the Creative Commons Attribution 4.0 License.
the Creative Commons Attribution 4.0 License.
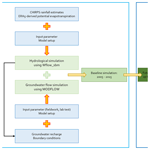
The impact of future changes in climate variables and groundwater abstraction on basin-scale groundwater availability
Steven Reinaldo Rusli
Victor F. Bense
Syed M. T. Mustafa
Albrecht H. Weerts
Groundwater is under pressure from a changing climate and increasing anthropogenic demands. In this study, we project the effect of these two processes onto future groundwater status. Climate projections of Representative Concentration Pathway 4.5 (RCP4.5) and Representative Concentration Pathway 8.5 (RCP8.5) from the Coupled Model Intercomparison Project Phase 6 (CMIP6) drive a one-way coupled fully distributed hydrological and groundwater model. In addition, three plausible groundwater abstraction scenarios with diverging predictions from increasing, constant, and decreasing volumes and spatial distributions are used. Groundwater status projections are assessed for short-term (2030), mid-term (2050), and long-term (2100) periods. We use the Bandung groundwater basin as our case study; it is located 120 km from the current capital city of Indonesia, Jakarta, which is currently scheduled for relocation. It is selected as the future anthropogenic uncertainties in the basin, related to the projected groundwater abstraction, are in agreement with our developed scenarios. Results show that changes in the projected climate input, including intensifying rainfall and rising temperature, do not propagate notable changes in groundwater recharge. At the current unsustainable groundwater abstraction rate, the confined piezometric heads are projected to drop by maxima of 7.14, 15.25, and 29.51 m in 2030, 2050, and 2100, respectively. When groundwater abstraction expands in proportion to present population growth, the impact is worsened almost 2-fold. In contrast, if groundwater abstraction decreases because of the relocated capital city, groundwater storage starts to show replenishment potential. As a whole, projected groundwater status changes are dominated by anthropogenic activity and less so by changes in climatic forcing. The results of this study are expected to show and inform responsible parties in operational water management about the issue of the impact of projected climate forcing and anthropogenic activity on future groundwater status.
- Article
(5446 KB) - Full-text XML
- BibTeX
- EndNote
Groundwater, as one of the major sources of water on Earth, has been known to be overexploited in many basins worldwide (Bierkens and Wada, 2019; Gleeson et al., 2020), which has caused further global depletion of groundwater resources (Wada et al., 2010). In more than half of the subdistricts located in the northwestern part of Bangladesh, the estimated groundwater abstraction has a higher volume than the simulated groundwater recharge (Shahid et al., 2015), and overexploitation of groundwater for irrigation, identified as the primary factor, contributes to the decline in groundwater levels in these areas (Mustafa et al., 2017). Even more drastically, 21 (out of 23) provinces in China were diagnosed with groundwater-overexploitation-related problems (Lili et al., 2020). In the northeastern part of Brazil, the intensification of groundwater exploitation has caused piezometric surface drawdowns of up to 100 m (de Luna et al., 2017). In the Canary Islands archipelago of Spain, in Gran Canaria, the currently accumulated groundwater depletion would require a few decades to recover (Custodio et al., 2016). From all such cases, we can see the severe impact on the groundwater regime of anthropogenic activities driven by groundwater abstraction. Dwindling groundwater tables, depleting groundwater storage, and degrading groundwater quality have led to various consequences, such as land subsidence (Chen et al., 2022), wetland deterioration (Mancuso et al., 2020), groundwater pollution (Meng et al., 2022), and seawater intrusion (Momejian et al., 2019).
Besides anthropogenic activities such as the groundwater abstraction discussed above, climatic variability may also play an important role in a changing groundwater regime since groundwater recharge is the dominant driver of groundwater flow. Surface and soil properties aside, groundwater recharge is modulated by precipitation, temperature, radiation, and other climate variables. The recent changes in these variables' patterns, frequencies, and extremes, therefore, have led to the alteration of the groundwater table distribution. Several studies suggest that changes in climate can contribute positively to an increase in groundwater recharge (Tillman et al., 2016; Patle et al., 2018; Gaye and Tindimugaya, 2019). This occurs when rainfall intensification provides a higher volume of water, thus providing an opportunity for more abundant groundwater resources. Others advocate the opposite and estimate a decline in groundwater recharge as a consequence of climate change (Pardo-Igúzquiza et al., 2019; Anurag and Ng, 2022; Trásy-Havril et al., 2022). For the most part, such a reduction is related to the higher potential evapotranspiration driven by the increasing temperature. Others have found the trend to be less definitive, varying per case depending on various factors and involving large uncertainties in its quantification (Meixner et al., 2016; Smerdon, 2017; Yawson et al., 2019; Wu et al., 2020b; Wang et al., 2021).
As much as both anthropogenic and climatic factors have influenced the groundwater regime in the past centuries even at the global scale (Döll et al., 2012), they have also, to various extents, controlled the current and future statuses of the subsurface resource (Stevenazzi et al., 2017; Liu et al., 2022). Therefore, future groundwater resource prediction relies greatly on climate projections and anthropogenic scenarios. However, the degree to which these two factors influence the groundwater resource varies in each case. Some studies suggest that changes in groundwater abstraction (anthropogenic factors) are more influential at the groundwater level compared to changes driven by climatic factors (Varouchakis et al., 2015; Brewington et al., 2019; Mustafa et al., 2019). On the other hand, Davamani et al. (2024) compiled a list of studies that propose otherwise. Within the list, it is shown that groundwater recharge will drop significantly under the impact of climate change (Olarinoye et al., 2020; Soundala and Saraphirom, 2022). This signals the uncertainties and high spatial variability in terms of the impact of both climatic and anthropogenic factors on groundwater recharge and, further, the groundwater level and availability. Therefore, it is important to address studies on the influences of these factors, which encompass various spatial scales – global, regional, and even local ones (Jyrkama and Sykes, 2007; Hughes et al., 2021).
Regarding climate projection studies, the Coupled Model Intercomparison Project (CMIP) takes up an important position in coordinating global climate models (GCMs) worldwide. In its current sixth development phase, CMIP6 (Eyring et al., 2016), it distributes climate model outputs from numerous GCMs run by various model groups in different representative concentration pathway (RCP) scenarios (IPCC, 2021), a set of pathways developed specifically for the span of projected radiative forcing values by the year 2100 (van Vuuren et al., 2011). With numerous hydrological forcing projections available in multiple scenarios, it is possible to simulate future groundwater recharge using hydrological models, from catchment to global scales (Yuan et al., 2015; Zhao et al., 2021; Hua et al., 2022).
While climate variables only have partial repercussions for groundwater recharge as this is also controlled by other factors, in particular basin surface and subsurface properties, groundwater abstraction directly removes groundwater from subsurface storage. Regarding anthropogenic projection scenarios, many studies develop scenarios in which groundwater abstraction rates increase (Chang et al., 2020; Ansari et al., 2021; Aslam et al., 2022), in line with rising populations. Only a few studies have projected the abstraction to decrease in the future (Mustafa et al., 2019; Siarkos et al., 2021), and when they do, this is estimated not to be a likely scenario but a recommended policy to achieve sustainable abstraction rates. Nevertheless, in some specific basin-scale areas, decreasing future groundwater abstraction might be a real possibility and hence is no less important for study in comparison to the increasing abstraction scenario. This is one of the novelties of this study, along with the coupled modeling approach and the assessment of shallow and deep groundwater availability.
In this study, we aim to envisage future groundwater availability in several climatic and anthropogenic scenarios, especially considering the spatially volatile variability of the influences of these factors on groundwater resources. We test the approach on the Bandung groundwater basin in Java, Indonesia. This would contribute novel findings on the degree of influence of both climatic and anthropogenic factors in such a highly groundwater-dependent and tropical area. While currently developed in a rising population trajectory, the Indonesian capital city's relocation plan could, in reverse, steer future groundwater abstraction down in the Bandung groundwater basin. This is a rather unique situation, as there are not many basins in the world that are seriously facing the possibility of future reduced groundwater abstraction. The Bandung groundwater basin and the city of Jakarta are closely connected. The urban and industrial sector development in the study area is highly influenced by the demographic and socioeconomic activity within and around the capital city. With the plan to relocate the capital to Borneo, it is predicted that many aspects of the study area will be impacted, including the decrease in the groundwater abstraction volume, rate, and spatial distribution. However, there have been several challenges in the early phase of the relocation that might lead to further repercussions. Should any consequences arise in the project, e.g., delay, postponement, or, even worse, revocation, the groundwater abstraction in the Bandung groundwater basin would still be projected to increase. Such uncertain circumstances of future groundwater abstraction are reflected in the developed anthropogenic scenarios of this study.
With the future climatic forcing and groundwater abstraction uncertainties, in our analysis, we simulate the groundwater level and storage changes using a one-way coupled distributed hydrological model (Wflow_sbm) and groundwater flow model (MODFLOW – the Modular three-dimensional finite-difference ground-water flow model) (Rusli et al., 2023a, b). By applying multiple climatic forcing and abstraction scenarios, we aim to specifically (1) quantify the impact of future climate projection on groundwater recharge and (2) assess the impact of changing groundwater abstraction on groundwater status in the study area. It is expected that the outcome of this study will be useful for understanding basin-scale future groundwater availability as well as the controlling factors and their processes. We also believe that this study will provide valuable input for the Bandung groundwater basin authorities to improve current and future groundwater policy and management.
2.1 Study area
2.1.1 Hydrological situation, hydrogeological setting, and current state of the art
The Bandung groundwater basin is located in the western part of Java, Indonesia, close to the current capital city of Jakarta (see Fig. 1). It covers a total area of over 1699 km2 and was populated by approximately 10 million people in 2020. Topographically, it is surrounded by steep mountains around its perimeter but mostly has plains in its middle part, where the urban areas have developed. Its elevation ranges from 640 to 2500 m a.s.l. Its average annual rainfall between 2005 and 2018 has been estimated to be between 1970 and 2850 mm per year (Rusli et al., 2021). The average discharge at the surface catchment outlet during the same period is observed to be 73.86 m3 s−1 but with a large temporal variation, with highest and lowest recorded discharges of 469.29 and 4.4 m3 s−1, respectively. Our previous study also reported the spatial distribution and pumping volume of the groundwater abstraction within the basin's boundary (Rusli et al., 2023a). In summary, the water demand from the domestic and industrial sectors grew at a swift rate of up to 1.6 times over these 14 years. On average, the volume of groundwater abstraction from the upper and lower aquifers was estimated to be 122 million and 255 million cubic meters per year, respectively. The upper and lower aquifers are different, as hydrogeologically the Bandung groundwater basin consists of multiple subsurface layers (see the conceptual groundwater flow model in Fig. 1, which is taken from Rusli et al., 2023b). Although the main aquifer is formed by a solitary geological formation named the Cibeureum Formation, it is interspersed in many locations by thin clay layers known as the Kosambi Formation. This aquitard zone conceptually divides the aquifer into two stratifications, hence the upper and lower aquifers. Detailed data on the basin climate, hydrology, and hydrogeological features are presented in our previous studies (Rusli et al., 2021, 2023a, b).
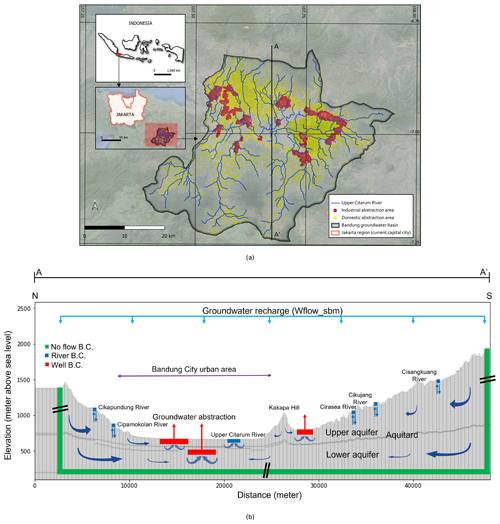
Figure 1(a) The geographical location of the study area. The yellow and red areas denote the domestic and industrial groundwater abstraction areas, respectively. The study area's relative position is highlighted on the locator map in the top-left corner, as indicated by the red square in Java. The second locator map just below the main locator map highlights the close distance between our study area of the Bandung groundwater basin and Jakarta, the current capital city of Indonesia. This image is adapted from Rusli et al. (2023b). (b) The conceptual model of the Bandung groundwater basin from the north–south cross section. Interspersing aquitard layers are found between the upper and lower aquifers, interpolated based on the available borehole data. The figure also includes groundwater fluxes entering, leaving, and flowing within the domain, together with their boundary conditions. This image is taken from Rusli et al. (2023b).
