which dominantly constitute the western climate cluster. In the future scenarios, this is also assigned with consistently greater discharge reductions in these catchments. Of course, these discharge simulations strongly rely on the validity of our statistical models and, as we only show the mean ensemble values, on the variability of simulations between individual models. During the hindcasting period, these simulations generally fit well with the Safran-based simulations (Figs. S2; S3), and all models produce greater discharge reductions in the western than in the eastern cluster (Table 4). The Safran-based hindcasting simulations produce however a rather equilibrated discharge reduction in both clusters (Labrousse et al., 2020). This is related to that fact that the stronger precipitation decrease in the western cluster is compensated for by a stronger temperature increase in the eastern cluster. Notice that the GCM–RCM models fail in reproducing these temperature differences and produce a rather homogeneous temperature increase (Fig. 6). Also seasonally, the models do not reproduce the warming trends well compared to observations (Fig. 5).
The importance of the WeMO and MO patterns for the western Mediterranean P was first reported by Gonzalez-Hidalgo et al. (2009), highlighting that negatives phases of both TPs play in favour of higher P, and that predominates the influence of NAO, which is mainly restricted to the winter season (Dünkeloh and Jacobeit, 2003). We confirm this but also show that this mainly holds for the eastern cluster. Unfortunately, compared to observations, the representation of both TPs in the considered GCM–RCM simulations is less satisfactory than for NAO and Scand, giving less credit to these simulations. This is especially problematic in the case of WeMO. This TP already showed during the recent past a strong negative trend, which is not reproduced by the considered climate models. In other words, during the forthcoming decades, the decrease of WeMO could in reality be much more important than the models predict, increasing hence the influence of air masses from Mediterranean origins and consequently the contrast between both climate clusters. Reliable representation of the Mediterranean TPs in climate models may be more complicated than representation of the Atlantic TPs since this probably requires the coupling with oceanic circulation models at much finer spatial scales in the Mediterranean area in order to catch the heat and water fluxes. For the prediction of the evolution of extreme precipitation events in this area, which is one of major challenges for climate change research, further progress in this field seems to be mandatory.
The overall goal of this study was to investigate the relationships between a series of selected climatic and hydrological parameters and several teleconnection patterns in order to better understand the hydroclimatic functioning in the study region during past and future climate conditions. The study area is made up of six watersheds in the south of France on the Mediterranean side. Previous studies that worked on the evolution of climatic and hydrological conditions for the same watersheds showed different responses to climate change and a decrease in the water discharge of the six corresponding coastal rivers. In this study we propose to statistically divide the study area into subunits, each characterized by its specific climatic conditions and connections to the atmospheric circulation. We further employ an ensemble of CMIP5 model projections to study the annual evolution of water discharge for the six coastal rivers under a future RCP8.5 scenario. This allows us to come to a number of conclusions which can be summarized as follows:
-
There is a complex interplay between the seasonal evolution of the major hydroclimatic parameters T, P, and Q and the dominant atmospheric TPs NAO, Scand, MO, and WeMO. Clustering based on the RDI-03 drought index and the statistical k-means clustering technique however allows for the identification of two major climatic clusters, which respectively represent the areas which are under the influence of remote Atlantic (western cluster) and more local Mediterranean (eastern cluster) air masses.
-
Our future simulations on the hydroclimatic evolution in our study catchments confirm that the decrease of surface water resources which has been detected in the recent past is likely to continue during the forthcoming decades. Under extreme conditions, average annual water discharge could decrease by about −49 % to −88 %. This evolution is mainly driven by the strong temperature increase, which uniformly applies to all catchments, in combination with a moderate precipitation decrease of max. −14 %, which is however restricted to the southern catchments.
-
The future climate simulations predict an antagonistic evolution in both clusters, which are significantly related to decreasing trends of the TPs Scand and WeMO. The former provokes a general tendency of lower P in both clusters during spring, summer, and autumn, whereas the latter might compensate for this evolution by enhanced P in the eastern cluster during autumn and winter.
-
Compared to observations, representation of the Mediterranean TPs WeMO and MO in the considered climate models is less satisfactory compared to the Atlantic TPs NAO and Scand. Further improvement of the model simulations therefore requires better representations of the Mediterranean TPs, for example, through coupling of high-resolution models of oceanic circulation in the Mediterranean Sea.
The main code commands are given in the Supplement.
