the Creative Commons Attribution 4.0 License.
the Creative Commons Attribution 4.0 License.
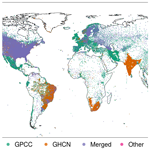
Rainfall Estimates on a Gridded Network (REGEN) – a global land-based gridded dataset of daily precipitation from 1950 to 2016
Steefan Contractor
Markus G. Donat
Lisa V. Alexander
Markus Ziese
Anja Meyer-Christoffer
Udo Schneider
Elke Rustemeier
Andreas Becker
Imke Durre
Russell S. Vose
We present a new global land-based daily precipitation dataset from 1950 using an interpolated network of in situ data called Rainfall Estimates on a Gridded Network – REGEN. We merged multiple archives of in situ data including two of the largest archives, the Global Historical Climatology Network – Daily (GHCN-Daily) hosted by National Centres of Environmental Information (NCEI), USA, and one hosted by the Global Precipitation Climatology Centre (GPCC) operated by Deutscher Wetterdienst (DWD). This resulted in an unprecedented station density compared to existing datasets. The station time series were quality-controlled using strict criteria and flagged values were removed. Remaining values were interpolated to create area-average estimates of daily precipitation for global land areas on a 1∘ × 1∘ latitude–longitude resolution. Besides the daily precipitation amounts, fields of standard deviation, kriging error and number of stations are also provided. We also provide a quality mask based on these uncertainty measures. For those interested in a dataset with lower station network variability we also provide a related dataset based on a network of long-term stations which interpolates stations with a record length of at least 40 years. The REGEN datasets are expected to contribute to the advancement of hydrological science and practice by facilitating studies aiming to understand changes and variability in several aspects of daily precipitation distributions, extremes and measures of hydrological intensity. Here we document the development of the dataset and guidelines for best practices for users with regards to the two datasets.
- Article
(15004 KB) - Full-text XML
- BibTeX
- EndNote
Earth's climate is changing, leading to spatial and temporal variations in precipitation. These changes in precipitation are strongly linked to social, economic and environmental prosperity due to the role precipitation plays in global food production and maintaining biodiversity. Theoretical expectations are that the global hydrological cycle would intensify in a warmer climate, associated with increases in mean and extreme precipitation (whereby mean and total precipitation would increase at lower rate than extreme precipitation due to energetic constraints; Allen and Ingram, 2002). In addition to changes in precipitation due to climate change, precipitation is also characterised by strong variability in many regions. Reliable observations are necessary to understand these short- and long-term changes and to evaluate climate models which help understand the processes driving these changes. Hence in some ways gridded observations of the past also help us to better plan for and adapt to these changes in the future.
All observations have errors – for example, gauge-based precipitation measurements are subject to undercatch, wind related errors, evaporation loss, wetting loss, splash in/out errors and tipping errors (see McMillan et al., 2012 for details). However, alternatives to gauge-based measurements such as satellite observations, model reanalysis products and radar-based observations have additional limitations. Reanalysis products assimilate observations and models to create a synthesised estimate of the state of the earth system. They are often misused as observations but in fact inherit issues from the incomplete observations and imperfect models and are based on complex assimilation techniques. Furthermore, none of the reanalysis products assimilate surface precipitation observations (MERRA2 however incorporates satellite infrared and microwave measurements) and as such are not representative of reality. This is evidenced by the classification of precipitation as the least reliable class by Kalnay et al. (1996). Reanalyses also contain temporal inhomogeneities due to the changing amount of assimilated observations over time (Compo et al., 2006). According to Lorenz and Kunstmann (2012) even the state-of-the-art reanalyses are unsuitable for climate trend and long-term water budget analysis. Radar estimates provide high spatial and temporal resolution estimates of rainfall over local regions; however, these estimates can be inaccurate compared to rain gauges (Krajewski et al., 2010; Villarini and Krajewski, 2010; McKee and Binns, 2016), and very few national networks of radar observations exist.
Satellite products have become available in recent years. These datasets are gridded and boast a global or quasi-global coverage. The Tropical Rainfall Measuring Mission (TRMM) 3B42 (Huffman et al., 2007), Global Precipitation Climatology Projects 1 Degree Daily (GPCP-1DD) (Huffman et al., 2001), Climate Hazards Group InfraRed Precipitation with Stations (CHIRPS) (Funk et al., 2015) and the Precipitation Estimates from Remotely Sensed Information using Artificial Neural Networks – Climate Data Record (PERSIANN-CDR) (Ashouri et al., 2014) are some examples of popular satellite-based precipitation products. These satellite-based datasets, however, use complex algorithms to derive precipitation estimates from indirect radiation measurements, resulting in large uncertainties in precipitation estimates. For example GPCP-1DD measures infrared reflectivity of clouds to infer the cloud thickness and then estimates precipitation rates based on the poor relationship between clouds and rainfall (Kidd and Levizzani, 2011). This estimate is also adjusted based on monthly gauge observations; however, the uncertainties remain high. In general satellite products perform well in the tropics where the rain rates are higher but struggle with snow and ice and on complex terrain (Bytheway and Kummerow, 2013; Tian and Peters-Lidard, 2010; Contractor et al., 2015). New satellite missions and technology will be able to overcome these shortcomings over time. For example, the recently launched Global Precipitation Measurement (GPM) mission is an international satellite mission that aims to improve the detection of light rain and snowfall as well as provide quantitative estimates of precipitation particle size distribution (Hou et al., 2014). The biggest limitation of satellite products, however, is also their brevity. It was only after the Tropical Rainfall Measurement Mission (TRMM) in 1997 that we entered an era of multi-sensor measurements across multiple satellites to produce a globally consistent and complete map of precipitation (Tian and Peters-Lidard, 2010). Thus the satellite products do not allow for an analysis of global rainfall changes that effectively separates the natural variability from anthropogenic climate change. Very recently, datasets that blend together precipitation estimates from multiple sources such as gauge observations, satellite observations and even reanalyses have become available. Examples include MSWEP V2 (Beck et al., 2019), CHIRPS (Funk et al., 2015) and Shen et al. (2014). These datasets offer very high spatial and temporal resolution data with a reasonably long temporal record. However, these datasets may exhibit increased temporal variability due to the incorporation of various observational sources over time and do not include as many in situ station observations as the gauge-only datasets.
Observations have shown spatially varying changes in mean precipitation across the globe (Trenberth, 2011; Hartmann et al., 2013) and robust increases in extreme precipitation across various regions and in the global average (Groisman et al., 2005; Westra et al., 2013; Donat et al., 2016). These global analyses of observed precipitation changes were based on datasets of monthly precipitation accumulations (such as Climatic Research Unit's CRU TS; Harris et al., 2014; Mitchell and Jones, 2005, Global Precipitation Climatology Centre's GPCC Full Data Monthly; Becker et al., 2013; Schneider et al., 2015, Global Historical Climatology Network's GHCN-Monthly; Peterson and Vose, 1997, Global Precipitation Climatology Project GPCP-Monthly; Adler et al., 2003; Huffman et al., 1997; and the Smith et al., 2012 dataset), or datasets providing indices representing specific aspects of extreme precipitation (such as GHCNDEX; Donat et al., 2013a, HadEX; Alexander et al., 2006 and HadEX2, Donat et al., 2013b). Availability of daily precipitation data, however, would allow analysis of precipitation at different parts of the distribution, and for a wider range of temporal aggregations. A daily resolution dataset would also enable a more robust estimate of the extremes since monthly datasets average out the extremes and dampen the variability in daily observations. Existing gauge-based quasi-global gridded datasets of daily precipitation are short (such as CPC Global Precipitation dating back to 1979 (Chen and Xie, 2008; Xie et al., 2007; Chen et al., 2008) and GPCC Full Data Daily V1 which dates back to 1988 (Schamm et al., 2015). An updated version, GPCC Full Data Daily V2018, was released in June 2018, covering from 1982 to 2016) and therefore do not allow for robust analysis of long-term variability or trends. The main reason for this is the lack of data sharing between countries, which results in poor spatial coverage earlier in time. Even in cases where meteorological organisations have agreements in place with countries to obtain gauge data (such as GPCC on behalf of Deutscher Wetterdienst – DWD), the length of their analysis is limited due to the lack of high-quality data extending back in time. To reach a high level of quality, the GPCC applies a quality control procedure with manual inspection of questionable values, which is very time consuming but preserves the real extremes in the data. Many regional- or continental-scale products are also available which are produced by local meteorological organisations or researchers who have a more complete set of daily gauge data available to them and thus have longer temporal records. Examples of such datasets include E-OBS for Europe (Haylock et al., 2008), CPC for the United States (Chen and Xie, 2008; Chen et al., 2008; Xie et al., 2007), AWAP for Australia (Jones et al., 2009), APHRODITE for Asia (Yatagai et al., 2012), CLARIS for South America (Menendez et al., 2010), and national and regional products for UK (Perry and Hollis, 2005), Spain (Herrera et al., 2012), Germany (Rauthe et al., 2013), Switzerland (Frei and Schär, 1998; Isotta et al., 2013), Norway (Lussana et al., 2018), India (Rajeevan et al., 2006) and the Middle East (Yatagai et al., 2008).
Spatially regular gridded data, rather than irregular station data, facilitate many studies (such as climate variability studies investigating connections between regional or global precipitation phenomena and large-scale changes) that are not spatially biased. Furthermore, climate models also rely on gridded data. Gridded datasets are needed for initialising, forcing and validating global and regional climate models. Since the models also produce outputs representative of area averages (Osborn and Hulme, 1998) as opposed to point-based processes, gridded datasets are also necessary to evaluate them. Finally, gridded observations can provide reasonable estimates in regions where local station data are unavailable but stations within the typical length scales of precipitation systems in that region may be present.
Given all the limitations of existing datasets noted above, our aim here was to create a new long-term global land-based dataset with increased raw station density back to the mid-twentieth century. In this study we present the data and methods used to create such a dataset, called Rainfall Estimates on a Gridded Network (REGEN), and evaluate it against existing daily and monthly, global and regional products. We also describe how uncertainty estimates are calculated and finally provide guidelines for how to best use (and not use) the dataset.
REGEN was created by acquiring daily station precipitation data from various sources, quality controlling them using an automated algorithm and merging them into a single archive, which was then interpolated with ordinary block kriging. We created two related datasets: the first dataset (REGEN AllStns V1-2019) interpolated the entire station network referred to henceforth as REGEN and the second dataset (REGEN LongTermStns V1-2019) interpolated only the long-term stations referred to henceforth as REGEN40YR. Stations considered long-term here are those with at least 40 complete years of data, described in more detail in Sect. 2.4. Both datasets cover the period 1950–2016. In this section the various raw data sources, automated quality control, automated station matching algorithm and the interpolation method are described.
2.1 Raw gauge data
The raw station data for REGEN has three sources:
-
the Global Precipitation Climatology Centre (GPCC), operated by Deutscher Wetterdienst (DWD) (approximately 100 000 stations),
-
the Global Historical Climatology Network – Daily (GHCN-Daily) version 3.22-upd-2017092104: stations hosted by National Centers for Environmental Information (NCEI) in the USA (Menne et al., 2012) (103 635 stations), and
-
other stations Argentina and Russian (approximately 1000 stations).
