the Creative Commons Attribution 4.0 License.
the Creative Commons Attribution 4.0 License.
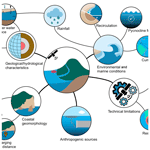
Applicability of Landsat 8 thermal infrared sensor for identifying submarine groundwater discharge springs in the Mediterranean Sea basin
Albert Folch
Jordi Garcia-Orellana
Submarine groundwater discharge (SGD) has received increasing attention over the past 2 decades as a source of nutrients, trace elements and ocean pollutants that may alter coastal biogeochemical cycles. Assessing SGD flows and their impact on coastal marine environments is a difficult task, since it is not easy to identify and measure these water flows discharging into the sea. The aim of this study is to demonstrate the significant usefulness of the freely available thermal infrared (TIR) imagery of the Landsat 8 thermal infrared sensor (TIRS) as an exploratory tool for identifying SGD springs worldwide, from local to regional scales, for long-term analysis. The use of satellite thermal data as a technique for identifying SGD springs in seawater is based on the identification of thermally anomalous plumes obtained from the thermal contrasts between groundwater and sea surface water. In this study, we use the TIR remote sensing (TIR-RS) imagery provided by Landsat 8 at a regional scale and discuss the principle limiting factors of using this technique in SGD studies. The study was developed in karstic coastal aquifers in the Mediterranean Sea basin during different seasons and under diverse meteorological conditions. Although this study demonstrates that freely available satellite TIR remote sensing is a useful method for identifying coastal springs in karst aquifers both locally and regionally, the limiting factors include technical limitations, geological and hydrogeological characteristics, environmental and marine conditions and coastal geomorphology.
- Article
(10352 KB) - Full-text XML
-
Supplement
(2226 KB) - BibTeX
- EndNote
Submarine groundwater discharge (SGD) is an important component of the hydrological cycle and has been commonly defined as any flow of water across the continental margin in the ocean–aquifer interface, regardless of fluid composition or driving force, with spatial scale lengths of meters to kilometers (Burnett and Dulaiova, 2003; Moore, 2010; Taniguchi et al., 2019). This definition includes meteoric fresh groundwater resulting from inland recharge, but also seawater circulated through the sediments of coastal aquifers (Burnett and Dulaiova, 2003). Both water flows mix in coastal aquifers, where biogeochemical reactions may occur when this groundwater interacts with the geological matrix (Moore, 1999; Moosdorf et al., 2021; Rocha et al., 2021; Ruiz-González et al., 2021). This dynamic mixing zone influences the transfer of chemical compounds such as nutrients, trace metals and other contaminants to coastal waters (Alorda-Kleinglass et al., 2019; Boehm et al., 2004; Rodellas et al., 2015; Trezzi et al., 2016). SGD-derived inputs from chemical compounds can highly impact coastal ecosystems by influencing productivity, biomass, species composition and sonification (Andrisoa et al., 2019; Garcés et al., 2011; Garcia-Orellana et al., 2016; Krest et al., 2000). According to Garcia-Orellana et al. (2021), groundwater discharge pathways of SGD can be grouped into the following five different SGD pathways according to the characteristics of the processes: (1) terrestrial groundwater discharge, (2) density-driven seawater circulation, (3) seasonal exchange of seawater, (4) shoreface circulation of seawater and (5) centimeter-scale porewater exchange (PEX). The discharge of fresh groundwater (pathway 1) and, to a lesser extent, density-driven seawater circulation (pathway 2) is the only mechanism that represents a net source of freshwater, usually mixed with seawater, to the coastal ocean. The SGD discharge process related to this fresher fraction of SGD occurs mainly through three different ways, namely coastal onshore springs, which discharge on the coastline via surface sinkholes (Garcia-Solsona et al., 2010; Mejías et al., 2012), submarine springs, where the discharge occurs via deep sinkholes (Bakalowicz, 2015; Fleury et al., 2007), and diffuse discharge, a type of discharge which is not concentrated and occurs throughout sediments (Rodellas et al., 2014).
Identifying and mapping groundwater discharge areas is challenging, despite the number of traditional methods available for locating the main groundwater discharge locations and quantifying their flow rates. These methods include simple procedures such as deploying traditional local knowledge, conducting visual observations, monitoring changes in vegetation, as well as in water temperature and salinity, and using seepage meters or radioactive isotope tracers (Garcia-Orellana et al., 2021; Mejías et al., 2012; Rosenberry et al., 2020; Schubert et al., 2014). Apart from these methods, several authors have suggested thermal infrared remote sensing (TIR-RS) as an alternative methodology for identifying potential SGD spring sites, since it enables the screening and study of inaccessible zones and/or areas with a scarcity of hydrogeological information (Wilson and Rocha, 2012). Temperature has been used successfully to study SGD by comparing the relatively constant temperature of groundwater with that of surface seawaters, which fluctuates seasonally (Dale and Miller, 2007). In general, groundwater maintains a relatively constant temperature between depths of 5 and 100 m, approximately 1–2 ∘C higher than the mean annual air temperature (Anderson, 2005). The detection of SGD springs via TIR-RS is possible in any environment where there is thermal contrast between the discharging fluid and the receiving surface water body (Kelly et al., 2013). TIR images have the potential to identify the location of major SGD springs, and to study their spatial and temporal variability, by exploring the temperature difference between coastal seawater and brackish groundwater discharges at different times.
There are two types of platforms for obtaining thermal infrared information, i.e., airborne TIR-RS (airplane, helicopter and drone) and satellite TIR-RS (Modis, Aster and Landsat). Airborne TIR has been used for different applications; for example, Shaban et al. (2005) and Akawwi et al. (2008) conducted aerial TIR surveys along the Mediterranean Sea and Dead Sea coastlines to identify potential SGD sites. Kelly et al. (2013) used TIRS images from localized point source SGD to demonstrate that groundwater plume areas are linearly and highly correlated to in situ groundwater fluxes. Airborne TIR-RS has also been applied in combination with other methods, not only for qualitative SGD recognition, but also for quantifying groundwater flows from freshwater springs (Danielescu et al., 2009; Mejías et al., 2012). In the coastal carbonate aquifer of El Maestrazgo (Iberian Peninsula), a combination of complementary techniques was used to locate submarine springs via airborne high-resolution thermal infrared, radon measurements and physical–chemical anomalies and to quantify the groundwater discharge by direct quantification with flowmeters and Ra isotopes (Mejías et al., 2012). Tamborski et al. (2015) combined airborne TIR overflights with coastal radionuclide surveys to investigate the significance of SGD along the northern shore of Long Island (New York, USA) to provide quantitative evidence for TIR-RS as a tool to remotely identify and measure SGD. Finally, Danilescu et al. (2009) assessed total freshwater discharge in two small nutrient-sensitive estuaries in Prince Edward Island (Canada), using a combination of TIR images, direct discharge measurements and numerical simulations.
Compared to airplane, helicopter and drone platforms, satellite TIR-RS has some characteristics that limit its use for coastal water observation. The temporal resolution is fixed and varies depending on the satellite, with a minimum daily revisit frequency and a maximum frequency of every 16 d for a specific area. Furthermore, the spatial resolution, which varies between 30 and 1000 m, is lower than the airborne resolution. This results in the fact that small thermal anomalies, induced by small flows of SGD, are likely not to be detected. Additionally, satellite TIR-RS images are affected by atmospheric conditions (i.e., clouds and shadows). However, satellite TIR-RS imagery has the great advantage of being free of charge (Landsat), easily accessible, globally available, multi-temporal and covering a regional scale instantaneously. These advantages turn satellite TIR-based approaches into a viable and promising option for detecting SGD worldwide.
There are several satellite missions capable of measuring sea surface temperatures (SST) with a moderate spatial resolution and acquisition, namely the Advanced Spaceborne Thermal Emission and Reflection Radiometer (ASTER) and the Landsat satellite, among others, which provide an appropriate spatial and temporal resolution for large-scale SGD monitoring. For those sensors suitable for SGD research, Landsat is the one with the longest TIR data provision (from 1982 until today and already planned to go beyond 2030; Wulder et al., 2019) and is the one most widely applied for the purposes of SGD research (Wilson and Rocha, 2012, among others).
The use of satellite imagery in SGD studies has evolved in parallel with the launch of new sensors that feature spatial resolution improvements over previous sensors. However, the application of satellite TIR images is neither extensive nor widespread compared to airborne TIR images. Several SGD studies used Landsat 7 to locate groundwater discharge areas (e.g., Wang et al., 2008), to detect known but previously unmapped SGD locations (Varma et al., 2010), to determine the spatial extent and scale of SGD-derived temperature anomalies (Wilson and Rocha, 2012) and to infer SGD temporal variation using long-term thermal anomaly size variations (Mallast et al., 2014). More recent studies used data obtained by the Landsat 8 thermal infrared sensor (TIRS) to identify and characterize SGD sites using the sensor's technical improvements. For example, McCaul et al. (2016) proposed a multi-approach methodology for understanding submarine and intertidal groundwater discharge patterns. Xing et al. (2016) evaluated the ability of satellite remote sensing methods (Landsat 7 and 8) to detect thermal anomalies related to SGD as a possible index of the presence of offshore low-salinity groundwater storage at local scale. To the best of our knowledge, there is no study that thoroughly compares SGD locations with satellite-data-derived thermal anomalies over large spatial scales in order to assess the suitability of satellite TIR-RS data for conducting SGD research.
The aim of this work is to study the usefulness of satellite TIR images at different sites, covering a large scale and in different seasons to assess whether Landsat 8 TIR-RS can be used as an exploratory tool for identifying SGD springs worldwide, from local to regional scales, for long-term analysis. The second aim is to discuss the influencing factors to be considered in the identification of SGD at the local and regional level.
