the Creative Commons Attribution 4.0 License.
the Creative Commons Attribution 4.0 License.
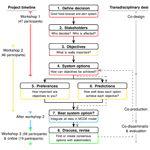
The role of multi-criteria decision analysis in a transdisciplinary process: co-developing a flood forecasting system in western Africa
Jafet C. M. Andersson
Daniel Hofmann
Francisco Silva Pinto
Martijn Kuller
Climate change is projected to increase flood risks in western Africa. In the FANFAR project, a pre-operational flood early warning system (FEWS) for western Africa was co-designed in workshops with 50–60 stakeholders from 17 countries, adopting multi-criteria decision analysis (MCDA). We aimed at (i) designing a FEWS with western African stakeholders using MCDA and (ii) evaluating participatory MCDA as a transdisciplinary process. To achieve the first aim (i), we used MCDA methods for problem structuring and preference elicitation in workshops. Problem structuring included stakeholder analysis, creating 10 objectives to be achieved by the FANFAR FEWS and designing 11 possible FEWS configurations. Experts predicted FEWS configuration performance, which we integrated with stakeholder preferences. We tested MCDA results in sensitivity analyses. Three FEWSs showed good performance, despite uncertainty, and were robust across different preferences. For stakeholders it was most important that the FEWS produces accurate, clear, timely, and accessible flood risk information. To achieve the second aim (ii), we clustered common characteristics of collaborative governance frameworks from the sustainability science and transdisciplinary literature. Our framework emphasizes issues crucial to the earth systems sciences, such as uncertainty and integrating interdisciplinary knowledge. MCDA can address both well. Other strengths of MCDA are co-producing knowledge with stakeholders and providing a consistent methodology with unambiguous, shared results. Participatory MCDA including problem structuring can contribute to co-designing a project but does not achieve later phases of transdisciplinary processes well, such as co-disseminating and evaluating results. We encourage colleagues to use MCDA and the proposed framework for evaluating transdisciplinary hydrology research that engages with stakeholders and society.
- Article
(4351 KB) - Full-text XML
-
Supplement
(5845 KB) - BibTeX
- EndNote
1.1 Floods in western Africa
Western Africa is vulnerable to projected impacts of climate change, particularly concerning runoff quantities (Aich et al., 2016; Roudier et al., 2014). Climate change projections and mechanisms remain uncertain for western Africa, but there is growing evidence for increased frequency, magnitude, and impact of floods (Nka et al., 2015). Western Africa is already heavily impacted by floods. Preliminary United Nations data estimate that 465 people died from floods in western and central Africa in 2020. More than 1.7 million people were affected, 94 000 people displaced, and 152 000 houses destroyed (OCHA, 2020). Good flood early warning systems (FEWSs) help minimize flood impacts (Perera et al., 2019); “good” means they give accurate, timely, and understandable information and are affordable. Several FEWSs have been set up in western Africa, some being very useful. However, none sufficiently meet stakeholder needs regarding (i) timeliness (e.g., annual frequency of PRESASS/PRESAGG forecasts; WMO, 2021), (ii) coverage (systems propagating streamflow measurements cover small parts of western Africa and no ungauged basins, e.g., SLAPIS, OPIDIN, or FEWS-Oti; Massazza et al., 2020), (iii) up-to-date operational production without failures (e.g., interrupted production and access to SATH-NBA during the major 2020 floods; NBA, 2020), (iv) accuracy (e.g., global modeling systems such as GloFAS; Passerotti et al., 2020), and (v) openness and ownership (e.g., proprietary closed-source consultancy systems may limit the independence of western African stakeholders and hence the FEWS' long-term sustainability). An overview of gaps, needs, and recommendations is provided by the WMO (2020). Moreover, feedback from a stakeholder survey, interviews, and the literature indicated that the perceived overall effectiveness of FEWSs was very low in all but one western African country, receiving the lowest score of 1 of 3 possible (Fig. 5 in Lumbroso et al., 2016).
1.2 Developing a FEWS with stakeholders in the FANFAR project
The EU Horizon 2020 project FANFAR aimed at co-developing a pre-operational FEWS for western Africa (FANFAR, 2021; Andersson et al., 2020a). This FEWS is currently based on three open-source hydrological HYPE models (Andersson et al., 2017; Arheimer et al., 2020; Santos et al., 2022) in a cloud ICT environment. It includes daily meteorological forecasting, data assimilation, hydrological forecasting, flood alert derivation, and distribution through email, SMS, API (application programming interface), and an interactive visualization portal (https://fanfar.eu/ivp/, last access: 1 June 2022). Rather than the technical system (Andersson et al., 2020b), this paper addresses stakeholder engagement in an iterative co-design process, which is needed to address FEWS development (Sultan et al., 2020).
To organize such a transdisciplinary endeavor involving many stakeholders, a comprehensive multi-criteria decision analysis (MCDA) process can be suitable (Belton and Stewart, 2002; Eisenführ et al., 2010; Keeney, 1982). It should include problem-structuring methods (Rosenhead and Mingers, 2001). Participatory MCDA can help focus FEWS development such that it best meets stakeholder expectations. Indeed, MCDA has been used in flood risk management (reviewed by de Brito and Evers, 2016; Abdullah et al., 2021) but rarely as a participatory process. Stakeholders were not even mentioned in a review of 149 papers (Abdullah et al., 2021). de Brito and Evers (2016) concluded that stakeholder participation was fragmented and that stakeholders were rarely involved in the entire decision process despite being reported in 51 % of 128 papers.
2.1 Sustainability science and transdisciplinary research frameworks
Disaster management increasingly acknowledges that FEWS development should closely involve users to adapt it to their needs, thus increasing usefulness, effectiveness, and uptake (Basher, 2006; Bierens et al., 2020; UNISDR, 2010). Participatory processes to address global environmental challenges are at the core of transdisciplinary research and the sustainability sciences. However, this literature lacks systematic integration and conceptualization of empirical evidence (e.g., Lang et al., 2012; Caniglia et al., 2021). Mechanisms of sustainability transformations are still not well understood (Schneider et al., 2019; Wuelser et al., 2021). Various frameworks for collaborative governance have been proposed, several using three main phases: (i) problem framing, (ii) collaborative research and co-producing knowledge, and (iii) evaluating and co-disseminating results (Jahn et al., 2012; Lang et al., 2012; Mauser et al., 2013). These elements are shared by other frameworks, but some proposed another structure (e.g., Caniglia et al., 2021; Lemos and Morehouse, 2005). Many authors stress the iterative nature of transdisciplinary processes, where progress is achieved in cycles. From a practical perspective, four guiding principles for evaluating co-production processes have been proposed (Norstrom et al., 2020): (i) to situate the process in a context, place, or issue, (ii) pluralistic by recognizing multiple ways of knowing and doing, (iii) goal-oriented, and (iv) interactive with ongoing learning of actors and frequent, active engagement. Recent systematic analyses of transdisciplinary projects revealed seven common characteristics: (i) transdisciplinary principles such as taking practitioners on board, (ii) transdisciplinary approaches such as joint problem identification or alliances with regional partners, (iii) systematic procedures and specific methodologies, (iv) product formats for communicating and using results in practice and capacity building, (v) personal learning and skills development, (vi) framings, and (vii) results including insights, data, and information (Wuelser et al., 2021). Moreover, societal impacts can be classified along three generic mechanisms: (i) promote systems, target knowledge, and transformation knowledge, (ii) foster social learning for collective action, and (iii) enhance competences for reflective leadership (Schneider et al., 2019).
We clustered shared characteristics from this literature in our own framework (Table 1). We found that some elements received less attention in the social-science-oriented literature but are, however, highly relevant to the earth system sciences. These include the explicit consideration of uncertainty and the interdisciplinary effort needed for tackling technically complex problems (Lemos and Morehouse, 2005; Mauser et al., 2013). Our framework follows a stepwise timeline, as proposed by many (Jahn et al., 2012; Lang et al., 2012; Mauser et al., 2013). We used the terminology by Mauser et al. (2013), (i) co-design, (ii) co-production, and (iii) co-dissemination of knowledge, to which we added evaluation, involving academia and stakeholders throughout. We will use the proposed framework for evaluating and discussing the role of MCDA in a transdisciplinary process, specifically how well the different elements are met by MCDA.
2.2 MCDA in flood risk research
MCDA is well suited to addressing the challenge of stakeholder participation in FEWS development and embraces various methodologies to support complex decisions (e.g., Belton and Stewart, 2002; de Brito and Evers, 2016). We chose multi-attribute value theory (MAVT; Eisenführ et al., 2010; Keeney, 1982) for reasons well documented in the literature: (i) developing a complex FEWS requires many decisions, such as identifying hydrological models and data sources to produce forecasts or defining appropriate flood hazard thresholds, visualizations, and distribution channels to reach people. MCDA allows one to address such choices. (ii) To adapt the FEWS to stakeholder needs, collaboration with nonacademic partners is required. MCDA allows close stakeholder interaction, offering various methods for each stage of decision making (e.g., Eisenführ et al., 2010; Keeney, 1982; Marttunen and Hamalainen, 2008; Zheng et al., 2016; Marttunen et al., 2017). (iii) MAVT and value-focused thinking (Keeney, 1996) base decisions on the objectives that are of fundamental importance to stakeholders. (iv) To evaluate FEWS configurations, MCDA allows integration of different kinds of scientific and technical data from experts such as forecast accuracy or development costs in step 6 of the MCDA process (see Methods and Fig. 1). The stakeholder preferences are elicited separately in step 5: in complex decisions, not all objectives can be fully achieved, and MCDA explicitly asks stakeholders which trade-offs they are willing to make. Preferences are combined with the prediction data in step 7. Especially in case of conflicting interests, it can be helpful to disentangle stakeholder values from facts (Gregory et al., 2012a; Keeney, 1982; Reichert et al., 2015). (v) MAVT and multi-attribute utility theory (MAUT) are mathematically very flexible. Usually linear additive aggregation is applied, but many non-compensatory models are possible, which may better represent stakeholder preferences (Haag et al., 2019a; Reichert et al., 2015, 2019). (vi) MAVT and MAUT allow inclusion of various types of uncertainty, e.g., of expert predictions with probability theory or stakeholder preferences with sensitivity analyses (Reichert et al., 2015; Haag et al., 2019b; Zheng et al., 2016). (vii) MCDA is done stepwise to reduce complexity and increase transparency.
MCDA is increasingly popular in hydrology and flood risk research. Our brief literature search revealed around 50 articles, but only a few included stakeholders (Web of Science 25 August 2021; keywords: “MCDA” AND “hydrolog*” AND/OR “flood*”). This corroborates the results of two reviews (de Brito and Evers, 2016; Abdullah et al., 2021). Both confirmed a significant growth in MCDA applications, especially for flood mitigation, while flood preparedness, response, or recovery phases were understudied. Most papers lacked uncertainty analysis and stakeholder participation (de Brito and Evers, 2016). We found that MCDA was mainly used as a technical method to integrate indicators, e.g., for calibrating flood forecasting models (Pang et al., 2019). Recent methodologically interesting papers addressed MCDA coupled with artificial intelligence (Pham et al., 2021), machine learning (Nachappa et al., 2020), or portfolio decision analysis (Convertino et al., 2019). Combining GIS (geographic information systems) with MCDA is a trend, also in hydrology. Examples include flood risk assessment focusing on uncertainty (Tang et al., 2018) and flood risk analyses producing risk maps (e.g., Ronco et al., 2015; Samanta et al., 2016).
Among the few studies including stakeholders are a MCDA concept to improve urban resilience in flood risk management (Evers et al., 2018) and a participatory case study for flood vulnerability assessment (de Brito et al., 2018). In most cases with participation, stakeholders only assigned weights, but further participatory processes were not documented (de Brito and Evers, 2016; Ronco et al., 2015). However, several papers stated that MCDA results are highly susceptible to model assumptions, especially weights (de Brito and Evers, 2016). For instance, the sensitivity of MCDA results to weight variability was assessed with a global sensitivity analysis by Tang et al. (2018). To increase decision-making quality and implementation success, MCDA applications require uncertainty analysis and stakeholder participation (de Brito and Evers, 2016).
2.3 Aims and research questions
The research gaps identified in the literature review lead to two complementary aims: (1) define what constitutes a good FEWS for western Africa using a participatory MCDA process that includes uncertainty and document empirical evidence from the FANFAR project, hereby contributing to knowledge production, learning, and scientific praxis in hydrology; (2) evaluate the suitability of participatory MCDA as a transdisciplinary process. Concretely, we address two research questions.
-
RQA. What characterizes a good regional FEWS for western Africa? Is it possible to identify a robust FEWS configuration despite uncertainty of expert predictions of FEWS performance, despite uncertainty of the MCDA model, and despite possibly different preferences of stakeholders regarding what the FEWS should achieve?
-
RQB. How suitable is participatory MCDA as a transdisciplinary process in a large, international project? What worked well or less well in FANFAR? Is the proposed framework useful for this type of evaluation?