According to previous studies, the groundwater level in the Bandung groundwater basin has been decreasing in the last 3 decades. The groundwater table decreases, on average, from less than 1 m per year around urban areas close to rivers and streams to 2.45 m per year around industrial areas (Abidin et al., 2013; Gumilar et al., 2015). A correlation between the groundwater abstraction location and the decreasing groundwater table is visually apparent: the drop in the groundwater level is distinctly higher in areas where the groundwater is abstracted from both the upper and lower aquifers (Rusli et al., 2023b). In recent years, the rising pressure of water demand has still increased groundwater abstraction, while the intensified rainfall pattern has caused the groundwater recharge to fluctuate. Furthermore, this is worsened by the fact that groundwater abstraction from the bottom aquifer is partially compensated for by the loss of groundwater in the upper aquifer through vertical flow, which negatively impacts the unconfined groundwater table's disproportionate drawdown (Rusli et al., 2023b). All of the mentioned features of groundwater flow were simulated in our previous studies (Rusli et al., 2023a, b). Not only are the characteristics of the subsurface flow numerically reconstructed, but the one-way coupled hydrological-groundwater flow model is also supplemented, considering the limited number of calibration data, by additional validation of groundwater storage change using satellite-gravimetry-based estimates (GRACE – Gravity Recovery and Climate Experiment) (Rusli et al., 2023a) and semiquantitative model evaluation using environmental water tracer data (Rusli et al., 2023b).
2.1.2 The influence of Jakarta and the new capital city plan on the Bandung groundwater basin
Jakarta is a metropolitan city, with an area of 661.5 km2 and a population density of 13 000 people per square kilometer. Its area extends to the greater Jakarta Metropolitan Area (JMA), with a total area of 4384 km2. In 2019, the number of daily commuters entering JMA was reported to be 3.2 million (Martinez and Masron, 2020). Undoubtedly, Bandung, the largest city within the Bandung groundwater basin, is one of the cities with tangible mutual dependencies on Jakarta due to its close distance (Fig. 1). Bandung was populated by 2.7 million people, while the greater area of the Bandung groundwater basin had a total population of 10.5 million people, both in 2023. The flows of demographic and socioeconomic activities between these two cities often lead them to be referred to as the Jakarta–Bandung mega-urban region (Pravitasari et al., 2018).
The future of the groundwater regime in the Bandung groundwater basin has become uncertain with the latest geospatial planning of Indonesia. Indonesia intends to move its capital city from Jakarta to Nusantara, which is located on the separate island of Borneo (Nugroho, 2020; Mutaqin et al., 2021; Hackbarth and de Vries, 2021). Under the current schedule where the capital city will begin relocation in 2024 (de Vries and Schrey, 2022), the urban and industrial development in cities surrounding Jakarta, including those in the Bandung groundwater basin, is predicted to be impacted, as the relocation would move not only the center of government, but also some of the residents (Kodir et al., 2021). As per the Presidential Regulation of Indonesia number 63, 2022 (Indonesia, 2022), the relocation is scheduled for completion in 2045, with the first two phases ending in 2030. By 2030, up to 750 000 people are predicted to have been fully relocated from JMA and its surroundings, including the Bandung groundwater basin, to Nusantara.
Considering the capital city's relocation plan and the close proximity between our study area and Jakarta, it is reasonable to imagine that the former issue will dampen the growth of urban and industrial areas in the Bandung groundwater basin. Indirectly, it will also be possible to forecast that, in the future, the pressure to fulfill water demands by pumping groundwater will be reduced. Having said that, such a trend did not always happen in other former capital cities, such as Rio de Janeiro (Silva Jr. and Pizani, 2003), Lagos (Healy et al., 2020), and Yangon (Hashimoto et al., 2022). In these three former capital cities, economic growth continues despite being at different rates and translates into increasing groundwater abstraction. The uncertainties involving future water resources, specifically groundwater abstraction, in the Bandung groundwater basin are greatly unsettling, and thus it is necessary to explore multiple and diverging scenarios. Therefore, while the common conceptual understanding of groundwater abstraction projection will increase in the future, in this study we define three scenarios with wide ranges, from increasing to decreasing future groundwater abstractions as described in Sect. 2.3.2.
2.2 Simulation workflow and temporal framework
There are two numerical simulations involved in the modeling framework of this study: (1) surface hydrological flow and (2) groundwater flow. The hydrological simulation is performed using the Wflow_sbm model (Sect. 2.4.2), with two climate variables as its main forcings: rainfall and potential evapotranspiration. This produces two outputs: simulated river discharge and groundwater recharge. The former is compared with observation data to evaluate the performance of the hydrological Wflow_sbm model simulation. At the same time, the latter is used to force the one-way coupled MODFLOW groundwater flow model (Sect. 2.4.3). Aside from having simulated groundwater recharge as its input, the groundwater flow model is also regulated by boundary conditions of groundwater abstraction. The outputs of the groundwater flow model include transient groundwater tables for the unconfined layer and piezometric heads for the confined layer, which allow the associated groundwater storage changes to be calculated.
We consider the simulation setup described in this study in two phases based on a temporal categorization: the baseline period and the future period. The baseline period is used as a “control” of changes in the latter period; it is very important to note this, especially for variables whose projected changes are expressed as a percentage relative to the benchmark. For the hydrological simulation using Wflow_sbm and the groundwater flow model using MODFLOW, the benchmark period is set to between 2005 and 2015. The CHIRPS – Climate Hazards Group InfraRed Precipitation with Station data – rainfall estimates forced the baseline hydrological simulation (Funk et al., 2015), and the groundwater abstraction defined in the groundwater flow model was estimated based on the population number (Rusli et al., 2021). For the climate data's temporal classification, the baseline period's temporal coverage is from 1981 to 2015 following the categorization in the Copernicus Climate Data Store of the MRI-ESM2-0 model group (Copernicus Climate Change Service, 2021). For the future period, we place the temporal setting into three categories: the short-term future (up to 2030), the mid-term future (up to 2050), and the long-term future (up to 2100). These temporal classifications serve not only as analysis checkpoints but also as milestones for the projected groundwater abstraction spatial distribution described in Sect. 2.3.2. On the whole, Fig. 2 outlines the role of the temporal setup in this study (in the horizontal direction) from the baseline period (left box) to the future period (right box), together with the setup for subsequent hydrological and groundwater flow simulations (in the vertical direction) in each period.
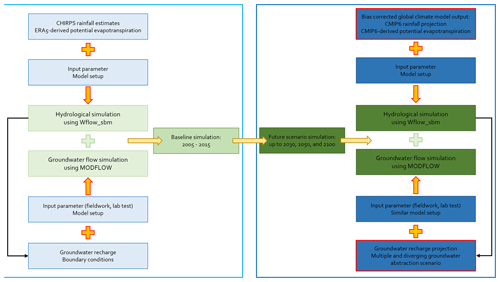
Figure 2Overview of the modeling workflow. The left and right boxes show, respectively, the model setup during the baseline period and the future period. While both the hydrological and groundwater flow models' setups are similar during the two periods, the forcing data and boundary conditions are different and are determined according to their respective configurations.
2.3 Future scenario development
In this study, we are going to independently develop two climatic scenarios (Sect. 2.3.1) and three anthropogenic scenarios (Sect. 2.3.2). The climatic scenarios involve changes in projected rainfall and potential evapotranspiration that are influenced by temperature and radiation. The anthropogenic scenarios involve changes in not only the groundwater abstraction rate but also the spatial distribution of the pumping activities. These scenarios result in six combinations of outcomes that are analyzed, compared, and discussed in depth in further sections.
2.3.1 Climate projection data and scenario
For the future period's hydrological simulation, we use the CMIP6 (Coupled Model Intercomparison Project Phase 6) climate model runs (Eyring et al., 2016) in two greenhouse gas concentration trajectory scenarios: RCP4.5 and RCP8.5 (IPCC, 2021). RCP4.5 is selected as the intermediate scenario, while RCP8.5 is the extreme one. The considered variables are those required as input to the Wflow_sbm model, specifically precipitation and near-surface air temperature as well as surface downwelling shortwave radiation and top-of-atmosphere (TOA) incident shortwave radiation. The latter three variables are used to estimate potential evapotranspiration using the method proposed by de Bruin et al. (2016). All the mentioned climate model products are publicly available from the Copernicus Climate Data Store (Copernicus Climate Change Service, 2021). Consistent with Sect. 2.2 and Fig. 2, we use the data on both the baseline and future scenarios' temporal frameworks: up to 2015 for the baseline period and up to 2100 for the future period. The used data have a daily temporal resolution, following the daily resolution for the hydrological Wflow_sbm model simulation, and are aggregated to a monthly resolution for the groundwater MODFLOW model simulation.
To select the model group, we apply criteria that the product should have: (a) a spatial resolution of approximately 1° × 1° considering the size of the study area, (b) a daily temporal resolution for rainfall and temperature following the hydrological model setup, and (c) a projection of historical, RCP4.5, and RCP8.5 scenarios applicable to the four mentioned variables. Considering the criteria and other climate projection studies, we use the climate model outputs from the MRI-ESM2-0 model group (Yukimoto et al., 2019) for the precipitation and near-surface air temperature. It has been suggested to perform well in CMIP6 outputs (Oruc, 2022), especially those related to cloud processes (Kawai et al., 2019). It has also been shown to be one of the best performing models in other studies and proven through multi-metric assessments, including in Southeast Asia (Iqbal et al., 2021; Baghel et al., 2022), which is very close to our study area. It is not used for the global radiation data, however, as it does not cover the TOA incident shortwave radiation projection for RCP8.5. Therefore, for the global radiation data, we use the climate model output from the Geophysical Fluid Dynamics Laboratory Earth System Model (GFDL-ESM4) group (Krasting et al., 2018).
2.3.2 Groundwater abstraction scenarios
For the groundwater abstraction projection, we consider three diverging scenarios where the groundwater abstraction (a) increases, (b) stays constant, and (c) decreases in the future. Meanwhile, the groundwater abstraction during the baseline period is set according to estimates from our previous studies (Rusli et al., 2023a), increasing annually from 300 Mm3 per year in 2005 to 495 Mm3 per year in 2020. For all the boundary conditions, the groundwater abstraction is distributed horizontally based on land use and vertically based on domestic or industrial water demand classifications.
In this study, we propose a new approach to establishing the scenario where the future groundwater abstraction increases (scenario 1). Commonly, the projected groundwater abstraction rate increases in proportion to the projected population growth. We indeed apply such a method to estimate the annual volume of the future groundwater abstraction in the study area, using an annual population growth rate of 1.36 % as shown in Fig. 3a. In this scheme, the annual groundwater abstraction volume in 2030, 2050, and 2100 is projected to be 529, 839, and 1346 Mm3 per year. Considering the current high population density in the Bandung groundwater basin, it is only logical that a surge in the groundwater abstraction volume is accompanied by an enlargement of the abstraction area. In this paper, we expand the groundwater abstraction location by increasing the area of the initial abstraction location proportionally to the volume of abstraction.
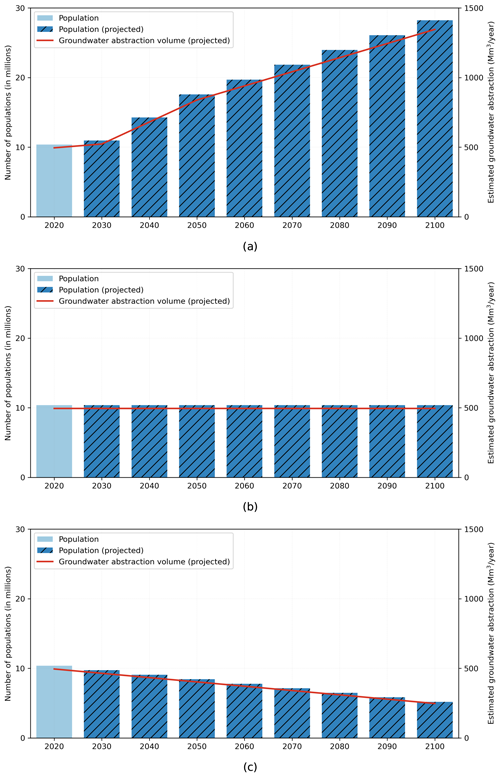
Figure 3The three diverging scenarios of estimated groundwater abstraction volumes (right axis, Mm3 yr−1) based on the projected population (left axis, millions). (a) Abstraction scenario 1: increasing abstraction. (b) Abstraction scenario 2: constant abstraction. (c) Abstraction scenario 3: decreasing abstraction.