The study was carried out on the coastal karstic aquifers of the Mediterranean Sea basin, where there are many local studies that describe the discharge processes thanks to the significant connectivity between this coastal aquifer type and the sea (e.g., Bakalowicz, 2005; Barberá and Andreo, 2015; Worthington, 1999). In this hydrogeological context, SGD takes place mainly through submarine or aerial springs (point source). Although the groundwater discharge from submarine springs represents a negligible fraction of the global SGD (Luijendijk et al., 2020), in some areas, such as the Mediterranean Sea, this fraction can be locally important, strongly influencing marine ecosystems and serving as a freshwater resource for the population (Rodellas et al., 2015; Alorda-Kleinglass et al., 2021). To validate the temporal effectiveness of this technique, Landsat 8 images from 2017 and 2018 on the coasts of the Mediterranean Sea basin were used to locate SGD springs previously described in the scientific literature, showing in which period of the year these SGD springs are observable via satellite. In addition, we will hypothesize and discuss those factors that may condition the identification of SGD springs in order that future studies might take them into account when using satellite remote sensing TIR techniques.
2.1 Study area
The Mediterranean basin has been selected for this study because it is one of the areas of the world where numerous SGD studies have been carried out and where dozens of coastal springs have been described dating back to ancient times in countries such as Spain, France, Italy, Croatia, Greece, Turkey, Syria, Lebanon and Libya (Fig. 1). In this study, we focus on a set of 54 springs mentioned in the scientific peer review literature published in English (Basterretxea et al., 2010; Bakalowicz, 2018; Fleury et al., 2007; Mejías et al., 2012; Garcia-Solsona et al., 2010) where groundwater discharge is known to occur, and where there is a description of the hydrogeological context of each spring (Supplement 1). The number of springs included in the study represents at least 88 % of the submarine karst springs described in the English peer review literature concerning the Mediterranean basin.
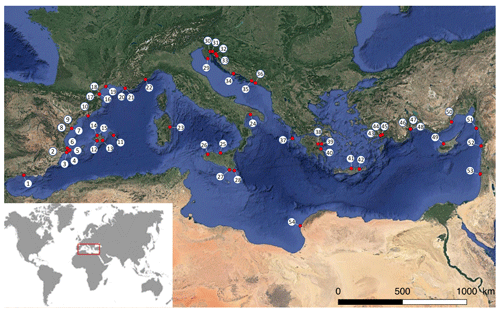
Figure 1Location of the 54 SGD springs used for this study. The SGD springs shown in the figure are described in Bakalowicz (2018), Basterretxea et al. (2010), Fleury et al. (2007), Garcia-Solsona et al. (2010) and Mejías et al. (2012). © Google Earth 2021.
The SGD contribution to the Mediterranean ranges from 3 to 50×1011 m3 yr−1, where fresh groundwater inputs represent 1 %–25 % of the total SGD inputs (Rodellas et al., 2015). SGD has been described and studied in several locations along the Mediterranean coast (e.g., Bakalowicz, 2015; Mejías et al., 2012; Tulipano et al., 2005; Bejannin et al., 2017). The Mediterranean basin is characterized by 46 % of its coastline being formed by karstic aquifers (Bakalowicz, 2015; Fleury et al., 2007; Trezzi et al., 2016). Its narrow continental shelves prevent large tidal amplification along the coast; tidal amplitude is usually less than 0.2 m (Werner et al., 2013). The Mediterranean climate is seasonal, characterized by windy, mild, wet winters and by relatively calm, warm and dry summers. Strong local winds, such as the cold and dry tramontane, mistral and bora from the north and the hot and dry sirocco from the south, are typical of the region. These strong regional and seasonal wind regimes provide a substantial amount of vertical mixing in the seawater column. In general, the main rainfall season is during fall and spring, with an average annual precipitation of 500 mm yr−1 (Andreo and Carrasco, 1993). The sea temperature is approximately between 26–30 and 14–19 ∘C in the summer and winter, respectively.
The available information for each of the 54 studied springs (Supplement 1) shows that the mean flow rates range between 0.009 and 50 m3 s−1, the distances from the shore range from the coastline to 1 km offshore and that discharge depths vary between 7 m a.s.l. (above sea level) and 150 m b.s.l. (below sea level). In accordance with these characteristics, we might classify the studied springs into five groups. The first group of springs discharge inland near the seashore and reach the sea through small streams; these karstic springs are located between 300 and 500 m inland and at elevations of 2, 3 and 15 m a.s.l. for Patan in Croatia, Almyros of Heraklion, Crete, in Greece and Maro in Spain, respectively. The second group of springs discharges in coastal lagoons at a distance of 100 m from the sea shore and a depth of 4 m b.s.l. (Font Dame and Font Estramar in Salses-Leucate lagoon in France) and at an unknown shore distance and 30 m b.s.l. (Vise in Thau lagoon, respectively). The third group of springs is located between 0 and 10 m from the shoreline and in shallow sea waters of between 0 and 7 m b.s.l. (Torre Badum, Las Fuentes and Font de Dins in Spain, Ain Zayana in Libya, Agios Nikolaos, Kefalonia and Anavalos Kiveri in Greece, and Ovacık and Gökova in Turkey). The fourth group of springs is also located close to the shoreline but at a water column depth of 12 m b.s.l. The two springs of this group are Moraig in Spain and Port Miou and Bestouan in France. The fifth and last group consists of Mortola in Italy and Chekka in Lebanon, in which discharges occurs offshore between 100 m and 1 km and with a water column depth of between 35 and 150 m b.s.l.
The type of coastal karst aquifer studied has been defined using the same classification as in Tulipano et al. (2005) for Mediterranean coastal karst aquifers. The first type has systems with poorly developed, but highly fractured, karstification. This karst type included three different subsystems in which (1) faults dissect the aquifer, such as in the Gökova (four springs; Bayari and Kurttaş, 2002) and Ovacık spring, where the faults are located in the underlying beds that extend towards the sea (Elhatip, 2003), (2) groundwater flows along the zones of cracks, fractures and karst hollows, such as in the Donnalucata spring (Povinec et al., 2006), and (3) groundwater flows through stratification joints, such as in the Mortola spring (Fleury et al., 2007). The second type has systems with well-developed karstification connected to the sea (e.g., Moraig, Port Miou, Bestouan, Almyros of Heraklion, Almyros of Agios Nikolaos, Kefalonia, Ain Zayana and Chekka). The last type of defined karst system is a well-developed karstification but with low connectivity with the sea. This group is represented by only two springs, i.e., Kiveri Anavalos in Greece and Vise in France.
2.2 Landsat 8 TIRS data acquisition
To determine the optimal time period for SGD detection using remote sensing, the SST of a series of images covering all seasons was compared. The TIRS instrument of Landsat 8 is a thermal imager with two thermal infrared bands centered at 10.8 and 12.0 µm and a ground sampling distance (GSD) of 100 m. However, all thermal images are resampled using a cubic convolution to 30 m (Roy et al., 2014). To carry out this study, only the thermal band 10 TIRS 1 (10.6–11.2 µm) of the 11 Landsat 8 bands was used to study the SGD sites. The other Landsat 8 thermal band, 11 TIRS 2 (11.50–12.51 µm), was not used because the data collected in this band had some large calibration uncertainties (US Geological Survey, 2014).
A total of 27 path and row combinations were analyzed with the Landsat 8 TIR images of the Mediterranean coast between January 2017 and December 2018 to cover all 54 known SGD sites. To that end, a total of 1296 images (two images per month for the 2 years of study) were acquired from the US Geological Survey (USGS), with cloud coverage between 0 % and 90 % in each image. A manual inspection of all images resulted in finer selection of 413 images with cloud-free conditions above the areas of interest (Supplement 2). The finer selection of cloud-free images was used for subsequent steps.
2.3 Deriving SST values from Landsat 8 TIRS data
Data processing included the conversion of digital numbers to SST, including an atmospheric correction of each image following the methodology presented by Chander et al. (2009). Image processing began with radiometric correction, which was performed by converting the digital number (DN) to sensor spectral radiance through band-specific rescaling gain and bias factors according to Eq. (1).
where Lλ is the sensor spectral radiance (W m−2 sr−1 µm−1), Grescale is the band-specific rescaling gain factor (W m−2 sr−1 µm−1 DN−1), Qcal is the quantized and calibrated standard product pixel values (DN) and Brescale is the band-specific rescaling bias factor (W m−2 sr−1 µm−1).
The next step was an atmospheric correction to remove the atmospheric component of the recorded thermal signal which strongly depends on atmospheric conditions (aerosol content, humidity, temperature, etc.) at a specific recording time and place. To atmospherically correct images at sensor spectral radiance, it was necessary to transform them into surface radiance of an ideal blackbody, considering the scene-specific up- and downwelling radiance and transmission values and the emissivity of the water surface, according to Eq. (2) (Barsi et al., 2003).
where Lt is the radiance of an ideal blackbody (W m−2 sr−1 µm−1), Lλ is the sensor spectral radiance (W m−2 sr−1 µm−1), LU is the upwelling radiance (W m−2 sr−1 µm−1), LD is the downwelling radiance (W m−2 sr−1 µm−1), ε is the emissivity of the surface (–), and τ is the atmospheric transmission (–).
A web-based atmospheric correction tool (Atmospheric Correction Parameter Calculator) developed by Barsi et al. (2003), based on MODTRAN, was used to obtain values for atmospheric transmissivity and the upwelling and downwelling radiances of the atmosphere. The emissivity of water in the Landsat 8 TIR bands ranges from 0.98 (band 11) to 0.99 (band 10), and in this study, we assume a constant emissivity of 0.99 (Wen-Yao et al., 1987).
