the Creative Commons Attribution 4.0 License.
the Creative Commons Attribution 4.0 License.
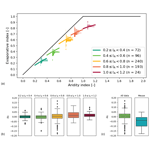
Catchment response to climatic variability: implications for root zone storage and streamflow predictions
Nienke Tempel
Laurène Bouaziz
Riccardo Taormina
Ellis van Noppen
Jasper Stam
Eric Sprokkereef
Markus Hrachowitz
This paper investigates the influence of multi-decadal climatic variability on the temporal evolution of root zone storage capacities (Sr,max) and its implications for streamflow predictions in the Meuse basin. Through a comprehensive analysis of 286 catchments across Europe and the US that are hydro-climatically comparable to the Meuse basin, we construct inter-decadal distributions of past deviations in evaporative ratios (IE) from expected values based on catchment aridity (IA). These distributions of ΔIE were then used to estimate inter-decadal changes in Sr,max and to quantify the associated consequences for streamflow predictions in the Meuse basin. Our findings reveal that, while catchments do not strictly adhere to their specific parametric Budyko curves over time, the deviations in IE are generally very minor, with an average ΔIE=0.01 and an interquartile range (IQR) of −0.01 to 0.03. Consequently, these minor deviations lead to limited inter-decadal changes in Sr,max, mostly ranging between −10 and +21 mm (−5 % to +10 %). When these changes (ΔSr,max) are accounted for in hydrological models, the impact on streamflow predictions in the Meuse basin is found to be marginal, with the most significant shifts in monthly evaporation and streamflow not exceeding 4 % and 12 %, respectively. Our study underscores the utility of parametric Budyko-style equations for first-order estimates of future Sr,max in hydrological models, even in the face of climate change and variability. This research contributes to a more nuanced understanding of hydrological responses to changing climatic conditions and offers valuable insights for future climate impact studies in hydrology.
- Article
(6749 KB) - Full-text XML
-
Supplement
(8560 KB) - BibTeX
- EndNote
Transpiration from vegetation is, on average, the largest water flux that leaves terrestrial hydrological systems (Jasechko, 2018). In spite of some uncertainty (Coenders-Gerrits et al., 2014), its magnitude is controlled by the interplay between sub-surface water supply and canopy water demand (Eagleson, 1982; Milly, 1994; Rodriguez-Iturbe et al., 2007; Donohue et al., 2007; Jaramillo et al., 2018). Both individual plants and the composition of plant communities within given spatial domains (hereafter referred to as vegetation for brevity) have, over time, adapted to local environmental and hydro-climatic conditions to ensure continuous and sufficient access to water, nutrients, and light, which has allowed their survival (Yuan et al., 2019; Ma et al., 2021). Adaptation strategies include, amongst others, the regulation of water use efficiency (e.g. Troch et al., 2009; Flo et al., 2021) or the adaptation of the extent of root systems so that roots penetrate large-enough sub-surface pore volumes for water supply to satisfy transpiration demand during dry periods (e.g. Gao et al., 2014; Fan et al., 2017). This sub-surface pore volume between field capacity and permanent wilting point defines the maximum water volume that is within the reach of roots and that is thus available for plant transpiration, hereafter referred to as root zone storage capacity Sr,max (mm). Indeed, Sr,max is a core property of terrestrial hydrological systems as it regulates, to a large extent, the partitioning of water fluxes into drainage of liquid water and, thus, eventually, streamflow Q (mm d−1), vapour released to the atmosphere as transpiration ET (mm d−1), and interception or soil evaporation EI (mm d−1) (Savenije and Hrachowitz, 2017).
At the catchment scale, Sr,max has, in the past, been quantified with three methods: firstly, by calibration as parameter of hydrological models (e.g. Fenicia et al., 2009; Coxon et al., 2020; Fowler et al., 2020; Bouaziz et al., 2021; Hanus et al., 2021; Wang et al., 2023); secondly, as product of estimates of average root depth, soil porosity, and water content at field capacity (e.g. Clark et al., 2008; Maxwell et al., 2015); and, thirdly, by following optimality principles and thus maximising variables such as net primary production, transpiration rates, or others (e.g. Kleidon, 2004; Guswa, 2008; Sivandran and Bras, 2012; Speich et al., 2020). Although all three methods mentioned above are correct in principle, insufficient data often limits their use. For example, although there are observations of root depth for several thousand individual plants worldwide (Guerrero-Ramírez et al., 2021), it is difficult to meaningfully upscale these values to plant communities with different compositions, ages, or densities. In addition, these estimates are mostly snapshots in time reflecting past conditions and, similarly to the calibration method, do not give any indication of the potential future evolution of Sr,max.
Alternatively, there is increasing evidence that Sr,max can be robustly estimated exclusively based on water balance data, i.e. long-term estimates of precipitation P (mm d−1) and actual evaporation (e.g. Donohue et al., 2012; Gentine et al., 2012; Gao et al., 2014, 2016; de Boer-Euser et al., 2016; Wang-Erlandsson et al., 2016; Dralle et al., 2021; Hrachowitz et al., 2021; McCormick et al., 2021; van Oorschot et al., 2021; Stocker et al., 2023). Under the assumption that vegetation allocates resources in an efficient way between above- and sub-surface growth (Guswa, 2008; Schymanski et al., 2008), root systems and, thus, Sr,max will not be larger than necessary to guarantee access to sufficient water during dry periods with certain return periods. The water of volume that, in the past, has been transpired during the driest periods and that can be estimated via the water balance must have been accessible to roots and therefore must reflect the magnitude of the water volume that was stored in the sub-surface and that was accessible to plants during these dry periods, i.e. Sr,max.
This approach offers the advantage that an evolution of Sr,max over time, either through natural adaptation to changing hydro-climatic conditions (e.g. Jaramillo et al., 2018) or through human interventions such as deforestation (e.g. Nijzink et al., 2016a; Hrachowitz et al., 2021) and irrigation (van Oorschot et al., 2024), is manifest in changes in dry-period transpiration ET. This offers an opportunity not only to trace the past evolution of Sr,max over time but also, together with projections of future hydro-climatic conditions, including P and EP, to quantify its potential future trajectories and the associated effects of this temporal evolution of Sr,max with regard to the hydrological response.
More specifically, estimating Sr,max from the water balance requires knowledge of EA. For past conditions, this can be robustly estimated from the water balance by assuming negligible storage change, i.e. , which is satisfied for the vast majority of catchments worldwide over timescales of around 10 years (Han et al., 2020). Climate model projections can generate, in addition to estimates of future P and potential evaporation EP (mm d−1), estimates of future EA. However, the latter are subject to major uncertainties (e.g. van Oorschot et al., 2021). As an alternative method, non-parametric formulations of the Budyko hypothesis demonstrate that the long-term partitioning of water fluxes – expressed as the evaporative index (–) – and, thus, of the hydrological response of catchments globally is, to the first order, controlled by the aridity index (Schreiber, 1904; Oldekop, 1911; Budyko and Miller, 1974). To reduce the scatter around these non-parametric Budyko-style curves and to assign catchments a unique position in the IA–IE space, parametric re-formulations such as the Tixeront–Fu equation (Tixeront, 1964; Fu, 1981; Zhang et al., 2004) and similar expressions (see Andréassian et al., 2016) were developed:
where ω (–) [1, ∞) is a catchment-specific effective parameter that aggregates all other influences on IE next to IA (Berghuijs and Woods, 2016). Higher values of ω indicate higher .
As this relationship has emerged from catchment responses and, thus, also vegetation, having adapted to past hydro-climatic conditions, expressed by IA, it is plausible to assume that the hydrological partitioning IE of a catchment will eventually adapt to a changing future IA in a corresponding way by moving along its catchment-specific curve defined by ω. This reasoning then allows us to estimate future EA based on future projections of P and EP (Roderick and Farquhar, 2011; Wang et al., 2016; Liu et al., 2020). As a consequence, the effects of a changing future EA on the future root zone storage capacity Sr,max can be quantified. In contrast to the vast majority of climate impact studies, which, in the absence of further information, assume time-invariant Sr,max even under changing future climate (e.g. Prudhomme et al., 2014; Brunner et al., 2019; Hakala et al., 2020; Rottler et al., 2021; Hanus et al., 2021), the use of such a time-variant formulation of Sr,max as parameter in hydrological models has the potential to provide more reliable predictions of the future hydrological response of catchments, as, for example, demonstrated in a recent proof-of-concept study by Bouaziz et al. (2022) for the Meuse basin in northwestern Europe. They found with model simulations that the adaptation of Sr,max to future climate conditions, expressed as IA, can cause major shifts in seasonal water supply. This involved future increases in Sr,max and, thus, increases in vegetation-accessible sub-surface water volumes, which lead to increases in summer EA of up to 15 %; these, in turn, reduced groundwater recharge, which resulted in 10 % decreases in late-summer and autumn groundwater storage, eventually causing winter flows that could be up to 20 % lower as compared to model runs that used constant values of Sr,max estimated from past hydro-climatic conditions. These findings are qualitatively consistent with the results of Speich et al. (2020), who reported significant changes in modelled streamflow when replacing a static parameter used to describe Sr,max with a forest dynamics model. More generally, Wagener et al. (2003) and Merz et al. (2011) documented the role of time-variant model parameters, including Sr,max (in their papers referred to as root constant and FC, respectively), by comparing model calibrations over multiple time windows. In a different approach, the importance of time-variable vegetation dynamics was demonstrated by Duethmann et al. (2020), who used remotely sensed vegetation indices, including the normalised difference vegetation index (NDVI), to account for temporal variations in evaporation surface resistance in the Penman equation, leading to considerably improved model skill with regard to reproducing observed river flow over multiple decades.
A major assumption underlying the approach of Bouaziz et al. (2022) is that, under changing future conditions, catchments will indeed follow their specific Budyko curve as defined by the time-invariant parameter ω, which describes the long-term average past conditions. The resulting IE is, in the following, referred to as the expected IE,exp. Several recent studies have pointed out that this assumption may not strictly hold and that ω itself may be subject to fluctuations over time (e.g. Berghuijs and Woods, 2016; Reaver et al., 2022). While of minor relevance to humid environments, IE and, thus, EA become proportionally more sensitive to fluctuations in ω with increasing aridity IA (Gudmundsson et al., 2016). As a consequence, it has to be expected that estimates of future EA and the associated Sr,max are subject to uncertainties or deviations ΔIE,exp from IE,exp that are not accounted for by Bouaziz et al. (2022).
