the Creative Commons Attribution 4.0 License.
the Creative Commons Attribution 4.0 License.
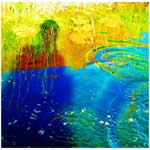
Making a case for power-sensitive water modelling: a literature review
Rozemarijn ter Horst
Rossella Alba
Jeroen Vos
Maria Rusca
Jonatan Godinez-Madrigal
Lucie V. Babel
Gert Jan Veldwisch
Jean-Philippe Venot
Bruno Bonté
David W. Walker
Tobias Krueger
Models are widely used to research hydrological change and risk. However, the power embedded in the modelling process and outcomes is often concealed by claiming their neutrality. Our review shows that in the scientific literature relatively little attention is given to the influence of models on development processes and outcomes in water governance. At the same time, an emerging body of work offering critical insights into the political implications of hydrological models and a nuanced understanding of their application in context has begun to flourish. Drawing on this work, we call for power-sensitive modelling which includes the following considerations: take a holistic approach to modelling beyond programming and coding; foster accountability; work towards just and equitable water distributions; be transparent about the expectations and choices made; and democratise modelling by giving space to and being mindful of representations of multiple bodies of knowledge and multiple stakeholders and by incorporating marginalised people and nature into the modelling process. Our call should not be understood as a suggestion to do away with modelling altogether, but rather as an invitation to interrogate how quantitative models may help to foster transformative pathways towards more just and equitable water distributions.
- Article
(676 KB) - Full-text XML
-
Supplement
(1211 KB) - BibTeX
- EndNote
Water flows and storages are increasingly researched and governed through quantitative (hydrological, hydrodynamic, socio-hydrological, and hydro-economic) models. These models are used with different purposes, including documenting water distribution, exploring causal dynamics, simulating changes, predicting future conditions, and informing policy-making. Far from being neutral tools, models are shaped by policy projects, institutional backgrounds, specific traditions and practices of modellers, and gendered relations and experiences (Sismondo, 1999; Knorr-Cetina, 1999; Lane, 2012; MacKenzie, 2006; Melsen et al., 2018a; Addor and Melsen, 2019). Since models are complex and the places and people that develop a model may be disconnected from the places and people that use the model, unravelling how and why a model functions, and with what influence, is complicated (Kouw, 2016). However, we argue in this paper that this complexity is an often-missed piece of the puzzle in model commission and development, and consciously engaging with it can help to improve the models' fit for purpose or support a modelling process that contributes towards more just and equitable water distributions.
Models are not neutral, and those who commission and develop models do have choices in whether modelling should be done and how. The hydrological modelling community is well aware that any one model could have turned out differently with different assumptions, simplifications, or data and if different people had developed it. An iconic example is the study by Holländer et al. (2014), in which 10 research teams were presented with increasing amounts of data from an artificially constructed catchment in order to model runoff from rainfall, leading to results varying initially by 2 orders of magnitude. Reflections on modelling as a social practice and the political consequences of models in the hydrological community have been primarily in terms of how a model could be considered fit for purpose and in terms of model adequacy, uncertainty, and subjectivity (Krueger and Alba, 2022).
Beven (2019) distinguished between two kinds of purpose: accurate representation of hydrological processes and mere forecasting of hydrological variables. The latter does not necessarily require any process understanding to develop output, as recently shown for instance with the resurgence of machine learning in hydrology (Nearing et al., 2021). However, Beven (2019) argued that an accurate process representation is needed if models are to be used for decision-making. Addor and Melsen (2019) and Melsen (2022) showed that institutional factors play a greater role in modellers choosing models than model adequacy in the sense of fitness for purpose. The question of model adequacy began to gain an overtly political connotation when Beven (2019) and Hamilton et al. (2022) considered the possibility of policy-makers or stakeholders being involved in assessing whether a model is fit for purpose. Further developing this point, we would add that the developments (including increasing model complexity, attention for uncertainty, fitness for purpose, and involvement of stakeholders) will bring to the fore ever more clearly the political nature of models as something to utilise and as something to challenge.
One pitfall could be that discussions remain disconnected from the context the models are used in, while this could improve the modelling practice itself. Naturally, the discussions described above take the model as their starting and end points, as the aim is to improve models, but the challenge will be to step out of model land (Thompson and Smith, 2019). Since hydrological science is inherently bound to societal needs (Lane, 2014), being more explicit about the political influence of models is relevant not only from a science studies perspective, but also for hydrology as a discipline and for societies at large. The aim of this article, therefore, is two-fold. First, we research how the academic literature discusses the many ways in which models and modelling processes can gain in influence, even beyond their intended reach. We start with the hypothesis that there is indeed still limited scholarship attending to the influence of models and modelling practices. Second, we draw lessons on how to engage with this political charge of water models and eventually how to harness the influence of models for progressive transformation. We begin the article by introducing our understanding of what models are. We then describe the methodology of the study and present the findings of our analysis. Based on the results, we define and call for a power-sensitive approach to modelling and discuss possible methods to facilitate its implementation in practice.
