the Creative Commons Attribution 4.0 License.
the Creative Commons Attribution 4.0 License.
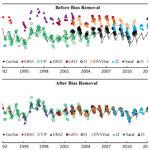
Evaluation of historic and operational satellite radar altimetry missions for constructing consistent long-term lake water level records
Song Shu
Hongxing Liu
Richard A. Beck
Frédéric Frappart
Johanna Korhonen
Minxuan Lan
Min Xu
Bo Yang
Yan Huang
A total of 13 satellite missions have been launched since 1985, with different types of radar altimeters on board. This study intends to make a comprehensive evaluation of historic and currently operational satellite radar altimetry missions for lake water level retrieval over the same set of lakes and to develop a strategy for constructing consistent long-term water level records for inland lakes at global scale. The lake water level estimates produced by different retracking algorithms (retrackers) of the satellite missions were compared with the gauge measurements over 12 lakes in four countries. The performance of each retracker was assessed in terms of the data missing rate, the correlation coefficient r, the bias, and the root mean square error (RMSE) between the altimetry-derived lake water level estimates and the concurrent gauge measurements. The results show that the model-free retrackers (e.g., OCOG/Ice-1/Ice) outperform the model-based retrackers for most of the missions, particularly over small lakes. Among the satellite altimetry missions, Sentinel-3 gave the best results, followed by SARAL. ENVISAT has slightly better lake water level estimates than Jason-1 and Jason-2, but its data missing rate is higher. For small lakes, ERS-1 and ERS-2 missions provided more accurate lake water level estimates than the TOPEX/Poseidon mission. In contrast, for large lakes, TOPEX/Poseidon is a better option due to its lower data missing rate and shorter repeat cycle. GeoSat and GeoSat Follow-On (GFO) both have an extremely high data missing rate of lake water level estimates. Although several contemporary radar altimetry missions provide more accurate lake level estimates than GFO, GeoSat was the sole radar altimetry mission, between 1985 and 1990, that provided the lake water level estimates. With a full consideration of the performance and the operational duration, the best strategy for constructing long-term lake water level records should be a two-step bias correction and normalization procedure. In the first step, use Jason-2 as the initial reference to estimate the systematic biases with TOPEX/Poseidon, Jason-1, and Jason-3 and then normalize them to form a consistent TOPEX/Poseidon–Jason series. Then, use the TOPEX/Poseidon–Jason series as the reference to estimate and remove systematic biases with other radar altimetry missions to construct consistent long-term lake water level series for ungauged lakes.
- Article
(4349 KB) - Full-text XML
- BibTeX
- EndNote
About 3 % percent of the Earth's land surface is covered by lakes (Pekel et al., 2016). These lakes are the habitats for a great number of aquatic and terrestrial species (Schindler and Scheuerell, 2002). They are also the major freshwater sources for various human activities (Postel et al., 1996). The long-term variations in lake water levels were identified as sentinel for climate change (Adrian et al., 2009; Williamson et al., 2009). The lake water level change can also have significant influences on the local ecosystem and environment, for example, the breeding success of fish (Probst et al., 2009), the drainage of thaw lakes (Pohl et al., 2009; Marsh et al., 2009; Jones and Arp, 2015), and landslide at lake coastal areas (Tyszkowski et al., 2015). Monitoring lake water levels is important for a better understanding of their impact on the environment and for the wise management of freshwater resources.
At present, only a very small portion of lakes are monitored by gauge stations. The number of gauged lakes has decreased in recent years owing to the high cost of installation and maintenance of gauge stations (Hannah et al., 2011). The overwhelming majority of the lakes on Earth remain ungauged, particularly those located in remote areas with harsh environments, for example, the Arctic and the sub-Arctic regions. Many previous studies show that the lakes in these remote areas have been experiencing dramatic changes with regard to the lake water balance (Turner et al., 2014), the timing and magnitude of spring/early summer flooding (Rokaya et al., 2018), and the lake ice cover phenology (Surdu et al., 2014) due to rapid climate warming (Karl et al., 2015). There is an urgent need to develop an alternative approach for the effective monitoring of lake water levels at the global scale.
Satellite radar altimeters make surface elevation measurements by tracking the satellite orbit position and the range between the satellite and the Earth's surface at nadir direction. They have been used widely to monitor lake water levels since the 1980s. A total of 13 satellite missions have been launched with different radar altimeters on board in the past 3 decades. Those include the Geodetic/Geophysical Satellite (GeoSat; 1985–1989; McConathy and Kilgus, 1987) and GeoSat Follow-on (GFO; 1998–2008; Barry et al., 1995) developed by US Navy, ERS-1 (1991–2000; Cheney et al., 1991), ERS-2 (1995–2011; Zandbergen et al., 1997), ENVISAT (2002–2012; Zelli, 1999), CryoSat-2 (2010–present; Wingham et al., 2006) and Sentinel-3 (2016–present; Donlon et al., 2012) developed by ESA (European Space Agency), the Satellite with ARgos and ALtika (SARAL; 2013–present; Verron et al., 2015) developed jointly by CNES (Centre National d'Etudes Spatiales – the French Space Agency) and ISRO (Indian Space Research Organisation), the TOPEX/Poseidon (T/P; 1992–2005; Lee-Lueng, 1994), Jason-1 (2001–2013; Menard et al., 2003), Jason-2 (2008–present; Lambin et al., 2010) and Jason-3 (2016–present) developed jointly by NOAA (National Oceanic and Atmospheric Administration), NASA (National Aeronautics and Space Administration), CNES and EUMETSAT (European Organization for the Exploitation of Meteorological Satellites), and the HY-2A (2011–present) developed by CNSA (China National Space Administration). Most of the radar altimeters operate in a conventional low-resolution mode (LRM), whereas Sentinel-3 and CryoSat-2 operate in synthetic aperture radar (SAR) mode. CryoSat-2 is also able to operate in the interferometric SAR (SARin) mode.
Based on the elevation measurements collected by different satellite radar altimeters, five online databases have also been developed to offer the time series of altimetry-derived water level estimates for major inland lakes around the world. These include the Hydroweb database (http://www.legos.obs-mip.fr/soa/hydrologie/hydroweb/, last access: 15 February 2021) developed by the Laboratoire d'Etudes en Géophysique et Océanographie Spatiales (LEGOS; Crétaux et al., 2011), the River and Lake database (http://www.cse.dmu.ac.uk/EAPRS/products_riverlake.html, last access: 15 February 2021) built by the ESA and De Montfort University (ESA-DMU; Berry et al., 2005), the Global Reservoir and Lake Monitor (GRLM; https://ipad.fas.usda.gov/cropexplorer/global_reservoir/, last access: 15 February 2021) developed by the Foreign Agricultural Service of the United States Department of Agriculture (USDA) (Birkett et al., 2011), the Hydrosat developed by the Institute of Geodesy from the University of Stuttgart (http://hydrosat.gis.uni-stuttgart.de, last access: 15 February 2021), and the Database for Hydrological Time Series over Inland Waters (DAHITI; https://dahiti.dgfi.tum.de/en/, last access: 15 February 2021) launched by the Deutsches Geodätisches Forschungsinstitut der Technischen Universität München (DGFI-TUM) in 2013 (Schwatke et al., 2015b). The time series of water level estimates in these databases are produced by merging the elevation measurements from multiple satellite radar altimeters with different processing strategies (Birkett and Beckley, 2010; Ričko et al., 2012; Schwatke et al., 2015b).
For each satellite radar altimeter, one or more dedicated algorithms have been designed to retrieve the surface elevations. Each algorithm is often designed to handle one type of Earth surface. These radar altimetry algorithms are also known as retracking algorithms or simply retrackers. For example, there are four different retrackers designed for ENVISAT altimeter, including the Ocean retracker for ocean open water surface, the Ice1 retracker for general continental ice sheet surface, the Ice2 retracker for continental internal flat ice surface, and the sea ice retracker for ocean ice surface (Frappart et al., 2006).
Many previous studies have evaluated different satellite radar altimeters in the retrieval of water levels over inland lakes with different sizes and environmental surroundings. Morris (1994) examined the performance of GeoSat over the Great Lakes (Erie, Huron, Michigan, Ontario and Superior), and the root mean square error (RMSE) between the altimetry-derived water level estimates and the gauge measurements ranged from 9.4 to 13.8 cm. Birkett (1995) assessed TOPEX/Poseidon over lakes Ontario, Michigan and Superior, and its RMSE ranged from 4.69 to 6.2 cm. Also, Birkett et al. (2010) evaluated Jason-2 water level estimates against gauge measurements over five lakes. They found that its RMSE was 2.95 cm for Lake Ontario (with an area of ∼20 000 km2) and 33.2 cm for Lake Yellowstone (with an area of ∼350 km2). Frappart et al. (2006) investigated the performance of the four retrackers of ENVISAT over three small lakes (with an area from 100 to 300 km2) near Curuai in Amazon basin. They observed that the Ice1 retracker was the best for retrieving lake water levels with ENVISAT altimetry observations. Jarihani et al. (2013) compared five different satellite radar altimetry missions (ENVISAT, GFO, T/P, Jason-1, and Jason-2) and assessed the performance of different retrackers adopted by these missions over Lake Eildon (138 km2) and Lake Argyle (1000 km2) in Australia. They found out that, among the five missions, Jason-2 gave the best results with a RMSE of 28 cm for Ice1 retracker and 32 cm for MLE3 retracker, while T/P yielded the largest RMSE of 150 cm for its sole Ocean retracker. Schwatke et al. (2015a) evaluated the performance of ENVISAT and SARAL over the Great Lakes and found that both missions can achieve very low RMSE, ranging from 2–6 cm for these large lakes. Villadsen et al. (2016) reprocessed CryoSat-2 data with several non-official retrackers and assessed their performance over Lake Vänern (5550 km2) and Lake Okeechobee (1436 km2). They demonstrated that the Multiple Waveform Persistent Peak (MWaPP) retracker produced the lowest RMSE of 9.1 cm over Lake Vänern and 13.4 cm over Lake Okeechobee. Crétaux et al. (2018) evaluated Sentinel-3 and Jason-3 over Lake Issyk-Kul (6236 km2), and found that both missions achieved a very low RMSE of 3 cm with the Ocean retracker. Shu et al. (2020) assessed the performance of the Sentinel-3 SAR retrackers over 15 lakes, and they reported that the SAR Altimetry Mode Studies and Applications-2 (SAMOSA-2) retracker has the lowest mean RMSE of 8.08 cm. Jiang et al. (2020) also evaluated four retrackers (including official and non-official) for Sentinel-3 and demonstrated that the MWaPP+ retracker can significantly improve the accuracy of water level estimates over large rivers.
Apparently, each of those previous evaluations only focused on a few of radar altimetry missions. Those individual evaluations are not strictly comparable, since each study was conducted over a different set of lakes. The differences in lake size, geographic location, surrounding topography, and land cover type could significantly influence the accuracy of lake water levels retrieved by satellite radar altimeters (Maillard et al., 2015).
Despite the previous research efforts, many questions remain as to the construction of a long-term time series of water level for ungauged inland lakes, particularly for those located in remote areas (e.g., the Arctic coastal plains). As described above, each radar altimetry mission spans different time periods and has different levels of measurement accuracy, and there are systematical differences (biases) between different mission measurements. In order to construct a long-term consistent time series of lake water level estimates, the question is which radar altimetry mission can be used as a high-confidence initial reference to remove the biases between missions and to tie different missions together? For a certain time period, one lake may be visited by multiple radar missions. In this case, which satellite radar altimetry mission may provide more reliable lake water level estimates? Most radar altimetry missions have several retrackers that can be used to estimate lake water level. For a given radar altimetry mission, which retracker is most reliable and accurate for lake water level retrieval? The pursuit of answers to these questions entails a comprehensive and consistent evaluation of all radar altimetry missions over the same set of lakes.
