the Creative Commons Attribution 4.0 License.
the Creative Commons Attribution 4.0 License.
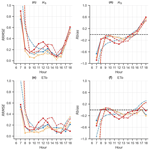
Assessment of upscaling methodologies for daily crop transpiration using sap flows and two-source energy balance models in almonds under different water statuses and production systems
Manuel Quintanilla-Albornoz
Xavier Miarnau
Ana Pelechá
Héctor Nieto
Joaquim Bellvert
Daily transpiration (Td) is crucial for both irrigation water management and increasing crop water productivity. The use of the remote-sensing-based two-source energy balance model (TSEB) has proven to be robust in estimating plant transpiration and evaporation separately for various crops. However, remote sensing models provide instantaneous estimations, and so daily upscaling approaches are needed to estimate daily fluxes. Daily upscaling methodologies have not yet been examined to upscale solely transpiration in woody crops. In this regard, this study aims to evaluate the proper image acquisition time throughout the day and four methodologies used to retrieve Td in almond trees with different production systems and water statuses. Hourly transpiration (Th) was estimated using the TSEB contextual approach (Th–TSEB) with high-resolution imagery five times during two diurnal courses. The tested methodologies were the following: the simulated evaporative fraction variable (EFsim), irradiance (Rs), reference evapotranspiration (ETo), and potential evapotranspiration (ETp). These approaches were first evaluated with in situ sap flow (T–SF) data and were then applied to the Th–TSEB. Daily T–SF showed significant differences among production systems and levels of water stress. The EFsim and ETp methods correlated better with measured T–SF and reduced the underestimation observed using the Rs and ETo methods, especially at noon in the severely water-stressed trees. However, the daily upscaling approaches applied in the TSEB (Td–TSEB) failed to detect differences between production systems. The lack of sensibility of Th–TSEB among production systems poses a challenge when estimating Td in canopies with discontinuous architectural structures. The use of ETp as a reference variable could address this issue as it incorporates various aerodynamic and radiative properties associated with different canopy architectures that influence the daily Th–SF pattern. However, more accurate ETp estimates or more advanced ETp models are needed.
- Article
(10317 KB) - Full-text XML
- BibTeX
- EndNote
Almond is one of the high-value crops with the greatest water usage (Goldhamer and Fereres, 2017; López-López et al., 2018). In Spain, a paradigm change is taking place with the introduction of new intensified almond production systems with more planar designs (Iglesias and Echeverria, 2022), which may complicate the accurate estimation of evapotranspiration (ET) using remote sensing models. Thus, since the expansion of almond production is occurring in a context of increasing water scarcity, many studies have focused on quantifying its water usage in different environments and under different water regimes. From a water management point of view, there is particular interest in validating the daily ET and its components, plant transpiration (Tp) and evaporation (E), in this crop and under different production systems and water statuses. This is relevant because almond is considered to be a drought-tolerant species able to control water loss through stomatal closure, which has been identified as a common and early event in plant responses to water deficits (Castel and Fereres, 1982; Escalona et al., 1999, Chaves et al., 2002). Romero and Botía (2006) also showed that the influence of the evaporative demand of the atmosphere on stomatal behavior was higher in well-watered almonds compared to in water-stressed almonds. The same study also demonstrated that water-stressed almonds restricted stomatal activity earlier in the morning when the atmospheric vapor pressure deficit (VPD) was still low. As a result, maximum Tp values occurred during this period and were significantly higher than those observed in well-watered almonds.
Accurate in-field quantification of crop ET and the partitioning components E from soil and Tp from vegetation is very useful for both irrigation water management and increasing crop water productivity (Zhang et al., 2021). Consequently, several methodologies have been developed to address this objective (Evett and Tolk, 2009). Of these, remote sensing thermal-based surface energy balance models have shown their utility in retrieving ET in a wide range of environments and ecosystems (Shuttleworth and Wallace, 1985; Bastiaanssen et al., 1998; Drexler et al., 2004; Overgaard et al., 2006; Allen et al., 2007; Timmermans et al., 2007; Kalma et al., 2008; Kustas and Anderson, 2009). The advantage of using remote sensing lies in the possibility of monitoring heterogeneous surfaces over a wide range of spatial resolutions and thereby generating operational ET products (Kalma et al., 2008). One such model that calculates Tp and E explicitly is the two-source energy balance (TSEB), which was initially developed by Norman et al. (1995) and Kustas and Norman (1999). The separate Tp and E outputs provide the advantage of simultaneously evaluating canopy stress and directly quantifying plant water consumption. This information can be valuable for enhancing water use efficiency in agricultural and environmental management. Moreover, Tp is also linked to plant productivity as the exchange of both water and carbon between the atmosphere and the plant is conveyed via the leaf stoma. The TSEB approach has demonstrated its robustness in accurately estimating plant ET across diverse surface conditions and a wide range of landscapes (Kustas and Anderson, 2009; Kustas et al., 2019; Gómez-Candón et al., 2021; Gao et al., 2023; Knipper et al., 2023). To estimate Tp, the use of very-high-resolution thermal and multispectral imagery allows for the direct estimation of canopy and soil temperatures, facilitating the retrieval of ET partitioning through the use of, for example, the TSEB contextual approach (TSEB-2T) model (Nieto et al., 2019; Nassar et al., 2020; Gao et al., 2023; Quintanilla-Albornoz et al., 2023).
Models for estimating ET fluxes based on remote sensing, however, can only be used to derive an instantaneous ET at the time of clear-sky satellite or aircraft overpass. Thus, the selection of a proper overpass time and the development of upscaling algorithms to extrapolate ET from instantaneous to daily scale are of special interest for the management of crop water consumption. Current thermal-infrared (TIR) polar-orbiting satellites, such as Landsat, Sentinel-3, or the moderate-resolution imaging spectroradiometer (MODIS) on board Terra, have an overpass time close to 10:00 UTC (mean locator solar time). However, several studies suggest that the best accuracies in ET retrievals would be captured better in the early afternoon (Delogu et al., 2012; Anderson et al., 2021). Bellvert et al. (2014) also showed that early afternoon was the most appropriate moment to detect maximum differences in canopy temperature between well-watered and water-stressed crops. For this reason, in coming years, new TIR satellite missions including TRISHNA (Thermal infraRed Imaging Satellite for High Resolution Natural resource Assessment) (Lagouarde et al., 2018), SBG (Surface Biology and Geology) (Basilio et al., 2022), or LSTM (Land Surface Temperature Monitoring) (Koetz et al., 2018) are planned at an overpass time around 13:00 UTC (GMT time).
Daily upscaling of ET fluxes is commonly performed by assuming a constant relationship over the course of the day between instantaneous ET and a reference meteorological forcing that can be computed at hourly and daily time steps (Crago and Brutsaert, 1996; Van Niel et al., 2011; Cammalleri et al., 2014). This hypothesis is generally known as self-preservation (Crago and Brutsaert, 1996). Generally, the most commonly used methods for upscaling ET are the evaporative fraction (EF) method, the solar radiation (Rs) method, the stress factor method, and the canopy resistance method (Hoedjes et al., 2008; Delogu et al., 2012; Cammalleri et al., 2014; Jiang et al., 2021; Nassar et al., 2021). The EF method assumes that the ratio of latent heat flux (LE) to surface available energy (AE) remains relatively stable throughout the day under clear-sky conditions. However, studies have shown that the EF method can lead to systematic underestimation for estimating daily evapotranspiration fluxes under wet conditions, as the ratio between LE and AE tends to be stable around midday but significantly higher during the early morning and late afternoon (Shuttleworth et al., 1989; Brutsaert, 1992; Crago and Brutsaert, 1996; Lhomme and Elguero, 1999; Gentine et al., 2007). To address this challenge, Hoedjes et al. (2008) introduced a parameterization of the diurnal EF pattern based on the primary atmospheric forcing parameters: Rs and relative humidity (RH). Implementing this approach, known as EFsim, Delogu et al. (2012) successfully reduced the overestimation associated with the EF method from 15.8 % to 6.5 %.
Additionally, while estimating the instantaneous AE at a specific time can be relatively straightforward using thermal imagery and meteorological data, determining daily AE needs daily course measurements or estimates of net radiation (Rn) and soil heat flux (G), which can be challenging. Given that the diurnal pattern of AE is primarily influenced by Rs, it has become a common practice to use Rs as a reference variable for the estimation of daily ET fluxes from instantaneous measurements (Jackson et al., 1983; Zhang and Lemeur, 1995). The use of Rs in the context of remote sensing applications has fewer requirements than the EF method, with the latter needing auxiliary information, such as Rn, that can be complex to measure and may further limit operational utility. When using the Rs upscaling method, both Cammalleri et al. (2014) and Nassar et al. (2021) improved daily ET compared to when using EF methods.
Another upscaling method that has been proposed is the stress factor method. This approach employs the reference evapotranspiration (ETo) or potential evapotranspiration (ETp) as a reference variable, which inherently accounts for the key meteorological factors influencing the evaporative process (Trezza, 2002; Delogu et al., 2012). Trezza (2002) found a constant ratio between ET and ETo during the daytime and employed it to estimate daily ET using remote sensing estimations, achieving better results compared to EF upscaling methods. However, Cammalleri et al. (2014) obtained similar results when using both the EF method and the ETo to estimate daily ET in sites without stress conditions. For their part, Delogu et al. (2012) evaluated the use of ETp as a reference variable and obtained worse results compared to the EF method for a dataset with stress events. This was attributed to the fact that the AE followed both stressed and unstressed ET patterns, whereas ETp often increased independently of the water stress levels.
Nevertheless, the aforementioned upscaling methods reported in the literature for agricultural ecosystems have only been validated against daily ET, usually over sites with eddy covariance flux towers, with a footprint with mixed information on the spatial variability (Cammalleri et al., 2014; Xu et al., 2018; Jiang et al., 2021). Therefore, to the best of our knowledge, the use of upscaling methodologies to estimate daily Tp (Td) based on instantaneous Tp values has not been previously examined. Furthermore, the diurnal pattern of Tp has a different response between well-watered and water-stressed crops (Poni et al., 2009; Tuzet et al., 2003), and this different response would also depend on the stomatal control of each species in relation to soil water and vapor pressure deficits. Thus, the hypothesis of this study is that upscaling methods may have different responses for water-stressed and well-watered almond trees (Sánchez et al., 2021; Jofre-Čekalović et al., 2022; Iglesias and Echeverria, 2022; Peddinti and Kisekka, 2022; Knipper et al., 2023). Therefore, the main purpose of this study is to evaluate different Td upscaling methodologies in almond trees under different production systems and water regimes using sap flow measurements. This study aims to contribute to our understanding and to establish a reference for upscaling remote sensing canopy Tp in woody crops, which is crucial in mapping daily ET partitioning from field to global scales.
2.1 Trial location and design
This study was conducted in an almond orchard situated at the experimental station of the IRTA (Institute of Agrifood Research and Technology) in Les Borges Blanques, Spain (41°30′31.89′′ N, 0°51′10.70′′ E; 323 m elevation) (Fig. 1a). The almond orchard was planted in June 2009, with “Marinada” used as the scion cultivar onto an INRA GF-677 rootstock. Additionally, the orchard was planted with different planting distances and was subjected to different pruning techniques. The combination of planting distance and pruning techniques will be referred to as the “production system”. Three almond production systems were evaluated: open vase with minimal pruning (MP) spaced at 5.5 × 3.5 m, central axis spaced at 5 × 3 m, and hedgerow spaced at 4.5 × 3 m (Fig. 1b and c). The orchard was situated on a clay-loam-textured soil, with a depth ranging from 1.6 to 2 m. The study site has a Mediterranean climate, with an average annual rainfall of 364 mm and an average annual evapotranspiration of 1088 mm. Two different dates were selected to assess the diurnal course of Tp: 29 June and 19 August 2022. Figure 2 displayed the meteorological conditions during the campaign.
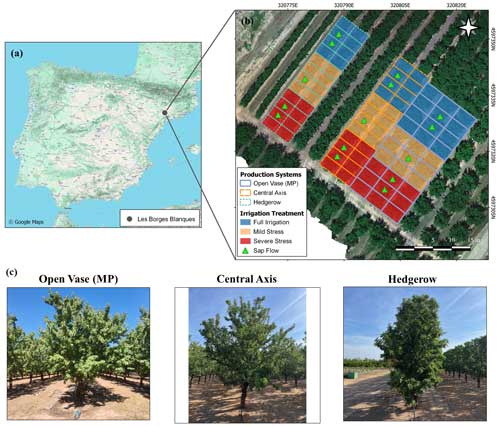
Figure 1(a) Location of the almond orchard in Les Borges Blanques; (b) experimental design of the orchard, showing in different colors the three production systems and the three irrigation treatments; and (c) photographs from summer 2022 of production systems.