The overall objectives of this paper are thus (1) to quantify the deviations in IE following changes in IA based on historical observations and (2) to analyse how this has, in the past, propagated further into uncertainties in time-variant estimates of Sr,max in contrasting environments over multiple decades in a large-sample approach using long-term water balance data from 286 catchments from Great Britain (GB), the US, and the Meuse basin. In a direct follow up to Bouaziz et al. (2022), who modelled the impact of a changing future climate on the hydrological response in several catchments of the Meuse basin without accounting for deviations in IE and, thus, uncertainties in Sr,max, we will, in a third step, using the same model, (3) quantify the additional effect of deviations in IE and, thus, uncertainties in Sr,max on the hydrological response in the Meuse basin and compare it to previous streamflow predictions in the Meuse basin (Bouaziz et al., 2022) that do not account for these uncertainties. Specifically, we will test the hypothesis that the inter-decadal evolution of Sr,max, reflecting vegetation adaptation to factors other than IA, which is manifest in deviations from the expected future IE,exp and thus from the associated future Sr,max, lead to significant changes in the predicted future hydrological response in the Meuse basin and needs to be accounted for in hydrological climate impact studies.
2.1 Study area
The hydrological model experiment in this study is done for the Meuse River basin upstream of Borgharen at the border between Belgium and the Netherlands (Fig. 1), which spans an area of 21 300 km2 in northwestern Europe. Largely located in the Ardennes, a rolling-hill landscape characterised by ridges and incised valleys, the elevation reaches up to around 650 m. Approximately 60 % of the basin is used for agriculture, while 30 % is covered by forests (Bouaziz et al., 2022).
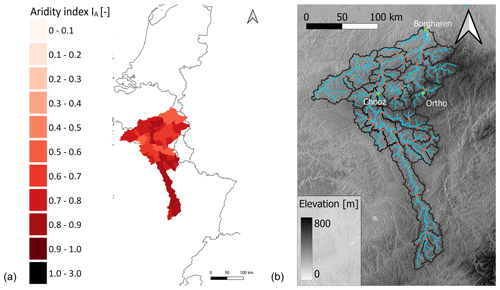
Figure 1(a) The location of the Meuse basin in northwestern Europe, where the red colour indicates the aridity index IA of the catchment; (b) the elevation range, river trajectory, and gauges in the Meuse River basin. Gauges are indicated with orange dots. The catchments of Borgharen, Ortho, and Chooz are specifically highlighted (with green) as they are separately emphasised in some of the results and analyses.
The Meuse basin is characterised by a temperate humid climate with average annual precipitation of around 920 mm yr−1, potential evaporation of around 610 mm yr−1, and streamflow of around 400 mm yr−1. The Meuse is a rain-fed river with a response time of several hours up to a few days. Transient snowpacks can be present for a few days in some parts of the basin but are overall of minor importance (Bouaziz et al., 2021). The streamflow has strong seasonality, with low summer flows and high winter flows, which are, on average, 4 times higher than the summer flow (De Wit et al., 2007). Precipitation falls relatively homogenously throughout the year, and the seasonality of the streamflow is thus mainly caused by the seasonal differences in solar-energy input and, thus, evaporation.
2.2 Data
To quantify the deviations ΔIE,exp from expected IE,exp, we adopted a large-sample strategy using long-term water balance data from catchments in contrasting environments.
Table 1Segmentation of data by 10-year periods, with the exception of the CAMELS USA, which has two periods of 9 years. Note the extra time period for the France Meuse data in comparison with the Belgium and Netherlands data.

For the Meuse river basin, daily precipitation, temperature, and radiation were obtained for the 1989–2018 period from the E-OBS v20.0 data set (Cornes et al., 2018) and were pre-processed as described by Bouaziz et al. (2022). Temperature was downscaled using a digital elevation model and a fixed temperature lapse rate, while potential evaporation was estimated using the Makkink method (Hooghart and Lablans, 1988). This method was chosen to balance the availability of the required data with their suitability for hydrological model applications (Oudin et al., 2005). Monthly bias correction was applied to address underestimation of precipitation. Daily streamflow data were available for 23 catchments within the Meuse basin from water authorities in Belgium (Service publique de Wallonie), France (Eau France), and the Netherlands (Rijkswaterstaat) for various time periods between 1989–2018 (Table 1). Note that the streamflow data at the station of Borgharen in the Netherlands are constructed by combining observations from the nearby stations St. Pieter on the Meuse and Kanne on the Albert canal (De Wit et al., 2007).
Long-term temporal changes in IE,exp and deviations ΔIE,exp therefrom that can be quantified in the Meuse basin remain limited to the 23 catchments that are gauged and streamflow records of 30 years at most. To increase the sample size in space and time and to encompass a broader range of climates, we have, in addition, included data from catchments in GB and the US, available from the CAMELS GB (Coxon et al., 2020) and CAMELS US (Addor et al., 2017) databases for the time periods indicated in Table 1. To ensure consistency, potential evaporation across all data sets was recalculated using the Makkink equation based on mean daily temperature and shortwave radiation (Hooghart and Lablans, 1988). From the full set of 671 catchments available in each of the two CAMELS databases, we excluded from the analysis those that exhibited long-term IE>IA, indicating that EA exceeds EP and, thus, the energy limit, which is an indicator of major data errors or significant unaccounted water export to adjacent catchments via, for example, groundwater exchange or irrigation water abstraction (Bouaziz et al., 2018). This does not imply that catchments retained for our analysis are not subject to data errors or unaccounted water exports. Although the effect of spurious results cannot be completely avoided, removing catchments which exhibit clear evidence of water balance deficits can at least reduce the impact of spurious effects.
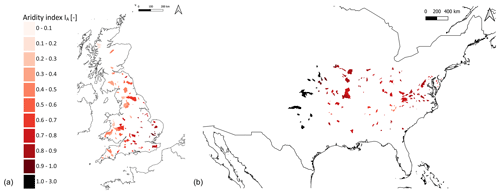
Figure 2The locations of the catchments that are provided by the large-sample data sets (a) CAMELS GB and (b) CAMELS USA. The red colour indicates the aridity index IA of the catchment.
In addition, catchments were excluded from the analysis if they did not meet the criteria of minimal human impact or if they received more than 10 % of their annual precipitation as snow. The exclusion of catchments with snowfall was necessary due to the temporary water storage capacity of snow, which can lead to inaccurate estimation of root zone storage capacity due to delayed water input (Dralle et al., 2021). This resulted in a total of 286 catchments being used for the subsequent analysis (23 – Meuse basin; 94 – CAMELS GB; 169 – CAMELS USA; Fig. 2), covering a wide range of hydro-climatic conditions, with the catchments in the Meuse basin being located at an intermediate position between the GB and US catchments (Fig. 3). Finally, the data were segmented into distinct 10-year periods (Table 1), allowing us to quantify decadal changes in IA, IE, and the associated Sr,max, as well as their decadal deviations from the expected values, i.e. ΔIE,exp and .
Following the three specific research objectives as formulated in Sect. 1, the experiment of this study is executed in several subsequent steps, shown in Fig. 4: for each of the 286 study catchments, (a) estimate IE,obs and, thus, ωobs and EA,obs from the water balance data of multiple past individual decades in the period 1989–2018 (Sect. 3.1); (b) quantify the distributions of deviations ΔIE,exp and, thus, ΔEA,exp from the expected IE,exp and EA,exp between subsequent decades (Sect. 3.1); (c) estimate from the past water balance data and, thus, from EA,obs of multiple past individual decades (Sect. 3.2); (d) quantify the distribution of deviations from the expected between subsequent decades based on ΔEA,exp (Sect. 3.2); (e) for the 23 Meuse catchments for the 2009–2018 decade, sample from the distribution (Sect. 3.2); and, finally, (f) quantify the effect of uncertainties in Sr,max on the hydrological response by using the sampled values as parameters in multiple runs and compare the results to model runs that assume and, thus, (Sect. 3.3).
3.1 Estimate IE, EA, and their deviations from expected values over time
For each of the 286 study catchments, we estimated for each individual decade i with a data record (see Table 1) the decadal average evaporation and the associated decadal average evaporative indices from the observed decadal average balance data:
Together with Pobs,i and , expressed as aridity index , we then use to solve the parametric Tixeront–Fu formulation of the Budyko hypothesis (Eq. 1) for ωobs,i for each decade for each individual catchment.
In a next step, assuming that and of the following decade i+1 are projections of an unknown future, we solve Eq. (1) for IE to “predict” the expected evaporative index of that following decade, i.e. , based on together with ωobs,i from the current decade, implying that each catchment will follow its specific curve defined by ωobs,i. The difference in the expected in relation to the actual observed then represents the deviation and, thus, for that decade i+1 for each individual catchment. The deviations for all decades of all catchments are then aggregated to an individual distribution of deviations for each of the three data sets, i.e. ΔIE,Meuse, ΔIE,GB, and ΔIE,US, and for one distribution of all three data sets combined, i.e. ΔIE. The general procedure is illustrated in Fig. 5.
3.2 Estimate root zone storage capacity Sr,max and its deviations from expected values over time
For each study catchment and each decade i with a data record we estimated the root zone storage capacity . This was done on the basis of observed decadal water balance data, as described in detail elsewhere (e.g. Nijzink et al., 2016a; Bouaziz et al., 2020; Hrachowitz et al., 2021).
Briefly, the decadal averages (Eq. 2) of each study catchment were redistributed to daily values by rescaling daily observed values of according to the following:
where t is any given day within a decade i. Note that the rescaling in Eq. (4) is based on the simplifying assumption of a constant ratio. This does not account for the effects of vegetation water stress and may cause inflated Sr,max estimates in regions with pronounced dry periods (e.g. van Oorschot et al., 2021). The catchments of the Meuse basin for which Sr,max was estimated in this study are characterised by abundant summer precipitation and rather short dry spells. The effect of a constant scaling factor on Sr,max is therefore minor.
These daily estimates of evaporation were then used together with daily observed precipitation Pobs,i(t) to compute the time series of daily cumulative storage deficits for a specific year j according to the following:
where t0 is the last preceding day on which the cumulative storage deficit . Note that the effects of interception evaporation EI on the estimation of storage deficits are negligible, as demonstrated by Bouaziz et al. (2020), and we therefore assumed that EA=ET.