3.1 MCDA within a transdisciplinary process
A typical transdisciplinary process starts with co-design and joint problem framing in step 1 of our proposed framework (Table 1). This was done at the beginning of the FANFAR project with joint proposal writing and a kick-off meeting with European and western African consortium partners (Sect. 3.2). Co-design can be further divided into building the collaborative research team (step 1a), defining research questions and the methodological framework (1b), and finding the boundary object, which is a FEWS for western Africa in our case (1c). To support participation, legitimacy, inclusion of bridging organizations, and balanced ownership from science and practice (Table 1; steps 1a and 1b), we carried out a stakeholder analysis (e.g., Grimble and Wellard, 1997; Lienert et al., 2013; Reed et al., 2009). Although often neglected in MCDA, stakeholder analysis can be a suitable early step in the problem-structuring phase of MCDA (Fig. 1; step 2). Identifying stakeholders is crucial in any participatory project. In FANFAR, the main identified stakeholders that participated in the workshops were representatives from hydrological services, emergency management agencies, river basin organizations, and regional expert agencies. Together with these priority stakeholders, we identified objectives: “What is of fundamental importance to be achieved by a FEWS?” Furthermore, we identified options: “Which FEWS configurations are potentially suitable for achieving objectives?” These early steps of MCDA (Fig. 1; steps 3 and 4) can be classified under co-design step 1 of the transdisciplinary processes (Table 1). To support identification of objectives and options, diverse problem-structuring methods (PSMs) are available (Rosenhead and Mingers, 2001). It is common to combine MCDA with PSMs (reviewed by Marttunen et al., 2017). Similar PSMs to those used in FANFAR were described in a wastewater infrastructure planning example (Lienert et al., 2015).
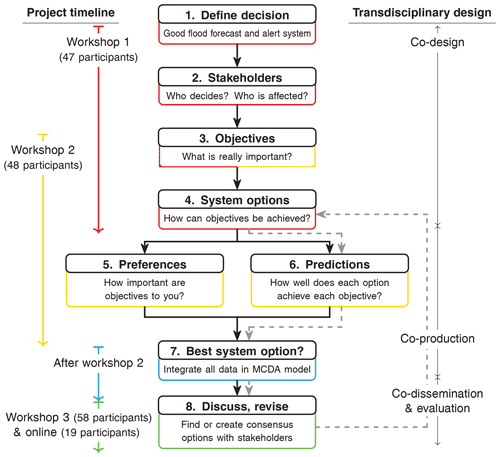
Figure 1Multi-criteria decision analysis (MCDA) was carried out stepwise in the FANFAR project. For explanations, see the text.
The next steps 5–7 in MCDA (Fig. 1) belong to the transdisciplinary co-production step 2 (Table 1). Hereby, research produces new knowledge in continuous exchange between scientists from different disciplines and stakeholders. A transdisciplinary process is often iterative (e.g., Jahn et al., 2012; Lang et al., 2012), captured in FANFAR with cycles of stakeholder workshops to test, discuss, and improve the pre-operational FEWS. In the co-dissemination and evaluation step 3 (Table 1), new knowledge is critically reflected, integrated, and disseminated, which is captured in step 8 of MCDA (Fig. 1). In the following, after summarizing the workshops (Sect. 3.2), we focus on the MCDA steps (Sects. 3.3–3.10). We present MCDA methods such that they are easily adaptable to other transdisciplinary projects, e.g., in hydrology research, and provide extensive details as a blueprint in the Supplement.
3.2 Co-design workshops in western Africa
We carried out three workshops in western Africa and a FANFAR consortium kick-off meeting (Norrköping, Sweden, 17–18 January 2018). A fourth workshop was replaced with two half-day online workshops due to COVID-19 (20–21 January 2021) and a final online workshop (1 June 2021). The workshops are documented in reports (FANFAR, 2021; Lienert et al., 2020). At each workshop, western African stakeholders presented the rainy season flood situation in their country and their experience with the FANFAR FEWS. Each workshop hosted extensive technical sessions for experimenting with the latest FEWS configuration and included structured technical feedback. Between workshops, the FEWS was adapted to meet requests as well as possible (Andersson et al., 2020a). We also conducted sessions with emergency managers, e.g., about their understanding of flood risk representation to improve FEWS visualizations (Kuller et al., 2020). Here, we focus only on interactions at the core of MCDA.
The first workshop (Niamey, Niger, 17–20 September 2018) hosted 47 participants from 21 countries, including European and African consortium members and representatives from regional and national hydrological service and emergency management agencies from 17 western and central African countries. The main aim was to initiate the co-design process. For MCDA, we used problem structuring (Fig. 1): stakeholder analysis (Sect. 3.3), identifying fundamentally important objectives of stakeholders (Sect. 3.4), and FEWS configurations that meet objectives (Sect. 3.5). The second workshop (Accra, Ghana, 9–12 April 2019) hosted 48 participants from 21 countries. For MCDA, we consolidated objectives and elicited participants' preferences regarding achieving these objectives (Sect. 3.7). Additionally, we collected preference data on the importance of objectives from each stakeholder with questionnaires. This provided interesting insights into preference formation over time (Kuller et al., 2022). For the third workshop (Abuja, Nigeria, 10–14 February 2020), participant numbers increased to 58, including representatives from the WMO (World Meteorological Organization; https://public.wmo.int/, last access: 1 June 2022), ECOWAS (Economic Community of West African States; https://www.ecowas.int/, last access: 1 June 2022), and 16 western and central African countries. We discussed the main MCDA results. During a last online workshop, which was attended by 10–19 participants (varying numbers due to Internet connection problems), stakeholders completed a survey, providing some feedback for MCDA (Sect. 3.10).
3.3 Stakeholder analysis
For the stakeholder analysis (Grimble and Wellard, 1997; Reed et al., 2009), we followed Lienert et al. (2013). Workshop participants filled in a pen-and-paper questionnaire in French or English, assisted by two experts. The survey was completed in 2.5 h by 31 participants in 18 groups, clustered in countries. After receiving information, the participants completed two tables, one for identifying key western African organizations that produce and operate FEWSs and one for downstream stakeholders. As an example, we asked “Who might play a role because they use information from such systems in society?” Each table contained eight tasks: (1) listing key organizations or stakeholders, (2) specifications such as names, (3) their presumed main interests, (4) why they might use FEWSs, and (5) appropriate distribution channels. We used a 10-point Likert scale, asking participants to (6) rate the importance of considering each listed stakeholder in the FANFAR co-design process, (7) the presumed influence or power of each stakeholder in implementing the FEWS, and (8) how strongly each would be affected by the FEWS performance level. We cleaned the raw data and categorized stakeholders according to forecast/alert producers or users, decisional level, sector, and perceived main interest (for details, see Silva Pinto and Lienert, 2018).
3.4 Generating objectives and attributes
Generating objectives is key to MCDA (Belton and Stewart, 2002; Eisenführ et al., 2010; Keeney, 1982), since this choice can alter results. Value-focused thinking guides this step by focusing on what is fundamentally important to stakeholders (Keeney, 1996). However, simply asking is insufficient, and often too few (Bond et al., 2008; Haag et al., 2019c) or too many objectives are produced; we refer to the guidelines in Marttunen et al. (2019). Our stepwise procedure started at the FANFAR kick-off meeting in Sweden and continued in the first two western African workshops (for details, see Lienert et al., 2020). In the first workshop, one stakeholder group individually used an interactive online survey to first brainstorm and then select objectives from a master list (Haag et al., 2019c). Individuals in a second group used the same procedure as a pen-and-paper survey, assisted by a moderator. The third group used a means–ends network in a moderated group discussion to find consensus objectives (Eisenführ et al., 2010). Each participant (or group) ranked and rated objectives according to importance. Objectives were discussed in the plenary, and the most important ones were chosen by majority vote. We post-processed objectives to avoid common mistakes such as double counting, overlaps, or means objectives (Eisenführ et al., 2010). MCDA objectives are only useful if they discriminate options, in our case FEWS configurations. We dismissed objectives not fulfilling this requirement. In the second workshop, we presented a revised list of the 10 most important objectives, including a clear definition of the best and worst possible cases for each (for attribute descriptions, see Sect. S2.4.1 in the Supplement). For instance, the FEWS being available in several languages is the best case and only in English the worst. After discussion, stakeholders agreed on the final objectives as a basis for MCDA. To operationalize objectives, attributes (synonym indicators) are required (Eisenführ et al., 2010). These were developed by experts from the FANFAR consortium. In most cases, we constructed attributes from several sub-attributes (Sect. 3.6). Sub-attributes or attributes were transformed to a value using marginal value functions (Sect. 3.7).
3.5 Generating FEWS configurations
Different plausible FEWS configurations were generated in the first workshop in three moderated group sessions. Two groups used the “Strategy Generation Table” (Gregory et al., 2012b; Howard, 1988) and one “Brainwriting 635” (Paulus and Yang, 2000) combined with “Cadavre Exquis”, where participants wrote words on a paper and gave it to the next person. The Strategy Generation Table allowed pre-structuring of FEWS elements such as observed variables, forecast production models, and language. Stakeholders chose elements forming suitable FEWS configurations with the help of questions: “The most easy to use FEWS” or the “Most robust FEWS working well given western African boundary conditions such as Internet or power supply problems”. Brainwriting 635 allowed for interactive brainstorming using the same questions. We discussed all FEWS configurations in the plenary. As part of post-processing, FANFAR consortium members created technically interesting FEWS configurations. We provide details in the Supplement for readers unfamiliar with the methods (Sect. S1.1 in the Supplement).
3.6 Predicting the performance of each FEWS configuration
Part of the MCDA input data are scientific predictions (Fig. 1) based on estimates or models of the performance level for each objective (Eisenführ et al., 2010). We used expert estimates by interviewing FANFAR consortium members in July–August 2019 (O'Hagan, 2019). First, experts developed attributes (Sect. 3.4), mostly constructed from sub-attributes. They then estimated the most probable level of each FEWS configuration for each (sub-)attribute and gave uncertainty ranges, for example, for operation costs. For constructed attributes, we integrated the predictions of the sub-attributes into one value using a weighted sum, whereby the weights were defined by experts (Sect. 3.7). We aggregated the uncertainty of each sub-attribute into a single uncertainty distribution with 1000 Monte Carlo simulations. To characterize the resulting aggregated uncertainty, we used a normal distribution with the mean of the Monte Carlo simulation and as standard deviation of the 95 % confidence interval from the simulation. This was used as input in the MCDA (Sect. 3.8).
As an example, the objective 1.1 High accuracy of information consists of three sub-attributes, the KGE index for 1, 3, and 10 d forecasts (Kling–Gupta efficiency; Gupta et al., 2009). The KGE is one possible accuracy index for hydrological model evaluation, e.g., to estimate the error of predicted vs. observed values. For each FEWS configuration and lead day, the expert estimated the KGE. The KGE index number was transformed into a value ranging from 0 (worst) to 1 (best), with a nonlinear marginal value function elicited from the expert. We aggregated the lead-day values into a single value [0:1] with a weighted sum, where the accuracy of the 1 d forecast received a weight of 0.5, the 3 d forecast 0.4, and the 10 d forecast 0.1. Details for predicting system performance, i.e., the expected attribute level, are given in Sect. S2.4 in the Supplement.
3.7 Eliciting stakeholder or expert preferences
Marginal value functions. Subjective preferences of stakeholders enter the MCDA model on an equal footing to expert predictions (Fig. 1). Preference elicitation is an important, sensitive step during which many biases can occur (Montibeller and von Winterfeldt, 2015). Marginal value functions convert the attribute levels for each objective to a common scale, where 0 is the worst possible achievement of this objective and 1 is the best achievement. As an example, the KGE index is an attribute for the objective 1.1 High accuracy of information. The conversion allows integration of attributes with different units into one model, e.g., the KGE index with operation costs (EUR yr−1) and development time (d). As a default, a linear marginal value function can be used. However, nonlinear value functions usually capture preferences better. In FANFAR, most attributes are technical, requiring expert knowledge. We thus elicited shapes of value functions from experts (Sect. 3.6; for details, including figures of value functions, see Sect. S2.4.1). For each sub-attribute, we mostly created seven evenly spaced levels (worst, very bad, bad, neutral, good, very good, and best). Experts then assigned attribute numbers (e.g., KGE index for 3 d forecasts) to each level. We transformed attribute levels to [0:1] values using linear interpolation between levels. As an example, the KGE index ranges from minus infinity (worst case, value 0) to 1 (best case, value 1; Table S8 in the Supplement). For each sub-attribute, we elicited a nonlinear marginal value function (Fig. S5 in the Supplement), allowing aggregation into one value. Because we already used elicited nonlinear value functions to construct the composite attribute, we used a linear value function for these in MCDA (Sect. 3.8).