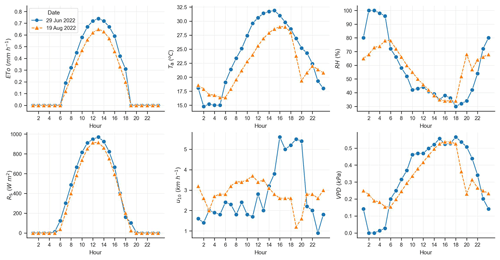
Figure 2Meteorological conditions at an hourly scale during the flight campaign: reference evapotranspiration (ETo), air temperature (Ta), relative humidity (RH), solar radiation (Rs), wind speed at 10 m (u10), and vapor pressure deficit (VPD). The x axis represents the time in UTC+0, with solar noon occurring around 12:00 UTC at the experimental site.
The orchard was irrigated using a drip irrigation system. In the open-vase (MP) system, two lateral pipes were positioned on each side of the tree at 40 cm, with a dripper placed every 70 cm and a water discharge rate of 2.2 L h−1. The central axis and hedgerow systems had a lateral pipe along the row line, with drippers placed at 60 cm intervals with a water discharge rate of 3.8 L h−1 per dripper. Daily irrigation was scheduled on a weekly basis to complement potential crop evapotranspiration (ETc) using ETc = (ETo × Kc) − effective rainfall, as described by Allen et al. (1998). ETo was obtained from a meteorological station within Catalonia's official network of meteorological stations (SMC, https://ruralcat.gencat.cat/web/guest/agrometeo, last access: 20 December 2023), situated 500 m away from the study site. The ETo is estimated using the FAO-56 Penman–Monteith method (Allen et al., 1998). Kc refers to the crop coefficient. The Kc was assigned based on different phenological stages following Goldhamer and Girona (2012). The assigned Kc values were as follows: Kc1= 0.70 (April), Kc2= 0.95 (May), Kc3= 1.09 (June), Kc4= 1.15 (July), Kc5= 1.17 (August), and Kc6= 1.12 (September). Effective rainfall was determined following the method outlined by Olivo et al. (2009), which considers half of the precipitation for days with a single event exceeding 10 mm; otherwise, it is considered to be zero. Three irrigation treatments were implemented for each production system during the 2021 and 2022 growing seasons: (i) full irrigation, where irrigation matches ET requirements (100 % ETc); (ii) mild stress, irrigated at 50 % ETc; and (iii) severe stress, irrigated at 20 % ETc. The water applied was quantified using digital water meters (CZ2000-3M, Contazara, Zaragoza, Spain).
2.2 Sap flow measurement
Sap flow sensors offer substantial advantages, enabling the continuous and automated measurement of sap movements for each plant with a high temporal resolution (Smith and Allen, 1996; Forster, 2017; Fernandez et al., 2001). When properly calibrated, these sensors can measure the Tp for the entire plant (López-Bernal et al., 2010; Forster, 2017; Noun et al., 2022). Among the sap-flow-measuring methods available, the compensation heat pulse (CHP) has been suggested as a tool for detecting water stress and for irrigation-scheduling purposes (Fernandez, 2001; Alarcón et al., 2005). Therefore, the CHP sap flow method combined with the calibrated average gradient technique was employed to estimate the Tp. The sap flow system consists of a 2 mm diameter 4.8 W stainless-steel heater and two temperature sensors positioned 10 and 5 mm downstream and upstream of the heater, respectively. Each temperature sensor is embedded with two E-type thermocouples (chrome-constantan wire) spaced 10 mm apart along the needle. The heat pulse velocity at 5 and 15 mm below the cambium is used to calculate the sap flow density across the trunk radius. The sap flow system was developed by the IAS-CSIC laboratory. For further specifications, refer to Villalobos et al. (2009). Sap flow data were collected every 15 min and stored in a CR1000 data logger (Campbell Scientific Inc., Logan, UT, USA).
Sap flow sensors were installed in each production system, monitoring two trees from the full-irrigation and severe-stress treatments and one tree from the mild-stress treatments, as shown in Fig. 1b. They were installed at 0.5 m above the ground. Each sap flow transpiration (T–SF) underwent correction for wound and azimuthal effects (López-Bernal et al., 2010) using actual Tp measured by a water balance method (Twb) on 13 July 2022. The Twb was calculated using Eq. (1):
where P is precipitation, IR is the amount of water applied through irrigation, ΔSWC is the difference in soil water content (SWC) between 2 consecutive days, DP is deep percolation, and E corresponds to evaporation. P, DP, and IR were considered to be zero because the water balance was calculated for days without P and IR applied. Additionally, the soil was covered with plastic sheeting during these days to prevent E fluxes (E ≈ 0). Differences between Twb and T–SF measurements were assumed to remain constant throughout the season, as demonstrated by Espadafor et al. (2015). The calibrated T–SF was used to calculate both the accumulated hourly Tp (Th–SF) and the accumulated daily Tp (Td–SF).
The SWC was measured using a neutron probe at intervals of 20 cm down to a depth of 180 cm (Campbell Pacific Nuclear Scientific, Model 503). The tubes used for SWC measurements were installed to cover one-quarter of the planting area. In each tree, two groups of three tubes were installed in parallel, positioned below the emitter, at a quarter of the inter-row distance and at half of the inter-row distance. Soil samples were taken at the time of tube installation to estimate the volumetric moisture content (cm3 of water cm−3 of soil). This measurement was then used to calibrate the neutron probe readings.
2.3 Field measurement
2.3.1 Stem water potential, stomatal conductance, leaf transpiration, and leaf area index
Stem water potential (Ψs), stomatal conductance (gs), and leaf transpiration (Eleaf) were measured at 07:00, 09:00, 12:00, 14:00, and 16:00 UTC solar time during the UAV flight campaign and in the same trees where sap flow sensors were installed. The measurement of Ψs followed the protocol outlined by McCutchan and Shackel (1992). The Ψs was determined by measuring three shaded leaves from each tree. Prior to measurement, each leaf was enclosed in a plastic bar covered with aluminum foil for 1 h to equalize the water potential between the leaf, stem, and branches. A pressure chamber (Plant Water Status Console, Model 3500; SoilMoisture Equipment Corp., Santa Barbara, CA) was utilized to obtain the Ψs in all measurements within 1 h. The gs and Eleaf were measured using the LI-600 porometer/fluorometer (LI-COR Inc., Lincoln, NE, USA). Three sunny leaves were measured in each tree concomitant to image acquisition. The leaf area index (LAI) was determined for trees equipped with sap flow sensors using the LAI-2200 Plant Canopy Analyzer (PCA) (LI-COR Inc., Lincoln, NE, USA). The LAI was measured for each flight date around midday. The LAI measurement procedure involved one measurement being taken above the tree and four being taken below the tree. The incident radiation above the tree was recorded in an open area using five sensor rings. A single measurement was taken in each cardinal direction (N, S, E, and W) beneath the tree. The LAI was subsequently estimated from the vertical profile of the crown using the FV2200 v.2.1.1 software. The accuracy of LAI estimations was 0.57 m2 m−2 (Quintanilla-Albornoz et al., 2023).
2.3.2 Image acquisition campaign
A total of 10 flights were conducted on 29 June and 29 August 2022 with UAV DRONEHEXA XL (DroneTools, Seville, Spain). On each day, five flights were conducted at 07:00, 09:00, 12:00, 14:00, and 16:00 UTC (GMT). The UAV was outfitted with a MicaSense RedEdge-MX multispectral camera (MicaSense, Northlake Way, Seattle, USA) and a FLIR SC655 thermal camera (FLIR Systems, Wilsonville, OR, United States). MicaSense RedEdge-MX captures images in five spectral bands at wavelengths of 475 ± 20, 560 ± 20, 668 ± 10, 717 ± 10, and 840 ± 40 nm. FLIR SC655 has a spectral response in the range of 7.5–13 µm. The flights were carried out at a height of 50 m above ground level to capture multispectral and thermal images with spatial resolutions of 0.03 and 0.06 m, respectively.
All images were subjected to radiometric, atmospheric, and geometric correction. The FieldSpec 4 Standard-Res Spectroradiometer (Malvern Panalytical, Inc., United Kingdom) was used to acquire in situ spectral measurements of various ground targets simultaneously with the image acquisition for radiometric calibration. The FieldSpec 4 Standard-Res Spectroradiometer has an optical resolution of 3–10 mm and a wavelength response between 350 and 2500 nm. Before conducting spectral measurements on the ground targets, the spectroradiometer was calibrated using a white reference panel (white-color Spectralon) and a dark reference. The thermal sensor underwent radiometric calibration in the laboratory using a blackbody (model P80P, Land Instruments, Dronfield, United Kingdom). Additionally, in situ temperature measurements were acquired using an SI-111-SS Apogee infrared radiometer connected to an Apogee AT-100 microCache Bluetooth micrologger (Apogee Instruments Inc., Logan, UT, USA). The mosaicking process and the generation of the digital elevation model (DEM) and the digital surface model (DSM) were performed using Agisoft Metashape Professional software (Agisoft LLC., St. Petersburg, Russia). Geometric and radiometric corrections was conducted using QGIS 3.4 (QGIS 3.4.15).
2.4 TSEB model description
The TSEB scheme, initially introduced by Norman et al. (1995) and further refined by Kustas and Anderson (2009), was utilized to estimate Tp employing high-resolution images. The TSEB is an energy balance model that assumes that net surface radiation (Rn) is primarily distributed among sensible heat flux (H), latent heat flux (LE), and soil heat flux (G). Consequently, the LE (W m−2) is calculated as the residual of the surface energy equation using Eqs. (2a), (2b), and (2c):
where the subscripts c and s refer to the energy fluxes of the canopy and soil, respectively. The Campbell and Norman (1998) canopy transfer model, considering a rectangular clumping index, was employed to estimate Rn,s and Rn,c, as described by Parry et al. (2019) and Quintanilla-Albornoz et al. (2023). G was assumed to be a constant fraction of Rn,s of around 0.35. A series resistance scheme was utilized, dividing H into soil (Hs) and canopy (Hc), as shown in Eqs. (3a), (3b), and (3c):
where ρ is the air density; Cp is the specific heat of the air; Ts is the soil temperature; Tc is the canopy temperature; Ta is the air temperature; Tac is the temperature in the canopy air space, equivalent to the aerodynamic temperature; rs is the resistance to heat flow in the boundary layer immediately above the soil surface; rx is the total boundary layer resistance of the complete canopy leaves; and ra is the aerodynamic resistance to turbulent heat transport between the air canopy layer and the overlying air layer. The resistances were derived according to Kustas and Norman (1999) and Norman et al. (1995).
The contextual approach of the TSEB model (TSEB–2T) was evaluated in this study and is available online at https://doi.org/10.5281/zenodo.8134956 (Nieto et al., 2023). The TSEB–2T was applied with direct measurements of Tc and Ts from high-resolution thermal images. Tc and Ts were obtained with a supervised image classification based on use of the DSM and the soil-adjusted vegetation index (SAVI). SAVI was chosen due to its ability to reduce the impact of ground brightness in the near- and shortwave-infrared wavelengths, which enhances the contrast between vegetation and the ground surface (Qi et al., 1994). Pixels were classified as canopy if they exhibited a DSM greater than 1.5 m and a SAVI greater than 0.2. Pixels that did not meet these conditions were classified as pure soil. These layers were employed to retrieve the Tc and Ts from thermal images. Finally, the hourly Tp in millimeters (Th-TSEB) was estimated using 1000 × 3600 × LEc (ρwλ), where ρw is the density of water (assumed to be 1000 kg m−3), and λ is the latent heat of vaporization (J kg−1): λ= 1×106 × (2.501 – 0.002361 Ta). All biophysical traits required for TSEB models, namely the fractional canopy cover (fc), canopy height (hc), and canopy width (wc), were obtained using the multispectral and DSM high-resolution images. For additional details on the biophysical traits' procedures, refer to Quintanilla-Albornoz et al. (2023).
2.5 Models evaluated to upscale daily transpiration
The self-conservation method is the most commonly used approach to upscale ET fluxes from instantaneous measurements. This assumes a constant relationship between the instantaneous ET and some meteorological variables over time under certain conditions. According to Cammalleri et al. (2014), the relationship between instantaneous measurement of ET fluxes and a reference variable can be illustrated using Eq. (4):
where λLEt is the instantaneous latent heat flux at the acquisition time t, Xt and Xd are the values of the reference variable at the acquisition time t and during the day d, and β represents a correction factor to account for potential biases or nighttime ET. This paper evaluates four self-preservation approaches, elucidated below, along with their implications for estimating Td in almond crops.