Finally, to obtain the sea surface temperature (SST), the corrected radiances were introduced into Eq. (3).
where T is the effective sensor brightness temperature (K), Lt is the radiance of an ideal blackbody (W m−2 sr−1 µm−1), K1 is prelaunch calibration constant 1 (W m−2 sr−1 µm−1), and K2 is prelaunch calibration constant 2 (K).
The resulting atmospherically corrected SST data represent temperature with an error of less than 1.3 K for the temperature range 270–330 K. This temperature represents the skin temperature of the water (<1 mm of the upper most water layer), which differs from the bulk temperature below it by about 0.1 K due to sensible heat fluxes, evaporative heat loss and longwave radiation (Donlon et al., 2002; Wloczyk et al., 2006).
2.4 Site inter-comparison between a single image and multiple images
Temperature maps of coastal waters were created from temperature data to assess the significance of the SST anomalies. The identification of SGD spring sites was based on the assumption that temperatures of discharging groundwater may be different than seawater and less variable than seawater temperatures throughout the year. SGD spring sites were analyzed using two different procedures. First, single images were used to identify SGD springs by means of the water temperature anomalies. As a second step of the single image approach, the change between images along the study period was also evaluated. This qualitative analysis allows us to observe variations in the morphology and temperature range of the known discharge plume between images. As a second approach, called multiple imaging, SGD spring sites were detected by evaluating the pixel-by-pixel standard deviation (SD) across all image sets. Lower values of SD were used as indicators for groundwater discharge using a sea surface temperature (SST) data series. This statistical parameter has been previously applied in semi-arid areas to study groundwater and surface water interactions and identify spring discharge into lakes or enclosed seas (Mallast et al., 2014; Tcherepanov et al., 2005). It was assumed that groundwater tends to be less variable than surface water, which varies seasonally and daily. The applied multi-temporal thermal remote sensing approach was based on a variable number of Landsat 8 TIRS images. The images used to calculate the standard deviation varied between 5 and 17 images, depending on the number of images without clouds available for each studied site. The resulting thermal maps were combined with satellite imagery from Google Earth (only the land part) using GIS (geographic information system; QGIS Las Palmas) to show the location the SGD springs at the identified sites.
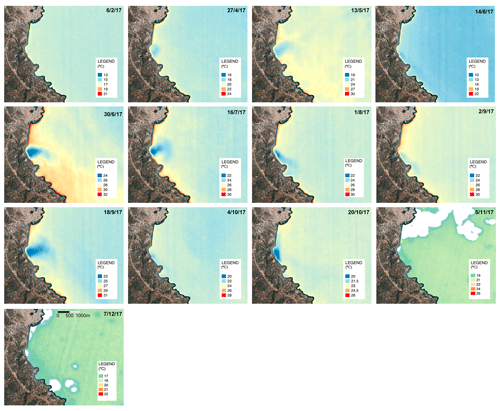
Figure 2SST images (degrees Celsius) obtained via band 10 of Landsat 8 OLI/TIRS of the region of Almyros of Agios Nikolaos (Crete, Greece) throughout 2017. Of the 23 images analyzed, the presence of an SGD spring was clearly visible in nine images that represent a 37 % ratio of success. In January, March and November 2017, it was not possible to obtain an SST image due to the presence of clouds. © Google Earth 2021.
3.1 Overall identifications
As an example of the Landsat 8 TIR images analyzed in the Mediterranean Sea basin, the thermal images of the Almyros SGD spring in Agios Nikolaos (Crete, Greece) throughout the year 2017 are shown in Fig. 2. In general, a thermal anomaly plume is observed in several of the 23 images (one of the July images is missing) occurring near and perpendicular to the coastline, reflecting the continuous discharge of groundwater. Since a satellite image provides the sea surface temperature (SST) of the first millimeter of seawater (Donlon et al., 2002; Wloczyk et al., 2006), the thermal contrast due to SGD can be observed as the fresh groundwater flows over seawater due to its lower density (i.e., lower salinity; Wilson and Rocha, 2012). The images series of Almyros Agios Nikolaos (Fig. 2) show how thermal contrast caused by the groundwater discharge cannot be observed throughout the whole year. The thermal contrast is more identifiable from the second half of April until the end of October, but the best thermal plume observations were from June to October (Fig. 2). Conversely, this spring cannot be identified from November to March. The groundwater discharge was not identified in February and December, due to the absence of thermal contrast, and in the other months (January, March and November) because clouds made identification difficult. Thus, the overall percentage of time for optimal SGD spring identification was 37 % in 2017.
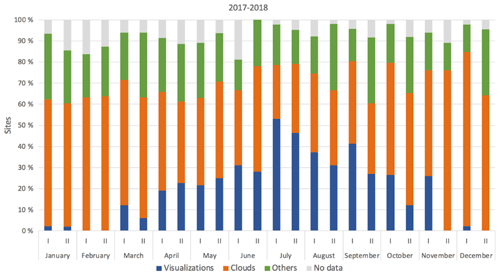
Figure 3Percentage of successful and unsuccessful SGD identifications for all SGD springs that were identified at least once (23 springs representing 44 %) throughout the year 2017 and during the year 2018 (based on the selection of 720 images) are shown individually in the Supplement 3. The x axis shows the months of the year. Roman numerals (I and II) represent the two satellite passes per month that cover every area. No image available is represented in gray, while the presence of clouds is in orange and blue for successful SGD identifications. Those for which the images were not covered by clouds, and where SGD springs were not identified, are shown in the figure as “others” in green.
If the same approach is applied for the total of 54 SGD springs studied, only 23 springs were identified in individual images, representing a 44 % success rate for the technique over the entire study period (2017 and 2018). The success percentage for identifying the 23 SGD springs, according to the period of the year, is shown in Supplement 3. The highest success percentage for SGD identification was during the summer, specifically from June to September (Fig. 3), which corresponds to an average of 21 % of the images analyzed. Conversely, the SGD springs were not identified in the remaining 79 % of the images. The winter period, from December to February (Fig. 3), had the lowest percentage of SGD spring identifications.
3.2 Influencing factors to consider in the identification of SGD springs
The analysis of the images of the Mediterranean coast obtained by Landsat 8 TIRS during 2017 and 2018 was successful for several studied springs but did not identify SGD springs in all the images analyzed. Thus, identifying SGD springs using Landsat 8 TIRS presents some limitations, as the success rate was slightly less than 50 %. The following potential limitations were previously reported in the literature in some local studied areas: only information for the first millimeters of seawater is available (Donlon et al., 2002; Wloczyk et al., 2006), spatial resolution (Wilson and Rocha, 2012), period of the year (Bayari and Kurttaş, 2002; Wilson and Rocha, 2012; Xing et al., 2016), the results are highly dependent on atmospheric temperature, seawater currents, wind speed and direction, sea surface effects (Kelly et al., 2013) and cloud cover, and the need for specialist knowledge to convert the data into accessible (visualized) information (McCaul et al., 2016).
Given the abovementioned limitations, we propose a conceptual framework of factors representing technical limitations in order to assess those issues that should be considered when applying TIR-RS to the study of SGD. These technical limitation factors can be grouped as follows: (1) technical limitations, (2) geological and hydrogeological characteristics, (3) environmental and marine conditions, (4) coastal geomorphology, and (5) anthropogenic sources (Fig. 4).
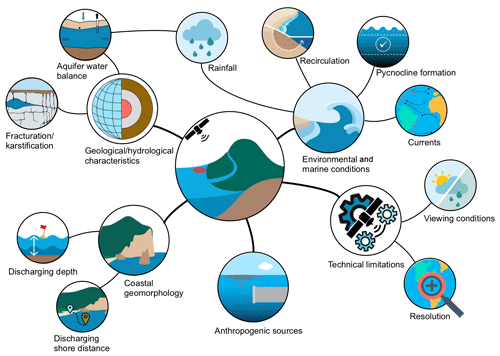
Figure 4Conceptual framework of technical limitation factors that can limit the identification of SGD springs by using Landsat 8 TIRS images.
3.2.1 Technical limitation factors
Some of the main limitations of the technique are related to the temporality of obtaining images, the spatial resolution, the availability of images in the desired period and the atmospheric conditions during the image capture. Each satellite has an image acquisition spatial and temporal resolution, and therefore, the results are subject to these pre-established conditions. For Landsat 8 TIRS, the temporal resolution is 2 weeks, and the spatial resolution is 100 m resampled to 30 m, so a smaller thermal anomaly plume than this produced by an SGD spring will not be identified. For example, in the 20 m wide semi-enclosed cove of Alcaufar (Menorca; western Mediterranean), there are several described springs (García-Solsona et al., 2010) that were not identified by satellite due to the small dimensions of the cove (García-Solsona et al., 2010). The availability of satellite images depends, in some cases, on technical problems that the satellite experiences during image collection, which implies that there are annual series of images with some missing images. In the example used in Fig. 2, where the Almyros of Agios Nikolaos (Greece) SGD spring is identified, only 23 of the 24 images that should have been produced during 2017 were obtained. The striping noise that can be seen in the images (Fig. 2), and which affect TIR bands especially, is another technical limitation. Sometimes the difference is so large that it would hinder a proper SGD detection (Gerace and Montanaro, 2017).
Other important limitations related to the technique include the atmospheric conditions at the time of the image capture. Clouds and clouds shadows change radiometric information leaving the sea surface and prevent a correct analysis of the images. For the Mediterranean Sea basin, of 80 % of the images in which SGD springs were not identified, 60 % were due to the high presence of clouds. Thus, clouds are the main factor limiting the identification of SGD springs. The presence of clouds is higher in winter than in summer; therefore, warmer months are much better for identifying SGD springs in the Mediterranean Sea. However, in at least 20 % of the cloudless images, it was not possible to observe and locate SGD springs described in the literature. Therefore, a detailed analysis of the cloudless imagery is necessary to confirm the optimal conditions for locating SGD springs in the area of interest (Anderson, 2005).