In this study, we will examine the performance of all historical and currently operational satellite radar altimetry missions, except for HY-2A and CryoSat-2 missions. HY-2A was excluded from this study because of the difficulty in obtaining its data product (the data are not available online for public access). The exclusion of CryoSat-2 was due to its long repeat cycle orbit that does not allow the production of frequent co-located observations for evaluation. Water level estimates retrieved by different retrackers of the 11 radar altimetry missions will be assessed by using the corresponding gauge measurements on 12 lakes of various sizes distributed across four countries. After this introductory section, we will briefly describe these lakes and the gauge measurements in Sect. 2. In Sect. 3, we will introduce the data sets collected by the 11 satellite radar altimetry missions and the different retrackers adopted by each mission. Then, in Sect. 4, we present the methods for processing the satellite radar altimetry data to determine lake water levels. Next, we evaluate each altimetry mission and its retrackers, in comparison with the gauge measurements in Sect. 5, and discuss the performance of each mission and the relevant issues in integrating different radar altimetry missions to construct consistent long-term time series in Sect. 6. The research findings are summarized in Sect. 7.
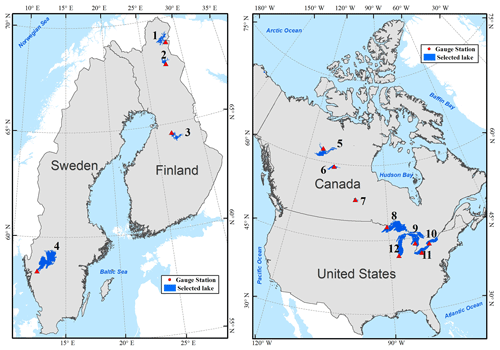
Figure 1Geographic distribution of the case study lakes. Lakes are labeled with an identification number listed in Table 1. This figure is adapted from Fig. 1 in Shu et al. (2020).
2.1 Case study lakes
Our case study sites include 12 lakes/reservoirs in four countries (as shown in Fig. 1). The geographic location, the winter ice condition, and the gauge station for these lakes are summarized in Table 1. The largest one is Lake Superior in North America (over 80 000 km2), while the smallest one is the Lokka reservoir in Finland (about 500 km2). The three lakes in Finland (Inarijärvi, Lokka, and Oulujärvi) and Lake Cedar in Canada all have numerous islands scattered within the lake, fragmenting the water surfaces of these lakes. Therefore, the surface condition of these lakes is very similar to small lakes, over which the satellite radar altimetry signal is contaminated easily by the surrounding land surfaces. These lakes are treated as small lakes to evaluate the performance of each satellite altimetry mission in contrast to the large lakes (e.g., the Great Lakes, Great Slave Lake, and Lake Vänern). The boundary polygons of these 12 lakes were obtained from the Global Lakes and Wetland Databases (GLWD; Lehner and Döll, 2004). The lake polygons were then used to extract measurements from each mission in the subsequent analysis.
A majority of the lakes on Earth are located between 45 and 75∘ N (Verpoorter et al., 2014). Those lakes have varying ice cover conditions in winter seasons, due to the differences in their latitudes and local climates. Among the selected case study lakes, Lake Inarijärvi in Finland is the northernmost, with a latitude of 69.02∘, and Lake Erie is the southernmost, with a latitude of 42.16∘. The three lakes in Finland and the three lakes in Canada are fully ice covered in winter seasons. The ice cover usually lasts more than 7 months for Lake Inarijärvi (Korhonen, 2006) and more than 5 months for Great Slave Lake (Howell et al., 2009). The duration of ice cover decreases for the lakes at more southern locations. In comparison with Canadian lakes, the ice cover on Finnish lakes is often much thinner (Shu et al., 2020) due to the heating effect of the North Atlantic Current (Rahmstorf, 2003; Korhonen, 2019).
Lake Vänern in Sweden and the Great Lakes of North America could be fully covered, partly covered, or totally free from ice in winter seasons, depending on the winter air temperature. Lake Vänern often remains completely ice free in winter. From 1979 to 2002, it was only covered by ice in nine winters (Weyhenmeyer et al., 2008). In a cold winter, Lake Superior and Lake Erie are often fully covered by ice, and the other three (Huron, Ontario, and Michigan) Great Lakes are partly covered (Assel and Wang, 2017), while in warmer winters all of them are partly covered.
2.2 Gauge data
In situ water level measurements for the 12 lakes were collected, respectively, at the gauge stations listed in Table 1, which are obtained from four online databases. Those include the Finnish Environment Information Management System – Hertta – operated by Finnish Environment Institute (SYKE) (http://www.syke.fi/fi-FI/Avoin_tieto/Ymparistotietojarjestelmat, last access: 15 February 2021), the SMHI (Swedish Meteorological and Hydrological Institute; http://vattenwebb.smhi.se/station/, last access: 15 February 2021), Canada Real-time Hydrometric Data (https://wateroffice.ec.gc.ca/mainmenu/real_time_data_index_e.html, last access: 15 February 2021), and the Center for Operational Oceanographic Products and Services (https://tidesandcurrents.noaa.gov/, last access: 15 February 2021) operated by NOAA. These gauge stations measure the water-equivalent lake levels when the lake is ice covered (Shu et al., 2020). Note that the gauge data are referenced to different datum. In this study, only the gauge data on the Great Lakes are converted to EGM2008 using the tool VDatum (https://vdatum.noaa.gov/, last access: 15 February 2021).
In this study, we evaluate the performance of radar altimeters on board 11 satellite missions. Those include all historical and currently operational satellite radar altimetry missions, except for HY-2A and CryoSat-2. No data are available from the HY-2A mission launched by China. CryoSat-2 operates on a long-term repeat orbit (369 d) in order to obtain spatially dense coverage in polar regions, and it is difficult to form frequent time series of co-located water level observations for inland lakes. Most of the altimetry data products of the 11 satellite radar altimetry missions have gone through several rounds of updating and refinements. We used the most up-to-date version of the data product of each mission for the evaluation. The geographical coverage, operational time period, repeat cycle, sampling rate, and retrackers of these radar altimetry missions are summarized in Table 2. The temporal coverage and the overlapping time periods of the 11 missions are illustrated in Fig. 2.
Satellite radar altimeters measure elevation through transmitting radar signal pulses to the nadir surface and timing the echoes. The transmitted and echoed radar pulse is sampled as pulse strength over the elapsed time, which is known as radar altimetry waveform. Most of the 11 missions (except for GeoSat, TOPEX/Poseidon, and GFO) adopted two or more retracking algorithms (retrackers) to process the echoed waveforms in order to produce accurate elevation measurements for different types of Earth surfaces. These retrackers can be divided into two general categories, namely the empirical/model-free retrackers and the physical/model-based retrackers. The model-based retrackers fit a physically based model to the echoed waveform to produce elevation measurements. For example, the ENVISAT Ocean retracker is based on the Brown (1977) model and the Sentinel-3 ice sheet retracker is based on a five-part piecewise analytical function (MSSL/UCL/CLS, 2019). The model-free retrackers have no assumption on the model of the echoed waveform, and the examples include the offset center of gravity (OCOG, also known as Ice1 or ice) developed by Wingham (1986) and the sea ice retracker developed by Laxon (1994). There are also many efficient non-official retrackers (model based or model free) developed in previous studies for different surface conditions (Jiang et al., 2020). In this study, we only focus on the official retrackers that were adopted by each mission to generate the official data products.
A total of 10 of the 11 missions (except for Sentinel-3) utilize the conventional pulse-limited altimeter to measure surface elevation. The diameter of the radar pulse footprint on the Earth's surface varies from 1.6 to 13.4 km, according to the satellite orbit, the echoing surface roughness, and the duration of radar pulse (Chelton et al., 1989). Among the 10 conventional pulse-limited altimetry missions, SARAL utilizes a Ka band (35.75 GHz) as the primary band, with a bandwidth of 480 MHz, to measure the Earth's surface elevation, while the others use a Ku band (e.g., 13.6 GHz) as the primary band, with a bandwidth of 320 MHz. Due to the adoption of the Ka band and the higher bandwidth, the footprint generated by SARAL is about 0.8 times smaller than the other Ku-band altimeters for a given pulse length and orbit altitude (Raney and Phalippou, 2011). Sentinel-3 uses a synthetic aperture radar (SAR) altimeter to measure the Earth's surface elevation. This SAR altimetry technology decreases the along-track footprint size from several kilometers to about 300 m, which improves the retrieval of elevation information over more variable surfaces, e.g., coastal areas (Donlon et al., 2012).
GeoSat was launched on 12 March 1985 by the US Navy, and its operations consisted of two distinct mission phases, namely the Geodetic Mission (GM) and the Exact Repeat Mission (ERM; McConathy and Kilgus, 1987). The GM phase lasted about 18 months, from 31 March 1985 to 30 September 1986, and the ERM phase lasted about 3.5 years, from 8 November 1986 to January 1990. In the GM phase, the satellite operated on a geodetic drifting orbit, while in the ERM phase, it operated on an exact repeat orbit, with a repeat cycle of 17 d. In both phases, the satellite collected elevation measurements of the Earth's surface between 72∘ N and 72∘ S latitudes. GeoSat used a single ocean retracker, based on the Brown (1977) model, to produce elevation measurements for all different types of the Earth's surface (Lillibridge et al., 2006). The georeferenced measurements were originally provided at a 1 Hz rate by the National Centers for Environmental Information (NCEI) at NOAA (https://accession.nodc.noaa.gov/0053056, last access: 15 February 2021). For this study, we obtained GeoSat data from the Radar Altimeter Database System (RADS; Scharroo et al., 2013). RADS provides the most up-to-date harmonized geophysical and systematic corrections for all the satellite radar altimeters. The limitation of RADS is that all the data are provided only at 1 Hz rate. Since the original georeferenced data were also at the 1 Hz rate, the RADS GeoSat data product, instead of the NOAA/NCEI product, was therefore chosen for the evaluation. At the 1 Hz data rate, the sampling interval along the satellite track is 6–7 km, depending on the latitude. GeoSat Follow-On (GFO) was launched on 10 February 1998 and ended on 22 October 2008. Since it was a follow-on mission of GeoSat, it retained the GeoSat ERM orbit with a repeat cycle of 17 d and covered Earth's surface between 72∘ N and 72∘ S latitudes along the satellite ground tracks (Naval Oceanographic Office and NOAA Laboratory for Satellite Altimetry, 2002). The elevation measurements were produced by the same retracking algorithm used for GeoSat. The georeferenced data were provided at a 10 Hz rate and distributed by US Navy and NOAA at https://accession.nodc.noaa.gov/0085960 (last access: 15 February 2021). With the 10 Hz sampling rate, the distance between two adjacent measurements is about 700 m.
ERS-1 and ERS-2 were launched by ESA on 17 July 1991 and 21 April 1995, and retired on 10 March 2000 and 5 September 2011, respectively. ERS-2 was the tandem mission of ERS-1 and carried basically the same set of instruments on board ERS-1. ERS-1 had eight mission phases (Phase A, B, R, C, D, E, F, and G) with different repeat cycles during its lifetime (http://www.deos.tudelft.nl/ers/phases, last access: 15 February 2021), including the 3 d cycle for the commissioning and the ice phases (phase A, B, and D), the 35 d cycle for the nominal observation phase (phase R, C, and G), and the 168 d cycle for the geodetic drifting phases (phase E and F). ERS-2 had two phases, namely the 35 d nominal observation phase (from 29 April 1995 to 21 February 2011) and the 3 d phase (from 10 March to 6 July 2011). Elevation measurements collected by both missions cover the Earth's surface between 81.5∘ N and 81.5∘ S latitude (Brockley, 2014). After the retirement of ERS-2, the data collected by the two missions between August 1991 and July 2003 were reprocessed to generate an improved homogeneous long-term data set, which is called the REAPER (the REprocessing of Altimeter Products for ERS) products (Brockley et al., 2017). In the reprocessing, the four retrackers used for ENVISAT (ocean, Ice1, Ice2 and sea ice) were adopted to refine elevation measurements. Ice1 and sea ice are model-free retrackers developed by Wingham (1986) and Laxon et al. (1994). The other two are model-based retrackers. Later, the ERS-2 data were further reprocessed by the Centre de Topographie des Océans et de l'Hydrosphère (CTOH) at the Laboratoire d'Etudes en Géophysique et Océanographie Spatiales (LEGOS; Frappart et al., 2016). The CTOH ERS-2 product contains elevation measurements generated by two retrackers, i.e., Ice1 and Ice2. In this study, we chose the ERS-1 REAPER data product from ESA (https://earth.esa.int/, last access: 15 February 2021) and the further improved ERS-2 data product from CTOH (http://ctoh.legos.obs-mip.fr/, last access: 15 February 2021) for the evaluation. Both products provide georeferenced elevation measurements at a 20 Hz rate. At this data rate, the distance between two adjacent measurements along the satellite track is about 350 m.
