the Creative Commons Attribution 4.0 License.
the Creative Commons Attribution 4.0 License.
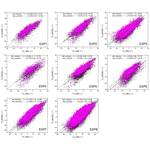
Improved parameterization of snow albedo in Noah coupled with Weather Research and Forecasting: applicability to snow estimates for the Tibetan Plateau
Massimo Menenti
Rongmingzhu Su
Nan Yao
Weiqiang Ma
Snow albedo is important to the land surface energy balance and to the water cycle. During snowfall and subsequent snowmelt, snow albedo is usually parameterized as functions of snow-related variables in land surface models. However, the default snow albedo scheme in the widely used Noah land surface model shows evident shortcomings in land–atmosphere interaction estimates during snow events on the Tibetan Plateau. Here, we demonstrate that our improved snow albedo scheme performs well after including snow depth as an additional factor. By coupling the Weather Research and Forecasting (WRF) and Noah models, this study comprehensively evaluates the performance of the improved snow albedo scheme in simulating eight snow events on the Tibetan Plateau. The modeling results are compared with WRF run with the default Noah scheme and in situ observations. The improved snow albedo scheme significantly outperforms the default Noah scheme in relation to air temperature, albedo and sensible heat flux estimates by alleviating cold bias estimates, albedo overestimates and sensible heat flux underestimates, respectively. This in turn contributes to more accurate reproductions of snow event evolution. The averaged root mean square error (RMSE) relative reductions (and relative increase in correlation coefficients) for air temperature, albedo, sensible heat flux and snow depth reach 27 % (5 %), 32 % (69 %), 13 % (17 %) and 21 % (108 %), respectively. These results demonstrate the strong potential of our improved snow albedo parameterization scheme for snow event simulations on the Tibetan Plateau. Our study provides a theoretical reference for researchers committed to further improving the snow albedo parameterization scheme.
- Article
(4456 KB) - Full-text XML
- BibTeX
- EndNote
The surface albedo directly determines the proportion of incident solar radiation that is absorbed by the surface and is an important parameter in climate and land surface models (LSMs) (Sellers et al., 1996). Small changes in surface albedo can affect the energy balance in the land–atmosphere system and can drive both local and global climate change (Bloch, 1964).
Surface albedo changes dramatically during snowfall and snowmelt cycles. Much research has been carried out to identify the factors that influence these changes, including the effects of terrain shielding, altitude, sky conditions, vegetation, and snow properties such as grain size, liquid water content, depth, and impurities (Warren and Wiscombe, 1980; Wiscombe and Warren, 1980; Aoki et al., 2003; Jonsell et al., 2003; Hansen and Nazarenko, 2004; Liang et al., 2005; Wang et al., 2015; He et al., 2018a). This body of research has led to the development of many parameterization schemes for surface albedo (Oerlemans and Knap, 1998; Wang et al., 2007; Bao et al., 2008; Li and Hu, 2009; Gardner and Sharp, 2010; Kuipers Munneke et al., 2011; Malik et al., 2014; Dang et al., 2015; He et al., 2017, 2018b; Meng and Li, 2019; Saito et al., 2019; Wang et al., 2020). Most snow albedo parameterization schemes depend on statistical empirical formulas and constant parameters rather than on representing physical snow albedo feedback processes. To improve the performance of snow albedo parameterization schemes for simulating land–atmosphere interactions, Bao and Lü (2009) added consideration of solar zenith angle to a regional climate model, which resulted in a 1.2 ∘C temperature increase and considerably improved the cold bias in East Asia and improved the representation of diurnal ground temperature changes in northwestern China. Park and Park (2016) investigated the effect of vegetation on snow-covered surface albedo and improved the winter surface albedo estimates from their LSM by including leaf and stem indices in the snow albedo parameterization scheme, which reduced the root mean square error (RMSE) by 69 %. Zhong et al. (2017) considered aerosol radiative effects on snow processes in their simulations and successfully reproduced the snow albedo and snow depth. Fresh snow albedo depends on snow depth, and albedo parameterization schemes that fail to account for this generally overestimate the snow depth. To address this, Wang et al. (2020) developed a new albedo scheme for fresh snow, which accounts for the relationship between fresh snow albedo, snow grain size and snow depth, resulting in improved snow depth estimates during the snow ablation period on the Tibetan Plateau. This highlights the importance of accounting for the effect of snow depth on fresh snow albedo.
A coupled land–atmosphere model can provide useful insights into conditions on the Tibetan Plateau, where the terrain is complex and there are few and unevenly distributed observation stations (Maussion et al., 2011; Yuan et al., 2016; Norris et al., 2017; Bonekamp et al., 2018; Rahimi et al., 2019). However, the parameterization scheme for surface albedo in the Noah LSM, which is currently the most widely used LSM, does not account for all the factors that influence albedo. It includes many predetermined parameters and an approximate treatment of vegetation, soil and snow, which can result in some inaccuracies in the estimated surface albedo (Wen et al., 2011; Liu et al., 2019). For example, the surface albedo parameterization scheme in the Noah LSM considers snow cover and age but ignores other snow-related factors, such as snow depth, that can drive dramatic changes in albedo (Ek et al., 2003). This makes it inappropriate to use the Noah LSM to characterize changes in snow albedo that follow from snowfall and snowmelt processes in complex topographic areas. However, the Noah LSM appears to be the most readily available snow albedo scheme for long-term climate modeling research (Rai et al., 2019). Despite its shortcomings, the Noah albedo parameterization scheme does provide substantial improvements to estimates of the magnitude and timing of both the peak snowfall amount and the maximum snow cover extent, following from the scheme's consideration of snow albedo decay and liquid water refreezing (Livneh et al., 2010). The above issues represent opportunities for improvements to be made to the snow albedo parameterization scheme in the Noah LSM.
The use of an advanced snow albedo parameterization scheme is crucial for accurate estimation of land–atmosphere interactions over the Tibetan Plateau, where the snow albedo effect is extremely strong. It has been shown that the Weather Research and Forecasting (WRF; Skamarock et al., 2008) model, when coupled with the default Noah albedo parameterization scheme, results in an apparent cold bias over the Tibetan Plateau (Gao et al., 2015; Meng et al., 2018; Liu et al., 2019). This bias can be reduced by including albedo products from the Moderate Resolution Imaging Spectroradiometer (MODIS) and an additional snow depth parameter as independent variables in the Noah albedo parameterization scheme (Liu, 2020). This approach is not the same as assimilating satellite-retrieved snow-related products into the LSM, which has also been shown to lead to improvements (Xu and Shu, 2014; Zhang et al., 2014; Lin et al., 2016; Xue et al., 2019). This improved snow albedo scheme has been successfully implemented in the WRF model, coupled with Noah, to simulate land–atmosphere interactions during a regional heavy snow event on the Tibetan Plateau (Liu, 2020). However, it has not been shown that the improvements that follow from the improved snow albedo scheme are universal over the Tibetan Plateau, and this should be studied further. Severe snowfall occurs often over the southern Tibetan Plateau, while snowfall over the eastern Tibetan Plateau is generally of relatively weak intensity, and the rate of snowmelt varies widely depending on the heterogeneous underlying surfaces. This makes it necessary to carry out numerical experiments that focus on snow events over the eastern and southern Tibetan Plateau to assess how reliably the improved scheme can be used to characterize different snowfall intensities and snowmelt processes.
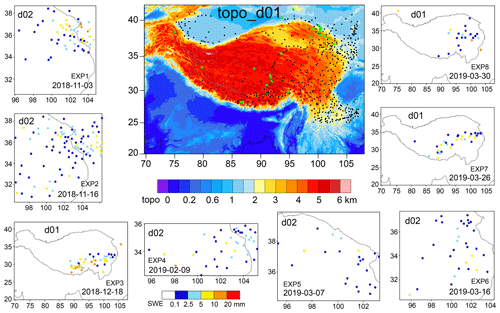
Figure 1WRF domains of d01 and d02 and CMA observations of daily snowfall amount in colored solid circles for the eight experiments. The topographical height of d01 is shaded with black solid circles indicating the locations of the CMA stations and green solid circles indicating the locations of the CAS stations and the Qilian Mountains integrated observatory network.
