the Creative Commons Attribution 4.0 License.
the Creative Commons Attribution 4.0 License.
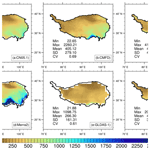
Last-decade progress in understanding and modeling the land surface processes on the Tibetan Plateau
Donghai Zheng
Fan Yang
Land surface models (LSMs) that simulate water and energy exchanges at the land–atmosphere interface are a key component of Earth system models. The Tibetan Plateau (TP) drives the Asian monsoon through surface heating and thus plays a key role in regulating the climate system in the Northern Hemisphere. Therefore, it is vital to understand and represent well the land surface processes on the TP. After an early review that identified key issues in the understanding and modeling of land surface processes on the TP in 2009, much progress has been made in the last decade in developing new land surface schemes and supporting datasets. This review summarizes the major advances. (i) An enthalpy-based approach was adopted to enhance the description of cryosphere processes such as glacier and snow mass balance and soil freeze–thaw transition. (ii) Parameterization of the vertical mixing process was improved in lake models to ensure reasonable heat transfer to the deep water and to the near-surface atmosphere. (iii) New schemes were proposed for modeling water flow and heat transfer in soils accounting for the effects of vertical soil heterogeneity due to the presence of high soil organic matter content and dense vegetation roots in surface soils or gravel in soil columns. (iv) Supporting datasets of meteorological forcing and soil parameters were developed by integrating multi-source datasets including ground-based observations. Perspectives on the further improvement of land surface modeling on the TP are provided, including the continuous updating of supporting datasets, parameter estimation through assimilation of satellite observations, improvement of snow and lake processes, adoption of data-driven and artificial intelligence methods, and the development of an integrated LSM for the TP.
- Article
(8347 KB) - Full-text XML
- BibTeX
- EndNote
Land surface models (LSMs) are a key component of Earth system models, which simulates the water and energy exchanges at the land–atmosphere interface (Dickinson et al., 2006; Hurrell et al., 2013). Moreover, the LSM is a powerful tool to provide a better understanding of the interactions among hydrological, ecological, and biogeochemical processes (Pan et al., 2012). Over the past decades, the LSMs have evolved from a simple bucket model (Manabe, 1969) to more sophisticated model systems (Dai et al., 2003; Dickinson et al., 1993; Oleson et al., 2010; Sellers et al., 1986, 1996) that have incorporated many physical and physiological processes occurring in the atmosphere–snow–vegetation–soil–aquifer system. Meanwhile, several offline multi-model intercomparison projects have been conducted to identify the strength and weakness of LSMs, such as the Project for the Intercomparison of Land-Surface Parameterization Schemes (PILPS) (Henderson-Sellers et al., 1995, 1993), the Global Soil Wetness Project (GSWP) (Dirmeyer et al., 1999; Dirmeyer, 2011), and the African Monsoon Multidisciplinary Analysis (AMMA) Land Surface Model Intercomparison Project (ALMIP) (Boone et al., 2009). A general conclusion is that there are still big differences among various LSMs, and none of them outperforms others for all conditions. Jimenez et al. (2011) and Xia et al. (2014) also reported that large discrepancies exist between various LSM simulations even when driven with the same meteorological forcing. Therefore, further improvement of LSMs remains imperative.
The Tibetan Plateau (TP), well-known as the Third Pole and the Asian water tower, shows very strong interactions among its hydrosphere, cryosphere, biosphere, and atmosphere that manifest TP's impact on surrounding regions and regional or global climate system (Wu et al., 2015; Yao et al., 2015). The land surface processes in the TP thus show unique features due to the multispherical interactions. The land–atmospheric exchange on the TP is generally intense due to strong solar radiation, which is further considerably regulated by the complex terrain. Typical processes of the cryosphere (such as glacier, soil freeze–thaw, and snow processes), lake–air interactions, and vertical soil heterogeneity coexist and consist of the TP's complex land surface processes that are not well represented by current LSMs. The coupled land–atmospheric numerical experiments (Gao et al., 2015; Zhao et al., 2018) demonstrate also the important role of the TP's land surface processes on affecting the simulations of atmospheric processes and variables. These numerical experiments further highlight the necessity to improve current LSMs to better represent the TP's complex land surface processes.
After Yang et al. (2009) identified key issues in the understanding and modeling of land surface processes on the TP, many endeavors have been made to evaluate and validate the performance of current LSMs, as well as to identify the model deficiencies and to propose solutions (L. Wang et al., 2016; Zheng et al., 2017b, 2016). Based on the evaluation results, the LSMs have been improved from two aspects. One is to improve the existing schemes, such as improvement of parameter values (Dai et al., 2013; Shangguan et al., 2014, 2013, 2017), development of more realistic and reliable process representations (Wang and Yang, 2018; W. Wang et al., 2020), and usage of better mathematic solutions (Wang et al., 2017; Luo et al., 2018). The other is to extend modeling capability through adding new schemes and functions that are missing or ignored in current LSMs (Chen et al., 2012; Zheng et al., 2015a, b; Zhu et al., 2017). In addition, new meteorological forcing datasets have also been developed by integrating multi-source datasets including ground-based observations (He et al., 2020).
To the best of our knowledge, there is no systematic review of the last decade's progress in understanding and modeling the land surface processes on the TP. The presented topics in this paper cover the new development of land surface schemes for the TP (Sect. 2) and the supporting datasets such as meteorological forcing and soil datasets (Sect. 3). Finally, perspectives on the further improvement of land surface modeling on the TP are given (Sect. 4).
2.1 Cryosphere processes
Deficiencies in representing soil freeze–thaw and glacier and snow processes in cold and high mountainous regions are the main sources of uncertainties in applying the LSMs to the TP's cryosphere. Land surface processes in the cryosphere, including the phase change of water and water–heat interactions, are more complex than those in unfrozen areas. Zheng et al. (2017b) showed that the rates of water phase change are different in freezing and thawing periods, and the concept of phase change efficiency is introduced for improving the simulation of soil freeze–thaw processes as confirmed by Yang et al. (2018). A major advance in representing the transition process of water phase change on the TP is the introduction of enthalpy in the energy governing equations for heat transfer. The introduction of enthalpy not only makes the governing equation simpler but also makes the solution more stable. Enthalpy was used as a prognostic variable instead of temperature to simulate snow accumulation and melting (Sun et al., 1999), lake ice formation and melting (Sun et al., 2007), as well as soil freeze–thaw processes (Li and Sun, 2008). Based on these pioneer practices, Bao et al. (2016) developed a frozen soil scheme, and Wang et al. (2017) further coupled this scheme with a snow scheme originally from Sun et al. (1999), in which enthalpy was adopted as a prognostic variable instead of snow–soil temperature for simulating heat transfer. The coupled model of Wang et al. (2017) was proven to be able to realistically capture the measured soil–snow water and temperature dynamics on the TP, confirming the advantage of adopting the enthalpy as the prognostic variable for snow and frozen soil simulations.
The enthalpy concept was also applied to the development of a glacier mass balance model, i.e., the Water and Enthalpy Budget-based Glacier mass balance Model (WEB-GM) (Ding et al., 2017). The WEB-GM contains a dynamic scheme to judge precipitation type (rain, sleet, snow) based on wet-bulb temperature, relative humidity, and elevation (Ding et al., 2014), a new albedo scheme to consider the impacts of shallow snow, and a parameterization scheme for turbulent heat transfer (Yang et al., 2008). Compared to the traditional temperature-based glacier mass balance model (i.e., Fujita model), the WEB-GM model performs better in simulating glacier surface temperature, turbulent heat fluxes, and glacier mass balance at the Parlung No. 4 Glacier in the southeast TP (Fig. 1).
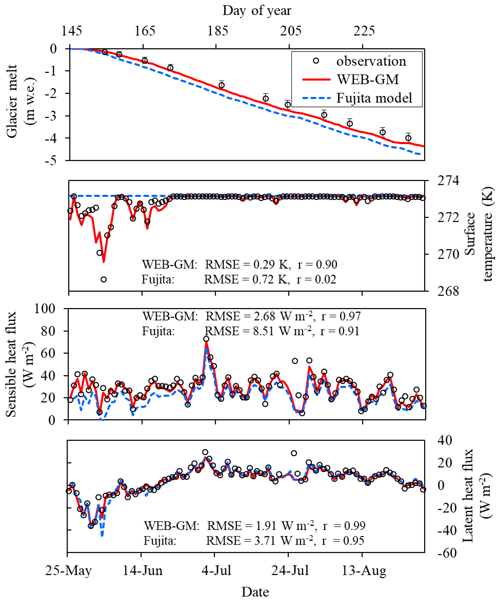
Figure 1Comparisons of the WEB-GM and Fujita model in simulating glacier melt (a), surface temperature (b), sensible (c), and latent heat fluxes (d) at the Parlung No. 4 Glacier. The figure is reproduced from Ding et al. (2017).
Since soil water flow and heat transport are strongly coupled in the freeze–thaw transition processes, Wang and Yang (2018) incorporated a fully coupled soil water–heat transport scheme into the CLM4.5 model, whereby the interaction between soil water flow and heat transport, as well as the soil water vapor diffusion process, is considered. The results show that the measured frozen soil dynamics (e.g., soil moisture and temperature) in the TP are better captured in comparison to the original isothermal scheme, which also highlight the importance of considering the process of water vapor diffusion in cold regions that is generally neglected in current LSMs. Considering that the snow cover on the TP is generally thin (∼ a few centimeters) and therefore the subsurface albedo has a large influence on the snow surface albedo, a new snow albedo scheme was developed recently, with which simulations of snow ablation were improved (W. Wang et al., 2020).