The other two groundwater abstraction scenarios are based on Indonesia's capital city relocation plan. In the “stay constant” scenario (scenario 2), we assume that the urban and industrial areas that are currently settled will continue to remain where they are now, with linear development (Fig. 3b). It is also possible that there will be tangible development, but its impact will be compensated for by technological advancement, e.g., increasing efficiency or reducing losses of (groundwater) use and distribution. Therefore, the groundwater abstraction rate is made constant from 2020 to 2100. In the decreasing groundwater abstraction scenario (scenario 3), we assume that the capital city's relocation will gradually decrease the population of the Bandung groundwater basin and water demand in the future (Fig. 3c). By 2100, it is assumed that the population will be half that of 2020, thereby also decreasing the groundwater abstraction rate by 50 % to 248 Mm3 per year, linearly interpolated. In scenarios 2 and 3, the spatial distribution of the groundwater abstraction area remains the same as applied in the baseline period.
2.4 One-way coupled model simulation setup
2.4.1 Bias adjustment and statistical downscaling method of the Inter-Sectoral Impact Model Intercomparison Project phase 3b (ISIMIP3b)
In climate-related research, it is common to apply bias correction to climate simulation data, as they generally have statistical attributes that are different from climate observation data (Lange, 2019). The discrepancies occur due to various factors, such as differences in spatial resolution and systematic biases. Therefore, bias correction, involving a two-step method of bias adjustment and statistical downscaling, is necessary to bridge and minimize this gap. In this study, we apply a method tailored to ISIMIP3b for our bias correction (Lange, 2019, 2021).
In ISIMIP3b, the data required to be specified as the benchmark are the (a) historical “ground truth” data and (b) high-resolution data for the bias adjustment and statistical downscaling, respectively. In this study, we use CHIRPS estimates (Funk et al., 2015), with a spatial resolution of 0.25° × 0.25°, as the historical ground truth rainfall estimates. The MRI-ESM2-0 model output is available at a lower spatial resolution than the CHIRPS estimates. Therefore, it is first regridded and resampled to match the CHIRPS spatial resolution before the bias adjustment is applied. As CHIRPS is available at an even higher resolution of 0.05° × 0.05°, we continue to use it as a benchmark for the statistical downscaling. The historical ground truth estimates for the other variables (near-surface air temperature, surface downwelling shortwave radiation, and TOA incident shortwave radiation) are based on ERA5-Land hourly data (Copernicus Climate Change Service, 2019). All the datasets used for the application of ISIMIP3b in this paper are listed in Table 1.
2.4.2 Wflow_sbm model setup
The Wflow_sbm model (van Verseveld et al., 2024) is used to perform the hydrological simulation. With model parameters that mostly represent physical characteristics, using Wflow_sbm makes it easier to intuitively interpret and correlate the parameter values with physical catchment properties. In the last decade, Wflow_sbm has been widely used in hydrological modeling studies (López et al., 2016; Hassaballah et al., 2017; Gebremicael et al., 2019), including those in tropical regions like Southeast Asia (Wannasin et al., 2021; Rusli et al., 2021), delivering good performance as shown by the Kling–Gupta efficiency (KGE), Nash–Sutcliffe efficiency (NSE), and RMSE metrics.
We use the same Wflow_sbm model as in our previous studies (Rusli et al., 2023a, b). Starting with high-resolution model parameterization based on point-scale (pedo)transfer functions (PTFs) (Imhoff et al., 2020), it is followed by downscaling designated to a model resolution of 0.008° × 0.008°. We use (a) the SoilGrids database (Hengl et al., 2017) for soil-related parameter estimation, (b) the monthly leaf area index climatology for daily interception calculation (Gash, 1979), (c) the MERIT-DEM dataset (Yamazaki et al., 2017) for river network delineation (Eilander et al., 2021), and (d) the VITO land use map (Buchhorn et al., 2020) for deriving land-use-related parameters. After the simulation of the surface processes, the infiltrated water flow is controlled by the MaxLeakage parameter. Such a parameter influences the amount of water flowing from the pseudo water table to the deep groundwater (van Verseveld et al., 2024) and hence the groundwater recharge. It is usually only used for linking to a dedicated groundwater model, representing the water that is “lost” to the model. Normally set to zero in all other cases, when the MaxLeakage parameter is defined as greater than zero, the simulated water is treated as lost from the saturated zone and runs out of the model. As the Wflow_sbm model only considers the first couple of meters of soil below the surface level, the water that leaves the saturated zone is then treated as the groundwater recharge. To calibrate the MaxLeakage parameter, considering its importance, we optimized the KGE value between the observed and simulated river discharges (Rusli et al., 2023a).
Precipitation and potential evapotranspiration are the primary forcing data to run the Wflow_sbm model. Following the temporal setting, we prepare and split the forcing data into the baseline and future projection periods. For the baseline period, CHIRPS rainfall estimates (Funk et al., 2015) and potential evapotranspiration, derived from ERA5 temperature and global radiation data using the method from de Bruin et al. (2016), are used. Using the extended triple-collocation method (McColl et al., 2014), CHIRPS products were found to perform well in estimating rainfall in the study area in our previous study (Rusli et al., 2021). For the future period, the forcing data are obtained from the bias-corrected climate projection: (1) the bias-corrected rainfall projections from the CMIP6 model output of the MRI-ESM2-0 model group and (2) the potential evapotranspiration derived from the bias-corrected near-surface air temperature projections from the CMIP6 model output of the MRI-ESM2-0 model group and the bias-corrected surface downwelling shortwave radiation and TOA incident shortwave radiation projections from the CMIP6 model output of the GFDL-ESM4 model group, based on two RCP scenarios (RCP4.5 and RCP8.5).
2.4.3 Groundwater flow model setup
The MODFLOW6 model, which solves the Darcy three-dimensional groundwater flow equation using the control-volume finite-difference (CVFD) method, is used to perform the groundwater flow simulation in this study. The model is built using the MODFLOW Python package (Bakker et al., 2016).
The MODFLOW model parameterization is based on the combination of literature reviews, fieldwork, and laboratory experiments. The model's subsurface vertical discretization is based on collated borehole data (Rahiem, 2020), interpreted as a three-layer model: the upper aquifer as the top layer, the thin interspersing aquitard as the middle layer, and the lower aquifer as the bottom layer. The hydraulic conductivities of the soil were measured using a combination of slug tests in the field, laboratory tests, and private company reports, which were recalibrated later (Rusli et al., 2023a). The Kh of the upper and lower aquifers is in relatively similar ranges between 0.15 and 0.58 m per day, as it is formed by a solitary geological formation. The Kv ranges between 3.0 × 10−4 and 6.0 × 10−4 m per day. The aquitard is 10 times less permeable than the aquifer. The storage parameters are obtained from private company pumping test reports, and the river-related parameters were previously calibrated under steady-state conditions. The well package, related to groundwater abstraction, is set according to the scenario described in Sect. 2.3.2. The full description of the groundwater flow model parameterization, including the model parameter recalibration, is also reported in our previous studies (Rusli et al., 2023a, b).
Similar to the hydrological simulation, we split the forcing data and the simulation period into two windows: the baseline period between 2005 and 2015 and the future projection period of short-term (up to 2030), mid-term (up to 2050), and long-term (up to 2100) futures. Both periods are forced by the groundwater recharge simulated by the Wflow_sbm model, resulting in three different inputs as the drivers of the groundwater flow model: the baseline groundwater recharge, the future RCP4.5 groundwater recharge, and the future RCP8.5 groundwater recharge. With the combinations of different inputs and boundary conditions, six different outputs are produced from the groundwater flow simulation.
3.1 Climate projection
The results for the climate projection are visualized by comparing the discussed climate variables – rainfall, temperature, solar radiation, or potential evapotranspiration – during the baseline period and the future period. Quantitatively, the variables are compared using their statistical attributes: minimum and maximum values; mean; and lower, middle, and upper quartiles. Visually, they are also represented in boxplots for easier interpretation and are categorized by monthly estimates.
3.1.1 Bias-corrected rainfall projection
The statistical attributes of the rainfall estimates pre and post bias correction, as well as the ones of the future scenarios, are summarized in Table 2. In the MRI-ESM2-0 model group's “historical” output pre bias correction, the rainfall is, surprisingly, projected to decrease in general compared to the CHIRPS estimates (column 1) in both RCP4.5 (column 4) and RCP8.5 (column 6). These values are, however, prior to bias correction. The same trend of climate model underestimation in almost every statistical distribution is also found during the baseline period (column 1 and column 2). Therefore, it is essential to implement bias correction. By applying the ISIMIP3b bias adjustment, we come up with baseline rainfall estimates that represent a better statistical fit to the CHIRPS estimates (column 3). Consequently, we apply the bias correction to the future scenarios of RCP4.5 (column 5) and RCP8.5 (column 7).
Table 2Application of ISIMIP3b bias correction to the rainfall estimate projection from the MRI-ESM2-0 model group (mm).
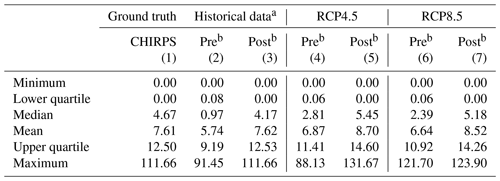
a Historical data of the climate projection products have the temporal coverage of the baseline period (1981–2015). b The pre and post columns represent the values pre and post bias adjustment in the future periods (2015–2100).
To observe the seasonal impact on the projected climatic scenario, we plot the monthly rainfall of the baseline period of CHIRPS and the future period of the bias-corrected RCP4.5 and RCP8.5 projections in Fig. 4. The boxplots represent the median and interquartile range of the interannual estimates, while the whiskers show the estimates' range. During the rainy season between October and March, we can see that the projected rainfall has an increasing trend, with higher monthly rainfall especially from November to January. A contrasting trend is shown in the dry season between April and September, with lower monthly rainfall especially from July to September. In short, the wet period is projected to become wetter, and the dry period is projected to become drier. We can also observe only small differences in the statistical quartiles between the two RCPs with similar widths, mostly between the orange and red boxes. The width of the minimum and maximum values, however, is more apparent. The magnitudes of the hydrological extremes are projected to be more pronounced in the future. Therefore, floods and droughts are predicted to be more severe than they currently are.
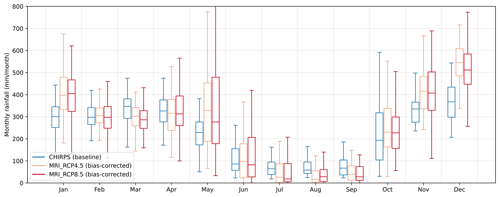
Figure 4The comparison between the rainfall monthly statistics in the baseline period and the two future climatic scenarios (RCP4.5 and RCP8.5). The future rainfall estimates are bias-corrected using the ISIMIP3b bias correction method. The box and whisker plots represent the median, interquartile range, and range of the interannual estimates.
3.1.2 Bias-corrected potential evapotranspiration projection
We apply the bias correction method of ISIMIP3b to the near-surface air temperature, surface downwelling shortwave radiation, and TOA incident shortwave radiation in a similar fashion to the one of the rainfall estimates. Figure 5a and b show the monthly near-surface air temperature and radiation projections, respectively. The monthly average temperature sharply increases from the baseline period of the aggregated ERA5-Land hourly estimates to the future period of the bias-corrected CMIP6 projections in all statistical attributes: quartiles, average, interquartile range, and extreme values. The temperature, on the long-term average, is projected to be warmer by 2.21 and 2.72 °C in RCP4.5 and RCP8.5, respectively. The projection onto global radiation is based on the GFDL-ESM4 model group, with a tendency towards slightly lower surface downwelling shortwave radiation in the future. The TOA incident shortwave radiation remains almost constant throughout. In the radiation variable, the two different climatic scenarios of RCP4.5 and RCP8.5 do not seem to differ a lot in their statistical values.
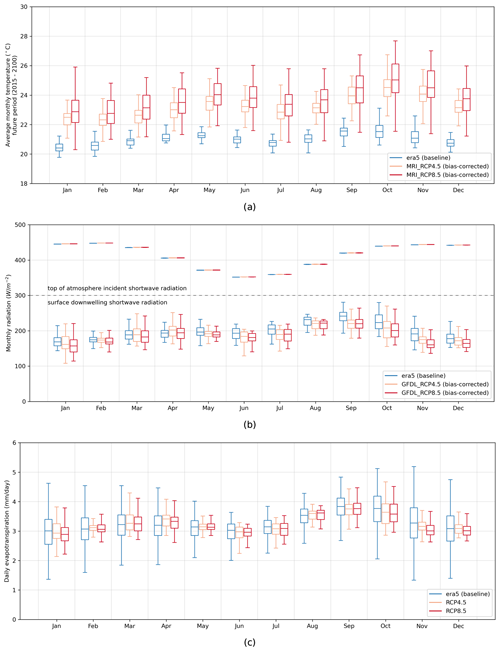
Figure 5The results for bias-corrected projections onto three variables: (a) temperature, (b) radiation, and (c) potential evapotranspiration, all in the baseline period and the two future climatic scenarios (RCP4.5 and RCP8.5. The box–whisker plots represent the median, interquartile range, and range of the interannual estimates.