In this study, we selected eight moderate to snowstorm events that occurred over the southern and eastern Tibetan Plateau to assess the universality of the improvements offered by our improved snow albedo scheme in WRF coupled with the Noah LSM. For each snow event, two numerical experiments were carried out: one implementing the default Noah snow albedo scheme and the other implementing our improved snow albedo scheme. The model performance was assessed through comparison of the modeled air temperature, albedo, snow depth, turbulent heat and vapor fluxes with ground observations. The aim of this study is to explore the potential of our improved snow albedo parameterization scheme to simulate snow events over the whole Tibetan Plateau more accurately than can be done using the standard default scheme. We hope that this study will also provide a useful reference for researchers working to develop and improve this and other albedo parameterization schemes.
2.1 Description of snow events
We selected China Meteorological Administration (CMA) national observation stations on the Tibetan Plateau and in the surrounding regions, with elevations exceeding 1000 m. This resulted in a total of 502 stations (Fig. 1 shows their distribution). Snowfall events were identified from the hourly air temperature and precipitation observations from all 502 stations when the air temperature was below 0 ∘C. Daily snowfall amounts were calculated using 08:00 BST (Beijing Standard Time) as the start and end time for each day. The standards to define snowfall grade were taken from the China Meteorological Standardization Network (http://www.cmastd.cn/standardView.jspx?id=479, last access: 3 September 2021). Using these standards, eight different grades of snowfall event were considered in our study, including moderate, heavy and snowstorm. Most of the snowfall events took place over the eastern Tibetan Plateau, and some occurred in a large region across the south and center to the eastern Tibetan Plateau. The maximum daily snowfall amount from all snowfall events exceeded 8 mm, and four events resulted in more than 10 mm daily snowfall, making these snowstorm-grade events. Snow depth is much greater on the southern Tibetan Plateau (>50 cm) than it is on the eastern and central Tibetan Plateau ( cm). The description of eight snowfall events, including the date and location of moderate- to snowstorm-grade events, the maximum snow depth and daily snowfall amount, is detailed in Table 1. The daily snowfall amounts from the eight snowfall events are shown in Fig. 1.
2.2 Model description and experiment configuration
The WRF model (Skamarock et al., 2008), version 3.7.1, coupled with the Noah LSM, was used to simulate the eight snowfall events in this study. It is a fully compressible, non-hydrostatic model and includes a run-time hydrostatic option. Vertical levels are determined using a mass-based terrain-following hydrostatic pressure coordinate, and calculations are performed on an Arakawa C grid. The model uses second- and third-order Runge–Kutta time integration schemes and second- to sixth-order advection schemes in both the horizontal and vertical directions.
The extremely steep terrain on the central and southern Tibetan Plateau led to model instability and failure for snowfall events 3, 7 and 8 when a relatively fine horizontal resolution of 1 km was used; however, the calculations remained stable when the resolution was increased to 5 km. We therefore used two-way nested modeling domains in our model configuration for snowfall events 1, 2, 4, 5 and 6 and a single modeling domain for snowfall events 3, 7 and 8. The coarse domain (d01) was used to simulate synoptic-scale atmospheric conditions over 20.0–42.0∘ N and 69.7–108.0∘ E with a horizontal resolution of 5 km. The inner domain (d02) had a horizontal resolution of 1 km, and event 1 occupied 876×966 grid cells, event 2 occupied 976×1001 cells, event 4 was resolved by 966×451 cells, event 5 was calculated over 781×686 cells, and event 6 covered 926×881 cells. The vertical structure of both domains included 35 unevenly spaced layers and extended up to 50 hPa. The model was configured to use the Noah LSM in d01 and d02 to describe all land–atmosphere interactions, the Thompson scheme to represent microphysical processes, the Dudhia scheme to represent shortwave radiation, the RRTM scheme to describe longwave radiation, the YSU scheme to describe the planetary boundary layer, and only in d01 to use the Kain–Fritsch cumulus parameterization scheme for clouds.
We conducted numerical experiments to simulate snow event 1 (EXP1), event 2 (EXP2), event 3 (EXP3), event 4 (EXP4), event 5 (EXP5), event 6 (EXP6), event 7 (EXP7) and event 8 (EXP8). The model domains for the experiments are shown in Fig. 1. Each experiment included two model simulations: one implementing the default Noah snow albedo parameterization scheme and the other implementing a new improved snow albedo scheme. The new improved snow albedo was developed based on a conversion formula from MODIS narrowband spectral reflectance to broadband albedo following Liang (2000) and an albedo calculation formula about fresh snow, firn and bare-ground albedo, snow age and depth following Oerlemans and Knap (1998). MODIS broadband albedo and WRF modeled snow depth and age were used to estimate the related parameters, i.e., firn albedo and scales of snow depth and age through nonlinear fitting of the above albedo calculation formula. The final nonlinear fitting results produced the new improved snow albedo scheme; see Eqs. (3) and (4). The snow albedo is parameterized using Eqs. (1) and (2) in the default Noah land surface scheme (Livneh et al., 2010) and using Eqs. (3) and (4) in the improved scheme (Liu, 2020):
where A and B are constants equal to 0.94 and 0.58, respectively, for snow accumulation periods, and are 0.82 and 0.46, respectively, for other periods; αbg is the background albedo, which depends on the land cover type; “sc” is snow cover and ranges from 0 to 1; αmax is fresh snow albedo; αsnow is snow albedo; t is the snow age in units of days; d is snow depth in meters.
The fifth-generation European Centre for Medium-Range Weather Forecasts (ECMWF) reanalysis data set (ERA5), with a spatial resolution of and 3 h temporal resolution, provided initial and boundary conditions for our numerical experiments. The ERA data were calculated using 4DVar data assimilation in CY41R2 from the ECMWF's Integrated Forecast System, with 137 vertical hybrid sigma/pressure levels, extending to 0.01 hPa. ERA5 is freely available from the website https://www.ecmwf.int/en/forecasts/datasets/reanalysis-datasets/era5 (3 September 2021). The near-real-time MODIS land cover product was used to replace the outdated land cover in the WRF preprocessing system. We ran the model from before the onset of each snowfall event until and after all snowmelt following the event had ceased. EXP1 was run from 1 to 8 November 2018, EXP2 was run from 13 to 18 November 2018, EXP3 was run from 16 to 20 December 2018, EXP4 was run from 5 to 11 February 2019, EXP5 was run from 2 to 10 March 2019, EXP6 was run from 12 to 18 March 2019, EXP7 was run from 24 to 29 March 2019, and EXP8 was run from 28 March to 7 April 2019. The model results were output at 3 h intervals, and the first day was used for model spinup.
Table 2Locations of stations from the Chinese Academy of Sciences (CAS) and the Qilian Mountains integrated observatory network and whether or not observations of albedo, SH and LH were used from each station.
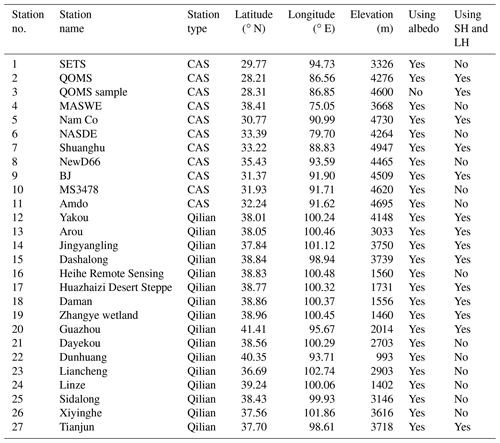
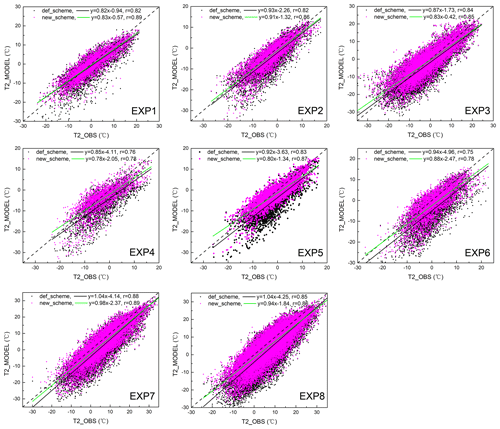
Figure 2Scatterplot of air temperature comparing the CMA observations and model estimates for the eight experiments in the inner (high-resolution) model domain from WRF, using the default Noah snow albedo scheme (def_scheme, black solid circle) and using the improved snow albedo scheme (new_scheme, red solid circle). The black solid line is a linear fit to the black solid circles. The green solid line is a linear fit to the red solid circles. The black dotted line is the line y=x. r is the correlation coefficient between the CMA observations and the model estimates. The correlation coefficient (r) is significant at the 0.01 significance level.