2.2 Lake processes
In recent years, modeling of lake–air interactions has drawn more and more attention, and hydrometeorological models such as WRF and CLM have included lake models as a component. The WRF-lake model generally performs well for shallow lakes (Gu et al., 2015). However, Fang et al. (2017) showed that the WRF-lake model performs poorly when applied to Lake Nam Co, a deep lake, on the TP. Compared to the in situ measurements, the simulated lake surface temperature is too low, the lake ice appears too early, and the seasonality of lake temperature in deep water is too smooth. These biases indicate that the WRF-lake model underestimates the process of strong vertical mixing between surface water and deep water. On the other hand, Lazhu et al. (2016) found that a semiempirical lake model, FLake, can reproduce well the measurements of surface temperature, surface turbulent fluxes, and ice phenology in the Lake Nam Co. The performance evaluations of the above two models indicate that a semiempirical model like FLake may be superior for the application to deep lakes on the TP. The FLake simulations also showed that the actual lake evaporation is less than the potential evaporation that is traditionally used as a surrogate of the former (Lazhu et al., 2016). Huang et al. (2019) also compared the performance of FLake, WRF-lake, and CoLM-lake models for their applications to Lake Nam Co, which further investigated the sensitivity of lake models to several key parameters, such as light extinction coefficient, turbulent mixing, and temperature of maximum water density. The results demonstrated that the simulations can be largely improved via model calibrations.
Based on the above model evaluations, lake models have been improved through better representing the processes of lake–air interaction and lake interior vertical mixing on the TP. Based on in situ measurements, B. Wang et al. (2015) evaluated the performance of two lake–air exchange models in the small Lake Nam Co and showed that the simulated surface heat fluxes can be enhanced by increasing the values of Charnock and roughness Reynolds numbers from 0.013 and 0.11 to 0.031 and 0.54, respectively. Zhang et al. (2019) developed a new scheme to improve vertical mixing in the lake interior based on a K-profile parameterization (KPP) associated with internal wave activity and shear instability. The KPP was incorporated into the CLM lake model (CLM-KPP), which represents better the transition between stratification and turnover and effectively reproduces strong mixing between the lake surface and deep water during windy conditions, leading to improved simulation of water surface temperature in comparison to the original model (CLM-ORG) (Fig. 2).
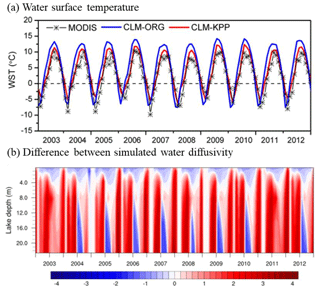
Figure 2Comparisons of the original (CLM-ORG) and revised (CLM-KPP) CLM lake models in simulating water surface temperature (WST) (a) and their differences in simulating water diffusivity (log10Kw) (b) in Lake Nam Co. The figure is reproduced from Zhang et al. (2019).
In addition to the improvements in those general processes, lake models were developed to consider some typical processes for the TP lakes. Dai et al. (2018) developed the CoLM-Lake with a focus on freezing and thawing transition of lakes, which was tested at 10 lakes worldwide, and the results show that the model can perform well if model parameters are calibrated. Considering that there are a number of salt lakes in the TP, Su et al. (2019) incorporated the salinity effects on the temperatures of maximum water density and freezing point into the FLake model and validated its performance in the brackish endorheic Qinghai Lake. The results showed that consideration of salinity effects reduces the simulated maximum ice thickness and advances the break-up date, which are closer to the in situ buoy measurements.
2.3 Soil subsurface processes
Soil subsurface processes in the TP are largely affected by the vertical heterogeneity. The vertical stratification is caused by the presence of high soil organic matter (SOM) content and dense vegetation roots in surface soils (Yang et al., 2005; Zheng et al., 2015a, b) or gravel (Yi et al., 2018) in soil columns. Their existences considerably influence soil thermal and hydraulic properties and therefore soil state and land surface fluxes.
The existence of SOM in the surface soils leads to lower thermal conductivity and larger heat capacity (Zheng et al., 2015b; Chen et al., 2012). Chen et al. (2012) evaluated three widely used thermal conductivity schemes (e.g., Farouki, 1981; Johansen, 1975; Yang et al., 2005) implemented by current LSMs using laboratory measurements. The results showed that all three schemes overestimate the measured thermal conductivity for Tibetan soils including SOM content, and the scheme of Yang et al. (2005) was further modified to include the SOM effect that reduces the noted overestimations (Fig. 3). Similar work was done by Zheng et al. (2015b) to incorporate the SOM effect into the scheme of Johansen (1975). Luo et al. (2017) showed that consideration of SOM effect in the thermal scheme can also improve the soil temperature simulations produced by the CLM4.5 model for two Tibetan grassland sites. Zheng et al. (2015b) further demonstrated that consideration of SOM effect tends to produce larger sensible heat flux and smaller latent heat flux, and the amplitude of the diurnal surface temperature cycle is increased.
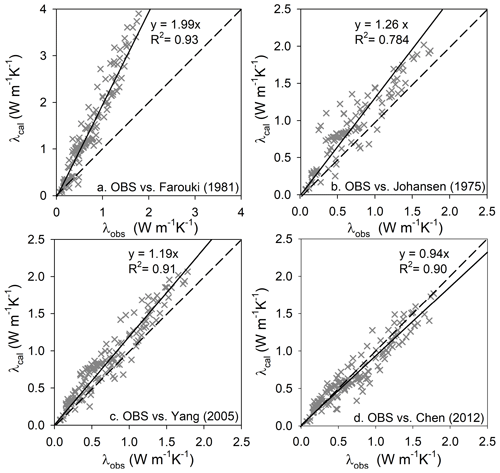
Figure 3Scatterplots of laboratory-measured (OBS, shown on the x axis) and estimated thermal conductivity produced by the schemes of Farouki (1981) (a), Johansen (1975) (b), Yang et al. (2005) (c), and Chen et al. (2012) (d) for four alpine grassland stations. The figure is reproduced from Chen et al. (2012).
The existence of SOM in the surface soils also increases soil porosity and water-holding capacity (Chen et al., 2012; Zheng et al., 2015a). Previous studies (Chen et al., 2013; C. W. Li et al., 2018, 2017; Su et al., 2013) have shown that current LSMs generally underestimate the surface soil moisture in the TP by ignoring the SOM effect in the hydraulic parameterization. Chen et al. (2012) and Zheng et al. (2015a) showed that the presence of SOM content in the surface layer of Tibetan soils leads to larger porosity and higher water-holding capacity, and the saturated hydraulic conductivity is found exponentially decreased with soil depth (Zheng et al., 2015a). Accordingly, a new hydraulic parameterization was developed by Zheng et al. (2015a) to include the above SOM effect, which reduces the noted underestimation of surface soil moisture under wet conditions. However, an overestimation of surface soil moisture was noted for the drying period, which can be addressed by implementing either the exponential or asymptotic vertical root distribution function that is able to represent the presence of abundant roots in the surface layer (Zheng et al., 2015a, c). Besides affecting the water uptake, Gao et al. (2015) inferred that the presence of the rhizosphere in the surface layer could reduce the saturated hydraulic conductivity and infiltration that increases the water-holding capacity, which can also mitigate the dry biases noted for the simulation of surface soil moisture on the TP.
The existence of gravels is another characteristic that should be considered in some part of the TP (Yi et al., 2018), in which soils are usually not well-developed and large gravels (particle size ≥2 mm) may occupy considerable volumes. The existence of gravels tends to decrease the water-holding capacity and increase the hydraulic conductivity and thermal conductivity. Laboratory experiments were conducted by Yi et al. (2018) that for the first time the impact of gravels on soil hydraulic and thermal parameters as well as the soil water content and frozen soil depth was quantified. A new soil hydraulic and thermal scheme was also developed by Pan et al. (2017) for simulating the freeze–thaw process in gravel-containing soils. The scheme was incorporated into the CLM4.0 model that captures well the measured freeze–thaw dynamics in two typical Tibetan sites that contain different levels of gravel content in the soil columns.
2.4 Hydrological processes
The understanding and modeling of hydrological characteristics on the TP, such as the runoff seasonality and parameterization of subsurface and groundwater flow, was also much improved. Bai et al. (2016) evaluated the performance of Global Land Data Assimilation System (GLDAS) products in representing the streamflow dynamics of five Tibetan river basins based on the gauged measurements. It demonstrated that the four LSMs (CLM, VIC, Noah, and MOSAIC) implemented by the GLDAS capture well the trend of annual streamflow dynamics but fail to reproduce the seasonal pattern such as the timing of peak flow. Zheng et al. (2018, 2016) showed that the seasonality of the runoff regime in the eastern Tibetan river basins is mainly controlled by the soil freeze–thaw mechanism (Fig. 4a–b). An annual hysteresis loop between measured or simulated runoff and precipitation was also reported (Fig. 4c–d), which is related to both the amount and the state of water stored in the soils. Zheng et al. (2017a) further showed that the soil water storage-based runoff parameterizations outperform the groundwater table-based schemes in representing the runoff dynamics in the seasonally frozen Tibetan river basins as confirmed by Yuan et al. (2018). Both Bai et al. (2016) and Zheng et al. (2017a) also highlight the importance of including groundwater and lateral flow schemes in current LSMs. Recently, a groundwater scheme (Xie et al., 2012; Y. Zeng et al., 2016; Y. J. Zeng et al., 2016; Xie et al., 2018) has been developed and coupled into the CLM4.5 model, and the results demonstrated that the scheme can simulate well the groundwater level and lateral flow. Nevertheless, it is still a big challenge to simulate well the hydrological processes across all the Tibetan rivers due to the lack of gauged measurements (including precipitation and streamflow) in most parts of the TP.