ENVISAT was launched on 28 February 2002, as the successor to ERS-1 and ERS-2. In the nominal observation phase, ENVISAT operated on the same orbit as ERS-1 and ERS-2, with a 35 d repeat cycle from 2002 to 2010. In October 2010, it was maneuvered to a new orbit, with a repeat cycle of 30 d, to extend its mission lifetime until 8 April 2012. This new phase is referred to as the extension phase. In both phases, the elevation measurements were provided at an 18 Hz rate, with a sampling interval of about 370 m along the satellite ground track. ENVISAT mission used four retrackers (ocean, Ice1, Ice2, and sea ice) to generate elevation measurements for different types of the Earth's surface. In 2018, the ENVISAT altimetry data were reprocessed and released by ESA as the ENVISAT V3 product. We obtained this most recent version 3 product from ESA (https://earth.esa.int/, last access: 15 February 2021) for the evaluation.
SARAL is a joint altimetry mission of CNES (Space Agency of France) and ISRO (Indian Space Research Organisation). It was launched on 25 February 2013 by ISRO and is the first satellite mission with a Ka-band (35.75 GHz) radar altimeter on board (Frappart et al., 2015; Bonnefond et al., 2018). During its exact repetitive phase from the launch to 4 July 2016, SARAL flew on ENVISAT nominal orbit with a 35 d exact repeat cycle. Due to technical issues with the reaction wheels, the repetitive orbit has no longer been maintained since 4 July 2016, and the orbit of the satellite decayed naturally, leading to irregular drifting ground tracks on the Earth's surface. This new phase is known as the SARAL drifting phase (Dibarboure et al., 2018). The four ENVISAT retrackers (Ice1, Ice2, sea ice, and ocean) were adopted by SARAL in the creation of different data products for different types of the Earth's surfaces. The data are provided at a rate of 40 Hz by AVISO+ (Archiving, Validation and Interpretation of Satellite Oceanographic data) at the CNES (https://aviso-data-center.cnes.fr/, last access: 15 February 2021). The distance between two adjacent measurements along the satellite track is about 180 m. In this study, we only evaluated the SARAL data collected in the exact repetitive phase.
TOPEX/Poseidon (T/P), Jason-1, Jason-2, and Jason-3 are four continuous missions that provide long-term consistent altimetry observations of the Earth's surface along the same fixed ground tracks. The operation of each satellite is usually composed of two phases, namely the phase with nominal orbit and the phase with interleaved orbit (Fu et al., 1994). Both orbits have an exact repeat cycle of 10 d and cover Earth's surface between 66∘ N and 66∘ S latitudes. Each satellite in this series firstly flies on the nominal orbit after its launch, and was usually maneuvered to a new orbit a number of months after the launch of its successor satellite. The ground tracks generated by this new orbit phase are on the midway between its nominal ground tracks; hence, the new orbit is referred to as interleaved orbit. The period between the launch of the successor satellite and the maneuver of the predecessor satellite is often called the tandem phase. During this phase, the two satellites fly on the same orbit, separated by 60–70 s (see the Jason-3 product handbook). TOPEX/Poseidon was launched on 10 August 1992 and then maneuvered to the interleaved orbit on 15 August 2002 after the launch of Jason-1 on 7 December 2001. TOPEX/Poseidon was decommissioned on 9 October 2005. The TOPEX/Poseidon data products were generated with their sole Brown-model-based retracker (herein after referred to as the ocean retracker; Rodríguez and Martin, 1994) for all different types of surfaces. In the original TOPEX/Poseidon data products, the geographic coordinates were provided for the 1 Hz elevation measurements. In this study, we utilized the data products created by RADS for the evaluation. The distance between two adjacent 1-Hz measurements along the satellite track is about 6 km. Jason-1 was shifted to the interleaved orbit on 10 February 2009, after the launch of Jason-2 on 20 June 2008. Jason-1 stayed on the interleaved orbit for 3 years until 7 May 2012 when it was adjusted to a geodetic orbit. It was finally decommissioned on 1 July 2013. Jason-2 was transferred to the interleaved orbit on 17 October 2016, after the launch of Jason-3 on 17 January 2016. It maintained the interleaved orbit for 8 months and then transferred to a geodetic orbit on 10 July 2017. It was decommissioned on 1 October 2019. Jason-3 is now operating on the nominal orbit. A total of two retrackers have been used by all three missions to generate elevation measurements, i.e., the Brown-model-based MLE4 retracker for ocean surfaces and the model-free ice retracker (similar to OCOG/Ice1 retracker) for non-ocean surfaces (see the Jason-1, 2, and 3 product handbook for details). Another Brown-model-based retracker MLE3 has also been adopted for Jason-2 and Jason-3. Due to its apparent inferior performance in comparison with MLE4 (Thibaut et al., 2010; Vu et al., 2018), it is not included for our evaluation. All three of these radar altimetry missions provide elevation measurements at a rate of 20 Hz. The ground distance between two adjacent measurements is about 350 m. We obtained the altimetry data products of these three missions from AVISO+ for the evaluation.
The Sentinel-3 mission consists of two identical satellites, i.e., the Sentinel-3A and Sentinel-3B, which were launched on 16 February 2016 and 25 April 2018, respectively. The ground tracks of Sentinel-3B fall exactly in the middle of the ground tracks of Sentinel-3A. In other words, the Sentinel-3B is operated on an interleaved orbit and in parallel with the Sentinel-3A on the nominal orbit. The two orbits have the same 27 d repeat cycle and collect elevation measurements along their ground tracks between 81.35∘ N and 81.35∘ S latitudes (Donlon et al., 2012). Both satellites carry a synthetic aperture radar altimeter instrument (SRAL) for the elevation measurements. The SRAL works primarily on the synthetic aperture radar (SAR) mode with the low resolution mode (LRM) as a backup (https://sentinel.esa.int/web/sentinel/user-guides/sentinel-3-altimetry/resolutions/sampling, last acess: 15 February 2021). A total of four retrackers are used in the SAR mode to produce elevation measurements, including SAR Altimetry Mode Studies and Applications-3 (SAMOSA-2), offset center of gravity (OCOG), sea ice, and ice sheet (MSSL/UCL/CLS, 2019). The OCOG (also known as Ice1) is a model-free retracker developed by Wingham (1986). The other three are model-based fully analytic or semi-analytic retrackers. Due to the high rate of missing data (Shu et al., 2020), the sea ice retracker is not included for the evaluation in this study. The elevation measurements are provided at a rate of 20 Hz. The interval between two adjacent measurements along the satellite track is about 300 m (https://sentinel.esa.int/web/sentinel/user-guides/sentinel-3-altimetry/resolutions/sampling, last acess: 15 February 2021). We obtained the Sentinel-3 altimetry data from the ESA Copernicus Open Access Hub (https://scihub.copernicus.eu/, last acess: 15 February 2021) for the evaluation.
In this study, the altimetry data collected by each mission in geodetic phase (or drifting phase) are not included in the evaluation. In the geodetic phase, the drifting ground tracks do not generate frequent observations for a specific lake to form a time series of water level measurements. In this study, for all the completed missions, only the data collected in their exact repeat phase are used for the evaluation. For instance, the data collected in the ERM phase were used for GeoSat and the data collected in phases R, C, and G were used for ERS-1. In the extension phase of the ENVISAT mission and in the intermittent phases of TOPEX/Poseidon, Jason-1, and Jason-2 missions, the satellites all operated on an exact repeat orbit. Therefore, the data collected in these phases were also included in the evaluation. For the two currently operational missions, i.e., Sentinel-3 and Jason-3, the observations for longer than a full year (including winter and summer) are used for the evaluation, including Jason-3 data between February 2016 and March 2018 and Sentinel-3 data between June 2016 and September 2017.
In addition to the altimeter instrument, most of the 11 satellite missions (except for GeoSat) also carried a passive microwave radiometer (MWR) to simultaneously measure the brightness temperature (referred to as TB) of Earth's surface. The microwave bands adopted by each mission are listed in Table 2.
The method used to determine lake water level from satellite radar altimetry in this study consists of three technical data processing steps. First, the surface elevation measurements are retrieved from altimetry data products of the 11 satellite missions for the 12 case study lakes, and the most recent release of the altimetry data products with the up-to-date geophysical corrections has been used. Second, spurious surface elevation measurements are filtered out through statistical analysis, and the remaining valid surface elevation measurements within a lake are statistically aggregated to determine lake water level at different time points. Third, the ice cover condition is examined using the simultaneous TB measurements from the MWR instruments, and those lake water level estimates during the ice-covered period are excluded in the subsequent accuracy evaluations. To evaluate the performance of each satellite altimeter and its retrackers, three accuracy measures, including the Pearson's correlation coefficient r, the bias and the RMSE, have been calculated by comparing the radar-altimetry-derived lake water level estimates with the corresponding gauge measurements.
4.1 Retrieval of lake surface elevation measurements
Following Crétaux et al. (2017), the surface elevation is determined for each satellite radar altimetry mission according to Eq. (1) as follows:
where hretrk is the surface elevation generated by a retracker, H is the height of satellite orbit, Rretrk is the range between the satellite and the nadir Earth's surface generated by a retracker, ΔRiono, and ΔRwet, and ΔRdry compensate for the delay of the radar pulse due to the ionosphere, the wet troposphere, and the dry troposphere, respectively. ΔRsolidEarth and ΔRpole are for solid Earth tide correction and pole tide correction, and geoid converts the reference surface from ellipsoid to geoid (orthometric height). In this study, the geoid model EGM2008 (Pavlis et al., 2012) is adopted.
Due to the variable nature of the Earth's atmosphere, the three atmospheric components (ΔRiono, ΔRwet, and ΔRdry) have significant influence on the accuracy of altimetry measurements (Fernandes et al., 2014; Fernandes and Lázaro, 2016; Crétaux et al., 2009; Scharroo and Smith, 2010). Many global atmospheric models have been used to quantify the biases induced by the three atmospheric components at different locations and times. For the ionospheric correction (ΔRiono), it has been recommended to use the NIC09 (New Ionosphere Climatology) model for the radar altimetry measurements acquired before September 1998 (Scharroo and Smith, 2010) and to use the GIM (global ionosphere map) model for the measurements acquired after that time (Iijima et al., 1999). For the dry and the wet tropospheric corrections (ΔRdry and ΔRwet), the three most commonly used atmospheric models are produced by the European Centre for Medium-Range Weather Forecasts (ECMWF) and the National Centers for Environmental Prediction (NCEP). Those include the ECMWF model (Miller et al., 2010), the ECMWF Re-Analysis Interim (ERA) model (Dee et al., 2011), and the NCEP model (Caplan et al., 1997). The magnitude of the dry and the wet tropospheric corrections depends linearly on the height of the surface over which the altimetry measurement is made. The higher the surface elevation, the smaller the magnitude of the dry and the wet tropospheric correction terms. The difference between the dry tropospheric corrections computed at the sea surface, with an elevation of 0 m, and at the land surface, with an elevation of 5000 m, could be as high as 1 m (Fernandes et al., 2014). Fernanders and Lázaro (2016) also developed a new algorithm to improve the wet tropospheric corrections that can be applied to different radar altimetry missions. In this study, since we focus mainly on the official data products generated by each satellite mission, we adopted the dry and the wet tropospheric corrections that were contained in the official data products and were computed with the height of the surface where the altimetry measurements were taken. Table 3 lists the version of each altimetry data product and the models of the three atmospheric corrections utilized in this study.
4.2 Statistical determination of lake water levels
The 12 lakes in this study were all overpassed by the 11 satellite radar altimetry missions. The number of each mission's ground tracks on these lakes is determined by the size of the lake and the satellite orbit. The large lakes (e.g., Lake Superior) usually have multiple ground tracks for each mission, while the small lakes (e.g., Lokka reservoir) may have only one ground track for a satellite mission. For a large lake (e.g., the Great Lakes), strong wind, big wave, diurnal tide, geoid undulation, and other factors may significantly influence lake water level at different locations in the lake. The in situ water level measurements from a gauge station may not reflect the actual water level of those ground tracks far away from the gauge station. Thus, the overall RMSE of the altimetry-derived estimates will increase when altimetry observations from distant ground tracks are included for the evaluation (Birkett, 1995). To minimize the possible influence of wind, waves, tide, and other environmental factors for an objective comparison between different satellite missions, we thus select the ground track nearest to the gauge station and exclude distant ground tracks in the performance evaluation, as listed in Table 4. CryoSat-2 uses a geodetic orbit (long-term repeat orbit). It is difficult to form a frequent time series of co-located water level estimates for the evaluation. Although a time series of water level estimates from CryoSat-2 observations can be derived for a large lake by including many different ground tracks, this will inevitably introduce uncertainties to the evaluation due to the factors explained above. This is the reason that we did not include the CryoSat-2 data and the data collected by other satellite missions during their geodetic phases or drifting phases.