We are aware that there are different viewpoints on what models are and subsequently what their influence on development processes looks like and where accountability lies. It is therefore necessary to clarify the theoretical starting point of this article. First, for the purpose of this article, we adopt a broad definition of models to capture a wide range of modelling practices that resonate with the representational view many modellers share. This view understands models as simplifications of the world that support the processing of input in various ways to create output that is informative about the input and the process. In other words, the output is influenced by the process and input (based on Losee, 1997). The simplifications of the world are based on ideas about how the world functions or should function, enabled or limited by technology and sustained by particular forms of (expert) knowledge, values, and understanding (Haas, 1992; MacKenzie and Wajcman, 1999; Krueger and Alba, 2022). Examples are the different ways in which water is understood, from a purely physical understanding that is often applied in hydrology (taking human influences into account, which is common in socio-hydrology) to seeing a deep entanglement of people and water (Linton and Budds, 2014; Sivapalan et al., 2011). Modelling and models are used for different purposes, including to consolidate ideas about what the world is or to explore unknown parts thereof, for instance through prediction (Morgan and Morrison, 1999; Pielke, 2003; Lane, 2014). Modelling can be done in laboratory or applied settings, for narrowly prescribed purposes such as calculating the height of a dam, or to relate to broader questions of whether, where, or for whom that same dam should be built. These questions have a potential impact (in the case of the dam, a very imminent one) on how modellers and model users engage with and shape the world around them (King and Kraemer, 1993).
Second, to unpack both how power is inscribed in models and how this might gain in influence, it is essential to place our analysis in scientific and technological debates about what knowledge is and how it is produced. This philosophical perspective has significant implications for the way modelling is understood and conceptualised. From this perspective, the modelling process, i.e. problem identification and the development or application of the model to the generation of new information and the support of (policy) decisions, is not linear, although it is often designed or portrayed as such (Macnaghten, 2020; Babel and Vinck, 2022). Different parts of the model development process can run simultaneously or feed back onto one another. Few processes run exactly as designed on paper, and models are not made in neutral laboratory settings devoid of funding, norms, values, and ideas of what the world is and should be.
The constructivist epistemologies we build on conceptualise scientific knowledge as historically contingent, situated, and socially constructed (Latour, 2003). Science and technology studies have long argued that scientific knowledge is to be understood “primarily as a human product, made with locally situated cultural and material resources, rather than as simply the revelation of a pre-given order of nature” (Golinski, 2005, p. xvii). In contrast to mainstream interpretations of science as neutral and objective, science and technology studies conceptualise environmental knowledge as political and shaped by power relations; this determines which knowledge claims are considered more relevant and usable, how and where research should be published, and, in turn, which criteria and norms scientists need to conform with (Demeritt, 2001; Law, 2004; Stengers, 2018; Turner, 2011; Zwarteveen et al., 2017). Thus, power is an inevitable component of any piece of scientific investigation. Paying attention to what is seen and how can be illustrated by the different disciplinary, ontological, and epistemological perspectives of socio-hydrological and hydro-social research (Wesselink et al., 2017). While socio-hydrology takes hydrology as its starting point and adds social components to improve its representation of complex social dynamics (Lane, 2014), hydro-sociology takes sociology and the complex interactions between values, significance, and power relations as its starting points to explain how water and society interact. An example of this different way of thinking is the hydro-social cycle in which water is depicted as being able to flow upstream, driven for instance by economic incentives (Linton and Budds, 2014).
All the models, including the “purely” physical-science-based and quantitative ones, are shaped by people and their norms, values, and institutions, and the models shape these in return (Bijker, 2017; Bijker et al., 1987; Latour, 2000; Latour and Woolgar, 1986; MacKenzie and Wajcman, 1999; Krueger and Alba, 2022; Saltelli and Di Fiore, 2023). This societal influence is clearest and most direct through the visual output of models, such as graphs and maps, used in decision-making processes. However, there are many clearly recognizable or more hidden ways in which models also interact with social processes. It may be that specific elements of the modelling process have more influence than the final product (Lane et al., 2013), for instance by (re-)producing or challenging discourses, either more or less implicitly (Krueger and Alba, 2022). In this process it matters whose information and knowledge are taken into account, who and what are represented in the process, and how. Information and knowledge enter and exit models at every stage of the development process, so the relationship of models with social processes happens throughout the model development chain. However, it is important not to essentialise the influence of models in society and to recognise that their influence might vary from case to case. As Woolgar and Cooper (1999, p. 443) argue on technology more broadly, “technology is good and bad; it is enabling and it is oppressive; it works and it does not; and, as just part of all this, it does and does not have politics”.
Our constructivist theoretical approach and broad definition of models and modelling processes help to make visible that modelling is a process that is susceptible to outside influences in which different choices are made that shape the process and output (Demeritt, 2006; Lane, 2012). Based on the above, we argue that analysing the potential influence of models requires engagement with questions on why modelling is chosen as a method to produce information, which assumptions are included in the problematisation phase and the data and model that are used, how the available technology enables or excludes, and how the process and output are communicated and questioned and by whom. The articles that are included in the analysis do not necessarily apply a constructivist approach, but they do discuss one or all of the aforementioned aspects.