The maximum annual storage deficit represents the volume of water that needs to be stored within the reach of roots to provide vegetation with continuous access to water in that year j; this is obtained as follows:
Previous studies suggested that, in a wide spectrum of environments, vegetation develops root systems that allow access to sufficient water to bridge dry spells with return periods of around 20 years (Gao et al., 2014; Nijzink et al., 2016a). The annual storage deficits of all years j in a specific decade i and catchment were therefore used to fit the generalised-extreme-value distribution. This then allowed us to estimate the storage deficit with a 20-year return period, which, here, was defined as the root zone storage capacity for that decade: . Note that, strictly seen, Sr,max is a lower limit of the magnitude of vegetation-accessible sub-surface water volumes. Based on Eqs. (2)–(6), it is an estimate of the water volume that was required in the past to meet the estimated EA (Eq. 2). In principle, the total volume of Sr,max could be higher. However, several previous studies have shown that estimating Sr,max as a parameter of a hydrological model calibrated according to observed streamflow leads to very similar values of Sr,max in many regions worldwide (e.g. Gao et al., 2014; de Boer-Euser et al., 2016; Nijzink et al., 2016a; Hrachowitz et al., 2021; Wang et al., 2024). In other words, this is evidence that models can only reproduce observed streamflow if Sr,max does indeed represent a maximum vegetation-accessible water volume and, thus, an upper limit.
In a next step, assuming that of the following decade i+1 is a projection of an unknown future, we follow the same procedure described above by Eqs. (2)–(4) but using to “predict” the expected root zone storage capacity of the following decade . The difference between the expected and the actually observed then represents the deviation for that specific catchment for decade i+1. The deviations for all decades for all catchments are then aggregated to an individual distribution of deviations for each of the three data sets, i.e. , , and .
3.3 Effect of ΔSr,max on streamflow
To isolate and quantify the effect of uncertainties ΔSr,max in predicted Sr,max on predictions of the hydrological response, we run several simulation scenarios with a process-based model for the 23 study catchments in the Meuse basin.
3.3.1 Hydrological model
The hydrological model used in this study is wflow-FLEXTopo (van Verseveld et al., 2024), a fully distributed process-based model designed to represent spatial variability in hydrological processes. The modular model uses flexible model structures for the selection of hydrological response units (HRUs), which are delineated based on topography and land use. See Fig. 6 for a schematic representation of wflow-FLEXTopo within one HRU.
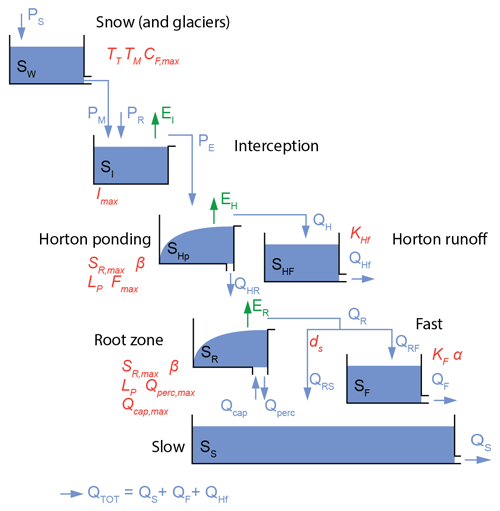
Figure 6Schematic representation of the wflow-FLEXTopo model for a single-class model including all storages and fluxes (van Verseveld et al., 2024).
Briefly, each HRU consists of several storage components linked by fluxes, similarly to comparable process-based models successfully used in previous studies (e.g. Fenicia et al., 2006; Euser et al., 2015; Gao et al., 2016; Fowler et al., 2020). Here, we have defined three HRUs that represent wetlands, hillslopes, and plateaus and which are connected through a common groundwater storage (e.g. Hulsman et al., 2021). The HRUs were delineated using the MERIT Hydro data set at 60 m × 90 m resolution (Yamazaki et al., 2019), with a threshold of 5.9 m for the height above the nearest drainage (HAND; Rennó et al., 2008) and a slope threshold of 0.13 following the methodology proposed by Gharari et al. (2011). The hillslopes are associated with forest, and the largest parts of the plateaus are used for crop cultivation agriculture in the study region, as identified using CORINE land cover data (European Environment Agency, 2018). The areal fraction of each of the three HRUs was derived for each cell at a model resolution of approximately 600 m × 900 m, similarly to the sub-grid landscape variability implemented by Nijzink et al. (2016b) in the distributed mesoscale hydrologic model (mHM). All relevant model equations are given in Table 2. Note that Horton ponding and runoff processes (Fig. 6) have minor importance in the study region and were therefore switched off for the model implementation in this study.
Table 2Water balance and flux equations used in the hydrological model, with variables: PS is snowfall (mm t−1), QM is snowmelt (mm t−1), QR is refreezing snow (mm t−1), Tthresh is melting-temperature threshold (°C), Trange is the range over which precipitation partly falls as snow and partly falls as rainfall (°C), T is air temperature (°C), QR,pot is potential snowmelt (mm t−1), sDDF is the degree-day factor (mm t−1 °C), sRF is a coefficient of refreezing (–), PR is rainfall (mm t−1), Imax is the maximum interception storage for each class (mm), EI is interception evaporation (mm t−1), PE is effective precipitation (mm t−1), LP is the threshold parameter for water stress (–), Fmax is the maximum infiltration capacity (mm t−1), Fdec is the decay coefficient (–), SR,max is the root zone storage capacity (mm), QR,direct is direct runoff (mm t−1), is net infiltration in the root zone storage (mm t−1), ER is the evaporation from the root zone storage (mm t−1), QR is runoff (mm t−1), Qperc is percolation to the slow groundwater (mm t−1), Qcap is capillary rise from the slow groundwater (mm t−1), Qperc,max is the maximum percolation parameter (mm t−1), Qcap,max is the maximum capillary rise flux parameter (mm t−1), QRF is inflow in the fast storage (mm t−1), QF is fast runoff (mm t−1), KF is the recession constant (t−1), QRS is preferential recharge from the outflow of the root zone storage (mm t−1), QS is linear outflow from the slow groundwater storage (mm t−1), KS is the recession timescale coefficient, QTOT is the total streamflow (mm t−1), and Fhrufrac is the fraction of each class in a cell (–).
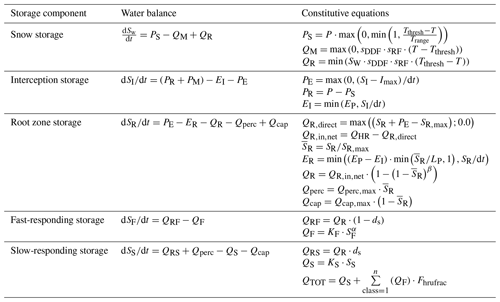
The model was previously calibrated for, at most, the downstream gauge at Borgharen (Bouaziz et al., 2022) using a multi-objective calibration strategy based on the Nash–Sutcliffe efficiencies of flows (ENS), as well as on the logarithm of flows (ENS,log), the Kling–Gupta efficiency of flows (EKG), and the monthly runoff coefficients as performance metrics (Bouaziz et al., 2022). The model was subsequently evaluated for its skill in reproducing streamflow for all of the other 22 stream gauges in the Meuse basin on the basis of the same performance metrics.
3.3.2 Scenarios
The effect of uncertainties ΔSr,max in the predicted Sr,max on the predictions of the hydrological response in the 23 study catchments was then quantified by running the calibrated model for the 2009–2018 period and replacing Sr,max with different “predictions” thereof for that period. Following the procedure used to predict Sr,max and ΔSr,max (Sect. 3.2) from IE and ΔIE (Sect. 3.1), we have, for this experiment, used the decadal period 1999–2008 (p1) as the basis to predict Sr,max and ΔSr,max for the period 2009–2018 (p2) in three scenarios.
Baseline scenario ()
We estimate of the first decade p1 based on observed data , , and of that period. Subsequently, we have used these values together with and of the second decade p2 to predict the expected and, finally, for that second period p2 in each catchment. The calibrated Sr,max values are then replaced in the model by the predicted values . Re-running the model with for period p2 then provides the baseline output of the hydrological response assuming that the catchments follow their specific Budyko curves as defined by their individual and that, therefore, and . This scenario is equivalent to the approach used by Bouaziz et al. (2022).
Scenario A ()
The catchments do not follow their specific Budyko curves. In this case, we used the combined distributions of historical deviations ΔIE from the Meuse, GB, and US data sets to determine . The predicted for decade p2 was thus estimated by sampling 100 times from the distribution of deviations ΔIE and adding the sampled values to the expected value . This sample of 100 values of then allowed us to generate a distribution of 100 values to be used in 100 model re-runs. Thus, each model run represents the effect of an error ΔIE on the estimation of . The differences in the hydrological responses with respect to the baseline scenario were then quantified (Fig. 7a). The distributions of ΔIE sampled in this scenario are based on all 286 catchments. They reflect a plausible overall distribution of ΔIE in rather cool, humid climates with comparable aseasonal precipitation distributions, similarly to the Meuse basin.
Scenario B ()
Scenario B is the same as scenario A, with the only difference being that we did not use the full distribution of ΔIE from all three data sets combined. Instead, here, we have limited the sampling distribution of deviations to ΔIE,Meuse and, thus, to historical deviations in the Meuse basin only to account for potential effects of regionally different distributions of ΔIE (Fig. 7b).
4.1 Historical IE and deviations ΔIE from expected values over time
For historical water balance observations of all three data sets, i.e. Meuse, GB, and US, the decadal IE,obs exhibits deviations ΔIE from the expected values IE,exp (Figs. 8b, d, f and 9) following decadal shifts in IA, with medians of between to 0.11 (Figs. 8a, c, e and S1–S3 in the Supplement) or % to 18.12 % in relative terms. Overall, the ΔIE remains minor, and the distributions are largely centred around zero, although they do not generally follow normal distributions, as indicated by an analysis of Q–Q plots and Shapiro–Wilk tests for normality (Shapiro and Wilk, 1965). Differences are evident, as indicated by non-parametric Wilcoxon rank sum tests that suggest significant differences (p<0.05) in the ΔIE distributions of the different data sets and different decades. The median ΔIE,GB for GB is rather stable and varies only between 0 and 0.01 with rather narrow spread, as shown by the interquartile ranges IQR ∼ 0.03. This narrow scatter around zero and the stability over time allow balanced and rather robust predictions of IE with this data set. On the other hand, the distributions ΔIE,US for the US catchments are characterised by stronger fluctuations, with medians changing from −0.01 to 0.04 between the decades, and a somewhat wider spread, with IQR ∼ 0.04.