Weights. In the second FANFAR workshop, we elicited weights from five groups, according to language (French F, English E) and professional background (emergency managers, hydrologists). The two French-speaking groups used the Swing method (Eisenführ et al., 2010): eight emergency managers (group 1. Emergency-F) and 11 hydrologists (two sub-groups 2A. and 2B. Hydrology-F). The two English-speaking groups used an adapted Simos revised card procedure (Figueira and Roy, 2002; Pictet and Bollinger, 2008), hereafter Simos card: 14 hydrologists (3. Hydrology-E) and three emergency managers (4. Emergency-E). We elicited weights from three AGRHYMET experts with the Simos card (5. AGRHYMET-E). Stakeholders can be uncertain about preferences, or groups may disagree. For Swing, we avoided forcing participants to reach group consensus and encouraged discussion of diverging opinions, resulting in a range of weights. We took the mean as the main weight and considered strong deviations in sensitivity analyses (Sect. 3.9). These were weights that differed by more than 0.2 from the mean. For the Simos card, two additional weight sets resulted from eliciting a range for one variable. The moderator recorded important comments to inform sensitivity analyses (Table S3 in the Supplement). For French-speaking hydrologists, two diverging preference sets emerged from the start, which we analyzed separately (2A, 2B). For interested readers, we give details of standard MCDA weight elicitation (Sect. S1.2 in the Supplement). To check for the validity of the additive aggregation model (Sect. 3.8), we briefly discussed implications in the weight sessions using elicitation procedures from our earlier work (Haag et al., 2019a; Zheng et al., 2016).
3.8 MCDA model integrating predictions and preferences
The MCDA model integrates expert predictions with stakeholder preferences and calculates the total value of each FEWS configuration (alternatives; Eisenführ et al., 2010). A finite set of FEWS alternatives is evaluated regarding the predicted outcomes of every objective or attribute. We denote predicted outcomes (Sect. 3.6) as , with xa,i the level of an attribute i that measures a predicted consequence of FEWS a (or b, c, etc.). The total value v(xa) of FEWS a is calculated with a multi-attribute value function, . The resulting total value v(xa) of each FEWS is between 0 (all objectives achieve the worst level) and 1 (all objectives achieve the best level given the attribute ranges). A rational decision maker chooses the FEWS with the highest value. Commonly, an additive model is used:
with parameters , where wi is the weight of attribute i, with , and
and where vi(xi,θ) is the value for the predicted consequence xi of attribute i of FEWS a. This value is inferred with the help of the marginal value function (Sect. 3.7).
While easy to understand, the additive model entails strong assumptions, e.g., that objectives are preferentially independent (Eisenführ et al., 2010). Increasing evidence indicates that many stakeholders do not agree with model implications (Haag et al., 2019a; Reichert et al., 2019; Zheng et al., 2016). Additive aggregation implies that good performance on one objective can fully compensate for poor performance on another. In the FANFAR weight elicitation sessions, we asked stakeholders, using some examples, whether they agree with objectives being preferentially independent and as a consequence with the full compensatory effect. In all five groups this was not the case. We therefore used a non-additive model with less strict requirements, the weighted power mean with an additional parameter γ that determines the degree of non-compensation:
If γ=1, we are back to the additive model in Eq. (1). We used γ=0.2, based on stakeholder input (Sect. 3.7), close to a weighted geometric mean (γ→0). We visualize implications of the power mean in Sect. S1.3 in the Supplement (for details, see Haag et al., 2019b).
We calculated MCDA results in our new open-source software ValueDecisions (Haag et al., 2022), based on R (R Core Team, 2018), with earlier R scripts developed in our group (e.g., Haag et al., 2019b) and the R “utility” package (Reichert et al., 2013). We rendered R scripts as a web application for ValueDecisions with the “shiny” package (Shiny, 2020). We used R for additional analyses: aggregating uncertainty of sub-attributes, weight visualization, and statistical analysis of sensitivity analyses.
3.9 Uncertainty of predictions and preferences
Uncertainty of predictions. Probability theory is used in MAVT (Reichert et al., 2015). We defined uncertainty distributions from expert predictions for each attribute (Sect. 3.6). We calculated aggregated values of each FEWS configuration across all objectives (Sect. 3.8), drawing randomly from the attributes' uncertainty distributions in 1000 Monte Carlo simulation runs. We analyzed rank frequencies: how many times in 1000 runs each FEWS configuration achieved each rank.
Sensitivity analyses of the aggregation model and weights. Local sensitivity analyses are common to check the sensitivity of MCDA results to diverging preferences (e.g., Eisenführ et al., 2010; Haag et al., 2022; Zheng et al., 2016). We checked weights and aggregation models. We used setting S0 as the default, comparing it with a separate MCDA for each setting with changed preference input parameters (settings are summarized in the results in Table 3; for details, see Sect. S1.4 in the Supplement). For each setting, we compared the mean ranks of FEWS configurations from 1000 Monte Carlo runs with the default MCDA (S0). We used the nonparametric Kendall τ correlation coefficient (Kendall, 1938) to measure rank reversals (as in Zheng et al., 2016). To test the aggregation model (Sect. 3.8), we recalculated the MCDA for other reasonable models (Haag et al., 2019a; settings S11–S14; Table 3). For weights, we changed the weight of one objective, while the ratios of all the others were kept constant and renormalized. For more method explanations, see Eisenführ et al. (2010); for details for readers not familiar with MCDA, see Sect. S1.4. Consistency checks during weight elicitation with group 1. Emergency-F revealed an inconsistency and strongly different weights (Fig. S3 in the Supplement). We tested it in sensitivity analysis S21 (Table 3). For Swing weights, stakeholders stated ranges, for which we tested whether the difference between the maximum or minimum from the average weight exceeded Δ=0.02 (S22). For Simos' card, we tested alternative weight sets resulting from the ranges (S23). It is common to test interesting objectives by doubling the elicited weight. We did this for the objective several languages because its importance might have been underestimated (S31).
Cost–benefit visualizations are an additional way of checking the robustness of results (e.g., Liu et al., 2019). We used the standard setting S0 without prediction uncertainty (Table 3) for this visual analysis. For reasons of space, we refer to Sect. S2.9 in the Supplement.
3.10 Discuss results with stakeholders, feedback
We discussed the first MCDA results in the third stakeholder workshop. Workshop four was carried out online due to COVID-19, and we were not able to thoroughly discuss results. We did assess stakeholder perceived satisfaction with FEWS performance during the 2020 rainy season with an online survey, asking the following questions for each objective. (a) “How much does the FANFAR FEWS currently fulfill this objective?” (b) “Would you use the FEWS in future if it remains as is?” (c) “What is the minimum acceptable to you? This means: below which level would you NOT use the FEWS?” (For details, see Sect. S1.5 in the Supplement.)
We ordered MCDA results as in the Methods section. RQB is based on MCDA results, and we address it in the Discussion.
4.1 Stakeholder analysis
Of 249 stakeholders listed by the workshop participants, 68 distinct types remained after data cleaning (for details, see Silva Pinto and Lienert, 2018). Stakeholders perceived to have high influence and to be highly affected by the FANFAR FEWS were national entities for disaster management, water resources, and infrastructure, who were well represented in FANFAR (details in Table S4 in the Supplement). Specific organizations were also perceived to be highly important and affected, e.g., Autorité du Bassin de la Volta (ABV), who participated in workshops, and the consortium member AGRHYMET representing 13 western African states. Other important/affected parties were mainly stakeholders receiving forecasts and alerts such as NGOs, electricity utilities, dam managers, and the agricultural sector. The Red Cross and environmental protection agencies were perceived to have slightly lower importance/affectedness, among others. Civil societies such as communities would be strongly affected but have limited decisional influence on developing the FEWS. In contrast, the media, industry, and commerce were perceived to have more influence but would not be strongly affected. Such outlier stakeholders could potentially provide a different view of the FEWS.
4.2 Objectives and attributes
Objectives covered issues of fundamental importance to stakeholders in view of a good FEWS for western Africa (Fig. 2). Some objectives concerned quality requirements, grouped as 1. High information accuracy and clarity and 2. Good information access, such as accounting for language diversity. Aspects of 3. Low costs and 4. High sustainability were also important, e.g., 42. Skilled labor in western Africa, capable of maintaining, operating, and accessing the FEWS. Each objective is characterized by an attribute for operationalizing the objectives' performance level (Fig. 2; for attribute calculations, see Sect. S2.4).
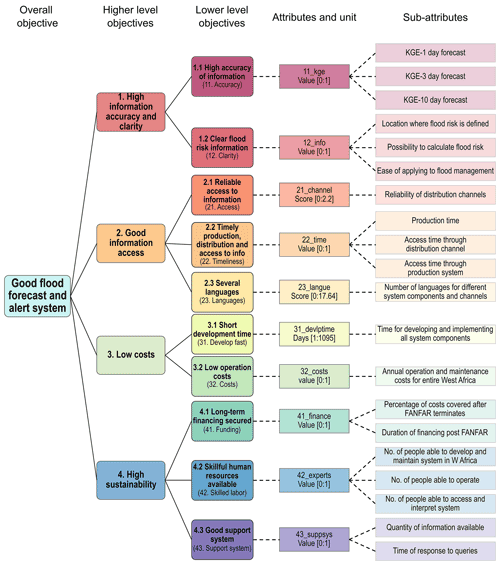
Figure 2Objectives hierarchy. From left to right: overall objective, 4 higher-level fundamental objectives, 10 lower-level fundamental objectives (short names in brackets) and corresponding attributes, and the attributes' unit (usually a value) and range (square brackets), from worst (usually value 0) to best (usually value 1). Most attributes were constructed from sub-attributes (far right).
4.3 FEWS configurations
Stakeholders generated six FEWS configurations in workshop sessions (b–g; Table 2). Experts of the FANFAR consortium developed five configurations (h–k) to cover important technical aspects, such as using refined hydrological models, e.g., redelineation and recalibration of the worldwide HYPE model to western Africa (Andersson et al., 2020b), and including earth observations (EOs) from satellites. FEWSs were constructed in separate sessions with experts from AGRHYMET for the forecast production system and with stakeholders for the user interface IVP (Interactive Visualization Portal). They were combined to form plausible combinations of various FEWS elements (summary of important features in Table 2; for all FEWS elements, see Tables S6 and S7 in the Supplement). Configuration a. Status quo represents roughly the state of the initial FEWS version, when stakeholders started experimentation and giving feedback in the first workshop.
Table 2Overview of 11 FEWS configurations. Selected main characteristics: recent hydrological observation data types (HydObs; WL: water level, Q: river discharge, EOs: earth observations) and meteorological input/forcing data (MetF; HydroGFD; HydroGFD3 – Berg et al., 2021, improved version; HydroGFD-WA: HydroGFD2 adjusted by western African meteorological observations; Am: American meteorological forecasts (e.g., GFS); Ens: ECMWF ensemble meteorological forecasts); hydrological models (WWH: World-Wide HYPE); forecast output variables (Q: river discharge; WL: water level; P: precipitation; E: evaporation; SM: soil moisture; WQ: water quality); data download (Excel: table for the selected station); distribution channels (Web: web visualization; H-TEP: login to H-TEP to download data; FTP: FANFAR and national FTP; API: application programming interface; SoMed: social media, e.g., WhatsApp; ConMed: conventional media, e.g., radio, TV; Tradit: traditional word of mouth) and automatization (Automatic: automatic push of data to distribution channels; Manual: automatic processing with manual control of distribution by the operator); flood hazard reference threshold types (RP Sim: return period based on simulations; RP Obs: return periods based on observations at gauged locations; HistY: selected historic year; Local: user-defined thresholds for a specific location); language of user interface (En: English; Fr: French; Pt: Portuguese; Ar: Arabic).
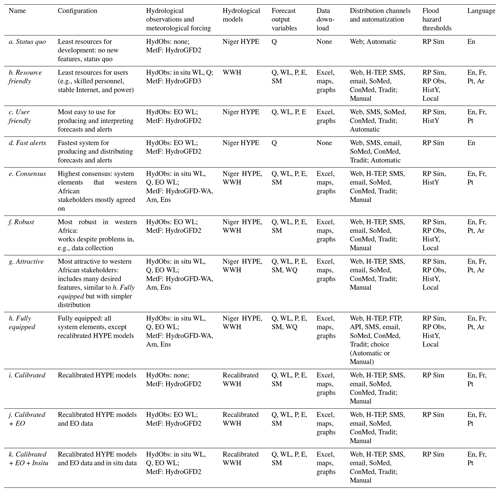
4.4 Predicted performance of each FEWS configuration
Based on expert predictions but excluding stakeholder preferences, no FEWS configuration achieved the best levels of all the objectives (Fig. 3; for details, see Sect. S2.4; raw input data for MCDA modeling in Table S30 in the Supplement). This illustrates the impossibility of designing a perfect FEWS given the inherent trade-offs between achieving objectives. For instance, the status quo pre-operational FEWS a. Status quo achieved the highest values for the objectives 31. Develop fast (short development time) and 32. Costs but scored low on many others, such as 11. Accuracy, 12. Clarity, 21. Access, and 22. Timeliness of information. FEWS achieving high levels for objectives of 1. High information accuracy and clarity cannot achieve 31. Develop fast well at low 32. Costs. Therefore, it is not possible to clearly determine the “best” FEWS based on only the predicted performance (Fig. 3). We require stakeholder input about the importance of objectives (Sects. 4.5 and 4.6).