3.2.2 Geology and hydrogeology limitation factors
The characteristics of coastal aquifers and the different driving forces that allow groundwater discharge into the sea are other factors that can limit the identification of SGD springs. According to Garcia-Orellana et al. (2021), SGD is the combination of fresh terrestrial groundwater discharge driven by hydraulic gradient between land and sea and saline groundwater discharge from the seawater recirculation through the coastal and continental margin. Thus, the thermal signal of both types of groundwater will define the intensity and shape of the plume that can be observed in the sea.
For coastal aquifer SGD springs, geological factors such as the lithology (e.g., type of rock, degree of karstification, presence of faults and/or fractures, etc.) determine the hydraulic properties that greatly influence the groundwater flow that discharges into the sea (Brunner et al., 2007; Edet et al., 1998). Another aspect to consider is the connectivity of the aquifer with the sea. Coastal aquifers may be totally or partially hydraulically connected to the sea, depending on several factors such as the geological structure of the aquifer and the seabed, as well as the hydraulic properties of both onshore and offshore geological formations. In addition, the amount of SGD depends on the aquifer water budget, which produces variations in groundwater discharge flow. The main factors that influence this budget are the natural variations in recharge at different temporal scales (rainfall events, seasonality and inter-annual changes) and the abstraction of groundwater, which can reduce the discharge of the fresh groundwater component of the SGD or even induce seawater intrusion into the coastal aquifer. Unfortunately, these factors are linked since, in most cases, when recharge is low, abstractions tend to be higher.
In the presented research, from the 54 karstic SGD studied springs, information on the type of coastal karst aquifer is only available for 37 % of the springs (Supplement 4). From those, the first karst-type system, characterized by poorly developed but highly fractured karstification, represents 11 % of the springs, and 7 % of the total were successfully identified. The second type of karst system, characterized by well-developed karstification connected to the sea, represents 22 % of the springs, and half of those were identified (11 % of the total). Finally, the third karst type system, with well-developed karstification but low connectivity with the sea, represents only 4 % of the springs, and half of those were identified (1.8 % of the total). Therefore, the best geological and hydrogeological settings for identifying SGD springs are karst systems with well-developed karstification and that are well-connected to the sea, as well as those systems with poorly developed but highly fractured karstification. This is because these systems have high transmissivities that can induce high local SGD rates, making it easy to detect their thermal plumes. However, it depends on the recharge area and its rate.
Conversely, low groundwater discharge water volumes may limit the identification of thermal anomalies because they could attenuate the thermal anomaly produced by the SGD. Therefore, low discharge water volumes are unlikely to be identified using this technique. Of the 54 karstic SGD studied springs, the flow rate information was only available for 59 % of the springs (Supplement 4). Springs with a flow rate lower than 10 m3 s−1 represent 40 % of the springs. From those, only 50 % were identified. The springs with a flow rate higher than 10 m3 s−1 represent 15 % of the springs, and from those, 60 % were identified. This indicates that, apart from flow rate, other factors affect the failure to identify these SGD springs on the coastline.
Inter-annual and annual changes, such as humid–dry periods, overexploitation and extreme events such as strong rainfall storms, etc., can also modify SGD flows. Thus, the research period in which the images were collected is also important because seasonal variations (Lee et al., 2016) can lead to SGD thermal plume variations, not only due to the temperature difference between groundwater and seawater (Lee et al., 2016) but also the annual SGD flow rate variations (Michael et al., 2005) that allow their identification.
Regarding hydrogeological conditions in the Mediterranean Sea basin, it was expected that the best seasons for identifying SGD springs using satellite TIR-RS would be spring and autumn, when rainfall is higher, and therefore, a higher discharge flow rate is expected. However, results showed that late spring and summer were the best seasons for identifying SGD springs, indicating that other factors influence the identification of SGD springs using this technique.
3.2.3 Environmental and marine conditions
Other aspects that could affect the thermal contrast between the groundwater plume and seawater include environmental and marine conditions. Both factors can make the identification of SGD difficult because they cause seawater mixing with the discharging groundwater, reducing the thermal contrast between them and modifying the sea surface temperature. The main environmental condition that can affect SGD identification is the action of wind, which can mix the first millimeters of the sea surface water, limiting the identification of SGD springs using remote sensing techniques. Similarly, marine conditions can affect SGD identification if they reduce the thermal contrast between the groundwater plume and the seawater. These marine conditions are basically reduced to the coastal water hydrodynamic conditions that can be affected by processes such as the influence of tides or coastal currents, the formation of a pycnocline in surface seawater or the fetch due to wind action. Tides, coastal currents and fetch generate seawater movement and mix groundwater with seawater, causing a thermal contrast attenuation. Similarly, the presence of a pycnocline can result in less vertical mixing of the water column. In subtropical areas with cold winters and hot summers, such as the Mediterranean Sea, coastal waters often develop a pycnocline during the summer months, as high temperatures increase the evaporation of seawater, generating an increase in salinity and therefore water density. This effect causes cold, fresh groundwater to flow over salty and dense seawater, generating an SGD layer on the sea surface.
The temperature of the Mediterranean Sea oscillates seasonally between 26–30 ∘C in summer and 14–19 ∘C in winter, while the groundwater temperature remains relatively constant over time, implying a greater contrast between summer and winter. However, SGD spring visualizations in cloud-free images decrease significantly in winter months compared to warmer months (Fig. 4). In total, 35 % of the springs were identified in summer, and only 4 % were identified in winter (Supplement 4). Consequently, the number of SGD spring visualizations is much higher in summer than in winter. In the winter months, wind, water column mixing, currents, etc., are more intense and reduce the thermal contrast. Therefore, environmental and marine conditions during the winter months are unfavorable for the identification of coastal springs.
For this reason, areas where the influence of various marine factors such as tides, coastal currents or fetch is small are ideal for identifying SGD springs because such factors induce mixing attenuating the thermal contrast needed to identify SGD springs. Similarly, in subtropical areas such as the Mediterranean Sea, it is easier to identify SGD springs because coastal waters often develop a pycnocline during the summer months, resulting in less vertical mixing of the water column and, therefore, better identification of SGD springs. Therefore, in the Mediterranean basin, environmental and marine conditions in summer months are much more favorable for the identification of coastal springs than in winter months.
3.2.4 Coastal morphology limitation factors
Another aspect that influences SGD thermal plume visualization is the coastal morphology; depending on its characteristics, the seawater residence time in the study zone may allow the formation of a thermal plume. In semi-enclosed areas, such as bays or coves, where the residence time of discharging water in the sea is in the range of 1 to several days (Tamborski et al., 2020), the formation of thermal plumes from SGD can easily be detected by satellite, in comparison with open-sea areas, where the seawater residence time is shorter (less than 1 d), due to the effect of marine or weather factors such as waves, coastal currents or fetch. Groundwater discharge can occur below sea level, either at shallow (<10 m) or at greater depths, right on the shoreline or a few meters from it (Zektser et al., 2006). The morphology of the coastal seabed combined with the geological characteristics of the aquifer (e.g., karstification degree and coastal hydraulic gradient) determine both the depth and offshore distance of the groundwater discharge. These two characteristics are very significant, since the location of the SGD spring on the coast is critical for the correct identification of the thermal contrast between groundwater and seawater using satellite thermal images. While groundwater discharges produced at coastal level or several meters inland usually generate thermal contrasts that are easily detectable by satellite (Mejías et al., 2012), submarine springs located several meters deep often represent a challenge for satellite detection. This is because, when groundwater discharges below sea level, it rises to the sea surface, generating a buoyant thermal layer of several millimeters due to its lower density that arises from its temperature and salinity characteristics. Therefore, a greater discharge depth requires more time to reach the sea surface, adapting the SGD's thermal signal at the sea surface to the surrounding water temperature. Thus, marine factors may have a greater influence in balancing the seawater and groundwater temperatures, preventing the recognition of thermal anomalies on the sea surface, as stated by Mallast et al. (2013).
However, the time required for groundwater to reach the sea surface depends not only on the depth of the discharge below sea level but also on hydrogeological factors, such as the discharge flow. Thus, SGD springs characterized by shallow depths and large flow rates will favor the detection of SGD-induced thermal plumes on the sea surface, while deep areas with small flows may be undetectable. Intermediate situations, such as shallow areas with small flows or deep areas with large flows, may be detected, depending on the relative importance of the environmental and/or marine limiting factors.
For studied springs in the Mediterranean Sea basin, 52 % of the springs were located in semi-enclosed coastal areas (Supplement 4). Of these, 31 % of the studied springs were identified (Port Miou and Bestouan in France; Kefalonia, Anavalos Kiveri and Almyros of Agios Nikolaos in Greece; Martinšćica, Perilo, Novijanska, Jurjevska and Patan in Croatia; Gökova (three springs), Ovacık and Antalya (three springs) in Turkey). These results show that, when discharge occurs in semi-enclosed areas where the seawater residence time is large, the springs can be identified until late autumn, even though the flow of groundwater is relatively small (0.75 m3 s−1 was reported for the Ovacık spring in Turkey). Therefore, the success in identifying SGD springs in semi-enclosed areas also is higher throughout the year.