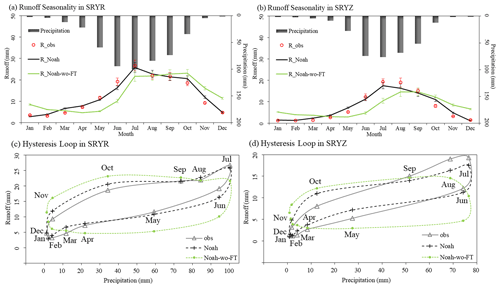
Figure 4Monthly mean measured (R_obs) and simulated runoff (a, b) and annual precipitation–runoff hysteresis loops (c, d) plotted from measured and simulated runoff produced by the improved Noah LSM with (Noah) and without (Noah-wo-FT) the soil freeze–thaw mechanism for the source regions of Yellow (SRYR, 1984–2009) (a, c) and Yangtze rivers (SRYZ, 1984–2005) (b, d). The figure is reproduced from Zheng et al. (2018).
2.5 Representation of subgrid topographic effects
The TP is characterized by high elevation and complex terrain, which exert obvious subgrid topographic effects on radiative and moment transfer processes. A 3D Monte Carlo photon tracing program for radiation transfer was developed by Liou et al. (2007) and applied to simulate the surface solar fluxes on the TP. It was found that the inclusion of subgrid variability can lead to a significant solar flux deviation from the conventional smoothed topography simulation. Lee et al. (2013) further improved this 3D model and applied it to investigate the topographic effect on surface radiative energy budget on the TP. The results demonstrated that the TP would receive more solar flux that leads to stronger convection and enhanced snowmelt rate when the 3D topographic effects were considered. Recently, Lee et al. (2019) investigated the climate effects of the 3D radiation–topography interactions by incorporating their model into the Community Climate System Model version 4 (CCSM4). After including this topographic effect, the energy budget and air temperature simulated by the CCSM4 could be significantly improved. Gu et al. (2020) also coupled a subgrid terrain radiative forcing scheme into the Regional Climate Model Version 4.1 (RegCM4.1). They found that adopting this scheme in the RegCM4.1 could produce a better simulation of the energy budget on the TP, which thus led to a better simulation of East Asian summer monsoon and precipitation over China.
Representing the effect of subgrid terrain variability on the moment transfer is very important for improving the simulation of wind speed, water vapor transfer, and precipitation over the TP. Wang et al. (2017) demonstrated that water vapor transfer over the southern Tibetan Plateau is overestimated by current climate models. Lin et al. (2018) also found similar biases in WRF simulations and demonstrated that the complex terrain of the Himalaya can retard water vapor transfer through exerting an orographic drag on the atmosphere. Han et al. (2015) suggested an aerodynamic length of about 10 m is needed to reflect the drag, which, however, is difficult to apply such a “big number” directly to the framework of Monin–Obukhov similarity theory. Alternatively, Zhou et al. (2018, 2019) implemented a turbulent orographic form drag scheme (Beljaars et al., 2004) in the WRF model, which reduce the positive biases in the surface wind simulation, leading to the reduction of water vapor transfer from the surroundings to the TP. This parameterization is also critical for the successful simulation of precipitation distribution from the low altitudes to high latitudes of the central Himalaya (Y. Wang et al., 2020).
Offline simulation is generally adopted to test model performance, and meteorological forcing, as well as static land parameter datasets, is required for running the LSM. There are several global forcing datasets dedicated to driving the LSMs, such as GLDAS (Rodell et al., 2004), ERA-Interim (Dee et al., 2011), and Modern-Era Retrospective Analysis for Research and Applications (MERRA) (Gelaro et al., 2017; Rienecker et al., 2011). Wang and Zeng (2012) compared the performance of several precipitation datasets derived from GLDAS, MERRA, NCEP/NCAR-1, CFSR, ERA-40, and ERA-Interim for their applications to the TP. The results showed that the GLDAS performs better than other datasets due to the fact that the GLDAS has merged products from the ground- and satellite-based measurements. However, the development of these datasets only utilized measurements from a limited number of Chinese weather stations (∼200 stations), and their performance can be improved if more ground-based measurements are used (Wang and Zeng, 2012; A. H. Wang et al., 2016; W. Wang et al., 2016). On the other hand, the global land parameter datasets such as the soil data derived from the FAO Soil Map (FAO, 2003), Harmonized World Soil Database (HWSD) (FAO/IIASA/ISRIC/ISS-CAS/JRC, 2012), and SoilGrid (Hengl et al., 2014) show similar problems. Accordingly, several meteorological forcing and soil datasets have been developed specifically for mainland China via merging more available ground-based measurements during the last decade. These improved forcing data and other supporting data generally outperform the corresponding global dataset not only in accuracy but also in both spatial and temporal resolution, which make a finer-scale land surface simulation possible and contribute to substantial improvements of land surface modeling in China.
3.1 Improvement of meteorological forcing data
The accuracy of LSM simulations highly depends on the quality of meteorological forcing data. Among them, the accuracy of precipitation and radiation data is more often addressed. For lake simulations, particularly for modeling lake ice, it is also crucial to provide accurate information on wind speed.
Table 1 lists several commonly used precipitation datasets in China. Among them, the CN05.1 was produced by China Meteorological Administrator through the interpolation from more than 2000 gauge stations over mainland China (Wu and Gao, 2013). The China Meteorological Forcing Dataset (CMFD) was developed by the Institute of Tibetan Plateau Research, Chinese Academy of Sciences (ITPCAS), via merging a variety of data sources including reanalysis data, satellite-based products, and ground-based measurements (He et al., 2020; Yang and He, 2019). Figure 5 shows the comparison of mean annual precipitation derived from the six forcing datasets for the TP during the period between 1985 and 2014. Large differences can be noted among the six precipitation datasets. According to the CN05.1 that produced based on the interpolation of ground-based measurements, the mean annual precipitation is about 410 mm on the TP with a larger amount in the southeast and smaller amount in the northwest. Compared to the CN05.1, both the ERA-Interim and MERRA2 largely overestimate the precipitation in the southeastern part of the Himalaya, and the precipitation in the southeastern part of the TP is generally overestimated by the MERRA2. On the contrary, the GLDAS-1 underestimates the precipitation in the southeastern part of the TP, which is resolved in the GLDAS-2. However, the overestimation of precipitation in the central part of Himalaya can be noted for the GLDAS-2. Overall, the CMFD integrating the ground-based measurements produces spatial distribution and variation of precipitation comparable to the CN05.1. The reliability of the CMFD precipitation data has also been validated by other studies (He et al., 2015; Xie et al., 2017; Yang et al., 2017). Yang et al. (2017) evaluated the precipitation data of the CMFD, CN05.1, and GLDAS using independent ground-based measurements collected by the China Ministry of Water Resources (MWR). The results demonstrated that the CMFD generally outperforms the CN05.1 and GLDAS in capturing the spatial and temporal variability of precipitation in China. Similar findings were also reported by He et al. (2015) for their applications to the upper reach of Heihe River basin.
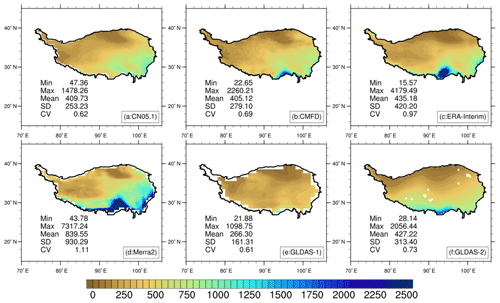
Figure 5Spatial distribution of mean annual precipitation derived from six forcing datasets for the Tibetan Plateau (1985–2014): (a) CN05.1, (b) CMFD, (c) ERA-Interim, (d) MERRA2, (e) GLDAS version1, and (f) GLDAS version 2. Statistic values, such as minimum (Min), maximum (Max), mean (Mean), standard deviation (SD), and coefficient of variation (CV), are also shown in each plot.
Until now, the CMFD forcing data have been adopted in hundreds of research publications with respect to land surface simulations, ecological modeling, and climate change studies. For instance, the data were used to (i) improve the land surface temperature simulation in China (Chen et al., 2011), (ii) simulate the distribution of permafrost and seasonal frozen soil on the TP (Guo and Wang, 2013), (iii) drive the LSM to obtain a high spatial resolution simulation of soil moisture on the TP (C. W. Li et al., 2018), and (iv) drive a land data assimilation system to improve the soil moisture simulation in China (Yang et al., 2020, 2016).
3.2 Improvement of static land parameter data
Recently, a series of land parameter datasets have been developed for running the LSMs by the Land-Atmosphere Interaction Research Group at Beijing Normal University and Sun Yat-sen University available from http://globalchange.bnu.edu.cn/research/data (last access: 1 November 2020). These datasets include (i) a soil particle-size distribution dataset of China (Shangguan et al., 2012), (ii) a China dataset of soil properties (Shangguan et al., 2013), (iii) a China dataset of soil hydraulic parameters (Dai et al., 2013), (iv) a Global Soil Dataset for use in Earth System Models (GSDE) (Shangguan et al., 2014), (v) a global depth to bedrock dataset (Shangguan et al., 2017), (vi) a global dataset of soil hydraulic and thermal parameters (Dai et al., 2019), and (vii) a reprocessed global leaf area index dataset (Yuan et al., 2011). Among these datasets, the GSDE has been widely used, which provides soil information including soil texture, organic carbon, and bulk density at a spatial resolution of 30 arcsec for eight vertical soil layers to a depth of 2.3 m.
Figures 6 and 7 show respectively the spatial distribution of bulk density and clay content on the TP derived from the GSDE and two other widely used soil datasets, i.e., the HWSD and SoilGrid provided by the ISRIC (International Soil Reference and Information Centre). Large differences can be also noted among the three soil datasets. Compared to the GSDE, which was produced based on more soil samples taken from China, the SoilGrid tends to provide larger values in the northwest and smaller values in the southeast for the bulk density, and the HWSD tends to provide lower values of clay content. Since soil hydraulic parameters are closely related to the bulk density and clay content, different values of bulk density and clay content derived from different soil datasets would affect the soil hydraulic properties and thus the water budget simulation by the LSMs. For instance, J. D. Li et al. (2018) investigated the impact of soil texture on the LSM simulations over the central Tibetan Plateau, in which the FAO Soil Map and GSDE were used. The results showed that soil parameters play a dominative role in simulating energy and water fluxes. Therefore, additional efforts are needed to investigate the accuracy of these newly developed soil datasets, as well as to investigate their impacts on the LSM simulations.