This literature review is primarily based on the ROSES (RepOrting standards for Systematic Evidence Syntheses) method (Haddaway et al., 2018), which is specifically developed for the field of environmental management. It uses a similar approach to systematic reviews that is often used in the social sciences (Petticrew and Roberts, 2006). The method provides a three-stage approach that includes searching, screening, and critical appraisal, and it explicitly allows for additional articles to be included during the screening process to accommodate the multi-disciplinary nature of environmental research. In our preliminary attempt to define the query, we collected articles that discussed the influence of models. For this selection, we drew on our diverse set of expertise as an interdisciplinary group. In our final inclusion and exclusion strategy we selected papers that engage explicitly with how models gain and have influence or, put differently, have socially and ecologically differentiating effects. Following Petticrew and Roberts (2006), we included doctoral research in addition to published articles, as these often comprise studies that unpack longitudinal modelling processes in detail. This resulted in 136 articles, of which 60 discuss water models. We finally identified 30 that reflect on the influence the models have. We formed the first query based on the keywords of these 30 articles. However, we were not able to define a comprehensive query that would capture the majority of pre-selected articles in this first selection due to their disciplinary diversity.
To ensure the replicability of the study, we defined a query based on words that related to the influence of water models. The final query is defined as TITLE-ABS-KEY (“water model*” OR “hydr* model*” OR “groundwater model*”) AND TITLE-ABS-KEY (“justice” OR “equit*” OR “politic*” OR “ethic*”). “Politic*” and “equit*” were chosen as keywords because they broadly relate to how models influence issues of distribution in relation to who gets what, when, and how (Lasswell, 1936). “Justice” and “ethic*” were chosen to capture those articles that reflect on why certain actors – including nature – receive or are deprived of water. The query necessarily excludes words such as “influence”, “power”, “values”, “reflexivity”, “accountability”, and “responsibility”; earlier attempts to define a suitable query that included these keywords resulted in large quantities of articles that did not reflect on the influence models have due to the multiple meanings of these words.
Results were taken from SCOPUS and Web of Science, based on the English language literature for the period January 1993–December 2023. The query resulted in 408 unique documents. Following the ROSES protocol, we screened the articles to identify those that explicitly addressed or analysed the (potential) influence of water models. A first screening by title excluded 40 documents that had no author listed, were not in English, or did not discuss water or water models; 368 articles were screened by abstract, of which 98 showed that the article may reflect on the influence of water models. These were subsequently selected for screening of the full text. Of the 98 articles, 27 were finally selected through the query. In addition, we had pre-selected 30 articles and added 4 suggested by the Hydrology and Earth System Sciences (HESS) community based on the review of this paper, which we included for the critical appraisal stages following the ROSES method after the elimination of one duplicate. This approach is akin to a mix of a systematic literature review and a narrative review (Cronin et al., 2008).
Figure 1 provides a graphic overview of the systematic literature review process, and the Appendix provides an overview of the 61 articles included in the literature review. Those marked with “*” were pre-selected through the narrative literature review.
As the first step of the critical appraisal, we identified shared relationships within and between the reviewed studies (Haddaway et al., 2018). We did this by comparing keywords and by listing common patterns in the included literature, based on our own assessment. By comparing the keywords and main issues, we iteratively identified 13 mechanisms through which models have influence. We identified four overarching themes that represent different phases in a modelling process (for other ways to represent and structure, see for instance Refsgaard and Henriksen, 2004; Melsen et al., 2018b). The first three themes unpack different activities of model-making and its relation to the world, from mental models and policy projects, the influence of modellers' choices on the model, and the ways in which models relate to the world around us. The last theme includes studies in which people explicitly apply changes in a modelling process to account for the (potential) influence of models. The four overarching themes form the structure of the narrative synthesis, in which we elaborate on how all the themes and topics play out in practice. The themes and related mechanisms of influence we identified are the following.
-
Mental models and policy projects
- -
Problem framing: exploration versus consolidation
- -
Knowing the world in specific ways
- -
Working towards different versions of the world
- -
Representation: mental models translated into, and shaped by, categories
- -
-
The influence of modellers' choices
- -
How modellers' choices matter
- -
Familiarity, habits, and standardisation of practices and technological requirements
- -
Modelling developed through interactions and institutional interests
- -
-
The “real-world” impact models have
- -
Naturalising and legitimising world views through models
- -
Exclusive and inclusive assessments
- -
The influence of presentation: colours, maps, and graphs
- -
-
Engaging with non-modellers through models
- -
Connecting to and disconnecting from people and places
- -
Stakeholders confronted with different realities of modelling and measuring
- -
Representation and fairness
- -
Intent: building reflection on engaging with the real world from a modeller's perspective
- -
This review identifies four interrelated dimensions of the modelling process that explain how models gain influence: (a) mental models and policy projects; (b) the influence of modellers' choices; (c) the real-world impact models; and (d) engagement with non-modellers through models (Table 1). We present the main argument of each article reviewed in one of these four dimensions while being aware that several articles present more than one argument. Appendix A provides more details of the articles reviewed, including the different topics discussed, as well as information on the models and case studies discussed in the articles.