We use the three variables above to calculate potential evapotranspiration using the method of de Bruin et al. (2016), with the result shown in Fig. 5c. The range of estimates is visually inconsistent between the baseline and future periods, as the future period is calculated in monthly time steps according to the temporal resolution of the radiation projections. Therefore, the variation between the statistical distribution is lower compared to the ones of the baseline period, where it is available at a daily time step. The difference in magnitude is considered low, as the highest difference in average daily potential evapotranspiration is less than 0.5 mm per day. Without looking at the seasonal variance, the annual daily potential evapotranspiration averages of the baseline period, the future RCP4.5 scenario, and the future RCP8.5 scenario are 3.24, 3.26, and 3.23 mm per day, respectively, which are relative to insignificant differences.
3.2 Groundwater recharge projection
The projected rainfall and potential evapotranspiration are used to force the Wflow_sbm model, resulting in the projected groundwater recharge. As the change in groundwater recharge in millimeters per day is relatively small, we accumulate the daily recharge to monthly recharge to produce more intuitive figures. The seasonal pattern of the monthly groundwater recharge is shown in Fig. 6a. The values intrinsically represent the number of days in each month. Therefore, the groundwater recharge in the months without 31 d is lower than that in the months with 31 d. The results are confirmed to be consistent in all the short-term, mid-term, and long-term future assessments of groundwater recharge, with consistent and slightly increasing groundwater recharge during the wet and dry seasons, respectively.
It can be seen that, after the start of the wet season when the soil moisture starts to be saturated (December) to the beginning of the dry season when the soil's maximum capacity for storing water is still attained (May), the groundwater recharge can no longer increase despite the increasing rainfall projection during the wet season. On the other hand, after the start of the dry season when the soil moisture starts to dry up (June) to the beginning of the wet season when the void spaces in the subsurface are still available for water to fill into (November), the magnitude of the groundwater recharge is relatively more subject to change. Having said that, during the latter period, there is only a small difference in the median and extreme values of the groundwater recharge, although the quartile values vary. Based on the median values, the largest difference in groundwater recharge is projected to occur in either September (for RCP4.5) or October (for RCP8.5), in an order of magnitude of minuscule increases of 1.27 % and 1.79 %. In all other months except June, the groundwater recharge is projected to either, despite being a very small change, increasing, or remaining relatively constant. Annually, the average groundwater recharge during the baseline period of 315.1 mm per year is projected to insignificantly increase to 316.1 mm per year for RCP4.5 and 316.4 mm per year for RCP8.5. On average, the absolute relative change in the rainfall, temperature, and groundwater recharge estimates in RCP4.5 are 31.03 %, 10.71 %, and 0.36 %. Similar changes for RCP8.5 are 28.36 %, 13.10 %, and 0.44 %. Figure 6b displays a comparison of the magnitude of change in the rainfall and potential evapotranspiration on the left axis as well as the groundwater recharge on the right axis with a 10 times larger scale, all in monthly average values. The average value is chosen over the median value, specifically for this figure, to take into account the projected extreme values. From the results, the change in the groundwater recharge is found to be far less responsive compared to its driver, especially for rainfall.
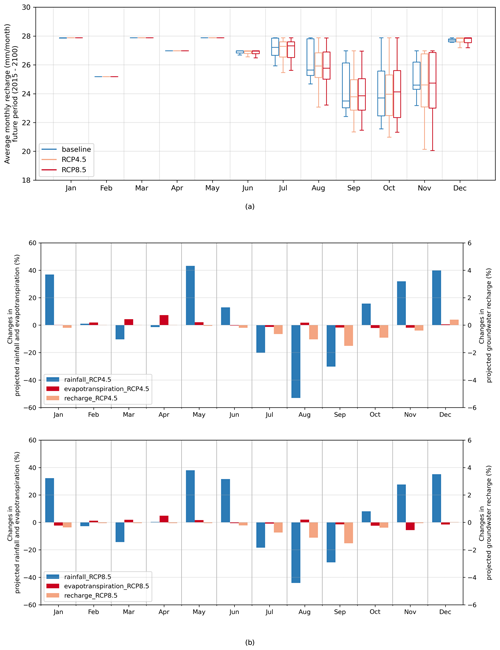
Figure 6The results of (a) groundwater recharge projection and (b) its value relative to its driver of rainfall and potential evapotranspiration. The values are averaged over the future period, annually, up to 2100.
The results could indicate two possibilities. First, this reveals the dominant role of the already saturated soil in controlling the groundwater recharge processes in the Bandung groundwater basin. When the soil's maximum capacity is already reached, more rainfall does not directly affect the recharge processes. Instead, it increases surface runoff, river discharge, and water loss to evapotranspiration; the latter occurs only when the potential evapotranspiration (PET) allows it. However, as shown in Fig. 5c, the projected change in the PET varies between months. During the wet season, in particular, the PET is even projected to slightly decrease. This concept is agreed upon by the hydrological model simulation in the projected climatic scenarios. Figure 7a and b, respectively, show the boxplot of the simulated river discharge and actual evaporation in the baseline and future periods. These graphs indicate an increase in the river discharge variable and a slight decrease in the actual evaporation. Specifically for the river discharge, the projected amplification is remarkably apparent, and the magnitude of the rise is larger the further the projections are, following the trend shown in the rainfall projection. The results for actual evaporation follow the ones of the projected PET, with largely a minor decrease in values. These changes balance out the increment of the rainfall forcing, supporting the outcome of groundwater recharge that shows relatively steady consistencies despite the increase in the hydrological forcing dataset. In the water balance term, the amplified magnitude of inflow in the future is followed by the rising outflow, causing the changes in the other outflux of groundwater recharge to be minimal. Secondly, the impact of changing climate variables on groundwater recharge could be hindered by the model's coupling limitation, causing the model to underestimate the degree of influence. The fact that the hydrological and groundwater flow models are one-way coupled, instead of fully coupled, caps the two-way feedback nature of groundwater recharge. Physically, groundwater recharge is controlled by both the surface processes (represented by the hydrological simulation in this study) and the amount of space available to be recharged (represented by the groundwater flow model). However, in our one-way model coupling scheme, groundwater recharge is fully determined by the surface processes. These two notions are discussed further in Sect. 4.2.
3.3 Groundwater level projection
The combination of two climatic and three groundwater abstraction scenarios results in six outcomes. For each outcome, there are three temporal checkpoints assigned as milestones of the assessment: the short-term, mid-term, and long-term futures in 2030, 2050, and 2100, respectively. There are also two layers of aquifers to be assessed, i.e., the unconfined and confined aquifers. As there are a lot of numbers to unpack, we discuss the results as per the abstraction scenario.
Generally, we focus on the maximum drawdown values of the groundwater table or piezometric head, as the change in the groundwater head is found to be highly localized from both the simulation and observation perspectives. Furthermore, the baseline groundwater abstraction area is estimated at “only” 27.3 % of the total basin area for the domestic groundwater abstraction and even at 4.7 % for the more intensive industrial abstraction. Taking the average or median value for the whole groundwater basin, therefore, would not be suitable for representing the severity of the groundwater abstraction impact, considering the high number of cells involved in the numerical simulation.
In the increasing groundwater abstraction scenario, the groundwater level is projected to continue decreasing. The numbers are worse for the confined aquifer, as the groundwater abstraction is spatially more concentrated with higher abstraction rates while having a much smaller storage coefficient compared to the one in the unconfined layer. In RCP4.5, the maximum piezometric head drawdowns for the confined aquifer are projected to be 10.04 m in 2030, 19.98 m in 2050, and 48.79 m in 2100. In RCP8.5, the numbers are also concerning for the unconfined aquifers, as the groundwater table is projected to decrease to 3.38 and 3.40 m in the long run in RCP4.5 and RCP8.5, respectively. Based on the drawdown area, the trend shows that the impacted area is enlarged as the groundwater abstraction area expands. In the unconfined aquifer, 74.6 %, 80.5 %, and 87.2 % of the groundwater basin area are projected to experience groundwater table drawdown in 2030, 2050, and 2100 in RCP4.5, obviously with varying magnitudes. For the confined aquifers, the numbers representing the depression area are 70.5 %, 72.9 %, and 75.4 %. In RCP8.5, these numbers are pretty much consistent, with a maximum difference of less than 1 % from the RCP4.5 results.
The second abstraction scenario shows the projection of the current situation in the study area. Should the anthropogenic pressure remain the same, the groundwater level, as expected, is projected to remain decreasing. The maximum confined piezometric head drawdowns for 2030, 2050, and 2100 in RCP4.5 are 7.14, 15.25, and 29.51 m. The numbers for RCP8.5 are very similar: 7.14, 15.28, and 29.51 m. Similar to the increasing abstraction scenario, the unconfined aquifer groundwater table drawdown is noticeably lower compared to that of the confined aquifer, with maximum drawdowns of 2.58 and 2.60 m in 2100 for RCP4.5 and RCP8.5, respectively. The drawdown area increases, with up to 84.7 % and 75.0 % of the groundwater basin area experiencing long-term dwindling groundwater heads in the unconfined and confined aquifers. Despite the lesser extent of the impacted area relative to the previous scenario, the area of the decreasing groundwater head remains relatively dominant in comparison to the basin's total area. To visualize the time series increasing the impact of the groundwater head drawdown, Fig. 8 shows the propagation of the groundwater head decline in the Bandung groundwater basin in the RCP4.5 climatic scenario and the constant groundwater abstraction anthropogenic scenario.
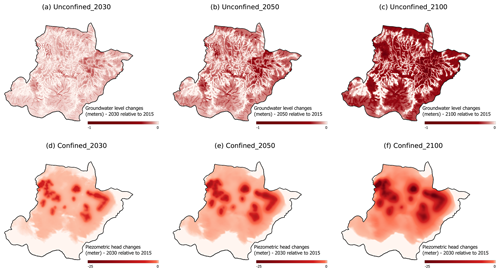
Figure 8The spatial distribution of the projected groundwater head change in RCP4.5 and the constant groundwater abstraction scenario. Panels (a), (b), and (c) represent the unconfined aquifers in the short-term, mid-term, and long-term futures, respectively. Meanwhile, panels (d), (e), and (f) represent the confined aquifers in the short-term, mid-term, and long-term futures, respectively.
The third abstraction scenario projects the groundwater abstraction to decrease, which is influenced by the relocation of Indonesia's capital city. In this scenario, the groundwater is simulated as partially replenished between 2050 and 2100. This is indicated by the projected piezometric head drawdown that reaches 12.61 m in 2050 but is calculated at 11.75 m in 2100. Granted that 11.75 m remains a net negative of groundwater head in the future, subsurface flow (and therefore replenishment) requires a long time to reach an equilibrium. The fact that the piezometric head drawdown decreases signals an improving situation in scenario 3. Having said that, the groundwater table in the unconfined aquifer is projected to decrease by up to 2.58 and 2.93 m in RCP4.5 and RCP8.5, respectively. However, its drawdown area is projected to be smaller, going from 80.8% and 83.6% in 2030 for RCP4.5 and RCP8.5, respectively, to 75.5% and 76.7% in 2100. The response of groundwater replenishment is unique between the aquifer layers: the unconfined aquifer primarily reduces the drawdown area, while the confined aquifer relaxes the magnitude of the piezometric head drawdown. Nevertheless, it presents an opportunity for groundwater replenishment in the future given the right policy and management in the study area.
Table 3 summarizes the maximum groundwater head drawdown in the climatic and anthropogenic scenarios, checkpoints (short-term, mid-term, and long-term futures), and layers (unconfined and confined aquifers) under study. The table shows that the anthropogenic factor has a more dominant influence on future groundwater storage relative to the climatic factor. This is indicated as different RCPs in an abstraction scenario result in smaller changes in values compared to different abstraction scenarios in a climatic scenario. We can also see an increase in the impact over time and a more severe impact on the confined groundwater head compared to the unconfined groundwater table.
3.4 Groundwater storage projection
As stated above and shown in Fig. 8, the drawdown due to the groundwater abstraction is highly congregated spatially. In the unconfined layer, the drawdown area is distributed under the abstraction area, while the area close to water bodies is less impacted. This occurs due to surface water and groundwater interaction, where the groundwater table is also regulated by the surface water elevation aside from the subsurface flow. In the confined aquifer, the highly elevated area on the surface is much less impacted compared to the one in the overlying aquifer, and the drawdown area is, in general, located directly under the abstraction area. Using only the groundwater head, although useful, could not capture the whole picture of the basin's groundwater regime projection. Therefore, we also assess the groundwater projections from the perspective of the integrated aquifer water balance and the integrated cumulative storage changes over time.