Data of climatic projections (temperature, precipitation, and sea-level pressure) can be found at https://doi.org/10.24381/cds.9d44a987 (Copernicus, 2021). Historical atmospheric and hydrological data are available in the Supplement.
The supplement related to this article is available online at: https://doi.org/10.5194/hess-26-6055-2022-supplement.
CL designed and conducted all experiments and analysed results with advice from WL, SP, MS, and AT. Analyses were done by CL, WL, SP, and MS. Funding was acquired by WL and GL. CL wrote the paper, and all co-authors agreed to the published version.
The contact author has declared that none of the authors has any competing interests.
Publisher's note: Copernicus Publications remains neutral with regard to jurisdictional claims in published maps and institutional affiliations.
We are especially grateful to Météo-France for supply of the Safran–Isba climate data in the framework of the Publithèque agreement between MF and UPVD.
This research has been supported by the doctoral school ED 305 at UPVD through the attribution of a PhD grant to Camille Labrousse.
This paper was edited by Niko Wanders and reviewed by two anonymous referees.
Arnell, N. W., van Vuuren, D. P., and Isaac, M.: The implications of climate policy for the impacts of climate change on global water resources, Global Environ. Chang., 21, 592–603, https://doi.org/10.1016/j.gloenvcha.2011.01.015, 2011.
BD Carthage Métropole: Hydrological Data Bank Hosted at the French Ministry of the Environment and of Sustainable Development, https://geo.data.gouv.fr/fr/datasets/38d5c85219924e0f5355b551b0453ed942a03b8f, last access: 21 February 2021.
Beranová, R. and Kyselý, J.: Links between circulation indices and precipitation in the Mediterranean in an ensemble of regional climate models, Theor. Appl. Climatol., 123, 693–701, https://doi.org/10.1007/s00704-015-1381-6, 2016.
Bholowalia, P. and Kumar, A.: EBK-Means: A Clustering Technique based on Elbow Method and K-Means in WSN, Int. J. Comp. Appl., 105, 17–24, 2014.
Bueh, C. and Nakamura, H.: Scandinavian pattern and its climatic impact, Q. J. Roy. Meteor. Soc., 133, 2117–2131, https://doi.org/10.1002/qj.173, 2007.
Burgess, M. G., Ritchie, J., Shapland, J., and Pielke, R.: IPCC baseline scenarios have over-projected CO2 emissions and economic growth, Environ. Res. Lett., 16, 014016, https://doi.org/10.1088/1748-9326/abcdd2, 2020.
Cam, L. M. L. and Neyman, J.: Proceedings of the Fifth Berkeley Symposium on Mathematical Statistics and Probability: Weather modification, University of California Press, 690 pp., https://www.worldcat.org/formats-editions/25234809 (last access: 22 November 2020), 1967.
Chazette, P., Flamant, C., Raut, J.-C., Totems, J., and Shang, X.: Tropical moisture enriched storm tracks over the Mediterranean and their link with intense rainfall in the Cevennes-Vivarais area during HyMeX, Q. J. Roy. Meteor. Soc., 142, 320–334, https://doi.org/10.1002/qj.2674, 2016.
Chiodo, G., Oehrlein, J., Polvani, L. M., Fyfe, J. C., and Smith, A. K.: Insignificant influence of the 11-year solar cycle on the North Atlantic Oscillation, Nat. Geosci., 12, 94–99, https://doi.org/10.1038/s41561-018-0293-3, 2019.
Comas-Bru, L. and McDermott, F.: Impacts of the EA and SCA patterns on the European twentieth century NAO–winter climate relationship, Q. J. Roy. Meteor. Soc., 140, 354–363, https://doi.org/10.1002/qj.2158, 2014.
Compo, G. P., Whitaker, J. S., Sardeshmukh, P. D., Matsui, N., Allan, R. J., Yin, X., Gleason, B. E., Vose, R. S., Rutledge, G., Bessemoulin, P., Brönnimann, S., Brunet, M., Crouthamel, R. I., Grant, A. N., Groisman, P. Y., Jones, P. D., Kruk, M. C., Kruger, A. C., Marshall, G. J., Maugeri, M., Mok, H. Y., Nordli, Ø., Ross, T. F., Trigo, R. M., Wang, X. L., Woodruff, S. D., and Worley, S. J.: The Twentieth Century Reanalysis Project, Q. J. Roy. Meteor. Soc., 137, 1–28, https://doi.org/10.1002/qj.776, 2011.