The total number of stations interpolated each day in REGEN range from a minimum of 35 460 to a maximum of 56 190, with an average of 50 530 (Fig. 1a). Regionally, the number of stations per day doubles in North America after 2000 and decreases substantially in South America from the late 1990s. There are no Chinese stations in 1950 and there is a large drop in stations in India in 1970, affecting the total number of stations per day in Asia. Stations in Africa are sparse throughout the time period of REGEN; however, there are still more stations compared to other existing global rainfall products. However, this highlights a very important issue regarding the sharing of meteorological data between countries. Global datasets of observations are limited by the amount of station data available. Regions of poor station coverage are most abundant in Africa and Asia because of limited capability or readiness of countries to share data, despite the World Meteorological Organisation (WMO) data policy encouraging free and unrestricted exchange of meteorological data and products. Therefore, even the in situ data held by GPCC can only be distributed in the form of derived products such as the gridded dataset described in this article. We encourage maintainers and providers of data to advocate for increased and more open sharing of meteorological data within their organisations.
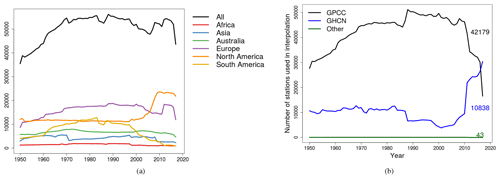
Figure 1Final (interpolated), quality-controlled number of stations over time by (a) region and (b) source. Figure 4c shows a map of the regions. Due to the varying station network over time, the total number of stations over the entire temporal domain sums to 135 178 stations. The numbers in black, blue and green in (b) refer to the average number of stations from GPCC, GHCN and Other sources respectively.
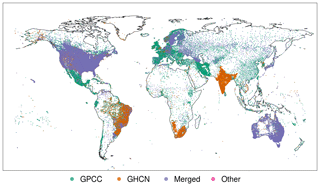
Figure 2Distribution of stations colour coded by source. “GPCC” refers to stations hosted by Deutsche Wetterdienst, “GHCN” refers to stations hosted by National Centers for Environmental Information (NCEI), “Merged” refers to stations that have been identified as identical in two or more archives resulting in a merger of the time series and finally “Other” refers to the Russian and Argentinian stations that were added by us.
The majority of the underlying station data for REGEN is sourced from the stations hosted by GPCC (Fig. 1b). Note that Fig. 1b does not show the actual number of stations in GHCN-Daily or Other archives, but rather the number of daily records from stations in GHCN-Daily or Other that were unique with respect to the stations in the GPCC archive. Due to the large overlap between the archives, the number of stations from GHCN-Daily is higher when fewer stations from GPCC are available. There is a gradual increase in stations from GPCC until 1990 and a steep decline after 2010. All quality-controlled station data hosted by GPCC are eventually archived in a relational database (henceforth referred to as GPCC database); however, there were additional ASCII data files for various countries that were not processed at the time of the analysis (henceforth referred to as GPCC ASCII data files).
Figure 2 shows that most of the station data for Central America, western South America, Europe, Africa, Middle East and East Asia were sourced from GPCC.
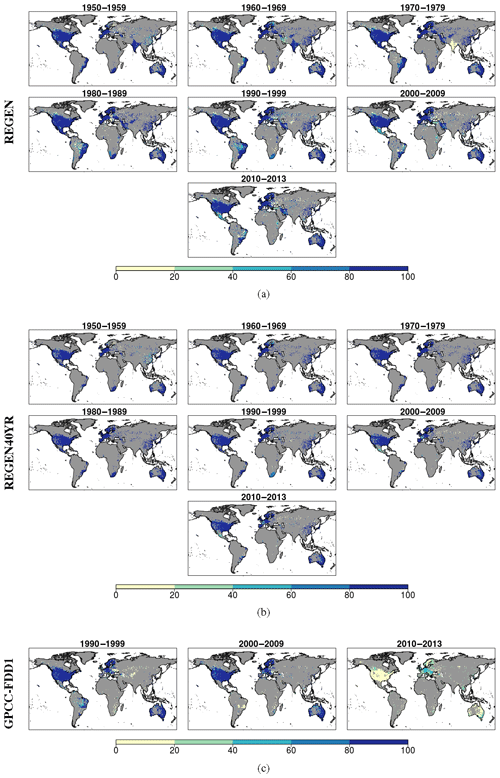
Figure 3Grids showing percentage of days with at least one station in each decade for (a) REGEN, (b) REGEN40YR and (c) GPCC-FDD1. Grey areas indicate grids where no stations are present.
We summarise the spatial and temporal distribution of the station network comprising REGEN in Fig. 3a. Each map in Fig. 3 refers to a decade and shows for each grid the percentage of days in each decade with at least one station, based on REGEN (Fig. 3a), REGEN40YR (Fig. 3b) and also GPCC's Full Data Daily V1 (GPCC-FDD1; Schamm et al., 2015) for comparison (Fig. 3c). We compare REGEN's station network with GPCC-FDD1's because until REGEN, GPCC-FDD1 was the global dataset of daily precipitation with the highest station density. It can be seen that not only is REGEN's station network density higher than GPCC-FDD1 in all the decades, but even the REGEN40YR station network with a much stricter completeness criterion has more stations in all three comparable decades relative to GPCC-FDD1.
2.2 Quality control
The quality control procedures used in REGEN were adopted from NCEI, part of National Oceanic and Atmospheric Administration (NOAA) in the USA (Durre et al., 2010). The quality control is done in two stages and climatologies generated in an auxiliary step are used in both stages. At the end of the quality control process all data are written in a common format identical to the GHCN-Daily format (see README file, ftp://ftp.ncdc.noaa.gov/pub/data/ghcn/daily/readme.txt, last access: 22 September 2017). For a thorough account of the validation of each quality control (QC) check including the respective false-positive rates, see Durre et al. (2010). The total false-positive rate based on all checks is 1 % (Durre et al., 2010).
The first quality control stage involves basic integrity checks such as checks for erroneous zeros, conflicts between multi-day accumulations and daily reports, duplication of entire years or months, repetition or frequent occurrence of values, and world record exceedances. Only minor changes (to account for different data formats) were made to the original QC procedures from Durre et al. (2010) before applying them. In addition, this test stage also checks for outliers by checking for gaps in tails of distributions and checks for climatological outliers. The test also performs some temporal consistency checks by comparing values with consecutive days to look for unrealistic spikes in precipitation. The second quality control stage does spatial corroboration checks, which determines whether the value at each station is consistent with the values at neighbouring stations. For further information and detail on the quality control algorithms, refer to Durre et al. (2010). Data failing any tests at any point of the quality control process are flagged (see GHCN-Daily README file (ftp://ftp.ncdc.noaa.gov/pub/data/ghcn/daily/readme.txt, last access: 22 September 2017) for a list of quality flags and their meanings). In order to ensure a high-quality final dataset, all flagged data are removed prior to interpolation. Although the QC procedures were designed to minimise the number of instances in which true extremes are flagged as errors (Durre et al., 2010), it is possible that a few such extremes are among the flagged values that were withheld from the REGEN input data. Future versions of REGEN may consider methods for recognising and saving possible flagged extremes.
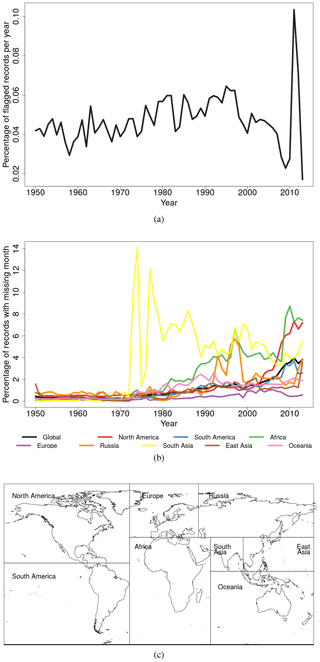
Figure 4Percentage of records that (a) failed one or more quality control tests and were flagged and (b) were not used as input for interpolation due to missing monthly totals and hence missing anomaly values. Panel (c) shows a map of regions as used for (a) and (b).
All data sources (each country in the GPCC ASCII data, the GPCC database and Other data) were quality controlled individually before merging. Since our QC procedures are identical to the GHCN-Daily, we used the flags already included with the GHCN-Daily data. The percentage of flagged records per year in the final merged input data average around 0.05 %–0.06 % throughout the time period, spiking to 0.1 % around 2010 (Fig. 4a). This may be because the number of stations in the final merged station network sourced from GHCN-Daily increase in time in the last decade of the temporal record while the number of stations sourced from GPCC decrease. Since GPCC data are assumed to be of higher quality compared to GHCN-Daily due to the manual quality control they are subjected to, the flag rate increases with time as well due to the higher percentage of GHCN-Daily stations in the last decade of the final merged station network. In general we also see a trend of increasing missing months with time in all regions (Fig. 4b). A month is marked as missing if it contains fewer than 70 % of the possible number of daily data records. We chose a threshold of 70 % as it was used by GPCC for creating their daily gridded products (Schamm et al., 2014). Haylock et al. (2008) also use a similar threshold of 80 %. As a result the percentage of missing months is also an indicator of the completeness of the daily data records. The spike in missing month percentage in South Asia is because there are no Indian stations available after 1970.
2.3 Merger of GHCN-daily, GPCC and other smaller data archives
Once the station data from various sources were quality controlled individually they were merged with each other in multiple steps. First, the manually and automatically quality-controlled data in GPCC's database were merged with ASCII data files for various countries that at the time of the analysis were not integrated into the GPCC database, to create a combined archive of quality-controlled GPCC stations. This GPCC archive was then merged with the GHCN-Daily archive and subsequently the Argentinian and Russian data.
For consistent comparison GPCC shifts data for certain countries so the daily amount always represents the day closest to 07:00 LT on the day of the timestamp to 07:00 LT the next day, local time. For example, if the source in situ data timestamp represents the day from 09:00 LT the previous day to 09:00 LT on the day of the source timestamp, then the resulting GPCC timestamps are shifted a day back compared to the source timestamps. This results in climatologically consistent timestamps. In our case, while merging the GHCN data, we shifted the GHCN data timestamps identically to the way GPCC shifted their timestamps, for all countries whose timestamps were shifted by GPCC. The countries for which the data are shifted a day back (e.g. data from 2 January are saved as 1 January) are listed in the Appendix. So far no countries' data have been shifted forward. This data shifting is important to keep in mind when comparing REGEN with regional datasets. For example when comparing REGEN with the precipitation from the Australian Water Availability Project regional dataset (AWAP; Jones et al., 2009) we shifted AWAP a day backward. This may also result in inconsistent comparisons between REGEN and satellite datasets which represent 00:00 UTC on the day of the timestamp to 00:00 UTC the next day, and also inconsistent comparisons across political borders where the time zone changes. Figure 7b highlights this timestamp shifting by plotting the unshifted precipitation amount from AWAP averaged across Australia during Cyclone Yasi as a dashed line, and the shifted AWAP and REGEN estimates as solid lines. Note that some countries maintain a mix of manually monitored and automated weather stations which may represent precipitation over differing 24 h windows that may not be suitable for being shifted identically. For example, around 10 % of observations in the US and around 30 stations in the Netherlands are midnight observations, i.e. observations over the 24 h period from midnight to midnight UTC, which are assigned to the day on which the observing period ends. Although these observations have not been manually adjusted in this version of REGEN, they will be taken care of in the next iteration. Globally more countries may exist whose gauge observations may represent a mix of reporting times (due to the use of automatic weather stations for example); however, without proper metadata about these reporting times it is not possible for us to adjust their timestamp accordingly.