Of the 54 SGD studied springs, the distance and depth information are available for 41 % of the springs (Supplement 4). The first group of springs, which discharge near the seashore and reach the sea through small streams, represents 6 % of the springs (Patan in Croatia, Almyros of Heraklion in Greece and Maro in Spain), and from those, 66 % were identified (Patan and Almyros of Heraklion). The second group of springs, which represent 6 % (Font Dame, Font Estramar and Vise) and are characterized by discharge in coastal lagoons, was not identified. The third group of springs, which discharge close to the shoreline and in shallow water, represents 20 % of the springs (Torre Badum, Las Fuentes, Font de Dins in Spain, Ain Zayana in Libya, Agios Nikolaos, Kefalonia and Anavalos Kiveri in Greece, and Ovacık (3 springs) and Gökova in Turkey), and we were able to identify 91 % (all of them except the Ain Zayana spring). No springs were identified from the fourth group, which represents 6 % of the springs (Moraig in Spain and Port Miou and Bestouan in France) and is characterized by discharge close to the shoreline but at a water column depth of 12 m b.s.l. None of the springs in the fifth group (Mortola in Italy and Chekka in Lebanon), which represent 4 % of the springs and are characterized by discharge offshore with a water column depth between 35 and 150 m b.s.l., were identified.
From the analysis of the images obtained by Landsat 8 TIRS, and considering the hydrogeological data of the various coastal springs, we conclude that groundwater discharges that occur at significant depths (>12 m b.s.l.) are unlikely to be identified by this technique, probably because the thermal anomaly generated between groundwater and seawater does not reach the sea surface. This is the probable reason for the failure to identify the springs of Vise in France, Mortola in Italy and Chekka in Lebanon. For the Vise spring, which discharges at 30 m b.s.l., the hydrogeological information indicates that it is a mixture of karstic water, thermal water and seawater (Aquilina et al., 2002); most likely, the discharging groundwater does not produce enough thermal contrast to be detected with a satellite sensor. On the other hand, SGD springs located in very shallow areas, such as the Salses-Leucate lagoon, which has an average maximum depth of 2 m b.s.l. (Bejannin et al., 2017), and where there are two well-known springs (Font Dame and Font Estramar), were not identified. A possible reason is that these types of shallow coastal lagoons are highly influenced by atmospheric temperature, since the small water column has little capacity to buffer temperature fluctuations (Lee et al., 2016). However, Anavalos Kiveri and Torre Badum, identified during winter months, are shallow (0 to 10 m b.s.l.) SGD springs. Therefore, although initially these types of springs should be easily detectable by satellite, atmospheric factors can greatly influence the formation of the thermal contrast needed to identify them.
The shore distance at which groundwater discharge occurs is another factor that affects the identification of groundwater discharge in coastal areas. SGD springs discharging further than 500 m from the seashore were not recognized, such as the Mortola spring with a distance of 800 m. This may be related to the fact that, as the distance to the coast increases, the depth of the water column increases, and the travel time of the fresher groundwater also increases, allowing a complete temperature equilibrium. Furthermore, these zones are more affected by ocean currents that increase water mixing, attenuating the thermal signal.
In some cases, there is not one single factor affecting SGD spring identification but rather a combination of several factors (flow rate, season, coastal morphology, etc.) which makes it difficult to explain why an SGD spring was not identified.
3.2.5 Thermal anomalies from streams and anthropogenic sources
When looking for coastal thermal anomalies by using satellite TIR images, some anomalies can be detected that are not necessarily related to SGD. These anomalies can be from natural sources, such as rivers or streams, or civilian facilities such as ports, thermal power plants, fish farms or wastewater treatment plants. These thermal anomalies may misidentify or mask some SGD springs when discharge occurs in shallow areas with high seawater residence times.
For example, natural water sources, such as small rivers or streams, also produce a thermal signal at sea. As SGD, they may have a different temperature than seawater. This is the case for the small river near Split in Croatia (Supplement 5), which produces a thermal anomaly that could be misinterpreted as an SGD spring. Furthermore, when SGD and rivers discharge at the same location, the thermal signal of SGD will be masked and/or modified by surface water discharge. In the case of civil facilities, the most common facilities that could be confused with SGD springs would be the discharges from wastewater treatment plants as they usually discharge water with a different thermal signal than the sea. For example, in the Hyperion Treatment Plant (HTP) and the Orange County Sanitation District (OCSD) wastewater diversion events in Southern California, where treated water discharge takes place at depth, it is possible to detect its thermal effect on the coastal waters (DiGiacomo et al., 2004) with SST differences of at least 0.5 ∘C identifiable with TIR-RS (Gierach et al., 2017). Other examples of civilian facilities that can generate thermal signals and induce non-identification or masking of SGD springs are power plants or ports. In the case of the Mediterranean Sea basin, it was observed that facilities located along NE of the Iberian Peninsula, such as the Vandellós II Nuclear Power Plant, the Port of Barcelona and the Port of Benicarló, showed thermal anomalies similar to those produced by the SGD springs (Supplement 5). These outflows can also mask and modify the SGD thermal signal of the plume when both outflows take place at the same location, such as the case of Peñíscola in Spain, where the SGD discharge takes place at the same location as the port (Supplement 5).
3.3 Application of the multi-temporal SST series method
In some studied areas, there may be low thermal contrast that can prevent the identification of SGD springs. In these cases, it is possible to deepen the analysis of the images by using the multi-temporal SST series proposed by Mallast et al. (2014) in the Dead Sea, which minimizes the effect of the previously indicated limiting factors. Unlike single images, the multi-temporal SST series method allows several images and, thus, several points in time to be integrated, accentuating the small thermal anomalies for easy identification. An example of the usefulness of the multi-temporal SST series method is in the Serra d'Irta (eastern Iberian Peninsula; Mejías et al., 2012; Garcia-Solsona et al., 2010; Trezzi et al., 2016). At this site, Mejías et al. (2012) identified four large thermal anomalies (Torre Badum, Punta del Pebret and Les Fonts springs), by using airborne thermal remote sensing, that were not identified using Landsat 7. Using single images with Landsat 8 TIRS, only the Torre Badum spring was identified when compared with the four thermal anomalies identified by aerial thermal infrared images in 2012. The multi-temporal SST series method, which integrates 10 cloud-free images from 2017 to 2018, enables the identification of Torre Badum and Les Fonts, two of the four springs identified in 2012 by Mejías et al. (2012; Fig. 5).
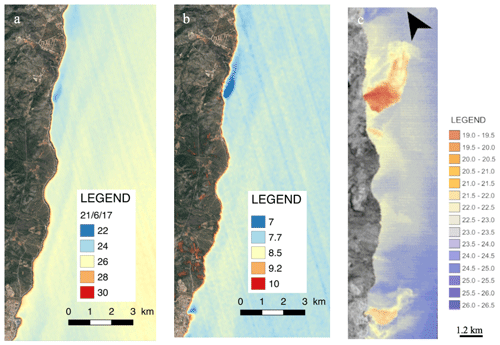
Figure 5(a) Serra d'Irta single image map using an image taken on 21 June 2017. One spring in the middle of the Torre Badum spring was identified. (b) Serra d'Irta standard deviation (SD) map using 10 cloud-free images acquired between 2017 and 2018. In total, two springs in Serra d'Irta, in the south of the Les Fonts beach at the Alcossebre spring and in the middle at the Torre Badum spring, were identified. (c) Image modified from Mejías et al. (2012), where SGD springs were identified using aerial thermal infrared images. © Google Earth 2021.
Although the multi-temporal SST series method is better for identifying SGD springs because the thermal contrast is enhanced, this method did not enable SGD spring identification in other places where the single-image method did not identify them. Furthermore, with multi-temporal SST series, the temporal morphological information, such as the shape and size of the discharge plume, is lost (Mallast et al., 2013). Conversely, single images allow the identification of morphological variations in the discharge plume and the temperature variations along that plume. Therefore, if the study objective is to identify SGD springs, the multi-temporal SST series method is the best option, but if the objective is to study the SGD plume variation, single images are better.
3.4 Identification of new SGD springs: challenges and recommendations for future SGD studies
One of the main objectives of this study is to demonstrate the great usefulness of satellite TIR imagery at local and regional scales, identifying SGD springs not previously described in the scientific literature. An example of this usefulness is the identification of 21 SGD springs undescribed in the bibliography along the Croatian coast using single images (Fig. 6). This demonstrates that the analysis of single images obtained from Landsat 8 TIRS allows the identification of new SGD springs. Therefore, this economical technique is very useful in inaccessible areas (Gumma and Pavelic, 2013; Edet et al., 1998). For this reason, in the design of SGD studies at both local and regional levels, it is recommended that the coastal water temperatures for thermal anomalies first be screened using the presented satellite-based TIR-RS approach, as this will help narrow the sampling surveys. Although the study of the thermal images during a single year should be sufficient to identify potential coastal springs, it is highly advisable to analyze thermal images over several years, since there may be one or several factors (technical, marine, environmental, hydrogeological, etc.) that may alter the SGD thermal signal.
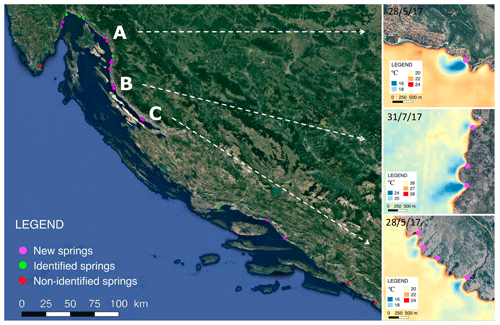
Figure 6Springs reported in the literature (described SGD spring) vs. a new SGD spring identified with Landsat 8 TIRS. New SGD springs, not reported in the literature, are represented in pink. Springs reported in the literature are represented in green and white. © Google Earth 2021.
Although this study focused on the discharge of groundwater from springs located in karst aquifers, the study of satellite TIR-RS images could be extrapolated to other types of aquifers where the discharge is more diffused and where the proportion of meteoric water is lower. To identify thermal plumes, water discharging into the sea, whether of meteoric origin or marine circulation, must have acquired sufficient thermal contrast in the coastal aquifer before discharge. This thermal contrast can also occur in places where coastal aquifers are volcanic rocks or fractured granites and even in sedimentary formations. However, in each case, the specific geological context (aquifer matrix, hydraulic parameter distribution, etc.) should be considered in the analysis.