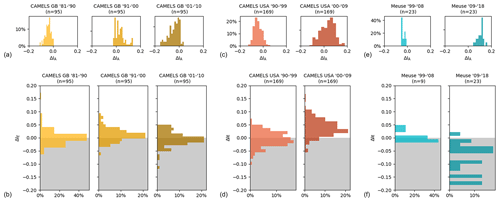
Figure 8Distributions of ΔIA per decade and per data set, CAMELS GB (a), CAMELS USA (c), and Meuse (e), and the deviations in estimating IE (ΔIE) for the different data sets of CAMELS GB (b), CAMELS USA (d), and Meuse (f).
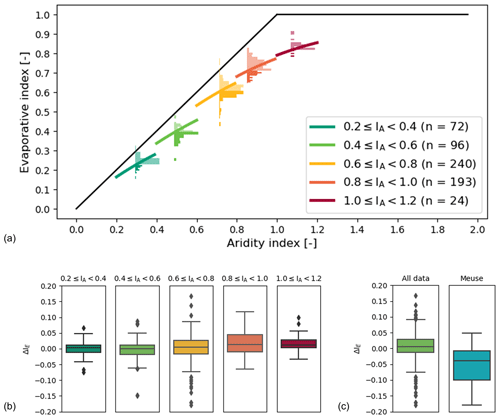
Figure 9(a) IE deviations (ΔIE) plotted per aridity index in the Budyko framework for all data sets combined, (b) distributions of IE deviations (ΔIE) corresponding to the aridity index groups from (a), (c) distributions of IE deviations (ΔIE) for all data combined and for Meuse data.
The noticeable and significant shift towards higher, i.e. more positive, ΔIE,US between these decadal distributions entails proportionally higher-than-expected evaporation for the later decade. In contrast, while, for the first decade, the catchments in Meuse basin have a median that is broadly consistent with those of the GB and the US catchments, the distribution experiences a major shift towards lower, i.e. more negative, values, with a median in the second decade, suggesting lower-than-expected proportional evaporation. However, this pattern may be an artefact of the limited sample sizes, consisting of only 9 and 23 catchments in the first and second decades, respectively, in the Meuse basin and should be interpreted with due care to avoid misinterpretations.
In the deviations ΔIE from the expected IE, no apparent geographical pattern can be distinguished through visual analysis (Fig. S4). In particular, for GB, adjacent catchments do frequently display opposing signs in ΔIE, indicating positive and negative deviations from expected IE within very close distances.
Similarly, aggregating the individual distributions of ΔIE of all three data sets and all decades into one full distribution and stratifying this distribution into individual distributions according to their aridity index IA in bins of 0.2 width (Fig. 9) also does not exhibit systematic differences between the distributions. The median of all five distributions with ΔIE=0.00–0.01 close to zero and their spreads are characterised by only minor differences, with values of IQR ∼ 0.02 for IA=0.2–0.4 and IQR ∼ 0.06 for IA=0.8–1.0. For additional context, the individual values of ΔIE for each catchment are plotted against the corresponding ΔIA, illustrating that, overall, the variability from catchment to catchment is much greater than for single catchments through time (Fig. S8).
To avoid the need to base the further analysis in the Meuse exclusively on the small sample of ΔIE,Meuse, we initially intended to construct more robust estimates of ΔIE in the Meuse by further stratifying the above according to hydro-climatic and landscape indicators. However, this further attempt to find multi-variate relationships that link ΔIE with hydro-climatic and landscape indicators did not show clear and consistent results and is not further reported on here. As an alternative, we therefore decided to use two extreme cases of distributions to sample ΔIE for the Meuse basins, i.e. scenario A and scenario B (Fig. 9c; Sect. 3.2.2), in the subsequent modelling experiment. Based on the results above, the rationale behind using scenario A is that the full distribution ΔIE describes a large sample of catchments. In the absence of a clear pattern with regard to which distribution of deviations ΔIE is more suitable for which type of environment, the full distribution, combing all data, allows a conservative perspective as it contains a wide range of historically observed ΔIE in a wide range of different environments. In addition, note that the full distribution of ΔIE from all data, with a median ΔIE=0.01 and IQR = 0.04, is very similar to the distribution associated with the IA bin 0.6–0.8, into which most of the Meuse basins fall. The use of the full ΔIE distribution in scenario A is contrasted by scenario B and its small sample distribution ΔIE,Meuse. The rationale of scenario B is to conserve potentially relevant regional information that is contained in ΔIE,Meuse and which may be under-represented in the full distribution due to the differences in the sample sizes.
4.2 Historical Sr,max and its deviations ΔSr,max from expected values over time
The general pattern of Sr,max estimated from historical water balance data following the procedure described in Sect. 3.2 is broadly consistent with previous studies (Gao et al., 2014; Wang-Erlandsson et al., 2016; de Boer-Euser et al., 2016; Stocker et al., 2023) and reflects the overall role of hydro-climatic conditions as a control in water storage in the root zone of vegetation (Fig. 10a). While, in catchments in humid regions with low IA, root zone storage capacities as low as mm are predominant, more arid regions with IA>1 are characterised by significantly higher values of mm. In other words, vegetation in humid climates has developed smaller root systems due to shorter and less frequent dry spells, which ensure more regular rainwater supply that can be directly used for transpiration. In contrast, vegetation in arid climates requires more extensive root systems to access sufficient water throughout the longer and more frequent dry spells.
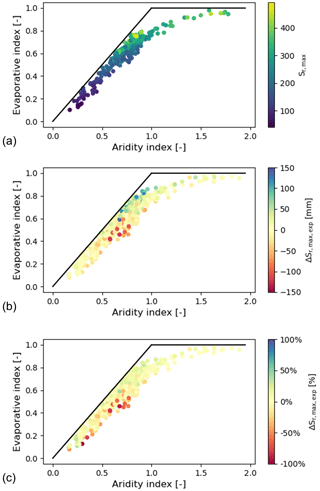
Figure 10(a) Root zone storage capacities (Sr,max) in the Budyko framework for the Meuse, CAMELS GB, and CAMELS USA catchments. Panels (b) and (c) show the error in estimating the root zone storage capacity () plotted in the Budyko framework by colour scale in absolute values (mm) and in percentages (%), respectively.
The changing hydro-climatic conditions between periods p1 and p2, expressed as changes in IA in Fig. 8, resulted in shifts in for p2. Depending on the data set, the median was between −24.9 for the Meuse data set and 13.6 mm for the US data set (Fig. 11). The deviations ΔIE (Sect. 4.1) between p1 and p2 then caused corresponding deviations from the expected . The results illustrate that the absolute magnitudes and spreads of the deviations from expected root zone storage capacities, i.e. , remain, in general, rather limited and closely centred around zero (Fig. 11), with a median mm (IQR = 19.2 mm) in GB and slightly more pronounced values of mm (IQR = 43.7 mm) in the US. The relative deviations show a similar picture, with medians of 0.8 % (IQR = 11.9 %) in GB and 4.8 % (IQR = 16.5 %) in the US. Reflecting the higher ΔIE,Meuse, , with a median of −24.9 mm, is characterised by more marked negative deviations in the Meuse basin, suggesting that the expected root zone storage capacity is overestimated and therefore smaller than expected.
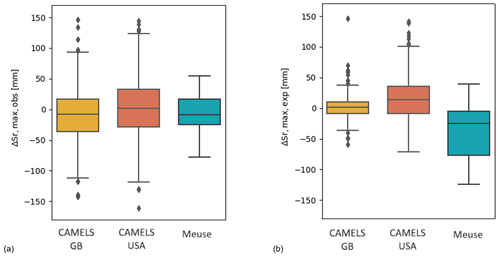
Figure 11The distributions of shifts in (a) observed root zone storage capacity and (b) expected root zone storage capacity for the different data sets.
As shown in Fig. 10, both the absolute and relative magnitudes of do not show a clear relationship with IA. Throughout all types of environments, from humid to arid, most deviations remain closely confined to the range of −25 to 25 mm or −5 % to 5 % in relative terms. The only exception is that the highest positive and negative values occur in the 0.75–1.0 aridity bin, with absolute values reaching extremes of −124 and +147 mm. However, it is noteworthy that the positive extremes in absolute changes concur with the highest magnitudes of Sr,max (Fig. 10a and b) so that the relative values of in that aridity bin are largely consistent with those in more arid and more humid environments (Fig. 10c).
4.3 Effect of ΔSr,max on streamflow predictions
4.3.1 Overall model performance
The model calibrated according to observed streamflow at the station of Borgharen at the outlet of the Meuse basin captures the main features of the hydrological response at that location. Slightly underestimating low flows and overestimating a few peaks, such as in January 2011, the model performance at Borgharen was obtained as ENS=0.85, , and EKG=0.88 (Fig. S9a). This is mirrored by the model's ability to reproduce streamflow in the remaining 22 sub-catchments (Fig. S9b and c), which largely exhibit only moderately lower performances, with medians of ENS=0.72, , and EKG=0.80 (Fig. S9d). However, for two of the catchments (Modave and Jemelle), the model could not reproduce well the hydrological response. The underlying geology of these catchments is complex, and they are likely to experience major groundwater losses which are not accounted for in this model (Bouaziz et al., 2018).
4.3.2 Changes in the hydrological response due to ΔSr,max – scenario A
Sampling from the full distribution ΔIE of all 286 catchments, as described in Sect. 3.3.2, and re-running the model for period p2 with the associated values ΔSr,max, the resulting modelled evaporation and streamflow were compared to that of the baseline scenario (ΔIE=0 and ). Overall, it was found that the modelled annual average evaporation and streamflow were affected only to a minor degree by ΔSr,max with respect to the baseline scenario, with median values of between mm yr−1 (<1 %) and mm yr−1 (−1 %) for all catchments.
However, minor but distinguishable shifts in the seasonal re-distribution of water fluxes compared to the baseline scenario could be observed. EA from early summer to early autumn increases, on average, by up to ∼0.5 mm per month, depending on the catchment. In relative terms, this is equivalent to increases of up to ∼0.6 % (Fig. 12a). More specifically, at the station of Borgharen, ΔSr,max causes the highest annual change in June, with a median ΔEA of approximately 0.4 mm per month (∼0.5 % Fig. 12b). Similar changes can be observed in other catchments (Figs. 12c and d and S10–S32). Higher summer EA due to ΔSr,max is contrasted by reduced winter streamflow, which generally reaches values of ΔQ that do not exceed mm per month ( %; Fig. 12e). At Borgharen, the most pronounced changes occur in December, with median ΔQ of approximately -0.1 mm per month ( %; Fig. 12f).