Table 4The indices of ground tracks selected for each satellite mission over each lake.
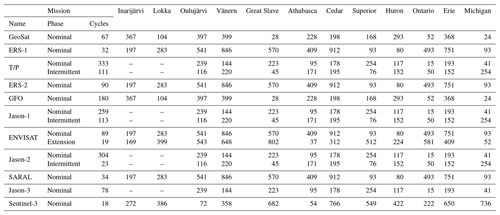
Note that there are no T/P and Jason overpasses for Lake Inarijärvi and Lokka, since their geographic coverage is up to a latitude of 66∘ N.
The total number of completed cycles for each mission depends on its operational lifetime and the temporal length of a repeat cycle. For a mission with a long lifetime and short repeat cycle, the overpass number could be much higher. As listed in Table 4, TOPEX/Poseidon has the highest number of complete cycles (333 in the nominal phase and 111 in the intermittent phase). In each repeat cycle, there is one satellite overpass along the selected ground track for each mission.
Spurious elevation measurements could be generated when the satellite ground track passes over lake islands or when it is close to the lake shore. In particular, the complex surrounding topography could have considerable influences on the elevation measurements over very small lakes (width less than 2 km) or over rivers, when considering the tracking modes (e.g., the open/closed loop of Sentinel-3 and Jason-3) and the receiving window sizes (e.g., the three different window sizes of ENVISAT) of the radar altimeter (Jiang et al., 2020; Biancamaria et al., 2018). The smallest case study lake in our evaluation is the Lokka reservoir in Finland, with a surface area of about 500 km2. For each mission, the ground track over the lake is at least 10 km long. In this study, two steps were adopted to minimize the influences. First, for each satellite overpass during the exact repeating phase, we extracted the surface elevation measurements along a ground track falling within lakes using lake polygons from the GLWD. Considering the footprint size of the radar pulses over relatively homogeneous surface (usually 1–2 km) and the seasonal fluctuation of lake surface area, only elevation measurements over 2 km away from the polygon boundary are selected. Then, the extracted elevation measurements along each ground track were combined to form a surface elevation profile, which was examined to filter out the spurious measurements with the robust median absolute deviation (MAD) statistic (Shu et al., 2018, 2020; Liu et al., 2012). The spurious measurements deviate significantly from the other measurements of the lake surface elevation profile. The MAD method calculates a statistic score for each measurement of the surface elevation profile to indicates its deviation from the rest of the measurements. The higher the score, the stronger deviation is. The measurements with a score value larger than or equal to three are excluded. The median of the remaining elevation measurements along the track is then used as the estimate of the lake water level on the day of each satellite overpass. Finally, the time series of water level estimates were evaluated through comparison with the concurrent gauge measurements.
4.3 Identification of lake level estimates affected by ice cover
Lakes located in a high latitude in this study are more frequently overpassed by satellite missions, but the ice cover on these lakes in the winter season may introduce significant errors to the elevation measurements of satellite altimetry missions. It has been demonstrated that the lake ice cover in winter could have strong influences on the radar altimetry signal pulse, resulting in lower elevation measurements than the real lake surface elevation (Birkett and Beckley, 2010; Ziyad et al., 2020). The mechanism on how lake ice deforms the Sentinel-3 altimetry signal pulse and fails the official waveform retracking algorithms has been investigated in Shu et al. (2020). Shu et al. (2020) also developed a non-official correction algorithm to accurately retrieve the water-equivalent lake levels in ice-covered condition from Sentinel-3 altimetry observations. Since the official retrackers of all the satellite altimetry missions (not only Sentinel-3) are not designed to handle the ice cover on lakes, we identified and excluded the measurements obtained in the ice-covered condition in order to have a fair comparison between different altimetry missions.
In this study, we followed the method in Shu et al. (2020) to examine the ice cover condition for all satellite radar altimetry missions over the case study lakes. In other words, we examine the temporal variations of brightness temperature (TB) over lake surface to detect the lake ice cover. Similar to the pre-processing of radar altimetry surface elevation measurements, we first filter the simultaneous microwave TB measurement profile along the track over a lake. Then, all the remaining valid microwave TB measurements were averaged to represent the temperature for the day of each satellite overpass. The time series curve of TB was then analyzed to determine the dates of ice on and ice off for each winter, indicated by the sudden increase and rapid decrease in TB on the curve. Those radar altimetry measurements collected in the ice-covered condition were identified and then excluded from the subsequent evaluations.
4.4 Accuracy measures for the performance evaluation
The performance of a satellite altimetry mission and its retrackers were evaluated in terms of three accuracy measures as in Shu et al. (2020), including the Pearson's correlation coefficient (r), the bias, and the root mean square error (RMSE). The bias and the RMSE were computed as below.
where n is overpasses along the selected track on a lake, i is the index of an overpass, is the altimetry-derived lake level estimate for satellite overpass i given by a specific retracker, and is the concurrent gauge measurement at the time of overpass i.
These three accuracy measures are computed for each retracker of each mission over each lake. The bias represents the systematic (positive or negative) difference between the series of altimetry-derived estimates and the gauge measurements. If both are referenced to the same vertical datum (e.g., EGM2008), then the smaller the bias, the closer altimetry-derived estimates are to the real lake water level. Since the data of the altimetry-derived water levels and the gauge measurements were consistent only for the Great Lakes, as mentioned in Sect. 2.2, we compared and evaluated the biases of all the retrackers of the 11 missions for these five lakes. The Pearson correlation coefficient r indicates each retracker's capability in depicting lake water level temporal variation. A high r value shows that the retracker captures the lake water level variation very well. Note that the correlation coefficient r is not affected by systematic errors/biases or vertical datum differences. In our evaluation, the RMSE is calculated after the bias of each retracker over each lake was removed (Shu et al., 2020). The RMSE, hence, represents the relative accuracy (precision) of the altimetry-derived lake level estimates. By removing the bias, the inconsistency between the vertical data of the altimetry-derived water levels and the gauge measurements would not affect RMSE values, making all the retrackers over the 12 lakes comparable to each other in terms of RMSE value.
5.1 Radar altimetry-derived lake water level estimates
Figure 3 shows the time series of TB and altimetry-derived water levels over Great Slave Lake collected by ENVISAT, Jason-2, and Sentinel-3 in the winters of 2003–2004, 2011–2012, and 2016–2017, respectively. The ice-covered duration is determined by the sudden increase and the decrease in TB, as indicated by the vertical dashed lines in Fig. 3a–c. The similar temporal variation of TB was also observed for other satellite missions over other lakes when they were covered by ice. As shown in Fig. 3d–f, the lake water level estimates during the ice-covered periods deviate significantly from the gauge measurements, while, during the ice-free seasons, the lake water level estimates correlate very well with the gauge measurements.
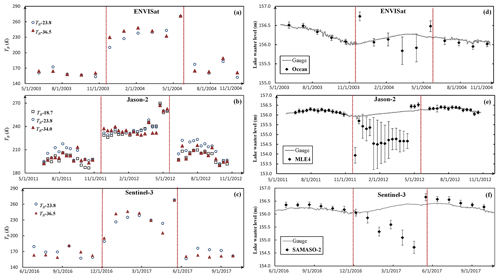
Figure 3Time series of mean brightness temperature (TB) and lake level estimates produced by ENVISAT, Jason-2, and Sentinel-3 over Great Slave Lake in the winter. (a) TB in the 2003–2004 winter, (b) TB in the 2011–2012 winter, (c) TB in the 2016–2017 winter, (d) lake level estimate in the 2003–2004 winter, (e) lake level estimates in the 2011–2012 winter, and (f) lake level estimates in 2016–2017 winter.
Table 5 summarizes the number of lake level estimates during ice-free (open water) and ice-covered seasons over each lake for each retracker of the 11 missions. For some satellite missions, the number of valid lake water level estimates over a certain lake during ice-free season was too small to perform an evaluation. For example, the number of GeoSat estimates over Lake Inarijärvi, Lokka, Lake Oulujärvi, and Lake Cedar are all less than three. Therefore, the evaluation of GeoSat over these lakes was not conducted. As shown in Table 5, the total number of lake water level estimates (sum of the ice-covered number and the ice-free number) for some satellite missions, such as GeoSat and GFO, are considerably smaller than the number of completed orbit cycles due to satellite data loss. The reasons for satellite data loss could be the malfunction of the sensor, the maneuver of the satellite during the phase transition, the failure of the retracker to reach convergence when processing complex waveforms (e.g., multi peaks) from inhomogeneous reflecting surfaces in the altimeter footprint, saturation of the sensor over very bright targets, or the rapid changes in the topography that are larger than the size of tracking window, causing tracking losses (Biancamaria et al., 2017).
Table 5The number of lake water level estimates during ice-free (open water) and ice-covered seasons.
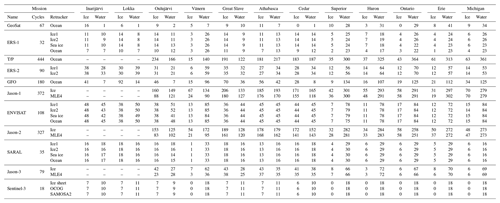
We calculated the data loss rate of lake level estimates over each lake for each retracker of the 11 missions. For each satellite repeat cycle, there is a satellite overpass along the selected ground track on each lake, and there is supposed to be a lake water level estimate if valid surface elevation measurements exist. In this study, the data loss rate of lake level estimates (not the data loss rate of elevation measurements) is calculated through dividing the total number of water level estimates (sum of the ice-covered number and the ice-free number) by the total number of repeat cycles. As shown in Table 6, GeoSat has very high data loss rate for almost all the lakes. The average data loss rate is 65.42 %. There are seven lakes with a loss rate higher than 70 %. In particular, the data loss rate over small lakes is much higher than that for large lakes. The highest data loss rate is 98.51 % over Lake Cedar. The high data loss rate could be partly due to GeoSat's low sampling rate (1 Hz). The other possible reason is the failure of the lock-on to return pulse during transition from land to water, as documented in Sect. 5 of GeoSat user handbook (https://www.nodc.noaa.gov/archive/arc0024/0053056/2.2/about/userhandbook.pdf, last access: 15 February 2021; Cheney, 1997). Similarly, GFO also has very high data loss rate for small lakes. The highest data loss rate is 80 % over Lake Cedar. The high data loss rates of GeoSat and GFO hamper their usefulness for retrieving lake water levels. In contrast, SARAL and Sentinel-3 have a very low data loss rate over both large lakes and small lakes. For ERS-2 and ENVISAT, the data loss rates over small lakes are slightly higher than those over large lakes. Another interesting observation is that, on average, the model-based retrackers have a relatively higher data loss rate than model-free retrackers for all missions. For example, the data loss rates of the MLE4 retracker of Jason-1, Jason-2 and Jason-3 missions are 21.48 %, 16.45 %, and 15.7 %, respectively, which is about twice as high as the loss rates of the ice retracker of these three missions (12.8 %, 6.61 %, and 6.20 %). It suggests that the model-free retrackers are more reliable than model-based retrackers for producing continuous lake water level estimates, confirming the observations of Frappart et al. (2006) and Sulistioadi et al. (2015).
5.2 The biases of altimetry-derived lake water level estimates
We construct a long-term series of lake water levels for each of the 12 lakes, using the altimetry-derived estimates during ice-free seasons. Figure 4a shows the lake water level time series over Great Slave Lake. For many satellite missions, there is more than one water level time series from different retrackers. Figure 4a displays only the water level time series produced by the retracker of each mission that has the lowest data loss rate (see Table 6). For example, the water level time series produced by Jason-1 ice retracker, rather than MLE4 retracker, was displayed.