The merging algorithm used is described below. Two stations were considered identical if either of the following conditions were fulfilled:
-
The latitude and longitudes matched to three decimal places, and their elevation (to the nearest integer, if non-missing) and World Meteorological Organisation (WMO) station IDs either match or are missing. Alternatively the stations were also considered a match if the WMO IDs were non-missing and matched and the latitude and longitude matched to one decimal place.
-
If the coordinates were within 1∘ of each other, WMO IDs either matched or were missing, the correlation between the time series that overlap was greater than 0.99 and the overlapping time series themselves had at least 365 daily data records with a minimum of 10 d with precipitation greater than 1 mm. A search radius of 1∘ was necessary to allow for many stations to be compared with each other in order to account for possible inaccuracies in station metadata (coordinates).
Note that the above algorithm can result in false matches as nearby stations can be highly correlated; however, this will mainly be an issue in highly dense networks such as US. For the future version, a more quantitative measure of similarity between station time series will be used. Also note that WMO station IDs do not change after a station is relocated to a site in the vicinity, which can result in two stations in different locations being merged together according to our criteria. On occasions where precipitation amount from a station was different between multiple sources, we prioritised data from higher-quality sources and accepted values from these sources. The data qualities and hence priorities in descending order (highest quality first) are GPCC database, GPCC ASCII data files, Other data and GHCN-Daily data. This way if data from a higher-quality source were missing, they were replaced with data from a matching station from a lower-quality source but not vice versa. Note that this approach may induce inhomogeneities in the raw station data.
2.4 Interpolation method
Station data were interpolated using ordinary block kriging, exactly as in the method used by GPCC's Full Data Daily V1 (GPCC-FDD1; Schamm et al., 2015) product. Ordinary block kriging is a stochastic interpolation method which means it accounts for the statistical structure of precipitation in terms of the spatial autocorrelation function. The autocorrelation function models the statistical relationship between the Euclidean distances between the observations and their correlation. The interpolation method calculates a weighted average of the nearest station values based on their distance to the grid point and the autocorrelation function. This interpolation method was chosen by Schamm et al. (2014) after a comparison with various different methods. It produces area-average precipitation estimates implicitly by estimating the interpolated field at various points inside the grid box and then calculating their weighted sum. This results in estimates directly comparable to other forms of data that produce area-average estimates such as satellite products or climate models. More details on the interpolation method, including the autocorrelation function and its parameters, equations to calculate kriging estimates and their numerical implementation, are described in Schamm et al. (2014) and Rubel (1996).
We interpolated ratios of the daily precipitation to the total monthly precipitation. If both the daily records and monthly totals were zero, the ratio was set to zero as well to ensure consistency with monthly datasets. The monthly totals for calculating daily ratios in the station time series were obtained by summing the daily station data as well. A month was considered complete if it had at least 70 % of non-missing days. The absolute values were retrieved post-interpolation by superimposing the interpolated ratios on the GPCC Full Data Monthly V2018 product (Ziese et al., 2018). This dataset was chosen because it is a well established dataset recommended for historical precipitation, global water cycle and trend analysis (Becker et al., 2013; Schneider et al., 2014, 2017). Furthermore, GPCC-FDD1 also calculates ratios using an older version of this dataset (GPCC Full Data Monthly V7, Schneider et al., 2015; the newer version stops in 2016 whereas the older version stops in 2013) and it was readily available on the GPCC High Performance Computer (HPC) where the interpolation was performed. This approach is commonly known as climatology-aided interpolation (CAI) and has two advantages. Firstly CAI reduces the influence of elevation and other variables (Hofstra et al., 2008) which allows us to interpolate with only latitude and longitude as input variables. Secondly, because monthly gridded datasets are often based on much more reliable and stable station networks, especially in areas with problematic daily station coverage, the final absolute values may be more reliable in these regions. A disadvantage of interpolating anomalies was that even if a daily record existed, it was not used for interpolation if the monthly total was missing because of the completeness criteria. Finally, since we use GPCC Full Data Monthly V2018 to retrieve daily absolute precipitation values, our analysis is also limited to the temporal extent of this monthly dataset, which is currently up to the year 2016 (a previous version of REGEN, Version 1.0, used GPCC Full Data Monthly V7 and hence stops in 2013). The interpolation parameters and auto-correlation function were also identical to the GPCC-FDD1 product and are described in Schamm et al. (2014). The interpolation scheme uses the nearest 4 to 10 stations for interpolation (the numbers were chosen to have similar settings as the modified SPHEREMAP scheme utilised for the monthly analysis) and stations within 1 km are averaged to remove station duplicates as well as reduce the impact of such nearby stations on the estimate. For complete coverage, however, the search radius is increased until the minimum station requirement is met. This means that for these stations in data-sparse regions, the search radius can be much bigger than the decorrelation length scale of 347 km which is reflected in the kriging error (see below). The decorrelation length scale is calculated from the autocorrelation function and is indicative of the extent of a station's influence.
Besides the interpolated fields, three other fields characterising the underlying data or uncertainty are provided with the dataset. These are as follows:
-
Kriging error. This is not an absolute error but rather can be interpreted as percentage of variance (Rubel, 1996). It is a result of solving the kriging equations and is dependent on the density of the observations and size of the grid (Schamm et al., 2014).
-
Yamamoto standard deviation. This can be interpreted as an absolute error as it is the variance between the estimate and the observations used in interpolation, weighted by the kriging weights (Yamamoto, 2000).
-
The field of number of stations inside each grid cell Note that these are the actual number of observations inside a grid box, and that this is not the number of stations used for interpolation of that grid cell estimate as stations outside the grid cell may be used for interpolation in some cases where density is low.
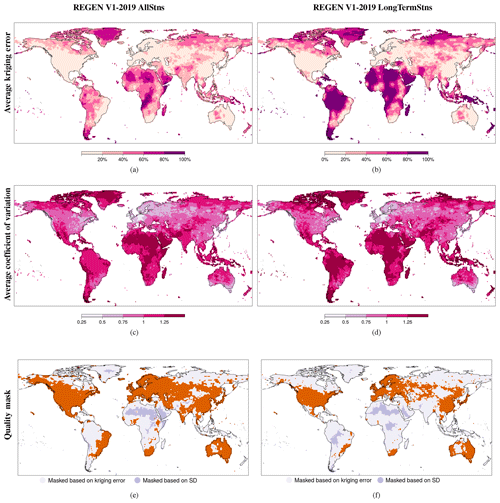
Figure 5Kriging error (KE) (a, b) and coefficient of variation (CoV) (c, d) defined by the ratio of the Yamamoto standard deviation (Yamamoto, 2000) averaged over 1950–2016 and the daily precipitation averaged over 1950–2016, and masks based on the KE and CoV (e, f) based on REGEN (a, c, e) and REGEN40YR (b, d, f) data. SD: standard deviation.
The 1950–2016 average kriging error (KE) and coefficient of variation (CoV), and the data quality mask based on KE and CoV are shown for REGEN and REGEN40YR in Fig. 5. The CoV, defined as the ratio of the Yamamoto standard deviation and the precipitation estimate, is a normalised measure of the variance at each grid cell. The kriging error is largest in regions with a low station density such as Greenland, Africa and South America and is larger for REGEN40YR compared to REGEN as expected (Fig. 5a and b). The coefficient of variation, however, is comparable between REGEN and REGEN40YR. The largest CoV values (maximum of 2.33) are once again seen in Africa, South America, Greenland and Southeast Asia (Fig. 5c and d). This means that the variance between the grid cell estimate and the observations used for interpolation is more than twice as large as the average precipitation for these grids. Grids with CoV greater than 1.9 make up less than 0.05 % (22 all together) of the grid cells, with the mode of CoV being around 1.25. The resulting data quality mask based on kriging error and coefficient of variation for REGEN40YR has a smaller global land coverage, with particularly sparse coverage in Africa, South America and Asia in both version of the dataset (Fig. 5e and f).
As mentioned earlier, we interpolated two different sets of underlying station data to create two related datasets. The first interpolates all available station data while the second interpolates only the long-term data defined by stations with at least 40 complete years of data, where a year was considered complete if all 12 months were non-missing, i.e. each month had at least 70 % non-missing days. The All station dataset (REGEN) is useful for those users who do not have access to a regional precipitation product based on a high station density and would like an approximate estimate of precipitation as well as for users interested in the best estimate (based on as many stations as possible) of precipitation amounts at each time step, accepting that this may result in a decrease in temporal homogeneity. It is also useful for users seeking more complete fields of precipitation over global land areas and less interested in the uncertainties introduced due to station network variability. REGEN40YR is useful for users conducting a climate-scale analysis of precipitation such as looking at changes in various precipitation indices over several decades, since the use of long-term stations minimises artificial variability of grid cell values due to network variations. Users must use a dataset (REGEN or REGEN40YR) that is suitable to their needs in conjunction with a quality mask (described below).
We provide a quality mask for both datasets where the masked grids are of lower quality. The masks were prepared based on the kriging error and coefficient of variation. Figure 5e and f shows the data quality masks for the two REGEN datasets. A grid cell was left unmasked if it either contained at least 60 % of the days in every decade from 1950 to 2016 (7 in total) with at least one station, or both the grid cell coefficient of variation and kriging error were under the 95th percentile threshold of the 1950–2016 average coefficient of variation and 1950–2016 average kriging error respectively. For ease of use we provide a single mask for the entire data period; however, we recognise that the coefficient of variation, kriging error and number of stations per grid vary over time, meaning a different mask could be calculated for each day. Such a mask would keep all grid cells with at least one station in addition to all grid cells with the coefficient of variation and kriging error within the 95th percentile of all the grids on the day. A possible recommended use case for the unmasked (high-quality) grids of REGEN would be the evaluation of or comparison with another dataset (such as a satellite product) or climate model output.
In this section we evaluate REGEN and REGEN40YR with existing monthly and daily precipitation datasets by showing comparisons of maps and time series.
3.1 Comparison with global gridded datasets of monthly precipitation
Traditionally, global trends in historical precipitation are analysed with monthly datasets since no other suitable long-term daily datasets existed (e.g. Hartmann et al., 2013). Here we reproduce the trend comparison from Hartmann et al. (2013) while including REGEN. Annual precipitation anomalies are compared in Fig. 6 between REGEN, REGEN40YR, GPCC Full Data Monthly Version 7 (GPCC; Schneider et al., 2015), CRU TS v4.01 (CRU; Mitchell and Jones, 2005) and GHCN Monthly Version 2 dataset (GHCN; Peterson and Vose, 1997). Anomalies were calculated by subtracting the average of total annual precipitation from 1950 to 2010 from the total annual precipitation for each dataset respectively. The variability in annual precipitation totals between REGEN and the other datasets is very similar, especially when compared to GPCC-FDD1 and CRU. GHCN has higher variability in many years compared to the other datasets including REGEN and REGEN 40YR.