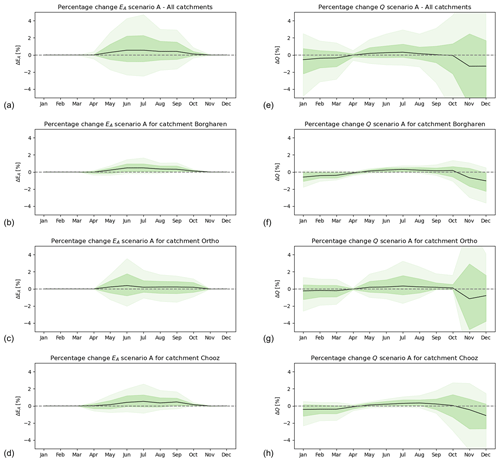
Figure 12Change in evaporation and streamflow for scenario A. The change is calculated for every run as the difference between the evaporation (a–d) or streamflow (e–h) in relation to the reference run (ΔIE=0). The outputs for all years and runs have been put together. The lightly shaded area represents the 90th and 10th percentiles, while the slightly darker shaded area represents the 25th to 75th percentiles. The black line represents the median. Images (a) and (e) display all catchments, (b) and (f) display Borgharen, (c) and (g) display Ortho, and (d) and (h) display Chooz.
Differences between the baseline scenario and scenario A also remain rather limited in terms of modelled annual maximum flow with a median ΔQmax of approximately -0.1 mm d−1 ( %) across all 23 study catchments in the Meuse basin (Fig. 13a). The most pronounced ΔQmax of approximately -0.3 mm d−1 ( %) was observed in the Le Mouzon Circourt-sur-Mouzon catchment, while, at Borgharen, mm d−1 ( %) was found (Fig. 13b). Annual minimum flows experienced only negligible overall increases of ΔQmin≪1 % caused by ΔSr,max (Fig. 13a).
4.3.3 Changes in the hydrological response due to ΔSr,max – scenario B
Alternatively, sampling from the sparse, regional distribution ΔIE,Meuse as described in Sect. 3.3.2 to estimate ΔSr,max for model re-runs provided a perspective on how more extreme, regionally confined distributions of ΔIE may affect the hydrological response. Similarly to scenario A, the modelled average annual changes of ΔEA does not exceed mm yr−1 ( %) and ΔQ does not exceed ∼44 mm yr−1 (∼12 %) compared to the baseline scenario. The effects of ΔSr,max thus remained modest across all study catchments in the Meuse basin, albeit being slightly more pronounced than for scenario A.
In contrast, major differences were detected in the modelled seasonal water fluxes. On average, catchments experienced a reduction in summer evaporation, particularly in the months June and July, with ΔEA reaching up to mm per month (−4 %), as shown in Fig. 14a. Zooming in to the selected stations of Borgharen, Ortho, and Chooz, a corresponding pattern of ΔEA can be found (Fig. 14b–d), with the most pronounced ΔEA at -0.56 mm per month (−1 %), at station La Meuse Goncourt (Fig. S29). Seasonal streamflow experienced partly considerable increases. The modelled increases ΔQ were, on average, most pronounced in late autumn and early winter across all catchments, with median increases of up to ∼3 mm per month (∼12 %) in November and ∼4 mm per month (∼8 %) in December (Fig. 14e). Similarly, at Borgharen, median increases of mm per month (∼12 %) in November, accompanied by minor decreases in the summer months, were found (Fig. 14f).
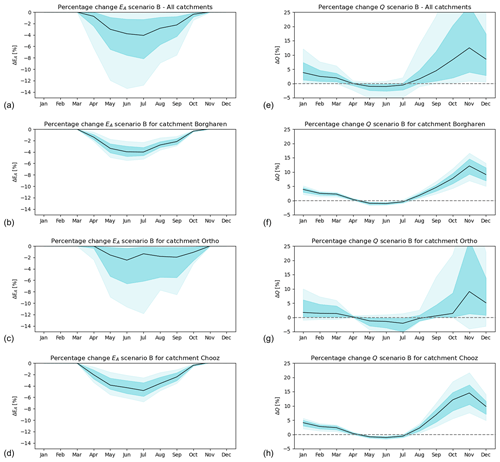
Figure 14Change in evaporation and streamflow for scenario B. The change is calculated for every run as the difference between the evaporation (a–d) or streamflow (e–h) in relation to the reference run (ΔIE=0). The outputs for all years and runs have been put together. The lightly shaded area represents the 90th and 10th percentiles, while the slightly darker shaded area represents the 25th to 75th percentiles. The black line represents the median. Images (a) and (e) display all catchments, (b) and (f) display Borgharen, (c) and (g) display Ortho, and (d) and (h) display Chooz.
The modelled annual maximum flows increased through for scenario B, with a median ΔQmax of approximately 0.35 mm d−1 (∼5 %) across all study catchments in the Meuse basin (Fig. 13a). The most pronounced ΔQmax of 1 mm d−1 (∼6 %) was observed in the La Meuse Goncourt catchment, while, at Borgharen, ΔQmax∼0.2 mm d−1 (∼5 %) was found (Fig. 13b). In spite of these partly marked increases in Qmax, the effect of ΔSr,max on annual minimum flows in scenario B remained low and comparable to that from scenario A. For all catchments, ΔQmin was found to be close to zero (Fig. 13a and b).
Parametric formulations of the Budyko hypothesis, such as the Tixeront–Fu equation (Eq. 1; Tixeront, 1964; Fu, 1981), have, in the past, been used to predict IE and, thus, future water partitioning based on changes in IA under the assumption that catchments follow their specific curves in the IA–IE space, as defined by parameter ω that is obtained from long-term historical water balance data (Roderick and Farquhar, 2011; Wang et al., 2016; Liu et al., 2020). Recently, several studies correctly observed that catchments do not necessarily follow their specific curves under changing environmental conditions, raising the concern that parametric Budyko-style equations may therefore have little predictive power (Berghuijs and Woods, 2016; Reaver et al., 2022; Jaramillo et al., 2022). The absolute decadal fluctuations to 0.11 across all data sets in our study are rather minor and closely correspond to those reported by previous studies (e.g. Jaramillo et al., 2022; Ibrahim et al., 2024), although the relative changes may be somewhat higher. Following these absolute values ΔIA, our results indeed provide further evidence that such deviations ΔIE from expected IE are a widespread phenomenon. However, our results also illustrate that, although catchments do not strictly follow their specific curves at decadal timescales, the magnitude of deviations remains, overall, rather minor, with a median of ΔIE=0.01 and an IQR = −0.01to 0.03 across all catchments in this study (Fig. 10). In spite of some differences in detail, the general distributions of ΔIE from different data sets, regions, and hydro-climatic conditions are broadly similar, and no systematic differences linked to catchment properties or hydro-climatic conditions could be identified (Figs. 9 and 10).
The root zone storage capacity Sr,max as a core property of terrestrial hydrological systems and parameters in hydrological models can, together with its evolution over time, be robustly estimated at the catchment-scale based on water balance data (Gao et al., 2014; de Boer-Euser et al., 2016; Wang-Erlandsson et al., 2016; Dralle et al., 2021; Hrachowitz et al., 2021; McCormick et al., 2021; van Oorschot et al., 2021, 2024; Stocker et al., 2023). This offers an opportunity to account for vegetation adaptation to changing hydro-climatic conditions with a time-variable parameter Sr,max for predictions with hydrological models. Bouaziz et al. (2022) were the first to demonstrate the potential of doing that in a recent proof-of-concept study. However, they estimated future Sr,max under the assumption that their catchments will strictly follow their specific curves in the IA–IE space as determined by parameter ω, which was obtained from historical water balance data. In other words, they did not account for deviations ΔSr,max that result from deviations ΔIE. In addition, the analysis of Bouaziz et al. (2022), predicting future streamflow based on projected future water balance data, remained a scenario analysis which they could not evaluate against actual observations. Sequentially addressing these knowledge gaps, here, we quantify distributions ΔIE from historical observed water balance data and used these observed past deviations ΔIE to estimate past deviations ΔSr,max, which were not accounted for by Bouaziz et al. (2022). In a first step, we found that, for the vast majority of the 286 catchments analysed in this study, characterised by a median historical Sr,max of 239.2 mm, the limited deviations ΔIE also resulted in ΔSr,max that remained narrowly confined between to 25 mm or −5 % to 10 % (Fig. 11), although some regional outliers can reach higher values.
In a second step, using samples of ΔIE from two distinct distributions in scenarios A and B, we estimated ΔSr,max for use as parameter in model simulations. Overall, it was found with the more balanced scenario A that ΔSr,max caused shifts in seasonal EA and Q; however, this was characterised by marginal magnitudes, with the most pronounced changes in ΔEA<1 % occurring, on average, in June and those in % occurring in December, with a similar pattern for the annual maximum and minimum flows, ΔQmax and ΔQmin, respectively. For scenario B, with an example of a rather extreme, regionally confined ΔIE, the deviations showed somewhat higher magnitudes, with % in July and ΔQ∼12 % in November, and a comparable pattern for ΔQmax and ΔQmin.
Notwithstanding the above, it is important to bear in mind that, as in any catchment-scale hydrological experiment, the available data may be subject to various types of uncertainties, which can be further exacerbated by decisions made in the modelling process (e.g. Beven, 2016; Nearing et al., 2016; Hrachowitz and Clark, 2017; McMillan et al., 2018), so that results have to be interpreted with due care. This is particularly true for the use of long time series of data records generated by different data providers, potentially also using changing observation methods over time and for which, in many cases, homogenisation to make them comparable is not a trivial task. In this study, the use of E-OBS precipitation data, together with streamflow data, from various data providers in Belgium, France, and the Netherlands for the Meuse basin, as well as the initial analysis of the CAMELS GB and US data sets, illustrated the presence of systematic differences in the water balances between the three individual groups of data sets. These differences could, in the preliminary analysis conducted here, largely be attributed to different methods to estimate EP. While, for the data record of the Meuse basin, the lack of more detailed consistent long-term data dictated the use of the Makkink equation based on temperature and incoming shortwave radiation, EP was estimated using the Penman–Monteith method in the CAMELS GB catchments and using the Priestley–Taylor method in the CAMELS US catchments. In an attempt to homogenise across the data sets, we therefore re-estimated EP in the GB and US catchments with the Makkink equation. Its simplicity and the exclusion of factors such as vapour pressure deficit or wind speed may have the potential to cause a certain level of uncertainty, although this has previously been shown to produce plausible estimates of EP for use in hydrological models (Oudin et al., 2005).