2.5.1 Simulated evaporative fraction variable (EFsim) method
The EFsim is based on the evaporative fraction (EF) method. The EF method assumes that the ratio between LE and AE is relatively constant during the day. Following Eqs. (5a), (5b), and (5c), we can obtain the daily LE fluxes:
where LEd and AEd correspond to daily accumulated LE and AE, respectively. Rn can be determined from remote sensing data using Eq. (6):
where α corresponds to the albedo, ε corresponds to the surface emissivity, Ratm corresponds to the atmospheric longwave radiation, σ corresponds to the Stefan–Boltzmann constant, and Trad corresponds to the radiometric temperature. To avoid daily measurement of Rn and G, the AE can be extrapolated from instantaneous AE estimated through thermal imagery and Rs following the methods proposed by Jackson et al. (1983) and Delogu et al. (2012), as expressed in Eq. (7):
where AEt represents the instantaneous AE estimated through thermal imagery, Rs,d represents the daily Rs, and Rs,t is the Rs at the measurement time. According to Hoedjes et al. (2008), the daily pattern of EF can be simulated as a function of Rs and RH, as in Eq. (8a). However, EFsim is a theoretical curve and must be adjusted using real EF values with Eq. (8b):
where Rs is in W m−2, and RH is in percentage. Additionally, EFt,obs represents actual EF values estimated using remote sensing imagery based on Eq. (5a), and EFt,sim is the EFsim at the time of EFt,obs. Finally, the EFsim method employs Eq. (5c) with an EF estimated using Eq. (8b) and an AEd estimated using Eq. (7) to estimate LEd.
2.5.2 Incoming shortwave solar radiation (Rs) approach
An alternative strategy consists of replacing AE as a reference variable with the Rs. This method is founded on the principle that Rs is the primary radiation flux during the day, resulting in a strong correlation and associated variations between actual ET and Rs (Jackson et al., 1983; Delogu et al., 2012; Nassar et al., 2021). Thus, LEd can be estimated with Eq. (9):
where Rs,d corresponds to daily Rs, and Rs,t is the Rs at the time that LE was estimated.
2.5.3 Stress factor approach
The stress factor approach involves upscaling the instantaneous ET using either the reference (ETo) or potential evapotranspiration (ETp), as depicted in Eq. (10):
The stress factor is defined as the ratio between ET and instantaneous ETo or ETp (SF = ET ETo (or ETp)). The ETo was obtained using the FAO-56 method (Allen et al., 1998). ETp was estimated using the Penman–Monteith one-source energy balance model and by forcing it with meteorological data and the actual LAI (Allen et al., 1998). The ETp obtained from the Penman–Monteith model is available in the Python programming language at https://doi.org/10.5281/zenodo.8134956 (Nieto et al., 2023).
The minimum bulk canopy resistance for the ETp model was determined through a method that parameterizes the relationship between gs and VPD, as described by Kustas et al. (2022). Meteorological data were obtained from the weather station of the Meteorological Service of Catalonia located near the experimental orchard.
The EFsim, Rs, ETo, and ETp upscaling methods were used to estimate Td from Th–SF measurements and from Th–TSEB estimations. The Td obtained using the EFsim, Rs, ETo, and ETp upscaling methods from Th–SF measurements was called Td–SF–ETsim, Td–SF–Rs, Td–SF–ETo, and Td–SF–ETp, while the Td estimated from Th-TSEB estimations was called Td–TSEB–ETsim, Td–TSEB–Rs, Td–TSEB–ETo, and Td–TSEB–ETp, respectively.
3.1 Biophysical traits and physiological measurements
Table 1 shows an analysis of variance (ANOVA) of the main biophysical traits, and Table 2 shows the average of each biophysical variable for each production system and irrigation treatment. The fractional canopy cover (fc) significantly varied between production systems, with open vase (MP) and hedgerows presenting the highest and lowest values, respectively. The average fc for each production system was 0.56, 0.50, and 0.47 for open vase (MP), central axis, and hedgerow, respectively. Canopy height (hc) varied significantly between production systems, irrigation treatments, and their interaction. Overall, taller trees were observed in the open-vase (MP) system. However, the open-vase (MP) and hedgerow systems led to smaller trees in the severe-stress treatment, whereas the central axis had the smallest trees in the mild-stress treatment. The measured LAI did not show significant differences among production systems or irrigation treatments.
Table 1Analysis of variance (three-way ANOVA) testing the effect of date, production system (PS), irrigation treatment (TRT), and their interactions on fractional canopy cover (fc), canopy height (hc), leaf area index (LAI), stem water potential (Ψs), and hourly (Th–SF) and daily transpiration (Td–SF) measured by sap flow sensors. The p values less than 0.05 were considered to be statistically significant, while “ns” represents a statistically non-significant interaction.
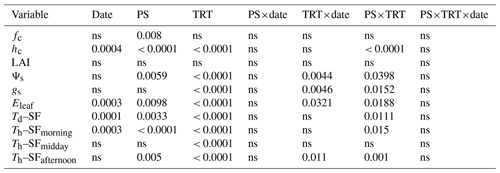
Table 2Comparison of fractional canopy cover (fc), canopy height (hc), leaf area index (LAI), and daily transpiration (Td–SF) measured during the flight campaign. Different letters mean significant differences at p < 0.05 using Tukey's honest significant difference test considering the interaction between production system and irrigation treatment.
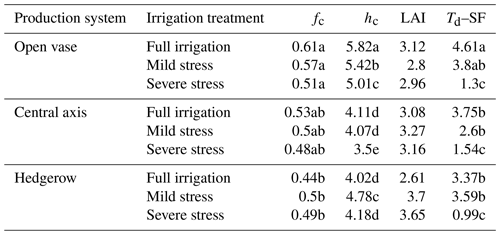
3.1.1 Stem water potential, stomatal conductance, and leaf transpiration
The diurnal patterns of Ψs, gs, and Eleaf exhibited variations primarily attributed to the irrigation treatment (Fig. 3). These variations led to significant differences in tree daily average Ψs, gs, and Eleaf among the different irrigation treatments (Table 1). Moreover, the interaction between production system and irrigation treatment (PS×TRT) had a significant impact, primarily attributable to the central axis subjected to the mild-stress treatment. The central axis under the mild-stress treatment exhibited values comparable to those observed in the severe-stress treatment. The daily pattern of Ψs exhibited significant differences between irrigation treatments as early as 07:00 UTC. In contrast, discernible significant differences between irrigation treatments for gs and Eleaf were evident as early as 09:00 UTC. Differences in Ψs, gs, and Eleaf between irrigation treatments remained evident until 16:00 UTC. The peak disparities in Ψs, gs, and Eleaf among irrigation treatments were observed around 12:00 UTC. During this time, Ψs had its most reduced values, with an average of −1.35 MPa in the full-irrigation treatment, −1.86 MPa in the mild-stress treatment, and −2.30 MPa in the severe-stress treatment. Simultaneously, gs attained its maximum values with an average of 0.41, 0.25, and 0.12 mol m−2 s−1 for the full-irrigation, mild-stress, and severe-stress treatments, respectively. The most pronounced variations in Eleaf among irrigation treatments occurred at 12:00 UTC, and the highest Eleaf values were recorded at 14:00 UTC, with, respectively, averaged values of 10.61, 6.96, and 5.24 mmol m−2 s−1 for the full-irrigation, mild-stress, and severe-stress treatments. Finally, on average, the tree daily mean Ψs for the fully irrigated treatment was −1.18 MPa, while the mild-stress and severe-stress treatments showed values of −1.65 and −1.99 MPa, respectively. Similarly, the tree daily averaged values of gs were 0.32, 0.21, and 0.13 mol m−2 s−1 for the full-irrigation, mild-stress, and severe-stress treatments, respectively. Additionally, the tree daily Eleaf values were 7.74, 5.77, and 4.12 mol m−2 s−1 for the full-irrigation, mild-stress, and severe-stress treatments, respectively.
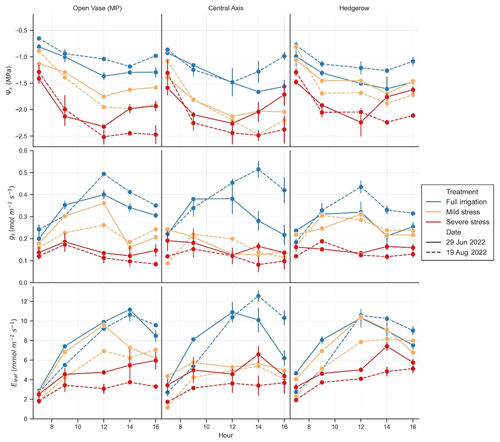
Figure 3Daily course of stem water potential (Ψs), stomatal conductance (gs), and leaf transpiration (Eleaf) for 29 June and 19 August 2022 in almond trees with three different production systems (open vase (MP), central axis, and hedgerow) and irrigation treatments (full irrigation, mild stress, and severe stress). The x axis represents the time in UTC+0, with solar noon occurring around 12:00 UTC at the experimental site.
3.1.2 Sap flow transpiration
The Td–SF showed significant differences among production systems, irrigation treatments, PS×TRT, and dates (Table 1). The open-vase (MP) system transpired significantly higher, with an average of 3.13 mm d−1 compared to 2.64 mm d−1 for the central-axis system and 2.46 mm d−1 for the hedgerow system. Notably, in hedgerow, the mild-stress treatment showed higher Td–SF values compared to the full-irrigation treatment, although the difference was not statistically significant (Table 2).
Figure 4 shows the daily patterns of Th–SF. The Th–SF patterns exhibited variations based on production system, irrigation treatment, and date. The daily pattern may vary between days due to differences in the main weather forcing parameters (see Fig. 2), as well as an irrigation scheduling error that occurred on 29 June at 12:00 UTC, coinciding with the ongoing measurements. The error in the irrigation schedule resulted in significant pattern variations, particularly in the severe-stress treatment. In this treatment, Th–SF exhibited a notable increase at 13:00 UTC, reaching its peak at 14:00 and 15:00 UTC on 29 June in all production systems. The maximum Th–SF rates recorded in the severe-stress treatment on 29 June were 0.14, 0.20, and 0.23 mm h−1 for the open-vase (MP), central-axis, and hedgerow systems, respectively. Conversely, the maximum Th–SF rates in the severe-stress treatment on 29 August were observed between 10:00 and 12:00 UTC, with 0.10, 0.12, and 0.07 mm h−1 for the open-vase (MP), central-axis, and hedgerow systems, respectively.
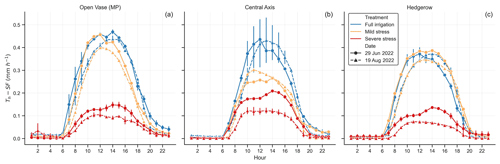
Figure 4Daily course of hourly sap flow transpiration (Th–SF) for different irrigation treatments in the (a) open-vase (MP), (b) central-axis, and (c) hedgerow production systems for the dates of 29 June and 19 August 2022. The x axis represents the time in UTC+0, with solar noon occurring around 12:00 UTC at the experimental site.
In the full-irrigation treatment, the maximum Th–SF rates varied depending on the day and the production system, occurring between 12:00 and 14:00 UTC. In the open-vase (MP) system, the highest Th–SF values, averaging 0.45 mm h−1, were recorded at 14:00 UTC. In the central-axis system under full irrigation, the maximum Th–SF occurred at 12:00 UTC on 29 June and at 14:00 UTC on 19 August, with a Th–SF rate of 0.43 mm h−1 for both dates. In the hedgerow system, the full-irrigation treatment yielded a maximum Th–SF of 0.37 mm h−1 on both days, observed at 12:00 UTC on 29 June and at 14:00 UTC on 19 August.
Similarly to the full-irrigation treatment, in the mild-stress treatment, the timing of maximum Th–SF depended on the day and the production system. In the mild-stress treatment for the open vase (MP), the maximum Th–SF was recorded at 12:00 UTC, corresponding to 0.45 mm h−1 on 29 June and to 0.39 mm h−1 on 19 August. In contrast, the mild-stress treatment for the central-axis system reached its peak at 14:00 UTC on 19 June and at 12:00 UTC on 19 August, with Th–SF rates of 0.26 and 0.30 mm h−1, respectively. In the hedgerow system, under the mild-stress treatment, the maximum Th–SF rates of approximately 0.38 and 0.34 mm h−1 were observed at 14:00 UTC on 29 June and at 12:00 UTC on 19 August, respectively.