2.3 Data for model evaluation and comparison
CMA hourly observations of air temperature and snow depth from 502 stations were used to assess the WRF model estimates of 2 m air temperature and snow depth that were made using the improved snow albedo parameterization scheme. Albedo is a key factor in net radiation calculations and is defined as the ratio of reflected shortwave radiation (upwards) to received shortwave radiation (downwards). It determines the distribution of turbulent land surface heat fluxes between sensible (SH) and latent (LH) heat. There are many meteorological observations available that have been continuously recorded on the Tibetan Plateau at atmospheric boundary layer towers, eddy covariance systems (Ma et al., 2018, 2020) and the Qilian Mountains integrated observatory network (Li et al., 2018; Liu et al., 2018; Li, 2019; Liu et al., 2020; Zhao and Zhang, 2020). These provide in situ data that are assumed to constitute “truth” for the model validation in this study. In situ observations of albedo, SH and LH from 11 Chinese Academy of Sciences (CAS) stations/samples and from 16 stations in the Qilian Mountains integrated observatory network are used to evaluate the accuracy of the improved snow albedo parameterization scheme for modeling snow events on the Tibetan Plateau. It is reasonable to compare observations of albedo, SH and LH with model estimates at 5 km resolution because there are only a few in situ observation stations in d02 but a total of 27 observation stations in d01. At local solar noon in Lhasa (14:00 BST), the observed albedo value is closer to the Lambertian albedo that is described by the WRF model when coupled with LSMs. We therefore used albedo observations made at 14:00 BST to evaluate the model-calculated albedo. Quality control codes 1, 2 and 3 were selected when using the Turbulence Knight version 3 (TK3) software, and 0 was used when using the Eddypro software to calculate SH and LH. Details of the 27 stations from the CAS and the Qilian Mountains integrated observatory network that were used in our study are provided in Table 2, and their locations on the Tibetan Plateau are shown in Fig. 1. In order to compare WRF simulations against in situ observations, sampling the gridded model estimates and interpolating to given ground stations' locations were done by bi-linear interpolation of the four surrounding model grid points. The RMSE and the correlation coefficient were calculated for the assessment of the model performance in relation to albedo, air temperature, SH, LH, and snow depth estimates.
Table 3RMSE and correlation coefficient (r) for air temperature between CMA observations and model estimates, calculated using the default Noah snow albedo scheme (def_scheme) and the improved albedo scheme (new_scheme). The difference in RMSE is new_scheme RMSE minus def_scheme RMSE. The difference in r is new_scheme r minus def_scheme r. P value < 0.05 is the significance test for the correlation.
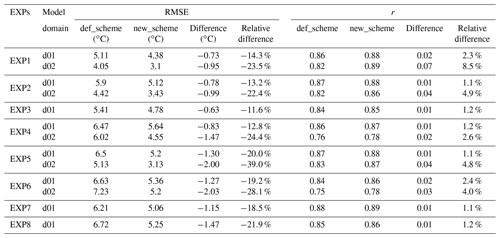
3.1 Air temperature
Albedo is a key factor in determining the net radiation received at the surface, which determines the land surface energy balance and influences air temperature. Scatterplots of the air temperatures estimated by the WRF model and observed at the CMA stations are shown in Fig. 2. In all eight modeling experiments, implementing the improved snow albedo scheme in the WRF model greatly reduces the cold bias that occurs when the default Noah snow albedo scheme is used. Where the default Noah scheme results in a warm bias at the observed lower air temperature for EXP1 and EXP3, however, the improved albedo scheme does not improve the accuracy of the WRF estimates. Compared with cold bias caused by the default Noah albedo scheme at the observed lower air temperature, the improved snow albedo scheme results in a warm bias for EXP2, EXP4 and EXP5. On the whole, scatterplots comparing air temperature observations from the CMA station with WRF estimates made using the improved snow albedo scheme estimates show the data to be concentrated near the ideal fitting line, where the model has exactly reproduced the observations. Using the improved snow albedo scheme results in a marked reduction in the cold bias for the WRF model estimates for EXP1, EXP2, EXP4, EXP5 and EXP6, and the greatest reduction in the cold bias, for all eight experiments, occurs for EXP6 (Fig. 2).
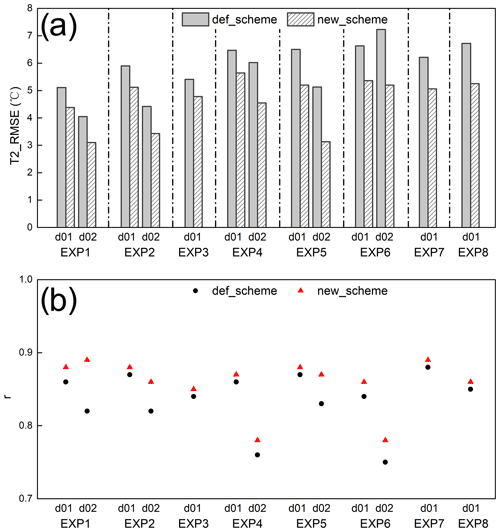
Figure 3RMSE (a) and correlation coefficient (b) for air temperature (T2) between observations and model estimates in d01 and d02. The correlation coefficient is significant at the 0.01 significance level. For def_scheme and new_scheme, see Fig. 2.
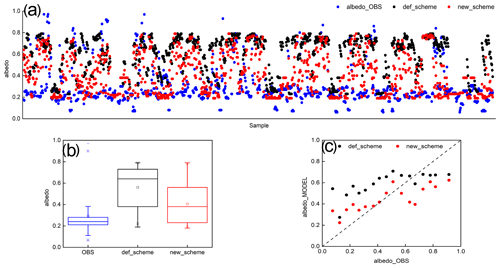
Figure 4Scatterplot of albedo from observations and model estimates for the eight experiments (a: horizontal axis denotes samples from EXP1 to EXP8), corresponding box-and-whisker plot (b) with lower and upper boundaries of the box indicating the first and third quartiles of albedo, respectively, and the line inside the box indicating the median of albedo and averaged albedo observations every 0.05 segments (i.e., 0–0.049, 0.05–0.099, 0.1–0.149, 0.15–0.199, …, 0.85–0.899, 0.9–0.949, 0.95–0.999) and model estimates at the same time as observations for all experiments (c). For def_scheme and new_scheme, see Fig. 2.
To quantify the improvement to air temperature estimates that follows from implementation of the improved snow albedo scheme, the RMSE and correlation coefficient were calculated between CMA observations and model estimates of air temperature shown in Fig. 3. The differences between the accuracy of the new scheme and the default scheme are shown in Table 3. The accuracy of WRF air temperature estimates varies between the different snowfall events, varies between the different snow albedo schemes, and also varies with model resolution. The lowest air temperature RMSE and the highest correlation coefficient are 3.1 ∘C and 0.89, respectively, and both occur for EXP1. The highest air temperature RMSE and the lowest correlation coefficient occur for EXP6, reaching 7.2 ∘C and 0.75, respectively. Air temperature RMSE generally ranges from 4.1 to 7.2 ∘C for the WRF estimates that were made using the default Noah snow albedo scheme estimates, with correlation coefficients ranging from 0.75 to 0.88. In contrast, when the improved snow albedo scheme is implemented in WRF, the RMSE ranges from 3.1 to 5.6 ∘C, and the correlation coefficients range from 0.78 to 0.89. Compared with when the default Noah snow albedo scheme is used, the maximum decrease in air temperature RMSE when the new scheme is used reaches 2.03 ∘C, which represents an improvement of 28.1 %, and the average decrease in air temperature RMSE is 1.2 ∘C, representing an improvement of 20.7 %. There is an improvement of more than 11 % in the RMSE for all eight experiments, with the maximum improvement of 39 % when the new albedo scheme is used relative to when the default scheme is used. Implementing the improved snow albedo scheme in WRF for all eight experiments also increased the correlation coefficient between observed and modeled air temperature by 0.01–0.07, which represents an improvement of 1 %–9 % (Fig. 3, Table 3).