Copernicus: CMIP5 monthly data on single levels, Copernicus Climate Change Service [data set], https://doi.org/10.24381/cds.9d44a987, 2021.
Cramer, W., Guiot, J., Fader, M., Garrabou, J., Gattuso, J.-P., Iglesias, A., Lange, M. A., Lionello, P., Llasat, M. C., Paz, S., Peñuelas, J., Snoussi, M., Toreti, A., Tsimplis, M. N., and Xoplaki, E.: Climate change and interconnected risks to sustainable development in the Mediterranean, Nat. Clim. Change, 8, 972–980, https://doi.org/10.1038/s41558-018-0299-2, 2018.
DeGaetano, A. T. and Shulman, M. D.: A climatic classification of plant hardiness in the United States and Canada, Agr. Forest Meteorol., 51, 333–351, https://doi.org/10.1016/0168-1923(90)90117-O, 1990.
Dünkeloh, A. and Jacobeit, J.: Circulation dynamics of Mediterranean precipitation variability 1948–98, Int. J. Climatol., 23, 1843–1866, https://doi.org/10.1002/joc.973, 2003.
Durand, Y., Brun, E., Merindol, L., Guyomarc’h, G., Lesaffre, B., and Martin, E.: A meteorological estimation of relevant parameters for snow models, Ann. Glaciol., 18, 65–71, https://doi.org/10.3189/S0260305500011277, 1993.
Folton, N. and Lavabre, J.: Approche par modélisation PLUIE-DEBIT pour la connaissance régionale de la ressource en eau: application à la moitié du territoire français, Houille Blanche, 93, 64–70, https://doi.org/10.1051/lhb:2007037, 2007.
Fovell, R. G. and Fovell, M.-Y. C.: Climate Zones of the Conterminous United States Defined Using Cluster Analysis, J. Climate, 6, 2103–2135, https://doi.org/10.1175/1520-0442(1993)006<2103:CZOTCU>2.0.CO;2, 1993.
Giorgi, F.: Climate change hot-spots, Geophys. Res. Lett., 33, L08707, https://doi.org/10.1029/2006GL025734, 2006.
Giorgi, F., Torma, C., Coppola, E., Ban, N., Schär, C., and Somot, S.: Enhanced summer convective rainfall at Alpine high elevations in response to climate warming, Nat. Geosci., 9, 584–589, https://doi.org/10.1038/ngeo2761, 2016.
Gonzalez-Hidalgo, J. C., Lopez-Bustins, J.-A., Štepánek, P., Martin-Vide, J., and Luis, M. de: Monthly precipitation trends on the Mediterranean fringe of the Iberian Peninsula during the second-half of the twentieth century (1951–2000), In. J. Climatol., 29, 1415–1429, https://doi.org/10.1002/joc.1780, 2009.
Grinsted, A., Moore, J. C., and Jevrejeva, S.: Application of the cross wavelet transform and wavelet coherence to geophysical time series, Nonlin. Processes Geophys., 11, 561–566, https://doi.org/10.5194/npg-11-561-2004, 2004.
Habets, F., Boone, A., Champeaux, J. L., Etchevers, P., Franchistéguy, L., Leblois, E., Ledoux, E., Moigne, P. L., Martin, E., Morel, S., Noilhan, J., Seguí, P. Q., Rousset-Regimbeau, F., and Viennot, P.: The SAFRAN-ISBA-MODCOU hydrometeorological model applied over France, J. Geophys. Res.-Atmos., 113, D06113, https://doi.org/10.1029/2007JD008548, 2008.
Hausfather, Z. and Peters, G. P.: RCP8.5 is a problematic scenario for near-term emissions, P. Natl. Acad. Sci. USA, 117, 27791–27792, https://doi.org/10.1073/pnas.2017124117, 2020.
Holman, I. P., Rivas-Casado, M., Bloomfield, J. P., and Gurdak, J. J.: Identifying non-stationary groundwater level response to North Atlantic ocean-atmosphere teleconnection patterns using wavelet coherence, Hydrogeol. J., 19, 1269, https://doi.org/10.1007/s10040-011-0755-9, 2011.
hydroweb: Hydrological Data Bank Hosted at the French Ministry of the Environment and of Sustainable Development, http://www.hydro.eaufrance.fr/, last access: 9 June 2020.