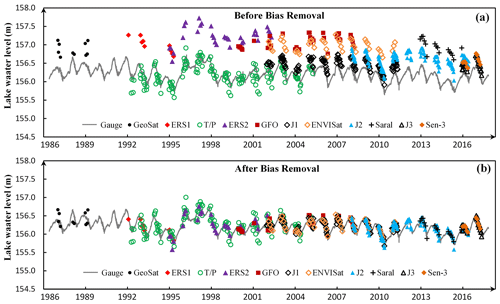
Figure 4The long-term time series of lake water level derived from the 11 satellite radar altimetry missions for Great Slave Lake in Canada. (a) The biases between altimetry-derived estimates and gauge measurements were not removed. (b) The biases were removed.
Clearly, biases exist between the altimetry-derived estimates and the gauge measurements for all missions. The magnitude of the biases varies among the missions. If altimetry-derived water levels and gauge data are both references to the same vertical datum, the small magnitude of bias indicates that the absolute values of altimetry-derived lake water level are close to the ground truth represented by gauge measurements. As shown in Fig. 4a, the time series of T/P water level estimates (given by the ocean retracker) has the least difference to the gauge data on Great Slave Lake in absolute values, while the time series of ERS-2 estimates (produced by Ice1 retracker) has the largest absolute difference from the gauge measurements. As shown in Fig. 4b, after removing the biases, the altimetry-derived estimates match the gauge measurements well for most of the missions over Great Slave Lake.
The bias value for each retracker of the 11 missions over the 12 lakes are reported in Table 7. Since only the Great Lakes' gauge measurements are referenced to the same vertical datum as altimetry-derived lake water levels, we will then focus our discussion of the bias on these five Great Lakes. For a specific lake (e.g., Lake Erie) the different missions and different retrackers of the same mission could have very different magnitudes of biases. The mean bias, with respect to gauge data, is calculated for each retracker by averaging the biases over the five Great Lakes. As shown in Table 7, the retrackers with a mean bias less than 10 cm include the ocean retracker of the TOPEX/Poseidon mission, the MLE4 retracker of the Jason-1, Jason-2 and Jason-3 missions, and the ice sheet and SAMOSA-2 retracker of the Sentinel-3 mission. The mean bias of the Jason-3 MLE 4 retracker is less than 1 cm. Note that all those low bias retrackers are model based. Actually, for all missions with multiple retrackers, the model-based retrackers outperforms the model-free retrackers in terms of mean bias over the Great Lakes.
5.3 The performance of radar altimetry missions in capturing lake water level dynamics
The Pearson correlation coefficient r was calculated for all the retrackers of each mission over every lake that has more than three lake water level estimates. A high correlation coefficient of the lake water level estimates from a retracker with gauge measurements indicates a strong capability of the retracker to reconstruct the temporal variation of lake water levels. As shown in Table 8, all the retrackers of the 11 missions, except for the ERS-1 sea ice retracker, have a good performance on large lakes (e.g., the Great Lakes). In contrast, many retrackers give an r value of less than 0.7 over small lakes. The ERS-1 ocean retracker gives the lowest r value of 0.07 over Lake Oulujärvi.
Table 8Pearson's correlation coefficient r between altimetry-derived lake level estimates and gauge measurements.
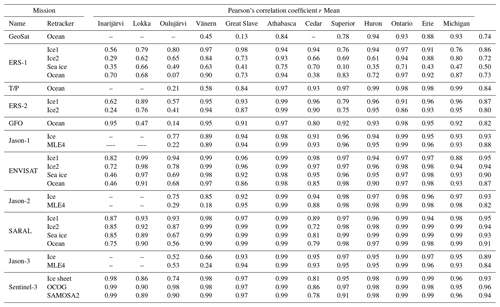
The performances of SARAL and Sentinel-3 missions in capturing the lake water level dynamics are outstanding. Almost all of their retrackers produce a very high r value over both large and small lakes. Their stronger capabilities, compared to other satellite radar missions, for retrieving water levels for small waterbodies were previously reported in Bogning et al. (2018) and Normandin et al. (2018). The Sentinel-3 Ice1 retracker gives the highest mean r value (0.96) across the 12 lakes. In contrast, the ERS-1 sea ice retracker has very poor performance over almost all the lakes, even on very large lakes, resulting in the lowest mean r value of 0.50.
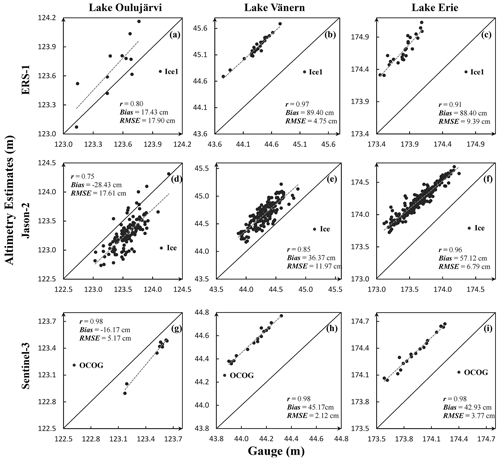
Figure 5Scatterplots of lake water level estimates given by model-free retrackers against gauge measurements. The scatterplots of the same mission are arranged in the same row, and the scatterplots of the same lake are arranged in the same column.
As indicated in Table 8, for all the missions the model-free retrackers (except for the ERS-1 sea ice retracker) outperform the model-based retrackers in depicting water level variations over small lakes. The model-free retrackers, including the Ice1 (or OCOG) retracker of ERS-1, ERS-2, ENVISAT, SARAL, and Sentinel-3 missions and the ice retracker of Jason-1, Jason-2, and Jason-3 missions all yield higher r values than model-based retrackers of the same missions over small lakes. The performance contrast between model-free and model-based retrackers is particularly conspicuous over Lake Oulujärvi and Lake Vänern. Figure 5 shows the scatterplots produced by the model-free retrackers of ERS-1, Jason-2, and Sentinel-3 over lakes Oulujärvi, Vänern, and Erie. Figure 6 shows the corresponding scatterplots produced by the model-based retrackers (ERS-1 Ocean, Jason-2 MLE4, and Sentinel-3 SAMOSA-2) of the same missions over the three lakes. Apparently, the estimates given by model-free retrackers correlate very well with the gauge measurements for all three missions over the three lakes. The correlation is higher on large lakes (e.g., Lake Erie) than on small lakes (e.g. Lake Oulujärvi). In contrast, no clear correlation can be observed between the water level estimates from ERS-1 ocean retracker and Jason-2 MLE4 retracker and gauge measurements on Lake Oulujärvi. The correlation of Jason-2 MLE4 retracker estimates with gauge measurements on Lake Vänern is very low. It suggests that, in comparison with the model-based retrackers, the model-free retrackers (OCOG/Ice1/ice) are less affected by the contamination of land surface surrounding small lakes.
5.4 Overall precision of altimetry-derived lake water level estimates from different missions
As introduced in Sect. 4.4, the RMSE was computed for each retracker after removing the bias, which contains the vertical datum difference between satellite and ground measurements and systematic error between the gauge station and retrackers. Such calculated RMSE represents the precision of altimetry-derived lake water level estimates as compared with gauge measurements. A small RMSE of a retracker means a small random error; hence, a high precision of the retracker in retrieving lake water levels. The RMSE values for all retrackers of the 11 missions over the 12 lakes are listed in Table 9. Similar to the pattern that we observed for the correlation coefficient r, the RMSE values for large lakes are significantly smaller than those for small lakes. Most retrackers of the 11 missions have a RMSE of less than 10 cm for large lakes. The RMSEs for small lakes, however, may exceed 30 cm. Among all retrackers and all missions, SARAL Ice2 retracker gives the lowest RMSE (of 1.92 cm) over Lake Ontario, while GFO produces the highest RMSE (of 132.81 cm) over Lake Oulujärvi. Again, it reflects the adverse influences of land surface on the accuracy of satellite altimeters in the retrieval of lake water levels for small lakes.
As compared to other missions, Sentinel-3 and SARAL clearly have better measurement precision in terms of RMSE over small lakes, such as Lake Inarijärvi, Lokka and Lake Oulujärvi, which is largely due to the smaller footprint of the altimeters on board these two missions. Most retrackers of these two missions yielded a RMSE less than 30 cm over the three lakes. In contrast, the RMSEs of ERS-1 retrackers over these three lakes are mostly higher than 50 cm. The mean RMSEs of the three Sentinel-3 retrackers (7.31 cm for ice sheet, 6.08 cm for OCOG, and 6.57 cm for SAMOSA-2) are much smaller than other missions. The mean RMSEs of the SARAL retrackers (7.89 cm for Ice1, 7.30 cm for Ice2, 8.85 cm for sea ice, and 10.46 cm for the ocean retracker) are slightly higher than Sentinel-3 retrackers.
For the same mission, model-free retrackers often have lower RMSEs than the model-based retrackers. For example, the average RMSEs across the 12 lakes are 14.76 cm for ERS-1 (Ice1), 11.28 cm for Jason-1 (ice), 7.74 cm for ENVISAT (Ice1), 8.18 cm for Jason-2 (ice), and 8.03 cm for Jason-3 (ice) retrackers. In contrast, the average RMSEs are 35.17 cm for ERS-1 (ocean), 18.68 cm for Jason-1 (MLE4), 14.66 cm for ENVISAT (ocean), 19.22 cm for Jason-2 (MLE4), and 17.15 cm for Jason-3 (MLE4) retrackers. The mean RMSE of the model-based retrackers is approximately twice as large as that of the model-free retrackers. The performance contrast, in terms of RMSE between the two types of retrackers, is striking for small lakes. On Lake Oulujärvi, the RMSEs for the ice retracker of Jason-1, Jason-2 and Jason-3 missions are 17.42, 17.16, and 24.65 cm. But, the RMSEs of the MLE retracker of these three missions are 124.98, 99.91, and 110.32 cm, which is 5–6 times higher than the model-free retrackers. Again, it highlights the fact that model-free retrackers are more precise choices for the retrieval of water levels for small lakes. For large lakes, both types of retrackers have similar performance in lake level estimates. Therefore, the selection of either a model-free or model-based retracker does not make much difference in the precision of water level estimates for large lakes.
Among the 11 satellite radar altimetry missions, eight of them have more than one retracker to measure the Earth's surface elevation. It should be noted that none of these retrackers were dedicated to the surface elevation measurements of inland lakes. Our evaluation intention is to identify which retrackers have relatively better performance. As shown in Tables 6, 8, and 9, all the retrackers of the same mission have similarly good performance for large lakes (e.g., the Great Lakes) in terms of the data loss rate, the correlation coefficient r, and RMSE. In other words, any of the retrackers for the same mission (except for the ERS-1 sea ice retracker) could be used to retrieve water levels for a large lake. However, for small lakes, the model-free retrackers, such as the Ice1 (OCOG) retracker of ERS-1, ERS-2, ENVISAT, and SARAL and the ice retracker of Jason-1, Jason-2, and Jason-3, are clearly better choices than the model-based retrackers, such as the ocean retracker of ERS-1, ERS-2, ENVISAT, and SARAL and the MLE4 retracker of Jason-1, Jason-2, and Jason-3 or the non-model based sea ice retracker. Our evaluation result is contrary to Sulistioadi (2015), who found comparable performances between sea ice and OCOG retrackers over a couple of small lakes (Lake Matano and Lake Towuti in Indonesia) using ENVISAT data. In a previous study, Frappart (2006) concluded that the model-free Ice1 retracker was the best among the four ENVISAT retrackers in the retrieving lake water levels. Our evaluation results consistently demonstrate that, for all radar altimetry missions, model-free retrackers tend to have high correlation coefficients and lower data loss rates and RMSEs than the model-based retrackers over small lakes. The model-free retrackers are, therefore, recommended for the retrieval of water levels over small lakes.
It is evident that the performance of the satellite radar altimetry missions has been improving with the time, as observed from Tables 6, 8, and 9. In general, the new generation of the radar altimetry mission performs better than historical missions. The data loss rate decreases from 65.42 %, for the first-generation mission of GeoSat, to 2.32 %, for the currently operational Sentinel-3 mission. The mean RMSE decreases from 35.17 cm in the early ERS-1 mission to 6.08 cm in the current Sentinel-3 mission. Among the 11 missions, the most recent Sentinel-3 mission has the best performance. All three retrackers (particularly the OCOG retracker) produced the lowest mean RMSEs and the lowest mean data loss rate among all historical and currently operational missions. The SAMOSA-2 retracker has a slightly higher RMSE and clearly lower bias than OCOG retracker. The reason is that the SAR altimeter on board Sentinel-3 increases the along-track sampling resolution (∼300 m) and maximizes the information retrieval over variable terrain surfaces (Donlon et al., 2012).