Compared with using the default Noah snow albedo scheme, using the improved scheme results in improved model estimates for all eight EXPs, decreasing the air temperature RMSE and increasing the correlation coefficient when compared with observations. These improvements occur for air temperature estimates calculated at both 5 and 1 km resolution. The improvement to WRF model estimates is greater for calculations made at 1 km resolution than at 5 km resolution, and air temperature estimates are more accurate at 1 km resolution than at 5 km resolution by implementing the improved albedo scheme (Fig. 3, Table 3). Therefore, fine resolution (i.e., 1 km) is strongly recommended for future snowfall event modeling studies.
3.2 Albedo
Using the improved albedo scheme in the WRF model greatly reduces the cold air temperature bias that otherwise occurs, indicating an improvement to model performance. It is necessary to compare albedo estimates with in situ observations. There are very few observation stations located in the finer model domain, and so a total of 26 stations in d01 was used to evaluate the performance of the WRF simulations of albedo at 5 km resolution (Table 2).
Scatterplots comparing observations and WRF estimates for albedo in the eight experiments, when both the default and improved snow albedo schemes were used, are shown alongside our statistical analysis in Fig. 4. Albedo higher than 0.7 is interpreted as snowfall. Albedo in the range of 0.4 to 0.6 is interpreted as snowmelt. Albedo lower than 0.3 indicates sparse or patchy snow cover at the in situ stations. For all eight snowfall events, the observed albedo is concentrated at low values, with a median of 0.24, while WRF-estimated albedo using the default Noah snow albedo scheme has higher values, with a median of 0.64. Compared with albedo estimates calculated using the default scheme, WRF estimates made using the improved scheme result in a prolonged snowmelt period, which increases the number of snowmelt samples and leads to a median albedo of 0.38, which is closer to that for the in situ observations. The mean albedo estimated from WRF using the improved scheme is 0.4, which is also closer to the observed mean of 0.3 than the mean of 0.6 calculated from WRF using the default scheme (Fig. 4a and b). In general, the accuracy of the WRF estimates when the new scheme is used is closely related to the observed albedo. Compared with the WRF estimates made using the default Noah scheme, the WRF estimates made using the improved scheme greatly reduce the overestimation of albedo when the observed values are below 0.6 but seem to increase the underestimation of albedo when the observed values are higher than 0.6 (Fig. 4c).
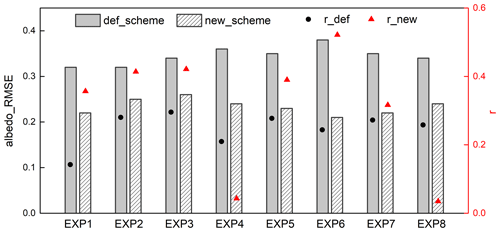
Figure 5RMSE in column and correlation coefficient (r) in the scatterplot for albedo, comparing observations and model estimates. r_def and r_new mean r between the observations and WRF estimates using the default albedo scheme and using the improved albedo scheme, respectively. Except for EXP4 and EXP8, the correlation coefficient is significant at the 0.01 significance level. For def_scheme and new_scheme, see Fig. 2.
To further evaluate the accuracy of WRF albedo estimates when the different snow albedo schemes are used, we calculated the RMSE and correlation coefficients between the observations and the model estimates (Fig. 5). The RMSE for the WRF estimates, when compared to the observations, ranges from 0.32 to 0.38 for the eight experiments when the default scheme is used and ranges from 0.21 to 0.26 when the improved scheme is used. Compared with the default Noah snow albedo scheme, the improved scheme results in a 0.1 decrease in the albedo RMSE in EXP1, representing a relative decrease of 31.3 %, a 0.07 decrease in EXP2, representing a relative decrease of 21.9 %, a 0.08 decrease in EXP3, representing a relative 23.5 % decrease, a 0.12 decrease in EXP4 and EXP5, representing relative decreases of 33.3 % and 34.3 %, respectively, a 0.17 decrease in EXP6, representing a relative 44.7 % decrease, a 0.13 decrease in EXP7, representing a relative 37.1 % decrease, and a 0.1 decrease in EXP8, representing a relative 29.4 % decrease. With the exceptions of EXP4 and EXP8, correlations between the modeled and observed albedo are significant at the 0.01 significance level. Implementing the improved albedo scheme in WRF increases the albedo correlation coefficient by 0.21 in EXP1, a relative increase of 151 %, by 0.13 in EXP2, a relative increase of 47.6 %, by 0.13 in EXP3, a relative increase of 42.5 %, by 0.11 in EXP5, a relative increase of 40.7 %, by 0.28 in EXP6, a relative increase of 114 %, and by 0.04 in EXP7, a relative increase of 16.2 % (Fig. 5). In general, during snowfall and the snowmelt period that follows it, implementing WRF using the improved snow albedo scheme outperforms implementing WRF using the default Noah scheme and results in more accurate albedo estimates, demonstrated by considerable decreases in RMSE and increases in the correlation coefficients.
3.3 Snow depth
There is a feedback between albedo and snow. Snow accumulation and snowmelt influence the proportion of solar irradiance that is reflected; albedo indirectly and non-negligibly influences snow accumulation and snowmelt by affecting the land surface energy budget. In this study, we use an improved snow albedo scheme in which albedo is parameterized as a function of snow depth and age. WRF model estimates of albedo calculated using the improved snow albedo scheme outperform those calculated using the default Noah scheme when snowfall events are simulated, and this leads to improved representation of snowfall and the subsequent snowmelt processes in WRF when the improved scheme is used. Instantaneous direct measurements of snow depth are recorded during snowfall events and over the subsequent snowmelt period. We use these to quantity the improvement that using the new albedo scheme makes to snow estimates calculated in WRF. We assess this by calculating the RMSE and correlation coefficient between the model snow depth estimates and CMA observations, as shown in Fig. 6.
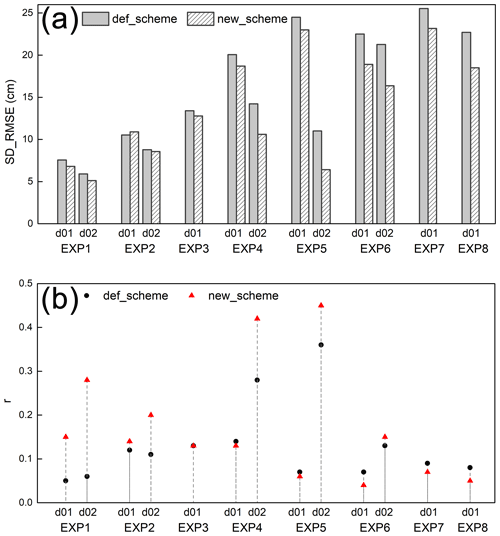
Figure 6Same as Fig. 3 but for RMSE (a) and correlation coefficient (b) for snow depth (SD). The correlation coefficient is significant at the 0.01 significance level, except for d01 estimates in EXP6.
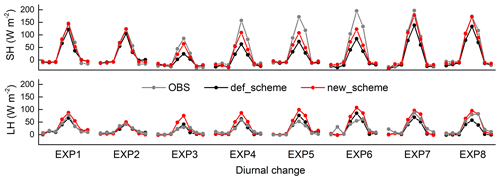
Figure 7Diurnal change in sensible (SH) and latent (LH) heat fluxes from ground observations and model estimates. Beginning at 02:00 BST with a 3 h interval. For def_scheme and new_scheme, see Fig. 2.
Comparing the accuracy of WRF snow depth estimates calculated using the new scheme to the accuracy achieved using the default Noah scheme, the greatest relative decrease in RMSE is 41.7 %, which occurs for estimates made at finer resolution in EXP5. Replacing the default albedo scheme with the new scheme in WRF results in an average decrease in snow depth RMSE of 2.2 cm, which is a 13.4 % improvement. In areas covered by the higher-resolution model domain, the average RMSE decrease is 2.8 cm, which is a 21.2 % improvement. This shows that the impact of replacing the albedo scheme with an improved scheme is more significant for areas in the higher-resolution d02 model domain than for areas in the coarser d01 model domain (Fig. 6a). Using the improved albedo scheme in WRF increases the correlation coefficient between observed and modeled snow depth in areas within the d02 model domain, but this increase is not consistent for areas in the d01 domain. The greatest increase, both relative and absolute, in the snow depth correlation coefficient occurs in the finer simulation domain in EXP1, where the correlation between observed and modeled snow depth increases by 0.22, which is a 366.7 % increase. The mean and relative increases in the correlation coefficient between observed and modeled snow depth for areas in the d02 simulations are 0.14 and 107.8 %, respectively (Fig. 6b). WRF snow depth estimates are more accurate at finer resolution (i.e., 1 km resolution) than in coarser simulations (i.e., 5 km resolution), regardless of which albedo scheme is implemented (Fig. 6). Implementing the improved albedo scheme in WRF improves the agreement between model estimated and observed snow depth relative to implementing the default Noah albedo scheme, as seen by the decreased RMSE and the increased correlation coefficient.