IPCC: Climate Change 2014: Synthesis Report. Contribution of Working Groups I, II and III to the Fifth Assessment Report of the Intergovernmental Panel on Climate Change, edited by: Core Writing Team, Pachauri, R. K. and Meyer, L. A., Synthesis Report, Geneva, Switzerland, https://www.ipcc.ch/report/ar5/syr/ (last access: 12 July 2021) 2014.
Kalimeris, A. and Kolios, S.: TRMM-based rainfall variability over the Central Mediterranean and its relationships with atmospheric and oceanic climatic modes, Atmos. Res., 230, 104649, https://doi.org/10.1016/j.atmosres.2019.104649, 2019.
Kang, S. and Lin, H.: Wavelet analysis of hydrological and water quality signals in an agricultural watershed, J. Hydrol., 338, 1–14, https://doi.org/10.1016/j.jhydrol.2007.01.047, 2007.
Kendall, M. G.: Rank correlation methods, Griffin, Oxford, England, ISBN 9780852641996, 1975.
Köppen, W.: Das geographisca System der Klimate, in: Handbuchder Klimatologie, Borntraeger, 1–44, 1936.
Krichak, S. O., Breitgand, J. S., Gualdi, S., and Feldstein, S. B.: Teleconnection–extreme precipitation relationships over the Mediterranean region, Theor. Appl. Climatol., 117, 679–692, https://doi.org/10.1007/s00704-013-1036-4, 2014.
Labrousse, C., Ludwig, W., Pinel, S., Sadaoui, M., and Lacquement, G.: Unravelling Climate and Anthropogenic Forcings on the Evolution of Surface Water Resources in Southern France, Water, 12, 3581, https://doi.org/10.3390/w12123581, 2020.
Lespinas, F., Ludwig, W., and Heussner, S.: Impact of recent climate change on the hydrology of coastal Mediterranean rivers in Southern France, Climatic Change, 99, 425–456, https://doi.org/10.1007/s10584-009-9668-1, 2010.
Lespinas, F., Ludwig, W., and Heussner, S.: Hydrological and climatic uncertainties associated with modeling the impact of climate change on water resources of small Mediterranean coastal rivers, J. Hydrol., 511, 403–422, https://doi.org/10.1016/j.jhydrol.2014.01.033, 2014.
Liesch, T. and Wunsch, A.: Aquifer responses to long-term climatic periodicities, J. Hydrol., 572, 226–242, https://doi.org/10.1016/j.jhydrol.2019.02.060, 2019.
Lionello, P., Malanotte-Rizzoli, P., and Boscolo, R.: Mediterranean Climate Variability, Elsevier, 438 pp., ISBN 9780080460796, 2006.
Lloyd, S.: Least squares quantization in PCM, IEEE T. Inform. Theory, 28, 129–137, https://doi.org/10.1109/TIT.1982.1056489, 1982.
Lopez-Bustins, J.-A., Martin-Vide, J., and Sanchez-Lorenzo, A.: Iberia winter rainfall trends based upon changes in teleconnection and circulation patterns, Global Planet. Change, 63, 171–176, https://doi.org/10.1016/j.gloplacha.2007.09.002, 2008.
López-Moreno, J. I. and Vicente-Serrano, S. M.: Atmospheric circulation influence on the interannual variability of snow pack in the Spanish Pyrenees during the second half of the 20th century, Hydrol. Res., 38, 33–44, https://doi.org/10.2166/nh.2007.030, 2007.
López-Moreno, J. I., Vicente-Serrano, S. M., Morán-Tejeda, E., Lorenzo-Lacruz, J., Kenawy, A., and Beniston, M.: Effects of the North Atlantic Oscillation (NAO) on combined temperature and precipitation winter modes in the Mediterranean mountains: Observed relationships and projections for the 21st century, Global Planet. Change, 77, 62–76, https://doi.org/10.1016/j.gloplacha.2011.03.003, 2011.
Ludwig, W., Dumont, E., Meybeck, M., and Heussner, S.: River discharges of water and nutrients to the Mediterranean and Black Sea: Major drivers for ecosystem changes during past and future decades?, Prog. Oceanogr., 80, 199–217, https://doi.org/10.1016/j.pocean.2009.02.001, 2009.
Mann, H. B.: Nonparametric Tests Against Trend, Econometrica, 13, 245–259, https://doi.org/10.2307/1907187, 1945.
Manzano, A., Clemente, M. A., Morata, A., Luna, M. Y., Beguería, S., Vicente-Serrano, S. M., and Martín, M. L.: Analysis of the atmospheric circulation pattern effects over SPEI drought index in Spain, Atmos. Res., 230, 104630, https://doi.org/10.1016/j.atmosres.2019.104630, 2019.