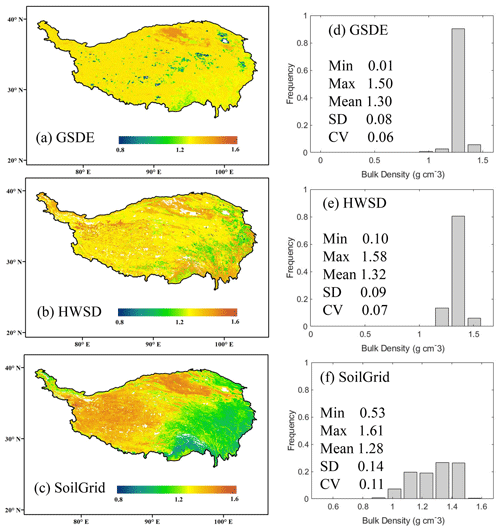
Figure 6Spatial distribution of soil bulk density on the TP derived from (a) GSDE, (b) HWSD, and (c) SoilGrid. Corresponding histograms are also shown in (d)–f).
Besides the abovementioned improved forcing and soil datasets, other remote sensing or reanalysis datasets have also been widely used for land surface modeling on the TP, especially in ungauged or poor-gauged areas. They were used to drive hydrological simulation (S. Wang et al., 2015; Tong et al., 2014; Qi et al., 2018) or land data assimilation system (Lu et al., 2012) to validate model simulation (Li et al., 2020) and to improve understanding of physical processes (Liu et al., 2018). On the other hand, current in situ observation networks also made contributions to understanding the land surface process by serving as calibration and validation sources. For example, the multiscale soil moisture and freeze–thaw monitoring network on the central TP (CTP-SMTMN) (Yang et al., 2013) and the Tibetan Plateau observatory of plateau-scale soil moisture and soil temperature (Tibet-Obs) (Su et al., 2011) provide a valuable dataset to validate and calibrate remote sensing products and model simulations. However, the density of in situ observation networks on the TP is still very sparse. Consequently, integration of the ground observation, remote sensing products, and reanalysis data may provide a complete and reliable supporting dataset for studying the land surface processes on the TP.
After Yang et al. (2009) identified key issues in the understanding and modeling of land surface processes on the TP, much progress has been made in developing new land surface schemes and supporting datasets in the past decade. The main advances are summarized in this mini-review:
-
(i) an enthalpy-based approach was adopted to enhance the description of cryosphere processes such as glacier and snow mass balance and soil freeze–thaw transitions;
-
(ii) parameterization of vertical mixing process was improved in lake models to ensure reasonable heat transfer into deep water;
-
(iii) new schemes were proposed for modeling water flow and heat transfer in the soils accounting for the effect of vertical soil heterogeneity due to the presence of high soil organic matter content and dense vegetation roots in surface soils or gravel in soil columns;
-
(iv) supporting datasets of meteorological forcing and soil parameter datasets were developed by integrating more ground-based measurements.
Based on the review, the following research perspectives in land surface modeling on the TP are given.
-
Continuous improvement of meteorological forcing and land parameter datasets. The quality of current datasets is generally good for eastern China but not so reliable for the Tibetan Plateau and northwestern China, because of limited access to station data. With the progress in data sharing policy, it is possible to merge more station data from these remote regions. In addition, new experimental activities (e.g., the Second Scientific Expedition to the Tibetan Plateau and the Third Atmospheric Science Experiment on the Tibetan Plateau) may provide new observations to improve and validate the quality of the forcing datasets. Also, high-resolution modeling can provide a new meteorological data source to improve the data quality in regions with complex terrain (Li et al., 2020; Y. Wang et al., 2020).
-
Estimation of land parameters at model grid scale through data assimilation and/or multi-objective optimization. Parameter upscaling faces big challenges with field sampling. By contrast, satellite data can avoid this kind of issues, although the remote sensing products may also encounter uncertainties. Satellite data assimilation and/or multi-objective optimization may provide a new feasible way to estimate model parameters. A recent work (Luo et al., 2020) proves the concept, showing inverse estimation with in situ data is indeed able to reproduce the spatial pattern of soil organic carbon content measured in a mesoscale area of the TP, and some pioneering studies (Corbari et al., 2015; Pinnington et al., 2018; Yang et al., 2016) show the potential of this type of methods.
-
Development of new schemes and integration of multiple schemes into a single LSM. Some parameterizations are still questionable or even excluded, such as rhizosphere effect, complex terrain, and high-elevation lakes, which are typical for the TP. For example, the parameterization of the light extinction coefficient and turbulent mixing in lakes are critical to freeze-up simulation, but their values are empirically given (Huang et al., 2019). Meanwhile, since the vegetation types on the TP have unique characteristics and are very sensitive to climate change, the parameterization of vegetation-related processes should be improved as demonstrated by Li et al (2019). The role of these processes should be understood and parameterized with new observations. Coupling new schemes into a LSM can increase the diversity of the LSM community and thus better quantify the uncertainties of LSMs.
-
Adoption of data-driven methods and artificial intelligence in land surface process study. Big-data methods could provide vast data and clues in model development, parameter optimization, and so on (Guo, 2017), while artificial intelligence could help in process understanding (Reichstein et al., 2019). As demonstrated by Jing et al. (2020), the integration of physical process-based models with data-driven approaches shows high potential to improve the understanding and simulation of land surface processes.
All data that were used were cited in Sect. 3. The CMFD is available at http://dx.doi.org/10.11888/AtmosphericPhysics.tpe.249369.file (Yang and He, 2019). CN05.1 data are available from Xuejie Gao (gaoxj@cma.gov.cn) upon reasonable request. The ERA-Interim datasets are distributed by ECMWF (Dee et al., 2011), available at http://apps.ecmwf.int/datasets/. GLDAS data are free from https://disc.gsfc.nasa.gov/datasets?page=1&keywords=GLDAS (Rodell et al., 2004). MERRA2 data are available from https://disc.gsfc.nasa.gov/datasets?keywords=MERRA-2&page=1 (Gelaro et al., 2017). The HWSD is available at http://webarchive.iiasa.ac.at/Research/LUC/External-World-soil-database/HTML (FAO/IIASA/ISRIC/ISS-CAS/JRC, 2012). The GSDE is available from http://globalchange.bnu.edu.cn/research/data (Shangguan et al., 2014).
HL and KY conceptualized the work. HL drafted the paper. DZ and KY revised the paper. HL, DZ, KY, and FY collected data and contributed to analyses and discussions.
The authors declare that they have no conflict of interest.
The authors would like to thank Xuejie Gao, Baohong Ding, Yingying Chen, Wei Shangguan, and Jiming Jin for their assistance.
This research has been supported by the National Key Research and Development Program of China (grant no. 2017YFA0603703), the National Natural Science Foundation of China (grant no. 91747101), the Second Tibetan Plateau Scientific Expedition and Research Program (STEP) (grant no. 2019QZKK0206), and the Strategic Priority Research Program of Chinese Academy of Sciences (grant no. XDA20100103).
This paper was edited by Xing Yuan and reviewed by three anonymous referees.
Bai, P., Liu, X. M., Yang, T. T., Liang, K., and Liu, C. M.: Evaluation of streamflow simulation results of land surface models in GLDAS on the Tibetan plateau, J. Geophys. Res.-Atmos., 121, 12180–12197, 2016.
Bao, H., Koike, T., Yang, K., Wang, L., Shrestha, M., and Lawford, P.: Development of an enthalpy-based frozen soil model and its validation in a cold region in China, J. Geophys. Res.-Atmos., 121, 5259–5280, https://doi.org/10.1002/2015jd024451, 2016.
Beljaars, A. C. M., Brown, A. R., and Wood, N.: A new parametrization of turbulent orographic form drag, Q. J. Roy. Meteor. Soc., 130, 1327–1347, https://doi.org/10.1256/qj.03.73, 2004.
Boone, A., de Rosnay, P., Balsamo, G., Beljaars, A., Chopin, F., Decharme, B., Delire, C., Ducharne, A., Gascoin, S., Grippa, M., Guichard, F., Gusev, Y., Harris, P., Jarlan, L., Kergoat, L., Mougin, E., Nasonova, O., Norgaard, A., Orgeval, T., Ottle, C., Poccard-Leclercq, I., Polcher, J., Sandholt, I., Saux-Picart, S., Taylor, C., and Xue, Y. K.: The Amma Land Surface Model Intercomparison Project (Almip), B. Am. Meteorol. Soc., 90, 1865–1880, 2009.
Chen, Y. Y., Yang, K., He, J., Qin, J., Shi, J. C., Du, J. Y., and He, Q.: Improving land surface temperature modeling for dry land of China, J. Geophys. Res.-Atmos., 116, D20104, https://doi.org/10.1029/2011JD015921, 2011.
Chen, Y. Y., Yang, K., Tang, W. J., Qin, J., and Zhao, L.: Parameterizing soil organic carbon's impacts on soil porosity and thermal parameters for Eastern Tibet grasslands, Sci. China Earth Sci., 55, 1001–1011, 2012.
Chen, Y. Y., Yang, K., Qin, J., Zhao, L., Tang, W. J., and Han, M. L.: Evaluation of AMSR-E retrievals and GLDAS simulations against observations of a soil moisture network on the central Tibetan Plateau, J. Geophys. Res.-Atmos., 118, 4466–4475, 2013.