3.4 Turbulent heat and vapor fluxes
Albedo plays a significant role in the land surface energy balance. It determines the proportioning of net radiation fluxes between turbulent heat and vapor fluxes. Our study shows that using the improved snow albedo scheme in WRF results in a good model representation of surface albedo in simulations of snow events. We now consider whether replacing the default scheme with the improved scheme may affect the proportioning of net radiation fluxes between turbulent heat and vapor fluxes. Since there are very few observation in situ stations located in the area covered by the finer model domain, a total of 14 in situ stations located in the area covered by d01 were selected, and WRF estimates of turbulent heat and vapor fluxes were assessed from simulations calculated at 5 km resolution (Table 2).
The diurnal changes in SH and LH recorded in the in situ observations and calculated in the eight modeling experiments are shown in Fig. 7. The WRF model successfully captures the diurnal changes in SH and LH, particularly in EXP1 and EXP2, where the model estimates of SH and LH are almost equal to the in situ observations. In the nighttime, the WRF model accurately estimates SH and LH in all eight experiments. However, during the day WRF consistently underestimates SH in all experiments except EXP1 and EXP2 and estimates LH with varying accuracy when the default Noah albedo scheme is used. For example, when the default Noah scheme is used, WRF accurately estimates LH in EXP3 and EXP4 but overestimates LH in EXP5 and EXP6 and underestimates LH in EXP7 and EXP8. Compared with WRF estimates calculated using the default scheme, WRF simulations calculated using the new albedo scheme result in increased estimates of the turbulent heat and vapor fluxes. This leads to SH estimates that are closer to observations for experiments EXP3 to EXP8, a greatly overestimated LH for experiments EXP3 to EXP6, and a slightly overestimated LH, which is closer to observations for EXP7 and EXP8 (Fig. 7). In general, WRF estimates of SH are improved through the implementation of the new albedo scheme relative to the default scheme, although SH is underestimated during snowfall events. The impact of the improved albedo scheme on LH estimates varies between the different snowfall events, but LH is consistently overestimated.
The RMSE and correlation coefficient for comparisons between WRF estimates and observations for SH and LH are shown in Fig. 8. Compared with when the default Noah scheme is used, WRF estimates using the improved scheme result in reduced RMSE for SH estimates in all experiments except EXP7. The absolute (and relative) reductions are 10.9 W m−2 (16.2 %) in EXP1, 3.9 W m−2 (7.6 %) in EXP2, 11.9 W m−2 (23.8 %) in EXP3, 15.7 W m−2 (21 %) in EXP4, 17.9 W m−2 (17.4 %) in EXP5, 16.6 W m−2 (15.8 %) in EXP6, and 10.0 W m−2 (10.3 %) in EXP8. There is a relative increase of 6.5 % in EXP7. Implementing the improved scheme in WRF significantly increases the correlation coefficients between observed and modeled SH, relative to using the default scheme, in all experiments except EXP7, where there is a slight decrease. The largest increase in the SH correlation coefficient, both absolute and relative, is 0.2 and 51.3 %, respectively, and occurs for EXP5. Implementing the improved scheme in WRF reduces the SH RMSE by an average of 10 W m−2, which is an improvement of 13.2 %, and increases the SH correlation coefficient by an average of 0.1, which is an improvement of 16.8 % (Fig. 8a). However, replacing the default scheme with the improved albedo scheme results in less accurate estimates of LH and corresponds to an increase in RMSE in almost all eight experiments, although the correlation coefficient increases for half of the snowfall event simulations (EXP3, EXP4, EXP6 and EXP8) (Fig. 8b).
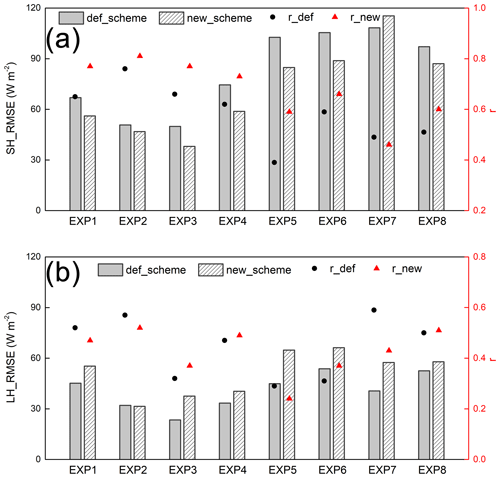
Figure 8Same as Fig. 5 but for sensible (a) and latent (b) heat fluxes. The correlation coefficient is significant at the 0.001 significance level.
In summary, the improved snow albedo scheme has a significant effect on the proportioning of radiative fluxes between turbulent heat and vapor fluxes. It significantly outperforms the default Noah scheme in relation to SH estimates, but there is no significant improvement in LH estimates, and these may be less accurate when the new scheme is used relative to the default scheme during snowfall and the subsequent snowmelt period.
The highly complex topography of the Tibetan Plateau means that WRF estimates of air temperature, albedo and snow depth are strongly sensitive to model resolution. Our study shows that WRF performs much more accurately when run at a finer resolution (1 km) than at a relatively coarse resolution (5 km) for snowfall events over the Tibetan Plateau, regardless of which snow albedo parameterization scheme is used. This difference may be explained by the ability of the model to resolve complex terrain (Rahimi et al., 2019) and/or by the implementation of the cumulus convective parameterization scheme. The more detailed representation of complex terrain and the explicit representation of convection mean that running WRF at finer resolution greatly improves model estimates of air temperature, surface pressure, and relative humidity (Singh et al., 2021) and provides small improvements in the magnitude of daytime convective precipitation (Collier and Immerzeel, 2015). Norris et al. (2017) pointed out that decreasing the grid spacing from 6.7 to 2.2 km likely improves estimates of mountain precipitation but does not fundamentally change the representation of the diurnal cycle. They indicated that the key difference between low and high model resolution is whether or not a cumulus convective scheme is required. Subkilometer grid resolution has been investigated in WRF and used for modeling meteorological variables over complex terrain (Horvath et al., 2012; Dimitrova et al., 2016). The 500 m resolution configuration of WRF results in the closest match between the model estimates and observations and gives the most plausible spatial distribution of precipitation over the complex topography. The performance of the WRF model has been similarly demonstrated to improve at 500 m, relative to coarser resolutions, for wind and air temperature estimates (Bonekamp et al., 2018). We therefore strongly suggest that subkilometer grid resolution should be considered when WRF is configured for simulations covering areas in High-Mountain Asia in future research.
Our improved snow albedo scheme parameterizes albedo as a function of snow depth and age by considering the relationship between MODIS albedo and the modeled snow depth and age. It is more physically plausible than the default Noah scheme, which considers snow cover, and outperforms the default Noah scheme for air temperature, snow depth, albedo and turbulent heat and vapor flux estimates during snowfall events. However, even when the improved albedo scheme is used, the RMSE for WRF estimates of albedo at 5 km spatial resolution remains around 0.21–0.26, although this represents a decrease of 22 %–45 % relative to when the default albedo scheme is used. It should be noted that the accuracy of the MODIS albedo retrieval algorithm is limited during snowfall events and snowmelt periods (Qin et al., 2011; An et al., 2020) and also that rugged mountain terrain not only affects the radiation absorbed by the land surface, but also affects the radiation reflected by the land surface to the satellite-borne sensor. Multiple reflection and scattering from adjacent mountains create challenges for the monitoring and retrieval of surface albedo in areas of complex terrain via remote sensing (Zhang and Gao, 2011; Roupioz et al., 2014, 2016). This reduces the accuracy of MODIS albedo retrieval over the complex topographic Tibetan Plateau and constitutes a limitation of the improved albedo parameterization investigated here, since it relies on MODIS albedo products. A terrain correction is required for the MODIS albedo retrieval to further improve the albedo parameterization scheme used here. However, it is difficult to establish a unified terrain correction model due to the large undulations of the Tibetan Plateau. How to effectively eliminate the influence of terrain factors from a specific mountain surface on quantitative retrievals from remote sensing data has long been a challenge and a focus for remote sensing research. A further challenge for the assessment presented here is the sparse and uneven distribution of available in situ albedo observation data over the bulk Tibetan Plateau. This paucity of data means that there is a mismatch in spatial resolution when comparing albedo estimates calculated at 5 km resolution with in situ observations.