Figure 9 shows the magnitude of the projected recharge relative to the projected groundwater abstraction. The groundwater recharge, as suggested by the above simulations, is relatively constant, with small fluctuations coming from the seasonal temporal variability. The less dominant effect of the climatic variables is also visible from the similarity of the groundwater recharge values between the two RCPs. On the other hand, the groundwater abstraction before 2020 was similar between the scenarios. After the baseline period, this started to diverge from increasing to decreasing projections. The figure also shows that, between 2020 and 2025, the volume of groundwater abstraction starts to surpass that of groundwater recharge, esurpassing the turning point in the aquifer water balance components. It appears that, before 2023, the total integrated volume of the groundwater abstraction is still slightly below the total integrated volume of the groundwater recharge. This does not mean that the whole basin water table is rising, though, as spatially neither of the displayed variables is uniformly distributed. Added to this is the fact that groundwater moves relatively slowly compared to surface water. Therefore, a long time is required for the groundwater in the mountainous region to flow downstream, compensating for the groundwater abstraction in the urban area. After 2023, in the scenario where the groundwater abstraction increases, the total volume of groundwater abstraction is projected to surpass the groundwater recharge, making it implausible to replenish the groundwater storage. In the other two groundwater abstraction scenarios, the total groundwater abstraction volume is projected to still be slightly below the groundwater recharge. However, this does not mean that the groundwater storage is replenished. As mentioned above, groundwater flows at a low velocity. Therefore, reaching an equilibrium over the integrated basin area would require a long time. Secondly, the groundwater storage is also controlled by other fluxes and, most importantly, the surface water and groundwater interaction. The fact that the baseflow of the river (partially indicated in Fig. 7a) does not project a decrease in values shows that the groundwater constantly supplies the river baseflow, thereby decreasing the groundwater storage.
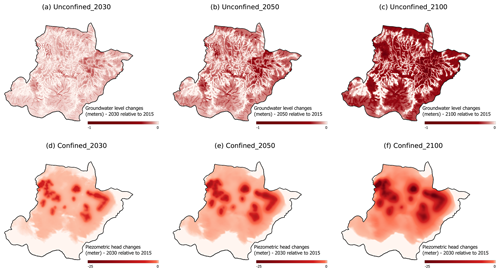
Figure 9The aquifer water balance of groundwater recharge and groundwater abstraction from 2015 to 2100 for all the climatic and anthropogenic scenarios.
Similar to the projected groundwater level assessment, six outcomes are produced from the combination of two climatic and three anthropogenic scenarios. Figure 10 shows the trajectory of each outcome in terms of its accumulated groundwater storage changes relative to the one in 2015 as a benchmark. Consistent with the aforementioned results, the difference between the climatic scenarios is very small, as lines with the same color almost intersect. However, the impact of the diverging abstraction scenario is visually apparent and even results in diverging groundwater storage change. Using the gradient of the storage depletion accumulation for scenario 2 as a benchmark, it becomes up to 3.43 times steeper for scenario 1 and up to 0.40 times milder for scenario 3 during the most extreme year, with both propagating in curve-shaped lines. The lines' shapes have important messages, as they indicate the uniquely diverging results of deteriorating, sustained, and improving groundwater storage depletion in the first, second, and third scenarios, respectively. It is also notable that, despite the indication of confined groundwater replenishment from the groundwater level perspective, assessment of the groundwater storage change suggests otherwise, as is discussed further in Sect. 4.2.
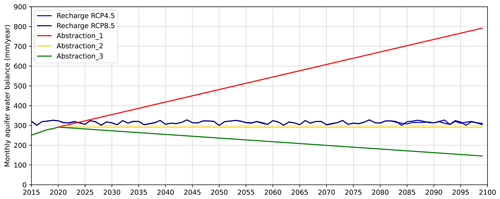
Figure 10The cumulative projected groundwater storage changes from 2015 to 2100 for all the climatic and anthropogenic scenarios.
In the constant abstraction scenario, the Bandung groundwater storage is projected to lose almost 2 × 1010 m3 in the upcoming 85 years. While the number might seem exaggerated, it is actually equivalent to an average of 0.54 mm per day of storage depletion. The rate of storage depletion is relatively constant throughout the short-term, mid-term, and long-term futures in this scenario, as per the abstraction rate. In the increasing abstraction scenario, the long-term storage depletion is projected to be 1.17 mm per day on average. This is a result of an escalating depletion, as the storage loss is averaged at 0.53 mm per day between 2015 and 2030, 0.86 mm per day between 2030 and 2050, and 1.48 mm per day between 2050 and 2100. In the decreasing abstraction scenario, the long-term storage depletion is projected at 0.40 mm per day on average. This is the result of a withering depletion, as the storage loss is averaged at 0.54 mm per day between 2015 and 2030, 0.49 mm per day between 2030 and 2050, and 0.33 mm per day between 2050 and 2100.
4.1 Projection uncertainty
In climate-projection-related studies, uncertainties are unavoidable as they are propagated from multiple sources: the natural climate variability, the climate model, and the emission scenario (Latif, 2011). The natural climate variability is highly uncertain, as Buser et al. (2010) suggested an extrapolative nature of climate variables, which involved different biases in the scenario and the control period. Each model also has different responses to climatology and perturbation component uncertainties, for example, as stated by Adachi et al. (2019). To tackle the wide range of uncertainty bounds, many studies propose using an ensemble of climate projection products (Hawkins et al., 2016; Rajczak and Schär, 2017). Meanwhile, other studies promote the efficiency of bias correction in reducing the uncertainties of climate projection products, as such a method that takes into account the ground truth estimates of the corresponding climate variables (Lange, 2019; Wu et al., 2022).
The climate projections resulting from different model groups have varying estimates and uncertainties. However, we believe that these uncertainties have been addressed in our study. First, the focus of the hydrological simulation is groundwater recharge, which is not solely influenced by climate variables. Indeed, climate variables play an important role, as they provide input to the basin, which further generates recharge. However, the control on effective groundwater recharge is also regulated by catchment features, such as the soil moisture capacity, surface water cycle, and subsurface properties. With the other simulation components' relatively known values, in this case the Wflow_sbm model parameterization, the groundwater recharge estimates, therefore, are not solely impacted by the uncertainties of the climate projections. Second, the primary goal of the study is to project the groundwater availability. With a relatively lower range of projected change in future groundwater recharge, previous studies suggested that the anthropogenic factor plays a larger role and involves a wider range of future uncertainties compared to the climatic factor (Mustafa et al., 2019; Aslam et al., 2022). In our scenario, the three abstraction scenarios that span increasing to decreasing projections are expected to be able to outweigh the uncertainty bounds propagated from the climatic scenario. All the results from our projection in this study support the claim: in every scenario, the change in groundwater abstraction always produces significantly higher influences on the groundwater table and storage compared to the climatic scenario. Third, we also apply a bias correction method to the climate projection products, which has been proven in previous studies to effectively reduce climate projection uncertainties (Rahimi et al., 2021; Wu et al., 2022). Table 2, columns (1), (2), and (3), shows a remarkable improvement in the bias-corrected climate model output. By bias-correcting the projected climate variables and taking into account the historical high-resolution ground truth data as the benchmark, we believe that the uncertainties of the climate projection have been significantly reduced.
4.2 Impact assessment on future groundwater level projections
As shown in the simulation workflow (Fig. 2), the future groundwater status is projected by altering the climate forcing input and groundwater abstraction as the boundary conditions. The former imparts its contribution to groundwater recharge estimates. However, as shown in Fig. 6b, there are significant differences in the impact of climate variables' changes on the surface and subsurface components of the water cycle. The rainfall median, not considering the seasonal fluctuation, is projected to change (either increase or decrease) up to 31.03 % and 28.36 % for RCP4.5 and RCP8.5, respectively. On the other hand, the projected change in the groundwater recharge is less than 1 %, indicating a slower response of subsurface components to the climate change projection.
This result is limited, however, by the nature of the model's one-way coupling. In our model setup, groundwater recharge is fully controlled by the surface processes and the pseudo water table. Physically, groundwater storage depletion due to groundwater abstraction might decrease the water table. In this process, there would be more space for water to infiltrate, indicating two-way feedback between groundwater abstraction and groundwater recharge. A one-way coupled model, unfortunately, is not capable of incorporating such two-way processes into its simulation. This further proves that groundwater abstraction and basin properties possess equal, if not more, importance compared to the climate forcing in groundwater recharge projection. This looks site-specific, however, depending on the basin features, especially the land use or land cover type, the soil maximum capacity, and the subsurface properties. In regions with higher margins between the groundwater recharge and soil capacity, meaning that the soil condition is not generally wet, changes in climatic factors (i.e., effective precipitation) will have a higher influence on changes in groundwater recharge. This also highlights the importance of basin-scale information in climate projection studies (Bhave et al., 2013; Jackson et al., 2015; Marcos-Garcia et al., 2023), which have been conducted largely for global-scale studies.
Additionally, the projected groundwater recharge is simulated under the assumption of constant soil characteristics represented by the MaxLeakage parameter in the Wflow_sbm model. Meanwhile, soil characteristics such as soil permeability, rock formation, soil infiltration capacity, and soil type and structure might evolve (Zhang and Wang, 2023), albeit on a much longer timescale than the surface features. In our opinion, changes in soil characteristics would influence the MaxLeakage parameter, thereby impacting the simulated projected groundwater recharge. While these changes are gradual, they are likely to affect recharge over time, potentially causing significant deviations from current projections. However, the tendency of soil characteristics to increasingly or decreasingly evolve long term, particularly regarding groundwater recharge generation, remains uncertain (Cook et al., 2022; Wu et al., 2020a). Accurate future projections will require constant soil monitoring and modeling. This further highlights the importance of data, especially soil-related data and hydrological information, as the benchmark for model calibration and verification. Consistent data assimilation that is validated through updated observation and simulation would decrease the uncertainties, making it possible to use the MaxLeakage parameter setup dynamic over time.
The uncertainties of future anthropogenic factors, considering their large influence, should be the primary focus of future groundwater management. Figure 9 emphasizes the importance of the current policies in managing groundwater abstraction, as between the years 2020 and 2025 the annual groundwater abstraction is estimated to surpass the total annual recharge volumetric-wise. This also shows that the previous depleting groundwater storage is controlled by the fluxes within the groundwater storage water balance components, mainly the surface–groundwater interaction. This is supported by Fig. 8, where the groundwater table in the unconfined storage depletes mostly in the mountainous region, contributing to conserving the river baseflow due to the groundwater abstraction along the river's downstream part. While the climatic factor is relatively intractable, groundwater abstraction activities, in terms of rates, volumes, and spatial distributions, are relatively manageable through regional or local groundwater policies, not to mention their more influential impact on the groundwater regime. A previous study suggests that groundwater abstraction has an even greater influence on river baseflow compared to changes in climate (Taie Semiromi and Koch, 2020). Improving understanding of the subsurface response and bridging the key gap between science and policy on the matter of groundwater abstraction should be the main focus and responsibility of all the involved stakeholders.
The simulation results also reveal the importance of multi-perspective assessment in groundwater regimes. On the one hand, Table 3 implies that the groundwater situation in the confined aquifer improves in scenario 3 of groundwater abstraction, where the maximum piezometric head drawdown from 2100 is lower than the one from 2050. On the other hand, Fig. 10 shows that the groundwater storage is still depleting, as shown by the negative gradient of all the lines, including ones from abstraction scenario 3. Such discrepancies occur as the two assessment variables, the groundwater head and the groundwater storage, represent two different dimensions of the groundwater status. The groundwater head represents a point, or a single grid, value that constitutes local features, while the groundwater storage evaluates the basin-integrated response. Referring to only one assessment variable could lead to a misunderstanding of the process of the groundwater flow system. We discuss the interpretation of the two conflicting numbers in the following section.
4.3 Opportunities for groundwater replenishment
As shown in Fig. 10, the different gradients represent diverging directions of the groundwater storage over time. While all the results accumulate negative changes, the rate at which the groundwater storage depletes differs among the scenarios. With a decreasing groundwater abstraction scenario in the future, the depletion rate is projected to decline, as expected. Admittedly, groundwater replenishment might take a comparably long time to reach a “new equilibrium”, considering the subsurface low-flow velocity. The current declining, but slower, groundwater storage depletion, therefore, could be interpreted in two ways: either (1) the groundwater storage is indeed still in a deteriorating trend or (2) the groundwater storage is actually replenished but has not yet reached the new equilibrium state as the subsurface timescale is longer than the surface's. The latter hypothesis is supported by the values in Table 3, which at first glance might seem inconsistent with the results in Fig. 10. By any means, the results in scenario 3, which are highly possible due to the capital city's relocation plan, suggest an opportunity for future groundwater replenishment, although it will take some time to yield a positive turning point. Consistent future groundwater head monitoring in the study area could provide crucial insight, which will assist in deriving adaptation policies in response to the capital city's relocation.