Another unresolved issue that emerged from our analysis is the considerable reduction in evaporation in the Meuse basin between the two study periods p1 (1999–2008) and p2 (2009–2018), as illustrated by the distribution of ΔIE that is characterised by remarkably more negative bias (Fig. 9) than in any other study catchment. The origin of this pattern is unclear, but similar anomalies in the hydrological response have previously been reported for the middle 20th century by others (Fenicia et al., 2009). They put forward the hypothesis that major decadal fluctuations in IE in the Meuse basin may have been the result of active, large-scale forest management. More specifically, forest rotation and a shift from deciduous to coniferous forest, together with an increase in average forest age towards the end of the 20th century, were hypothesised to have caused the IE fluctuations observed in the Meuse basin. While the relationship between stand age and evaporation is still under investigation (Teuling and Hoek van Dijke, 2020), there is evidence that young forests tend to evaporate more than mature forests (e.g. Vertessy et al., 2001; Brown et al., 2005). Together with Dirkse and Daamen (2004), who noted that, in the Netherlands, changes in forest management practices from clear-cutting and increased thinning resulted in a 10-year increase in the average age of trees between 1980 and 2001, specifically from 43 to 53 years; this may indeed explain at least some of the ΔIE observed in the Meuse basin, although it remains unclear why similar pattern were not observed elsewhere.
Together, the results of this study suggest that, although most catchments do not strictly follow their specific curves in the IA–IE space over time, the general magnitudes of deviations ΔIE are, in general, low enough to cause only very minor deviations ΔSr,max in predictions of root zone storage capacities. As a consequence, even under the assumption of rather exceptional ΔIE and thus ΔSr,max in scenario B, the effects on the hydrological response remain limited. This further suggests that vegetation adaptation to factors other than IA, which are manifest in the deviations ΔIE and, eventually, in ΔSr,max, does not lead to major changes in the predicted future hydrological response in the Meuse basin overall. However, it is plausible to assume that, in other regions that are characterised by stronger seasonal contrasts in liquid water supply (related to both seasonal rainfall distribution and snowmelt), similar deviations in ΔIE may lead to larger ΔSr,max and thus more pronounced effects on streamflow dynamics. Irrespective of that and in spite of not strictly following their specific curves, catchment estimates of future IE, based on changes in future IA, may therefore still be considered to be useful as first-order estimates to quantify the future evolution of parameter Sr,max in hydrological models for climate impact studies over decadal timescales.
In this study, we have quantified the cascading effects of uncertainties in decadal predictions of evaporative ratios IE as a function of changes in catchment aridity IA on predictions of root zone storage capacities Sr,max and, eventually, on predictions of streamflow. In this study in the Meuse basin, it was found that (1) when inferred from long-term data from 286 catchments in Europe and the US that are hydro-climatically similar to the Meuse basin, catchments do not strictly follow their specific curves defined by parameter ω in the IA–IE Budyko space over multiple decades, but these deviations are characterised by limited magnitudes with average ΔIE of 0.01 (0.89 %); (2) the deviations ΔIE have a minor impact on predictions of Sr,max, with the resulting deviations ΔSr,max ranging mostly between −10 to 20 mm or −5 % to 10 %; and, finally, (3) these uncertainties ΔSr,max have only a limited effect on the hydrological response in the Meuse basin because, in spite of causing shifts in the seasonal water supply, the magnitudes of these shifts in monthly EA and Q largely remain very minor (<1 %) and do not, even in the exceptional scenario B, exceed 4 % (EA) to 12 % (Q). Overall, this suggests that uncertainties in predictions of IE based on parametric Budyko-style equations and the associated uncertainties in predictions of model parameter Sr,max do not cause major uncertainties in streamflow predictions in the Meuse basin and can thus be considered to be useful first-order estimates in the absence of more detailed information.
The software for the WFLOW model (van Verseveld et al., 2024) is publicly accessible. The Python code used for the analyses in this work is available at https://doi.org/10.5281/zenodo.13969617 (Tempel, 2024).
The streamflow data for Belgian stations were provided by the Service Public de Wallonie (Service Public de Wallonie, 2018) and for French stations via the Banque Hydro portal (http://hydro.eaufrance.fr/, Banque Hydro, 2022). The E-OBS dataset (v20.0e) for daily precipitation, temperature, and radiation fields (Cornes et al., 2018) is available at https://www.ecad.eu/download/ensembles/download.php (ECA&D, 2024). Simulated historical and 2 K climate data were provided by the Royal Netherlands Meteorological Institute KNMI). All of the above-mentioned data were preprocessed and provided by Bouaziz et al. (2022). In addition, large-sample datasets, CAMELS GB (Coxon et al., 2020) and CAMELS US (Addor et al., 2017), were also used.
The supplement related to this article is available online at: https://doi.org/10.5194/hess-28-4577-2024-supplement.
MH, NT and LB designed the study. NT, LB and EvN performed the experiments. All the authors contributed to the discussion and writing of the manuscript.
At least one of the (co-)authors is a member of the editorial board of Hydrology and Earth System Sciences. The peer-review process was guided by an independent editor, and the authors also have no other competing interests to declare.
Publisher's note: Copernicus Publications remains neutral with regard to jurisdictional claims made in the text, published maps, institutional affiliations, or any other geographical representation in this paper. While Copernicus Publications makes every effort to include appropriate place names, the final responsibility lies with the authors.
The authors greatly appreciate the constructive and detailed comments by Andrew Guswa and two anonymous reviewers. This research was part of the CATAPUC II project funded by the Dutch Ministry of Infrastructure and Water Management (Rijkswaterstaat).
This paper was edited by Nunzio Romano and reviewed by Andrew Guswa and two anonymous referees.
Addor, N., Newman, A. J., Mizukami, N., and Clark, M. P.: The CAMELS data set: catchment attributes and meteorology for large-sample studies, Hydrol. Earth Syst. Sci., 21, 5293–5313, https://doi.org/10.5194/hess-21-5293-2017, 2017.
Andréassian, V., Mander, Ü., and Pae, T.: The Budyko hypothesis before Budyko: the hydrological legacy of Evald Oldekop, J. Hydrol., 535, 386–391, 2016.
Berghuijs, W. R. and Woods, R. A.: A simple framework to quantitatively describe monthly precipitation and temperature climatology, Int. J. Climatol., 36, 3161–3174, 2016a.
Berghuijs, W. R. and Woods, R. A.: Correspondence: Space-time asymmetry undermines water yield assessment, Nat. Commun., 7, 11603, https://doi.org/10.1038/ncomms11603, 2016b.
Beven, K.: Facets of uncertainty: epistemic uncertainty, non-stationarity, likelihood, hypothesis testing, and communication, Hydrolog. Sci. J., 61, 1652–1665, 2016.
Bouaziz, L., Weerts, A., Schellekens, J., Sprokkereef, E., Stam, J., Savenije, H., and Hrachowitz, M.: Redressing the balance: quantifying net intercatchment groundwater flows, Hydrol. Earth Syst. Sci., 22, 6415–6434, https://doi.org/10.5194/hess-22-6415-2018, 2018.
Bouaziz, L. J., Steele-Dunne, S. C., Schellekens, J., Weerts, A. H., Stam, J., Sprokkereef, E., and Hrachowitz, M.: Improved understanding of the link between catchment-scale vegetation accessible storage and satellite-derived Soil Water Index, Water Resour. Res., 56, e2019WR026365, https://doi.org/10.1029/2019WR026365, 2020.
Bouaziz, L. J. E., Fenicia, F., Thirel, G., de Boer-Euser, T., Buitink, J., Brauer, C. C., De Niel, J., Dewals, B. J., Drogue, G., Grelier, B., Melsen, L. A., Moustakas, S., Nossent, J., Pereira, F., Sprokkereef, E., Stam, J., Weerts, A. H., Willems, P., Savenije, H. H. G., and Hrachowitz, M.: Behind the scenes of streamflow model performance, Hydrol. Earth Syst. Sci., 25, 1069–1095, https://doi.org/10.5194/hess-25-1069-2021, 2021.
Bouaziz, L. J. E., Aalbers, E. E., Weerts, A. H., Hegnauer, M., Buiteveld, H., Lammersen, R., Stam, J., Sprokkereef, E., Savenije, H. H. G., and Hrachowitz, M.: Ecosystem adaptation to climate change: the sensitivity of hydrological predictions to time-dynamic model parameters, Hydrol. Earth Syst. Sci., 26, 1295–1318, https://doi.org/10.5194/hess-26-1295-2022, 2022.
Brown, A. E., Zhang, L., McMahon, T. A., Western, A. W., and Vertessy, R. A.: A review of paired catchment studies for determining changes in water yield resulting from alterations in vegetation, J. Hydrol., 310, 28–61, 2005.
Brunner, M. I., Farinotti, D., Zekollari, H., Huss, M., and Zappa, M.: Future shifts in extreme flow regimes in Alpine regions, Hydrol. Earth Syst. Sci., 23, 4471–4489, https://doi.org/10.5194/hess-23-4471-2019, 2019.
Budyko, M. I. and Miller, D. H.: Climate and life, Academic Press, New York, 508 pp., 1974.
Coenders-Gerrits, A. M. J., Van der Ent, R. J., Bogaard, T. A., Wang-Erlandsson, L., Hrachowitz, M., and Savenije, H. H. G.: Uncertainties in transpiration estimates, Nature, 506, E1–E2, 2014.
Cornes, R. C., van der Schrier, G., van den Besselaar, E. J., and Jones, P. D.: An ensemble version of the E-OBS temperature and precipitation data sets, J. Geophys. Res.-Atmos., 123, 9391–9409, 2018.
Coxon, G., Addor, N., Bloomfield, J. P., Freer, J., Fry, M., Hannaford, J., Howden, N. J. K., Lane, R., Lewis, M., Robinson, E. L., Wagener, T., and Woods, R.: CAMELS-GB: hydrometeorological time series and landscape attributes for 671 catchments in Great Britain, Earth Syst. Sci. Data, 12, 2459–2483, https://doi.org/10.5194/essd-12-2459-2020, 2020.
Clark, M. P., Rupp, D. E., Woods, R. A., Zheng, X., Ibbitt, R. P., Slater, A. G., and Uddstrom, M. J.: Hydrological data assimilation with the ensemble Kalman filter: Use of streamflow observations to update states in a distributed hydrological model, Adv. Water Resour., 31, 1309–1324, 2008.
de Boer-Euser, T., McMillan, H. K., Hrachowitz, M., Winsemius, H. C., and Savenije, H. H.: Influence of soil and climate on root zone storage capacity, Water Resour. Res., 52, 2009–2024, 2016.