Air temperature is a critical factor that is related to albedo and determines energy distribution between SH and LH. Using the improved snow albedo parameterization scheme significantly reduces the albedo overestimates during snowfall events that occur when the default scheme is used, and this leads to the reduction of the cold air temperature bias in the model. Therefore, in this study, the improved scheme reduces the underestimates for SH and improves the performance of WRF in simulating SH over the Tibetan Plateau relative to when the default scheme is used. These results indicate that the accurate simulation of surface albedo is very important for the accurate simulation of SH.
Implementing the improved albedo scheme results in little improvement to estimates of LH calculated, which is restricted by water content and increased only slightly relative to when the default albedo scheme is used. This may be explained by the LH parameterization scheme used in the Noah LSM (Chen and Dudhis, 2001). The total LH in the Noah LSM has three components (LH from the direct evaporation from the top surface layer, evaporation of precipitation intercepted by the canopy and transpiration via the canopy and roots, respectively). The factors affecting calculation of LH in the Noah LSM include not only the radiation balance (which is impacted by albedo), but also soil water, soil capillary conductivity and vegetation status, i.e., albedo, surface heat and water vapor exchange coefficient, saturated water vapor pressure, specific humidity, surface soil water content, field capacity, wilting point, canopy resistance, total precipitation, and canopy interception amount. In our current study, we have focused on the snow albedo parameterization scheme in the Noah LSM by considering the MODIS albedo product and the additional snow-related variable of snow depth. Therefore, the influence of our improved scheme on LH estimates calculated by the LSM is very limited.
Implementing the improved snow albedo scheme in place of the default scheme greatly decreases the overestimation of albedo from snowmelt to snow-free processes but does not remove the underestimation of albedo during snowfall. This means that the improvements mainly come from snowmelt and snow-free simulations, and model performance during snowfall may be worse when the improved albedo scheme is used. This suggests an opportunity to further investigate how albedo is characterized by snow depth and age in the snow albedo parameterization scheme.
We conducted several numerical experiments to evaluate the performance of the MODIS albedo-based snow albedo parameterization scheme (Liu, 2020) implemented in WRF. We assessed the RMSE and correlation coefficient between observed and modeled air temperature, albedo, snow depth and turbulent heat and vapor fluxes for simulations of eight snowfall events over the Tibetan Plateau. We compared the accuracy of WRF estimates made using the improved snow albedo scheme with that of WRF estimates made using the default Noah scheme, in both cases compared with ground observations.
The accuracy of WRF estimates of albedo is significantly improved when the new albedo scheme is implemented. The default Noah scheme tends towards higher albedo estimates and cannot accurately capture snowfall and snowmelt processes, resulting in a high RMSE and low correlation coefficient between modeled and observed albedo. Through consideration of snow-related variables, such as snow depth and age, and by being based on MODIS remote sensing albedo products, the improved scheme estimates albedo more accurately than the default scheme, improving the albedo RMSE by around 22 % to 45 %, with an average improvement of 32 %. Similarly, the improved scheme results in an increased correlation coefficient between modeled and observed albedo. Relative to the default scheme, the correlation coefficient relatively increases by around 16 % to 151 %, with an average improvement of 69 %. This may contribute to the relatively better performance of WRF in simulating air temperatures when the improved albedo scheme is used. The improved scheme relatively decreases (increases) the air temperature RMSE (correlation coefficient) by 16 % (1.5 %) for model estimates calculated at 5 km resolution and by 27 % (5 %) for model estimates calculated at 1 km resolution.
There are mutual feedbacks between snow and albedo. During snowfall and over the subsequent snowmelt period, snow depth and age affect changes in the albedo. The changes in albedo in turn affect the evolution of snow events by changing the surface energy budget and the proportional distribution of net radiation between turbulent heat and vapor fluxes and finally by changing the type of precipitation. Our study shows that when the default albedo scheme is replaced with the improved albedo scheme in WRF, the turbulent heat and vapor flux estimates increase. The improved scheme significantly outperforms the default Noah scheme for SH estimates, with a decrease (increase) in the RMSE (correlation coefficient) of 10 W m−2 (0.1), representing an improvement of 13 % (17 %). The overall accuracy with which WRF estimates turbulent heat and vapor fluxes improves when the improved albedo scheme replaces the default scheme, although there is no significant improvement in LH estimates. This overall improvement leads to a more accurate reproduction of the evolution of snowfall events and to more accurate snow depth estimates. Our study shows that using the improved albedo scheme in WRF reduces the RMSE and increases the correlation coefficient between modeled and observed snow depth, relative to using the default scheme. This improvement is more significant for simulations at 1 km resolution than for simulations at 5 km resolution, with maximum and averaged relative RMSE (correlation coefficient) decreases (increases) of 42 % (367 %) and 21 % (108 %), respectively, for 1 km resolution simulations.
The authors express thanks to the ECMWF for sharing the atmospheric reanalysis data set (the ERA5 data set is available from https://www.ecmwf.int/en/forecasts/datasets/reanalysis-datasets/era5), to NASA for offering MODIS reflectance products (https://doi.org/10.5067/MODIS/MOD09GA.006, Vermote and Wolfe, 2015), and to staff from CMA, CAS and the Qilian Mountains integrated observatory network for very hard work in meteorological observations and offering the data (CMA meteorological data are available from http://data.cma.cn/en; last access: 3 September 2021). Albedo and turbulent heat and vapor flux observations from CAS and the Qilian Mountains integrated observatory network are provided by the National Tibetan Plateau Data Center (http://data.tpdc.ac.cn, last access: 3 September 2021, National Tibetan Plateau Data Center).
LL, YM and MM were responsible for the conceptualization. RS, NY, and WM were responsible for data processing and drawing a part of the figures. LL prepared modeling analysis and the manuscript with contributions from all the co-authors.
The contact author has declared that neither they nor their co-authors have any competing interests.
Publisher's note: Copernicus Publications remains neutral with regard to jurisdictional claims in published maps and institutional affiliations.
The first author would like to thank all the group members for their help in completing this paper and thanks two reviewers for providing many constructive comments.
This research has been supported by the Strategic Priority Research Program of the Chinese Academy of Sciences (grant no. XDA20060101), the Second Tibetan Plateau Scientific Expedition and Research program (STEP) (grant no. 2019QZKK0103), the National Natural Science Foundation of China (grant nos. 91837208, 91637312, 41830650, 91737205), MOST High Level Talent (grant no. G20190161018), the Chinese Academy of Sciences President's International Fellowship Initiative (grant no. 2020VTA0001), the Key Research Program of Frontier Sciences of the Chinese Academy of Sciences (grant no. QYZDJ-SSW-DQC019) and Key Research and Development Projects of the Ministry of Science and Technology (grant no. 2018YFC1505701).
This paper was edited by Xing Yuan and reviewed by Enda Zhu and one anonymous referee.
An, Y., Meng, X., Zhao, L., Li, Z., Wang, S., Shang, L., Chen, H., Lyu, S., Li, G., and Ma, Y.: Performance of GLASS and MODIS satellite albedo products in diagnosing albedo variations during different time scales and special weather conditions in the Tibetan Plateau, Remote Sens., 12, 2456, https://doi.org/10.3390/rs12152456, 2020.
Aoki, T., Hachikubo, A., and Hori, M.: Effects of snow physical parameters on shortwave broadband albedos, J. Geophys. Res., 108, 4616, https://doi.org/10.1029/2003JD003506, 2003.
Bao, Y. and Lü, S.: Improvement of surface albedo parameterization within a regional climate model (RegCM3), Hydrol. Earth Syst. Sci. Discuss., 6, 1651–1676, https://doi.org/10.5194/hessd-6-1651-2009, 2009.