We also notice the different responses of groundwater “replenishment”, which are contrasting between the aquifer layers. In the unconfined aquifer, the primary response of the groundwater improvement is to reduce the impacted drawdown area. While the groundwater table is still decreasing in all future checkpoints, there are smaller (simulated) drawdown areas in 2100, even compared to the ones in 2030. In contrast, the confined aquifer relaxes the magnitude of the piezometric head drawdown while maintaining the drawdown area. This, presumably, is directly related to the spatial distribution of groundwater abstraction. The groundwater abstraction applied in the unconfined aquifer is more widespread with lower rates of abstraction. Therefore, the drawdown area is highly dependent on the spatial distribution. In contrast to the unconfined aquifer, the groundwater abstraction applied in the confined aquifer is more concentrated, with intense rates of abstraction. Decreasing the rate, consequently, has noticeable influences on the stressed piezometric head. This reveals an important opportunity for future groundwater policies: the governance of groundwater abstraction authorization should include not only the abstraction rate limitation, but also the consideration of future and integrated geospatial planning of the study area.
In this study, we develop groundwater status projection in multiple climatic and diverging anthropogenic scenarios. We simulate the groundwater recharge projection using the hydrological model Wflow_sbm. The climate projection forcing is taken from the CMIP6 MRI-ESM2-0 model group, including the projected rainfall and the projected temperature and radiation data to estimate potential evapotranspiration. We force the Wflow_sbm model with two RCP scenarios: RCP4.5 and RCP8.5. Further, using the groundwater recharge projection as the groundwater flow driver, we simulate the subsurface flow under groundwater abstraction as the boundary condition. We develop three diverging groundwater abstraction scenarios: increasing groundwater abstraction as the most common approach, constant abstraction as the benchmark, and decreasing abstraction as a possibility. We take the Bandung groundwater basin in Indonesia as our test case, which is located near Jakarta, the current capital city of Indonesia. The Bandung groundwater basin has a wide range of uncertainties in terms of future groundwater abstraction in response to the Indonesian capital city's relocation plan, thereby nicely covering the three developed anthropogenic scenarios.
We applied the bias correction method of ISIMIP3b to the CMIP6 climate projection data before forcing it to the Wflow_sbm model. The bias correction reduces the uncertainty of the climate variables' projection, as the bias-corrected historical data show consistent statistical distributions in the ground truth data. The future rainfall and temperature median are projected to change by 31.03 % and 10.71 % in RCP4.5 and by 28.36 % and 13.10 % in RCP8.5. Future groundwater recharge projection reveals the dominant control of the soil component in generating the groundwater recharge in the study area. The fact that there is a change of less than 1 % projected for the groundwater recharge variable in both climatic scenarios shows that, most of the time, the recharge is already at its maximum capacity. During the rainy season, rainfall intensification could not generate more recharge. On the other hand, during the dry season, increasing rainfall drove higher recharge. However, during the dry period that is projected to be even drier, the deficit of groundwater recharge almost balances out this additional recharge.
As expected, in the increasing and constant groundwater abstraction scenario, the groundwater status is projected to drop. The maximum groundwater head drawdown increases over time, the drawdown area expands, and the groundwater storage depletes. However, a positive sign of groundwater replenishment potential is shown in the decreasing groundwater abstraction scenario, despite the conflicting numbers between the point-based groundwater level assessment and the basin-integrated groundwater storage assessment. Despite being slow and occurring between 2050 and 2100, there is a sign that the groundwater storage is going in the right direction to be refilled. It is also clear that the groundwater table is highly regulated by not only the volume and rate, but also the spatial distribution of groundwater abstraction.
Comparing the results of climatic and anthropogenic impacts, we conclude that groundwater abstraction has a more significant impact on simulated future groundwater availability in areas with high groundwater dependency, such as the Bandung groundwater basin. However, this has to be approached on a case-by-case basis considering the spatial variability of the climatic factor, anthropogenic factor, and hydrology and hydrogeological properties of the area under study. The context of the projection of rainfall, evaporation, and river discharge is also important when applying such an analysis to a wider context beyond a case study. The findings of this study are expected to assist in deriving and improving the current and future groundwater policies and management strategies, in particular for the Bandung groundwater basin and in general for other groundwater basins around the world that face similar issues.
The climate model data are publicly available on the Copernicus Climate Data Store website https://doi.org/10.22033/ESGF/CMIP6.621 (Yukimoto et al., 2019), which can be accessed online at https://doi.org/10.24381/cds.c866074c (Copernicus Climate Change Service, 2021). The other data used in this study are stored in the 4TU Research Data repository, which is available online at https://doi.org/10.4121/d9706a2a-b77b-412f-a3aa-6e22bd8ddf4a (Rusli et al., 2024).
SRR, VFB, and AHW conceptualized the study together. SM provided the theoretical background and the related literature. SRR managed the input data for the one-way coupled model. AHW prepared the model setup for the hydrological simulation, and VFB supervised the development of the groundwater flow model. SRR ran the model simulations, while VFB, SMTM, and AHW validated the results. SRR wrote the original draft, while VFB, SMTM, and AHW contributed to reviewing the manuscript before its finalization.
At least one of the (co-)authors is a member of the editorial board of Hydrology and Earth System Sciences. The peer-review process was guided by an independent editor, and the authors also have no other competing interests to declare.
Publisher’s note: Copernicus Publications remains neutral with regard to jurisdictional claims made in the text, published maps, institutional affiliations, or any other geographical representation in this paper. While Copernicus Publications makes every effort to include appropriate place names, the final responsibility lies with the authors.
The first author would like to acknowledge the Indonesia Endowment Fund for Education (LPDP) of the Ministry of Finance, Republic of Indonesia, for its scholarship funding support. This research is also significantly supported by the Office of Energy and Mineral Resources (ESDM) of West Java through access to the available data. We acknowledge the World Climate Research Programme, which, through its Working Group on Coupled Modelling, coordinated and promoted CMIP6. We thank the climate modeling groups for producing and making available their model output, the Earth System Grid Federation (ESGF) for archiving the data and providing access to them, and the many funding agencies that support CMIP6 and ESGF.
This research has been supported by the Lembaga Pengelola Dana Pendidikan (grant no. LOG2429202221533732).
This paper was edited by Fadji Zaouna Maina and reviewed by three anonymous referees.
Abidin, H. Z., Gumilar, I., Andreas, H., Murdohardono, D., and Fukuda, Y.: On causes and impacts of land subsidence in Bandung Basin, Indonesia, Environ. Earth Sci., 68, 1545–1553, https://doi.org/10.1007/s12665-012-1848-z, 2013. a
Adachi, S. A., Nishizawa, S., Ando, K., Yamaura, T., Yoshida, R., Yashiro, H., Kajikawa, Y., and Tomita, H.: An evaluation method for uncertainties in regional climate projections, Atmos. Sci. Lett., 20, e877, https://doi.org/10.1002/asl.877, 2019. a
Ansari, A. H. M., Umar, R., us Saba, N., and Sarah, S.: Assessment of Current and Future Groundwater Stress through Varied Scenario Projections in Urban and Rural Environment in Parts of Meerut District, Uttar Pradesh in Ganges Sub-basin, J. Geol. Soc. India, 97, 927–934, https://doi.org/10.1007/s12594-021-1793-0, 2021. a
Anurag, H. and Ng, G.-H. C.: Assessing future climate change impacts on groundwater recharge in Minnesota, J. Hydrol., 612, 128112, https://doi.org/10.1016/j.jhydrol.2022.128112, 2022. a
Aslam, R. A., Shrestha, S., Usman, M. N., Khan, S. N., Ali, S., Sharif, M. S., Sarwar, M. W., Saddique, N., Sarwar, A., Ali, M. U., and Arshad, A.: Integrated SWAT-MODFLOW Modeling-Based Groundwater Adaptation Policy Guidelines for Lahore, Pakistan under Projected Climate Change, and Human Development Scenarios, Atmosphere, 13, 2001, https://doi.org/10.3390/atmos13122001, 2022. a, b
Baghel, T., Babel, M. S., Shrestha, S., Salin, K. R., Virdis, S. G., and Shinde, V. R.: A generalized methodology for ranking climate models based on climate indices for sector-specific studies: An application to the Mekong sub-basin, Sci. Total Environ., 829, 154551, https://doi.org/10.1016/j.scitotenv.2022.154551, 2022. a
Bakker, M., Post, V., Langevin, C. D., Hughes, J. D., White, J. T., Starn, J. J., and Fienen, M. N.: Scripting MODFLOW Model Development Using Python and FloPy, Groundwater, 54, 733–739, https://doi.org/10.1111/gwat.12413, 2016. a
Bhave, A. G., Mishra, A., and Groot, A.: Sub-basin scale characterization of climate change vulnerability, impacts and adaptation in an Indian River basin, Reg. Environ. Change, 13, 1087–1098, https://doi.org/10.1007/s10113-013-0416-8, 2013. a
Bierkens, M. F. P. and Wada, Y.: Non-renewable groundwater use and groundwater depletion: a review, Environ. Res. Lett., 14, 063002, https://doi.org/10.1088/1748-9326/ab1a5f, 2019. a
Brewington, L., Keener, V., and Mair, A.: Simulating Land Cover Change Impacts on Groundwater Recharge under Selected Climate Projections, Maui, Hawaii, Remote Sens., 11, 3048, https://doi.org/10.3390/rs11243048, 2019. a
Buchhorn, M., Smets, B., Bertels, L., Roo, B. D., Lesiv, M., Tsendbazar, N.-E., Li, L., and Tarko, A.: Copernicus Global Land Service: Land Cover 100m: version 3 Globe 2015–2019: Product User Manual, https://doi.org/10.5281/zenodo.3938963, 2020. a
Buser, C. M., Künsch, H. R., and Weber, A.: Biases and Uncertainty in Climate Projections, Scand. J. Stat., 37, 179–199, https://doi.org/10.1111/j.1467-9469.2009.00686.x, 2010. a
Chang, S. W., Chung, I.-M., Kim, M.-G., and Yifru, B. A.: Vulnerability assessment considering impact of future groundwater exploitation on coastal groundwater resources in northeastern Jeju Island, South Korea, Environ. Earth Sci., 79, 498, https://doi.org/10.1007/s12665-020-09254-2, 2020. a
Chen, H., Xue, Y., and Qiu, D.: Numerical simulation of the land subsidence induced by groundwater mining, Cluster Comput., 26, 3647–3656, https://doi.org/10.1007/s10586-022-03771-4, 2022. a
Cook, P., Black, E., Verhoef, A., Macdonald, D., and Sorensen, J.: Projected increases in potential groundwater recharge and reduced evapotranspiration under future climate conditions in West Africa, J. Hydrol. Regional Studies, 41, 101076, https://doi.org/10.1016/j.ejrh.2022.101076, 2022. a
Copernicus Climate Change Service: ERA5-Land hourly data from 2001 to present, Copernicus [data set], https://doi.org/10.24381/CDS.E2161BAC, 2019. a
Copernicus Climate Change Service: CMIP6 climate projections, Copernicus [data set], https://doi.org/10.24381/CDS.C866074C, 2021. a, b, c
Custodio, E., del Carmen Cabrera, M., Poncela, R., Puga, L.-O., Skupien, E., and del Villar, A.: Groundwater intensive exploitation and mining in Gran Canaria and Tenerife, Canary Islands, Spain: Hydrogeological, environmental, economic and social aspects, Sci. Total Environ., 557–558, 425–437, https://doi.org/10.1016/j.scitotenv.2016.03.038, 2016. a
Davamani, V., John, J. E., Poornachandhra, C., Gopalakrishnan, B., Arulmani, S., Parameswari, E., Santhosh, A., Srinivasulu, A., Lal, A., and Naidu, R.: A Critical Review of Climate Change Impacts on Groundwater Resources: A Focus on the Current Status, Future Possibilities, and Role of Simulation Models, Atmosphere, 15, 122, https://doi.org/10.3390/atmos15010122, 2024. a
de Bruin, H. A. R., Trigo, I. F., Bosveld, F. C., and Meirink, J. F.: A Thermodynamically Based Model for Actual Evapotranspiration of an Extensive Grass Field Close to FAO Reference, Suitable for Remote Sensing Application, J. Hydrometeorol., 17, 1373–1382, https://doi.org/10.1175/jhm-d-15-0006.1, 2016. a, b, c