Martin-Vide, J. and Lopez-Bustins, J.-A.: The Western Mediterranean Oscillation and rainfall in the Iberian Peninsula, Int. J. Climatol., 26, 1455–1475, https://doi.org/10.1002/joc.1388, 2006.
Mathbout, S., Lopez-Bustins, J. A., Royé, D., Martin-Vide, J., and Benhamrouche, A.: Spatiotemporal variability of daily precipitation concentration and its relationship to teleconnection patterns over the Mediterranean during 1975–2015, Int. J. Climatol., 40, 1435–1455, https://doi.org/10.1002/joc.6278, 2020.
MOI data: Climate Research Unit. Mediterranean Oscillation Indices (MOI) and Saharan Oscillation Index (SaOI), https://crudata.uea.ac.uk/cru/data/moi/, last access: 21 February 2021.
Molinié, G., Ceresetti, D., Anquetin, S., Creutin, J. D., and Boudevillain, B.: Rainfall Regime of a Mountainous Mediterranean Region: Statistical Analysis at Short Time Steps, J. Appl. Meteorol. Clim., 51, 429–448, https://doi.org/10.1175/2011JAMC2691.1, 2012.
NOAA: Climate Prediction Center, https://www.cpc.ncep.noaa.gov/products/precip/CWlink/daily_ao_index/teleconnections.shtml, last access: 21 February 2021.
NOAA Physical Sciences Laboratory: Climate Prediction Center, https://psl.noaa.gov/data/20thC_Rean/timeseries/monthly/NAO/index.html, last access: 21 February 2021.
Nuissier, O., Joly, B., Joly, A., Ducrocq, V., and Arbogast, P.: A statistical downscaling to identify the large-scale circulation patterns associated with heavy precipitation events over southern France, Q. J. Royal Meteor. Soc., 137, 1812–1827, https://doi.org/10.1002/qj.866, 2011.
Pascual, D., Pla, E., Lopez-Bustins, J. A., Retana, J., and Terradas, J.: Impacts of climate change on water resources in the Mediterranean Basin: a case study in Catalonia, Spain, Hydrolog. Sci. J., 60, 2132–2147, https://doi.org/10.1080/02626667.2014.947290, 2015.
Pearson, E. S.: The Test of Significance for the Correlation Coefficient, J. Am. Stat. Assoc., 26, 128–134, https://doi.org/10.1080/01621459.1931.10503208, 1931.
Pike, J. G.: The estimation of annual run-off from meteorological data in a tropical climate, J. Hydrol., 2, 116–123, https://doi.org/10.1016/0022-1694(64)90022-8, 1964.
Polanco-Martínez, J. M., Fernández-Macho, J., and Medina-Elizalde, M.: Dynamic wavelet correlation analysis for multivariate climate time series, Sci. Rep.-UK, 10, 21277, https://doi.org/10.1038/s41598-020-77767-8, 2020.
Quintana-Seguí, P., Le Moigne, P., Durand, Y., Martin, E., Habets, F., Baillon, M., Canellas, C., Franchisteguy, L., and Morel, S.: Analysis of Near-Surface Atmospheric Variables: Validation of the SAFRAN Analysis over France, J. Appl. Meteor. Clim., 47, 92–107, https://doi.org/10.1175/2007JAMC1636.1, 2008.
Ricard, D., Ducrocq, V., and Auger, L.: A Climatology of the Mesoscale Environment Associated with Heavily Precipitating Events over a Northwestern Mediterranean Area, J. Appl. Meteorol. Clim., 51, 468–488, https://doi.org/10.1175/JAMC-D-11-017.1, 2012.
Sadaoui, M., Ludwig, W., Bourrin, F., and Romero, E.: The impact of reservoir construction on riverine sediment and carbon fluxes to the Mediterranean Sea, Prog. Oceanogr., 163, 94–111, https://doi.org/10.1016/j.pocean.2017.08.003, 2018.
Sang, Y.-F.: A review on the applications of wavelet transform in hydrology time series analysis, Atmos. Res., 122, 8–15, https://doi.org/10.1016/j.atmosres.2012.11.003, 2013.
Schwalm, C. R., Glendon, S., and Duffy, P. B.: RCP8.5 tracks cumulative CO2 emissions, P. Natl. Acad. Sci. USA, 117, 19656–19657, https://doi.org/10.1073/pnas.2007117117, 2020.