Corbari, C., Mancini, M., Li, J., and Su, Z.: Can satellite land surface temperature data be used similarly to river discharge measurements for distributed hydrological model calibration?, Hydrolog. Sci. J., 60, 202–217, https://doi.org/10.1080/02626667.2013.866709, 2015.
Dai, Y., Wei, N., Huang, A., Zhu, S., Shangguan, W., Yuan, H., Zhang, S., and Liu, S.: The lake scheme of the Common Land Model and its performance evaluation, Chinese Sci. Bull., 63, 3002–3021, 2018.
Dai, Y., Xin, Q., Wei, N., Zhang, Y., Wei, S., Yuan, H., Zhang, S., Liu, S., and Lu, X.: A Global High-Resolution Data Set of Soil Hydraulic and Thermal Properties for Land Surface Modeling, J. Adv. Model. Earth Sy., 11, 2996–3023, https://doi.org/10.1029/2019ms001784, 2019.
Dai, Y. J., Zeng, X. B., Dickinson, R. E., Baker, I., Bonan, G. B., Bosilovich, M. G., Denning, A. S., Dirmeyer, P. A., Houser, P. R., Niu, G. Y., Oleson, K. W., Schlosser, C. A., and Yang, Z. L.: The Common Land Model, B. Am. Meteorol. Soc., 84, 1013–1023, 2003.
Dai, Y. J., Shangguan, W., Duan, Q. Y., Liu, B. Y., Fu, S. H., and Niu, G.: Development of a China Dataset of Soil Hydraulic Parameters Using Pedotransfer Functions for Land Surface Modeling, J. Hydrometeorol., 14, 869–887, 2013.
Dee, D. P., Uppala, S. M., Simmons, A. J., Berrisford, P., Poli, P., Kobayashi, S., Andrae, U., Balmaseda, M. A., Balsamo, G., Bauer, P., Bechtold, P., Beljaars, A. C. M., van de Berg, L., Bidlot, J., Bormann, N., Delsol, C., Dragani, R., Fuentes, M., Geer, A. J., Haimberger, L., Healy, S. B., Hersbach, H., Hólm, E. V., Isaksen, L., Kållberg, P., Köhler, M., Matricardi, M., McNally, A. P., Monge-Sanz, B. M., Morcrette, J.-J., Park, B.-K., Peubey, C., de Rosnay, P., Tavolato, C., Thépaut, J.-N., and Vitart, F.: The ERA-Interim reanalysis: configuration and performance of the data assimilation system, Q. J. Roy. Meteor. Soc., 137, 553–597, https://doi.org/10.1002/qj.828, 2011 (data available at: http://apps.ecmwf.int/datasets/, last access: 01 November 2020).
Dickinson, R. E., Kennedy, P. J., and Henderson-Sellers, A.: Biosphere-Atmosphere Transfer Scheme (BATS) version 1e as coupled to the NCAR community climate model, National Center for Atmospheric Research, Climate and Global Dynamics Division, 1993.
Dickinson, R. E., Oleson, K. W., Bonan, G., Hoffman, F., Thornton, P., Vertenstein, M., Yang, Z. L., and Zeng, X. B.: The Community Land Model and its climate statistics as a component of the Community Climate System Model, J. Climate, 19, 2302–2324, 2006.
Ding, B. H., Yang, K., Qin, J., Wang, L., Chen, Y. Y., and He, X. B.: The dependence of precipitation types on surface elevation and meteorological conditions and its parameterization, J. Hydrol., 513, 154–163, https://doi.org/10.1016/j.jhydrol.2014.03.038, 2014.
Ding, B. H., Yang, K., Yang, W., He, X. B., Chen, Y. Y., Lazhu, Guo, X. F., Wang, L., Wu, H., and Yao, T. D.: Development of a Water and Enthalpy Budget-based Glacier mass balance Model (WEB-GM) and its preliminary validation, Water Resour. Res., 53, 3146–3178, 2017.
Dirmeyer, P. A.: A History and Review of the Global Soil Wetness Project (GSWP), J. Hydrometeorol., 12, 729–749, 2011.
Dirmeyer, P. A., Dolman, A. J., and Sato, N.: The pilot phase of the Global Soil Wetness Project, B. Am. Meteorol. Soc., 80, 851–878, 1999.
Fang, N., Yang, K., Lazhu, Chen, Y., Wang, J., and Zhu, L.: Research on the Application of WRF-Lake Modeling at Nam Co Lake on the Qinghai-Tibetan Plateau, Plateau Meteorology, 36, 610–618, 2017.
FAO: The Digitized Soil Map of the World Including Derived Soil Properties (version 3.6), available at: http://www.fao.org/geonetwork/srv/en/metadata.show?id=14116&currTab=distribution, last access: 01 November 2020), FAO, Rome, Italy, 2003.
FAO/IIASA/ISRIC/ISS-CAS/JRC: Harmonized World Soil Database (version 1.2), available at: http://webarchive.iiasa.ac.at/Research/LUC/External-World-soil-database/HTML/ (last access: 1 November 2020), FAO, Rome, Italy and IIASA, Laxenburg, Austria, 2012.
Farouki, O. T.: The Thermal-Properties of Soils in Cold Regions, Cold Reg. Sci. Technol., 5, 67–75, 1981.
Gao, Y. H., Li, K., Chen, F., Jiang, Y. S., and Lu, C. G.: Assessing and improving Noah-MP land model simulations for the central Tibetan Plateau, J. Geophys. Rese.-Atmos., 120, 9258–9278, 2015.
Gelaro, R., McCarty, W., Suarez, M. J., Todling, R., Molod, A., Takacs, L., Randles, C. A., Darmenov, A., Bosilovich, M. G., Reichle, R., Wargan, K., Coy, L., Cullather, R., Draper, C., Akella, S., Buchard, V., Conaty, A., da Silva, A. M., Gu, W., Kim, G. K., Koster, R., Lucchesi, R., Merkova, D., Nielsen, J. E., Partyka, G., Pawson, S., Putman, W., Rienecker, M., Schubert, S. D., Sienkiewicz, M., and Zhao, B.: The Modern-Era Retrospective Analysis for Research and Applications, Version 2 (MERRA-2), J. Climate, 30, 5419–5454, https://doi.org/10.1175/JCLI-D-16-0758.1, 2017 (data available at: https://disc.gsfc.nasa.gov/datasets?keywords=MERRA-2&page=1, last access: 1 November 2020).
Gu, C., Huang, A., Wu, Y., Yang, B., Mu, X., Zhang, X., and Cai, S.: Effects of Subgrid Terrain Radiative Forcing on the Ability of RegCM4.1 in the Simulation of Summer Precipitation Over China, J. Geophys. Res.-Atmos., 125, e2019JD032215, https://doi.org/10.1029/2019jd032215, 2020.
Gu, H., Jin, J., Wu, Y., Ek, M. B., and Subin, Z. M.: Calibration and validation of lake surface temperature simulations with the coupled WRF-lake model, Climatic Change, 129, 471–483, https://doi.org/10.1007/s10584-013-0978-y, 2015.
Guo, D. L. and Wang, H. J.: Simulation of permafrost and seasonally frozen ground conditions on the Tibetan Plateau, 1981–2010, J. Geophys. Res.-Atmos., 118, 5216–5230, https://doi.org/10.1002/jgrd.50457, 2013.
Guo, H.: Big Earth data: A new frontier in Earth and information sciences, Big Earth Data, 1, 4–20, https://doi.org/10.1080/20964471.2017.1403062, 2017.
Han, C. B., Ma, Y. M., Su, Z. B., Chen, X. L., Zhang, L., Li, M. S., and Sun, F. L.: Estimates of effective aerodynamic roughness length over mountainous areas of the Tibetan Plateau, Q. J. Roy. Meteor. Soc., 141, 1457–1465, https://doi.org/10.1002/qj.2462, 2015.
He, J., Yang, K., Tang, W., Lu, H., Qin, J., Chen, Y., and Li, X.: The first high-resolution meteorological forcing dataset for land process studies over China, Scientific Data, 7, 25, https://doi.org/10.1038/s41597-020-0369-y, 2020.
He, S. W., Nan, Z. T., and Hou, Y. T.: Accuracy evaluation of two precipitation datasets over upper reach of Heihe River Basin, northwestern China, available at: http://www.scar.ac.cn/EN/10.3724/SP.J.1226.2015.00157 (last access: 1 November 2020), Sci. Cold Arid. Reg., 7, 157–169, 2015.
Henderson-Sellers, A., Yang, Z. L., and Dickinson, R. E.: The Project for Intercomparison of Land-Surface Parameterization Schemes, B. Am. Meteorol. Soc., 74, 1335–1349, 1993.
Henderson-Sellers, A., Pitman, A. J., Love, P. K., Irannejad, P., and Chen, T. H.: The Project for Intercomparison of Land Surface Parameterization Schemes (PILPS): Phase 2 and Phase 3, B. Am. Meteorol. Soc., 76, 489–503, 1995.
Hengl, T., de Jesus, J. M., MacMillan, R. A., Batjes, N. H., Heuvelink, G. B. M., Ribeiro, E., Samuel-Rosa, A., Kempen, B., Leenaars, J. G. B., Walsh, M. G., and Gonzalez, M. R.: SoilGrids1km – Global Soil Information Based on Automated Mapping, PLOS One, 9, e114788, https://doi.org/10.1371/journal.pone.0105992, 2014.
Huang, A., Lazhu, Wang, J., Dai, Y., Yang, K., Wei, N., Wen, L., Wu, Y., Zhu, X., Zhang, X., and Cai, S.: Evaluating and Improving the Performance of Three 1-D Lake Models in a Large Deep Lake of the Central Tibetan Plateau, J. Geophys. Res.-Atmos., 124, 3143–3167, https://doi.org/10.1029/2018jd029610, 2019.