De Wit, M. J., Van Den Hurk, B. J., Warmerdam, P. M., Torfs, P. J., Roulin, E., and Van Deursen, W. P.: Impact of climate change on low-flows in the river Meuse, Climatic Change, 82, 351–372, 2007.
Dirkse, G. M. and Daamen, W. P.: Dutch forest monitoring network, design and results, Commun. Ecol., 5, 115–120, 2004.
Donohue, R. J., Roderick, M. L., and McVicar, T. R.: On the importance of including vegetation dynamics in Budyko's hydrological model, Hydrol. Earth Syst. Sci., 11, 983–995, https://doi.org/10.5194/hess-11-983-2007, 2007.
Donohue, R. J., Roderick, M. L., and McVicar, T. R.: Roots, storms and soil pores: Incorporating key ecohydrological processes into Budyko's hydrological model, J. Hydrol., 436, 35–50, 2012.
Dralle, D. N., Hahm, W. J., Chadwick, K. D., McCormick, E., and Rempe, D. M.: Technical note: Accounting for snow in the estimation of root zone water storage capacity from precipitation and evapotranspiration fluxes, Hydrol. Earth Syst. Sci., 25, 2861–2867, https://doi.org/10.5194/hess-25-2861-2021, 2021.
Duethmann, D., Blöschl, G., and Parajka, J.: Why does a conceptual hydrological model fail to correctly predict discharge changes in response to climate change?, Hydrol. Earth Syst. Sci., 24, 3493–3511, https://doi.org/10.5194/hess-24-3493-2020, 2020.
Eagleson, P. S.: Ecological optimality in water-limited natural soil-vegetation systems: 1. Theory and hypothesis, Water Resour. Res., 18, 325–340, 1982.
ECA&D: E-OBS gridded dataset, ECA&D [data set], https://www.ecad.eu/download/ensembles/download.php (last access: 22 October 2024), 2024.
European Environment Agency: Corine Land Cover (CLC) 2018, Version 2020 20u1, European Environment Agency (EEA) under the framework of the Copernicus programme, http://land.copernicus.eu/pan-european/corine-land-cover/ (last access: 7 June 2019), 2018.
Euser, T., Hrachowitz, M., Winsemius, H. C., and Savenije, H. H.: The effect of forcing and landscape distribution on performance and consistency of model structures, Hydrol. Process., 29, 3727–3743, 2015.
Fan, Y., Miguez-Macho, G., Jobbágy, E. G., Jackson, R. B., and Otero-Casal, C.: Hydrologic regulation of plant rooting depth, P. Natl. Acad. Sci. USA, 114, 10572–10577, 2017.
Fenicia, F., Savenije, H. H. G., Matgen, P., and Pfister, L.: Is the groundwater reservoir linear? Learning from data in hydrological modelling, Hydrol. Earth Syst. Sci., 10, 139–150, https://doi.org/10.5194/hess-10-139-2006, 2006.
Fenicia, F., Savenije, H. H. G., and Avdeeva, Y.: Anomaly in the rainfall-runoff behaviour of the Meuse catchment. Climate, land-use, or land-use management?, Hydrol. Earth Syst. Sci., 13, 1727–1737, https://doi.org/10.5194/hess-13-1727-2009, 2009.
Flo, V., Martínez-Vilalta, J., Mencuccini, M., Granda, V., Anderegg, W. R., and Poyatos, R.: Climate and functional traits jointly mediate tree water-use strategies, New Phytol., 231, 617–630, 2021.
Fowler, K., Knoben, W., Peel, M., Peterson, T., Ryu, D., Saft, M., and Western, A.: Many commonly used rainfall-runoff models lack long, slow dynamics: Implications for runoff projections, Water Resour. Res., 56, e2019WR025286, https://doi.org/10.1029/2019WR025286, 2020.
Fu, B. P.: On the calculation of the evaporation from land surface, Sci. Atmos. Sin., 5, 23–31, 1981.
Gao, H., Hrachowitz, M., Schymanski, S. J., Fenicia, F., Sriwongsitanon, N., and Savenije, H. H.: Climate controls how ecosystems size the root zone storage capacity at catchment scale, Geophys. Res. Lett., 41, 7916–7923, 2014.
Gao, H., Hrachowitz, M., Sriwongsitanon, N., Fenicia, F., Gharari, S., and Savenije, H. H.: Accounting for the influence of vegetation and landscape improves model transferability in a tropical savannah region, Water Resour. Res., 52, 7999–8022, 2016.
Gentine, P., D'Odorico, P., Lintner, B. R., Sivandran, G., and Salvucci, G.: Interdependence of climate, soil, and vegetation as constrained by the Budyko curve, Geophys. Res. Lett., 39, 2–7, https://doi.org/10.1029/2012GL053492,, 2012.
Gharari, S., Hrachowitz, M., Fenicia, F., and Savenije, H. H. G.: Hydrological landscape classification: investigating the performance of HAND based landscape classifications in a central European meso-scale catchment, Hydrol. Earth Syst. Sci., 15, 3275–3291, https://doi.org/10.5194/hess-15-3275-2011, 2011.
Gudmundsson, L., Greve, P., and Seneviratne, S. I.: The sensitivity of water availability to changes in the aridity index and other factors – A probabilistic analysis in the Budyko space, Geophys. Res. Lett., 43, 6985–6994, 2016.
Guerrero-Ramírez, N. R., Mommer, L., Freschet, G. T., Iversen, C. M., McCormack, M. L., Kattge, J., and Weigelt, A.: Global root traits (GRooT) database, Global Ecol. Biogeogr., 30, 25–37, 2021.
Guswa, A. J.: The influence of climate on root depth: A carbon cost-benefit analysis, Water Resour. Res., 44, W02427, https://doi.org/10.1029/2007WR006384, 2008.
Hakala, K., Addor, N., Gobbe, T., Ruffieux, J., and Seibert, J.: Risks and opportunities for a Swiss hydroelectricity company in a changing climate, Hydrol. Earth Syst. Sci., 24, 3815–3833, https://doi.org/10.5194/hess-24-3815-2020, 2020.
Han, J., Yang, Y., Roderick, M. L., McVicar, T. R., Yang, D., Zhang, S., and Beck, H. E.: Assessing the steady-state assumption in water balance calculation across global catchments, Water Resour. Res., 56, e2020WR027392, https://doi.org/10.1029/2020WR027392, 2020.
Hanus, S., Hrachowitz, M., Zekollari, H., Schoups, G., Vizcaino, M., and Kaitna, R.: Future changes in annual, seasonal and monthly runoff signatures in contrasting Alpine catchments in Austria, Hydrol. Earth Syst. Sci., 25, 3429–3453, https://doi.org/10.5194/hess-25-3429-2021, 2021.
Hooghart, J. and Lablans, W.: Van Penman naar Makkink: een nieuwe berekeningswijze voor de klimatologische verdampingsgetallen, De Bilt, KNMI – Royal Netherlands Meteorological Institute, De Bilt, the Netherlands, 1988.
Hrachowitz, M. and Clark, M. P.: HESS Opinions: The complementary merits of competing modelling philosophies in hydrology, Hydrol. Earth Syst. Sci., 21, 3953–3973, https://doi.org/10.5194/hess-21-3953-2017, 2017.
Hrachowitz, M., Stockinger, M., Coenders-Gerrits, M., van der Ent, R., Bogena, H., Lücke, A., and Stumpp, C.: Reduction of vegetation-accessible water storage capacity after deforestation affects catchment travel time distributions and increases young water fractions in a headwater catchment, Hydrol. Earth Syst. Sci., 25, 4887–4915, https://doi.org/10.5194/hess-25-4887-2021, 2021.
Hulsman, P., Hrachowitz, M., and Savenije, H. H.: Improving the representation of long-term storage variations with conceptual hydrological models in data-scarce regions, Water Resour. Res., 57, e2020WR028837, https://doi.org/10.1029/2020WR028837, 2021.
Ibrahim, M., Coenders-Gerrits, M., van der Ent, R., and Hrachowitz, M.: Catchments do not strictly follow Budyko curves over multiple decades but deviations are minor and predictable, Hydrol. Earth Syst. Sci. Discuss. [preprint], https://doi.org/10.5194/hess-2024-120, in review, 2024.
Jaramillo, F., Cory, N., Arheimer, B., Laudon, H., van der Velde, Y., Hasper, T. B., Teutschbein, C., and Uddling, J.: Dominant effect of increasing forest biomass on evapotranspiration: interpretations of movement in Budyko space, Hydrol. Earth Syst. Sci., 22, 567–580, https://doi.org/10.5194/hess-22-567-2018, 2018.
Jaramillo, F., Piemontese, L., Berghuijs, W. R., Wang-Erlandsson, L., Greve, P., and Wang, Z.: Fewer basins will follow their Budyko curves under global warming and fossil-fueled development, Water Resour. Res., 58, e2021WR031825, https://doi.org/10.1029/2021WR031825, 2022.
Jasechko, S.: Plants turn on the tap, Nat. Clim. Change, 8, 562–563, 2018.
Kleidon, A.: Global datasets of rooting zone depth inferred from inverse methods, J. Climate, 17, 2714–2722, 2004.
Liu, Z., Cheng, L., Zhou, G., Chen, X., Lin, K., Zhang, W., and Zhou, P.: Global response of evapotranspiration ratio to climate conditions and watershed characteristics in a changing environment, J. Geophys. Res.-Atmos., 125, e2020JD032371, https://doi.org/10.1029/2020JD032371, 2020.
Ma, H., Mo, L., Crowther, T. W., Maynard, D. S., van den Hoogen, J., Stocker, B. D., and Zohner, C. M.: The global distribution and environmental drivers of aboveground versus belowground plant biomass, Nat. Ecol. Evol., 5, 1110–1122, 2021.
Maxwell, R. M., Condon, L. E., and Kollet, S. J.: A high-resolution simulation of groundwater and surface water over most of the continental US with the integrated hydrologic model ParFlow v3, Geosci. Model Dev., 8, 923–937, https://doi.org/10.5194/gmd-8-923-2015, 2015.
McCormick, E. L., Dralle, D. N., Hahm, W. J., Tune, A. K., Schmidt, L. M., Chadwick, K. D., and Rempe, D. M.: Widespread woody plant use of water stored in bedrock, Nature, 597, 225–229, 2021.