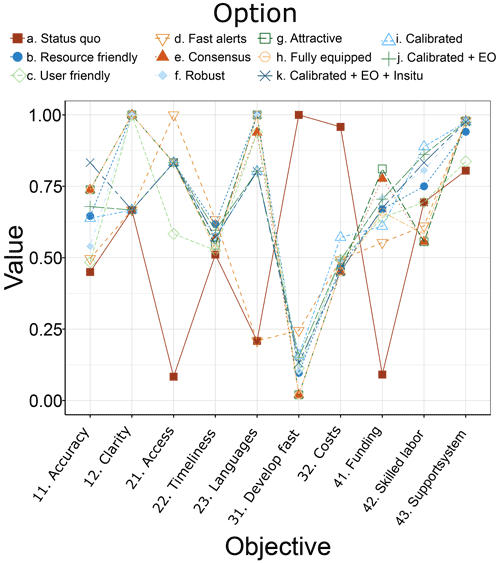
Figure 3Predicted values (y axis) of 11 FEWS configurations (options a–k; symbols) for 10 objectives (x axis), based on expert predictions but not including stakeholder preferences. Value 1: this FEWS configuration achieved the best level of this objective; 0: the FEWS achieved the worst level given the ranges of underlying attributes (i.e., it is a relative scaling from best to worst).
4.5 Stakeholder preferences
The elicited weights (w) for the four higher-level objectives were similar for all groups (w is total bar length; Fig. 4), except for the French-speaking emergency managers (1. Emergency-F). These gave a high weight (w=0.25) to 3. Low costs, which was least important for the others (0.1–0.12). They reasoned that all four higher-level objectives are equally important in emergency situations with a connected chain of events. In contrast, the higher-level objectives 1. High information accuracy and clarity and 2. Good information access were usually the most important for the other groups. There were some notable differences in the importance of lower-level objectives. Again, group 1. Emergency-F was exceptional in assigning much lower weights to objectives they considered unimportant (objectives 23, 31, 41, and 43). They argued that the goal in emergencies is to save lives, and FEWS development should focus on achieving fast access to flood alerts (22. Timeliness; w=0.21) and on personnel that can deal with this information (42. Skilled labor; w=0.25). Weights in the other groups were more balanced (details in Sect. S2.6 in the Supplement). There was varying agreement about weights within a group, reflected in the length of error bars (Fig. 4).
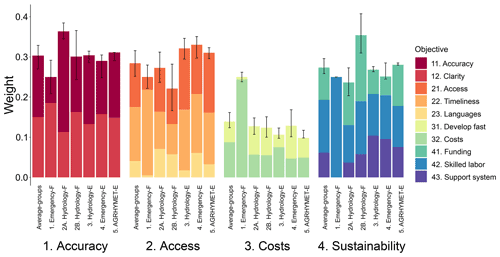
Figure 4Weights (y axis) assigned to higher-level objectives (blocks 1. Accuracy, 2. Access, etc.) colored by weights of lower-level objectives (11. Accuracy, 12. Clarity, etc.), averaged over all six stakeholder groups (Average groups) and for each group (1. Emergency-F, 2A. Hydrology-F, etc; x axis). Error bars: uncertainty of elicited preferences, i.e., the sum of uncertainties of all lower-level objectives within the branch of the respective higher-level objective. Per definition, all weights of a group sum up to 1.
4.6 MCDA model results
No FEWS configuration clearly outperformed the others for all the stakeholder groups in the standard MCDA (setting S0; Table 3) that did not consider uncertainty (Fig. 5; for details, see Tables S32 and S33 in the Supplement). The FEWS at the beginning of the project (a. Status quo) achieved the lowest total values (v<0.46) and the last ranks for all the stakeholder groups, except group 1. Emergency-F (v=0.64, rank 5). This was caused by their different weight preferences. All the other FEWSs generally reached high values for all the groups, with small differences between the groups. The total value ranged from v=0.55 in the worst case (d. Fast alerts for group 2A. Hydrology-F) to 0.70 (b. Resource friendly for 3. Hydrology-E). This FEWS, b. Resource friendly, seemed somewhat better than the others, achieving a high value for all the groups (v=0.65–0.70), thus reaching the first rank for all, again with the exception of group 1. Emergency-F, for which it still achieved the second rank. For better understanding, [0,1] values can be interpreted as percentages, and b. Resource friendly achieved 65 %–70 % of the ideal case over all the objectives in all the stakeholder groups. FEWS configurations f. Robust, i. Calibrated, j. Calibrated + EO, and k. Calibrated + EO + Insitu also performed well (v=0.63–0.70) for all the groups, while c. User friendly and d. Fast alerts achieved the lowest values (v=0.55–0.64).
Table 3Results of sensitivity analyses. Setting S0: default with elicited preferences of stakeholder groups and the weighted power mean model – Eq. (3). Settings S11–S14: effect of other aggregation models (varying γ). S21–S22: uncertainty of Swing weights. S231–S232: uncertainty of Simos' card weights. S31: increased (possibly underestimated) weight. S11–S31: all other parameters as S0. Column groups 1–5: Kendall's τ rank correlation coefficient between ranks of FEWS in the main MCDA (setting S0) and ranks resulting from MCDA using other settings (S11–S31) for stakeholder groups (e.g., group 1. Emergency-F). Column mean: correlation between S0 and the average rank over all groups for which analysis was done. Note: S21 was only done for group 1. Emergency-F (i.e., mean is group correlation). Kendall's τ 1: identical ranks; 0: no correlation; −1: inverse relationship; –: not applicable. Kendall's τ from 0.81 to 1.00: bold, indicating very good agreement between the changed setting and S0; τ from 0.61 to 0.80: italics.
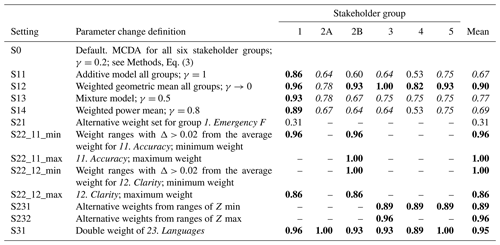
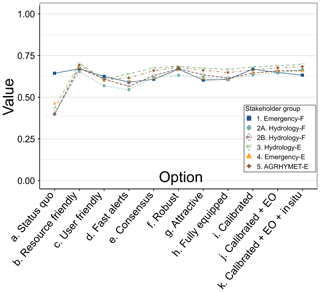
Figure 5Total aggregated value (y axis) of 11 FEWS configurations (x axis) for six stakeholder groups (symbols), without uncertainty. Higher values indicate that they better achieved the objectives, given expert predictions and stakeholders' preferences.
Including the uncertainty of expert predictions in MCDA with Monte Carlo simulation clarified results. The FEWSs b. Resource friendly and f. Robust performed well, achieving the highest ranks for all stakeholder groups in 1000 simulation runs (Fig. 6; details in Table S34 in the Supplement). The FEWSs i. Calibrated and j. Calibrated + EO achieved good to medium ranks for most groups in most runs. Poor performance was achieved by a. Status quo (except for group 1. Emergency-F) and d. Fast alerts, which hit the last ranks in most of the simulation runs. The remaining FEWSs performed somewhere in between.
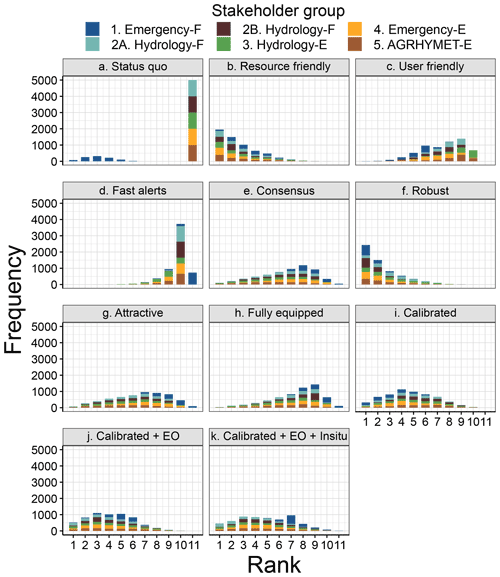
Figure 6Ranks of 11 FEWS configurations, including uncertainty of expert predictions. Frequency (y axis): how often each FEWS (blocks, a. Status quo, b. Resource friendly, etc.) achieved a rank (1: best rank, 11: worst; x axis) in each model run for each stakeholder group (stacked bars); 1000 Monte Carlo simulation runs drawing from uncertainty distributions of attribute predictions.
4.7 Sensitivity analyses of stakeholder preferences
FEWS performance was not sensitive to most model changes (Table 3). The least changes in rankings occurred between the standard MCDA (S0) and sensitivity analyses of extreme weight ranges elicited from stakeholders (S22–S232; Table 3): Kendall's τ rank correlations were high, ranging from 0.86 to 1 (1: identical ranking of all FEWSs). Doubling the weight of 23. Languages (S31) hardly impacted the rankings of any stakeholder group. Greater changes occurred using other models. The difference between the standard MCDA (S0) and changed aggregation models increased the more the aggregation parameter γ increased from 0 (geometric mean; S12) over mixture models (S13, S14) to 1 (additive model; S11). Rank correlations were still relatively high between the additive model and S0 (0.53–0.86). Importantly, rankings of the best-performing FEWS, b. Resource friendly and f. Robust, did not change (Sect. S2.8 in the Supplement). For the other configurations, including i. Calibrated, some differences were greater, depending on groups. The greatest changes occurred for alternative weights (S21) in group 1. Emergency-F. Interestingly, this moved the FEWS rankings and values to those of all the other groups. Hence, this group was no longer an outlier and, e.g., a. Status quo clearly performed worst also for 1. Emergency-F (Fig. S40 in the Supplement). Cost–benefit visualizations confirmed that b. Resource friendly, f. Robust, and i. Calibrated are suitable consensus FEWSs (see Sect. S2.9 for reasons of space).
4.8 Stakeholders' perceived satisfaction with current FEWSs
Participant numbers in the online workshop varied from 10 to 19 due to connection problems, which are frequent in western Africa, and related dropouts. The survey was filled out by 12 participants ( %), resulting for the 10 objectives in responses to each question. Most respondents perceived current performance as sufficient for all the objectives, based on the direct questions about future use of the FANFAR FEWS (b) and the inferred difference (c minus a) between how much the FEWS fulfills the respective objective (a) and the minimum acceptable level (c). Across all the objectives, 79 responses were positive, 16 negative, and 25 did not answer question b. For the most important objective, 11. Accuracy, all respondents would use the current FEWS in future (Fig. 7). However, 4 (of 12) respondents indicated that the FEWS does not currently meet their minimum acceptable performance requirements. This result is representative of results for all objectives (for details, see Sect. S2.10 in the Supplement).
The discussion follows the two research questions (Sect. 2.3). Addressing RQA, it was possible to find robust FEWS configurations despite large uncertainties and different stakeholder preferences (Sect. 5.1). Below, we discuss our experience with MCDA regarding uncertainty and eliciting stakeholder preferences. To address RQB, we use the proposed framework (Table 1) to evaluate and discuss participatory MCDA as a transdisciplinary process (Sect. 5.2).
5.1 Finding robust FANFAR FEWS configurations (RQA)
5.1.1 Main MCDA results
As the most important practical result of RQA, we identified three FEWS with good overall performance (Fig. 5). This would be difficult without MCDA given the uncertainty of expert estimates and the model (Fig. 6). Moreover, trade-offs between objectives had to be made (Fig. 3), and stakeholders had different preferences concerning the importance of objectives (Fig. 4). One well-performing FEWS, b. Resource friendly, was created by stakeholders in the first workshop. They chose FEWS components requiring the least resources for western Africa such as skilled personnel, a good Internet connection, or a stable power supply (Table 2). Similarly, stakeholders created f. Robust to reliably work under difficult western African conditions related to collecting in situ data and distributing information via various channels. The third FEWS, i. Calibrated, was created by FANFAR consortium members using refined HYPE models, including, e.g., adjusted delineation and parameter calibration (Andersson et al., 2020b) but excluding earth observation and in situ data. The latter were included in FEWS configurations j and k (Table 2), which, however, were not consistently among the three best-performing configurations. All three best FEWSs achieved 63 %–70 % of all objectives in all stakeholder groups. We consider this a very good value given the existing trade-offs. These FEWSs were robust (i) when including the uncertainty of expert predictions with Monte Carlo simulation (Fig. 6), (ii) in sensitivity analyses of the aggregation model and stakeholders' weight preferences (Table 3), and (iii) in dominance checks in cost–benefit visualizations (Sect. S2.9). Interestingly, these three FEWSs did not incorporate more advanced features: a FEWS that meets stakeholder preferences primarily needs to work accurately and reliably under difficult western African conditions.