Hurrell, J. W., Holland, M. M., Gent, P. R., Ghan, S., Kay, J. E., Kushner, P. J., Lamarque, J. F., Large, W. G., Lawrence, D., Lindsay, K., Lipscomb, W. H., Long, M. C., Mahowald, N., Marsh, D. R., Neale, R. B., Rasch, P., Vavrus, S., Vertenstein, M., Bader, D., Collins, W. D., Hack, J. J., Kiehl, J., and Marshall, S.: The Community Earth System Model A Framework for Collaborative Research, B. Am. Meteorol. Soc., 94, 1339–1360, 2013.
Jimenez, C., Prigent, C., Mueller, B., Seneviratne, S. I., McCabe, M. F., Wood, E. F., Rossow, W. B., Balsamo, G., Betts, A. K., Dirmeyer, P. A., Fisher, J. B., Jung, M., Kanamitsu, M., Reichle, R. H., Reichstein, M., Rodell, M., Sheffield, J., Tu, K., and Wang, K.: Global intercomparison of 12 land surface heat flux estimates, J. Geophys. Res.-Atmos., 116, D02102, https://doi.org/10.1029/2010jd014545, 2011.
Jing, W. L., Di, L. P., Zhao, X. D., Yao, L., Xia, X. L., Liu, Y. X., Yang, J., Li, Y., and Zhou, C. H.: A data-driven approach to generate past GRACE-like terrestrial water storage solution by calibrating the land surface model simulations, Adv. Water Resour., 143, 103683, https://doi.org/10.1016/j.advwatres.2020.103683, 2020.
Johansen, O.: Thermal conductivity of soils, PhD dissertation, University of Trondheim, Trondheim, Norway, 236 pp., 1975.
Lazhu, Yang, K., Wang, J. B., Lei, Y. B., Chen, Y. Y., Zhu, L. P., Ding, B. H., and Qin, J.: Quantifying evaporation and its decadal change for Lake Nam Co, central Tibetan Plateau, J. Geophys. Res.-Atmos., 121, 7578–7591, 2016.
Lee, W.-L., Liou, K. N., and Wang, C.-c.: Impact of 3-D topography on surface radiation budget over the Tibetan Plateau, Theor. Appl. Climatol., 113, 95–103, https://doi.org/10.1007/s00704-012-0767-y, 2013.
Lee, W.-L., Liou, K.-N., Wang, C.-c., Gu, Y., Hsu, H.-H., and Li, J.-L. F.: Impact of 3-D Radiation-Topography Interactions on Surface Temperature and Energy Budget Over the Tibetan Plateau in Winter, J. Geophys. Res.-Atmos., 124, 1537–1549, https://doi.org/10.1029/2018jd029592, 2019.
Li, C., Lu, H., Leung, L. R., Yang, K., Li, H., Wang, W., Han, M., and Chen, Y.: Improving Land Surface Temperature Simulation in CoLM Over the Tibetan Plateau Through Fractional Vegetation Cover Derived From a Remotely Sensed Clumping Index and Model-Simulated Leaf Area Index, J. Geophys. Res.-Atmos., 124, 2620–2642, https://doi.org/10.1029/2018jd028640, 2019.
Li, C. W., Lu, H., Yang, K., Wright, J. S., Yu, L., Chen, Y. Y., Huang, X. M., and Xu, S. M.: Evaluation of the Common Land Model (CoLM) from the Perspective of Water and Energy Budget Simulation: Towards Inclusion in CMIP6, Atmosphere-Basel, 8, 141, https://doi.org/10.3390/atmos8080141, 2017.
Li, C. W., Lu, H., Yang, K., Han, M. L., Wright, J. S., Chen, Y. Y., Yu, L., Xu, S. M., Huang, X. M., and Gong, W.: The Evaluation of SMAP Enhanced Soil Moisture Products Using High-Resolution Model Simulations and In-Situ Observations on the Tibetan Plateau, Remote Sens.-Basel, 10, 535, https://doi.org/10.3390/rs10040535, 2018.
Li, D., Yang, K., Tang, W. J., Li, X., Zhou, X., and Guo, D. L.: Characterizing precipitation in high altitudes of the western Tibetan plateau with a focus on major glacier areas, Int. J. Climatol., 40, 5114–5127, https://doi.org/10.1002/joc.6509, 2020.
Li, J. D., Chen, F., Zhang, G., Barlage, M., Gan, Y. J., Xin, Y. F., and Wang, C.: Impacts of Land Cover and Soil Texture Uncertainty on Land Model Simulations Over the Central Tibetan Plateau, J. Adv. Model. Earth Sy., 10, 2121–2146, https://doi.org/10.1029/2018MS001377, 2018.
Li, Q. and Sun, S.: Development of the universal and simplified soil model coupling heat and water transport, Sci. China Ser. D, 51, 88–102, https://doi.org/10.1007/s11430-007-0153-2, 2008.
Lin, C. G., Chen, D. L., Yang, K., and Ou, T. H.: Impact of model resolution on simulating the water vapor transport through the central Himalayas: implication for models' wet bias over the Tibetan Plateau, Clim. Dynam., 51, 3195–3207, https://doi.org/10.1007/s00382-018-4074-x, 2018.
Liou, K. N., Lee, W.-L., and Hall, A.: Radiative transfer in mountains: Application to the Tibetan Plateau, Geophys. Res. Lett., 34, L23809, https://doi.org/10.1029/2007GL031762, 2007.
Liu, W., Sun, F., Li, Y., Zhang, G., Sang, Y.-F., Lim, W. H., Liu, J., Wang, H., and Bai, P.: Investigating water budget dynamics in 18 river basins across the Tibetan Plateau through multiple datasets, Hydrol. Earth Syst. Sci., 22, 351–371, https://doi.org/10.5194/hess-22-351-2018, 2018.
Lu, H., Koike, T., Yang, K., Hu, Z. Y., Xu, X. D., Rasmy, M., Kuria, D., and Tamagawa, K.: Improving land surface soil moisture and energy flux simulations over the Tibetan plateau by the assimilation of the microwave remote sensing data and the GCM output into a land surface model, Int. J. Appl. Earth Obs., 17, 43–54, https://doi.org/10.1016/j.jag.2011.09.006, 2012.
Luo, Q., Yang, K., Chen, Y., and Zhou, X.: Method development for estimating soil organic carbon content in an alpine region using soil moisture data, Sci. China Earth Sci., 63, 591–601, https://doi.org/10.1007/s11430-019-9554-8, 2020.
Luo, S. Q., Fang, X. W., Lyu, S. H., Zhang, Y., and Chen, B. L.: Improving CLM4.5 Simulations of Land-Atmosphere Exchange during Freeze-Thaw Processes on the Tibetan Plateau, J. Meteorol. Res.-Prc., 31, 916–930, 2017.
Luo, S. Q., Chen, B. L., Lyu, S. H., Fang, X. W., Wang, J. Y., Meng, X. H., Shang, L. Y., Wang, S. Y., and Ma, D.: An improvement of soil temperature simulations on the Tibetan Plateau, available at: http://www.scar.ac.cn/EN/10.3724/SP.J.1226.2018.00080 (last access: 1 November 2020), Sci. Cold Arid. Reg., 10, 80–94, 2018.
Manabe, S.: Climate and Ocean Circulation: I. The Atmospheric Circulation and Hydrology of the Earth's Surface, Mon. Weather Rev., 97, 739–774, https://doi.org/10.1175/1520-0493(1969)097<0739:CATOC>2.3.CO;2, 1969.
Oleson, K. W., Lawrence, D. M., Gordon, B., Flanner, M. G., Kluzek, E., Peter, J., Levis, S., Swenson, S. C., Thornton, E., and Feddema, J.: Technical description of version 4.0 of the Community Land model (CLM), The National Center for Atmospheric Research (NCAR), 2010.
Pan, M., Sahoo, A. K., Troy, T. J., Vinukollu, R. K., Sheffield, J., and Wood, E. F.: Multisource Estimation of Long-Term Terrestrial Water Budget for Major Global River Basins, J. Climate, 25, 3191–3206, 2012.
Pan, Y. J., Lyu, S. H., Li, S. S., Gao, Y. H., Meng, X. H., Ao, Y. H., and Wang, S. J.: Simulating the role of gravel in freeze-thaw process on the Qinghai-Tibet Plateau, Theor. Appl. Climatol., 127, 1011–1022, 2017.
Pinnington, E., Quaife, T., and Black, E.: Impact of remotely sensed soil moisture and precipitation on soil moisture prediction in a data assimilation system with the JULES land surface model, Hydrol. Earth Syst. Sci., 22, 2575–2588, https://doi.org/10.5194/hess-22-2575-2018, 2018.
Qi, W., Liu, J. G., and Chen, D. L.: Evaluations and Improvements of GLDAS2.0 and GLDAS2.1 Forcing Data's Applicability for Basin Scale Hydrological Simulations in the Tibetan Plateau, J. Geophys. Res.-Atmos., 123, 13128–13148, https://doi.org/10.1029/2018jd029116, 2018.
Reichstein, M., Camps-Valls, G., Stevens, B., Jung, M., Denzler, J., Carvalhais, N., and Prabhat: Deep learning and process understanding for data-driven Earth system science, Nature, 566, 195–204, https://doi.org/10.1038/s41586-019-0912-1, 2019.
Rienecker, M. M., Suarez, M. J., Gelaro, R., Todling, R., Bacmeister, J., Liu, E., Bosilovich, M. G., Schubert, S. D., Takacs, L., Kim, G. K., Bloom, S., Chen, J. Y., Collins, D., Conaty, A., Da Silva, A., Gu, W., Joiner, J., Koster, R. D., Lucchesi, R., Molod, A., Owens, T., Pawson, S., Pegion, P., Redder, C. R., Reichle, R., Robertson, F. R., Ruddick, A. G., Sienkiewicz, M., and Woollen, J.: MERRA: NASA's Modern-Era Retrospective Analysis for Research and Applications, J. Climate, 24, 3624–3648, 2011.