The Th–SF exhibited significant differences between 06:00 and 21:00 UTC, attributed to the irrigation treatments. Th–SF for the severe-stress treatment was systematically lower than that for the other two treatments. These differences were more evident during daytime hours. Thus, the maximum differences between the full-irrigation and severe-stress treatments were observed at 12:00 UTC, reflecting an averaged difference of 0.28 mm h−1. Furthermore, nocturnal fluxes, which accounted for approximately 5 % of the total Td–SF, were observed, with the exception of one tree in the open-vase (MP) system and one tree in the hedgerow system (both under the severe-stress treatment), where nocturnal Th–SF contributed to 21.3 % and 10.6 % of the total Td–SF, respectively.
The statistical analysis showed that Th–SF during the morning (6:00 to 10:00 UTC) and afternoon (14:00 to 18:00 UTC) showed significant differences among production systems and PS×TRT (Table 1). During those daytime intervals, the open-vase (MP) production system demonstrated significantly higher Tp compared to the other production systems. The significance of PS × TRT is explained by the fact that the hedgerow, under the mild-stress treatment, exhibited higher Th–SF values than the full-irrigation treatment in both time periods. Notably, although there was no statistical difference between production systems at midday (11:00 to 13:00 UTC), the irrigation treatment was significant for the mean Td–SF (Table 1).
Figure 5 illustrates the relationship between the Th–SF measured during the days of the flight campaign and the key parameters utilized in the estimation of Td (Rs, ETo, and ETp) for all irrigation treatments. Th–SF was strongly correlated with Rs, ETo, and ETp for all irrigation treatments. Overall, the relationship between Th–SF and ETo had the highest Pearson correlation coefficient (r), with values of 0.95, 0.95, and 0.90 for the full-irrigation, mild-stress, and severe-stress treatments, respectively. Similarly, the correlation with Rs yielded r values of 0.94, 0.94, and 0.87, while ETp showed r values of 0.94, 0.94, and 0.85, respectively, for the full-irrigation, mild-stress, and severe-stress treatments. The ETp model exhibited a root mean squared error (RMSE) of 0.22 mm h−1 compared to Th–SF for the full-irrigation treatment. Additionally, the RMSE of the ETp model showed significant variation between production systems, with an error of 0.18 mm h−1 for the open-vase (MP) system, 0.19 mm h−1 for the central-axis system, and 0.27 mm h−1 for the hedgerow system.
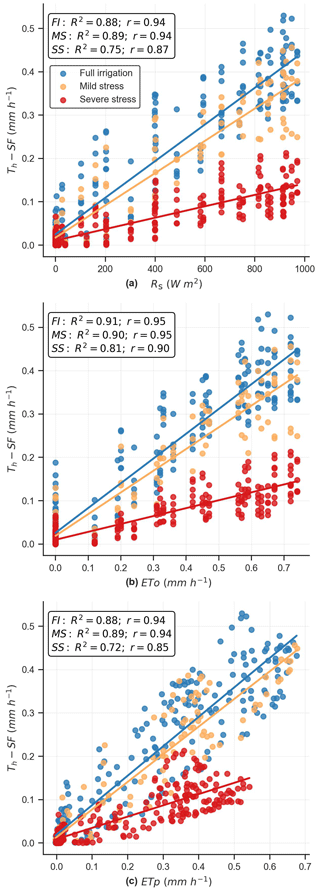
Figure 5Regression between hourly sap flow transpiration (Th–SF) with (a) solar irradiance (Rs), (b) reference evapotranspiration (ETo), and (c) potential evapotranspiration (ETp), separated by irrigation treatment. The box displays the statistical values for the determination coefficient (R2) and Pearson's correlation coefficient (r) across the full-irrigation, mild-stress, and severe-stress treatments.
The differences between the hourly and daily ratios of Rs (%), ETo (%ETo), and ETp (%ETp) and T–SF are shown in Fig. 6. The diurnal pattern in % was significantly different between irrigation treatments but not between production systems. The % displayed a relatively consistent trend between 09:00 and 15:00 UTC, fluctuating within the range of 28 % to 58 %, primarily influenced by irrigation treatment and date. However, during the interval from 12:00 to 15:00 UTC, the % did not show significant differences across production systems, irrigation treatments, and dates. During this interval of time, the overall average values of % were −14.47 %, −15.70 %, −10.2 %, and −2.47 % from 12:00 to 15:00 UTC, respectively.
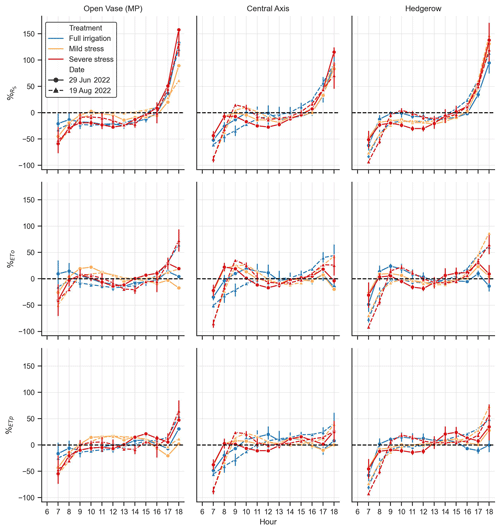
Figure 6Daily evolution of differences between hourly and daily means of αRs, αETo, and αETp. α represents the ratio between transpiration and the reference variable, while “ %” corresponds to the formula , where the subindex indicates the respective method. The x axis represents the time in UTC+0, with solar noon occurring around 12:00 UTC at the experimental site.
For its part, %ETo exhibited a distinct diurnal pattern between the two dates. Values remained relatively constant between 09:00 and 16:00 UTC, ranging from −16.44 % to 18.06 % on both dates. Similarly to %, %ETo showed no significant differences between irrigation treatments and dates from 12:00 to 14:00 UTC. During this time interval, the mean %ETo values were −4.87 % at 12:00 UTC, −8.52 % at 13:00 UTC, and −4.71 % at 14:00 UTC. The interaction between irrigation treatment and date began to display significant differences from 14:00 to 18:00 UTC, with the severe-stress treatment on 29 June showing significantly higher values. On the other hand, the %ETp pattern exhibited significant variations depending on irrigation treatment and date from 07:00 to 10:00 UTC and after 17:00 UTC. However, between 11:00 and 16:00 UTC, %ETp did not exhibit any significant effects attributable to the production system, irrigation treatment, or date. Between 11:00 and 16:00 UTC, %ETp ranged from −1.16 % to 13.07 %, with the minimum percentage difference being recorded at 11:00 UTC (1.16 %) on 29 June and at 12:00 UTC (3.10 %) on 19 August.
Figure 7 shows the relative RMSE (RRMSE) and bias (Rbias) when estimating Td from Th–SF. The Td–SF–EFsim exhibited an RRMSE ranging from 2.7 % to 26 % after 07:00 UTC. Overall, the lowest RRMSE for Td–SF–EFsim was observed at 14:00 UTC. However, the RRMSE of Td–SF–EFsim showed significant variability among irrigation treatments at this time, with values of 8.22 %, 5.28 %, and 17.4 % for the full-irrigation, mild-stress, and severe-stress treatments, respectively. Conversely, the RRMSE of Td–SF–EFsim varied as a function of date in the severe-stress treatment after 12:00 UTC. While, on 29 June, the RRMSE decreased, on 19 August, it increased, with values of 26.14 % at 14:00 UTC and 25.36 % at 16:00 UTC.
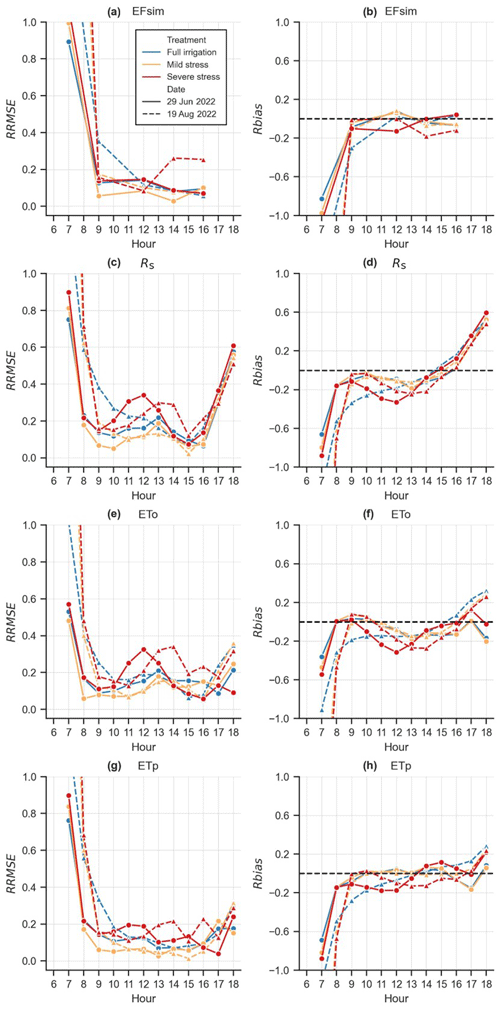
Figure 7Relative RMSE (RRMSE) and bias (Rbias) calculated for daily transpiration estimates obtained through the EFsim, Rs, ETo, and ETp methods using Th–SF at the time when ETo was greater than 0 mm h−1. The x axis represents the time in UTC+0, with solar noon occurring around 12:00 UTC at the experimental site.
The RRMSE of Td–SF–Rs and Td–SF–ETo varied with irrigation treatment. In the full-irrigation and mild-stress treatments, the RRMSE of Td–SF–Rs showed a convex shape throughout the day, with higher values in the early morning and late afternoon. In the full-irrigation treatment, the RRMSE of Td–SF–Rs steadily decreased until 15:00 UTC, reaching an average minimum value of 8.4 %. In the mild-stress treatment, the RRMSE of Td–SF–Rs remained relatively constant at around 10 % between 09:00 and 16:00 UTC, reaching its lowest point at 15:00 UTC (RRMSE of 4.18 %). In the severe-stress treatment, the RRMSE of Td–SF–Rs exhibited a sinusoidal-curve pattern. In this treatment, the RRMSE of Td–SF–Rs hovered around 15.3 % at 09:00 UTC and began to increase until 12:00 or 13:00 UTC, with a mean RMSE ranging between 29.83 % and 34 % depending on the date. On both dates, the RRMSE of Td–SF–Rs decreased at 15:00 UTC, with an RMSE of 7.37 % and 12.08 % on 29 June and 19 August, respectively.
The time at which the minimum RRMSE of Td–SF–ETo occurred varied depending on the interaction between irrigation treatment and date. In the full-irrigation treatment, the lowest RRMSE of Td–SF–ETo, corresponding to 8.59 %, was observed at 17:00 UTC on 29 June. Conversely, in the full-irrigation treatment, the minimum RRMSE of Td–SF–ETo (6.29 %) was recorded at 15:00 UTC on 29 August. For the mild-stress treatment, the minimum RRMSE of Td–SF–ETo on 19 June was 5.86 % and was recorded at 08:00 UTC, while the minimum RRMSE of Td–SF–ETo on 29 August was observed at 16:00 UTC, corresponding to 5.27 %. Similarly to Td–SF–Rs, the RRMSE of Td–SF–ETo in the severe-stress treatment presented a sinusoidal curve. The RRMSE of Td–SF–ETo decreased until approximately 10:00 or 11:00 UTC before gradually increasing around noon. On 29 June, it reached a maximum value of 32.50 % at 12:00 UTC. After 12:00 UTC, the RRMSE of Td–SF–ETo began to decrease and reached 5.70 % at 16:00 UTC. On 29 August, a maximum RRMSE of Td–SF–ETo was observed at 14:00 UTC, reaching 34.01 %, whereas the minimum RRMSE of Td–SF–ETo was recorded at 11:00 UTC (RRMSE of 12.83 %). Finally, the RRMSE of Td–SF–ETp exhibited higher values in the early morning and late afternoon but remained constant from 09:00 to 17:00 UTC. Although the severe-stress treatment exhibited the highest RMSE of Td–SF–ETp, no significant differences were detected among production systems, irrigation treatments, or dates. Overall, the minimum RRMSE of Td–SF–ETp of 7.51 % was recorded at 15:00 UTC.