5.1.2 Dealing with uncertainty of predictions, preferences, and model assumptions
Attributes operationalize objectives (Eisenführ et al., 2010). Seemingly trivial, this is often challenging. We illustrated this for the KGE index for 1, 3, and 10 d forecasts to measure the objective 11. Accuracy (Sect. 3.6). The uncertainty of expert predictions was relatively large for, e.g., the objectives 11. Accuracy, 22. Timeliness, or 42. Skilled labor but small to inexistent for, e.g., 12. Clarity and 23. Languages (Fig. S30 in the Supplement). The resulting overall uncertainty affected results less than expected (Fig. S35 in the Supplement).
The weights indicated that most groups preferred a FEWS producing accurate, clear, and reliable information, reaching recipients well before floods (11. Accuracy, 12. Clarity, 21. Access, 22. Timeliness; Fig. 4), and western African countries need the capability to handle this information (42. Skilled labor). We captured differences within groups with uncertainty ranges or separate preference sets (e.g., subgroups 2A, 2B; Sects. S1.2.3 and S2.6 in the Supplement). The French-speaking emergency managers (1. Emergency-F) had different preferences compared with all the others. All the groups regarded several languages as unimportant in weight elicitation despite discussing in the plenary that language diversity is crucial. When asked to make trade-offs, they were willing to give up language diversity to achieve accuracy. They were also willing to trade off higher operation and maintenance costs (except group 1. Emergency-F) and development time in return for receiving a functioning, precise FEWS.
Including the uncertainty of expert estimates and stakeholder preferences in MCDA can blur results. For FANFAR, including the uncertainty of predictions helped to better distinguish between FEWS performances (Fig. 6) compared with the standard analysis without uncertainty (Fig. 5). FEWS configurations b. Resource friendly and f. Robust consistently achieved the first ranks in 1000 simulation runs and, e.g., i. Calibrated good to medium ranks. However, some FEWSs, such as k. Calibrated + EO + Insitu, ranked last in numerous runs (Fig. 6) despite achieving good values when uncertainty was disregarded (0.63–0.70; Table S33). Because they ranked last in most runs, a. Status quo and d. Fast alerts would be an imprudent choice.
Local sensitivity analyses (e.g., Zheng et al., 2016) confirmed that b. Resource friendly, f. Robust, and i. Calibrated are robust choices. Changing stakeholder preferences hardly changed MCDA results compared with our standard model (S0; Table 3). Doubling the weight of 23. Languages (S31) did not affect the results in any group, thus avoiding costly translations as a priority. Operation and maintenance costs were candidates for doubling the weight but were already covered by the high weight of group 1. Emergency-F. In this group, sensitivity analyses of weight ranges given by group participants with a different opinion (S21; Table 3) changed the results in such a way that they aligned with results of the other stakeholder groups. This increases our confidence that the three proposed FEWSs are a good consensus. Moreover, the additive MCDA aggregation model (Eq. 1; Sect. 3.8) impacted the FEWS rankings (Table 3). As a standard, we assumed non-additive aggregation (Eq. 3), close to a weighted geometric mean model, based on feedback in weight elicitation sessions. After discussing examples, all the groups stated that poor performance on an important objective should not be compensated by good performance on others, a main implication of additive aggregation. This confirms that the additive model can unintentionally violate a stakeholder's preferences (e.g., Haag et al., 2019a; Reichert et al., 2019; Zheng et al., 2016). Thus, additive aggregation may not be the best model despite its popularity in MCDA applications. For FANFAR, sensitivity analyses sufficed to conclude that additive aggregation has an effect but does not alter the rankings of the best FEWSs. We can safely conclude that the three proposed FEWSs are suitable. We emphasize that the FEWS was continuously improved throughout the project, also after eliciting stakeholder preferences.
5.2 Evaluating participatory MCDA as a transdisciplinary process (RQB)
We critically evaluate participatory MCDA as a transdisciplinary process in a large project following our proposed framework (Table 1). We focus on important aspects of MCDA in a hydrology context, summarizing the main points in Table 4.
Table 4Summary evaluation of the MCDA process using a conceptual framework for transdisciplinary research (Table 1): (1) co-design, (2) co-production, and (3) co-dissemination of knowledge. Symbols: strength of MCDA; well possible with MCDA; + possible contribution by MCDA; 0 not achievable by MCDA; * remark. PSM: problem-structuring method; VFT: value-focused thinking.
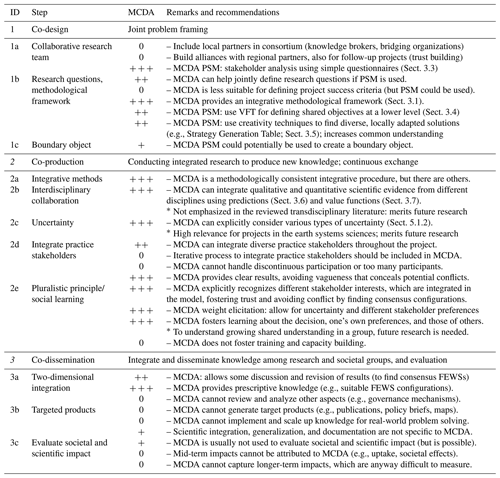
5.2.1 Evaluating the co-design step “joint problem framing”
MCDA does not fully meet all the requirements of this step. Building the collaborative research team cannot be attributed to MCDA, although it was achieved by the FANFAR project (step 1a, Table 1). Two key western African stakeholders were consortium partners from the start: AGRHYMET, who is mandated by 13 western African states and ECOWAS to provide, e.g., operational flood warnings, and NIHSA, the Nigerian Hydrological Services Agency. This follows a decade of collaboration between SMHI and AGRHYMET. Building alliances with regional partners is a transdisciplinary approach identified across projects and may lead to follow-up partnerships (Wuelser et al., 2021). Trust building is crucial, and AGRHYMET is clearly a bridging organization or knowledge broker between research and implementation (Norstrom et al., 2020; Wuelser et al., 2021; Lemos and Morehouse, 2005). FANFAR was co-led by western African partners and engaged stakeholders in workshops, thus meeting the principle of creating knowledge tailored to specific contexts (Caniglia et al., 2021; Norstrom et al., 2020). However, this cannot be attributed to MCDA, nor defining the research questions (step 1b) or boundary object (step 1c, Table 1). The boundary object was to produce an operational FEWS, which allowed stakeholders to commit (Jahn et al., 2012). Scientists and stakeholders both aimed to achieve this goal, which helped in overcoming unbalanced ownership (Lang et al., 2012). The FANFAR consortium agreed to use MCDA as an integrative methodological framework (1b) to achieve this goal and integrate different scientific disciplines (Lemos and Morehouse, 2005; Mauser et al., 2013; Lang et al., 2012). MCDA is one possible useful, stringent, and integrative methodology to produce transferable knowledge (Wuelser et al., 2021).
Narrowing the perspective to the concrete project with western African stakeholders, MCDA emphasizing early problem structuring is helpful (Marttunen et al., 2017; Rosenhead and Mingers, 2001). Taking practitioners on board from the start and avoiding insufficient legitimacy or underrepresentation of actors is crucial (Lang et al., 2012; Wuelser et al., 2021). Stakeholder mapping or social network analysis is suitable for identifying those to involve (Norstrom et al., 2020; Lang et al., 2012). As the first step of MCDA, we carried out stakeholder analysis (step 1a, Table 1). This is rarely done and was documented in only 9 % of 333 reviewed MCDA papers (Marttunen et al., 2017). We used relatively simple questionnaires (Sect. 3.3) to discover who has influence or is affected by a FEWS (Grimble and Wellard, 1997; Lienert et al., 2013; Reed et al., 2009). We identified 68 distinct stakeholder types (Sect. 4.1). In workshops, we included hydrologists from 17 countries and key supranational organizations such as AGRHYMET who produce flood information (Table S4; for details, see Silva Pinto and Lienert, 2018). The main receivers of FEWS information also participated: emergency managers from every country. Thanks to their experience, we elaborated on the alert dissemination chain and elements of effective FEWSs (Kuller et al., 2021). We identified missing parties, e.g., agriculture, industry, or humanitarian aid organizations. Some provided informal feedback on the FEWSs through social media. We did not invite them because more than 50 participants in workshops are ineffective. Indeed, pluralistic co-production while keeping processes manageable is a challenge in transdisciplinary projects (Norstrom et al., 2020; Lang et al., 2012).
Problem structuring is decisive because MCDA results critically depend on objectives and options, i.e., FEWS configurations in our case (Marttunen et al., 2017; Rosenhead and Mingers, 2001). These MCDA steps were carried out in the first workshop (Fig. 1; Sects. 3.4 and 3.5). They helped define shared goals and a success measure (e.g., Norstrom et al., 2020; step 1b, Table 1): to find a FEWS that achieves the objectives. Following value-focused thinking (Keeney, 1996), we first generated objectives in small groups using different methods (Sect. 3.4). This ensured a broad diversity and helped avoid “group think bias” (Janis, 1972). We are confident that we captured the 10 most important objectives that cover fundamental aims of western African stakeholders (Fig. 2). Moreover, many environmental applications of MCDA use too many objectives (Marttunen et al., 2018). This is ineffective and burdens MCDA weight elicitation. We excluded some objectives in plenary discussions.
We could not assume that all participants had sufficient technical knowledge to create FEWS configurations, but we aimed to avoid “myopic problem representation” (Montibeller and von Winterfeldt, 2015). The Strategy Generation Table is especially suitable (Gregory et al., 2012b; Howard, 1988). It allows pre-structuring of objectives while stimulating creative stakeholder inputs. The context-based principle of co-production includes asking for constraining factors (Norstrom et al., 2020): when creating FEWS, the necessity of considering the western African situation became evident, including frequent power cuts and slow Internet connections. Moreover, we realized that stakeholders had not created all potentially interesting FEWS configurations. An advantage of multi-attribute value theory is that options can be included later (Reichert et al., 2015; Eisenführ et al., 2010). The FANFAR consortium created additional FEWS covering technical aspects, e.g., ensemble meteorological forecasts, redelineation and calibration of hydrological models, and assimilation of EO and in situ water levels (FEWS h–k, Tables 2 and S6). During post-processing, we also created the FEWS at project start, a. Status quo, as a benchmark. Indeed, it performed poorly for most groups (Fig. 5). In summary, the three MCDA steps of stakeholder analysis, creating objectives, and FEWS took up large parts of the first workshop in western Africa. They were very helpful for stakeholders to exchange ideas, express their needs, and develop a common understanding, contributing to co-design step 1 (Table 4).
5.2.2 Evaluating the co-production step “integrated research to produce new knowledge”
Consistent integrative methods and systematic procedures for integrating bodies of knowledge are crucial (step 2a, Table 1) but less visible in the literature (Wuelser et al., 2021; Lang et al., 2012; Mauser et al., 2013). Recommendations include generating hazard maps or sensitivity and multi-criteria assessments (i.e., MCDA). Identifying stakeholders' positions and preferred options allows involvement of people in creating their future (Wuelser et al., 2021). In FANFAR, MCDA clearly helped in structuring the co-design process and integrating different knowledge types: expert estimates of how well each FEWS performs (Sect. 4.4) and stakeholder preferences (Sect. 4.5). Moreover, western African stakeholders experimented with the FEWS at each workshop, tested it in rainy seasons, and provided feedback (see Wuelser et al., 2021), which cannot be attributed to MCDA.
Transdisciplinary projects rely on interdisciplinary collaboration and on integrating evidence from different disciplines (step 2b, Table 1; Jahn et al., 2012; Lemos and Morehouse, 2005; Mauser et al., 2013). Integrating qualitative data for policy and decision making and quantitative data for models can be challenging (Lang et al., 2012). MCDA handles this by transforming attributes of different measurement units to a common value from 0 (objective not achieved) to 1 (fully achieved) using value functions (Sect. 3.7). The attributes can include qualitative scales. In FANFAR, experts provided attribute estimates: western African and European hydrologists, IT specialists, and decision analysts (Sect. S2.4.1). MCDA integrates very specific data, in our case the predictions about FEWS performance. Other evidence types also need integration in transdisciplinary projects, and other methods are available. This area merits future research given the lack of emphasis in the current literature.
“Questions of the uncertainty of the results” (Mauser et al., 2013, p. 428) were emphasized by these earth systems scientists for global sustainability but scarcely addressed by others (step 2c, Table 1). We included the uncertainty of expert predictions by eliciting probability distributions for each attribute (Sect. S2.4.1) and Monte Carlo simulation (Sect. 3.9). Local sensitivity analyses addressed uncertainty of the model and of stakeholder preferences (Sect. 5.1.2; discussed in Reichert et al., 2015). Handling uncertainty in a conceptually valid way is essential for transdisciplinary research in the earth systems sciences.