de Luna, R. M. R., Garnés, S. J. d. A., Cabral, J. J. d. S. P., and dos Santos, S. M.: Groundwater overexploitation and soil subsidence monitoring on Recife plain (Brazil), Nat. Hazards, 86, 1363–1376, https://doi.org/10.1007/s11069-017-2749-y, 2017. a
de Vries, W. T. and Schrey, M.: Geospatial Approaches to Model Renewable Energy Requirements of the New Capital City of Indonesia, Frontiers in Sustainable Cities, 4, https://doi.org/10.3389/frsc.2022.848309, 2022. a
Döll, P., Hoffmann-Dobrev, H., Portmann, F., Siebert, S., Eicker, A., Rodell, M., Strassberg, G., and Scanlon, B.: Impact of water withdrawals from groundwater and surface water on continental water storage variations, J. Geodyn., 59–60, 143–156, https://doi.org/10.1016/j.jog.2011.05.001, 2012. a
Eilander, D., van Verseveld, W., Yamazaki, D., Weerts, A., Winsemius, H. C., and Ward, P. J.: A hydrography upscaling method for scale-invariant parametrization of distributed hydrological models, Hydrol. Earth Syst. Sci., 25, 5287–5313, https://doi.org/10.5194/hess-25-5287-2021, 2021. a
Eyring, V., Bony, S., Meehl, G. A., Senior, C. A., Stevens, B., Stouffer, R. J., and Taylor, K. E.: Overview of the Coupled Model Intercomparison Project Phase 6 (CMIP6) experimental design and organization, Geosci. Model Dev., 9, 1937–1958, https://doi.org/10.5194/gmd-9-1937-2016, 2016. a, b
Funk, C., Peterson, P., Landsfeld, M., Pedreros, D., Verdin, J., Shukla, S., Husak, G., Rowland, J., Harrison, L., Hoell, A., and Michaelsen, J.: The climate hazards infrared precipitation with stations – a new environmental record for monitoring extremes, Scientific Data, 2, 1, https://doi.org/10.1038/sdata.2015.66, 2015. a, b, c
Gash, J. H. C.: An analytical model of rainfall interception by forests, Q. J. Roy. Meteor. Soc., 105, 43–55, https://doi.org/10.1002/qj.49710544304, 1979. a
Gaye, C. B. and Tindimugaya, C.: Review: Challenges and opportunities for sustainable groundwater management in Africa, Hydrogeol. J., 27, 1099–1110, https://doi.org/10.1007/s10040-018-1892-1, 2019. a
Gebremicael, T., Mohamed, Y., and der Zaag, P. V.: Attributing the hydrological impact of different land use types and their long-term dynamics through combining parsimonious hydrological modelling, alteration analysis and PLSR analysis, Sci. Total Environ., 660, 1155–1167, https://doi.org/10.1016/j.scitotenv.2019.01.085, 2019. a
Gleeson, T., Cuthbert, M., Ferguson, G., and Perrone, D.: Global Groundwater Sustainability, Resources, and Systems in the Anthropocene, Annu. Rev. Earth Pl. Sc., 48, 431–463, https://doi.org/10.1146/annurev-earth-071719-055251, 2020. a
Gumilar, I., Abidin, H. Z., Hutasoit, L. M., Hakim, D. M., Sidiq, T. P., and Andreas, H.: Land Subsidence in Bandung Basin and its Possible Caused Factors, Proced. Earth Plan. Sc., 12, 47–62, https://doi.org/10.1016/j.proeps.2015.03.026, 2015. a
Hackbarth, T. X. and de Vries, W. T.: An Evaluation of Massive Land Interventions for the Relocation of Capital Cities, Urban Science, 5, 25, https://doi.org/10.3390/urbansci5010025, 2021. a
Hashimoto, R., Kazama, S., Hashimoto, T., Oguma, K., and Takizawa, S.: Planning methods for conjunctive use of urban water resources based on quantitative water demand estimation models and groundwater regulation index in Yangon City, Myanmar, J. Clean. Prod., 367, 133123, https://doi.org/10.1016/j.jclepro.2022.133123, 2022. a
Hassaballah, K., Mohamed, Y., Uhlenbrook, S., and Biro, K.: Analysis of streamflow response to land use and land cover changes using satellite data and hydrological modelling: case study of Dinder and Rahad tributaries of the Blue Nile (Ethiopia–Sudan), Hydrol. Earth Syst. Sci., 21, 5217–5242, https://doi.org/10.5194/hess-21-5217-2017, 2017. a
Hawkins, E., Smith, R. S., Gregory, J. M., and Stainforth, D. A.: Irreducible uncertainty in near-term climate projections, Clim. Dynam., 46, 3807–3819, https://doi.org/10.1007/s00382-015-2806-8, 2016. a
Healy, A., Upton, K., Capstick, S., Bristow, G., Tijani, M., MacDonald, A., Goni, I., Bukar, Y., Whitmarsh, L., Theis, S., Danert, K., and Allan, S.: Domestic groundwater abstraction in Lagos, Nigeria: a disjuncture in the science-policy-practice interface?, Environ. Res. Lett., 15, 045006, https://doi.org/10.1088/1748-9326/ab7463, 2020. a
Hengl, T., de Jesus, J. M., Heuvelink, G. B. M., Gonzalez, M. R., Kilibarda, M., Blagotić, A., Shangguan, W., Wright, M. N., Geng, X., Bauer-Marschallinger, B., Guevara, M. A., Vargas, R., MacMillan, R. A., Batjes, N. H., Leenaars, J. G. B., Ribeiro, E., Wheeler, I., Mantel, S., and Kempen, B.: SoilGrids250m: Global gridded soil information based on machine learning, PLOS ONE, 12, e0169748, https://doi.org/10.1371/journal.pone.0169748, 2017. a
Hua, L., Zhao, T., and Zhong, L.: Future changes in drought over Central Asia under CMIP6 forcing scenarios, J. Hydrol. Regional Studies, 43, 101191, https://doi.org/10.1016/j.ejrh.2022.101191, 2022. a
Hughes, A., Mansour, M., Ward, R., Kieboom, N., Allen, S., Seccombe, D., Charlton, M., and Prudhomme, C.: The impact of climate change on groundwater recharge: National-scale assessment for the British mainland, J. Hydrol., 598, 126336, https://doi.org/10.1016/j.jhydrol.2021.126336, 2021. a
Imhoff, R. O., van Verseveld, W. J., van Osnabrugge, B., and Weerts, A. H.: Scaling Point-Scale (Pedo)transfer Functions to Seamless Large-Domain Parameter Estimates for High-Resolution Distributed Hydrologic Modeling: An Example for the Rhine River, Water Resour. Res., 56, 4, https://doi.org/10.1029/2019wr026807, 2020. a
Indonesia: Detail masterplan on Indonesia capital city, https://www.ikn.go.id/ (last access: 19 June 2024), 2022. a
IPCC: Climate Change 2021: The Physical Science Basis. Contribution of Working Group I to the Sixth Assessment Report of the Intergovernmental Panel on Climate Change, Cambridge University Press, Cambridge, United Kingdom and New York, NY, USA, in press, https://doi.org/10.1017/9781009157896, 2021. a, b
Iqbal, Z., Shahid, S., Ahmed, K., Ismail, T., Ziarh, G. F., Chung, E.-S., and Wang, X.: Evaluation of CMIP6 GCM rainfall in mainland Southeast Asia, Atmos. Res., 254, 105525, https://doi.org/10.1016/j.atmosres.2021.105525, 2021. a
Jackson, C. R., Bloomfield, J. P., and Mackay, J. D.: Evidence for changes in historic and future groundwater levels in the UK, Prog. Phys. Geog., 39, 49–67, https://doi.org/10.1177/0309133314550668, 2015. a
Jyrkama, M. I. and Sykes, J. F.: The impact of climate change on spatially varying groundwater recharge in the grand river watershed (Ontario), J. Hydrol., 338, 237–250, https://doi.org/10.1016/j.jhydrol.2007.02.036, 2007. a
Kawai, H., Yukimoto, S., Koshiro, T., Oshima, N., Tanaka, T., Yoshimura, H., and Nagasawa, R.: Significant improvement of cloud representation in the global climate model MRI-ESM2, Geosci. Model Dev., 12, 2875–2897, https://doi.org/10.5194/gmd-12-2875-2019, 2019. a
Kodir, A., Hadi, N., Astina, I., Taryana, D., Ratnawati, N., and Idris: The dynamics of community response to the development of the New Capital (IKN) of Indonesia, in: Development, Social Change and Environmental Sustainability, Routledge, 57–61, https://doi.org/10.1201/9781003178163-13, 2021. a
Krasting, J. P., John, J. G., Blanton, C., McHugh, C., Nikonov, S., Radhakrishnan, A., Rand, K., Zadeh, N. T., Balaji, V., Durachta, J., Dupuis, C., Menzel, R., Robinson, T., Underwood, S., Vahlenkamp, H., Dunne, K. A., Gauthier, P. P., Ginoux, P., Griffies, S. M., Hallberg, R., Harrison, M., Hurlin, W., Malyshev, S., Naik, V., Paulot, F., Paynter, D. J., Ploshay, J., Reichl, B. G., Schwarzkopf, D. M., Seman, C. J., Silvers, L., Wyman, B., Zeng, Y., Adcroft, A., Dunne, J. P., Dussin, R., Guo, H., He, J., Held, I. M., Horowitz, L. W., Lin, P., Milly, P., Shevliakova, E., Stock, C., Winton, M., Wittenberg, A. T., Xie, Y., and Zhao, M.: NOAA-GFDL GFDL-ESM4 model output prepared for CMIP6 CMIP, WDC Climate [data set], https://doi.org/10.22033/ESGF/CMIP6.1407, 2018. a
Lange, S.: Trend-preserving bias adjustment and statistical downscaling with ISIMIP3BASD (v1.0), Geosci. Model Dev., 12, 3055–3070, https://doi.org/10.5194/gmd-12-3055-2019, 2019. a, b, c
Lange, S.: ISIMIP3BASD, Zenodo [code], https://doi.org/10.5281/zenodo.4686991, 2021. a
Latif, M.: Uncertainty in climate change projections, J. Geochem. Explor., 110, 1–7, https://doi.org/10.1016/j.gexplo.2010.09.011, 2011. a
Lili, Y., Minhua, L., Fei, C., Yueyuan, D., and Cuimei, L.: Practices of groundwater over-exploitation control in Hebei Province, Water Policy, 22, 591–601, https://doi.org/10.2166/wp.2020.183, 2020. a
Liu, K., Zhang, J., and Wang, M.: Drivers of Groundwater Change in China and Future Projections, Remote Sens., 14, 4825, https://doi.org/10.3390/rs14194825, 2022. a
López López, P., Wanders, N., Schellekens, J., Renzullo, L. J., Sutanudjaja, E. H., and Bierkens, M. F. P.: Improved large-scale hydrological modelling through the assimilation of streamflow and downscaled satellite soil moisture observations, Hydrol. Earth Syst. Sci., 20, 3059–3076, https://doi.org/10.5194/hess-20-3059-2016, 2016. a
Mancuso, M., Santucci, L., and Carol, E.: Effects of intensive aquifers exploitation on groundwater salinity in coastal wetlands, Hydrol. Process., 34, 2313–2323, https://doi.org/10.1002/hyp.13727, 2020. a
Marcos-Garcia, P., Pulido-Velazquez, M., Sanchis-Ibor, C., García-Mollá, M., Ortega-Reig, M., Garcia-Prats, A., and Girard, C.: From local knowledge to decision making in climate change adaptation at basin scale. Application to the Jucar River Basin, Spain, Climatic Change, 176, 38, https://doi.org/10.1007/s10584-023-03501-8, 2023. a
Martinez, R. and Masron, I. N.: Jakarta: A city of cities, Cities, 106, 102868, https://doi.org/10.1016/j.cities.2020.102868, 2020. a
McColl, K. A., Vogelzang, J., Konings, A. G., Entekhabi, D., Piles, M., and Stoffelen, A.: Extended triple collocation: Estimating errors and correlation coefficients with respect to an unknown target, Geophys. Res. Lett., 41, 6229–6236, https://doi.org/10.1002/2014gl061322, 2014. a
Meixner, T., Manning, A. H., Stonestrom, D. A., Allen, D. M., Ajami, H., Blasch, K. W., Brookfield, A. E., Castro, C. L., Clark, J. F., Gochis, D. J., Flint, A. L., Neff, K. L., Niraula, R., Rodell, M., Scanlon, B. R., Singha, K., and Walvoord, M. A.: Implications of projected climate change for groundwater recharge in the western United States, J. Hydrol., 534, 124–138, https://doi.org/10.1016/j.jhydrol.2015.12.027, 2016. a
Meng, L., Shi, J., Zhai, Y., Zuo, R., Wang, J., Guo, X., Teng, Y., Gao, J., Xu, L., and Guo, B.: Ammonium Reactive Migration Process and Functional Bacteria Response along Lateral Runoff Path under Groundwater Exploitation, Sustainability, 14, 8609, https://doi.org/10.3390/su14148609, 2022. a
Momejian, N., Abou Najm, M., Alameddine, I., and El-Fadel, M.: Groundwater Vulnerability Modeling to Assess Seawater Intrusion: a Methodological Comparison with Geospatial Interpolation, Water Resour. Manag., 33, 1039–1052, https://doi.org/10.1007/s11269-018-2165-4, 2019. a
Mustafa, S. M. T., Abdollahi, K., Verbeiren, B., and Huysmans, M.: Identification of the influencing factors on groundwater drought and depletion in north-western Bangladesh, Hydrogeol. J., 25, 1357–1375, https://doi.org/10.1007/s10040-017-1547-7, 2017. a
Mustafa, S. M. T., Hasan, M. M., Saha, A. K., Rannu, R. P., Van Uytven, E., Willems, P., and Huysmans, M.: Multi-model approach to quantify groundwater-level prediction uncertainty using an ensemble of global climate models and multiple abstraction scenarios, Hydrol. Earth Syst. Sci., 23, 2279–2303, https://doi.org/10.5194/hess-23-2279-2019, 2019. a, b, c
Mutaqin, D. J., Muslim, M. B., and Rahayu, N. H.: Analisis Konsep Forest City dalam Rencana Pembangunan Ibu Kota Negara, Bappenas Working Papers, 4, 13–29, https://doi.org/10.47266/bwp.v4i1.87, 2021. a
Nugroho, H.: Pemindahan Ibu Kota Baru Negara Kesatuan Republik Indonesia ke Kalimantan Timur: Strategi Pemenuhan Kebutuhan dan Konsumsi Energi, Bappenas Working Papers, 3, 33–41, https://doi.org/10.47266/bwp.v3i1.53, 2020. a
Olarinoye, T., Foppen, J. W., Veerbeek, W., Morienyane, T., and Komakech, H.: Exploring the future impacts of urbanization and climate change on groundwater in Arusha, Tanzania, Water Int., 45, 497–511, https://doi.org/10.1080/02508060.2020.1768724, 2020. a
Oruc, S.: Performance of bias corrected monthly CMIP6 climate projections with different reference period data in Turkey, Acta Geophys., 70, 777–789, https://doi.org/10.1007/s11600-022-00731-9, 2022. a
Pardo-Igúzquiza, E., Collados-Lara, A. J., and Pulido-Velazquez, D.: Potential future impact of climate change on recharge in the Sierra de las Nieves (southern Spain) high-relief karst aquifer using regional climate models and statistical corrections, Environ. Earth Sci., 78, 598, https://doi.org/10.1007/s12665-019-8594-4, 2019.