3.2 Regression of measured and remotely estimated Th with the TSEB–2T
Figure 8 shows the concurrence between Th–TSEB and Th–SF. The most accurate estimation was obtained at 14:00 UTC, with an RRMSE of 29 % and an R2 value of 0.81. Comparable error statistics were obtained at 12:00 UTC, with an RRMSE of 39 % and an R2 value of 0.71. The least favorable outcomes were observed during early-morning flights, specifically at 07:00 UTC, when the TSEB–2T model provided null estimations for multiple trees. Overall, Th–TSEB showed an overestimation at all hours. However, these overestimations were more pronounced at 09:00 and 16:00 UTC, which, respectively, corresponded to RRMSE values of 77 % and 59 %.
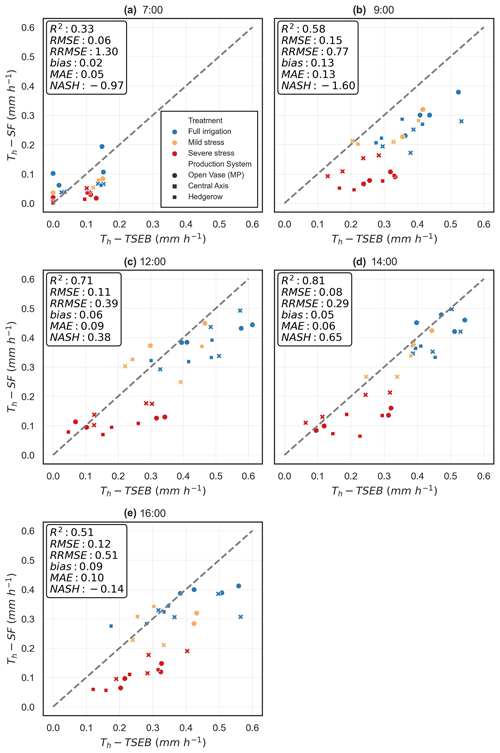
Figure 8Regressions between measured and estimated hourly transpiration with the TSEB–2T model and high-resolution images by hour, production system, and irrigation treatment.
Table 3 presents an ANOVA analysis aimed at assessing the sensitivity of Th–TSEB to the production system, irrigation treatment, and date. The estimations of Th–TSEB indicated significant differences among irrigation treatments across all flight times. The three irrigation treatments could be differentiated using the estimations at 09:00, 12:00, and 14:00 UTC. On the other hand, the Th–TSEB at 07:00 UTC in the mild-stress treatment presented similar values compared with the full-irrigation treatment. At 16:00 UTC, the mild-stress treatment showed comparable Th–TSEB estimations in relation to the full-irrigation and severe-stress treatments. The production system presented important differences in Th–TSEB when considering the flights conducted at 09:00 and 16:00 UTC. At these times, the open-vase (MP) production system exhibited significantly higher Th–TSEB. In contrast, the flights conducted at 07:00, 12:00, and 14:00 UTC estimated similar Th–TSEB values between production systems.
Table 3Analysis of variance (three-way ANOVA) testing the effects of date, production system (PS), and irrigation treatment (TRT) on Th–TSEB for each hour of flight conducted. The p values less than 0.05 were considered to be statistically significant, while “ns” represents a statistically non-significant interaction.
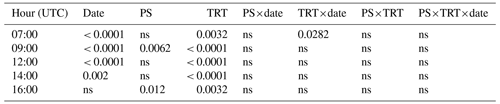
Table 4 shows the influence of production system, irrigation treatment, and date on the squared error of Th–TSEB compared with Th–SF. The results indicate that Th–TSEB using the flight performed at 07:00 UTC on 29 June exhibited a systematic error, generating important differences in the squared error due to the date. Furthermore, the squared error showed significant differences between irrigation treatments only for the flights conducted at 09:00 UTC. Additionally, a significant effect attributed to the interaction of production systems and irrigation treatments was observed at 9:00 UTC. Notably, the open vase (MP) system under the severe-stress treatment exhibited a higher error at 09:00 UTC. Moreover, while the production system, treatment, and date did not have a significant impact on the RMSE of Th–TSEB for flights conducted at 12:00, 14:00, and 16:00 UTC (Table 4), it is noteworthy that the severe-stress treatment consistently exhibited a higher error across all flight hours (Table 5).
Table 4Analysis of variance (three-way ANOVA) evaluating the effects of date, production system (PS), and irrigation treatment (TRT) on the root mean squared error (RMSE) of Th–TSEB for each hour of flight conducted. The p values less than 0.05 were considered to be statistically significant, while “ns” represents a statistically non-significant interaction.
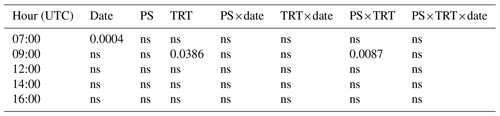
Table 5Root mean squared error (RMSE) of Th–TSEB (mm h−1), categorized by irrigation treatment, for each hour of flight conducted. Different letters mean significant differences at p value <0.05 using Tukey's honest significant difference test considering the interaction between production system and irrigation treatment.

3.3 Evaluation of daily upscaling methods to estimate Td with the TSEB–2T
The Td was estimated using the different upscaling methodologies and with the 14:00 UTC Th–TSEB estimation as a starting point (Fig. 9). The Th–TSEB at 14:00 UTC was selected due to it having the highest accuracy obtained (Fig. 8) when validated against the Th–SF. Overall, the results indicate that the EFsim, Rs, and ETo upscaling methods yielded similar results, even reducing the RRMSE obtained by Th–TSEB. In contrast, the ETp methods exhibited higher RMSE than those obtained by Th–TSEB. The Td–TSEB–Rs and Td–TSEB–ETo reached the highest accuracy, showing an RMSE (RRMSE) of 0.62 mm d−1 (23 %) and 0.61 mm d−1 (22 %), respectively. The Td–TSEB–EFsim and Td–TSEB–ETp approaches had RMSE (RRMSE) values of 0.72 mm d−1(26 %) and 0.89 mm d−1(32 %), respectively. In addition, the Td–TSEB–EFsim and Td–TSEB–ETp yielded larger overestimations, with biases of 0.38 and 0.61 mm d−1, compared to Td–TSEB–Rs and Td–TSEB–ETo, which had biases of 0.22 and 0.14 mm d−1.
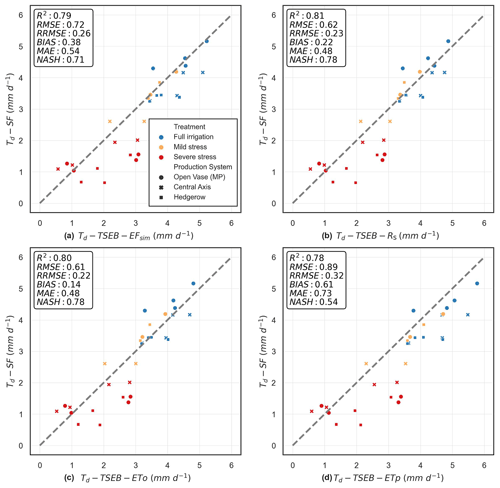
Figure 9Regressions between measured (Td–SF) and estimated daily transpiration (Td–TSEB) by production system and irrigation treatment with the following upscaling methodologies: (a) EFsim, (b) Rs, (c) ETo, and (d) ETp.
Table 6 shows an ANOVA analysis performed to detect the sensitivity of Td–TSEB–EFsim, Td–TSEB–Rs, Td–TSEB–ETo, and Td–TSEB–ETp to irrigation treatment, production system, and date. The results indicate that all approaches exhibited significant differences in the estimated Td, attributable to irrigation treatment and date. Finally, an ANOVA analysis was conducted to assess the influence of each upscaling method on the RMSE, considering production system, irrigation treatment, and date (Table 7). The RMSE in Td–TSEB–EFsim, Td–TSEB–Rs, Td–TSEB–ETo, and Td–TSEB–ETp varied significantly due to irrigation treatment. All the daily upscaling methods resulted in significantly higher RMSE values in the severe-stress treatment (Table 8).
Table 6Analysis of variance (three-way ANOVA) for the evaluation of the effects of date, production system (PS), and irrigation treatment (TRT) on Td estimated with TSEB–2T using flights conducted at 14:00 UTC and EFsim, Rs, ETo, and ETp upscaling methods. The p values less than 0.05 were considered to be statistically significant, while “ns” refers to a statistically non-significant interaction.

Table 7Analysis of variance (three-way ANOVA) evaluating the effects of date, production system (PS), and irrigation treatment (TRT) on the root mean squared error (RMSE) of Td estimated with TSEB–2T using flights conducted at 14:00 UTC and EFsim, Rs, ETo, and ETp upscaling methods. The p values less than 0.05 were considered to be statistically significant, while “ns” refers to a statistically non-significant interaction.

Table 8Root mean squared error (RMSE, mm d−1) of the estimated Td using the TSEB–2T and EFsim, Rs, ETo, and ETp upscaling approaches by irrigation treatment. Different letters mean significant differences at p < 0.05 using Tukey's honest significant difference test considering the irrigation treatments.
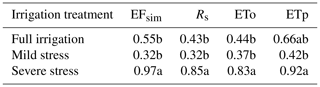
The timing of measurements is crucial for determining the level of water stress and accurately estimating Tp fluxes. Our observations indicate that the highest differences in Ψs, gs, Eleaf, and Th–SF between irrigation treatments were near solar noon (between 11:00 and 14:00 UTC), underlining the importance of considering diurnal variations in plant responses to water stress. This period is often when water stress is most pronounced and when plant physiological processes are most affected. Accurate measurements during this critical time frame can provide valuable insights into the impact of water stress on plant behavior and Tp rates. Therefore, our findings reinforce the conclusion that the best moment to determinate water stress is at noon or early afternoon, considering the maximum peaks of Tp (Gentine et al., 2007; Delogu et al., 2012) and the maximum differences between water status (Bellvert et al., 2014; Anderson et al., 2021; Tian and Schreiner, 2021).
In addition, the Th–TSEB values estimated using images obtained at 12:00 and 14:00 UTC yielded the most accurate results and were able to detect greater differences between irrigation treatments, while the irrigation treatment did not significantly affect the RMSE (Tables 3 and 4). This is in line with the findings of Anderson et al. (2021), who showed how earlier overpasses often created uniform maps of ET without differentiating between crop water demand. Additionally, Bellvert et al. (2014) showed that the optimal time for capturing high-resolution thermal images to minimize shade effects and to monitor leaf water potential and canopy temperature is around solar noon. The higher overestimations in Th–TSEB estimated using images obtained at 07:00, 09:00, and 16:00 UTC could be explained by the shadow that covered the thermal images. The thermal images captured at 07:00, 09:00, and 16:00 UTC were more susceptible to thermal radiation directionality (TDR) and shadow effects resulting from the higher zenith angle of the sun. Moreover, the significant contrast between inter-row soils and canopies leads to considerable directional variability in the thermal images (Mwangi et al., 2023). Although TSEB–2T accounts for radiation directionality when estimating H (Norman et al., 1995) and shortwave transmittance (Parry et al., 2019), it may still be susceptible to shadow effects because it does not distinguish between sunlit and shaded sources. To address this issue, Mwangi et al. (2022) proposed a four-component scheme (SPARSE4) as an option to account for sunlit vs. shaded soil and/or vegetation energy sources. This scheme couples a dual-source energy balance (SPARSE) model with the physically based unified four-component radiative transfer (UFR97) model. However, The Th–TSEB model demonstrates effective differentiation between irrigation treatments at all hours, particularly around solar noon, where differences between all irrigation treatments are evident. Conversely, it is noteworthy that Th–TSEB fails to exhibit significant differences between production systems around solar noon (Table 3). This poses a challenge when estimating Td using upscaling methods as no model detected variations in Td by production system, as evidenced by Td–SF (Table 6). This limitation is especially critical when estimating Td from Th–TSEB in canopies with diverse architectural structures.