Following Sentinel-3, SARAL gave the second-best performance among these missions. The Ice1 retracker of SARAL performed well for both small lakes and large lakes. For the period between February 2013 and June 2016, the SARAL Ice1 retracker provided the best retrieval of water levels for the overpassed lakes, due to its smaller footprint and larger bandwidth, owing to the use of Ka band (Bonnefond et al., 2018). Between February 2002 and April 2012, the Ice1 retracker of the ENVISAT mission provided very accurate retrieval of lake levels. Overall, the ENVISAT Ice1 retracker gave slightly better results than the ice retracker of the Jason-1 and Jason-2 missions. However, since the repeat cycle of ENVISAT is 35 d, and the data loss rate of ENVISAT Ice1 retracker is almost twice as high as that of Jason-1 and Jason-2 missions, the two Jason missions (with a repeat cycle of 10 d) provided temporally more frequent and continuous estimates of lake water levels than ENVISAT. It should be noted that Jason-1 and Jason-2 cover only the Earth's surface between 66∘ N and 66∘ S latitudes. For lakes located at high-latitude polar regions, ENVISAT is the best alternative option during its operational time.
GFO has a much higher data loss rate than other contemporary missions. For the lakes overpassed by GFO, ERS-2, and TOPEX/Poseidon in the same period of time, GFO is the least desirable choice. For the period from 1991 to 2001, ERS-1 and ERS-2 are better choices for small lakes than TOPEX/Poseidon. But for large lakes, TOPEX/Poseidon should be adopted, since it has much more frequent overpasses than ERS-1 and ERS-2 satellites, although comparable accuracy for lake level estimates. GeoSat exhibited a good performance for large lakes (e.g., the Great Lakes). Even though it has an extremely high data loss rate for almost all 12 lakes, the water level estimates given by GeoSat are still valuable since it was sole satellite radar altimetry mission between 1985 and 1989.
To construct a long-term time series of lake water level for an ungauged lake, one critical step is to determine a reference mission to tie all satellite missions together by compensating the biases between them. A reference mission should meet two requirements. First, the reference mission should be able to provide precise lake level estimates that are at least comparable with other missions. Second, the reference mission should have a long operational time period so that it has temporal overlaps with many other missions. Both Sentinel-3 and SARAL meet the first requirement, due to their high performance for both large and small lakes. However, they have a relatively short temporal overlap with other missions and do not satisfy the second requirement. Among 11 radar altimetry missions, there are four missions that have a nominal operational time over 10 years (the geodetic phase not counted), including TOPEX/Poseidon, Jason-1, ENVISAT, and Jason-2. TOPEX/Poseidon does not meet the first requirement well, since its performance is apparently inferior to Jason-1, ENVISAT, and Jason-2 in terms of the r, RMSE, and the data loss rate. Despite its long data duration, ENVISAT has a higher data loss rate and longer repeat cycle; hence, it has less frequent water level estimates than Jason-1 and Jason-2 missions, which reduces the chance of concurrent overpasses of ENVISAT with other missions over the same lake. In comparison, Jason-2 is a better choice as the reference mission than Jason-1. First, the ice retracker of Jason-2 has a much smaller RMSE and lower data loss rate than Jason-1, as shown in Tables 6 and 9. The Jason-2 ice retracker's performance (r=0.93; RMSE = 8.18 cm) in retrieving lake water levels is close to the best performance retracker of Sentinel-3 OCOG (r=0.96; RMSE = 6.47 cm). Second, Jason-2 temporally overlapped with seven other missions, including ERS-2, GFO, Jason-1, ENVISAT, SARAL, Jason-3, and Sentinel-3. Jason-1 has six overlapping missions, as shown in Table 2. Third, Jason-2 has a short repeat cycle of 10 d; hence, it has a better chance to find concurrent overpasses with other missions over the same lake. Moreover, for the four TOPEX/Poseidon–Jason satellites, the predecessor and the successor satellites measure the same location at almost the same time (separated by 60–70 d) during their tandem phases. This allows for the accurate estimation of the inter-mission biases between them over the large lakes around the world. For example, based on the measurements during the tandem phases over the five Great Lakes, the estimated biases (with the successor satellite as the benchmark) are 0.48±4.48 cm for TOPEX/Poseidon and Jason-1, 19.56±5.38 cm for Jason-1 and Jason-2, and cm for Jason-2 and Jason-3. Using Jason-2 as the initial reference, we are able to form a consistent TOPEX/Poseidon–Jason series of water level estimates that overlaps with all other radar altimetry missions (except for GeoSat). This consistent series of water level estimates can be further used as the reference for other missions to estimate the biases between them, and then construct the long-term time series of water level records at a global scale. As discussed above, the model-free retrackers outperform the model-based retrackers over small lakes. For the purpose of constructing consistent long-term time series of lake water levels, it is better to use the same model-free retracker (e.g., OCOG/ice/Ice1) for both large and small lakes to avoid possible inter-mission retracker-induced biases.
When a lake was visited by more than one satellite mission on the same day, the best water level estimate among the overlapping missions should be selected to form a long-term series of records in terms of the performance (r and RMSE) of the missions. The water level estimates from the satellite mission with higher r value and lower RMSE should be used. For the period before 2002, the order of selection priority should be ERS-2, ERS-1, and TOPEX/Poseidon. For the period of 2002–2013, the order of selection priority should be ENVISAT, Jason-2, Jason-1, ERS-2, and GFO. For the period 2013–2020, the order of selection priority should be Sentinel-3, SARAL, Jason-3, and Jason-2.
It should be noted that the lake sample size in this study is limited by two criteria. First, each case study lake must be overpassed by all the satellite missions evaluated in this study. Second, simultaneous in situ gauge data are available for the sample lakes. After our thorough search, we identified the 12 lakes in this study that satisfy these two conditions. As compared to previous, similar evaluation studies (as mentioned in the introduction), the 12 lakes still represent the largest sample size. More importantly, these lakes are located on different continents, along different latitudes, and in different geographical environments. They include both natural lakes and reservoirs. These lakes have different sizes and winter ice conditions. They form a representative sample of the majority of inland lakes around the world. Nevertheless, we agree that it is even better if we have a much larger sample size that satisfies the above conditions, and we hope to include more sample lakes in our future research when their in situ gauge data become available.
A total of 13 satellite radar altimetry missions have been launched to measure the Earth's surface elevation since 1985. The satellite radar altimetry data collected by these missions have been widely utilized for retrieving lake water levels. Although some previous studies assessed some missions in retrieving lake water levels, our knowledge and understanding are still limited as to the comparative advantages of different retrackers across different radar altimetry missions and the effective strategy of tying all missions together to reconstruct a long-term time series to support the investigation of lake water level dynamics. In this study, we made a comprehensive evaluation on the performances of the different retrackers of 11 missions using a consistent data processing procedure and algorithms over the same set of 12 case study lakes, where the gauge measurements are available. These 12 lakes are representative for different areal size, local climate and surrounding environment.
Among the 11 missions, the most recent mission of Sentinel-3 gave the most accurate estimates, largely due to the adoption of new SAR altimetry technology. All three retrackers (particularly the OCOG retracker) of Sentinel-3 yielded very accurate lake level estimates for both large and small lakes. These SAR altimetry echoes can be coherently processed in the future to further reduce the along-track sampling resolution, which is called fully focused SAR (FF-SAR) altimetry (Kleinherenbrink et al., 2020). This could significantly increase the accuracy of lake water level estimates and would be a worthy direction for future investigation. SARAL's performance is the second best in retrieving lake water levels, owing to the advantages of the Ka band. Its Ice1 retracker works for both large and small lakes too. The ENVISAT Ice1 retracker is slightly better than the ice retracker of Jason-1 and Jason-2 in terms of r and RMSE. However, Jason-1 and -2 can provide more consistent, frequent, and continuous lake water level estimates due to their low data loss rates and short repeat cycle. Although ERS-1 and ERS-2 (e.g., the Ice1 retracker) clearly had better performance over small lakes than T/P between 1991 and 2005, TOPEX/Poseidon is still recommended for retrieving water levels for large lakes, since it had much more frequent estimates than ERS-1 and ERS-2. Both GeoSat and GFO exhibited extremely high data loss rates of lake water level estimates. GFO can be replaced by several other contemporary missions, such as T/P, ERS-2, Jason-1, and ENVISAT. However, GeoSat was the earliest sole mission in the 1980s; therefore, it is still valuable for extending the time series of lake water level as early as possible.
In order to reconstruct long-term time series of lake water level, a reference mission needs to be selected to tie all other missions together. The best strategy for constructing long-term lake water level records should be a two-step bias correction and normalization procedure. In the first step, use Jason-2 as the initial reference to estimate the systematic biases with TOPEX/Poseidon, Jason-1, and Jason-3 and then normalize them to form a consistent TOPEX/Poseidon–Jason series. Then, use the TOPEX/Poseidon–Jason series as the reference to estimate and remove systematic biases with other radar altimetry missions to construct consistent long-term lake water level series for ungauged lakes. We found that the model-free retrackers (Ice1/OCOG/ice) evidently perform better than the model-based retrackers in terms of the RMSE, the Pearson's correlation coefficient r, and the data loss rate. For the missions with more than one retracker, the model-free retracker is recommended in the construction of the long-term time series of lake water level, particularly for small lakes. For different time periods, multiple missions may have overpassed the same lake on the same day. We have worked out the priority order to select the measurements among overlapping missions in three time periods to construct the best possible lake water level time series.
All data used in this study, including the radar altimetry data and the in situ water level data, can be found at the websites listed in Sects. 2 and 3.
SoS conceptualized the study and developed the methodology in addition to conducting the formal analysis and writing the original draft. HL assisted by supervising the project and developing the methodology. HL, FF, JK, ML, MX, BY, YH, and RAB reviewed and edited the paper. RAB gathered the resources and ML curated the data, while FF and JK conducted the validation.
The authors declare that they have no conflict of interests.
The authors would like to thank the two anonymous journal reviewers for their constructive and insightful comments which helped improve the quality of this paper.
This paper was edited by Christa Kelleher and reviewed by two anonymous referees.
Adrian, R., O'Reilly, C. M., Zagarese, H., Baines, S. B., Hessen, D. O., Keller, W., Livingstone, D. M., Sommaruga, R., Straile, D., and Van Donk, E.: Lakes as sentinels of climate change, Limnol. Oceanogr., 54, 2283–2297, 2009.
Assel, R. and Wang, J.: Great Lakes Ice Cover Data – Ice Year 2017, Produced by NOAA Great Lakes Environmental Research Laboratory, available at: https://www.glerl.noaa.gov/data/ice/ (last access: 15 February 2021), 2017.
Barry, R., Finkelstein, J., Kilgus, C., Mooers, C. N. K., Needham, B., and Crawford, M.: GeoSat follow-on satellite to supply ocean sciences data, Eos Trans. Am. Geophys. Union, 76, 33–36, 1995.
Berry, P. A. M., Garlick, J. D., Freeman, J. A., and Mathers, E. L.: Global inland water monitoring from multi-mission altimetry, Geophys. Res. Lett., 32, L16401, https://doi.org/10.1029/2005GL022814, 2005.
Biancamaria, S., Frappart, F., Leleu, A. S., Marieu, V., Blumstein, D., Desjonquères, J.-D., Boy, F., Sottolichio, A., and Valle-Levinson, A.: Satellite radar altimetry water elevations performance over a 200 m wide river: Evaluation over the Garonne River, Adv. Space Res., 59, 128–146, https://doi.org/10.1016/j.asr.2016.10.008, 2017.
Biancamaria, S., Schaedele, T., Blumstein, D., Frappart, F., Boy, F., Desjonqueres, J. D., Pottier, C., Blarel, F., and Nino, F.: Validation of Jason-3 tracking modes over French rivers, Remote Sens. Environ., 209, 77–89, https://doi.org/10.1016/j.rse.2018.02.037, 2018.
Birkett, C., Reynolds, C., Beckley, B., and Doorn, B.: From Research to Operations: The USDA Global Reservoir and Lake Monitor, in: Coastal Altimetry, edited by: Vignudelli, S., Kostianoy, A. G., Cipollini, P., and Benveniste, J., Springer, Berlin, Heidelberg, 19–50, 2011.