Several studies have shown the possibility of quantifying SGD through the study of thermal plumes obtained by aircraft (Tamborski et al., 2015; Danielescu et al., 2009; Mejías et al.,2012). By determining the thermal plume area, which is often directly related to the discharge volume, SGD may be quantitatively identified. However, there are several factors that can alter the thermal plume shape that could result in error. Furthermore, because the TIR-RS image only allows us to observe less than 1 mm of the sea surface, and thus prevents a determination of the volume beneath the thermal plume, the required information is missing. Moreover, quantification by means of images represents a major challenge because satellite images (e.g., Landsat 8 TIRS) can only be obtained once every 16 d, and many factors can alter the shape of the plume independent of the actual discharge. Therefore, unless the discharge occurs in locations where the environmental and marine conditions are well known, quantification by satellite images represents a major challenge that should be further investigated.
Although this study focused regionally on the Mediterranean Sea basin, it can be extrapolated to other parts of the world and in places where the SGD has sufficient thermal contrast when discharging into the sea. This technique has been successfully used in regional areas such as Ireland (Wilson and Rocha, 2012; McCaul et al., 2016) or Laizhou Bay in China (Xing et al., 2016). However, in areas where the thermal contrast between the sea and the aquifer is low and/or temperature does not fluctuate during the year, such as in tropical zones, the use of satellite images represents a challenge that must be explored in detail. Furthermore, it is important to perform an initial study to attempt to identify the previously described main factors that can limit SGD spring identification in the study area, to try to control/avoid these effects.
This study demonstrates that satellite remote sensing is a useful tool for the identification of coastal SGD springs in karst aquifers, both locally and regionally, by performing an initial screening of the coastal water temperature images to identify possible thermal anomalies that can help narrow SGD study sampling surveys. However, this study highlights the following limiting factors that should be considered: (1) technical limitations, (2) geological/hydrogeological characteristics, (3) environmental and marine conditions and (4) coastal geomorphology.
In the karstic coastal aquifer of the Mediterranean Sea basin, the highest success percentage of SGD visualizations was during June, July, August and September. The main factor limiting the identification of SGD springs was clouds, which are far more abundant in winter months. The best geological and hydrogeological settings for identifying SGD springs were karst systems with well-developed karstification that are well-connected to the sea and those systems with poorly developed, but highly fractured, karstification. Environmental and marine conditions (such as pycnocline formation, light winds, etc.) in the summer months were much more favorable for the identification of coastal springs than in the winter months. Semi-enclosed areas, where the seawater residence time is large, favor the identification of SGD springs throughout the year. Groundwater discharges that occurred at a depth of more than 12 m b.s.l. or in very shallow waters were not identified. Also, SGD springs that discharged further than 500 m from the seashore were not recognized.
Multi-temporal SST series are better for identifying SGD springs in coastal zones, but the information for changes in the discharge plume changes is lost, while single images are more suited to studying the morphological variations in the discharge plume and temperature variations along the plume.
Although this study focused regionally on the Mediterranean Sea basin, it can be extrapolated to other parts of the world and other aquifer types in places where the SGD has sufficient thermal contrast when discharging into the sea and where the specific geological contexts (e.g., aquifer matrix and hydraulic parameters distribution) are considered. Long time series are better for identifying SGD spring areas, since there may be one or several factors (technical, marine, environmental, hydrogeological, etc.) that can alter the SGD's thermal signal. It is recommended to first screen the coastal water temperature images obtained by satellite to identify possible thermal anomalies that will help narrow the sampling surveys. This technique allows the identification and quantification of SGD springs in zones without hydrogeological information.
All data are available from the corresponding author upon request.
The supplement related to this article is available online at: https://doi.org/10.5194/hess-25-4789-2021-supplement.
SJC, AF and JGO contributed to design and implementation of the research, to the analysis of the results and to the writing of the paper.
The authors declare that they have no conflict of interest.
Publisher's note: Copernicus Publications remains neutral with regard to jurisdictional claims in published maps and institutional affiliations.
The authors want to acknowledge Francisco Carreño Conde, from the Universidad Rey Juan Carlos, for the initial discussions on the topic and Ulf Mallast, from the Helmholtz Centre for Environmental Research – UFZ, for the review of the paper. Albert Folch is a Serra Húnter Fellow.
This work was partly funded by the Spanish Government (grant nos. PID2019-110212RB-C22 and PID2019-110311RB-C21) the “Maria de Maeztu” program for Units of Excellence (grant no. CEX2019-000940-M) of the Spanish Government and the project TerraMar (grant no. ACA210/18/00007) of the Catalan Water Agency. The authors want to thank the support of the Generalitat de Catalunya to GHS (grant no. 2017 SGR 1485) and MERS (grant no. 2018 SGR-1588) for additional funding.
This paper was edited by Marnik Vanclooster and reviewed by Alice Alonso and one anonymous referee.
Akawwi, E., Al-Zouabi, A., Kakish, M., Koehn, F., and Sauter, M.: Using thermal infrared imagery (TIR) for illustrating the submarine groundwater discharge into the eastern shoreline of the Dead Sea-Jordan, Am. J. Environ. Sci., 4, 693–700, https://doi.org/10.3844/ajessp.2008.693.700, 2008.
Alorda-Kleinglass, A., Garcia-Orellana, J., Rodellas, V., Cerdà-Domènech, M., Tovar-Sánchez, A., Diego-Feliu, M., Trezzi, G., Sánchez-Quilez, D., Sanchez-Vidal, A., and Canals, M.: Remobilization of dissolved metals from a coastal mine tailing deposit driven by groundwater discharge and porewater exchange, Sci. Total Environ., 688, 1359–1372, https://doi.org/10.1016/j.scitotenv.2019.06.224, 2019.
Alorda-Kleinglass, A., Ruiz-Mallén, I., Diego-Feliu, M., Rodellas, V. Bruach-Menchén, J. M., and Garcia-Orellana, J.: The social implications of Submarine Groundwater Discharge from an Ecosystem Services perspective: A systematic review, Earth Sci. Rev., 103742, https://doi.org/10.1016/j.earscirev.2021.103742, in press, 2021.
Anderson, M. P.: Heat as a ground water tracer, Ground Water, 43, 951–968, https://doi.org/10.1111/j.1745-6584.2005.00052.x, 2005.
Andreo, B. and Carrasco, F.: Estudio hidrogeológico del entorno de la Cueva de Nerja, in: Geología de la Cueva de Nerja, edited by: Carrasco, F., Trabajos sobre la Cueva de Nerja nº 3, Geología de la Cueva de Nerja, Patronato de la Cueva de Nerja, Málaga, 163–187, 1993.
Andrisoa, A., Stieglitz, T. C., Rodellas, V., and Raimbault, P.: Primary production in coastal lagoons supported by groundwater discharge and porewater fluxes inferred from nitrogen and carbon isotope signatures, Mar. Chem., 210, 48–60, https://doi.org/10.1016/j.marchem.2019.03.003, 2019.
Aquilina, L., Ladouche, B., Doerfliger, N., Seidel, J. L., Dupuy, C., and Le Strat, P.: Origin, evolution and residence-time of saline thermal fluids (Balaruc springs, S-France): Implications for large-scale fluid transfer across the continental shelf, Chem. Geol., 192, 1–21, 2002.
Bakalowicz, M.: Karst groundwater: A challenge for new resources, Hydrogeol. J., 13, 148–160, https://doi.org/10.1007/s10040-004-0402-9, 2005.
Bakalowicz, M.: Karst and karst groundwater resources in the Mediterranean, Environ. Earth Sci., 74, 5–14, https://doi.org/10.1007/s12665-015-4239-4, 2015.
Bakalowicz, M.: Coastal Karst groundwater in the mediterranean: A resource to be preferably exploited onshore, not from Karst Submarine springs, Geoscience, 8, 258, https://doi.org/10.3390/geosciences8070258, 2018.
Barberá, J. A. and Andreo, B.: Hydrogeological processes in a fluviokarstic area inferred from the analysis of natural hydrogeochemical tracers. The case study of eastern Serranía de Ronda (S Spain), J. Hydrol., 523, 500–514, https://doi.org/10.1016/j.jhydrol.2015.01.080, 2015.
Barsi, J. A., Barker, J. L., and Schott, J. R.: An Atmospheric Correction Parameter Calculator for a Single Thermal Band Earth-Sensing Instrument, Int. Geosci. Remote Sens. Symp., 5, 3014–3016, https://doi.org/10.1109/igarss.2003.1294665, 2003.
Basterretxea, G., Tovar-Sanchez, A., Beck, A. J., Masqué, P., Bokuniewicz, H. J., Coffey, R., Duarte, C. M., Garcia-Orellana, J., Garcia-Solsona, E., Martinez-Ribes, L., and Vaquer-Sunyer, R.: Submarine groundwater discharge to the coastal environment of a Mediterranean island (Majorca, Spain): Ecosystem and biogeochemical significance, Ecosystems, 13, 629–643, https://doi.org/10.1007/s10021-010-9334-5, 2010.
Bayari, C. S. and Kurttaş, T.: Coastal and submarine karstic discharges in the Gökova Bay, SW Turkey, Q. J. Eng. Geol. Hydrogeol., 35, 381–390, https://doi.org/10.1144/1470-9236/01034, 2002.
Bejannin, S., van Beek, P., Stieglitz, T., Souhaut, M., and Tamborski, J.: Combining airborne thermal infrared images and radium isotopes to study submarine groundwater discharge along the French Mediterranean coastline, J. Hydrol.: Reg. Stud., 13, 72–90, https://doi.org/10.1016/j.ejrh.2017.08.001, 2017.