4.1 Mental models and policy projects
We start by discussing the mental model (also called the “conceptual” or “perceptual” model in Beven, 2009, “mental images” in Beck, 1999, or “framing” in Odoni and Lane, 2010) that underlies any numerical model. Depending on the process, the mental model is not, or is less, influenced by limitations posed by data and technology and is more of an “ideal type” than an actual model, though Krueger et al. (2016) argue that technological possibilities of what can be modelled may already co-shape what can be imagined. We divide the mental model into two sets of elements, with the first being the ideas of how the world works, including any (causal) relations, and the second being the ideas of what this world should look like. Both elements are based on values, norms, and ideas about what is important and valid to a society in general and a modelling community in particular (Haas, 1992; Haraway, 1988; Jasanoff and Kim, 2009; Morgan and Morrison, 1999). Mental models are developed based on a multitude of factors, including the common interests, backgrounds, knowledge, and skills of those involved. Different communities may have very different ideas of how the world functions (Knorr-Cetina, 1999; Rusca and Di Baldassarre, 2019) or have experience with a particular way of conceptualising that is linked to an already familiar technology (Addor and Melsen, 2019; Babel et al., 2019; Melsen, 2022). In our systematic literature review, 22 articles paid specific attention to mental models. We discuss the main themes, illustrated with examples from the articles reviewed, including (1) problem framing, (2) how different ways of knowing the world influence modelling, (3) how different sociotechnical imaginaries influence why a model is made, and (4) how data and categories embody world views and influence what is included and excluded and in which ways.
4.1.1 Problem framing: exploration versus consolidation
Broadly speaking, there are two very distinct ways to use models. They can be used to explore unknowns or consolidate ideas about reality (Morgan and Morrison, 1999; Pielke, 2003). Several articles put forward how stakeholders that are part of the modelling process may have very different ideas about how the modelling process and outcomes should be used. These articles show that consolidation is often used for decision-making processes in which decision-makers seek to reduce uncertainty, while exploration is used in processes in which there is disagreement about the issue at hand. We use the article of Ramsey (2009) to highlight how world views, policy projects, and technology intertwine. This case study details how a geographical information system (GIS) surface water model was created with the hope of “generating shared understandings” among stakeholders as a key strategy in reducing water allocation conflicts in the Thousand Springs area in Idaho (USA) (Ramsey, 2009; pp. 1975–1976). The latter objective led the modellers to try to create a scientifically sound representation of the Thousand Springs area based on objective and measurable evidence. The model excluded some insights from inhabitants concerning the use of spring water as few measurable data were available on this issue, and the surface water model excluded groundwater from the discussions on water allocation. The exclusion of the experience of spring water users and groundwater prevented a deep exploration of the issues at hand, while this was clearly needed in the process of conflict reduction. The conclusion of the author is to call for dedicated time to explore “diverse problem understandings”, which entails clearly defining the mental model and modelling vision before engaging with a modelling effort.
To avoid disconnects between the model and user such as described by Ramsey (2009), Trombley (2017) suggested a multi-model approach to avoid a model serving one particular policy project at the expense of others in his PhD research. One of the suggestions they made is to design models for decision-making with the aim of facilitating exploration, models thus becoming mediators that foster a diversity of perspectives. Constanza and Ruth (1998) proposed engaging with the consolidating and exploratory functionality that models can have in the same modelling process by introducing a three-phase modelling approach. The first stage focuses on developing the model structure and “functional connections between variables” in discussions with stakeholders, the second stage focuses on replicating dynamics of interest realistically, and the third stage focuses on scenarios and management options. Alam et al. (2022) proposed a similar approach by calling for inclusion of positive and negative externalities, specifically in relation to agent-based modelling applied to understand the impact of agricultural water management interventions. They proposed such an approach as their review showed that there is limited attention paid to the spatially explicit and inequitable outcomes of interventions.
4.1.2 Knowing the world in specific ways
In the water sector, the way models are developed is often greatly influenced by specific “epistemic communities” that are bound by shared ideas about validity and causality and by ways of working that engender a particular vision of the world (Haas, 1992) or a particular way of doing things through communities of practice (Lane, 2012). Bouleau (2014) theorised about how expertise mixes with political priorities to influence the choice of tools and issues to be addressed and how this in turn influences the world. In the article, Bouleau contrasted the approaches of two different epistemic communities in two different river basins in France. In the Rhône River basin, model development was initially mainly guided by geographers and ecologists who focused on the floodplains. As a result, water was conceptualised as a “hydrosystem” linking hydrological and ecological processes in the river and floodplains. During the same time period in the Seine River basin, model development was led by engineers who assessed water quality in relation to the economic development of Paris. Water was conceptualised as a condition for economic development that should be closely monitored and modelled. The mental models, developed differently based on different expertise and political priorities on top of the material properties of the two river basins, influenced what was seen and how and consequently what the aquatic environment looked like (p. 253). Another example is provided by Andersson (2004), who confronted a project in which three models (HBV-N, STANK, and SOIL-N) were used to assess options for reducing riverine nitrogen loads in the upper Svartå Valley in Sweden with user opinions. The focus of the project on nitrogen, and not on phosphorus as well, for example, was found to be limiting and to not reflect decisions that had to be taken. Despite this limited focus, the overall modelling process was deemed to create a mutual learning environment for modellers, stakeholders, and decision-makers. A more philosophical reflection is provided by Laborde (2015), who compared their conceptualisation of a lake using MATLAB with a conceptualisation of the same lake by a fisher. By reflecting deeply on the underlying experiences and expertise that shape a (mental) model, they raise rhetorical questions on why the modelling version of the lake is (better) represented in decision-making and why the fisher's is not and whether there is space for complexity that is brought in through lived experiences, as is done by the fisher.