3.2 Comparison with regional gridded datasets of daily precipitation
Regional gridded datasets of daily precipitation are often created by local meteorological organisations and as such are often based on a much denser station network than global datasets (compare for e.g. Herrera et al., 2012 and Haylock et al., 2008) and an interpolation method optimised for the local regions. Furthermore, the local organisations also have a much better understanding of the station metadata. The result is a dataset with long temporal records ideal for analysing individual events and precipitation extremes. REGEN's skill in capturing individual significant precipitation events is highlighted by a comparison of time series of daily totals from various events between REGEN, REGEN40YR and other commonly used regional datasets available for Europe, the USA, Australia and Asia (Fig. 7). There is good agreement between the daily time series from REGEN, REGEN40YR, and both 0.25 and 1∘ (regridded from 0.25∘version using CDO remapcon2) versions of E-Obs Version 16 (Haylock et al., 2008) (note that E-Obs also uses CAI with global kriging to interpolate the daily anomalies) for the events of the Great Flood of 1968 in southeast England (Jackson, 1977) (Fig. 7a). Precipitation shown is spatially averaged over Ireland, southern England, northern France, Belgium and the Netherlands with the events occurring in mid-September. In Australia, the precipitation events around the landfall of Cyclone Yasi in 2011 are compared between REGEN, REGEN40YR and the AWAP (Jones et al., 2009) dataset, which is the most commonly used dataset of daily precipitation. Since the in situ data for Australia were shifted a day back during the production of REGEN, the AWAP daily averages were also shifted a day backward for this comparison and the agreement is high between the three datasets. Similarly, daily precipitation time series averaged over the Philippines during the Tropical Storm Thelma in 1991 are shown in Fig. 7c. In this case we compare REGEN and REGEN40YR against APHRODITE (Yatagai et al., 2012), which is the longest-running freely available dataset of daily precipitation in Asia at the moment, and SA-Obs V1 (van den Besselaar et al., 2017). REGEN and especially REGEN40YR contain a lot fewer stations compared to APHRODITE and SA-Obs in this region (Fig. 7c), which results in much larger differences in estimates between the datasets (Fig. 7a and b). REGEN captures the daily variability in APHRODITE well on most days; however, the long-term version (REGEN40YR) with a lot fewer stations (due to the strict completeness criteria) exhibits larger differences, substantially overestimating compared to APHRODITE on 1 and 9 November. On the other hand, REGEN40YR captures more of the variability of SA-Obs compared to REGEN, especially from 3 November onward. Interestingly, the spike on 27 October is present in APHRODITE, REGEN and REGEN40YR but not in SA-Obs and the spike on 8 November is present in SA-Obs, REGEN and REGEN40YR but not in APHRODITE. Finally, based on a comparison of daily rainfall rates during Tropical Storm Amelia, which made landfall in southern United States, there is also good agreement between REGEN, REGEN40YR and CPC CONUS (Chen and Xie, 2008; Chen et al., 2008; Xie et al., 2007) (Fig. 7d).
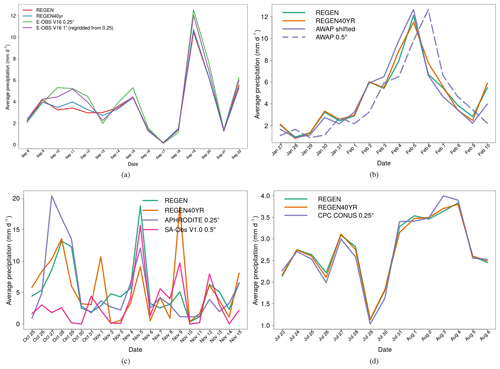
Figure 7Daily time series averaged over spatial regions of significant rainfall events. (a) Time series of daily rainfall during the great flood of 1968 over southeast England. (b) Time series of daily rainfall during Cyclone Yasi in northeast Australia in 2011. (c) Time series of daily rainfall during typhoon Thelma in Philippines in 1991. (d) Time series of daily rainfall during Tropical Storm Amelia in the USA in 1978.
As a more detailed comparison, we calculated the difference in daily estimates between REGEN and the five regional datasets mentioned above (CPC CONUS, E-Obs V16, AWAP, APHRODITE and SA-Obs). The five regional datasets were all regridded to the same 1∘ grid as REGEN and daily differences were calculated for each corresponding grid over the respective temporal periods of each regional dataset (CPC CONUS – 1950–2006, E-Obs V16 – 1950–2016, AWAP – 1950–2015, APHRODITE – 1950–2007, SA-Obs V1 – 1981–2014). Temporal correlations between REGEN and the respective regional datasets were also calculated at each grid. The mean difference between REGEN and CPC CONUS is positive in eastern United States and negative in the west (Fig. 8a), the standard deviation (SD) of the daily difference is high in coastal areas (Fig. 8b), and the temporal correlation is high everywhere (Fig. 8c). The mean difference between REGEN and E-Obs V16 is positive in most regions across the E-Obs domain (Fig. 8d), the SD of the difference is higher in the south and in Iceland compared to the northern parts of the E-Obs domain (Fig. 8e), and the temporal correlations are higher in regions of high station density (such as central Europe, the UK and Scandinavia) compared to low station density regions (such as northern Africa and Turkey) (Fig. 8f). The mean difference between REGEN and AWAP is positive in northern and central Australia and negative elsewhere (Fig. 8g), the SD of the difference is high in the northern and eastern coastal areas of Australia (Fig. 8h), and the temporal correlation is high everywhere except for the low station density regions of central Australia (Fig. 8i). Note that similar to Fig. 7b the AWAP daily data had to be shifted a day backward once again for a more suitable comparison. The mean of the daily difference between REGEN and APHRODITE is positive in most regions, and both the mean and SD of difference show higher values on the west coast of the Indian Peninsula, the maritime continent and the high-elevation Himalayan regions (Fig. 8j and k). The temporal correlation between REGEN and APHRODITE is high in continental Asia and low in the maritime continent (Fig. 8l). Finally, the mean difference between REGEN and SA-Obs is positive in most regions of the SA-Obs domain, with larger values of both the mean and SD of the difference in the maritime continent. Conversely, the temporal correlation between REGEN and SA-Obs is high in northern Australia and low in the maritime continent. High differences between REGEN and all regional datasets are observed in coastal areas. Note that it is possible that this is an artefact of the regridding of the regional datasets to a 1∘ resolution.
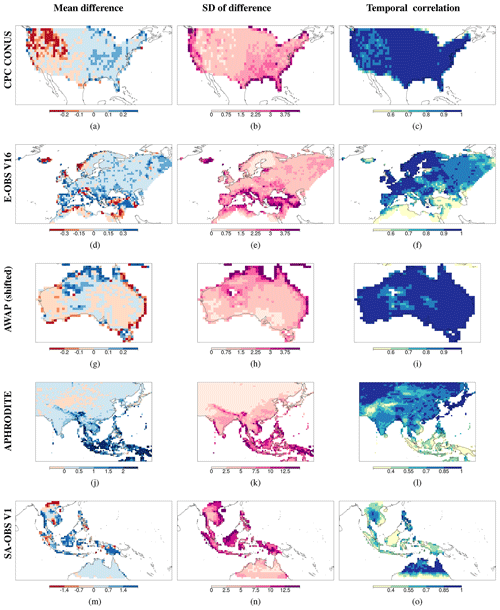
Figure 8Mean (a, d, g, j, m) and standard deviation (b, e, h, k, n) of the difference in daily values (mm d−1), and temporal correlation (c, f, i, l, o) between REGEN and CPC CONUS (a–c), REGEN and E-Obs V16 (d–f), REGEN and AWAP (g–i), REGEN and APHRODITE (j–l), and REGEN and SA-Obs V1 (m–o) over the respective periods of each regional dataset.
A comparison of the number of stations interpolated by each of the five regional datasets mentioned above (APHRODITE, SA-Obs V1, E-Obs V16, CPC CONUS and AWAP) and the corresponding stations interpolated by REGEN and REGEN40YR in the respective regions of each datasets is shown in Table 1. In some cases, due to a lack of available information, the daily maximum number of stations has been listed as opposed to the total number of stations for the entire time periods. In these cases, we also provide the daily maximum number of stations interpolated by REGEN and REGEN40YR. REGEN and REGEN40YR interpolate fewer stations compared to APHRODITE and SA-Obs. This is particularly striking in Southeast Asia, where REGEN40YR interpolates only 64 stations compared to 7956 interpolated by SA-Obs. On the other hand REGEN interpolates more stations compared to E-Obs and CPC CONUS. Note that some of these stations, especially in the US, may be duplicates missed by our merging algorithm. Finally, there is little difference in the station network interpolated by REGEN and AWAP.
Table 1Total number of stations interpolated by five regional datasets and the corresponding number of stations in each region interpolated by REGEN and REGEN40YR datasets over the entire time period of each respective regional dataset (CPC CONUS – 1950–2006, E-Obs V16 – 1950–2016, AWAP – 1950–2015, APHRODITE – 1950–2007, SA-Obs V1 – 1981–2014).
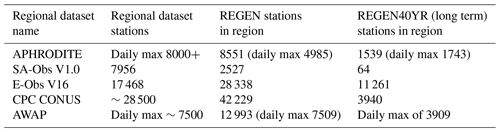
3.3 Case study over sub-Saharan Africa
Based on the maps of kriging error (Fig. 5a and b) the most data-sparse regions of REGEN are Africa, South America, Greenland and northern Russia. Despite the sparsity of data, REGEN can still be useful to get estimates of daily rainfall in some parts of these regions. We use the country of Benin in sub-Saharan Africa as an example. Benin has a tropical climate receiving the majority of rainfall around the summer months of June–August (JJA). In the summer of 2008 Benin experienced catastrophic flooding events which displaced around 150 000 people (WHO, 2010). The flooding started with heavy rainfall in the last week of July (IRIN, 2008). The time series of daily rainfall from 1950 to 2013 highlights 2008 as the year with the third highest rainfall on record based on REGEN (Fig. 9a), with the highest being in 1957. On comparison of the daily rainfall time series between 1957 and 2008 (Fig. 9b), the anomalous rainfall in late June and late July is apparent, even compared to 1957. This highlights REGEN's effectiveness in capturing the daily rainfall even in some parts of sub-Saharan Africa. Note that data from the region of Benin are of higher quality compared to surrounding regions as they are not masked in the data quality mask (Fig. 5e).
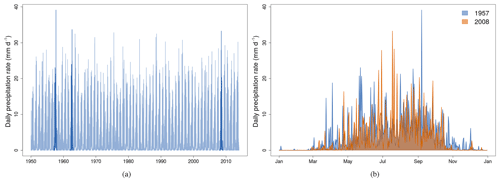
Figure 9Time series of daily precipitation from REGEN averaged over Benin in western Africa. Panel (a) shows the entire time series from 1950 to 2016 with the years containing the days with the highest three daily rainfall rates (1957, 1963 and 2008) shown in a darker shade. Panel (b) shows a comparison of the time series of daily rainfall between 1957 (year containing the day with the record highest rainfall based on REGEN) and 2008 (year during which the 2008 Benin floods occurred). Benin was chosen because of its good coverage of stations.