Birkett, C. M.: The contribution of TOPEX/POSEIDON to the global monitoring of climatically sensitive lakes, J. Geophys. Res., 100, 25179–25204, 1995.
Birkett, C. M. and Beckley, B.: Investigating the Performance of the Jason-2/OSTM Radar Altimeter over Lakes and Reservoirs, Mar. Geod., 33, 204–238, https://doi.org/10.1080/01490419.2010.488983, 2010.
Bogning, S., Frappart, F., Blarel, F., Niño, F., Mahé, G., Bricquet, J.-P., Seyler, F., Onguéné, R., Etamé, J., and Paiz, M.-C.: Monitoring water levels and discharges using radar altimetry in an ungauged river basin: The case of the Ogooué, Remote Sens., 10, 350, https://doi.org/10.3390/rs10020350, 2018.
Bonnefond, P., Verron, J., Aublanc, J., Babu, K. N., Bergé-Nguyen, M., Cancet, M., Chaudhary, A., Crétaux, J.-F., Frappart, F., Haines, B. J., Laurain, O., Ollivier, A., Poisson, J.-C., Prandi, P., Sharma, R., Thibaut, P., and Watson, C.: The Benefits of the Ka-Band as Evidenced from the SARAL/AltiKa Altimetric Mission: Quality Assessment and Unique Characteristics of AltiKa Data, Remote Sens., 10, 83, https://doi.org/10.3390/rs10010083, 2018.
Brockley, D. J.: REAPER – Product handbook for ERS Altimetry reprocessed products, available at: https://earth.esa.int/web/guest/document-library/browse-document-library/-/article/reaper-product-handbook-for-ers-altimetry-reprocessed-products (last access: 15 February 2021), 2014.
Brockley, D. J., Baker, S., Féménias, P., Martínez, B., Massmann, F., Otten, M., Paul, F., Picard, B., Prandi, P., Roca, M., Rudenko, S., Scharroo, R., and Visser, P.: REAPER: Reprocessing 12 Years of ERS-1 and ERS-2 Altimeters and Microwave Radiometer Data, IEEE T. Geosci. Remote, 55, 5506–5514, https://doi.org/10.1109/TGRS.2017.2709343, 2017.
Brown, G. S.: Average Impulse Responce Of A Rough Surface And Its Applications, IEEE T. Anten. Propag., AP-25, 67–74, 1977.
Caplan, P., Derber, J., Gemmill, W., Hong, S.-Y., Pan, H.-L., and Parrish, D.: Changes to the 1995 NCEP Operational Medium-Range Forecast Model Analysis–Forecast System, Weather Forecast., 12, 581–594, https://doi.org/10.1175/1520-0434(1997)012<0581:CTTNOM>2.0.CO;2, 1997.
Chelton, D. B., Walsh, E. J., and MacArthur, J. L.: Pulse Compression and Sea Level Tracking in Satellite Altimetry, J. Atmos. Ocean. Tech., 6, 407–438, https://doi.org/10.1175/1520-0426(1989)006<0407:PCASLT>2.0.CO;2, 1989.
Cheney, R.: The Geosat Altimeter JGM-3 GDRs on CD-ROM, Natl. Oceanic and Atmos. Admin., Silver Spring, 1997.
Cheney, R., Lillibridge, J., and McAdoo, D.: Preliminary analysis of ERS-1 fast delivery altimeter data, Office of ocean and earth sciences, NOAA National Ocean Service, Rockville, MD, 1991.
Crétaux, J. F., Calmant, S., Romanovski, V., Shabunin, A., Lyard, F., Bergé-Nguyen, M., Cazenave, A., Hernandez, F., and Perosanz, F.: An absolute calibration site for radar altimeters in the continental domain: Lake Issykkul in Central Asia, J. Geod., 83, 723–735, https://doi.org/10.1007/s00190-008-0289-7, 2009.
Crétaux, J. F., Jelinski, W., Calmant, S., Kouraev, A., Vuglinski, V., Bergé-Nguyen, M., Gennero, M. C., Nino, F., Abarca Del Rio, R., Cazenave, A., and Maisongrande, P.: SOLS: A lake database to monitor in the Near Real Time water level and storage variations from remote sensing data, Adv. Space Res., 47, 1497–1507, 2011.
Crétaux, J.-F., Nielsen, K., Frappart, F., Papa, F., Calmant, S., and Benveniste, J.: Hydrological Applications of Satellite AltimetryRivers, Lakes, Man-Made Reservoirs, Inundated Areas, in: Satellite Altimetry Over Oceans and Land Surfaces, CRC Press, Boca Raton, 459–504, 2017.
Crétaux, J.-F., Bergé-Nguyen, M., Calmant, S., Jamangulova, N., Satylkanov, R., Lyard, F., Perosanz, F., Verron, J., Samine Montazem, A., Le Guilcher, G., Leroux, D., Barrie, J., Maisongrande, P., and Bonnefond, P.: Absolute Calibration or Validation of the Altimeters on the Sentinel-3A and the Jason-3 over Lake Issykkul (Kyrgyzstan), Remote Sens., 10, 1679, https://doi.org/10.3390/rs10111679, 2018.
Dee, D. P., Uppala, S., Simmons, A., Berrisford, P., Poli, P., Kobayashi, S., Andrae, U., Balmaseda, M., Balsamo, G., and d. Bauer, P.: The ERA-Interim reanalysis: Configuration and performance of the data assimilation system, Q. J. Roy. Meteorol. Soc., 137, 553–597, 2011.
Dibarboure, G., Lamy, A., Pujol, M.-I., and Jettou, G.: The Drifting Phase of SARAL: Securing Stable Ocean Mesoscale Sampling with an Unmaintained Decaying Altitude, Remote Sens., 10, 1051, https://doi.org/10.3390/rs10071051, 2018.
Donlon, C., Berruti, B., Buongiorno, A., Ferreira, M. H., Féménias, P., Frerick, J., Goryl, P., Klein, U., Laur, H., Mavrocordatos, C., Nieke, J., Rebhan, H., Seitz, B., Stroede, J., and Sciarra, R.: The Global Monitoring for Environment and Security (GMES) Sentinel-3 mission, Remote Sens. Environ., 120, 37–57, https://doi.org/10.1016/j.rse.2011.07.024, 2012.
Fernandes, M. and Lázaro, C.: GPD+ Wet Tropospheric Corrections for CryoSat-2 and GFO Altimetry Missions, Remote Sens., 8, 851, https://doi.org/10.3390/rs8100851, 2016.
Fernandes, M., Lázaro, C., Nunes, A., and Scharroo, R.: Atmospheric Corrections for Altimetry Studies over Inland Water, Remote Sens., 6, 4952, https://doi.org/10.3390/rs6064952, 2014.
Frappart, F., Calmant, S., Cauhopé, M., Seyler, F., and Cazenave, A.: Preliminary results of ENVISAT RA-2-derived water levels validation over the Amazon basin, Remote Sens. Environ., 100, 252–264, 2006.
Frappart, F., Roussel, N., Biancale, R., Martinez Benjamin, J. J., Mercier, F., Perosanz, F., Garate Pasquin, J., Martin Davila, J., Perez Gomez, B., Gracia Gomez, C., Lopez Bravo, R., Tapia Gomez, A., Gili Ripoll, J., Hernandez Pajares, M., Salazar Lino, M., Bonnefond, P., and Valles Casanova, I.: The 2013 Ibiza Calibration Campaign of Jason-2 and SARAL Altimeters, Mar. Geod., 38, 219–232, https://doi.org/10.1080/01490419.2015.1008711, 2015.
Frappart, F., Legrésy, B., Niño, F., Blarel, F., Fuller, N., Fleury, S., Birol, F., and Calmant, S.: An ERS-2 altimetry reprocessing compatible with ENVISAT for long-term land and ice sheets studies, Remote Sens. Environ., 184, 558–581, https://doi.org/10.1016/j.rse.2016.07.037, 2016.
Fu, L. L., Christensen, E. J., Yamarone, C. A., Lefebvre, M., Menard, Y., Dorrer, M., and Escudier, P.: TOPEX/POSEIDON mission overview, J. Geophys. Res.-Oceans, 99, 24369–24381, 1994.
Hannah, D. M., Demuth, S., van Lanen, H. A. J., Looser, U., Prudhomme, C., Rees, G., Stahl, K., and Tallaksen, L. M.: Large-scale river flow archives: Importance, current status and future needs, Hydrol. Process., 25, 1191–1200, https://doi.org/10.1002/hyp.7794, 2011.
Howell, S. E. L., Brown, L. C., Kang, K.-K., and Duguay, C. R.: Variability in ice phenology on Great Bear Lake and Great Slave Lake, Northwest Territories, Canada, from SeaWinds/QuikSCAT: 2000–2006, Remote Sens. Environ., 113, 816–834, https://doi.org/10.1016/j.rse.2008.12.007, 2009.
Iijima, B. A., Harris, I. L., Ho, C. M., Lindqwister, U. J., Mannucci, A. J., Pi, X., Reyes, M. J., Sparks, L. C., and Wilson, B. D.: Automated daily process for global ionospheric total electron content maps and satellite ocean altimeter ionospheric calibration based on Global Positioning System data, J. Atmos. Sol.-Terr. Phy., 61, 1205–1218, https://doi.org/10.1016/S1364-6826(99)00067-X, 1999.
Jarihani, A., Callow, J. N., Johansen, K., and Gouweleeuw, B.: Evaluation of multiple satellite altimetry data for studying inland water bodies and river floods, J. Hydrol., 505, 78–90, https://doi.org/10.1016/j.jhydrol.2013.09.010, 2013.
Jiang, L., Nielsen, K., Dinardo, S., Andersen, O. B., and Bauer-Gottwein, P.: Evaluation of Sentinel-3 SRAL SAR altimetry over Chinese rivers, Remote Sens. Environ., 237, 111546, https://doi.org/10.1016/j.rse.2019.111546, 2020.
Jones, B. M. and Arp, C. D.: Observing a Catastrophic Thermokarst Lake Drainage in Northern Alaska, Permafrost Periglac. Process., 26, 119–128, https://doi.org/10.1002/ppp.1842, 2015.
Karl, T. R., Arguez, A., Huang, B., Lawrimore, J. H., McMahon, J. R., Menne, M. J., Peterson, T. C., Vose, R. S., and Zhang, H.-M.: Possible artifacts of data biases in the recent global surface warming hiatus, Science, 348, 1469–1472, https://doi.org/10.1126/science.aaa5632, 2015.
Kleinherenbrink, M., Naeije, M., Slobbe, C., Egido, A., and Smith, W.: The performance of CryoSat-2 fully-focussed SAR for inland water-level estimation, Remote Sens. Environ., 237, 111589, https://doi.org/10.1016/j.rse.2019.111589, 2020.
Korhonen, J.: Long-term changes in lake ice cover in Finland, Hydrol. Res., 37, 347–363, 2006.
Korhonen, J.: Long-term changes and variability of the winter and spring season hydrological regime in Finland, PhD thesis, Institute for Atmospheric and Earth System Research, University of Helsinki, Helsinki, 2019.
Lambin, J., Morrow, R., Fu, L. L., Willis, J. K., Bonekamp, H., Lillibridge, J., Perbos, J., Zaouche, G., Vaze, P., Bannoura, W., Parisot, F., Thouvenot, E., Coutin-Faye, S., Lindstrom, E., and Mignogno, M.: The OSTM/Jason-2 Mission, Mar. Geod., 33, 4–25, https://doi.org/10.1080/01490419.2010.491030, 2010.
Laxon, S.: Sea ice altimeter processing scheme at the eodc, Int. J. Remote Sens., 15, 915–924, https://doi.org/10.1080/01431169408954124, 1994.
Lee-Lueng, F.: TOPEX/POSEIDON mission overview, J. Geophys. Res., 99, 24369–24381, 1994.
Lehner, B. and Döll, P.: Development and validation of a global database of lakes, reservoirs and wetlands, J. Hydrol., 296, 1–22, https://doi.org/10.1016/j.jhydrol.2004.03.028, 2004.
Lillibridge, J., Smith, W. H., Sandwell, D., Scharroo, R., Lemoine, F., and Zelensky, N.: 20 Years of Improvements to GEOSAT Altimetry, ESA Special Publication, ESA, Venice, Italy, 2006.