4.1.3 Working towards different versions of the world
Sociotechnical imaginaries are visions of what the future can become, built on a notion that technology can assist in realising this envisioned future and be shaped by values (Haraway, 1988; Jasanoff and Kim, 2009). Working towards a certain envisioned future is also conceptualised as “policy projects” (Haas, 1992). Making values explicit is therefore useful in understanding what a modelling process aims to achieve. Deitrick et al. (2021, p. 12) identified and visualised which ethical and epistemological values inspired watershed modellers in the Chesapeake Bay in the USA by surveying and interviewing the modellers involved. To support modellers and those who use or are impacted by models, the authors made visible in a flowchart what kinds of choices in the modelling process related to ethics and knowledge production. These choices ranged from questions of funding and model selection, over how environmental processes were to be represented, to how users engaged with the model and how the results were interpreted while also scoping out available alternatives. The authors called for more openness and more explicitness by modellers when communicating these choices to contribute to transparency in decision-making. Rainwater et al. (2005) show how different epistemological values and policy projects influence data collection for groundwater modelling as well as how local political borders influence user engagement with modelling results for a shared groundwater body in Texas. Wheeler et al. (2018a, b) also emphasised the importance of making policy projects explicit and proposed a modelling approach for highly political and conflict-related contexts in which intended model users have very different world views and intended uses of the available water. The authors used the case of the Nile to explore possible future designs and operations of the Grand Ethiopian Renaissance Dam and its relation to operation of the High Aswan Dam in Egypt. The method did not focus on optimisation necessarily but started with identifying upstream and downstream state preferences as well as criteria (in this case scenarios based on acceptability and no harm) that guided the modelling exercise.
4.1.4 Representation: mental models translated into, and shaped by, categories
Definitions and categories are important mechanisms for translating world views into models. Building on feminist science and making gender explicit, two articles in our literature review call for more inclusive modelling. Haeffner et al. (2021) showed that available water data often disfavour women and local communities as few disaggregated data based on these categories are available. Disaggregation, which would entail collecting specific data related for instance to gender, class, and caste, can make differences and inequalities visible. When datasets are not aggregated or for instance create biases towards male water users (who are oftentimes more visible), the modelling exercises based on biased datasets inherit the same biases and knowledge gaps unless these are explicitly acknowledged and addressed. The solutions that the authors see to account for the limitations of modelling are to collect data that include specifications including race, class, and gender and to always contextualise results. This means that, in addition to presenting the outputs of the modelling process, the historical and cultural context of what is modelled is described too. Packett et al. (2020) emphasised that not only should the input into a model be of concern but that a balanced gender representation should also be achieved during the whole modelling process, including problem framing and conceptualisation, model construction, documentation and evaluation, and model interpretation and decision support.
Harvey and Chrisman (1998) unpacked the development of GIS technology to show how it can work inclusively and bring different groups together, but it can also work exclusively. Based on a case study on the mapping of wetlands in the USA, the authors argued that an important element that defines who and what are included or excluded is the mental model that underlies the GIS and modelling activities. Their case started with very different ideas about what wetlands are to American institutions. How different these understandings can be was highlighted in a 1995 report that compared four different datasets that represent the same wetland. The datasets disagreed in more than 90 % of the area on different purposes, procedures, sources, definitions, and logic that shaped the different inventory techniques (Shapiro, 1995, p. xiii). To address these discrepancies, one specific system (Cowardin et al., 1979) was chosen as a standard by the US Federal Government in 1997 to define wetlands. The authors warned, however, that even though a mental model is standardised to facilitate exchange, the introduction of different modes to collect data and different approaches to analyse these can again create different interpretations of the same area. In addition, the black-boxed nature of models can obscure these different interpretations, and an effort needs to be made to understand the influence of data collection methods and model choices.
4.2 The influence of modellers' choices
The following set of articles focuses on how a model is developed. Thirty-four of the articles in the review explicitly discuss modellers' choices. This includes the influence of modellers' familiarity with the models they use, their habits, and standardisation.