Patle, G. T., Singh, D. K., and Sarangi, A.: Modelling of climate-induced groundwater recharge for assessing carbon emission from groundwater irrigation, Curr. Sci. India, 115, 64–73, https://www.jstor.org/stable/26978149 (last access: 19 June 2024), 2018. a
Pravitasari, A. E., Rustiadi, E., Mulya, S. P., Setiawan, Y., Fuadina, L. N., and Murtadho, A.: Identifying the driving forces of urban expansion and its environmental impact in Jakarta-Bandung mega urban region, IOP C. Ser. Earth Env., 149, 012044, https://doi.org/10.1088/1755-1315/149/1/012044, 2018. a
Rahiem, M. A.: Hydrogeological Information of Bandung Basin, Indonesia, https://malikarrahiem.shinyapps.io/BandungBasin/ (last access: 19 June 2024), 2020. a
Rahimi, R., Tavakol-Davani, H., and Nasseri, M.: An Uncertainty-Based Regional Comparative Analysis on the Performance of Different Bias Correction Methods in Statistical Downscaling of Precipitation, Water Resour. Manag., 35, 2503–2518, https://doi.org/10.1007/s11269-021-02844-0, 2021. a
Rajczak, J. and Schär, C.: Projections of Future Precipitation Extremes Over Europe: A Multimodel Assessment of Climate Simulations, J. Geophys. Res.-Atmos., 122, 10773–10800, https://doi.org/10.1002/2017JD027176, 2017. a
Rusli, S., Weerts, A., Taufiq, A., and Bense, V.: Estimating water balance components and their uncertainty bounds in highly groundwater-dependent and data-scarce area: An example for the Upper Citarum basin, J. Hydrol. Regional Studies, 37, 100911, https://doi.org/10.1016/j.ejrh.2021.100911, 2021. a, b, c, d, e
Rusli, S., Bense, V., Taufiq, A., and Weerts, A.: Quantifying basin-scale changes in groundwater storage using GRACE and one-way coupled hydrological and groundwater flow model in the data-scarce Bandung groundwater Basin, Indonesia, Groundwater for Sustainable Development, 22, 100953, https://doi.org/10.1016/j.gsd.2023.100953, 2023a. a, b, c, d, e, f, g, h, i, j
Rusli, S., Weerts, A., Mustafa, S., Irawan, D., Taufiq, A., and Bense, V.: Quantifying aquifer interaction using numerical groundwater flow model evaluated by environmental water tracer data: Application to the data-scarce area of Bandung groundwater basin, West Java, Indonesia, J. Hydrol. Regional Studies, 50, 101585, https://doi.org/10.1016/j.ejrh.2023.101585, 2023b. a, b, c, d, e, f, g, h, i, j, k
Rusli, S., Bense, V., Mustafa, S., and Weerts, A.: Data and models used for paper 'The impact of future climate projections and anthropogenic activities on basin-scale groundwater availability, 4TU.ResearchData [data set], https://doi.org/10.4121/d9706a2a-b77b-412f-a3aa-6e22bd8ddf4a, 2024. a
Shahid, S., Wang, X.-J., Moshiur Rahman, M., Hasan, R., Harun, S. B., and Shamsudin, S.: Spatial assessment of groundwater over-exploitation in northwestern districts of Bangladesh, J. Geol. Soc. India, 85, 463–470, https://doi.org/10.1007/s12594-015-0238-z, 2015. a
Siarkos, I., Sevastas, S., Mallios, Z., Theodossiou, N., and Ifadis, I.: Investigating groundwater vulnerability variation under future abstraction scenarios to estimate optimal pumping reduction rates, J. Hydrol., 598, 126297, https://doi.org/10.1016/j.jhydrol.2021.126297, 2021. a
Silva Jr., G. and Pizani, T.: Vulnerability assessment in coastal aquifers between Niterói and Rio das Ostras, Rio de Janeiro State, Brazil, Rev. Lat. Am. Hidrogeol., 3, 93–99, 2003. a
Smerdon, B. D.: A synopsis of climate change effects on groundwater recharge, J. Hydrol., 555, 125–128, https://doi.org/10.1016/j.jhydrol.2017.09.047, 2017. a
Soundala, P. and Saraphirom, P.: Impact of climate change on groundwater recharge and salinity distribution in the Vientiane basin, Lao PDR, J. Water Clim. Change, 13, 3812–3829, https://doi.org/10.2166/wcc.2022.161, 2022. a
Stevenazzi, S., Bonfanti, M., Masetti, M., Nghiem, S. V., and Sorichetta, A.: A versatile method for groundwater vulnerability projections in future scenarios, J. Environ. Manage., 187, 365–374, https://doi.org/10.1016/j.jenvman.2016.10.057, 2017. a
Taie Semiromi, M. and Koch, M.: How Do Gaining and Losing Streams React to the Combined Effects of Climate Change and Pumping in the Gharehsoo River Basin, Iran?, Water Resour. Res., 56, e2019WR025388, https://doi.org/10.1029/2019WR025388, 2020. a
Tillman, F. D., Gangopadhyay, S., and Pruitt, T.: Changes in groundwater recharge under projected climate in the upper Colorado River basin, Geophys. Res. Lett., 43, 6968–6974, https://doi.org/10.1002/2016GL069714, 2016. a
Trásy-Havril, T., Szkolnikovics-Simon, S., and Mádl-Szőnyi, J.: How Complex Groundwater Flow Systems Respond to Climate Change Induced Recharge Reduction?, Water, 14, 3026, https://doi.org/10.3390/w14193026, 2022. a
van Verseveld, W. J., Weerts, A. H., Visser, M., Buitink, J., Imhoff, R. O., Boisgontier, H., Bouaziz, L., Eilander, D., Hegnauer, M., ten Velden, C., and Russell, B.: Wflow_sbm v0.7.3, a spatially distributed hydrological model: from global data to local applications, Geosci. Model Dev., 17, 3199–3234, https://doi.org/10.5194/gmd-17-3199-2024, 2024. a, b
van Vuuren, D. P., Edmonds, J., Kainuma, M., Riahi, K., Thomson, A., Hibbard, K., Hurtt, G. C., Kram, T., Krey, V., Lamarque, J.-F., Masui, T., Meinshausen, M., Nakicenovic, N., Smith, S. J., and Rose, S. K.: The representative concentration pathways: an overview, Climatic Change, 109, 1, https://doi.org/10.1007/s10584-011-0148-z, 2011. a
Varouchakis, E. A., Karatzas, G. P., and Giannopoulos, G. P.: Impact of irrigation scenarios and precipitation projections on the groundwater resources of Viannos Basin at the island of Crete, Greece, Environ. Earth Sci., 73, 7359–7374, https://doi.org/10.1007/s12665-014-3913-2, 2015. a
Wada, Y., van Beek, L. P. H., van Kempen, C. M., Reckman, J. W. T. M., Vasak, S., and Bierkens, M. F. P.: Global depletion of groundwater resources, Geophys. Res. Lett., 37, L20402, https://doi.org/10.1029/2010GL044571, 2010. a
Wang, S.-J., Lee, C.-H., Yeh, C.-F., Choo, Y. F., and Tseng, H.-W.: Evaluation of Climate Change Impact on Groundwater Recharge in Groundwater Regions in Taiwan, Water, 13, 1153, https://doi.org/10.3390/w13091153, 2021. a
Wannasin, C., Brauer, C., Uijlenhoet, R., van Verseveld, W., and Weerts, A.: Daily flow simulation in Thailand Part I: Testing a distributed hydrological model with seamless parameter maps based on global data, J. Hydrol. Regional Studies, 34, 100794, https://doi.org/10.1016/j.ejrh.2021.100794, 2021. a
Wu, T., Lin, H., Zhang, H., Ye, F., Wang, Y., Liu, M., Yi, J., and Tian, P.: Effects of Climatic Change on Soil Hydraulic Properties during the Last Interglacial Period: Two Case Studies of the Southern Chinese Loess Plateau, Water, 12, 511, https://doi.org/10.3390/w12020511, 2020a. a
Wu, W.-Y., Lo, M.-H., Wada, Y., Famiglietti, J. S., Reager, J. T., Yeh, P. J.-F., Ducharne, A., and Yang, Z.-L.: Divergent effects of climate change on future groundwater availability in key mid-latitude aquifers, Nat. Commun., 11, 3710, https://doi.org/10.1038/s41467-020-17581-y, 2020b. a
Wu, Y., Miao, C., Fan, X., Gou, J., Zhang, Q., and Zheng, H.: Quantifying the Uncertainty Sources of Future Climate Projections and Narrowing Uncertainties With Bias Correction Techniques, Earth's Future, 10, e2022EF002963, https://doi.org/10.1029/2022EF002963, 2022. a, b
Yamazaki, D., Ikeshima, D., Tawatari, R., Yamaguchi, T., O'Loughlin, F., Neal, J. C., Sampson, C. C., Kanae, S., and Bates, P. D.: A high-accuracy map of global terrain elevations, Geophys. Res. Lett., 44, 5844–5853, https://doi.org/10.1002/2017gl072874, 2017. a
Yawson, D., Adu, M., Mulholland, B., Ball, T., Frimpong, K., Mohan, S., and White, P.: Regional variations in potential groundwater recharge from spring barley crop fields in the UK under projected climate change, Groundwater for Sustainable Development, 8, 332–345, https://doi.org/10.1016/j.gsd.2018.12.005, 2019. a
Yuan, F., Tung, Y.-K., and Ren, L.: Projection of future streamflow changes of the Pearl River basin in China using two delta-change methods, Hydrol. Res., 47, 217–238, https://doi.org/10.2166/nh.2015.159, 2015. a
Yukimoto, S., Koshiro, T., Kawai, H., Oshima, N., Yoshida, K., Urakawa, S., Tsujino, H., Deushi, M., Tanaka, T., Hosaka, M., Yoshimura, H., Shindo, E., Mizuta, R., Ishii, M., Obata, A., and Adachi, Y.: MRI MRI-ESM2.0 model output prepared for CMIP6 CMIP, WDC Climate [data set], https://doi.org/10.22033/ESGF/CMIP6.621, 2019. a, b
Zhang, L. and Wang, J.: Prediction of the soil saturated hydraulic conductivity in a mining area based on CT scanning technology, J. Clean. Prod., 383, 135364, https://doi.org/10.1016/j.jclepro.2022.135364, 2023. a
Zhao, Y., Dong, N., Li, Z., Zhang, W., Yang, M., and Wang, H.: Future precipitation, hydrology and hydropower generation in the Yalong River Basin: Projections and analysis, J. Hydrol., 602, 126738, https://doi.org/10.1016/j.jhydrol.2021.126738, 2021. a