3.4 Comparison with existing global datasets of daily precipitation
Finally, in this section the only other existing global gridded gauge-based datasets of daily precipitation are compared. The temporally averaged annual total, annual maximum precipitation, trends in annual total and trends in annual maxima are compared against NOAA Climate Prediction Center's (CPC) Unified Gauge-Based Analysis of Daily Precipitation (CPC-Global) (Chen and Xie, 2008; Xie et al., 2007; Chen et al., 2008) and GPCC Full Data Daily V1 (GPCC-FDD1) (Fig. 10). For comparability CPC-Global, whose native resolution is 0.5∘ was regridded to 1∘ to match the GPCC-FDD1 and REGEN. The temporal coverage of CPC-Global and GPCC-FDD1 is 1979–2017 and 1988–2013 respectively. The temporal averaging and comparison was therefore done over 1988–2013, which is the longest common period between the three datasets. As expected REGEN is more similar to GPCC-FDD1 and REGEN40YR compared to CPC-Global for both the means and trends of both indices. This is because REGEN and GPCC-FDD1 use the same interpolation method and for the most part even the same underlying data. The largest differences between the three datasets arise in data-sparse regions in the high latitudes, Africa, Southeast Asia and the high-altitude regions in western South America. The spatial variability of the differences in annual total and annual maxima trends is higher compared to the spatial variability of differences in averages of the annual totals and annual maxima. Due to the lack of long-term stations in Saharan Africa, differences in all four indices between REGEN and the long-term-station-based REGEN40YR are larger compared to differences between REGEN and GPCC-FDD1 in northern Africa. Herold et al. (2016) showed CPC-Global produces lower annual totals compared to an ensemble of observational datasets including GPCC-FDD1, satellite products and reanalyses. This is consistent with our results since the difference in annual totals between REGEN and CPC-Global are positive in a majority of global land areas with the exception of northern North America and northern Africa.
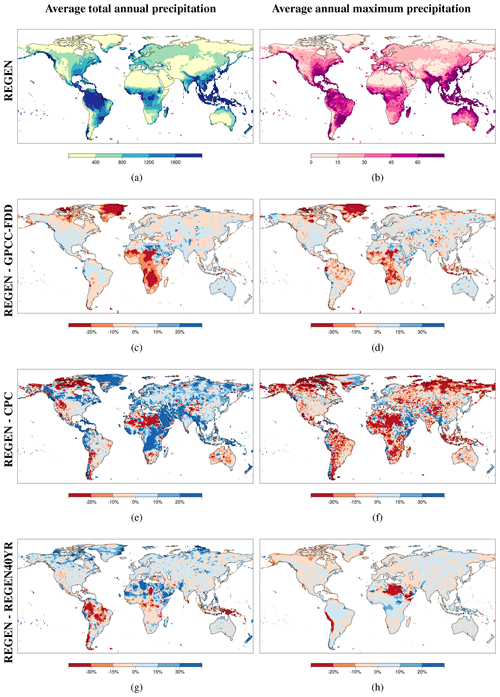
Figure 10Percentage difference in averaged total annual precipitation (c, e, g) and averaged maximum annual precipitation (d, f, h) between REGEN and GPCC (c, d), REGEN and CPC (e, f), and REGEN and REGEN40YR (g, h) data. The first row shows the absolute values of total annual precipitation (a) and annual maxima (b) averaged over 1988–2013 (the longest common time period between the three datasets).
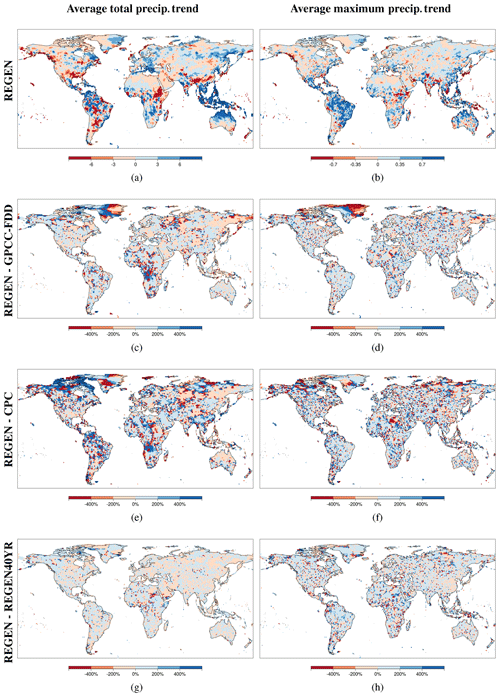
Figure 11Percentage difference in total annual precipitation trends (c, e, g), and annual maximum precipitation trends (d, f, h) between REGEN and GPCC (c, d), REGEN and CPC (e, f), and REGEN and REGEN40YR (g, h) data. The first column shows the absolute values of total annual precipitation trends (a) and annual maximum precipitation trends (b) averaged over 1988–2013 (the longest common time period between the three datasets).
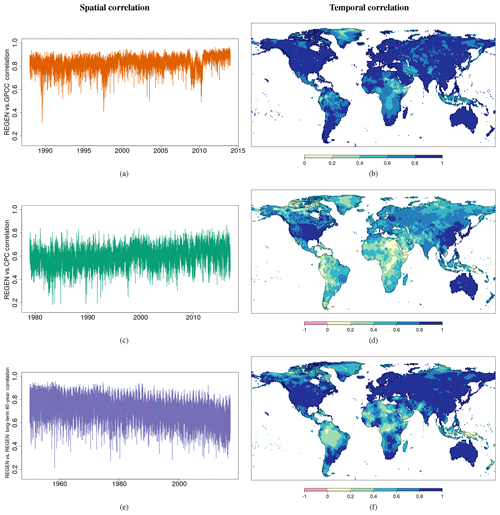
Figure 12Spatial (field) correlation at each daily time step (a, c, e) and temporal correlation between time series at each grid cell (b, d and, f) between REGEN and GPCC (a, b), REGEN and CPC (c, d), and REGEN and REGEN40YR long-term (e, f) data. Comparisons are over the entire common temporal period between each dataset pair (1988–2013 for REGEN vs. GPCC-FDD1, 1979–2016 for REGEN vs. CPC and 1950–2016 for REGEN vs. REGEN40YR).
Temporal and spatial correlations between REGEN and GPCC-FDD1 (Fig. 12a and b) are also higher compared to temporal and spatial correlations between REGEN and CPC-Global (Fig. 12c and d). Correlations between REGEN and CPC may be lower in parts where the underlying stations were shifted a day backward (see Appendix). Indeed, based on correlations between REGEN lagged +1 d and CPC (Fig. A1), the correlations are higher compared to Fig. 12d in and around the countries where data were shifted a day back (e.g. Vietnam, Brazil, Uruguay, Peru, Suriname, the Netherlands, Norway, Ukraine and Turkey). Correlations do not change compared to Fig. 12d in all regions where REGEN raw station data are not shifted. The spatial and temporal correlation between REGEN and GPCC-FDD1 is even higher than the correlation between REGEN and REGEN40YR (Fig. 12e and f) because REGEN's station network is more similar to GPCC-FDD1 than REGEN40YR. The areas with poor temporal correlation between REGEN and REGEN40YR correspond to areas with low station density such as the high latitudes, Africa and South America. Compared to the field correlation between REGEN and GPCC-FDD1, the correlation between REGEN and REGEN40YR is also more variable. This may be because the lower station density results in an increase in daily variability in interpolated fields. The drop in field correlation between REGEN and GPCC-FDD1 around 2010 corresponds to the higher percentage of GHCN stations in the last few years (Fig. 1b). There is also a decline in field correlation over time between REGEN and REGEN40YR, which may be related to the decline in the number of long-term stations over time. The temporal correlation between REGEN and CPC-Global is highest in the USA, Australia, East Asia and a small part of Europe. These regions all correspond to regions with good station density throughout the time period.
We present a new gauge-based dataset of gridded daily precipitation with a grid resolution of 1∘ × 1∘, global land coverage and temporal coverage from 1950 to 2013 called REGEN. REGEN was produced by interpolating quality-controlled in situ daily rainfall time series data using ordinary block kriging. The interpolation method for REGEN is identical to GPCC-FDD1 (another gridded dataset of daily precipitation from 1988–2016). REGEN also uses all the in situ daily data used by GPCC-FDD1 but expands on this raw data by combining it with GHCN-Daily and raw data from other sources. This resulted in an extended in situ daily precipitation network with coverage back to 1950. The raw data were subjected to comprehensive automated control procedures identical to the one used by the GHCN-Daily dataset and all suspicious data were removed, interpolating only the high-quality data. We used climatologically aided interpolation (CAI) which involved interpolating ratios of daily totals and monthly totals and retrieving absolute values by superimposing gridded monthly precipitation fields on the interpolated anomalies. This approach results in more reliable estimates in regions with sparse daily in situ data network and a comparatively denser monthly in situ data network. CAI also reduces the influence of variables such as elevation, distance to the coast etc., which allows us to interpolate using only the latitude and longitude as input variables. The gridded monthly fields used to retrieve the absolute daily precipitation rates came from GPCC Full Data Monthly V7 dataset.
REGEN is currently the longest dataset of daily precipitation based on gauge-only records with global land coverage, making it ideal for any global analysis at climatological scales. We therefore hope it will contribute to the advancement of hydrological science and practice by enabling a number of studies aiming to understand changes and variability in several aspects of daily precipitation distributions, including precipitation extremes, and measures of hydrological intensity. So far the only datasets that allowed global climatological-scale analyses of precipitation were monthly datasets or gridded ETCCDI indices; however, the monthly datasets tend to average out the extremes, in turn losing their usefulness when it comes to high-impact phenomena related to intense rainfall at shorter timescales. REGEN due to its daily temporal resolution fills this data gap. REGEN, like GPCC-FDD1, also provides various uncertainties related to the daily gridded fields. These include the Yamamoto standard deviation, which is indicative of the proximity of the estimated fields to the raw station values, the kriging error which is indicative of the density of stations inside the grid cell and finally also the exact number of stations inside each grid cell. Based on these measures a quality mask for REGEN that combines all three pieces of uncertainty information indicating the high-quality grid cells (with low uncertainties) is also presented. Users of REGEN should use the quality mask in all cases except when spatial completeness is of utmost importance. Alongside REGEN (which interpolates all station data) another related dataset that minimises artefacts due to station network variability by interpolating only the long-term stations (i.e. stations with at least 40 years of complete data) is also produced. Both datasets include bespoke data quality masks. As a result, although the station density is lower in the long-term version, users can use its quality mask to restrict their analysis to higher-quality areas. For analyses sensitive to the station network variability the long-term station version with the high-quality mask would be the most suitable. Note, however, due to the lower station density, the long-term station version may be less suitable for investigating individual events or short time series. The All station version on the other hand would be more suitable for analysis where a complete global coverage is important but temporal homogeneity is of lower priority. In any analysis it is recommended to use the data quality mask; however, in regions where no other daily datasets are available (such as parts of Africa), REGEN may provide a suitable rough estimate of precipitation even in lower-quality grids.