Rodell, M., Houser, P. R., Jambor, U., Gottschalck, J., Mitchell, K., Meng, C. J., Arsenault, K., Cosgrove, B., Radakovich, J., Bosilovich, M., Entin, J. K., Walker, J. P., Lohmann, D., and Toll, D.: The global land data assimilation system, B. Am. Meteorol. Soc., 85, 381–394, https://doi.org/10.1175/BAMS-85-3-381, 2004 (data available at: https://disc.gsfc.nasa.gov/datasets?page=1&keywords=GLDAS, last access: 1 November 2020).
Sellers, P. J., Mintz, Y., Sud, Y. C., and Dalcher, A.: A Simple Biosphere Model (Sib) for Use within General-Circulation Models, J. Atmos. Sci., 43, 505–531, 1986.
Sellers, P. J., Randall, D. A., Collatz, G. J., Berry, J. A., Field, C. B., Dazlich, D. A., Zhang, C., Collelo, G. D., and Bounoua, L.: A revised land surface parameterization (SiB2) for atmospheric GCMs .1. Model formulation, J. Climate, 9, 676–705, 1996.
Shangguan, W., Dai, Y. J., Liu, B. Y., Ye, A. Z., and Yuan, H.: A soil particle-size distribution dataset for regional land and climate modelling in China, Geoderma, 171, 85–91, 2012.
Shangguan, W., Dai, Y. J., Liu, B. Y., Zhu, A. X., Duan, Q. Y., Wu, L. Z., Ji, D. Y., Ye, A. Z., Yuan, H., Zhang, Q., Chen, D. D., Chen, M., Chu, J. T., Dou, Y. J., Guo, J. X., Li, H. Q., Li, J. J., Liang, L., Liang, X., Liu, H. P., Liu, S. Y., Miao, C. Y., and Zhang, Y. Z.: A China data set of soil properties for land surface modeling, J. Adv. Model. Earth Sy., 5, 212–224, 2013.
Shangguan, W., Dai, Y. J., Duan, Q. Y., Liu, B. Y., and Yuan, H.: A global soil data set for earth system modeling, J. Adv. Model. Earth Sy., 6, 249–263, 2014.
Shangguan, W., Hengl, T., de Jesus, J. M., Yuan, H., and Dai, Y. J.: Mapping the global depth to bedrock for land surface modeling, J. Adv. Model. Earth Sy., 9, 65–88, https://doi.org/10.1002/2016MS000686, 2017 (data available at: http://globalchange.bnu.edu.cn/research/data, last access: 1 November 2020).
Su, D., Hu, X., Wen, L., Lyu, S., Gao, X., Zhao, L., Li, Z., Du, J., and Kirillin, G.: Numerical study on the response of the largest lake in China to climate change, Hydrol. Earth Syst. Sci., 23, 2093–2109, https://doi.org/10.5194/hess-23-2093-2019, 2019.
Su, Z., Wen, J., Dente, L., van der Velde, R., Wang, L., Ma, Y., Yang, K., and Hu, Z.: The Tibetan Plateau observatory of plateau scale soil moisture and soil temperature (Tibet-Obs) for quantifying uncertainties in coarse resolution satellite and model products, Hydrol. Earth Syst. Sci., 15, 2303–2316, https://doi.org/10.5194/hess-15-2303-2011, 2011.
Su, Z., de Rosnay, P., Wen, J., Wang, L., and Zeng, Y.: Evaluation of ECMWF's soil moisture analyses using observations on the Tibetan Plateau, J. Geophys. Res.-Atmos., 118, 5304–5318, https://doi.org/10.1002/jgrd.50468, 2013.
Sun, S., Yan, J., Xia, N., and Sun, C.: Development of a model for water and heat exchange between the atmosphere and a water body, Adv. Atmos. Sci., 24, 927–938, https://doi.org/10.1007/s00376-007-0927-7, 2007.
Sun, S. F., Jin, J. M., and Xue, Y. K.: A simple snow-atmosphere-soil transfer model, J. Geophys. Res.-Atmos., 104, 19587–19597, https://doi.org/10.1029/1999jd900305, 1999.
Tong, K., Su, F. G., Yang, D. Q., and Hao, Z. C.: Evaluation of satellite precipitation retrievals and their potential utilities in hydrologic modeling over the Tibetan Plateau, J. Hydrol., 519, 423–437, https://doi.org/10.1016/j.jhydrol.2014.07.044, 2014.
Wang, A. H. and Zeng, X. B.: Evaluation of multireanalysis products with in situ observations over the Tibetan Plateau, J. Geophys. Res.-Atmos., 117, D05102, https://doi.org/10.1029/2011JD016553, 2012.
Wang, A. H., Zeng, X. B., and Guo, D. L.: Estimates of Global Surface Hydrology and Heat Fluxes from the Community Land Model (CLM4.5) with Four Atmospheric Forcing Datasets, J. Hydrometeorol., 17, 2493–2510, 2016.
Wang, B., Ma, Y., Chen, X., Ma, W., Su, Z., and Menenti, M.: Observation and simulation of lake-air heat and water transfer processes in a high-altitude shallow lake on the Tibetan Plateau, J. Geophys. Res.-Atmos., 120, 12327–12344, https://doi.org/10.1002/2015jd023863, 2015.
Wang, C. H. and Yang, K.: A New Scheme for Considering Soil Water-Heat Transport Coupling Based on Community Land Model: Model Description and Preliminary Validation, J. Adv. Model. Earth Sy., 10, 927–950, 2018.
Wang, L., Li, X. P., Chen, Y. Y., Yang, K., Chen, D. L., Zhou, J., Liu, W. B., Qi, J., and Huang, J. B.: Validation of the global land data assimilation system based on measurements of soil temperature profiles, Agr. Forest Meteorol., 218, 288–297, 2016.
Wang, L., Zhou, J., Qi, J., Sun, L. T., Yang, K., Tian, L. D., Lin, Y. L., Liu, W. B., Shrestha, M., Xue, Y. K., Koike, T., Ma, Y. M., Li, X. P., Chen, Y. Y., Chen, D. L., Piao, S. L., and Lu, H.: Development of a land surface model with coupled snow and frozen soil physics, Water Resour. Res., 53, 5085–5103, 2017.
Wang, S., Liu, S. X., Mo, X. G., Peng, B., Qiu, J. X., Li, M. X., Liu, C. M., Wang, Z. G., and Bauer-Gottwein, P.: Evaluation of Remotely Sensed Precipitation and Its Performance for Streamflow Simulations in Basins of the Southeast Tibetan Plateau, J. Hydrometeorol., 16, 2577–2594, https://doi.org/10.1175/Jhm-D-14-0166.1, 2015.
Wang, W., Cui, W., Wang, X. J., and Chen, X.: Evaluation of GLDAS-1 and GLDAS-2 Forcing Data and Noah Model Simulations over China at the Monthly Scale, J. Hydrometeorol., 17, 2815–2833, 2016.
Wang, W., Yang, K., Zhao, L., Zheng, Z., Lu, H., Mamtimin, A., Ding, B., Li, X., Zhao, L., Li, H., Che, T., and Moore, J. C.: Characterizing surface albedo of shallow fresh snow and its importance for snow ablation on the interior of the Tibetan Plateau, J. Hydrometeorol., 21, 815–827, https://doi.org/10.1175/JHM-D-19-0193.1, 2020.
Wang, Y., Yang, K., Pan, Z. Y., Qin, J., Chen, D. L., Lin, C. G., Chen, Y. Y., Lazhu, Tang, W. J., Han, M. L., Lu, N., and Wu, H.: Evaluation of Precipitable Water Vapor from Four Satellite Products and Four Reanalysis Datasets against GPS Measurements on the Southern Tibetan Plateau, J. Climate, 30, 5699–5713, https://doi.org/10.1175/Jcli-D-16-0630.1, 2017.
Wang, Y., Yang, K., Zhou, X., Chen, D. L., Lu, H., Ouyang, L., Chen, Y. Y., Lazhu, and Wang, B. B.: Synergy of orographic drag parameterization and high resolution greatly reduces biases of WRF-simulated precipitation in central Himalaya, Clim. Dynam., 54, 1729–1740, 2020.
Wu, G., Duan, A., Liu, Y., Mao, J., Ren, R., Bao, Q., He, B., Liu, B., and Hu, W.: Tibetan Plateau climate dynamics: recent research progress and outlook, Natl. Sci. Rev., 2, 100–116, https://doi.org/10.1093/nsr/nwu045, 2015.
Wu, J. and Gao, X.-J.: A gridded daily observation dataset over China region and comparison with the other datasets, available at: http://www.geophy.cn/EN/10.6038/cjg20130406 (last access: 1 November 2020), Chinese Journal of Geophysics-Chinese Edition, 56, 1102–1111, 2013.
Xia, Y., Sheffield, J., Ek, M. B., Dong, J., Chaney, N., Wei, H., Meng, J., and Wood, E. F.: Evaluation of multi-model simulated soil moisture in NLDAS-2, J. Hydrol., 512, 107–125, https://doi.org/10.1016/j.jhydrol.2014.02.027, 2014.
Xie, Z., Hu, Z., Gu, L., Sun, G., Du, Y., and Yan, X.: Meteorological Forcing Datasets for Blowing Snow Modeling on the Tibetan Plateau: Evaluation and Intercomparison, J. Hydrometeorol., 18, 2761–2780, https://doi.org/10.1175/JHM-D-17-0075.1, 2017.
Xie, Z. H., Di, Z. H., Luo, Z. D., and Ma, Q.: A Quasi-Three-Dimensional Variably Saturated Groundwater Flow Model for Climate Modeling, J. Hydrometeorol., 13, 27–46, 2012.