Boehm, A. B., Shellenbarger, G. G., and Paytan, A.: Groundwater discharge: Potential association with fecal indicator bacteria in the surf zone, Environ. Sci. Technol., 38, 3558–3566, https://doi.org/10.1021/es035385a, 2004.
Brunner, P., Hendricks Franssen, H. J., Kgotlhang, L., Bauer-Gottwein, P., and Kinzelbach, W.: How can remote sensing contribute in groundwater modeling?, Hydrogeol. J., 15, 5–18, https://doi.org/10.1007/s10040-006-0127-z, 2007.
Burnett, W. C. and Dulaiova, H.: Estimating the dynamics of groundwater input into the coastal zone via continuous radon-222 measurements, J. Environ. Radioact., 69, 21–35, https://doi.org/10.1016/S0265-931X(03)00084-5, 2003.
Chander, G., Markham, B. L., and Helder, D. L.: Summary of current radiometric calibration coefficients for Landsat MSS, TM, ETM+, and EO-1 ALI sensors, Remote Sens. Environ., 113, 893–903, https://doi.org/10.1016/j.rse.2009.01.007, 2009.
Dale, R. K. and Miller, D. C.: Spatial and temporal patterns of salinity and temperature at an intertidal groundwater seep, Estuar. Coast. Shelf Sci., 72, 283–298, https://doi.org/10.1016/j.ecss.2006.10.024, 2007.
Danielescu, S., MacQuarrie, K., and Faux, R.: The integration of thermal infrared imaging, discharge measurements and numerical simulation to quantify the relative contributions of freshwater inflows to small estuaries in Atlantic Canadae, Hydrol. Process., 23, 2847–2859, 2009.
DiGiacomo, P. M., Washburn, L., Holt, B., and Jones, B. H.: Coastal pollution hazards in southern California observed by SAR imagery: Stormwater plumes, wastewater plumes, and natural hydrocarbon seeps, Mar. Pollut. Bull., 49, 1013–1024, https://doi.org/10.1016/j.marpolbul.2004.07.016, 2004.
Donlon, C. J., Minnett, P. J., Gentemann, C., Nightingale, T. J., Barton, I. J., Ward, B., and Murray, M. J.: Toward Improved Validation of Satellite Sea Surface Skin Temperature Measurements for Climate Research, J. Climate, 15, 353–369, https://doi.org/10.1175/1520-0442(2002)015<0353:TIVOSS>2.0.CO;2, 2002.
Edet, A. E., Okereke, C. S., Teme, S. C., and Esu, E. O.: Application of remote-sensing data to groundwater exploration: A case study of the Cross River State, southeastern Nigeria, Hydrogeol. J., 6, 394–404, https://doi.org/10.1007/s100400050162, 1998.
Elhatip, H.: The use of hydrochemical techniques to estimate the discharge of Ovac1k submarine springs on the Mediterranean coast of Turkey, Environ. Geol., 43, 714–719, https://doi.org/10.1007/s00254-002-0668-y, 2003.
Fleury, P., Bakalowicz, M., and de Marsily, G.: Submarine springs and coastal karst aquifers: A review, J. Hydrol., 339, 79–92, https://doi.org/10.1016/j.jhydrol.2007.03.009, 2007.
Garcés, E., Basterretxea, G., and Tovar-Sánchez, A.: Changes in microbial communities in response to submarine groundwater input, Mar. Ecol. Prog. Ser., 438, 47–58, https://doi.org/10.3354/meps09311, 2011.
Garcia-Orellana, J., López-Castillo, E., Casacuberta, N., Rodellas, V., Masqué, P., Carmona-Catot, G., Vilarrasa, M., and García-Berthou, E.: Influence of submarine groundwater discharge on 210Po and 210Pb bioaccumulation in fish tissues, J. Environ. Radioact., 155–156, 46–54, https://doi.org/10.1016/j.jenvrad.2016.02.005, 2016.
Garcia-Orellana, J., Rodellas, V., Tamborski, J., Diego-Feliu, M., van Beek, P., Weinstein, Y., Charette, M., Alorda-Kleinglass, A., Michael, H. A., Stieglitz, T., and Scholten, J.: Radium isotopes as submarine groundwater discharge (SGD) tracers: Review and recommendations, Earth-Sci. Rev., 220, 103681, https://doi.org/10.1016/j.earscirev.2021.103681, 2021.
Garcia-Solsona, E., Garcia-Orellana, J., Masqué, P., Garcés, E., Radakovitch, O., Mayer, A., Estradé, S., and Basterretxea, G.: An assessment of karstic submarine groundwater and associated nutrient discharge to a Mediterranean coastal area (Balearic Islands, Spain) using radium isotopes, Biogeochemistry, 97, 211–229, https://doi.org/10.1007/s10533-009-9368-y, 2010.
Gerace, A. and Montanaro, M.: Remote Sensing of Environment Derivation and validation of the stray light correction algorithm for the thermal infrared sensor onboard Landsat 8, Remote Sens. Environ., 191, 246–257, https://doi.org/10.1016/j.rse.2017.01.029, 2017.
Gierach, M. M., Holt, B., Trinh, R., Jack Pan, B., and Rains, C.: Satellite detection of wastewater diversion plumes in Southern California, Estuar. Coast. Shelf Sci., 186, 171–182, https://doi.org/10.1016/j.ecss.2016.10.012, 2017.
Gumma, M. K. and Pavelic, P.: Mapping of groundwater potential zones across Ghana using remote sensing, geographic information systems, and spatial modeling, Environ. Monit. Assess., 185, 3561–3579, https://doi.org/10.1007/s10661-012-2810-y, 2013.
Kelly, J. L., Glenn, C. R., and Lucey, P. G.: High-resolution aerial infrared mapping of groundwater discharge to the coastal ocean, Limnol. Oceanogr. Meth., 11, 262–277, https://doi.org/10.4319/lom.2013.11.262, 2013.
Krest, J. M., Moore, W. S., Gardner, L. R., and Morris, J. T.: Marsh nutrient export supplied by groundwater discharge: Evidence from radium measurements, Global Biogeochem. Cy., 14, 167–176, https://doi.org/10.1029/1999GB001197, 2000.
Lee, E., Kang, K. M., Hyun, S. P., Lee, K. Y., Yoon, H., Kim, S. H., Kim, Y., Xu, Z., Kim, D. J., Koh, D. C., and Ha, K.: Submarine groundwater discharge revealed by aerial thermal infrared imagery: a case study on Jeju Island, Korea, Hydrol. Process., 30, 3494–3506, https://doi.org/10.1002/hyp.10868, 2016.
Luijendijk, E., Gleeson, T., and Moosdorf, N.: Fresh groundwater discharge insignificant for the world's oceans but important for coastal ecosystems, Nat. Commun., 11, 1260, https://doi.org/10.1038/s41467-020-15064-8, 2020.
Mallast, U., Siebert, C., Wagner, B., Sauter, M., Gloaguen, R., Geyer, S., and Merz, R.: Localisation and temporal variability of groundwater discharge into the Dead Sea using thermal satellite data, Environ. Earth Sci., 69, 587–603, https://doi.org/10.1007/s12665-013-2371-6, 2013.
Mallast, U., Gloaguen, R., Friesen, J., Rödiger, T., Geyer, S., Merz, R., and Siebert, C.: How to identify groundwater-caused thermal anomalies in lakes based on multi-temporal satellite data in semi-arid regions, Hydrol. Earth Syst. Sci., 18, 2773–2787, https://doi.org/10.5194/hess-18-2773-2014, 2014.
McCaul, M., Barland, J., Cleary, J., Cahalane, C., McCarthy, T., and Diamond, D.: Combining remote temperature sensing with in-situ sensing to track marine/freshwater mixing dynamics, Sensors, 16, 1402, https://doi.org/10.3390/s16091402, 2016.
Mejías, M., Ballesteros, B. J., Antón-Pacheco, C., Domínguez, J. A., Garcia-Orellana, J., Garcia-Solsona, E., and Masqué, P.: Methodological study of submarine groundwater discharge from a karstic aquifer in the Western Mediterranean Sea, J. Hydrol., 464–465, 27–40, https://doi.org/10.1016/j.jhydrol.2012.06.020, 2012.
Michael, H. A., Mulligan, A. E., and Harvey, C. F.: Seasonal oscillations in water exchange between aquifers and the coastal ocean, Nature, 436, 1145–1148, https://doi.org/10.1038/nature03935, 2005.
Moore, W. S.: The subterranean estuary: A reaction zone of ground water and sea water, Mar. Chem., 65, 111–125, https://doi.org/10.1016/S0304-4203(99)00014-6, 1999.
Moore, W. S.: The Effect of Submarine Groundwater Discharge on the Ocean, Annu. Rev. Mar. Sci., 2, 59–88, https://doi.org/10.1146/annurev-marine-120308-081019, 2010.
Moosdorf, N., Böttcher, M. E., Adyasari, D., Erkul, E., Gilfedder, B. S., Greskowiak, J., Jenner, A.-K., Kotwicki, L., Massmann, G., Müller-Petke, M., Oehler, T., Post, V., Prien, R., Scholten, J., Siemon, B., Ehlert von Ahn, C. M., Walther, M., Waska, H., Wunderlich, T., and Mallast, U.: A State-Of-The-Art Perspective on the Characterization of Subterranean Estuaries at the Regional Scale, Front. Earth Sci., 9, 95, https://doi.org/10.3389/feart.2021.601293, 2021.