The importance of integrating practice stakeholders in iterative processes (step 2d, Table 1) was underlined by many (e.g., Lemos and Morehouse, 2005; Norstrom et al., 2020). Our iterative workshop series to test and improve the FEWS cannot be attributed to MCDA. Practical MCDA projects often consist of three stakeholder workshops: for problem structuring, preference elicitation, and discussing results and revising options, i.e., FEWS (Fig. 1). Discontinuous participation can be a challenge (Lang et al., 2012), and FANFAR faced changing numbers and compositions of participants (Sect. 3.2). Like Lang et al. (2012), we also encountered the opposite: increasing requests over time and the challenge of keeping participant numbers manageable. We integrated new participants, e.g., by presenting the FEWS and MCDA objectives at each workshop. For MCDA, discontinuous participation was unproblematic, as new participants in the second workshop accepted the objectives (Fig. 2) and FEWSs (Table 2). Our participant sample was presumably sufficiently large and diverse to cover the main aspects. Another challenge can be vague results using methods such as sustainability visions, which may conceal potential conflicts (Lang et al., 2012). MCDA has the strength of providing clear results, even for uncertain data (Sect. 5.1.2).
The pluralistic principle aims at creating social learning across multiple axes (step 2e, Table 1). Sustained interaction with stakeholders, jointly searching for solutions, and joint learning foster trust, mutual understanding, and shared perspectives (e.g., Lemos and Morehouse, 2005; Norstrom et al., 2020; Schneider et al., 2019). It is not always necessary to reach consensus, but different expertise, perspectives, values, and interests must be recognized (e.g., Norstrom et al., 2020; Wuelser et al., 2021). Moreover, collaboratively engaging with conflicts is needed to rationalize contested situations (Schneider et al., 2019; Caniglia et al., 2021). A strength of MCDA is that opposing stakeholder interests are part of the methodology, hereby often avoiding conflict about solutions (Arvai et al., 2001; Gregory et al., 2012a, b; Marttunen and Hamalainen, 2008). During weight elicitation, we encouraged stakeholders to discuss diverging preferences (Sect. 3.7), and we recommend allowing for such uncertainty. Allowing for uncertainty can help participants to construct their own preferences (Lichtenstein and Slovic, 2006), can enable learning and understanding of alternative perspectives, and may inform sensitivity analyses (Sect. 4.7). In FANFAR, conflicting preferences did not change the rankings of FEWS, and we were able to identify consensus FEWSs (Sect. 5.1.2). In other cases, sensitivity analyses based on diverging preferences can help construct better FEWSs. Moreover, “Assessing the [interactive] principle should also focus on capturing learning, how the perceptions of actors change throughout the process, and the degree to which a shared perspective emerges” (Norstrom et al., 2020, p. 188). Such research is rare in MCDA but was attempted in FANFAR and a Swiss project (Kuller et al., 2022). Results were ambiguous, but we found shared agreement of FANFAR stakeholders about the most important objectives. More research to better understand individual cognitive and group decision-making processes is needed (Kuller et al., 2022).
Training and capacity building belong to the pluralistic principle (step 2e, Table 1). Many of the 31 analyzed transdisciplinary projects provided, e.g., training or attractive visualizations of recent research (Schneider et al., 2019). Capacity building can be promoted by working in the integrated ways discussed above or with capacity-building courses (Wuelser et al., 2021; Caniglia et al., 2021). FANFAR offered many training and capacity-building opportunities, which cannot be attributed to MCDA.
5.2.3 Evaluating the co-dissemination and evaluation step “integrating and disseminating knowledge”
Two-dimensional integration (step 3a, Table 1) implies that outcomes are discussed and revised from scientific and societal perspectives (Mauser et al., 2013; Lang et al., 2012). Discussing transformation knowledge includes measures, tools, or governance mechanisms to create change (Schneider et al., 2019). It can include prescriptive knowledge by recommending suitable options (Caniglia et al., 2021). This is a strength of MCDA: we provided detailed information about robust FEWS configurations (Sect. 5.1). Moreover, MCDA results are discussed with stakeholders, and new FEWSs could be constructed (Fig. 1). We could not carry out the fourth FANFAR workshop due to COVID-19 but collected online feedback. Stakeholders were quite satisfied with the FANFAR FEWS performance during the 2020 rainy season (Fig. 7). While not meeting the requirements of extensive discussions, it was the best available approach. We are currently carrying out a systematic daily reforecasting experiment covering 1991–2020 for five model configurations and aim to link results to expert satisfaction. Understanding governance mechanisms is beyond the scope of MCDA, in our case, ways to facilitate uptake of the FEWS in the whole of western Africa.
Target products (step 3b, Table 1) should address the original problem, be understandable, and be accessible to users (Lemos and Morehouse, 2005; Schneider et al., 2019; Lang et al., 2012). Products include technical publications, data visualizations, and open-access online databases (Schneider et al., 2019). In FANFAR, products cannot be attributed to MCDA. The main product is the FEWS, which includes operational data collection, assimilation, hydrological modeling, interpretation, and distribution through web visualization and APIs. Hereby, MCDA only supported the design. Additional products are a multilingual knowledge base (https://fanfar.eu/support/, last access: 2 June 2022), an open-source code (https://github.com/hydrology-tep/fanfar-forecast, last access: 2 June 2022), and video tutorials (https://www.youtube.com, search: HYPEweb FANFAR, last access: 2 June 2022). Ensuring consistent access, maintenance, updates, and improvements after project termination is challenging (Lemos and Morehouse, 2005). AGRHYMET has the authority to drive the FEWS uptake and already uses it, e.g., in their MSc curriculum or at PRESASS and PRESAGG forums (WMO, 2021), thus supporting the ECOWAS flood management strategy. Nevertheless, operationalization after EU financing is not secured.
Products should contribute to scientific progress, a major challenge being inadequate generalization of case study solutions (Lang et al., 2012; Jahn et al., 2012). Products are often not reported in the scholarly literature (Wuelser et al., 2021), the knowledge thus not advancing scientific progress and not being adopted in similar projects. We aimed to overcome this with this paper and other outputs (FANFAR, 2021). We document the MCDA process and provide details in the Supplement. We encourage hydrologists to use this material. We stress that it is not necessary to conduct a full MCDA in every case. The first problem-structuring steps can create useful insights and may be easier to apply (Sect. 5.2.1).
The last step 3c (Table 1) is to evaluate societal and scientific impact. Project evaluation is possible with MCDA, but MCDA was not used in FANFAR. Short-term impacts include increased citations or attention of nonacademic actors, e.g., high download rates or media coverage (Norstrom et al., 2020; Schneider et al., 2019). As an example, the FANFAR workshop in Nigeria was featured on the national TV news. Building social capacities and establishing stakeholder networks or communities of practice can be very helpful (Lemos and Morehouse, 2005; Schneider et al., 2019). As another example, a FANFAR social media group among western African stakeholders monitored the severe 2020 floods, which in many places were successfully forecasted by the FANFAR FEWS. Mid-term impacts include uptake of products and societal effects such as strategy implementation or amended legislation (Jahn et al., 2012; Norstrom et al., 2020). Long-term impacts are very difficult to measure as they are typically realized far beyond project termination (Norstrom et al., 2020; Schneider et al., 2019). Moreover, due to the complexity of problems in transdisciplinary projects, causal relationships are difficult to establish (Lang et al., 2012). To secure future sustainability of the FANFAR FEWS, several dialogs with potential financiers were held and 12 proposals submitted to date. Four were successful so far, providing funding for some parts of FANFAR, such as for hydrometric stations or additional training. The sustainability strategy focuses on the financing of operations, maintenance, dissemination, technical development, etc. More importantly, it addresses long-term collaboration, capacity development, transfer of responsibilities, and anchoring FANFAR in the routines of western African institutions. As one example of societal impact, NIHSA, the Nigeria Hydrological Services Agency, reported that an early FEWS warning in September 2020 saved approximately 2500 lives. The warning helped evacuate five communities before the flood destroyed more than 200 houses.
The MCDA process enabled us to find three good FANFAR FEWS configurations, which is important to western African stakeholders and people affected by floods. All stakeholder groups preferred a relatively simple FEWS producing accurate, clear, and accessible flood risk information that reaches recipients well before floods. To achieve this, most groups would trade off higher operation and maintenance costs, development time, and several languages. MCDA indicated that the three FEWSs are robust. They achieved 63 %–70 % of all 10 objectives despite diverging stakeholder preferences, model uncertainty, and uncertain expert predictions. Including uncertainty and stakeholders in MCDA is neglected in flood risk research. We highly recommend both: MCDA including uncertainty allowed us to better distinguish between FEWSs, and participatory MCDA focusing on stakeholders' objectives (value-focused thinking) helped avoid conflicts about FEWS configurations.
MCDA meets many but not all requirements of sustainability science and transdisciplinary research. Our proposed evaluation framework proved very useful for critically analyzing MCDA and specifically includes elements crucial to the earth systems sciences. We evaluated MCDA as a transdisciplinary process along the three framework steps. MCDA only partially contributes to co-design (step 1). However, if understood as a process including problem structuring, MCDA supports joint problem framing. Stakeholder analysis helps identify those to involve. Problem structuring includes creativity techniques for defining shared objectives and designing options, the FEWS configurations in our case. The main benefit of participatory MCDA lies in co-production (step 2). Interdisciplinary knowledge integration and uncertainty were rarely emphasized in the literature and could be research contributions of the earth systems sciences. Both are strengths of MCDA. MCDA also provides clear results and consensus FEWSs by integrating conflicting stakeholder interests into the model. However, MCDA does not achieve many aspects of co-dissemination well (step 3). MCDA results are discussed with stakeholders, but this focus is narrow. MCDA does not achieve important elements such as analyzing governance mechanisms and implementing actions and products. In FANFAR, we thus carried out complementary activities.
This paper documents in detail the participatory MCDA process for co-developing a good FEWS for western Africa, together with many stakeholders in the FANFAR project. The MCDA can serve as a blueprint for engaging in such transdisciplinary endeavors. Our MCDA emphasized the integration of stakeholders, of interdisciplinary expert knowledge, and of uncertainty, which is rarely done in flood risk research using MCDA. These aspects are certainly of high importance to other projects in the earth systems sciences. Moreover, we analyzed the strengths and limits of using MCDA in a large, international transdisciplinary project with the help of a framework based on the literature. This framework can generally support colleagues from the earth system sciences when engaging in complex transdisciplinary research with stakeholders and society.
The data are available in the Eawag Research Data Institutional Collection ERIC open (https://opendata.eawag.ch/, Eawag, 2022; https://doi.org/10.25678/000629, Lienert et al., 2022).
In the Supplement, we provide ample material to guide readers unfamiliar with MCDA through all steps. This includes a Methods section (generating FEWS configurations, eliciting weights, MCDA model, sensitivity analyses, stakeholder feedback), and a Results section (stakeholder analysis, objectives and attributes, FEWS, predictions, value functions, weights, MCDA results, and stakeholder feedback). The supplement related to this article is available online at: https://doi.org/10.5194/hess-26-2899-2022-supplement.
A video of an interview with Jafet Andersson and Aishat Ibrahim about the FANFAR project for the European Development Days (the Green Deal for a Sustainable Future; 15–16 June 2021, no. edd21) is available here: https://youtu.be/OrrpG6wZmhI (Andersson and Ibrahim, 2021). A video introducing the visualization portal of FANFAR is available here: https://youtu.be/GjGUkapNpus (FANFAR consortium, 2020).
JL was responsible for conceptualization of this research, funding acquisition, investigation and data collection, methodology development, project administration, acquiring resources, supervision, validation of data collection and analyses, writing of the original draft of the first manuscript, rewriting it, and revising this paper. JCMA was responsible for funding acquisition, investigation, project administration, and reviewing and editing all versions of this paper. DH carried out data curation, formal analyses, visualization of results, and validation and contributed to writing the original manuscript. FSP carried out the first formal analyses, collected data and developed the methodology, administrated the project, and reviewed and edited different versions of this paper. MK collected data, administrated the project, supervised collaborators, and reviewed and edited different versions of this paper.
The contact author has declared that neither they nor their co-authors have any competing interests.
Publisher's note: Copernicus Publications remains neutral with regard to jurisdictional claims in published maps and institutional affiliations.
This article is part of the special issue “Contributions of transdisciplinary approaches to hydrology and water resources management”. It is not associated with a conference.
FANFAR received funding from the EU Horizon 2020 research and innovation program under grant agreement no. 780118: FANFAR (Reinforced cooperation to provide operational flood forecasting and alerts in West Africa (FANFAR, 2021). We thank Eawag, the Swiss Federal Institute of Aquatic Science and Technology, for funding the COVID-19 prolongation period. We sincerely thank Abdou Ali, Addi Shuaib Olorunoje, Aishat Ibrahim, Alice Aubert, Aytor Naranjo, Berit Arheimer, Bernard Minoungou, Bode Gbobaniyi, Cletus Musa, David Gustafsson, Emilie Breviere, Emmanuel Mathot, Fabrizio Pacini, Fridolin Haag, Kevin Schönholzer, Léonard Santos, Lorna Little, Melissande Machefer, Mohamed Hamatan, Philipp Beutler, Sara Schmid, Tharcisse Ndayizigiye, and Umar Magashi for invaluable support of workshops, data collection, and analysis. We are truly grateful for the inspiring collaboration with workshop participants and sincerely thank them for their kindness and enthusiasm. We thank six anonymous reviewers and the editors of this special issue for helpful feedback.