McMillan, H. K., Westerberg, I. K., and Krueger, T.: Hydrological data uncertainty and its implications, Wiley Interdisciplin. Rev.: Water, 5, e1319, https://doi.org/0.1002/wat2.1319, 2018.
Merz, R., Parajka, J., and Blöschl, G.: Time stability of catchment model parameters: Implications for climate impact analyses, Water Resour. Res., 47, 1–17, https://doi.org/10.1029/2010WR009505, 2011.
Milly, P. C. D.: Climate, interseasonal storage of soil water, and the annual water balance, Adv. Water Resour., 17, 19–24, 1994.
Nearing, G. S., Tian, Y., Gupta, H. V., Clark, M. P., Harrison, K. W., and Weijs, S. V.: A philosophical basis for hydrological uncertainty, Hydrolog. Sci. J., 61, 1666–1678, 2016.
Nijzink, R., Hutton, C., Pechlivanidis, I., Capell, R., Arheimer, B., Freer, J., Han, D., Wagener, T., McGuire, K., Savenije, H., and Hrachowitz, M.: The evolution of root-zone moisture capacities after deforestation: a step towards hydrological predictions under change?, Hydrol. Earth Syst. Sci., 20, 4775–4799, https://doi.org/10.5194/hess-20-4775-2016, 2016a.
Nijzink, R. C., Samaniego, L., Mai, J., Kumar, R., Thober, S., Zink, M., Schäfer, D., Savenije, H. H. G., and Hrachowitz, M.: The importance of topography-controlled sub-grid process heterogeneity and semi-quantitative prior constraints in distributed hydrological models, Hydrol. Earth Syst. Sci., 20, 1151–1176, https://doi.org/10.5194/hess-20-1151-2016, 2016b.
Oldekop, E.: Collection of the Works of Students of the Meteorological Observatory, University of TartuJurjew-Dorpat Tartu, Estonia, p. 209, 1911.
Oudin, L., Hervieu, F., Michel, C., Perrin, C., Andréassian, V., Anctil, F., and Loumagne, C.: Which potential evapotranspiration input for a lumped rainfall–runoff model: Part 2 – Towards a simple and efficient potential evapotranspiration model for rainfall–runoff modelling, J. Hydrol., 303, 290–306, 2005.
Prudhomme, C., Giuntoli, I., Robinson, E. L., Clark, D. B., Arnell, N. W., Dankers, R., and Wisser, D.: Hydrological droughts in the 21st century, hotspots and uncertainties from a global multimodel ensemble experiment, P. Natl. Acad. Sci. USA, 111, 3262–3267, 2014.
Reaver, N. G. F., Kaplan, D. A., Klammler, H., and Jawitz, J. W.: Theoretical and empirical evidence against the Budyko catchment trajectory conjecture, Hydrol. Earth Syst. Sci., 26, 1507–1525, https://doi.org/10.5194/hess-26-1507-2022, 2022.
Rennó, C. D., Nobre, A. D., Cuartas, L. A., Soares, J. V., Hodnett, M. G., and Tomasella, J.: HAND, a new terrain descriptor using SRTM-DEM: Mapping terra-firme rainforest environments in Amazonia, Remote Sens. Environ., 112, 3469–3481, 2008.
Roderick, M. L. and Farquhar, G. D.: A simple framework for relating variations in runoff to variations in climatic conditions and catchment properties, Water Resour. Res., 47, W00G07, https://doi.org/10.1029/2010WR009826, 2011.
Rodriguez-Iturbe, I., D'Odorico, P., Laio, F., Ridolfi, L., and Tamea, S.: Challenges in humid land ecohydrology: Interactions of water table and unsaturated zone with climate, soil, and vegetation, Water Resour. Res., 43, W09301, https://doi.org/10.1029/2007WR006073, 2007.
Rottler, E., Bronstert, A., Bürger, G., and Rakovec, O.: Projected changes in Rhine River flood seasonality under global warming, Hydrol. Earth Syst. Sci., 25, 2353–2371, https://doi.org/10.5194/hess-25-2353-2021, 2021.
Savenije, H. H. G. and Hrachowitz, M.: HESS Opinions “Catchments as meta-organisms – a new blueprint for hydrological modelling”, Hydrol. Earth Syst. Sci., 21, 1107–1116, https://doi.org/10.5194/hess-21-1107-2017, 2017.
Schreiber, P.: Über die Beziehungen zwischen dem Niederschlag und der Wasserführung der Flüsse in Mitteleuropa, Z. Meteorol., 21, 441–452, 1904.
Schymanski, S. J., Sivapalan, M., Roderick, M. L., Beringer, J., and Hutley, L. B.: An optimality-based model of the coupled soil moisture and root dynamics, Hydrol. Earth Syst. Sci., 12, 913–932, https://doi.org/10.5194/hess-12-913-2008, 2008.
Service Public de Wallonie: Direction générale opérationnelle de la Mobilité et des Voies hydrauliques, Département des Etudes et de l'Appui à la Gestion, Direction de la Gestion hydrologique, Rapport annuel 2018, Tech. rep., Service Public de Wallonie, Namur, Belgium, https://doi.org/10.1029/2012WR012055, 2018.
Shapiro, S. S. and Wilk, M. B.: An analysis of variance test for normality (complete samples), Biometrika, 52, 591–611, 1965.
Sivandran, G. and Bras, R. L.: Identifying the optimal spatially and temporally invariant root distribution for a semiarid environment, Water Resour. Res., 48, W12525, https://doi.org/10.1029/2012WR012055, 2012.
Speich, M. J. R., Zappa, M., Scherstjanoi, M., and Lischke, H.: FORests and HYdrology under Climate Change in Switzerland v1.0: a spatially distributed model combining hydrology and forest dynamics, Geosci. Model Dev., 13, 537–564, https://doi.org/10.5194/gmd-13-537-2020, 2020.
Stocker, B. D., Tumber-Dávila, S. J., Konings, A. G., Anderson, M. C., Hain, C., and Jackson, R. B.: Global patterns of water storage in the rooting zones of vegetation, Nat. Geosci., 16, 250–256, 2023.
Tempel, N.: Paper-Catchment-Response, Zenodo [code], https://doi.org/10.5281/zenodo.13969617, 2024.
Teuling, A. J. and Hoek van Dijke, A. J.: Forest age and water yield, Nature, 578, E16–E18, 2020.
Tixeront, J.: Prévision des apports des cours d'eau, Publ. Assoc. Int. Hydrol. Sci., 63, 118–126, 1964.
Troch, P. A., Martinez, G. F., Pauwels, V. R., Durcik, M., Sivapalan, M., Harman, C., and Huxman, T.: Climate and vegetation water use efficiency at catchment scales, Hydrol. Process., 23, 2409, https://doi.org/10.1002/hyp.7358, 2009.
van Oorschot, F., van der Ent, R. J., Hrachowitz, M., and Alessandri, A.: Climate-controlled root zone parameters show potential to improve water flux simulations by land surface models, Earth Syst. Dynam., 12, 725–743, https://doi.org/10.5194/esd-12-725-2021, 2021.
van Oorschot, F., van der Ent, R. J., Alessandri, A., and Hrachowitz, M.: Influence of irrigation on root zone storage capacity estimation, Hydrol. Earth Syst. Sci., 28, 2313–2328, https://doi.org/10.5194/hess-28-2313-2024, 2024.
van Verseveld, W. J., Weerts, A. H., Visser, M., Buitink, J., Imhoff, R. O., Boisgontier, H., Bouaziz, L., Eilander, D., Hegnauer, M., ten Velden, C., and Russell, B.: Wflow_sbm v0.7.3, a spatially distributed hydrological model: from global data to local applications, Geosci. Model Dev., 17, 3199–3234, https://doi.org/10.5194/gmd-17-3199-2024, 2024.
Vertessy, R. A., Watson, F. G., and Sharon, K. O.: Factors determining relations between stand age and catchment water balance in mountain ash forests, Forest. Ecol. Manage., 143, 13–26, 2001.
Wagener, T., McIntyre, N., Lees, M. J., Wheater, H. S., and Gupta, H. V.: Towards reduced uncertainty in conceptual rainfall-runoff modelling: Dynamic identifiability analysis, Hydrol. Process., 17, 455–476, 2003.
Wang, S., Hrachowitz, M., Schoups, G., and Stumpp, C.: Stable water isotopes and tritium tracers tell the same tale: no evidence for underestimation of catchment transit times inferred by stable isotopes in StorAge Selection (SAS)-function models, Hydrol. Earth Syst. Sci., 27, 3083–3114, https://doi.org/10.5194/hess-27-3083-2023, 2023.
Wang, S., Hrachowitz, M., and Schoups, G.: Multi-decadal fluctuations in root zone storage capacity through vegetation adaptation to hydro-climatic variability have minor effects on the hydrological response in the Neckar River basin, Germany, Hydrol. Earth Syst. Sci., 28, 4011–4033, https://doi.org/10.5194/hess-28-4011-2024, 2024.
Wang, W., Zou, S., Shao, Q., Xing, W., Chen, X., Jiao, X., and Yu, Z.: The analytical derivation of multiple elasticities of runoff to climate change and catchment characteristics alteration, J. Hydrol., 541, 1042–1056, 2016.
Wang-Erlandsson, L., Bastiaanssen, W. G. M., Gao, H., Jägermeyr, J., Senay, G. B., van Dijk, A. I. J. M., Guerschman, J. P., Keys, P. W., Gordon, L. J., and Savenije, H. H. G.: Global root zone storage capacity from satellite-based evaporation, Hydrol. Earth Syst. Sci., 20, 1459–1481, https://doi.org/10.5194/hess-20-1459-2016, 2016.
Yamazaki, D., Ikeshima, D., Sosa, J., Bates, P. D., Allen, G. H., and Pavelsky, T. M.: MERIT Hydro: a high-resolution global hydrography map based on latest topography dataset, Water Resour. Res., 55, 5053–5073, 2019.
Yuan, W., Zheng, Y., Piao, S., Ciais, P., Lombardozzi, D., Wang, Y., and Yang, S.: Increased atmospheric vapor pressure deficit reduces global vegetation growth, Sci. Adv., 5, eaax1396, https://doi.org/10.1126/sciadv.aax1396, 2019.
Zhang, L., Hickel, K., Dawes, W. R., Chiew, F. H., Western, A. W., and Briggs, P. R.: A rational function approach for estimating mean annual evapotranspiration, Water Resour. Res., 40, W02502, https://doi.org/10.1029/2003WR002710, 2004.