Xie, Z. H., Liu, S., Zeng, Y. J., Gao, J. Q., Qin, P. H., Jia, B. H., Xie, J. B., Liu, B., Li, R. C., Wang, Y., and Wang, L. H.: A High-Resolution Land Model With Groundwater Lateral Flow, Water Use, and Soil Freeze-Thaw Front Dynamics and its Applications in an Endorheic Basin, J. Geophys. Res.-Atmos., 123, 7204–7222, https://doi.org/10.1029/2018JD028369, 2018.
Yang, F., Lu, H., Yang, K., He, J., Wang, W., Wright, J. S., Li, C., Han, M., and Li, Y.: Evaluation of multiple forcing data sets for precipitation and shortwave radiation over major land areas of China, Hydrol. Earth Syst. Sci., 21, 5805–5821, https://doi.org/10.5194/hess-21-5805-2017, 2017.
Yang, K. and He J.: China meteorological forcing dataset (1979-2018), National Tibetan Plateau Data Center, https://doi.org/10.11888/AtmosphericPhysics.tpe.249369.file, 2019.
Yang, K., Koike, T., Ye, B. S., and Bastidas, L.: Inverse analysis of the role of soil vertical heterogeneity in controlling surface soil state and energy partition, J. Geophys. Res.-Atmos., 110, D08101, https://doi.org/10.1029/2004JD005500, 2005.
Yang, K., Koike, T., Ishikawa, H., Kim, J., Li, X., Liu, H. Z., Liu, S. M., Ma, Y. M., and Wang, J. M.: Turbulent flux transfer over bare-soil surfaces: Characteristics and parameterization, J. Appl. Meteorol. Clim., 47, 276–290, 2008.
Yang, K., Chen, Y.-Y., and Qin, J.: Some practical notes on the land surface modeling in the Tibetan Plateau, Hydrol. Earth Syst. Sci., 13, 687–701, https://doi.org/10.5194/hess-13-687-2009, 2009.
Yang, K., Qin, J., Zhao, L., Chen, Y. Y., Tang, W. J., Han, M. L., Lazhu, Chen, Z. Q., Lv, N., Ding, B. H., Wu, H., and Lin, C. G.: A Multiscale Soil Moisture and Freeze–Thaw Monitoring Network on the Third Pole, B. Am. Meteorol. Soc., 94, 1907–1916, https://doi.org/10.1175/bams-d-12-00203.1, 2013.
Yang, K., Zhu, L., Chen, Y. Y., Zhao, L., Qin, J., Lu, H., Tang, W. J., Han, M. L., Ding, B. H., and Fang, N.: Land surface model calibration through microwave data assimilation for improving soil moisture simulations, J. Hydrol., 533, 266–276, 2016.
Yang, K., Wang, C. H., and Li, S. Y.: Improved Simulation of Frozen-Thawing Process in Land Surface Model (CLM4.5), J. Geophys. Res.-Atmos., 123, 13238–13258, https://doi.org/10.1029/2017jd028260, 2018.
Yang, K., Chen, Y. Y., He, J., Zhao, L., Lu, H., Qin, J., Zheng, D. H., and Li, X.: Development of a daily soil moisture product for the period of 2002–2011 in Chinese Mainland, Sci. China Earth Sci., 63, 1113–1125, https://doi.org/10.1007/s11430-019-9588-5, 2020.
Yao, T., Wu, F., Ding, L., Sun, J., Zhu, L., Piao, S. L., Deng, T., Ni, X., Zheng, H., and Ouyang, H.: Multispherical interactions and their effects on the Tibetan Plateau's earth system: a review of the recent researches, Natl. Sci. Rev., 2, 468–488, https://doi.org/10.1093/nsr/nwv070, 2015.
Yi, S., He, Y., Guo, X., Chen, J., Wu, Q., Qin, Y., and Ding, Y.: The physical properties of coarse-fragment soils and their effects on permafrost dynamics: a case study on the central Qinghai–Tibetan Plateau, The Cryosphere, 12, 3067–3083, https://doi.org/10.5194/tc-12-3067-2018, 2018.
Yuan, H., Dai, Y. J., Xiao, Z. Q., Ji, D. Y., and Shangguan, W.: Reprocessing the MODIS Leaf Area Index products for land surface and climate modelling, Remote Sens. Environ., 115, 1171–1187, 2011.
Yuan, X., Ji, P., Wang, L., Liang, X.-Z., Yang, K., Ye, A., Su, Z., and Wen, J.: High-Resolution Land Surface Modeling of Hydrological Changes Over the Sanjiangyuan Region in the Eastern Tibetan Plateau: 1. Model Development and Evaluation, J. Adv. Model. Earth Sy., 10, 2806–2828, https://doi.org/10.1029/2018ms001412, 2018.
Zeng, Y., Xie, Z., Yu, Y., Liu, S., Wang, L., Jia, B., Qin, P., and Chen, Y.: Ecohydrological effects of stream–aquifer water interaction: a case study of the Heihe River basin, northwestern China, Hydrol. Earth Syst. Sci., 20, 2333–2352, https://doi.org/10.5194/hess-20-2333-2016, 2016.
Zeng, Y. J., Xie, Z. H., Yu, Y., Liu, S., Wang, L. Y., Zou, J., Qin, P. H., and Jia, B. H.: Effects of anthropogenic water regulation and groundwater lateral flow on land processes, J. Adv. Model. Earth Sy., 8, 1106–1131, 2016.
Zhang, Q., Jin, J., Wang, X., Budy, P., Barrett, N., and Null, S. E.: Improving lake mixing process simulations in the Community Land Model by using K profile parameterization, Hydrol. Earth Syst. Sci., 23, 4969–4982, https://doi.org/10.5194/hess-23-4969-2019, 2019.
Zhao, P., Xu, X., Chen, F., Guo, X., Zheng, X., Liu, L., Hong, Y., Li, Y., La, Z., Peng, H., Zhong, L., Ma, Y., Tang, S., Liu, Y., Liu, H., Li, Y., Zhang, Q., Hu, Z., Sun, J., Zhang, S., Dong, L., Zhang, H., Zhao, Y., Yan, X., Xiao, A., Wan, W., Liu, Y., Chen, J., Liu, G., Zhaxi, Y., and Zhou, X.: The third atmospheric scientific experiment for understanding the Earth-atmosphere coupled system over the Tibetan Plateau and its effects, B. Am. Meteorol. Soc., 99, 757–776, https://doi.org/10.1175/bams-d-16-0050.1, 2018.
Zheng, D., van der Velde, R., Su, Z., Wang, X., Wen, J., Booij, M. J., Hoekstra, A. Y., and Chen, Y.: Augmentations to the Noah Model Physics for Application to the Yellow River Source Area. Part I: Soil Water Flow, J. Hydrometeorol., 16, 2659–2676, https://doi.org/10.1175/jhm-d-14-0198.1, 2015a.
Zheng, D., van der Velde, R., Su, Z., Wang, X., Wen, J., Booij, M. J., Hoekstra, A. Y., and Chen, Y.: Augmentations to the Noah Model Physics for Application to the Yellow River Source Area. Part II: Turbulent Heat Fluxes and Soil Heat Transport, J. Hydrometeorol., 16, 2677–2694, https://doi.org/10.1175/jhm-d-14-0199.1, 2015b.
Zheng, D., Van der Velde, R., Su, Z., Wen, J., Booij, M. J., Hoekstra, A. Y., and Wang, X.: Under-canopy turbulence and root water uptake of a Tibetan meadow ecosystem modeled by Noah-MP, Water Resour. Res., 51, 5735–5755, https://doi.org/10.1002/2015wr017115, 2015c.
Zheng, D., Van der Velde, R., Su, Z., Wen, J., and Wang, X.: Assessment of Noah land surface model with various runoff parameterizations over a Tibetan river, J. Geophys. Res.-Atmos., 122, 1488–1504, https://doi.org/10.1002/2016jd025572, 2017a.
Zheng, D., van der Velde, R., Su, Z., Wen, J., Wang, X., and Yang, K.: Evaluation of Noah Frozen Soil Parameterization for Application to a Tibetan Meadow Ecosystem, J. Hydrometeorol., 18, 1749–1763, https://doi.org/10.1175/jhm-d-16-0199.1, 2017b.
Zheng, D., van der Velde, R., Su, Z., Wen, J., Wang, X., and Yang, K.: Impact of soil freeze-thaw mechanism on the runoff dynamics of two Tibetan rivers, J. Hydrol., 563, 382–394, https://doi.org/10.1016/j.jhydrol.2018.06.024, 2018.
Zheng, D. H., Van der Velde, R., Su, Z. B., Wen, J., Wang, X., Booij, M. J., Hoekstra, A. Y., Lv, S. H., Zhang, Y., and Ek, M. B.: Impacts of Noah model physics on catchment-scale runoff simulations, J. Geophys. Res.-Atmos., 121, 807–832, 2016.
Zhou, X., Yang, K., and Wang, Y.: Implementation of a turbulent orographic form drag scheme in WRF and its application to the Tibetan Plateau, Clim. Dynam., 50, 2443–2455, https://doi.org/10.1007/s00382-017-3677-y, 2018.
Zhou, X., Yang, K., Beljaars, A., Li, H., Lin, C., Huang, B., and Wang, Y.: Dynamical impact of parameterized turbulent orographic form drag on the simulation of winter precipitation over the western Tibetan Plateau, Clim. Dynam., 53, 707–720, https://doi.org/10.1007/s00382-019-04628-0, 2019.
Zhu, S. G., Chen, H. S., Zhang, X. X., Wei, N., Wei, S. G., Yuan, H., Zhang, S. P., Wang, L. L., Zhou, L. H., and Dai, Y. J.: Incorporating root hydraulic redistribution and compensatory water uptake in the Common Land Model: Effects on site level and global land modeling, J. Geophys. Res.-Atmos., 122, 7308–7322, 2017.