This research has been supported by Horizon 2020 (FANFAR (grant no. 780118)) and Eawag the Eidgenössische Anstalt für Wasserversorgung Abwasserreinigung und Gewässerschutz (Eawag Discretionary Funds – to offset impacts of COVID-19).
This paper was edited by Thomas Thaler and reviewed by three anonymous referees.
Abdullah, M. F., Siraj, S., and Hodgett, R. E.: An overview of Multi-Criteria Decision Analysis (MCDA) application in managing water-related disaster events: Analyzing 20 years of literature for flood and drought events, Water, 13, 1358, https://doi.org/10.3390/w13101358, 2021.
Aich, V., Liersch, S., Vetter, T., Fournet, S., Andersson, J. C. M., Calmanti, S., van Weert, F. H. A., Hattermann, F. F., and Paton, E. N.: Flood projections within the Niger River Basin under future land use and climate change, Sci. Total Environ., 562, 666–677, https://doi.org/10.1016/j.scitotenv.2016.04.021, 2016.
Andersson, J. and Ibrahim, A.: Interview about the FANFAR project for the European Development Days (the Green Deal for a Sustainable Future; 15–16 June 2021, #edd21), https://youtu.be/OrrpG6wZmhI (last access: 9 June 2022), 2021.
Andersson, J., Ali, A., Arheimer, B., Crochemore, L., Gbobaniyi, B., Gustafsson, D., Hamatan, M., Kuller, M., Lienert, J., Machefer, M., Magashi, U., Mathot, E., Minoungou, B., Naranjo, A., Ndayizigiye, T., Pacini, F., Silva Pinto, F., Santos, L., and Shuaib, A.: Flood forecasting and alerts in West Africa – experiences from co-developing a pre-operational system at regional scale, EGU General Assembly 2020, online, 4–8 May 2020, https://doi.org/10.5194/egusphere-egu2020-7660, 2020a.
Andersson, J., Santos, L., Isberg, K., Gustafsson, D., Musuuza, J., Minoungou, B., and Crochemore, L.: Deliverable: D3.2. Report documenting and explaining the hydrological models, European Union, Horizon 2020, Innovation Action ICT programme, project 780118 FANFAR, https://doi.org/10.13140/RG.2.2.17369.85601, 41 pp., 2020b.
Andersson, J. C. M., Arheimer, B., Traoré, F., Gustafsson, D., and Ali, A.: Process refinements improve a hydrological model concept applied to the Niger River basin, Hydrol. Process., 31, 4540–4554, https://doi.org/10.1002/hyp.11376, 2017.
Arheimer, B., Pimentel, R., Isberg, K., Crochemore, L., Andersson, J. C. M., Hasan, A., and Pineda, L.: Global catchment modelling using World-Wide HYPE (WWH), open data, and stepwise parameter estimation, Hydrol. Earth Syst. Sci., 24, 535–559, https://doi.org/10.5194/hess-24-535-2020, 2020.
Arvai, J. L., Gregory, R., and McDaniels, T. L.: Testing a structured decision approach: Value-focused thinking for deliberative risk communication, Risk Anal., 21, 1065–1076, https://doi.org/10.1111/0272-4332.216175, 2001.
Basher, R.: Global early warning systems for natural hazards: systematic and people-centred, Philos. T. Roy. Soc. A, 364, 2167–2180, https://doi.org/10.1098/rsta.2006.1819, 2006.
Belton, V. and Stewart, T. S.: Multiple Criteria Decision Analysis: An Integrated Approach, Springer Science+Business Media Springer, Dordrecht, The Netherlands, https://doi.org/10.1007/978-1-4615-1495-4, ISBN 978-1-4613-5582-3, 978-1-4615-1495-4, 2002.
Berg, P., Almén, F., and Bozhinova, D.: HydroGFD3.0 (Hydrological Global Forcing Data): a 25 km global precipitation and temperature data set updated in near-real time, Earth Syst. Sci. Data, 13, 1531–1545, https://doi.org/10.5194/essd-13-1531-2021, 2021.
Bierens, S., Boersma, K., and van den Homberg, M. J. C.: The legitimacy, accountability, and ownership of an impact-based forecasting model in disaster governance, Politics Gov., 8, 445–455, https://doi.org/10.17645/pag.v8i4.3161, 2020.
Bond, S. D., Carlson, K. A., and Keeney, R. L.: Generating objectives: Can decision makers articulate what they want?, Manage. Sci., 54, 56–70, https://doi.org/10.1287/mnsc.1070.0754, 2008.
Caniglia, G., Luederitz, C., von Wirth, T., Fazey, I., Martin-Lopez, B., Hondrila, K., Konig, A., von Wehrden, H., Schapke, N. A., Laubichler, M. D., and Lang, D. J.: A pluralistic and integrated approach to action-oriented knowledge for sustainability, Nat. Sustain., 4, 93–100, https://doi.org/10.1038/s41893-020-00616-z, 2021.
Convertino, M., Annis, A., and Nardi, F.: Information-theoretic portfolio decision model for optimal flood management, Environ. Modell. Softw., 119, 258–274, https://doi.org/10.1016/j.envsoft.2019.06.013, 2019.
de Brito, M. M. and Evers, M.: Multi-criteria decision-making for flood risk management: a survey of the current state of the art, Nat. Hazards Earth Syst. Sci., 16, 1019–1033, https://doi.org/10.5194/nhess-16-1019-2016, 2016.
de Brito, M. M., Evers, M., and Almoradie, A. D. S.: Participatory flood vulnerability assessment: a multi-criteria approach, Hydrol. Earth Syst. Sci., 22, 373–390, https://doi.org/10.5194/hess-22-373-2018, 2018.
Eisenführ, F., Weber, M., and Langer, T.: Rational Decision Making, Springer, Berlin, Heidelberg, New York, ISBN 978-3-642-02850-2, 2010.
ERIC open (Eawag Research Data Institutional Collection), Eawag: Swiss Federal Institute of Aquatic Science and Technology, 8600 Dübendorf, Switzerland, https://opendata.eawag.ch/, last access: 2 June 2022.
Evers, M., Almoradie, A., and de Brito, M. M.: Enhancing Flood Resilience Through Collaborative Modelling and Multi-criteria Decision Analysis (MCDA), in: Urban Disaster Resilience and Security: Addressing Risks in Societies, edited by: Fekete, A. and Fiedrich, F., Urban Book Series, 221–236, https://doi.org/10.1007/978-3-319-68606-6_14, ISBN 978-3-319-68606-6, 978-3-319-68605-9, 2018.
FANFAR: Operational flood forecasting and alerts in West Africa. A transdisciplinary international project funded by the European Union, https://fanfar.eu and https://cordis.europa.eu/project/id/780118/results, last access: 30 September 2021.
FANFAR consortium: Video introducing the visualization portal of FANFAR, Youtube, https://youtu.be/GjGUkapNpus (last access: 9 June 2022), 2020.
Figueira, J. and Roy, B.: Determining the weights of criteria in the ELECTRE type methods with a revised Simos' procedure, Eur. J. Oper. Res., 139, 317–326, https://doi.org/10.1016/S0377-2217(01)00370-8, 2002.
Gregory, R., Failing, L., Harstone, M., Long, G., McDaniels, T., and Ohlson, D.: Structured decision making: A practical guide to environmental management choices, Wiley-Blackwell Publishing, ISBN 978-1-4443-3341-1, 978-1-4443-3342-8, 2012a.
Gregory, R., Long, G., Colligan, M., Geiger, J. G., and Laser, M.: When experts disagree (and better science won't help much): Using structured deliberations to support endangered species recovery planning, J. Environ. Manage., 105, 30–43, https://doi.org/10.1016/j.jenvman.2012.03.001, 2012b.
Grimble, R., and Wellard, K.: Stakeholder methodologies in natural resource management: A review of principles, contexts, experiences and opportunities, Agr. Syst., 55, 173–193, https://doi.org/10.1016/S0308-521X(97)00006-1, 1997.
Gupta, H. V., Kling, H., Yilmaz, K. K., and Martinez, G. F.: Decomposition of the mean squared error and NSE performance criteria: Implications for improving hydrological modelling, J. Hydrol., 377, 80–91, https://doi.org/10.1016/j.jhydrol.2009.08.003, 2009.
Haag, F., Lienert, J., Schuwirth, N., and Reichert, P.: Identifying non-additive multi-attribute value functions based on uncertain indifference statements, Omega-Int. J. Manage. S., 85, 49–67, https://doi.org/10.1016/j.omega.2018.05.011, 2019a.
Haag, F., Reichert, P., Maurer, M., and Lienert, J.: Integrating uncertainty of preferences and predictions in decision models: An application to regional wastewater planning, J. Environ. Manage., 252, 109652–109652, https://doi.org/10.1016/j.jenvman.2019.109652, 2019b.
Haag, F., Zuercher, S., and Lienert, J.: Enhancing the elicitation of diverse decision objectives for public planning, Eur. J. Oper. Res., 279, 912–928, https://doi.org/10.1016/j.ejor.2019.06.002, 2019c.
Haag, F., Aubert, A. H., and Lienert, J.: ValueDecisions, a web app to support decisions with conflicting objectives, multiple stakeholders, and uncertainty, Environ. Modell. Softw., 150, 105361, https://doi.org/10.1016/j.envsoft.2022.105361, 2022.
Howard, R. A.: Decision-analysis – Practice and promise, Manage. Sci., 34, 679–695, https://doi.org/10.1287/mnsc.34.6.679, 1988.
Jahn, T., Bergmann, M., and Keil, F.: Transdisciplinarity: Between mainstreaming and marginalization, Ecol. Econ., 79, 1–10, https://doi.org/10.1016/j.ecolecon.2012.04.017, 2012.
Janis, I. L.: Victims of Groupthink, Houghton Mifflin, New York, ISBN 13 978-0395140444, ISBN 10 0395140447, 1972.
Keeney, R. L.: Decision-Analysis – an Overview, Oper. Res., 30, 803–838, https://doi.org/10.1287/opre.30.5.803, 1982.
Keeney, R. L.: Value-focused thinking: Identifying decision opportunities and creating alternatives, Eur. J. Oper. Res., 92, 537–549, https://doi.org/10.1016/0377-2217(96)00004-5, 1996.
Kendall, M. G.: A new measure of rank correlation, Biometrika, 30, 81–93, https://doi.org/10.2307/2332226, 1938.
Kuller, M., Silva Pinto, F., Schönholzer, K., and Lienert, J.: Effective risk communication for early flood warning in West-Africa, EGU General Assembly 2020, online, 4–8 May 2020, https://doi.org/10.5194/egusphere-egu2020-9425, 2020.
Kuller, M., Schönholzer, K., and Lienert, J.: Creating effective flood warnings: a framework from a critical review, J. Hydrol., 602, 126708, https://doi.org/10.1016/j.jhydrol.2021.126708, 2021.
Kuller, M., Beutler, P., and Lienert, J.: Preference change in stakeholder group-decision processes in the public sector: extent, causes and implications, Eur. J. Oper. Res., in review, 2022.
Lang, D. J., Wiek, A., Bergmann, M., Stauffacher, M., Martens, P., Moll, P., Swilling, M., and Thomas, C. J.: Transdisciplinary research in sustainability science: practice, principles, and challenges, Sustain. Sci., 7, 25–43, https://doi.org/10.1007/s11625-011-0149-x, 2012.
Lemos, M. C. and Morehouse, B. J.: The co-production of science and policy in integrated climate assessments, Global Environ. Chang., 15, 57–68, https://doi.org/10.1016/j.gloenvcha.2004.09.004, 2005.
Lichtenstein, S. and Slovic, P. (Eds.): The Construction of Preference, Cambridge University Press, ISBN 978-0-521-83428-5, https://www.cambridge.org/ch/academic/subjects/psychology/cognition/construction-preference#OJjDl3E664yCXSzp.97 (last access: 9 June 2022), 2006.
Lienert, J., Schnetzer, F., and Ingold, K.: Stakeholder analysis combined with social network analysis provides fine-grained insights into water infrastructure planning processes, J. Environ. Manage., 125, 134–148, https://doi.org/10.1016/j.jenvman.2013.03.052, 2013.
Lienert, J., Scholten, L., Egger, C., and Maurer, M.: Structured decision-making for sustainable water infrastructure planning and four future scenarios, EURO Journal on Decision Processes, 3, 107–140, https://doi.org/10.1007/s40070-014-0030-0, 2015.