Bao, Y., Lyu, S., Zhang, Y., Meng, X., and Yang, S.: Improvement of surface albedo simulations over arid regions, Adv. Atmos. Sci., 25, 481–488, 2008.
Bloch, M. R.: Dust-induced albedo changes of polar ice sheets and glacierization, J. Glaciol., 5, 241–244, 1964.
Bonekamp, P. N. J., Collier, E., and Immerzeel, W. W.: The impact of spatial resolution, land use, and spinup time on resolving spatial precipitation patterns in the Himalayas, J. Hydrometeorol., 19, 1565–1581, 2018.
Chen, F. and Dudhis, J.: Coupling an advanced land surface-hydrology model with the Penn State-NCAR MM5 modeling system. Part I: Model implementation and sensitivity, Mon. Weather Rev., 129, 569–585, 2001.
Collier, E. and Immerzeel, W. W.: High-resolution modeling of atmospheric dynamics in the Nepalese Himalaya, J. Geophys. Res., 120, 9882–9896, 2015.
Dang, C., Brandt, R. E., and Warren, S. G.: Parameterizations for narrowband and broadband albedo of pure snow and snow containing mineral dust and black carbon, J. Geophys. Res., 120, 5446–5468, 2015.
Dimitrova, R., Silver, Z., Zsedrovits, T., Hocut, C. M., Leo, L. S., Di Sabatino, S., and Fernando, H. J. S.: Assessment of planetary boundary-layer schemes in the Weather Research and Forecasting mesoscale model using Materhorn field data, Bound.-Lay. Meteorol., 159, 589–609, 2016.
Ek, M. B., Mitchell, K. E., Lin, Y., Rogers, E., Grunmann, P., Koren, V., Gayno, G., and Tarpley, J. D.: Implementation of Noah land surface model advances in the National Centers for Environmental Prediction operational mesoscale Eta model, J. Geophys. Res., 108, 8851, https://doi.org/10.1029/2002JD003296, 2003.
Gao, Y., Xu, J., and Chen, D.: Evaluation of WRF mesoscale climate simulations over the Tibetan Plateau during 1979–2011, J. Climate, 28, 2823–2841, 2015.
Gardner, A. S. and Sharp, M. J.: A review of snow and ice albedo and the development of a new physically based broadband albedo parameterization, J. Geophys. Res., 115, F01009, https://doi.org/10.1029/2009JF001444, 2010.
Hansen, J. and Nazarenko, L.: Soot climate forcing via snow and ice albedos, P. Natl. Acad. Sci. USA, 101, 423–428, 2004.
He, C., Takano, Y., Liou, K.-N., Yang, P., Li, Q., and Chen, F.: Impact of snow grain shape and black carbon-snow internal mixing on snow optical properties: Parameterizations for climate models, J. Climate, 30, 10019–10036, 2017.
He, C., Liou, K.-N., and Takano, Y.: Resolving size distribution of black carbon internally mixed with snow: Impact on snow optical properties and albedo, Geophys. Res. Lett., 45, 2697–2705, 2018a.
He, C., Liou, K.-N., Takano, Y., Yang, P., Qi, L., and Chen, F.: Impact of grain shape and multiple black carbon internal mixing on snow albedo: Parameterization and radiative effect analysis, J. Geophys. Res., 123, 1253–1268, 2018b.
Horvath, K., Koracin, D., Vellore, R., Jiang, J., and Belu, R.: Sub-kilometer dynamical downscaling of near-surface winds in complex terrain using WRF and MM5 mesoscale models, J. Geophys. Res., 117, D11111, https://doi.org/10.1029/2012JD017432, 2012.
Jonsell, U., Hock, R., and Holmgren, B.: Spatial and temporal variations in albedo on Storglaciaren, Sweden, J. Glaciol., 49, 59–68, 2003.
Kuipers Munneke, P., van den Broeke, M. R., Lenaerts, J. T. M., Flanner, M. G., Gardner, A. S., and van de Berg, W. J.: A new albedo parameterization for use in climate models over the Antarctic ice sheet, J. Geophys. Res., 116, D05114, https://doi.org/10.1029/2010JD015113, 2011.
Li, X.: Qilian Mountains integrated observatory network: Dataset of Qinghai Lake integrated observatory network (eddy covariance system of Alpine meadow and grassland ecosystem Superstation, 2018), National Tibetan Plateau Data Center, https://doi.org/10.11888/Meteoro.tpdc.270802, 2019.
Li, X., Yang, X., Ma, Y., Hu, G., Hu, X., Wu, X., Wang, P., Huang, Y., Cui, B., and Wei, J.: Qinghai Lake Basin Critical Zone Observatory on the Qinghai-Tibet Plateau, Vadose Zone J., 17, 180069, https://doi.org/10.2136/vzj2018.04.0069, 2018.
Li, Y. and Hu, Z.: A study on parameterization of surface albedo over grassland surface in the northern Tibetan Plateau, Adv. Atmos. Sci., 26, 161–168, 2009.
Liang, S.: Narrowband to Broadband conversions of land surface albedo: I. Algorithms, Remote Sens. Environ., 76, 213–238, 2000.
Liang, X., Xu, M., Gao, W., Kunkel, K., Slusser, J., Dai, Y., Min, Q., Houser, P. R., Rodell, M., Schaaf, C. B., and Gao, F.: Development of land surface albedo parameterization based on Moderate Resolution Imaging Spectroradiometer (MODIS) data, J. Geophys. Res., 110, D11107, https://doi.org/10.1029/2004JD005579, 2005.
Lin, P., Wei, J., Yang, Z., Zhang, Y., and Zhang, K.: Snow data assimilation-constrained land initialization improves seasonal temperature prediction, Geophys. Res. Lett., 43, 11423—11432, 2016.
Liu, L.: Diagnostic analysis and numerical simulation of a regional heavy snowfall over the Tibetan Plateau, chap. 5, Doctoral dissertation, Institute of Tibetan Plateau Research, Chinese Academy of Sciences, Beijing, China, 2020.
Liu, L., Ma, Y., Menenti, M., Zhang, X., and Ma, W.: Evaluation of WRF modeling in relation to different land surface schemes and initial and boundary conditions: A snow event simulation over the Tibetan Plateau, J. Geophys. Res., 124, 209–226, 2019.
Liu, S., Li, X., Xu, Z., Che, T., Xiao, Q., Ma, M., Liu, Q., Jin, R., Guo, J., Wang, L., Wang, W., Qi, Y., Li, H., Xu, T., Ran, Y., Hu, X., Shi, S., Zhu, Z., Tan, J., Zhang, Y., and Ren, Z.: The Heihe Integrated Observatory Network: A Basin-Scale Land Surface Processes Observatory in China, Vadose Zone J., 17, 180072, https://doi.org/10.2136/vzj2018.04.0072, 2018.
Liu, S., Che, T., Xu, Z., Ren, Z., Tan, J., and Zhang, Y.: Qilian Mountains integrated observatory network: Dataset of Heihe integrated observatory network (Large aperture scintillometer of Daman Superstation, 2019), National Tibetan Plateau Data Center, https://doi.org/10.11888/Meteoro.tpdc.270673, 2020.
Livneh, B., Xia, Y., Mitchell, K. E., Ek, M. B., and Lettenmaier, D. P.: Noah LSM snow model diagnostics and enhancements, J. Hydrometeorol., 11, 721–738, 2010.
Ma, Y., Wang, Y., and Han, C.: Regionalization of land surface heat fluxes over the heterogeneous landscape: from the Tibetan Plateau to the Third Pole region, Int. J. Remote Sens., 39, 5872–5890, 2018.
Ma, Y., Hu, Z., Xie, Z., Ma, W., Wang, B., Chen, X., Li, M., Zhong, L., Sun, F., Gu, L., Han, C., Zhang, L., Liu, X., Ding, Z., Sun, G., Wang, S., Wang, Y., and Wang, Z.: A long-term (2005–2016) dataset of hourly integrated land–atmosphere interaction observations on the Tibetan Plateau, Earth Syst. Sci. Data, 12, 2937–2957, https://doi.org/10.5194/essd-12-2937-2020, 2020.
Malik, M. J., van der Velde, R., Vekerdy, Z., and Su, Z.: Improving modeled snow albedo estimates during the spring melt season, J. Geophys. Res., 119, 7311–7331, 2014.