Povinec, P. P., Aggarwal, P. K., Aureli, A., Burnett, W. C., Kontar, E. A., Kulkarni, K. M., Moore, W. S., Rajar, R., Taniguchi, M., Comanducci, J. F., Cusimano, G., Dulaiova, H., Gatto, L., Groening, M., Hauser, S., Levy-Palomo, I., Oregioni, B., Ozorovich, Y. R., Privitera, A. M. G., and Schiavo, M. A.: Characterisation of submarine groundwater discharge offshore south-eastern Sicily, J. Environ. Radioact., 89, 81–101, https://doi.org/10.1016/j.jenvrad.2006.03.008, 2006.
Rocha, C., Robinson, C. E., Santos, I. R., Waska, H., Michael, H. A., and Bokuniewicz, H. J.: A place for subterranean estuaries in the coastal zone, Estuar. Coast. Shelf Sci., 250, 107167, https://doi.org/10.1016/j.ecss.2021.107167, 2021.
Rodellas, V., Garcia-Orellana, J., Tovar-Sánchez, A., Basterretxea, G., López-Garcia, J. M., Sánchez-Quiles, D., Garcia-Solsona, E., and Masqué, P.: Submarine groundwater discharge as a source of nutrients and trace metals in a Mediterranean bay (Palma Beach, Balearic Islands), Mar. Chem., 160, 56–66, https://doi.org/10.1016/j.marchem.2014.01.007, 2014.
Rodellas, V., Garcia-Orellana, J., Masqué, P., Feldman, M., Weinstein, Y., and Boyle, E. A.: Submarine groundwater discharge as a major source of nutrients to the Mediterranean Sea, P. Natl. Acad. Sci. USA, 112, 3926–3930, https://doi.org/10.1073/pnas.1419049112, 2015.
Rosenberry, D. O., Duque, C., and Lee, D. R.: History and evolution of seepage meters for quantifying flow between groundwater and surface water: Part 1 – Freshwater settings, Earth-Sci. Rev., 204, 103167, https://doi.org/10.1016/j.earscirev.2020.103167, 2020.
Roy, D. P., Wulder, M. A., Loveland, T. R., C.E., W., Allen, R. G., Anderson, M. C., Helder, D., Irons, J. R., Johnson, D. M., Kennedy, R., Scambos, T. A., Schaaf, C. B., Schott, J. R., Sheng, Y., Vermote, E. F., Belward, A. S., Bindschadler, R., Cohen, W. B., Gao, F., Hipple, J. D., Hostert, P., Huntington, J., Justice, C. O., Kilic, A., Kovalskyy, V., Lee, Z. P., Lymburner, L., Masek, J. G., McCorkel, J., Shuai, Y., Trezza, R., Vogelmann, J., Wynne, R. H., and Zhu, Z.: Landsat-8: Science and product vision for terrestrial global change research, Remote Sens. Environ., 145, 154–172, https://doi.org/10.1016/j.rse.2014.02.001, 2014.
Ruiz-González, C., Rodellas, V., and Garcia-Orellana, J.: The microbial dimension of submarine groundwater discharge: current challenges and future directions, FEMS Microbiol. Rev., fuab010, 1–25, https://doi.org/10.1093/femsre/fuab010, 2021.
Schubert, M., Scholten, J., Schmidt, A., Comanducci, J. F., Pham, M. K., Mallast, U., and Knoeller, K.: Submarine groundwater discharge at a single spot location: Evaluation of different detection approaches, Water, 6, 584–601, https://doi.org/10.3390/w6030584, 2014.
Shaban, A., Khawlie, M., Abdallah, C., and Faour, G.: Geologic controls of submarine groundwater discharge: Application of remote sensing to north Lebanon, Environ. Geol., 47, 512–522, https://doi.org/10.1007/s00254-004-1172-3, 2005.
Tamborski, J., van Beek, P., Conan, P., Pujo-Pay, M., Odobel, C., Ghiglione, J. F., Seidel, J. L., Arfib, B., Diego-Feliu, M., Garcia-Orellana, J., Szafran, A., and Souhaut, M.: Submarine karstic springs as a source of nutrients and bioactive trace metals for the oligotrophic Northwest Mediterranean Sea, Sci. Total Environ., 732, 1–14, https://doi.org/10.1016/j.scitotenv.2020.139106, 2020.
Tamborski, J. J., Rogers, A. D., Bokuniewicz, H. J., Cochran, J. K., and Young, C. R.: Identification and quantification of diffuse fresh submarine groundwater discharge via airborne thermal infrared remote sensing, Remote Sens. Environ., 171, 202–217, https://doi.org/10.1016/j.rse.2015.10.010, 2015.
Taniguchi, M., Dulai, H., Burnett, K. M., Santos, I. R., Sugimoto, R., Stieglitz, T., Kim, G., Moosdorf, N., and Burnett, W. C.: Submarine Groundwater Discharge: Updates on Its Measurement Techniques, Geophysical Drivers, Magnitudes, and Effects, Front. Environ. Sci., 7, 1–26, https://doi.org/10.3389/fenvs.2019.00141, 2019.
Tcherepanov, E. N., Zlotnik, V. A., and Henebry, G. M.: Using Landsat thermal imagery and GIS for identification of groundwater discharge into shallow groundwater-dominated lakes, Int. J. Remote Sens., 26, 3649–3661, https://doi.org/10.1080/01431160500177315, 2005.
Trezzi, G., Garcia-Orellana, J., Rodellas, V., Santos-Echeandia, J., Tovar-Sánchez, A., Garcia-Solsona, E., and Maqué, P.: Submarine groundwater discharge: a significant source of dissolved trace metals to the North Western Mediterranean Sea. Marine Chemistry, Mar. Chem., 186, 90–100, https://doi.org/10.1016/j.marchem.2016.08.004, 2016.
Trezzi, G., Garcia-Orellana, J., Rodellas, V., Masqué, P., Garcia-Solsona, E., and Andersson, P. S.: Assessing the role of submarine groundwater discharge as a source of Sr to the Mediterranean Sea, Geochim. Cosmochim. Ac., 200, 42–54, https://doi.org/10.1016/j.gca.2016.12.005, 2017.
Tulipano, L., Panagopoulus, A., and Fidelibus, M. D.: Cost Action 621 “Groundwater management of coastal karstic aquifers”, Final Report, EU Publications Office (OPOCE), 366, 2005.
U.S. Geological Survey: Landsat 8 (L8) Operational Land Imager (OLI) and Thermal Infrared Sensor (TIRS): Calibration Notices, available at: https://www.usgs.gov/core-science-systems/nli/landsat/landsat-8-oli-and-tirs-calibration-notices (last access: 15 February 2019), 2014.
Varma, S., Turner, J., and Underschultz, J.: Estimation of submarine groundwater discharge into Geographe Bay, Bunbury, Western Australia, J. Geochem. Explor., 106, 197–210, https://doi.org/10.1016/j.gexplo.2010.02.003, 2010.
Wang, L. T., McKenna, T. E., and Deliberty, T. L.: Locating Ground-Water Discharge Areas In Rehoboth And Indian River Bays And Indian River, Delaware Using Landsat 7 Imagery, available at: http://udspace.udel.edu/handle/19716/3174 (last access: 20 October 2020), 2008.
Wen-Yao, L., Field, R. T., Gantt, R. G., and Klemas, V.: Measurement of the Surface Emissivity, Remote Sens. Environ., 5, 97–109, https://doi.org/10.1016/0034-4257(87)90009-5, 1987.
Werner, A. D., Bakker, M., Post, V. E. A., Vandenbohede, A., Lu, C., Ataie-Ashtiani, B., Simmons, C. T., and Barry, D. A.: Seawater intrusion processes, investigation and management: Recent advances and future challenges, Adv. Water Resour., 51, 3–26, https://doi.org/10.1016/j.advwatres.2012.03.004, 2013.
Wilson, J. and Rocha, C.: Regional scale assessment of Submarine Groundwater Discharge in Ireland combining medium resolution satellite imagery and geochemical tracing techniques, Remote Sens. Environ., 119, 21–34, https://doi.org/10.1016/j.rse.2011.11.018, 2012.
Wloczyk, C., Richter, R., Borg, E., and Nueberts, W.: Sea and lake surface temperature retrieval from Landsat thermal data in Northern Germany, Int. J. Remote Sens., 27, 2489–2502, https://doi.org/10.1080/01431160500300206, 2006.
Worthington, S. R. H.: A comprehensive strategy for understanding flow in carbonate aquifers, Karst Model., 5, 30–37, 1999.
Wulder, M. A., Loveland, T. R., Roy, D. P., Crawford, C. J., Masek, J. G., Woodcock, C. E., Allen, R. G., Anderson, M. C., Belward, A. S., Cohen, W. B., Dwyer, J., Erb, A., Gao, F., Griffiths, P., Helder, D., Hermosilla, T., Hipple, J. D., Hostert, P., Hughes, M. J., Huntington, J., Johnson, D. M., Kennedy, R., Kilic, A., Li, Z., Lymburner, L., McCorkel, J., Pahlevan, N., Scambos, T. A., Schaaf, C., Schott, J. R., Sheng, Y., Storey, J., Vermote, E., Vogelmann, J., White, J. C., Wynne, R. H., and Zhu, Z.: Current status of Landsat program, science, and applications, Remote Sens. Environ., 225, 127–147, https://doi.org/10.1016/j.rse.2019.02.015, 2019.
Xing, Q., Braga, F., Tosi, L., Lou, M., Zaggia, L., Teatini, P., Gao, X., Yu, L., Wen, X., and Shi, P.: Detection of Low Salinity Groundwater Seeping into the Eastern Laizhou Bay (China) with the Aid of Landsat Thermal Data, J. Coast. Res., 74, 149–156, https://doi.org/10.2112/si74-014.1, 2016.
Zektser, I. S., Everett, L. G., and Dzhamalov, R. G.: Submarine Groundwater, CRC Press, Boca Raton, USA, 2006.