Lienert, J., Andersson, J., Hofmann, D., Silva Pinto, F., and Kuller, M.: Deliverable D2.2. Report on the co-design workshops in FANFAR to create a flood forecast and alert system for West Africa European Union, Horizon 2020, Innovation Action ICT programme, project 780118 FANFAR, https://www.dora.lib4ri.ch/eawag/islandora/object/eawag:21545 (last access: 3 June 2022), Deliverable D2.2, 29 pp., 2020.
Lienert, J., Andersson, J. C. M. , Hofmann, D., Silva Pinto, F., and Kuller, M.: Data for scientific paper (on Eawag Research Data Institutional Collection, ERIC): The role of MCDA in a transdisciplinary process: Co-developing a flood forecasting system in West Africa, https://doi.org/10.25678/000629, 2022.
Liu, S., Maclean, K., and Robinson, C.: A cost-effective framework to prioritise stakeholder participation options, EURO Journal on Decision Processes, 7, 221–241, https://doi.org/10.1007/s40070-019-00103-7, 2019.
Lumbroso, D., Brown, E., and Ranger, N.: Stakeholders' perceptions of the overall effectiveness of early warning systems and risk assessments for weather-related hazards in Africa, the Caribbean and South Asia, Nat. Hazards, 84, 2121–2144, https://doi.org/10.1007/s11069-016-2537-0, 2016.
Marttunen, M. and Hamalainen, R. P.: The decision analysis interview approach in the collaborative management of a large regulated water course, Environ. Manage., 42, 1026–1042, https://doi.org/10.1007/s00267-008-9200-9, 2008.
Marttunen, M., Lienert, J., and Belton, V.: Structuring problems for Multi-Criteria Decision Analysis in practice: A literature review of method combinations, Eur. J. Oper. Res., 263, 1–17, https://doi.org/10.1016/j.ejor.2017.04.041, 2017.
Marttunen, M., Belton, V., and Lienert, J.: Are objectives hierarchy related biases observed in practice? A meta-analysis of environmental and energy applications of Multi-Criteria Decision Analysis, Eur. J. Oper. Res., 265, 178–194, https://doi.org/10.1016/j.ejor.2017.02.038, 2018.
Marttunen, M., Haag, F., Belton, V., Mustajoki, J., and Lienert, J.: Methods to inform the development of concise objectives hierarchies in multi-criteria decision analysis, Eur. J. Oper. Res., 277, 604–620, https://doi.org/10.1016/j.ejor.2019.02.039, 2019.
Massazza, G., Tarchiani, V., Andersson, J. C. M., Ali, A., Ibrahim, M. H., Pezzoli, A., De Filippis, T., Rocchi, L., Minoungou, B., Gustafsson, D., and Rosso, M.: Downscaling Regional Hydrological Forecast for Operational Use in Local Early Warning: HYPE Models in the Sirba River, Water, 12, 3504, https://doi.org/10.3390/w12123504 2020.
Mauser, W., Klepper, G., Rice, M., Schmalzbauer, B. S., Hackmann, H., Leemans, R., and Moore, H.: Transdisciplinary global change research: the co-creation of knowledge for sustainability, Curr. Opin. Env. Sust., 5, 420–431, https://doi.org/10.1016/j.cosust.2013.07.001, 2013.
Montibeller, G. and von Winterfeldt, D.: Cognitive and motivational biases in decision and risk analysis, Risk Anal., 35, 1230–1251, https://doi.org/10.1111/risa.12360, 2015.
Nachappa, T. G., Piralilou, S. T., Gholamnia, K., Ghorbanzadeh, O., Rahmati, O., and Blaschke, T.: Flood susceptibility mapping with machine learning, multi-criteria decision analysis and ensemble using Dempster Shafer Theory, J. Hydrol., 590, 125275, https://doi.org/10.1016/j.jhydrol.2020.125275, 2020.
NBA (Niger Basin Authority): SATH-NBA (Satellite based water monitoring and flow forecasting for the Niger River Basin, http://www.sath.abn.ne/, last access: 9 November 2020.
Nka, B. N., Oudin, L., Karambiri, H., Paturel, J. E., and Ribstein, P.: Trends in floods in West Africa: analysis based on 11 catchments in the region, Hydrol. Earth Syst. Sci., 19, 4707–4719, https://doi.org/10.5194/hess-19-4707-2015, 2015.
Norstrom, A. V., Cvitanovic, C., Lof, M. F., West, S., Wyborn, C., Balvanera, P., Bednarek, A. T., Bennett, E. M., Biggs, R., de Bremond, A., Campbell, B. M., Canadell, J. G., Carpenter, S. R., Folke, C., Fulton, E. A., Gaffney, O., Gelcich, S., Jouffray, J. B., Leach, M., Le Tissier, M., Martin-Lopez, B., Louder, E., Loutre, M. F., Meadow, A. M., Nagendra, H., Payne, D., Peterson, G. D., Reyers, B., Scholes, R., Speranza, C. I., Spierenburg, M., Stafford-Smith, M., Tengo, M., van der Hel, S., van Putten, I., and Osterblom, H.: Principles for knowledge co-production in sustainability research, Nat. Sustain., 3, 182–190, https://doi.org/10.1038/s41893-019-0448-2, 2020.
O'Hagan, A.: Expert knowledge elicitation: subjective but scientific, Am. Stat., 73, 69–81, https://doi.org/10.1080/00031305.2018.1518265, 2019.
OCHA: West and Central Africa: flooding situation, UN Office for the Coordination of Humanitarian Affairs (OCHA), https://reliefweb.int/report/niger/west-and-central-africa-flooding-situation-23-october-2020 (last access: 3 June 2022), 2, 2020.
Pang, B., Yue, J. J., Huang, Z. Q., and Zhang, R.: Parameter uncertainty assessment of a flood forecasting model using multiple objectives, J. Flood Risk Manag., 12, e12493, https://doi.org/10.1111/jfr3.12493, 2019.
Passerotti, G., Massazza, G., Pezzoli, A., Bigi, V., Zsótér, E., and Rosso, M.: Hydrological Model Application in the Sirba River: Early Warning System and GloFAS Improvements, Water, 12, 620, https://doi.org/10.3390/w12030620, 2020.
Paulus, P. B. and Yang, H. C.: Idea generation in groups: A basis for creativity in organizations, Organ. Behav. Hum. Dec., 82, 76–87, https://doi.org/10.1006/obhd.2000.2888, 2000.
Perera, D., Seidou, O., Agnihotri, J., Rasmy, M., Smakhtin, V., Coulibaly, P., and Mehmood, H.: Flood early warning systems: a review of benefits, challenges and prospects, United Nations University Institute for Water, Environment and Health (UNU-INWEH), https://inweh.unu.edu/flood-early-warning-systems-a-review-of-benefits-challenges-and-prospects/ (last access: 3 June 2022), Hamilton, Canada, 26, 2019.
Pham, B. T., Luu, C., Phong, T. V., Nguyen, H. D., Le, H. V., Tran, T. Q., Ta, H. T., and Prakash, I.: Flood risk assessment using hybrid artificial intelligence models integrated with multi-criteria decision analysis in Quang Nam Province, Vietnam, J. Hydrol., 592, 125815, https://doi.org/10.1016/j.jhydrol.2020.125815, 2021.
Pictet, J. and Bollinger, D.: Extended use of the cards procedure as a simple elicitation technique for MAVT. Application to public procurement in Switzerland, Eur. J. Oper. Res., 185, 1300–1307, https://doi.org/10.1016/j.ejor.2006.05.051, 2008.
R Core Team: R: A language and environment for statistical computing, Version 3.3.1, R Foundation for Statistical Computing, http://www.R-project.org/ (last access: 3 June 2022), Vienna, Austria, 2018.
Reed, M. S., Graves, A., Dandy, N., Posthumus, H., Hubacek, K., Morris, J., Prell, C., Quinn, C. H., and Stringer, L. C.: Who's in and why? A typology of stakeholder analysis methods for natural resource management, J. Environ. Manage., 90, 1933–1949, https://doi.org/10.1016/j.jenvman.2009.01.001, 2009.
Reichert, P., Schuwirth, N., and Langhans, S. D.: Constructing, evaluating and visualizing value and utility functions for decision support, Environ. Modell. Softw., 46, 283–291, https://doi.org/10.1016/j.envsoft.2013.01.017, 2013.
Reichert, P., Langhans, S. D., Lienert, J., and Schuwirth, N.: The conceptual foundation of environmental decision support, J. Environ. Manage., 154, 316–332, https://doi.org/10.1016/j.jenvman.2015.01.053, 2015.
Reichert, P., Niederberger, K., Rey, P., Helg, U., and Haertel-Borer, S.: The need for unconventional value aggregation techniques: experiences from eliciting stakeholder preferences in environmental management, EURO Journal on Decision Processes, 7, 197–219, https://doi.org/10.1007/s40070-019-00101-9, 2019.
Ronco, P., Bullo, M., Torresan, S., Critto, A., Olschewski, R., Zappa, M., and Marcomini, A.: KULTURisk regional risk assessment methodology for water-related natural hazards – Part 2: Application to the Zurich case study, Hydrol. Earth Syst. Sci., 19, 1561–1576, https://doi.org/10.5194/hess-19-1561-2015, 2015.
Rosenhead, J. and Mingers, J.: Rational Analysis for a Problematic World Revisited: Problem Structuring Methods for Complexity, Uncertainty and Conflict, John Wiley & Sons, Chichester, ISBN 978-0-471-49523-9, 2001.
Roudier, P., Ducharne, A., and Feyen, L.: Climate change impacts on runoff in West Africa: a review, Hydrol. Earth Syst. Sci., 18, 2789–2801, https://doi.org/10.5194/hess-18-2789-2014, 2014.
Samanta, S., Koloa, C., Pal, D. K., and Palsamanta, B.: Flood Risk Analysis in Lower Part of Markham River Based on Multi-Criteria Decision Approach (MCDA), Hydrology, 3, 29, https://doi.org/10.3390/hydrology3030029, 2016.
Santos, L., Andersson, J. C. M., and Arheimer, B.: Evaluation of parameter sensitivity of a rainfall-runoff model over a global catchment set, Hydrolog. Sci. J., 67, 342–357, https://doi.org/10.1080/02626667.2022.2035388, 2022.
Schneider, F., Giger, M., Harari, N., Moser, S., Oberlack, C., Providoli, I., Schmid, L., Tribaldos, T., and Zimmermann, A.: Transdisciplinary co-production of knowledge and sustainability transformations: Three generic mechanisms of impact generation, Environ. Sci. Policy, 102, 26–35, https://doi.org/10.1016/j.envsci.2019.08.017, 2019.
Shiny: Web Application Framework for R, R package version 1.5, https://cran.r-project.org/web/packages/shiny/index.html (last access: 3 June 2022), 2020.
Silva Pinto, F. and Lienert, J.: Report activities to establish co-design committee, stakeholder analysis, European Union, Horizon 2020, Innovation Action ICT programme, project 780118 FANFAR, https://www.dora.lib4ri.ch/eawag/islandora/object/eawag%3A23886 (last access: 3 June 2022), Deliverable D2.1, 48 pp., 2018.
Sultan, B., Lejeune, Q., Menke, I., Maskell, G., Lee, K., Noblet, M., Sy, I., and Roudier, P.: Current needs for climate services in West Africa: Results from two stakeholder surveys, Clim. Serv., 18, 100166, https://doi.org/10.1016/j.cliser.2020.100166, 2020.
Tang, Z. Q., Zhang, H., Yi, S. Z., and Xiao, Y. F.: Assessment of flood susceptible areas using spatially explicit, probabilistic multi-criteria decision analysis, J. Hydrol., 558, 144–158, https://doi.org/10.1016/j.jhydrol.2018.01.033, 2018.
UNISDR: Early warning practices can save lives: selected examples. Good practices and lessons learned, United Nations Secretariat of the International Strategy for Disaster Reduction (UNISDR), https://www.unisdr.org/files/15254_EWSBBLLfinalweb.pdf (last access: 3 June 2020), Bonn, Germany, 2010.
WMO: 2020 State of climate services: risk information and early warning systems, WMO (World Meteorological Organization), https://library.wmo.int/index.php?lvl=notice_display&id=21777#.YeU1A_go8ab (last access: 3 June 2022), 2020.
WMO: Regional Climate Outlook Forums, WMO (World Meteorological Organization), https://public.wmo.int/en/our-mandate/climate/regional-climate-outlook-products, last access: 25 February 2021.
Wuelser, G., Adler, C., Breu, T., Hadorn, G. H., Wiesmann, U., and Pohl, C.: On which common ground to build? Transferable knowledge across cases in transdisciplinary sustainability research, Sustain. Sci., 16, 1891–1905, https://doi.org/10.1007/s11625-021-01010-0, 2021.
Zheng, J., Egger, C., and Lienert, J.: A scenario-based MCDA framework for wastewater infrastructure planning under uncertainty, J. Environ. Manage., 183, 895–908, https://doi.org/10.1016/j.jenvman.2016.09.027, 2016.