Maussion, F., Scherer, D., Finkelnburg, R., Richters, J., Yang, W., and Yao, T.: WRF simulation of a precipitation event over the Tibetan Plateau, China – an assessment using remote sensing and ground observations, Hydrol. Earth Syst. Sci., 15, 1795–1817, https://doi.org/10.5194/hess-15-1795-2011, 2011.
Meng, C. and Li, H.: Solar radiation partitioning and surface albedo parameterization in the hinterland of Taklimakan Desert, Adv. Meteorol., 2019, 9098576, https://doi.org/10.1155/2019/9098576, 2019.
Meng, X., Lyu, S., Zhang, T., Zhao, L., Li, Z., Han, B., Li, S., Ma, D., Chen, H., Ao, Y., Luo, S., Shen, Y., Guo, J., and Wen, L.: Simulated cold bias being improved by using MODIS time-varying albedo in the Tibetan Plateau in WRF model, Environ. Res. Lett., 13, 44028, https://doi.org/10.1088/1748-9326/aab44a, 2018.
National Tibetan Plateau Data Center: Qilian Mountains integrated observatory network, available at: https://data.tpdc.ac.cn/, last access: 3 September 2021.
Norris, J., Carvalho, L. M. V., Jones, C., Cannon, F., Bookhagen, B., Palazzi, E., and Tahir, A. A.: The spatiotemporal variability of precipitation over the Himalaya: Evaluation of one-year WRF model simulation, Clim. Dynam., 49, 2179–2204, 2017.
Oerlemans, J. and Knap, W. H.: A 1 year record of global radiation and albedo in the ablation zone of Morteratschgletscher, Switzerland, J. Glaciol., 44, 231–238, 1998.
Park, S. and Park, S. K.: Parameterization of the snow-covered surface albedo in the Noah-MP Version 1.0 by implementing vegetation effects, Geosci. Model Dev., 9, 1073–1085, https://doi.org/10.5194/gmd-9-1073-2016, 2016.
Qin, J., Yang, K., Liang, S., Zhang, H., Ma, Y., Guo, X., and Chen, Z.: Evaluation of surface albedo from GEWEX-SRB and ISCCP-FD data against validated MODIS product over the Tibetan Plateau, J. Geophys. Res., 116, D24116, https://doi.org/10.1029/2011JD015823, 2011.
Rahimi, S. R., Wu, C., Liu, X., and Brown, H.: Exploring a variable-resolution approach for simulating regional climate over the Tibetan Plateau using VR-CESM, J. Geophys. Res., 124, 4490–4513, 2019.
Rai, A., Saha, S. K., and Sujith, K.: Implementation of snow albedo schemes of varying complexity and their performances in offline Noah and Noah coupled with NCEP CFSv2, Clim. Dynam., 53, 1261–1276, 2019.
Roupioz, L., Nerry, F., Jia, L., and Menenti, M.: Improved surface reflectance from remote sensing data with sub-pixel topographic information, Remote Sens., 6, 10356–10374, 2014.
Roupioz, L., Jia, L., Nerry, F., and Menenti, M.: Estimation of daily solar radiation budget at kilometer resolution over the Tibetan Plateau by integrating MODIS data products and a DEM, Remote Sens., 8, 504, https://doi.org/10.3390/rs8060504, 2016.
Saito, M., Yang, P., Loeb, N. G., and Kato, S.: A novel parameterization of snow albedo based on a two-layer snow model with a mixture of grain habits, J. Atmos. Sci., 76, 1419–1436, 2019.
Sellers, P. J., Randall, D. A., Collatz, G. J., Berry, J. A., Field, C. B., Dazlich, D. A., Zhang, C., Collelo, G. D., and Bounoua, L.: A revised land surface parameterization (SiB2) for atmospheric GCMs. Part I: Model formulation, J. Climate, 9, 676–705, 1996.
Skamarock, W., Klemp, J. B., Dudhia, J., Gill, D. O., Barker, D. M., Wang, W., and Powers, J. G.: A description of the advanced research WRF version 3, NCAR Technical Note NCAR/TN-475+STR, University Corporation for Atmospheric Research, https://doi.org/10.5065/D68S4MVH, 2008.
Singh, J., Singh, N., Ojha, N., Sharma, A., Pozzer, A., Kiran Kumar, N., Rajeev, K., Gunthe, S. S., and Kotamarthi, V. R.: Effects of spatial resolution on WRF v3.8.1 simulated meteorology over the central Himalaya, Geosci. Model Dev., 14, 1427–1443, https://doi.org/10.5194/gmd-14-1427-2021, 2021.
Vermote, E. and Wolfe, R.: MOD09GA MODIS/Terra Surface Reflectance Daily L2G Global 1 km and 500 m SIN Grid V006, NASA EOSDIS Land Processes DAAC [data set], doi10.5067/MODIS/MOD09GA.006, 2015.
Wang, J., Cui, Y., He, X., Zhang, J., and Yan, S.: Surface albedo variation and its influencing factors over Dongkemadi glacier, central Tibetan Plateau, Adv. Meteorol., 2015, 852098, https://doi.org/10.1155/2015/852098, 2015.
Wang, W., Yang, K., Zhao, L., Zheng, Z., Lu, H., Mamtimin, A., Ding, B., Li, X., Zhao, L., Li, H., Che, T., and Moore, J. C.: Characterizing surface albedo of shallow fresh snow and its importance for snow ablation on the interior of the Tibetan Plateau, J. Hydrometeorol., 21, 815–827, 2020.
Wang, Z., Zeng, X., and Barlage, M.: Moderate Resolution Imaging Spectroradiometer bidirectional reflectance distribution function-based albedo parameterization for weather and climate models, J. Geophys. Res., 112, D02103, https://doi.org/10.1029/2005JD006736, 2007.
Warren, S. G. and Wiscombe, W. J.: A model for the spectral albedo of snow: Snow containing atmospheric aerosols, J. Atmos. Sci., 37, 2734–2745, 1980.
Wen, J., Su, Z., Tian, H., Shi, X., Zhang, Y., Wang, X., Liu, R., Zhang, T., Kang, Y., Lyu, S., and Zhang, J.: Advances in observation and modeling of land surface processes over the source region of the Yellow River, Adv. Earth Sci., 26, 575–585, 2011.
Wiscombe, W. J. and Warren, S. G.: A model for the spectral albedo of snow. I: Pure snow, J. Atmos. Sci., 37, 2712–2733, 1980.
Xu, J. and Shu, H.: Assimilating MODIS-based albedo and snow cover fraction into the common land model to improve snow depth simulation with direct insertion and deterministic ensemble Kalman filter method, J. Geophys. Res., 119, 10684–10701, 2014.
Xue, Y., Houser, P. R., Maggioni, V., Mei, Y. W., Kumar, S. V., and Yoon, Y.: Assimilation of satellite-based snow cover and freeze/thaw observations over high mountain Asia, Front. Earth Sci., 7, 115, https://doi.org/10.3389/feart.2019.00115, 2019.
Yuan, W., Xu, W., Ma, M., Chen, S., Liu, W., and Cui, L.: Improved snow cover model in terrestrial ecosystem models over the Qinghai-Tibetan Plateau, Agr. Forest Meteorol., 218, 161–170, 2016.
Zhang, W. and Gao, Y.: Topographic correction algorithm for remotely sensed data accounting for indirect irradiance, Int. J. Remote Sens., 32, 1807–1824, 2011.
Zhang, Y., Hoar, T. J., Yang, Z., Anderson, J. L., Toure, A. M., and Rodell, M.: Assimilation of MODIS snow cover through the data assimilation research testbed and the community land model version 4, J. Geophys. Res., 119, 7091–7103, 2014.
Zhao, C. and Zhang, R.: Cold and Arid Research Network of Lanzhou university (eddy covariance system of Guazhou station, 2019), National Tibetan Plateau Data Center, https://doi.org/10.11888/Meteoro.tpdc.270709, 2020.
Zhong, E., Li, Q., Sun, S., Chen, W., Chen, S., and Nath, D.: Improvement of a snow albedo parameterization in the snow–atmosphere–soil transfer model: Evaluation of impacts of aerosol on seasonal snow cover, Adv. Atmos. Sci., 34, 1333–1345, 2017.