Liu, H., Wang, L., Tang, S. J., and Jezek, K. C.: Robust multi-scale image matching for deriving ice surface velocity field from sequential satellite images, Int. J. Remote Sens., 33, 1799–1822, 2012.
Maillard, P., Bercher, N., and Calmant, S.: New processing approaches on the retrieval of water levels in Envisat and SARAL radar altimetry over rivers: A case study of the São Francisco River, Brazil, Remote Sens. Environ., 156, 226–241, https://doi.org/10.1016/j.rse.2014.09.027, 2015.
Marsh, P., Russell, M., Pohl, S., Haywood, H., and Onclin, C.: Changes in thaw lake drainage in the Western Canadian Arctic from 1950 to 2000, Hydrol. Process., 23, 145–158, https://doi.org/10.1002/hyp.7179, 2009.
McConathy, D. R. and Kilgus, C. C.: The Navy GEOSAT mission: an overview, Johns Hopkins APL technical Digest, 8, 170–175, 1987.
Menard, Y., Fu, L. L., Escudier, P., Parisot, F., Perbos, J., Vincent, P., Desai, S., Haines, B., and Kunstmann, G.: The Jason-1 Mission, Mar. Geod., 26, 131–146, https://doi.org/10.1080/714044514, 2003.
Miller, M., Buizza, R., Haseler, J., Hortal, M., Janssen, P., and Untch, A.: Increased resolution in the ECMWF deterministic and ensemble prediction systems, ECMWF Newsletter, 124, 10–16, 2010.
Morris, C. S. and Gill, S. K.: Variation of Great Lakes water levels derived from Geosat altimetry, Water Resour. Res., 30, 1009–1017, https://doi.org/10.1029/94WR00064, 1994.
MSSL/UCL/CLS: Sentinel-3 Level 2 SRAL MWR Algorithm Theoretical Baseline Definition, Document Ref.: S3MPC.CLS.PBD.005, 138 pp., 2019.
Normandin, C., Frappart, F., Diepkilé, A., Marieu, V., Mougin, E., Blarel, F., Lubac, B., Braquet, N., and Ba, A.: Evolution of the performances of radar altimetry missions from ERS-2 to Sentinel-3A over the Inner Niger Delta, Remote Sens., 10, 833, https://doi.org/10.3390/rs10060833, 2018.
Naval Oceanographic Office and NOAA Laboratory for Satellite Altimetry: GeoSat Follow-On GDR User's Handbook, available at: https://www.star.nesdis.noaa.gov/socd/lsa/GFO/gfo_documentation.php, (last access: 15 February 2021), 2002.
Pavlis, N. K., Holmes, S. A., Kenyon, S. C., and Factor, J. K.: The development and evaluation of the Earth Gravitational Model 2008 (EGM2008), J. Geophys. Res.-Solid, 117, B04406, https://doi.org/10.1029/2011JB008916, 2012.
Pekel, J.-F., Cottam, A., Gorelick, N., and Belward, A. S.: High-resolution mapping of global surface water and its long-term changes, Nature, 540, 418–422, https://doi.org/10.1038/nature20584, 2016.
Pohl, S., Marsh, P., Onclin, C., and Russell, M.: The summer hydrology of a small upland tundra thaw lake: Implications to lake drainage, Hydrol. Process., 23, 2536–2546, https://doi.org/10.1002/hyp.7238, 2009.
Postel, S. L., Daily, G. C., and Ehrlich, P. R.: Human appropriation of renewable fresh water, Science, 271, 785–788, https://doi.org/10.1126/science.271.5250.785, 1996.
Probst, W. N., Stoll, S., Peters, L., Fischer, P., and Eckmann, R.: Lake water level increase during spring affects the breeding success of bream Abramis brama (L.), Hydrobiologia, 632, 211–224, https://doi.org/10.1007/s10750-009-9842-5, 2009.
Rahmstorf, S.: Thermohaline circulation: The current climate, Nature, 421, 699–699, https://doi.org/10.1038/421699a, 2003.
Raney, R. K. and Phalippou, L.: The future of coastal altimetry, in: Coastal Altimetry, edited by: Vignudelli, S., Kostianoy, A. G., Cipollini, P., and Benveniste, J., Springer, Berlin, Heidelberg, 535–560, https://doi.org/10.1007/978-3-642-12796-0_20, 2011.
Ričko, M., Birkett, C. M., Carton, J. A., and Crétaux, J. F.: Intercomparison and validation of continental water level products derived from satellite radar altimetry, J. Appl.Remote Sens., 6, 061710, https://doi.org/10.1117/1.JRS.6.061710, 2012.
Rodríguez, E. and Martin, J. M.: Assessment of the TOPEX altimeter performance using waveform retracking, J. Geophys. Res.-Oceans, 99, 24957–24969, https://doi.org/10.1029/94jc02030, 1994.
Rokaya, P., Budhathoki, S., and Lindenschmidt, K. E.: Trends in the Timing and Magnitude of Ice-Jam Floods in Canada, Scient. Rep., 8, 5834, https://doi.org/10.1038/s41598-018-24057-z, 2018.
Scharroo, R. and Smith, W. H. F.: A global positioning system-based climatology for the total electron content in the ionosphere, J. Geophys. Res.-Space, 115, A10318, https://doi.org/10.1029/2009ja014719, 2010.
Scharroo, R., Leuliette, E., Lillibridge, J., Byrne, D., Naeije, M., and Mitchum, G.: RADS: Consistent Multi-Mission Products, in: 20 Years of Progress in Radar Altimatry, 2012, Venice, Italy, 2013.
Schindler, D. E. and Scheuerell, M. D.: Habitat coupling in lake ecosystems, Oikos, 98, 177–189, https://doi.org/10.1034/j.1600-0706.2002.980201.x, 2002.
Schwatke, C., Dettmering, D., Borgens, E., and Bosch, W.: Potential of SARAL/AltiKa for Inland Water Applications, Mar. Geod., 38, 626–643, https://doi.org/10.1080/01490419.2015.1008710, 2015a.
Schwatke, C., Dettmering, D., Bosch, W., and Seitz, F.: DAHITI – an innovative approach for estimating water level time series over inland waters using multi-mission satellite altimetry, Hydrol. Earth Syst. Sci., 19, 4345–4364, https://doi.org/10.5194/hess-19-4345-2015, 2015b.
Shu, S., Liu, H., Frappart, F., Huang, Y., Wang, S., Hinkel, K. M., Beck, R. A., Yu, B., Jones, B. M., Arp, C. D., Wang, L., and Ye, Z.: Estimation of snow accumulation over frozen Arctic lakes using repeat ICESat laser altimetry observations – A case study in northern Alaska, Remote Sens. Environ., 216, 529–543, https://doi.org/10.1016/j.rse.2018.07.018, 2018.
Shu, S., Liu, H., Beck, R. A., Frappart, F., Korhonen, J., Xu, M., Yang, B., Hinkel, K. M., Huang, Y., and Yu, B.: Analysis of Sentinel-3 SAR altimetry waveform retracking algorithms for deriving temporally consistent water levels over ice-covered lakes, Remote Sens. Environ., 239, 111643, https://doi.org/10.1016/j.rse.2020.111643, 2020.
Sulistioadi, Y. B., Tseng, K. H., Shum, C. K., Hidayat, H., Sumaryono, M., Suhardiman, A., Setiawan, F., and Sunarso, S.: Satellite radar altimetry for monitoring small rivers and lakes in Indonesia, Hydrol. Earth Syst. Sci., 19, 341–359, https://doi.org/10.5194/hess-19-341-2015, 2015.
Surdu, C. M., Duguay, C. R., Brown, L. C., and Fernández Prieto, D.: Response of ice cover on shallow lakes of the North Slope of Alaska to contemporary climate conditions (1950–2011): radar remote-sensing and numerical modeling data analysis, The Cryosphere, 8, 167–180, https://doi.org/10.5194/tc-8-167-2014, 2014.
Thibaut, P., Poisson, J. C., Bronner, E., and Picot, N.: Relative Performance of the MLE3 and MLE4 Retracking Algorithms on Jason-2 Altimeter Waveforms, Mar. Geod., 33, 317–335, https://doi.org/10.1080/01490419.2010.491033, 2010.
Turner, K. W., Wolfe, B. B., Edwards, T. W. D., Lantz, T. C., Hall, R. I., and Larocque, G.: Controls on water balance of shallow thermokarst lakes and their relations with catchment characteristics: A multi-year, landscape-scale assessment based on water isotope tracers and remote sensing in Old Crow Flats, Yukon (Canada), Global Change Biol., 20, 1585–1603, https://doi.org/10.1111/gcb.12465, 2014.
Tyszkowski, S., Kaczmarek, H., Słowiński, M., Kozyreva, E., Brykała, D., Rybchenko, A., and Babicheva, V. A.: Geology, permafrost, and lake level changes as factors initiating landslides on Olkhon Island (Lake Baikal, Siberia), Landslides, 12, 573–583, https://doi.org/10.1007/s10346-014-0488-7, 2015.
Verpoorter, C., Kutser, T., Seekell, D. A., and Tranvik, L. J.: A global inventory of lakes based on high-resolution satellite imagery, Geophys. Res. Lett., 41, 6396–6402, https://doi.org/10.1002/2014GL060641, 2014.
Verron, J., Sengenes, P., Lambin, J., Noubel, J., Steunou, N., Guillot, A., Picot, N., Coutin-Faye, S., Sharma, R., Gairola, R. M., Murthy, D. V. A. R., Richman, J. G., Griffin, D., Pascual, A., Rémy, F., and Gupta, P. K.: The SARAL/AltiKa Altimetry Satellite Mission, Mar. Geod., 38, 2–21, https://doi.org/10.1080/01490419.2014.1000471, 2015.
Villadsen, H., Deng, X., Andersen, O. B., Stenseng, L., Nielsen, K., and Knudsen, P.: Improved inland water levels from SAR altimetry using novel empirical and physical retrackers, J. Hydrol., 537, 234–247, https://doi.org/10.1016/j.jhydrol.2016.03.051, 2016.
Vu, P. L., Frappart, F., Darrozes, J., Marieu, V., Blarel, F., Ramillien, G., Bonnefond, P., and Birol, F.: Multi-Satellite Altimeter Validation along the French Atlantic Coast in the Southern Bay of Biscay from ERS-2 to SARAL, Remote Sens., 10, 93, https://doi.org/10.3390/rs10010093, 2018.
Weyhenmeyer, G. A., Westöö, A. K., and Willén, E.: Increasingly ice-free winters and their effects on water quality in Sweden's largest lakes, Hydrobiologia, 599, 111–118, https://doi.org/10.1007/s10750-007-9188-9, 2008.
Williamson, C. E., Saros, J. E., Vincent, W. F., and Smol, J. P.: Lakes and reservoirs as sentinels, integrators, and regulators of climate change, Limnol. Oceanogr., 54, 2273–2282, 2009.
Wingham, D. J., Rapley, C. G., and Griffiths, H.: New Techniques In Satellite Altimeter Tracking Systems, in: Digest – International Geoscience and Remote Sensing Symposium (IGARSS), Zurich, Switzerland, 1339–1344, 1986.
Wingham, D. J., Francis, C. R., Baker, S., Bouzinac, C., Brockley, D., Cullen, R., de Chateau-Thierry, P., Laxon, S. W., Mallow, U., Mavrocordatos, C., Phalippou, L., Ratier, G., Rey, L., Rostan, F., Viau, P., and Wallis, D. W.: CryoSat: A mission to determine the fluctuations in Earth's land and marine ice fields, Adv. Space Res., 37, 841–871, 2006.
Zandbergen, R., Dow, J. M., Romay Merino, M., Píriz, R., and Martínez Fadrique, F.: ERS-1 and ERS-2 tandem mission: determination, prediction and maintenance, Adv. Space Res., 19, 1649–1653, https://doi.org/10.1016/S0273-1177(97)00319-0, 1997.
Zelli, C.: ENVISAT RA-2 advanced radar altimeter: instrument design and pre-launch performance assessment review, Acta Astronaut., 44, 323–333, https://doi.org/10.1016/S0094-5765(99)00063-6, 1999.
Ziyad, J., Goïta, K., Magagi, R., Blarel, F., and Frappart, F.: Improving the Estimation of Water Level over Freshwater Ice Cover using Altimetry Satellite Active and Passive Observations, Remote Sens., 12, 967, https://doi.org/10.3390/rs12060967, 2020.