While the comparison between actual Td–SF and Td–TSEB–EFsim, Td–TSEB–Rs, Td–TSEB–ETo, and Td–TSEB–ETp showed similar results (Fig. 9), Td–TSEB–Rs and Td–TSEB–ETo enhanced the accuracy of Td estimates, which is reflected in the reduced RMSE values of 0.62 and 0.61 mm d−1, respectively. It should be noted that both Cammalleri et al. (2014) and Nassar et al. (2021) reported the Rs method as yielding the best results when used as an upscaling parameter to estimate daily ET. However, our results suggest that the superior performance of Rs and ETo in estimating Td can be attributed to their capacity to rectify the overestimation observed in Th–TSEB estimates rather than their inherent alignment with the Th–SF pattern. The underestimation is clarified by the % at 14:00 UTC, which ranged from −2.47 % to −14.47 %, while the %ETo ranged from −4.71 % to −8.52 % (Fig. 6). Related to our results, Van Niel et al. (2012) and Cammalleri et al. (2014) observed a systematic underestimation of estimated daily ET values using the Rs approach for a wide range of ecosystems and weather conditions. In this regard, Anderson et al. (1997) proposed a correction factor of 1.1 to compensate for systematic bias, increasing by 10 % the daily ET estimations. Among the advantages of the Rs method, Cammalleri et al. (2014) highlighted its uniform bias around the acquisition time and throughout the season, which is in contrast to using the EF method, ETo, and the top-of-atmosphere radiance (RTOA) as reference variables. Nassar et al. (2021) also found that the Rs method exhibits less sensitivity to seasonal and climate variations compared to the EF approach and the use of the ratio of net radiation to solar radiation (). It is important to clarify that both the above-cited studies evaluated the EF approach with actual AE measurements using eddy covariance towers as validation data sources.
However, our findings indicate that the correction factor proposed by Anderson et al. (1997) of 1.1 for Td should be determined by water stress; otherwise, it may be deemed inadequate. In addition, the timing of the overpass is crucial in determining a correction factor for using the Rs and ETo approaches, particularly for trees experiencing water stress. For instance, although the minimum RRMSE of Td–SF–Rs of 10 % might be achievable at 15:00 UTC, the RRMSE of Td–SF–Rs could reach 25 %–30 % around midday in trees under waters stress. Given the variations in the RRMSE when estimating Td–SF–Rs throughout the day and between days, establishing an appropriate correction factor for water-stressed trees presents a challenge. These findings complement those of Cammalleri et al. (2014) and Nassar et al. (2021), who concluded that the Rs method is minimally influenced by the timing of the daytime overpass in unstressed vegetation.
The errors associated with the Rs and ETo approaches could be attributable to fluctuations in the daily patterns of Rs, ETo, and the physiological condition of the tree throughout the day. Rs and ETo exhibited an almost perfect concave shape, with their maximum values occurring at 13:00 UTC (Fig. 2), which was the local solar noon at our study site. Conversely, in the full-irrigation treatment, while stomatal closure was observed at 12:00 UTC, both Eleaf and Th–SF either remained steady or even increased until 14:00 UTC (Figs. 3 and 4). The maintenance or increase in Eleaf and Th–SF during the early afternoon can be attributed to the rise in air temperature and the decrease in RH, consequently leading to an increase in VPD during the afternoon (Fig. 2). The variation in patterns between Rs, ETo, and Th–SF resulted in lower hourly values of Th–SF Rs (or Th–SF ETo) at midday compared to those observed during the early afternoon (15:00–16:00 UTC). On the other hand, Th–SF Rs (or Th–SF ETo) in the early afternoon exhibited more representative values for estimating Td in the fully irrigated treatment from Th–SF (Fig. 6).
In contrast, the early water stress, as indicated by the Ψs, resulted in stomatal closure being detected at 09:00 UTC in the severe-stress treatment. The impact of stomatal closure can be observed in Fig. 4, where the maximum Th–SF was achieved before noon in the severe-stress treatment, specifically between 10:00 and 12:00 UTC. Despite the increase in Rs and ETo, the maximum Th–SF in the severe-stress treatment remained nearly constant between 10:00 and 16:00 UTC on 19 August. On 29 June, Th–SF in the severe-stress treatment increased rapidly, starting from 12:00 UTC and reaching its maximum value at 14:00 UTC, similarly to the full-irrigation treatment. As a result, the disparities between Th–SF and Rs (or ETo) were most pronounced at midday (Fig. 6), leading to significant potential underestimations when using midday measurements. While Rs and ETo decreased, Th–SF remained at its maximum until 16:00 UTC. Consequently, the relationship between Th–SF and Rs (or ETo) started to become more representative of Td–SF at 15:00 UTC on both dates. Therefore, it appears to be the case that the optimal time to estimate instantaneous Tp for daily estimations would fall in the early afternoon, specifically at 15:00 UTC, for both the Rs and ETo approaches in all irrigation treatments.
Regarding the ETo method, in line with the findings of Cammalleri et al. (2014), using ETo as a reference variable produced results similar to those of the Rs method, indicating that it does not represent an improvement. Moreover, the %ETo pattern varied from one date to another (Fig. 6), introducing uncertainty into the potential upscaling adjustments. Differences in the %ETo patterns between days may be attributable to variations in the aerodynamic properties of the canopy between the reference vegetation and the almond canopy. For instance, Colaizzi et al. (2006) obtained good results when applying the ETo method to alfalfa and irrigated cotton but poor results for bare soil in drying conditions. It should also be noted that the microclimatic conditions at the location of the weather station may differ from those of the study site, introducing uncertainty into estimation of the actual ETo of the orchard under study. These two issues may be a possible limitation when using ETo as a reference variable to estimate Td fluxes (Cammalleri et al., 2014).
The EFsim and ETp methods appear to enhance the Td, considering the better and more consistent RRMSE observed throughout the day for Td–SF–EFsim and Td–SF–ETp in contrast to Td–SF–Rs and Td–SF–ETo. This improvement was also noted in the RRMSE of Td–TSEB–EFsim and Td–TSEB–ETp, which yielded more similar RRMSE compared to Th–TSEB. This suggests that the EFsim and ETp methods induced fewer modifications to the error associated with TSEB itself compared to the Rs and ETo methods. The improvement in the EFsim method aligns with the daily EF curve observed in previous studies, which does not remain constant but instead exhibits an upward concave shape, especially in non-stressed vegetation (Hoedjes et al., 2008; Delogu et al., 2012; Lhomme and Elguero, 1999; Brutsaert, 1992). Delogu et al. (2012) showed an improvement in the reconstruction of daily ET for various sites and under different climatic conditions, including low water stress, using the EFsim methodology. This is in line with our findings if we consider the fact that their “low-water-stress” conditions align with the mild-stress treatment. However, it should be noted that the RRMSE of Td–SF–EFsim may increase when using AE estimations during the afternoon for trees under water stress. This is why the RMSE values are significantly higher in Td–TSEB–EFsim when using the TSEB–2T at 14:00 UTC (Table 7). The actual EF shape under water stress during the day differs from the EFsim shape, presenting a flatter profile (Hoedjes et al., 2008; Lhomme and Elguero, 1999). The larger differences between actual EF and EFsim during the afternoon could potentially lead to an underestimation of Td when using the EFsim method. However, EFsim was able to reduce the RRMSE of Td–SF–EFsim at noon in the severe-stress treatment by up to 15.35 % and 17.61 % compared to Td–SF–ETo and Td–SF–Rs, respectively. Additionally, the Td–SF–EFsim exhibited 5 % less RRMSE than Td–SF–ETo and Td–SF–Rs in the full-irrigation and mild-stress treatments. This indicates that the EFsim method might perform well under certain conditions but may have limitations, especially when applied to severely water-stressed trees using afternoon measurements.
The remarkable similarity in patterns between ETp and Th–SF is particularly surprising, considering the concerns raised by Delogu et al. (2012) regarding the applicability of the ETp method under stress conditions. Delogu et al. (2012) suggested that actual ET and ETp might exhibit different daily patterns due to stomatal closure, potentially causing a negative bias when using ETp as a daily upscaling parameter. However, the daily curve of the ratio between Th–SF and hourly ETp (%ETp) mitigates the sinusoidal shape of % and %ETo, which otherwise increases exponentially from noon to 18:00 UTC. Furthermore, the RRMSE of Td–SF–ETp improves compared to the other methodologies and shows less variability among irrigation treatments and hours compared to using Rs and ETo as the adjustment variables. This improvement was particularly evident at 12:00 UTC, where Td–SF–ETp showed approximately 5 % less RRMSE in the full-irrigation and mild-stress treatments while reducing RRMSE at noon by 10.6 % and 12.92 % in the severe-stress treatment compared to Td–SF–ETo and Td–SF–Rs, respectively.
Moreover, the ETp model may hold an advantage due to its incorporation of distinct aerodynamic and radiative properties associated with various canopy architectures, which influence the Th–SF pattern. The variation in RMSE in the estimation of ETp among production systems likely impacted the sensitivity of the ETp model fit to each specific production system. The absence of significant differences in LAI among production systems could affect the accuracy of ETp. Quintanilla-Albornoz et al. (2023) already showed a discrepancy between measured LAI and the fraction of intercepted photosynthetically active radiation (fIPAR) at the study site, where the hedgerow presented higher LAI values but low fIPAR levels. Considering the fact that fIPAR represents 45 % of the absorbed-light spectrum (Campbell and Norman, 1998), these results reinforce the idea of improving the shortwave transmittance model for estimating ET fluxes. Indeed, among the complexities, estimating parameters such as LAI, albedo, and the potential single-leaf stomatal resistance is considered to be challenging and can pose difficulties in making ETp estimations suitable for operational purposes (Delogu et al., 2012; Gao et al., 2022). However, enhanced ETp models and the refinement of crucial inputs like LAI and albedo can streamline and enhance ETp estimations, further enhancing their utility as a parameter for Td estimation in trees with different canopy architecture. For instance, implementing a more intricate model to estimate ETp, like the Shuttleworth and Wallace two-source model (Shuttleworth and Wallace, 1985), could enhance the daily upscaling method. This study was conducted over 2 measurement days with meteorological forcing conditions representative of typical summer days at the study site (Fig. 2). Additional measurement days would allow for the consideration of a wider range of meteorological forcing conditions and vegetative stages in almond trees for a more robust assessment of daily Tp patterns. However, the data collected effectively represent trees under varying levels of water stress (Fig. 3), consistently with the conditions necessary to address the hypothesis of this work.
This study evaluates four methodologies to estimate Td from instantaneous measurements. The daily upscaling methods were evaluated using sap flow measurements in almond trees under three different production systems and three irrigation treatments. Additionally, this study analyzed the daily pattern of physiological parameters, such as Ψs, gs, Eleaf, and Th–SF, to determine the best moment to estimate both Th and Td.
The Th–TSEB model effectively distinguished between irrigation treatments, especially at 12:00 and 14:00 UTC, when differences between the three irrigation treatments were apparent. However, the Th–TSEB did not show significant differences between the production systems at that time. Therefore, of the evaluated upscaling methods, none of the models could discern the significant differences in Td estimates across production systems, as observed in Td–SF. In addition, the upscaling methodologies were less accurate in severely stressed trees. Especially when using Rs and ETo as reference variables, the levels of underestimation exhibited significant variations between irrigation treatments and across different hours. Underestimation was as high as 30 % around noon for trees under water stress using the Rs and ETo methods. Therefore, it is advisable to carefully choose an appropriate time schedule. In this context, the EFsim and ETp methods demonstrated more consistent relationships with Th–SF and mitigated the underestimation observed in all irrigation treatments when using the other methods. For instance, both the ETsim and ETp models reduced the RRMSE by 5 % in the full-irrigation and mild-stress treatments using measurements at 12:00 UTC. In the severe-stress treatment, EFsim reduced the RRMSE by 17.61 % and 15.25 % at noon compared to the Rs and ETo methods, respectively, while ETp reduced it by 10.6 % and 12.92 % at noon compared to the Rs and ETo methods, respectively.
Moreover, ETp has the advantage of incorporating different aerodynamic and radiative properties associated with production systems. In this sense, the ETp method may be an option to better characterize the Td in trees with different canopy architectures. In this study, similar LAI estimates between production systems could affect the ETp model, where the hedgerow system showed a significantly higher error. This situation could impact the sensitivity of the ETp model with regard to differentiating Td between production systems. One approach to enhance Td estimations could involve refining the Penman–Monteith ETp model and improving the estimations of parameters such as the LAI, albedo, potential single-leaf stomatal resistance, and shortwave transmittance model. Alternatively, using more sophisticated models, such as the Shuttleworth and Wallace two-source model, could also be considered.
All data mentioned in this document have been generated by the IRTA Efficient Use of Water in Agriculture Program team. Datasets produced during this study can be made available upon reasonable request to the corresponding author and/or the Efficient Use of Water in Agriculture Program.
MQA: conceptualization, data curation, formal analysis, investigation, methodology, software, validation, visualization, and writing – original draft preparation. JB: conceptualization, funding acquisition, investigation, project administration, supervision, validation, and writing – original draft preparation. HN: software, validation, and writing – review and editing. XM: funding acquisition and writing – review and editing. AP: data curation and resources.