4.2.1 How modellers' choices matter
Modellers' choices matter, as they influence both the development and output of a model. Holländer et al. (2014) showed through a model comparison experiment that, when provided with the same data-scarce fictive watershed, 10 modellers essentially predicted 10 different (some of them very different) discharge time series based on the models of their own choosing. Within the same model, choices also matter greatly. Melsen et al. (2019) systematically demonstrated the impact of modelling decisions for the case of a flood and drought event in the Swiss Thur River basin, specifically for decisions on spatial resolution, spatial representation of forcing, calibration period, and performance metric. Mendoza et al. (2016) showed how hydrological modelling decisions can influence evaluations of climate change impacts. When comparing four different modelling structures and parameter estimation strategies applied to three watersheds of the Colorado River basin, the authors showed that calibration decisions may unexpectedly have more impact than the choice of model structure. Dobson et al. (2019), by comparing eight rival framings of two models of two water resource systems in the UK, showed how these specific representations of the systems influenced which water management decisions were suggested by the models. The choices of system boundaries and the statistical formulation of forcing generators were shown to have the greatest impact. Krueger and Alba (2022) discussed three types of models, a socio-hydrological human–flood model, an export coefficient type model, and a water security model, to showcase the interactions between modelling and policy. These case studies were used to analyse to what extent considerations of uncertainty, subjectivity, and fitness for purpose have led the hydrological community to engage with the political consequences of models and the powers inscribed in those models, be they world views, omissions, or vested interests. The authors especially see an opportunity for both modellers and social scientists to explore and engage with the political consequences of models together in relation to model uncertainty.
4.2.2 Why choices are made: familiarity, habits, standardisation of practices, and technological requirements
The choice of the modelling technology or model type has a great influence on the modelling outcomes. Addor and Melsen (2019) demonstrated, based on a survey of hydrological modellers, how familiarity with a model type is a better indicator of why a model is chosen than whether it is the best fit in terms of representing natural and social dynamics, contrary to what is typically depicted in scientific articles and consultancy reports. Babel et al. (2019) demonstrated that modellers inherit modelling choices from former supervisors and colleagues. This leads to long-lasting and sometimes unquestioned habits in model construction. Jenkins and McCauley (2006) made this visible by unpacking the GIS flow direction algorithm in the ESRI products ARC/INFO, ArcView, and ArcGIS, which can seemingly make wetlands disappear from maps. Without understanding why and how the GIS algorithm functions and without confronting the model world with the modelled world, this could mean that decisions are made that are ignorant of what is left invisible. Fernandez (2014) showed through historic research how the development and embedding of an indicator of minimum flow requirements (MFRs) are influenced by the financial and institutional needs of powerful water users in the Garonne River basin in France. Originally introduced in relation to water quality, the MFR indicator later became a stand-alone indicator in relation to river health and setting the conditions for the construction and management of hydropower dams to define sector-based water savings. This disconnect, as well as changes in decision-making processes for the host institutions of the indicator, led to the indicator becoming unquestioned and black-boxed.
Whatmore and Landström (2010) traced the adoption of a formula for calculating the velocity or surface inclination of water flowing in an open channel of given dimensions, or Manning's n, first presented in 1889. Although it is criticised as a simplification, the formula allows for simple tuning of a model that has incorporated it and limits the runtime. As such, attempts to replace this formula have failed so far. These six articles show how important the element of expertise is in modelling and warn of certain blind spots, which, once models become accepted and unquestioned tools, may be accepted as the way things are done. This does not mean that modellers are generally not reflexive. Kouw (2016) showed, for the case of hydraulic engineering in the Netherlands, different ways modellers include reflexivity in their modelling practice, including finding a balance between the detail of a model and the time needed to run it, engaging with models as “sparring partners” instead of “truth makers”, and knowing the basic structure of the model.
4.2.3 Modelling developed through interactions and institutional interests
Landström et al. (2011a) drew attention to a wide range of actors that influence modelling by assessing the practices of modelling flood risk of consultants for the Environment Agency of England and Wales. The authors showed how modelling processes are shaped by environmental managers, decision-makers, and developers, which is influenced by standardised modelling processes, including practices to visit the modelled field before and after a modelling exercise as well as long-term contractual agreements, such as the requirement to use a particular software package. The authors argued that the high level of standardisation limits the space for asking new questions, and therefore they recommended that the standard practices be routinely compared with new models developed by academics. In a connected paper, Lane et al. (2011a) discussed how models are used for predicting floods, taking into account climate change. By unpacking the modelling process, the authors showed that a primary assumption in the model was a guideline from the government which estimated that peak river flows for the 2080s will increase by 20 % compared to 2010. Published as part of the same research project, Lane et al. (2013) showed how technology has an influence on the choice of model. The authors discussed developments, from 1D (one-dimensional) modelling to represent water following a specific path to 2D (two-dimensional) modelling in which water can be represented as flowing both down and to the sides to mimic a floodplain. A specific event, such as a flood, provided a moment in which such developments and new sociotechnological constellations become apparent.
Munk (2010) and Junier (2017) also showed in their doctoral thesis how models are developed by a multitude of actors and occurrences. In their longitudinal studies based on interviews and observations, they unpacked the development process of the Hydraulic Engineering Center's River Analysis System used for flood risk analysis in the UK and the WFD (Water Framework Directive) Explorer in the Netherlands. Wesselink et al. (2009) did a similar analysis, in a research article, of how models are developed in conjunction with decision-making processes. They showed that, in the case of the Dutch Meuse River, political considerations have an unexpectedly great influence in relation to technical water expertise, especially in relation to transboundary water management.