Sen, P. K.: Estimates of the Regression Coefficient Based on Kendall's Tau, J. Am. Stat. Assoc., 63, 1379–1389, https://doi.org/10.1080/01621459.1968.10480934, 1968.
Smith, R. B.: The Influence of Mountains on the Atmosphere, in: Advances in Geophysics, vol. 21, edited by: Saltzman, B., Elsevier, 87–230, https://doi.org/10.1016/S0065-2687(08)60262-9, 1979.
Soubeyroux, J.-M., Martin, E., Franchisteguy, L., Habets, F., Noilhan, J., Baillon, M., Regimbeau, F., Vidal, J.-P., Moigne, P. L., and Morel, S.: Safran-Isba-Modcou (SIM): Un outil pour le suivi hydrométéorologique opérationnel et les études, La Météorologie, 63, 40–45, 2008.
Toreti, A., Desiato, F., Fioravanti, G., and Perconti, W.: Seasonal temperatures over Italy and their relationship with low-frequency atmospheric circulation patterns, Climatic Change, 99, 211–227, https://doi.org/10.1007/s10584-009-9640-0, 2010.
Torrence, C. and Compo, G. P.: A Practical Guide to Wavelet Analysis, B. Am. Meteorol. Soc., 79, 61–78, https://doi.org/10.1175/1520-0477(1998)079<0061:APGTWA>2.0.CO;2, 1998.
Tsakiris, G., Pangalou, D., and Vangelis, H.: Regional Drought Assessment Based on the Reconnaissance Drought Index (RDI), Water Resour. Manage., 21, 821–833, https://doi.org/10.1007/s11269-006-9105-4, 2007.
Tukey, J. W.: Comparing Individual Means in the Analysis of Variance, Biometrics, 5, 99–114, https://doi.org/10.2307/3001913, 1949.
Ulbrich, U., Lionello, P., Belušić, D., Jacobeit, J., Knippertz, P., Kuglitsch, F. G., Leckebusch, G. C., Luterbacher, J., Maugeri, M., Maheras, P., Nissen, K. M., Pavan, V., Pinto, J. G., Saaroni, H., Seubert, S., Toreti, A., Xoplaki, E., and Ziv, B.: Climate of the Mediterranean: Synoptic Patterns, Temperature, Precipitation, Winds, and Their Extremes, chap. 5, in: The Climate of the Mediterranean Region, edited by: Lionello, P., Elsevier, Oxford, 301–346, https://doi.org/10.1016/B978-0-12-416042-2.00005-7, ISBN 9780124160422, 2012.
Vautard, R., van Oldenborgh, G.-J., Thao, S., Dubuisson, B., Lenderink, G., Ribes, A., Planton, S., Soubeyroux, J.-M., and Yiou, P.: Extreme fall 2014 precipitation in the Cévennes mountains, B. Am. Meteorol. Soc., 96, S56–S60, 2015.
Verfaillie, D., Déqué, M., Morin, S., and Lafaysse, M.: The method ADAMONT v1.0 for statistical adjustment of climate projections applicable to energy balance land surface models, Geosci. Model Dev., 10, 4257–4283, https://doi.org/10.5194/gmd-10-4257-2017, 2017.
Vicente-Serrano, S. M., Beguería, S., López-Moreno, J. I., Kenawy, A. M. E., and Angulo-Martínez, M.: Daily atmospheric circulation events and extreme precipitation risk in northeast Spain: Role of the North Atlantic Oscillation, the Western Mediterranean Oscillation, and the Mediterranean Oscillation, J. Geophys. Res.-Atmos., 114, D08106, https://doi.org/10.1029/2008JD011492, 2009.
Wang, M. and Tan, B.: Two Types of the Scandinavian Pattern: Their Formation Mechanisms and Climate Impacts, J. Climate, 33, 2645–2661, https://doi.org/10.1175/JCLI-D-19-0447.1, 2020.
Weingartner, R., Viviroli, D., and Schädler, B.: Water resources in mountain regions: a methodological approach to assess the water balance in a highland-lowland-system, Hydrol. Process., 21, 578–585, https://doi.org/10.1002/hyp.6268, 2007.
Williamson, G.: Ecbtools: Environmental Change Biology Tools, GitHub [code], https://rdrr.io/github/ozjimbob/ecbtools/, 2016.