The contact author has declared that none of the authors has any competing interests.
Publisher’s note: Copernicus Publications remains neutral with regard to jurisdictional claims made in the text, published maps, institutional affiliations, or any other geographical representation in this paper. While Copernicus Publications makes every effort to include appropriate place names, the final responsibility lies with the authors.
The authors would like to thank the Efficient Use of Water in Agriculture team, at the IRTA, for their technical support, as well as the European Union Horizon 2020 Research and Innovation Program (H2020) of the European Commission in the context of the Marie Sklodowska-Curie Research and Innovation Staff Exchange (RISE) action and ACCWA project: grant agreement no. 823965. To enhance the readability and language of this work, the author(s) employed ChatGPT-3.5 throughout the writing process. Following the use of this tool, the author(s) assumed full responsibility for the papers content and reviewed and edited it as required.
This research has been supported by the projects ET4DROUGHT (grant no. PID2021-127345OR-C31) and DIGISPAC (grant no. TED2021-131237B-C21), both funded by the Ministry of Science and Innovation (MICINN-AEI) of Spain.
This paper was edited by Miriam Coenders-Gerrits and reviewed by Gilles Boulet and one anonymous referee.
Alarcón, J., Ortuño, M., Nicolás, E., Torres, R., and Torrecillas, A.: Compensation heat-pulse measurements of sap flow for estimating transpiration in young lemon trees, Biol. Plant. 49, 527–532, https://doi.org/10.1007/s10535-005-0046-1, 2005.
Allen, R., Pereira, L., Raes, D., and Smith, M.: Crops evapotranspiration: guidelines forcomputing crop water requirements. FAO Irrigation and Drainage Paper No. 56.FAO, Rome, Italy, 300, ISBN 92-5-104219-5, https://www.fao.org/4/x0490e/x0490e00.htm (last access: 13 December 2023), 1998.
Allen, R., Tasumi, M., Morse, A., Trezza, R., Wright, J., Bastiaanssen, W., Kramber, W., Lorite, I., and Robison, C. W.: Satellite-Based Energy Balance for Mapping Evapotranspiration with Internalized Calibration (METRIC) – Applications, J. Irrig. Drain. Eng., 133, 395–406, https://doi.org/10.1061/(asce)0733-9437(2007)133:4(395), 2007.
Anderson, M., Norman, J., Diak, G., Kustas, W., and Mecikalski, J.: A two-source time-integrated model for estimating surface fluxes using thermal infrared remote sensing, Remote Sens. Environ., 60, 195–216, https://doi.org/10.1016/S0034-4257(96)00215-5, 1997.
Anderson, M., Yang, Y., Xue, J., Knipper, K., Yang, Y., Gao, F., Hain, C., Kustas, W., Cawse-Nicholson, K., Hulley, G., Fisher, J., Alfieri, J., Meyers, T., Prueger, J., Baldocchi, D., and Rey-Sanchez, C.: Interoperability of ECOSTRESS and Landsat for mapping evapotranspiration time series at sub-field scales, Remote Sens. Environ., 252, 112189, https://doi.org/10.1016/j.rse.2020.112189, 2021.
Basilio, R., Hook, S., Zoffoli, S., and Buongiorno, M.: Surface Biology and Geology (SBG) Thermal Infrared (TIR) Free -Flyer Concept.: 2022 IEEE Aerospace Conference (AERO), Big Sky, MT, USA, 01–09, https://doi.org/10.1109/AERO53065.2022.9843292, 2022.
Bastiaanssen, W., Pelgrum, H., Wang, J., Ma, Y., and Moreno, J. F.: A remote sensing surface energy balance algorithm for land (SEBAL).: Part 2: Validation, J. Hydrol., 212, 213–229, https://doi.org/10.1016/S0022-1694(98)00254-6, 1998.
Bellvert, J., Zarco-Tejada, P., Girona, J., and Fereres, E.: Mapping crop water stress index in a “Pinot-noir” vineyard: Comparing ground measurements with thermal remote sensing imagery from an unmanned aerial vehicle, Precis. Agric., 15, 361–376, https://doi.org/10.1007/s11119-013-9334-5, 2014.
Brutsaert, W. and Sugita M.: Application of self-preservation in the diurnal evolution of the surface energy budget to determine daily evaporation, J. Geophys. Res., 97, 18377–18382, https://doi.org/10.1029/92JD00255, 1992.
Cammalleri, C., Anderson, M. C., and Kustas, W. P.: Upscaling of evapotranspiration fluxes from instantaneous to daytime scales for thermal remote sensing applications, Hydrol. Earth Syst. Sci., 18, 1885–1894, https://doi.org/10.5194/hess-18-1885-2014, 2014.
Campbell, G. and Norman, J.: An introduction to environmental biophysics, second ed., Springer New York, NY, 286 pp., https://doi.org/10.1007/978-1-4612-1626-1, 1998.
Crago, R. and Brutsaert, W.: Daytime evaporation and the self-preservation of the evaporative fraction and the Bowen ratio, J. Hydrol., 178, 241–255, https://doi.org/10.1016/0022-1694(95)02803-x, 1996.
Castel, J. and Fereres, E.: Responses of Young Almond Trees to Two Drought Periods in the Field, J. Hortic. Sci., 57, 175–187, https://doi.org/10.1080/00221589.1982.11515038, 1982.
Chaves, M., Pereira, J. S., Maroco, J., Rodrigues, M., Ricardo, C., Osório, M., Carvalho, I., Faria, T., and Pinheiro, C.: How plants cope with water stress in the field. Photosynthesis and growth, Ann. Bot., 89, 907–916, https://doi.org/10.1093/aob/mcf105, 2002.
Colaizzi, P., Evett, S., Howell, T., and Tolk, J.: Comparison of five models to scale daily evapotranspiration from one-time-of-day measurements, American Society of Agricultural and Biological Engineers, 49, 1409–1418, https://doi.org/10.13031/2013.22056, 2006.
Delogu, E., Boulet, G., Olioso, A., Coudert, B., Chirouze, J., Ceschia, E., Le Dantec, V., Marloie, O., Chehbouni, G., and Lagouarde, J.-P.: Reconstruction of temporal variations of evapotranspiration using instantaneous estimates at the time of satellite overpass, Hydrol. Earth Syst. Sci., 16, 2995–3010, https://doi.org/10.5194/hess-16-2995-2012, 2012.
Drexler, J., Snyder, R., Spano, D., and Paw U.: A review of models and micrometeorological methods used to estimate wetland evapotranspiration, Hydrol. Process., 18, 2071–2101, https://doi.org/10.1002/hyp.1462, 2004.
Escalona, J., Flexas, J., and Medrano, H.: Stomatal and non-stomatal limitations of photosynthesis under water stress in field-grown grapevines, Aust. J. Plant Physiol., 26, 421–433, https://doi.org/10.1071/PP99019, 1999.
Espadafor, M., Orgaz, F., Testi, L., Lorite, I., and Villalobos, F.: Transpiration of young almond trees in relation to intercepted radiation, Irrigation Sci., 33, 265–275, https://doi.org/10.1007/s00271-015-0464-6, 2015.
Evett, S. and Tolk, J.: Introduction: Can water use efficiency be modeled well enough to impact crop management?, Agron. J., 101, 423–425, https://doi.org/10.2134/agronj2009.0038xs, 2009.
Fernandez, J., Palomo, M., Díaz-Espejo, A., Clothier, B., Green, S., Girón, I., and Moreno, F.: Heat-pulse measurements of sap flow in olives for automating irrigation: tests root flow and diagnostics of water stress, Agr. Water Manage., 51, 99–123, https://doi.org/10.1016/S0378-3774(01)00119-6, 2001.
Forster, M.: How Reliable Are Heat Pulse Velocity Methods for Estimating Tree Transpiration?, Forests, 8, 350, https://doi.org/10.3390/f8090350, 2017.
Gao, R., Torres-Rua, A., Aboutalebi, M., White, W., Anderson, M., Kustas, W., Agam, N., Alsina, M., Alfieri, J., Hipps, L., Dokoozlian, N., Nieto, H., Gao, F., McKee, L., Prueger, J., Sanchez, L., Mcelrone, A., Bambach-Ortiz, N., Coopmans, C., and Gowing, I.: LAI estimation across California vineyards using sUAS multi-seasonal multi-spectral, thermal, and elevation information and machine learning, Irrigation Sci., 40, 731–759, https://doi.org/10.1007/s00271-022-00776-0, 2022.
Gao, R., Torres-Rua, A., Nieto, H., Zahn, E., Hipps, L., Kustas, W., Alsina, M., Bambach, N., Castro, S., Prueger, J., Alfieri, J., Mckee, L., White, W., Gao, F., Mcelrone, A., Anderson, M., Knipper, K., and Coopmans, C.: ET Partitioning Assessment Using the TSEB Model and sUAS Information across California Central Valley Vineyards, Remote Sens., 15, 756, https://doi.org/10.3390/rs15030756, 2023.
Gentine, P., Entekhabi, D., Chehbouni, A., Boulet, G., and Duchemin, B.: Analysis of evaporative fraction diurnal behaviour, Agr. Forest Meteorol., 143, 13–29, https://doi.org/10.1016/j.agrformet.2006.11.002, 2007.
Goldhamer, D. A. and Fereres, E.: Establishing an almond water production function for California using long-term yield response to variable irrigation, Irrigation Sci., 35, 169–179, https://doi.org/10.1007/s00271-016-0528-2, 2017.
Gómez-Candón, D., Bellvert, J., and Royo, C.: Performance of the Two-Source Energy Balance (TSEB) Model as a Tool for Monitoring the Response of Durum Wheat to Drought by High-Throughput Field Phenotyping, Front. Plant Sci., 12, 658357, https://doi.org/10.3389/fpls.2021.658357, 2021.
Hoedjes, J., Chehbouni, A., Jacob, F., Ezzahar, J., and Boulet, G.: Deriving daily evapotranspiration from remotely sensed instantaneous evaporative fraction over olive orchard in semi-arid Morocco, J. Hydrol., 354, 53–64, https://doi.org/10.1016/j.jhydrol.2008.02.016, 2008.
Iglesias, I. and Echeverria, G.: Scientia Horticulturae Current situation, trends and challenges for efficient and sustainable peach production, Sci. Hortic., 296, 110899, https://doi.org/10.1016/j.scienta.2022.110899, 2022.
Jackson, R., Hatfield, J., Reginato, R., Idso, S., and Pinter, P.: Estimation of daily evapotranspiration from one time-of-day measurements, Agr. Water Manage., 7, 351–362, https://doi.org/10.1016/0378-3774(83)90095-1, 1983.
Jiang, L., Zhang, B., Han, S., Chen, H., and Wei, Z.: Upscaling evapotranspiration from the instantaneous to the daily time scale: Assessing six methods including an optimized coefficient based on worldwide eddy covariance flux network, J. Hydrol., 596, 126135, https://doi.org/10.1016/j.jhydrol.2021.126135, 2021.
Jofre-Čekalović, C., Nieto, H., Girona, J., Pamies-Sans, M., and Bellvert, J.: Accounting for Almond Crop Water Use under Different Irrigation Regimes with a Two-Source Energy Balance Model and Copernicus-Based Inputs, Remote Sens., 14, 2106, https://doi.org/10.3390/rs14092106, 2022.
Kalma, J., McVicar, T., and McCabe, M.: Estimating land surface evaporation: A review of methods using remotely sensed surface temperature data, Surv. Geophys., 29, 421–469, https://doi.org/10.1007/s10712-008-9037-z, 2008.
Knipper, K., Anderson, M., Bambach, N., Kustas, W., Gao, F., Zahn, E., Hain, C., McElrone, A., Belfiore, O., Castro, S., Alsina, M., and Saa, S.: Evaluation of Partitioned Evaporation and Transpiration Estimates within the DisALEXI Modeling Framework over Irrigated Crops in California, Remote Sens., 15, 68, https://doi.org/10.3390/rs15010068, 2023.
Koetz, B., Bastiaanssen, W., Berger, M., Defourney, P., Bello, U. Del, Drusch, M., Drinkwater, M., Duca, R., Fernandez, V., Ghent, D., Guzinski, R., Hoogeveen, J., Hook, S., Lagouarde, J. P., Lemoine, G., Manolis, I., Martimort, P., Masek, J., Massart, M., Notarnicola, C., Sobrino, J., and Udelhoven, T.: High spatio-temporal resolution land surface temperature mission – A Copernicus candidate mission in support of agricultural monitoring, Int. Geosci. Remote Sens. Symp., 2018-July, 8160–8162, https://doi.org/10.1109/IGARSS.2018.8517433, 2018.