REGEN has been compared with global monthly and daily as well as regional daily gridded datasets of precipitation. The annual precipitation anomalies have been shown to resemble those from the other monthly datasets, and the spatial fields of annual totals and maxima as well as their trends more closely resemble GPCC-FDD1 than CPC. Even the daily time series of individual events of significant precipitation resemble the respective regional datasets closely in Europe, Australia and the USA. The larger inconsistencies between the long-term REGEN data and APHRODITE in Asia are indicative of the lower station densities in REGEN in this region. Also note that there is almost no raw in situ daily data in mainland China in 1950. As such any analysis focusing on China using this dataset should not go further back than 1951. Finally, note that despite our best efforts to homogenise station data before interpolating, because the raw data are sourced ultimately from various countries with different measurement practices (such as time of measurement, use of units, quality control and homogenisation steps etc.), inhomogeneities across political borders are possible (Trewin, 2010).
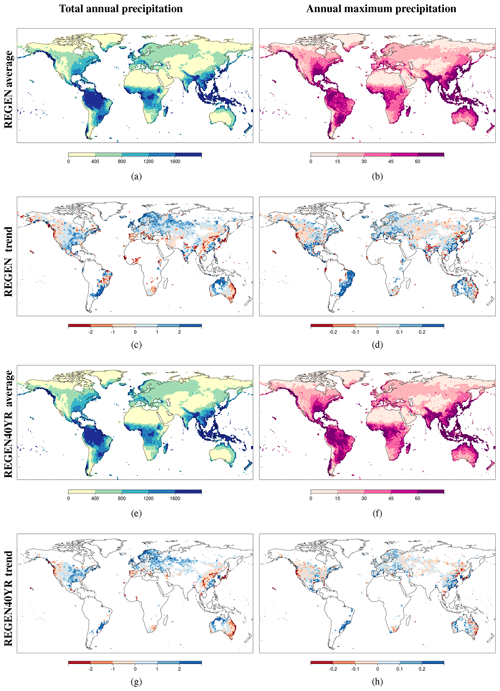
Figure 13Total annual precipitation (a, e), maximum annual precipitation (b, f) and respective trends (PRCPtot; c and g and RX1DAY; d and h) averaged over 1950 to 2016 based on REGEN data (a–d) and REGEN40YR data that only interpolates stations with at least 40 complete years of data (e–h).
Rainfall is highly variable and a 1∘ spatial resolution (roughly 10 000 km2) dataset such as REGEN is unlikely to contain the information necessary for many typical local-to-regional rainfall applications. However, we note the actual rainfall amounts in gridded datasets are subject to large uncertainties anyway (Herold et al., 2016), whereas estimates of variability are more robust. We therefore believe REGEN will prove itself valuable for climatological applications including studies of climate variability and long-term changes in daily precipitation intensity and extremes, as it provides long temporal coverage of quasi-global daily precipitation observations. The biggest strength of REGEN is the long temporal coverage of quasi-global daily precipitation observations. Regional datasets are often developed by national meteorological organisations and often have access to significantly more data than shared with Global archives such as GHCN-Daily and GPCC. For example the Spanish Meteorological Agency (AEMET) itself manages roughly over 9000 stations (Herrera et al., 2012), which is almost the same number of stations as those used by E-Obs for the entirety of Europe (around 12 000 gauges at its maximum). Furthermore, Herrera et al. (2012) only used the high-quality stations, which accounted for roughly 30 % of total stations available from AEMET. Often the respective meteorological organisation also have the resources to more thoroughly and in some cases even manually quality control the raw data. As a result, regional datasets (where available) may provide more accurate precipitation estimates than REGEN.
At the moment REGEN is not an operational product, meaning the analysis for REGEN was done as a single instance and there are currently no plans to update it regularly, such as on an annual or biennial basis.
Figure 13 reflects REGEN's strengths by showing annual totals and maxima and trends over the high-quality regions over the entire 67-year record of REGEN. Both the total annual precipitation and annual maxima based on REGEN are reasonable, with higher totals and maxima in the known wet regions such as the tropics and lower totals and maxima in the known dry regions such as Saharan Africa (Fig. 13a and b). Trends in total precipitation based on REGEN (Fig. 13c) are also comparable to the trends in total precipitation shown in the IPCC's 5th Assessment report (Fig. 2.29, Hartmann et al., 2013). The total annual precipitation, annual maxima and respective trends in the two indices based on the long-term REGEN data (REGEN40YR) (Fig. 13e–h) are also very similar to REGEN, which suggests that the effects of station variations appear negligible at this scale (for trends and averages over 1950–2013) for the high-quality grids. The trend maps shown in Fig. 13 have been masked based on the quality masks as shown in Fig. 5e and f.
REGEN provides precipitation estimates comparable to those from the currently most reliable datasets such as GPCC-FDD1. With a temporal coverage 152 % longer than that of GPCC-FDD1's and a similar global land coverage, REGEN is highly suitable for analysing climate change. We recognise that observations are not the “truth” but rather just our best estimates of it. REGEN and its variant REGEN40YR (which minimises station network variability) are therefore accompanied by various uncertainty estimates as well as a quality mask, allowing users a firm handle of the observational uncertainties in their analysis.
The countries for which the data are shifted a day back (e.g. data from 2 January are saved as 1 January) are Angola, Antarctica, Argentina, Australia, Azerbaijan, Bahamas, Bangladesh, Barbados, Benin, Bolivia, Botswana, Brazil, Bulgaria, Burkina Faso, Cameroon, Chad, Chile, Costa Rica, Croatia, Denmark/Greenland, Ethiopia, French Polynesia, Gabon, Georgia, Indonesia, islands in the Indian Ocean (IOT), Ivory Coast, Japan, Kenya, Libya, Madagascar, Malawi, Mali, Marshall Islands, Mauritania, Mozambique, the Netherlands, Niger, Norway, Peru, Senegal, Slovenia, Solomon Islands, Sudan, Suriname, Tanzania, Tunisia, Turkey, Ukraine, Uruguay, Vanuatu, Vietnam, Zambia and Zimbabwe.
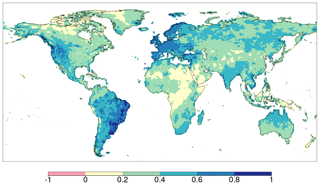
Figure A1Temporal correlations between REGEN and CPC, similar to Fig. 12d, but this time with CPC shifted a day backwards.
REGEN AllStns V1-2019 (REGEN) and REGEN LongTermStns V1-2019 (REGEN40YR) data have now been published with unique Digital Object Identifiers (DOIs) https://doi.org/10.25914/5ca4c380b0d44 (Contractor et al., 2019a) and https://doi.org/10.25914/5ca4c2c6527d2 (Contractor et al., 2019b) respectively. Older versions of both datasets, REGEN AllStns V1.0 and REGEN LongTermStns V1.0, are also available (https://doi.org/10.25914/5b9fa55a8298c, Contractor et al., 2019c and https://doi.org/10.25914/5b9fa67fce5d6, Contractor et al., 2019d respectively), however, we recommend users use the newer versions. Both datasets can be acquired in netcdf format along with netcdfs of the quality masks via the Research Data Australia (RDA) web pages https://researchdata.ands.org.au/rainfall-estimates-gridded-v1-2019/1408744 (last access: 15 January 2020) and https://researchdata.ands.org.au/rainfall-estimates-gridded-v1-2019/1408742 (last access: 15 January 2020) respectively. The RDA records contain further information about the datasets such as the dataset abstract, citation information, related organisations, grants, researchers and dataset managers (SC).
Dataset License and Rights. Non-Commercial License: CC-BY-NC-SA
Creative Commons – Attribution – Non Commercial – No Derivatives 4.0 International
http://creativecommons.org/licenses/by-nc-sa/4.0/legalcode
Access to this dataset is free, the users are free to download this dataset and share it with others and adapt it as long as they credit the dataset owners, provide a link to the license, and if changes were made, indicate it clearly and distribute their contributions under the same license as the original, commercial use is not permitted.
LVA, MD, SC and AB conceptualised the study. ID and RSV provided support during the quality control stage of the project by providing QC scripts, expertise and by hosting SC for two weeks at NCEI headquarters in Asheville, NC, USA. The GPCC team (ER, AM-C, US and MZ) provided support during the merger and interpolation stage of the project by providing data, computational resources, interpolation scripts, guidance and expertise, and by hosting SC for a month at DWD. SC wrote the manuscript with close input from LVA and MGD, and all authors commented on and improved the text.
The authors declare that they have no conflict of interest.
We are grateful to the National Centers for Environmental Information (NCEI) for providing QC scripts, raw GHCN-Daily data and hosting SC on a research visit, and we are grateful to the Global Precipitation Climatology Centre (GPCC) for providing interpolation scripts, computational resources and for hosting SC on a research visit to allow for on-site access to its data archive.
This research has been supported by the Australian Research Council (grant nos. DP160103439, CE110001028 and DE150100456) and the Spanish Ministry for Science and Innovation (grant no. RYC-2017-22964).
This paper was edited by Dimitri Solomatine and reviewed by two anonymous referees.