Jackson (2006) described in detail the process of how CalSim, a model used by the California Department of Water Resources to estimate and plan water delivery between 2001 and 2021, became a topic of public controversy. Developed in a sphere of trust based on similar professional expertise, it became apparent that the model was scrutinised based on different requirements in the public sphere. This necessitated changes in modelling practice towards more open and transparent processes. Jackson called for a broad take on modelling focusing on not only conceptual, mathematical, and computer-based aspects, but also organisational, political, and broadly sociological aspects, which could lead to decisions to “sacrifice a degree of analytic precision and granularity, but [..] gain in broader stakeholder accessibility and general analytic wieldiness” (Jackson, 2006, p. 8).
4.3 Modelling and real-world impact
Models are often discussed within the confinement of the model land they create (Thompson and Smith, 2019) or, in other words, under laboratory conditions insulated from the public and disconnected from the world that is being modelled. Whether developed under laboratory conditions or explicitly to inform (water) governance and management, models can have several unintended impacts. In our systematic literature review, 19 articles have paid specific attention to modelling and its real-world impact. The articles are all based on case studies and have paid particular attention to examining the context in which models are produced and how the model connects with, disconnects from, and influences the surrounding environment. The two main themes highlighted in the literature concern how models are mobilised to naturalise and legitimise certain policies and world views and how modelling processes can work to conceal or exclude some of the affected groups.
4.3.1 Naturalising and legitimising world views through models
Water governance processes are always contested and political, as stakeholders are likely to hold different world views, including contrasting visions of the way water should be managed and allocated and whose expertise and knowledge should be valued in decision-making processes (Zwarteveen et al., 2017). Models, therefore, can have the unintended consequence of legitimising one of these world views whilst concealing others. To illustrate this, coal mining is a contested process in which affected stakeholders might have different perceptions of the threats and potential of this development. To illustrate this, Connor et al. (2008) analysed the discourses related to a local debate on the development of an open-cast coal mine in Murrurundi, a town in the upper Hunter River basin in New South Wales, Australia. Models formed an integral part of the process by supporting the narrative of both the coal mine exploiter and the government. Despite the multiple distinct perspectives ensuing from this project, the models ended up legitimising the world views of the industry and state while concealing those of many affected groups who valued care of and cultural and spiritual connections to the place and water bodies. The paper thereby highlighted two real-world impacts of these models. First, they contribute to policy options grounded on notions of productivity and economic development promoted by the state and industry. Second, building on this first point, they also contributed to grounding the debates in scientific terminology and concepts, thereby forcing groups contesting these world views to draw on the same language and knowledge claims. Cornejo and Niewöhner (2021) showed a similar dynamic in the case of mining water abstraction in Tarapacá, Chile. Based on a groundwater model that depicted an aquifer as two separate water basins, it was decided to grant a mining company water rights as it was scientifically proven that the water resources would not be affected. Here too scientific knowledge generated through modelling was prioritised over local knowledge and everyday experiences. The way the modelling process was designed prevented the affected groups from questioning assumptions about future impacts of water abstraction. In addition, as the problem was framed in the scientific language generated by the model, local communities were forced to adapt to that language and generate data that spoke to the language and arguments of the scientific reports. The authors concluded that, in this contested process, the model became a “real” actor aligned with the interests of private companies and the neoliberal state. While this clearly shows the political nature of models, paradoxically, it is the notion that science is value-neutral that makes these models such powerful actors in water-related decision-making processes.
Kroepsch (2018) and Sanz et al. (2019) also discussed how groundwater models can be used to legitimise policies even if there is limited information available. Sanz et al. (2019) showed that, despite intrinsic uncertainties, and against the advice of the researchers who developed the model, a MODFLOW model was used by a governmental actor to legitimise boundaries drawn that determined which farmers were compensated for refraining from irrigation and which were not. Kroepsch (2018) questioned how it was decided to optimise space for groundwater abstraction instead of limiting it, even when impacts were unknown due to a long feedback time. Based on the analysis of 10 years of groundwater modelling and governance in the northern San Juan Basin in Colorado (USA), they argued that, in this project, in addition to quantitative measures, the “human values in risk-taking or precaution” should have been prominently included.
4.3.2 Exclusive and inclusive assessments
When modelling is presented as a neutral scientific process, a lack of attention to the context and its power relations can have negative effects on marginalised groups in society. An example of such a “desocialised assessment” was provided by Budds (2009) in a case of the La Ligua River basin in Chile. The author questioned the extent to which a hydrogeological model, used to represent the physical diversity in the La Ligua River basin, was representative. The model was based on data mainly available for the main river and not its tributaries, with limited information on actual water use (including illegal abstractions), and the modelling process included a limited assessment of the model's validity. Despite this, the model was used to define a generic policy for the additional allocation of water rights that could have led to aquifer depletion. Budds pointed out that this was possible partly due to the legitimacy given to the project by external consultants, whose expertise is generally held in high regard. She further argued that the model facilitated the implementation of a policy that reproduced pre-existing water inequalities in the basin. First, the allocation of the additional water rights did not take into consideration that commercial farmers were in a better position to acquire them. To illustrate this, obtaining legal rights for water abstraction required a lawyer and money, thereby favouring large and smaller commercial farmers over peasant farmers. Second, Budds (2009) argued that by excluding knowledge claims from peasant farmers, the model did not account for the fact that the increase in groundwater abstraction by peasant farmers was an adaptive response to the increased water use for agriculture in the valley and the 1996–1997 drought. Not recognising the vulnerability of these farmers by framing their actions as illegal ultimately increased their vulnerability. The author thus concluded that the fact that the water resource agency focused solely on hydrogeological modelling allowed the Chilean state to justify water allocation decisions that reproduced “unequal patterns of resource use” (p. 418).