Kustas, W. and Norman, J.: Evaluation of soil and vegetation heat flux predictions using a simple two-source model with radiometric temperatures for partial canopy cover, Agr. Forest Meteorol., 94, 13–29, https://doi.org/10.1016/S0168-1923(99)00005-2, 1999.
Kustas, W. and Anderson, M.: Advances in thermal infrared remote sensing for land surface modeling, Agr. Forest Meteorol., 149, 2071–2081, https://doi.org/10.1016/j.agrformet.2009.05.016, 2009.
Kustas, W., Alfieri, J., Nieto, H., Wilson, T., Gao, F., and Anderson, M.: Utility of the two-source energy balance (TSEB) model in vine and interrow flux partitioning over the growing season, Irrigation Sci. 37, 375–388, https://doi.org/10.1007/s00271-018-0586-8, 2019.
Kustas, W., Nieto, H., Garcia-Tejera, O., Bambach, N., McElrone, A., Gao, F., Alfieri, J., Hipps, L., Prueger, J., Torres-Rua, A., Anderson, M., Knipper, K., Alsina, M., McKee, L., Zahn, E., Bou-Zeid, E., and Dokoozlian, N.: Impact of advection on two-source energy balance (TSEB) canopy transpiration parameterization for vineyards in the California Central Valley, Irrigation Sci., 40, 575–591, https://doi.org/10.1007/s00271-022-00778-y, 2022.
Lagouarde, J., Bhattacharya, B., Crébassol, P., Gamet, P., Babu, S., Boulet, G., Briottet, X., Buddhiraju, K., Cherchali, S., Dadou, I., Dedieu, G., Gouhier, M., Hagolle, O., Irvine, M., Jacob, F., Kumar, A., Kumar, K., Laignel, B., Mallick, K., Murthy, C., Olioso, A., Ottlé, C., Pandya, M., Raju, P., Roujean, J., Sekhar, M., Shukla, M., Singh, S., Sobrino, J., and Ramakrishnan, R.: The Indian-French Trishna mission: Earth observation in the thermal infrared with high spatio-temporal resolution, Int. Geosci. Remote Sens. Symp., 2018-July, 4078–4081, https://doi.org/10.1109/IGARSS.2018.8518720, 2018.
Lhomme, J.-P. and Elguero, E.: Examination of evaporative fraction diurnal behaviour using a soil-vegetation model coupled with a mixed-layer model, Hydrol. Earth Syst. Sci., 3, 259–270, https://doi.org/10.5194/hess-3-259-1999, 1999.
López-Bernal, Á., Alcántara, E., Testi, L., and Villalobos, F.: Spatial sap flow and xylem anatomical characteristics in olive trees under different irrigation regimes, Tree Physiol., 30, 1536–1544, https://doi.org/10.1093/treephys/tpq095, 2010.
López-López, M., Espadador, M., Testi, L., Lorite, I., Orgaz, F., and Fereres, E.: Water use of irrigated almond trees when subjected to water deficits, Agr. Water Manage., 195, 84–93, https://doi.org/10.1016/j.agwat.2017.10.001, 2018.
McCutchan, H. and Shackel, K.: Stem-water potential as a sensitive indicator of water stress in prune trees (Prunus domestica L. cv. French), J. Am. Soc. Hortic. Sci., 117, 607–611, 1992.
Mwangi, S., Boulet, G., and Olioso, A.: Assessment of an extended SPARSE model for estimating evapotranspiration from directional thermal infrared data, Agr. Forest Meteorol., 317, 108882, https://doi.org/10.1016/j.agrformet.2022.108882, 2022.
Mwangi, S., Boulet, G., Le Page, M., Gastellu-Etchegorry, J., Bellvert, J., Lemaire, B., Fanise, P., Roujean, J., and Olioso, A.: Observation and Assessment of Model Retrievals of Surface Exchange Components over a Row Canopy Using Directional Thermal Data, IEEE J. Sel. Top. Appl., 16, 7343–7356, https://doi.org/10.1109/JSTARS.2023.3297709, 2023.
Nassar, A., Torres-Rua, A., Kustas, W., Nieto, H., McKee, M., Hipps, L., Stevens, D., Alfieri, J., Prueger, J., Alsina, M., McKee, L., Coopmans, C., Sanchez, L., and Dokoozlian, N.: Influence of model grid size on the estimation of surface fluxes using the two source energy balance model and sUAS imagery in vineyards, Remote Sens., 12, 342, https://doi.org/10.3390/rs12030342, 2020.
Nassar, A., Torres-Rua, A., Kustas, W., Alfieri, J., Hipps, L., Prueger, J., Nieto, H., Alsina, M., White, W., McKee, L., Coopmans, C., Sanchez, L., and Dokoozlian, N.: Assessing daily evapotranspiration methodologies from one-time-of-day Suas and EC information in the GRAPEX project, Remote Sens., 13, 2887, https://doi.org/10.3390/rs13152887, 2021.
Nieto, H., Kustas, W., Torres-Rua, A., Alfieri, J., Gao, F., Anderson, M., White, W., Song, L., Alsina, M., Prueger, J., McKee, M., Elarab, M., and McKee, L.: Evaluation of TSEB turbulent fluxes using different methods for the retrieval of soil and canopy component temperatures from UAV thermal and multispectral imagery, Irrigation Sci., 37, 389–406, https://doi.org/10.1007/s00271-018-0585-9, 2019.
Nieto, N., Guzinski, R., Graae, P., Jonas, ClaireBrenner, Mike, and gabrielmini: hectornieto/pyTSEB: v2.2 (v.2.2), Zenodo [code], https://doi.org/10.5281/zenodo.8134956, 2023.
Norman, J., Kustas, W., and Humes, K.: Source approach for estimating soil and vegetation energy fluxes in observations of directional radiometric surface temperature, Agr. Forest Meteorol., 77, 263–293, https://doi.org/10.1016/0168-1923(95)02265-Y, 1995.
Noun, G., Lo Cascio, M., Spano, D., Marras, S., and Sirca, C.: Plant-Based Methodologies and Approaches for Estimating Plant Water Status of Mediterranean Tree Species: A Semi-Systematic Review, Agronomy, 12, 2127, https://doi.org/10.3390/agronomy12092127, 2022.
Olivo, N., Girona, J., and Marsal, J.: Seasonal sensitivity of stem water potential to vapour pressure deficit in grapevine, Irrigation Sci., 27, 175–182, https://doi.org/10.1007/s00271-008-0134-z, 2009.
Overgaard, J., Rosbjerg, D., and Butts, M. B.: Land-surface modelling in hydrological perspective – a review, Biogeosciences, 3, 229–241, https://doi.org/10.5194/bg-3-229-2006, 2006.
Parry, C., Nieto, H., Guillevic, P., Agam, N., Kustas, W., Alfieri, J., McKee, L., and McElrone, A.: An intercomparison of radiation partitioning models in vineyard canopies, Irrigation Sci., 37, 239–252, https://doi.org/10.1007/s00271-019-00621-x, 2019.
Peddinti, S. and Kisekka, I.: Estimation of turbulent fluxes over almond orchards using high-resolution aerial imagery with one and two-source energy balance models, Agr. Water Manage., 269, 107671, https://doi.org/10.1016/j.agwat.2022.107671, 2022.
Poni, S., Bernizzoni, F., Civardi, S., Gatti, M., Porro, D., and Camin, F.: Performance and water-use efficiency (single-leaf vs. whole-canopy) of well-watered and half-stressed split-root Lambrusco grapevines grown in Po Valley (Italy), Agr. Ecosyst. Environ., 129, 97–106, https://doi.org/10.1016/j.agee.2008.07.009, 2009.
Qi, J., Chehbouni, A., Huete, A., Kerr, Y., and Sorooshian, S.: A modified soil adjusted vegetation index, Remote Sens. Environ., 48, 119–126, https://doi.org/10.1016/0034-4257(94)90134-1, 1994.
Quintanilla-Albornoz, M., Miarnau, X., Pelechá, A., Casadesús, J., García-Tejera, O., and Bellvert, J.: Evaluation of transpiration in different almond production systems with two-source energy balance models from UAV thermal and multispectral imagery, Irrigation Sci., https://doi.org/10.1007/s00271-023-00888-1, 2023.
Romero, P. and Botía, P.: Daily and seasonal patterns of leaf water relations and gas exchange of regulated deficit-irrigated almond trees under semiarid conditions, Environ. Exp. Bot., 56, 158–173, https://doi.org/10.1016/j.envexpbot.2005.01.012, 2006.
Sánchez, J, Simón L., González-Piqueras. J., and Montoya F.: Monitoring Crop Evapotranspiration and Transpiration/Evaporation Partitioning in a Drip-Irrigated, Water-Switzerland, 13, 2073, https://doi.org/10.3390/w13152073, 2021.
Shuttleworth, W. and Wallace, J.: Evaporation from sparse crops-an energy combination theory, Q. J. Roy. Meteor. Soc., 111, 839–855, https://doi.org/10.1002/qj.49711146910, 1985.
Shuttleworth, W., Gurney, R., Hsu, A., and Ormsby, J.: FIFE: the variation in energy partition at surface flux sites, Remote Sens. Large-Scale Glob. Porc. (IAHS Publ.), 186, 67–74, 1989.
Smith, D. and Allen, S.: Measurement of sap flow in plant stems, J. Exp. Bot., 47, 1833–1844, https://doi.org/10.1093/jxb/47.12.1833, 1996.
Tian, T. and Schreiner, R.: Appropriate time to measure leaf and stem water potential in north-south oriented, vertically shoot-positioned vineyards, Am. J. Enol. Viticult., 72, 64–72, https://doi.org/10.5344/ajev.2020.20020, 2021.
Timmermans, W., Kustas, W., Anderson, M., and French, A.: An intercomparison of the Surface Energy Balance Algorithm for Land (SEBAL) and the Two-Source Energy Balance (TSEB) modeling schemes, Remote Sens. Environ., 108, 369–384, https://doi.org/10.1016/j.rse.2006.11.028, 2007.
Trezza, R.: Evapotranspiration using a satellite-based surface energy balance with standardized ground control, Doctoral dissertation, Utah State University, https://doi.org/10.26076/675a-b84b, 2002.
Tuzet, A., Perrier A., and Leuning R.: A coupled model of stomatal conductance, photosynthesis and transpiration. Plant Cell Environ., 26, 1097–1116, https://doi.org/10.1046/j.1365-3040.2003.01035.x, 2003.
Van Niel. T., McVicar T., Roderick M., Van Dijk A., Renzullo L., Van Gorsel E.: Correcting for systematic error in satellite-derived latent heat flux due to assumptions in temporal scaling: Assessment from flux tower observations, J. Hydrol., 409, 140–148, https://doi.org/10.1016/j.jhydrol.2011.08.011, 2011.
Van Niel, T., McVicar, T., Roderick, M., Van Dijk, A., Beringer, J., Hutley, L., and Van Gorsel, E.: Upscaling latent heat flux for thermal remote sensing studies: Comparison of alternative approaches and correction of bias, J. Hydrol., 468–469, 35–46, https://doi.org/10.1016/j.jhydrol.2012.08.005, 2012.
Villalobos, F., Testi, L., and Moreno-Perez, M.: Evaporation and canopy conductance of citrus orchards, Agr. Water Manage., 96, 565–573, https://doi.org/10.1016/j.agwat.2008.09.016, 2009.
Xu, T., Guo, Z., Liu, S., He, X., Meng, Y., Xu, Z., Xia, Y., Xiao, J., Zhang, Y., Ma, Y., and Song, L.: Evaluating Different Machine Learning Methods for Upscaling Evapotranspiration from Flux Towers to the Regional Scale, J. Geophys. Res.-Atmos., 123, 8674–8690, https://doi.org/10.1029/2018JD028447, 2018.
Zhang, J., Guan, K., Peng, B., Jiang, C., Zhou, W., Yang, Y., Pan, M., Franz, T., Heeren, D., Rudnick, D., Abimbola, O., Kimm, H., Caylor, K., Good, S., Khanna, M., Gates, J., and Cai, Y.: Challenges and opportunities in precision irrigation decision-support systems for center pivots, Environ. Res. Lett., 16, 053003, https://doi.org/10.1088/1748-9326/abe436, 2021.
Zhang, L. and Lemeur, R.: Evaluation of daily evapotranspiration estimates from instantaneous measurements, Agr. Forest Meteorol., 74, 139–154, https://doi.org/10.1016/0168-1923(94)02181-I, 1995.