Adler, R. F., Huffman, G. J., Chang, A., Ferraro, R., Xie, P.-P., Janowiak, J., Rudolf, B., Schneider, U., Curtis, S., Bolvin, D., Gruber, A., Susskind, J., Arkin, P., and Nelkin, E.: The Version-2 Global Precipitation Climatology Project (GPCP) Monthly Precipitation Analysis (1979–Present), J. Hydrometeorol., 4, 1147–1167, https://doi.org/10.1175/1525-7541(2003)004<1147:TVGPCP>2.0.CO;2, 2003. a
Alexander, L. V., Zhang, X., Peterson, T. C., Caesar, J., Gleason, B., Klein Tank, A. M. G., Haylock, M., Collins, D., Trewin, B., Rahimzadeh, F., Tagipour, A., Rupa Kumar, K., Revadekar, J., Griffiths, G., Vincent, L., Stephenson, D. B., Burn, J., Aguilar, E., Brunet, M., Taylor, M., New, M., Zhai, P., Rusticucci, M., and Vazquez-Aguirre, J. L.: Global observed changes in daily climate extremes of temperature and precipitation, J. Geophys. Rese.-Atmos., 111, D05109, https://doi.org/10.1029/2005JD006290, 2006. a
Allen, M. R. and Ingram, W. J.: Constraints on future changes in climate and the hydrologic cycle, Nature, 419, 228–232, https://doi.org/10.1038/nature01092, 2002. a
Ashouri, H., Hsu, K.-L. L., Sorooshian, S., Braithwaite, D. K., Knapp, K. R., Cecil, L. D., Nelson, B. R., and Prat, O. P.: PERSIANN-CDR: Daily Precipitation Climate Data Record from Multisatellite Observations for Hydrological and Climate Studies, B. Am. Meteorol. Soc., 96, 69–83, https://doi.org/10.1175/BAMS-D-13-00068.1, 2014. a
Beck, H. E., Wood, E. F., Pan, M., Fisher, C. K., Miralles, D. G., van Dijk, A. I. J. M., McVicar, T. R., and Adler, R. F.: MSWEP V2 Global 3-Hourly 0.1∘ Precipitation: Methodology and Quantitative Assessment, B. Am. Meteorol. Soc., 100, 473–500, https://doi.org/10.1175/BAMS-D-17-0138.1, 2019. a
Becker, A., Finger, P., Meyer-Christoffer, A., Rudolf, B., Schamm, K., Schneider, U., and Ziese, M.: A description of the global land-surface precipitation data products of the Global Precipitation Climatology Centre with sample applications including centennial (trend) analysis from 1901–present, Earth Syst. Sci. Data, 5, 71–99, https://doi.org/10.5194/essd-5-71-2013, 2013. a, b
Bytheway, J. L. and Kummerow, C. D.: Inferring the uncertainty of satellite precipitation estimates in data-sparse regions over land, J. Geophys. Res.-Atmos., 118, 9524–9533, https://doi.org/10.1002/jgrd.50607, 2013. a
Chen, M. and Xie, P.: CPC Unified Gauge-based Analysis of Global Daily Precipiation, in: Western Pacific Geophysics Meeting, 29 July–1 August 2008, Cairns, Australia, 2008. a, b, c, d
Chen, M., Shi, W., Xie, P., Silva, V. B. S., Kousky, V. E., Wayne Higgins, R., and Janowiak, J. E.: Assessing objective techniques for gauge-based analyses of global daily precipitation, J. Geophys. Res.-Atmos., 113, D04110, https://doi.org/10.1029/2007JD009132, 2008. a, b, c, d
Compo, G. P., Whitaker, J. S., and Sardeshmukh, P. D.: Feasibility of a 100-year reanalysis using only surface pressure data, B. Am. Meteorol. Soc., 87, 175–190, https://doi.org/10.1175/BAMS-87-2-175, 2006. a
Contractor, S., Alexander, L. V., Donat, M. G., and Herold, N.: How Well Do Gridded Datasets of Observed Daily Precipitation Compare over Australia?, Adv. Meteorol., 2015, 1–15, https://doi.org/10.1155/2015/325718, 2015. a
Contractor, S., Donat, M. G., Alexander, L. V., Ziese, M., Meyer-Christoffer, A., Schneider, U., Rustemeier, E., Becker, A., Durre, I., and Vose, R. S.: Rainfall Estimates on a Gridded Network based on all station data v1-2019, NCI National Research Data Collection, https://doi.org/10.25914/5ca4c380b0d44, 2019a. a
Contractor, S., Donat, M. G., Alexander, L. V., Ziese, M., Meyer-Christoffer, A., Schneider, U., Rustemeier, E., Becker, A., Durre, I., and Vose, R. S.: Rainfall Estimates on a Gridded Network based on long-term station data v1-2019, NCI National Research Data Collection, https://doi.org/10.25914/5ca4c2c6527d2, 2019b. a
Contractor, S., Donat, M. G., Alexander, L. V., Ziese, M., Meyer-Christoffer, A., Schneider, U., Rustemeier, E., Becker, A., Durre, I., and Vose, R. S.: Rainfall Estimates on a Gridded Network based on all station data v1.0, NCI National Research Data Collection, https://doi.org/10.25914/5b9fa55a8298c, 2019c. a
Contractor, S., Donat, M. G., Alexander, L. V., Ziese, M., Meyer-Christoffer, A., Schneider, U., Rustemeier, E., Becker, A., Durre, I., and Vose, R. S.: Rainfall Estimates on a Gridded Network based on long-term station data v1.0, NCI National Research Data Collection, https://doi.org/10.25914/5b9fa67fce5d6, 2019d. a
Donat, M. G., Alexander, L. V., Yang, H., Durre, I., Vose, R., and Caesar, J.: Global land-based datasets for monitoring climatic extremes, B. Am. Meteorol. Soc., 94, 997–1006, https://doi.org/10.1175/BAMS-D-12-00109.1, 2013a. a
Donat, M. G., Alexander, L. V., Yang, H., Durre, I., Vose, R., Dunn, R. J. H., Willett, K. M., Aguilar, E., Brunet, M., Caesar, J., Hewitson, B., Jack, C., Klein Tank, A. M. G., Kruger, A. C., Marengo, J., Peterson, T. C., Renom, M., Oria Rojas, C., Rusticucci, M., Salinger, J., Elrayah, A. S., Sekele, S. S., Srivastava, A. K., Trewin, B., Villarroel, C., Vincent, L. A., Zhai, P., Zhang, X., and Kitching, S.: Updated analyses of temperature and precipitation extreme indices since the beginning of the twentieth century: The HadEX2 dataset, J. Geophys. Res.-Atmos., 118, 2098–2118, https://doi.org/10.1002/jgrd.50150, 2013b. a
Donat, M. G., Lowry, A. L., Alexander, L. V., O'Gorman, P. A., and Maher, N.: More extreme precipitation in the world's dry and wet regions, Nat. Clim. Change, 6, 508–513, https://doi.org/10.1038/nclimate2941, 2016. a
Durre, I., Menne, M. J., Gleason, B. E., Houston, T. G., and Vose, R. S.: Comprehensive automated quality assurance of daily surface observations, J. Appl. Meteorol. Clim., 49, 1615–1633, https://doi.org/10.1175/2010JAMC2375.1, 2010. a, b, c, d, e, f
Frei, C. and Schär, C.: A precipitation climatology of the Alps from high-resolution rain-gauge observations, Int. J. Climatol., 18, 873–900, https://doi.org/10.1002/(SICI)1097-0088(19980630)18:8<873::AID-JOC255>3.0.CO;2-9, 1998. a
Funk, C., Peterson, P., Landsfeld, M., Pedreros, D., Verdin, J., Shukla, S., Husak, G., Rowland, J., Harrison, L., Hoell, A., and Michaelsen, J.: The climate hazards infrared precipitation with stations – a new environmental record for monitoring extremes, Scient. Data, 2, 150066, https://doi.org/10.1038/sdata.2015.66, 2015. a, b
Groisman, P. Y., Knight, R. W., Easterling, D. R., Karl, T. R., Hegerl, G. C., and Razuvaev, V. N.: Trends in Intense Precipitation in the Climate Record, J. Climate, 18, 1326–1350, https://doi.org/10.1175/JCLI3339.1, 2005. a
Harris, I., Jones, P. D., Osborn, T. J., and Lister, D. H.: Updated high-resolution grids of monthly climatic observations – the CRU TS3.10 Dataset, Int. J. Climatol., 34, 623–642, https://doi.org/10.1002/joc.3711, 2014. a
Hartmann, D. L., Klein Tank, A. M., Rusticucci, M., Alexander, L. V., Brönnimann, S., Charabi, Y. A. R., Dentener, F. J., Dlugokencky, E. J., Easterling, D. R., Kaplan, A., Soden, B. J., Thorne, P. W., Wild, M., and Zhai, P.: Observations: Atmosphere and surface, in: Climate Change 2013 the Physical Science Basis: Working Group I Contribution to the Fifth Assessment Report of the Intergovernmental Panel on Climate Change, vol. 9781107057, edited by: Stocker, T. F., Qin, D., Plattner, G.-K., Tignor, M., Allen, S. K., Boschung, J., Nauels, A., Xia, Y., Bex, V., and Midgley, P. M., Cambridge University Press, Cambridge, UK and New York, NY, USA, 159–254, https://doi.org/10.1017/CBO9781107415324.008, 2013. a, b, c, d
Haylock, M. R., Hofstra, N., Klein Tank, A. M. G., Klok, E. J., Jones, P. D., and New, M.: A European daily high-resolution gridded data set of surface temperature and precipitation for 1950–2006, J. Geophys. Res., 113, D20119, https://doi.org/10.1029/2008JD010201, 2008. a, b, c, d
Herold, N., Alexander, L. V., Donat, M. G., Contractor, S., and Becker, A.: How much does it rain over land?, Geophys. Res. Lett., 43, 341–348, https://doi.org/10.1002/2015GL066615, 2016. a, b
Herrera, S., Gutiérrez, J. M., Ancell, R., Pons, M. R., Frías, M. D., and Fernández, J.: Development and analysis of a 50-year high-resolution daily gridded precipitation dataset over Spain (Spain02), Int. J. Climatol., 32, 74–85, https://doi.org/10.1002/joc.2256, 2012. a, b, c, d
Hofstra, N., Haylock, M., New, M., Jones, P., and Frei, C.: Comparison of six methods for the interpolation of daily, European climate data, J. Geophys. Res.-Atmos., 113, D21110, https://doi.org/10.1029/2008JD010100, 2008. a
Hou, A. Y., Kakar, R. K., Neeck, S., Azarbarzin, A. A., Kummerow, C. D., Kojima, M., Oki, R., Nakamura, K., and Iguchi, T.: The global precipitation measurement mission, B. Am. Meteorol. Soc., 95, 701–722, https://doi.org/10.1175/BAMS-D-13-00164.1, 2014. a
Huffman, G. J., Adler, R. F., Arkin, P., Chang, A., Ferraro, R., Gruber, A., Janowiak, J., McNab, A., Rudolf, B., and Schneider, U.: The Global Precipitation Climatology Project (GPCP) Combined Precipitation Dataset, B. Am. Meteorol. Soc., 78, 5–20, https://doi.org/10.1175/1520-0477(1997)078<0005:TGPCPG>2.0.CO;2, 1997. a
Huffman, G. J., Adler, R. F., Morrissey, M. M., Bolvin, D. T., Curtis, S., Joyce, R., McGavock, B., and Susskind, J.: Global Precipitation at One-Degree Daily Resolution from Multisatellite Observations, J. Hydrometeorol., 2, 36–50, https://doi.org/10.1175/1525-7541(2001)002<0036:GPAODD>2.0.CO;2, 2001. a
Huffman, G. J., Bolvin, D. T., Nelkin, E. J., Wolff, D. B., Adler, R. F., Gu, G., Hong, Y., Bowman, K. P., and Stocker, E. F.: The TRMM Multisatellite Precipitation Analysis (TMPA): Quasi-Global, Multiyear, Combined-Sensor Precipitation Estimates at Fine Scales, J. Hydrometeorol., 8, 38–55, https://doi.org/10.1175/JHM560.1, 2007. a
IRIN: Half million potential flood victims: WHO, available at: http://www.irinnews.org/news/2008/09/03 (last access: 29 January 2020), 2008. a
Isotta, F. A., Frei, C., Weilguni, V., Perčec Tadić, M., Lassègues, P., Rudolf, B., Pavan, V., Cacciamani, C., Antolini, G., Ratto, S. M., Munari, M., Micheletti, S., Bonati, V., Lussana, C., Ronchi, C., Panettieri, E., Marigo, G., Vertačnik, G. A. I. F., Christoph, F., Viktor, W., Melita, P. T., Pierre, L., Bruno, R., Valentina, P., Carlo, C., Gabriele, A. M. R. S., Michela, M., Stefano, M., Veronica, B., Cristian, L., Christian, R., Elvio, P., Gianni, M., and Gregor, V.: The climate of daily precipitation in the Alps: development and analysis of a high-resolution grid dataset from pan-Alpine rain-gauge data, Int. J. Climatol., 34, 1657–1675, https://doi.org/10.1002/joc.3794, 2013. a
Jackson, M. C.: Mesoscale and Small-Scale Motions As Revealed By Hourly Rainfall Maps of an Outstanding Rainfall Event: 14–16 September 1968, Weather, 32, 2–17, https://doi.org/10.1002/j.1477-8696.1977.tb04471.x, 1977. a
Jones, D. A., Wang, W., and Fawcett, R.: High-quality spatial climate data-sets for Australia, Aust. Meteorol. Oceanogr. J., 58, 233–248, 2009. a, b, c
Kalnay, E., Kanamitsu, M., Kistler, R., Collins, W., Deaven, D., Gandin, L., Iredell, M., Saha, S., White, G., Woollen, J., Zhu, Y., Chelliah, M., Ebisuzaki, W., Higgins, W., Janowiak, J., Mo, K. C., Ropelewski, C., Wang, J., Leetmaa, A., Reynolds, R., Jenne, R., and Joseph, D.: The NCEP/NCAR 40-year reanalysis project, B. Am. Meteorol. Soc., 77, 437–471, https://doi.org/10.1175/1520-0477(1996)077<0437:TNYRP>2.0.CO;2, 1996. a
Kidd, C. and Levizzani, V.: Status of satellite precipitation retrievals, Hydrol. Earth Syst. Sci., 15, 1109–1116, https://doi.org/10.5194/hess-15-1109-2011, 2011. a
Krajewski, W. F., Villarini, G., and Smith, J. A.: RADAR-Rainfall Uncertainties, B. Am. Meteorol. Soc., 91, 87–94, https://doi.org/10.1175/2009BAMS2747.1, 2010. a
Lorenz, C. and Kunstmann, H.: The Hydrological Cycle in Three State-of-the-Art Reanalyses: Intercomparison and Performance Analysis, J. Hydrometeorol., 13, 1397–1420, https://doi.org/10.1175/JHM-D-11-088.1, 2012. a
Lussana, C., Saloranta, T., Skaugen, T., Magnusson, J., Einar Tveito, O., and Andersen, J.: SeNorge2 daily precipitation, an observational gridded dataset over Norway from 1957 to the present day, Earth Syst. Sci. Data, 10, 235–249, https://doi.org/10.5194/essd-10-235-2018, 2018. a
McKee, J. L. and Binns, A. D.: A review of gauge–radar merging methods for quantitative precipitation estimation in hydrology, Can. Water Resour. J./Revue canadienne des ressources hydriques, 41, 186–203, https://doi.org/10.1080/07011784.2015.1064786, 2016. a
McMillan, H., Krueger, T., and Freer, J.: Benchmarking observational uncertainties for hydrology: rainfall, river discharge and water quality, Hydrol. Process., 26, 4078–4111, https://doi.org/10.1002/hyp.9384, 2012. a
Menendez, C. G., De Castro, M., Sorensson, A., and Boulanger, J. P.: CLARIS project: Towards climate downscaling in South America, Meteorol. Z., 19, 357–362, https://doi.org/10.1127/0941-2948/2010/0459, 2010. a
Menne, M. J., Durre, I., Vose, R. S., Gleason, B. E., and Houston, T. G.: An Overview of the Global Historical Climatology Network-Daily Database, J. Atmos. Ocean. Tech., 29, 897–910, https://doi.org/10.1175/JTECH-D-11-00103.1, 2012. a
Mitchell, T. D. and Jones, P. D.: An improved method of constructing a database of monthly climate observations and associated high-resolution grids, Int. J. Climatol., 25, 693–712, https://doi.org/10.1002/joc.1181, 2005. a, b
Osborn, T. J. and Hulme, M.: Evaluation of the European daily precipitation characteristics from the atmospheric model intercomparison project, Int. J. Climatol., 18, 505–522, https://doi.org/10.1002/(SICI)1097-0088(199804)18:5<505::AID-JOC263>3.0.CO;2-7, 1998. a
Perry, M. and Hollis, D.: The generation of monthly gridded datasets for a range of climatic variables over the UK, Int. J. Climatol., 25, 1041–1054, https://doi.org/10.1002/joc.1161, 2005. a
Peterson, T. C. and Vose, R. S.: An Overview of the Global Historical Climatology Network Temperature Database, B. Am. Meteorol. Soc., 78, 2837–2850, https://doi.org/10.1175/1520-0477(1997)078<2837:AOOTGH>2.0.CO;2, 1997. a, b
Rajeevan, M., Bhate, J., Kale, J. D., and Lal, B.: High resolution daily gridded rainfall data for the Indian region: Analysis of break and active monsoon spells, Current Science, 91, 296–306, 2006. a
Rauthe, M., Steiner, H., Riediger, U., Mazurkiewicz, A., and Gratzki, A.: A Central European precipitation climatology Part I: Generation and validation of a high-resolution gridded daily data set (HYRAS), Meteorol. Z., 22, 235–256, https://doi.org/10.1127/0941-2948/2013/0436, 2013. a
Rubel, F.: PIDCAP Quick Look Precipitation Atlas, Österr. Beitr. Meteorol. Geophys., 15, 1–95, 1996. a, b
Schamm, K., Ziese, M., Becker, A., Finger, P., Meyer-Christoffer, A., Schneider, U., Schröder, M., and Stender, P.: Global gridded precipitation over land: a description of the new GPCC First Guess Daily product, Earth Syst. Sci. Data, 6, 49–60, https://doi.org/10.5194/essd-6-49-2014, 2014. a, b, c, d, e
Schamm, K., Ziese, M., Raykova, K., Becker, A., Finger, P., Meyer-Christoffer, A., and Schneider, U.: GPCC Full Data Daily Version 1.0 at 1.0∘: Daily Land-Surface Precipitation from Rain-Gauges built on GTS-based and Historic Data, Global Precipitation Climatology Centre (GPCC, Deutscher Wetterdienst, https://doi.org/10.5676/DWD_GPCC/FD_D_V1_100, 2015. a, b, c
Schneider, U., Becker, A., Finger, P., Meyer-Christoffer, A., Ziese, M., and Rudolf, B.: GPCC's new land surface precipitation climatology based on quality-controlled in situ data and its role in quantifying the global water cycle, Theore. Appl. Climatol., 115, 15–40, https://doi.org/10.1007/s00704-013-0860-x, 2014. a
Schneider, U., Becker, A., Finger, P., Meyer-Christoffer, A., Rudolf, B., and Ziese, M.: GPCC Full Data Reanalysis Version 7.0 at 1.0∘: Monthly Land-Surface Precipitation from Rain-Gauges built on GTS-based and Historic Data, Global Precipitation Climatology Centre, Deutscher Wetterdienst, https://doi.org/10.5676/DWD_GPCC/FD_M_V7_100, 2015. a, b, c
Schneider, U., Finger, P., Meyer-Christoffer, A., Rustemeier, E., Ziese, M., and Becker, A.: Evaluating the hydrological cycle over land using the newly-corrected precipitation climatology from the Global Precipitation Climatology Centre (GPCC), Atmosphere, 8, 52, https://doi.org/10.3390/atmos8030052, 2017. a
Shen, Y., Zhao, P., Pan, Y., and Yu, J.: A high spatiotemporal gauge-satellite merged precipitation analysis over China, J. Geophys. Res.-Atmos., 119, 3063–3075, https://doi.org/10.1002/2013JD020686, 2014. a
Smith, T. M., Arkin, P. A., Ren, L., and Shen, S. S. P.: Improved Reconstruction of Global Precipitation since 1900, J. Atmos. Ocean. Tech., 29, 1505–1517, https://doi.org/10.1175/JTECH-D-12-00001.1, 2012. a
Tian, Y. and Peters-Lidard, C. D.: A global map of uncertainties in satellite-based precipitation measurements, Geophys. Res. Lett., 37, 1–6, https://doi.org/10.1029/2010GL046008, 2010. a, b
Trenberth, K. E.: Changes in precipitation with climate change, Clim. Res., 47, 123–138, https://doi.org/10.3354/cr00953, 2011. a
Trewin, B.: Exposure, instrumentation, and observing practice effects on land temperature measurements, Wiley Interdisciplin. Rev.: Clim. Change, 1, 490–506, https://doi.org/10.1002/wcc.46, 2010. a
van den Besselaar, E. J. M., van der Schrier, G., Cornes, R. C., Iqbal, A. S., and Klein Tank, A. M. G.: SA-OBS: A Daily Gridded Surface Temperature and Precipitation Dataset for Southeast Asia, J. Climate, 30, 5151–5165, https://doi.org/10.1175/JCLI-D-16-0575.1, 2017. a
Villarini, G. and Krajewski, W. F.: Sensitivity Studies of the Models of Radar-Rainfall Uncertainties, J. Appl. Meteorol. Clim., 49, 288–309, https://doi.org/10.1175/2009JAMC2188.1, 2010. a
Westra, S., Alexander, L. V., and Zwiers, F. W.: Global Increasing Trends in Annual Maximum Daily Precipitation, J. Climate, 26, 3904–3918, https://doi.org/10.1175/JCLI-D-12-00502.1, 2013. a
WHO: WHO|Floods in West Africa raise major health risks, available at: http://www.who.int/mediacentre/news/releases/2008/pr28/en/#.WweHryUc8nM.mendeley (last access: 29 January 2020), 2010. a
Xie, P., Chen, M., Yang, S., Yatagai, A., Hayasaka, T., Fukushima, Y., and Liu, C.: A Gauge-Based Analysis of Daily Precipitation over East Asia, J. Hydrometeorol., 8, 607–626, https://doi.org/10.1175/JHM583.1, 2007. a, b, c, d
Yamamoto, J. K.: An Alternative Measure of the Reliability of Ordinary Kriging Estimates, Math. Geol., 32, 489–509, https://doi.org/10.1023/A:1007577916868, 2000. a, b
Yatagai, A., Xie, P., and Alpert, P.: Development of a daily gridded precipitation data set for the Middle East, Adv. Geosci., 12, 165–170, https://doi.org/10.5194/adgeo-12-165-2008, 2008. a
Yatagai, A., Kamiguchi, K., Arakawa, O., Hamada, A., Yasutomi, N., and Kitoh, A.: APHRODITE: Constructing a Long-Term Daily Gridded Precipitation Dataset for Asia Based on a Dense Network of Rain Gauges, B. Am. Meteorol. Soc., 93, 1401–1415, https://doi.org/10.1175/BAMS-D-11-00122.1, 2012. a, b
Ziese, M., Rauthe-Schöch, A., Becker, A., Finger, P., Meyer-Christoffer, A., and Schneider, U.: GPCC Full Data Daily Version.2018 at 1.0∘: Daily Land-Surface Precipitation from Rain-Gauges built on GTS-based and Historic Data, Global Precipitation Climatology Centre, Deutscher Wetterdienst, https://doi.org/10.5676/DWD_GPCC/FD_D_V2018_100, 2018. a
- Abstract
- Introduction
- Data and methods
- Results and evaluation
- Summary, limitations and best practice recommendations for users
- Appendix A: List of countries for which the timestamps have been shifted a day back
- Data availability
- Author contributions
- Competing interests
- Acknowledgements
- Financial support
- Review statement
- References
- Abstract
- Introduction
- Data and methods
- Results and evaluation
- Summary, limitations and best practice recommendations for users
- Appendix A: List of countries for which the timestamps have been shifted a day back
- Data availability
- Author contributions
- Competing interests
- Acknowledgements
- Financial support
- Review statement
- References