Holifield (2009) described a similar dynamic in the case of groundwater modelling to understand the extent of pollution in St. Regis, Minnesota, USA. Modelling by the Champion International Corporation was challenged by a “counter-network” of local inhabitants and scientists, who had to prove that their representation was more scientifically viable. Holifield (2009) showed that this required them to include both disinterested “outsiders” and interested, locally accountable insiders and to make connections with “bigger” centres of power and calculation, which can multiply and amplify the locality's connections with equipment and resources (p. 371). Inspired by Holifield (2009, 2012), Meenar et al. (2018) applied an environmental justice perspective as a basis for (re-)developing flood mitigation and stormwater management plans in a watershed in south-eastern Pennsylvania, USA. Using the environmental justice dimensions of just distributions, procedure and participation, and recognition as entry points, the authors supported the redrawing of floodplains in a more inclusive way and in interaction with local inhabitants.
Similar dynamics were examined by Godinez-Madrigal et al. (2019), who showed how models supported top-down management of water-scarcity issues and related water allocation policies in the Lerma–Chapala Basin, Mexico. Outcomes of one modelling exercise were not accepted when they conflicted with the interest of an important actor, and a second modelling exercise excluded an important out-of-basin user which skewed the results. The decision on water allocation was eventually enforced through influence at the highest political level, the President of Mexico. Jensen (2020) also confirmed that the power of high-level decision-makers plays a key role. In the case of the Mekong River, the author showed that there is a certain saturation in knowledge developed by models, and there is a clear limitation on their impact as governments were unwilling to build on these insights. He argued that, “compared with the inventive energy deployed in modelling, moreover, it can also be observed that the efforts made by modellers to make this knowledge travel are rather less creative” (p. 88). These articles show that a model does not have influence on its own.
The previous examples show how models can work exclusively. The following articles show how pluralising data sources and methods can help to make the excluding nature of models visible, and they show how to mitigate this. Garcia-Cuerva et al. (2016) suggested a participatory modelling method aimed at including marginalised communities in the case of identifying opportunities for stormwater control measures in the Walnut Creek watershed in North Carolina (USA). Although not yet tested, Garcia-Cuerva et al. (2016) opted to first develop a modelled version of Walnut Creek and cooperated with an NGO, Partners for Environmental Justice, to facilitate discussions with stakeholders “to evaluate alternatives and to elicit preferences” (p. 43). Hasala et al. (2020) followed up on the study of Garcia-Cuerva et al. (2016) and compared the approach of collecting information through modelling with a method that relied on interviews. Specifically looking at identifying possible sites for green roofs in majority–minority neighbourhoods in relation to stormwater control measures, they reported significant differences on which roofs should be greened based on interviews of people living in the area and based on the model outputs. When used in conjunction, the authors showed how the model could be used as a tool to bring different stakeholders together to discuss which options fit a neighbourhood best.
4.3.3 The influence of presentation: colours, maps, and graphs
Interestingly, few articles discuss in depth what the influence is of specific ways of presenting the modelling results through illustrations such as graphs or maps. Most refer to this in passing. For instance, Bergström (1991) also concluded that ethics in modelling is becoming more and more important with the rising popularity of models, and he did so based on a review of the development and use of the Hydrologiska Byråns Vattenbalansavdelning (HBV) and PULSE models at the Swedish Meteorological and Hydrological Institute between 1971 and 1990. On illustrations, Bergström (1991) said that “Multi-colour graphical presentations are very useful for illustrative purposes but they should not be used to impress or convince where the scientific foundation is weak” (p. 134). Abbott and Vojinovic (2014) discussed illustrations as a way to connect with stakeholders, claiming that stakeholders are “challenged-out to exercise and develop their own inherent knowledges, imaginations and judgments, and to exercise these both independently and interactively” (p. 528). Abbott and Vojinovic also pointed out the responsibility of the modeller, claiming that the “quality of the character of the modeller, becomes inseparable from the quality of the model within the quality of the total production” (pp. 528–529).
4.4 Engaging with non-modellers through models
When it comes to modelling, we want to pay specific attention to the engagement of non-modellers in modelling processes. To counter the exclusionary nature of modelling, a popular approach is to engage those affected by the processes that the models aim to examine. Methods range from taking into account the needs and positions of different stakeholders in the design of and communication about the model (Cash et al., 2003; Harmel et al., 2014; Bremer et al., 2020) to different forms of participatory modelling (Étienne, 2011; Voinov et al., 2016; Venot et al., 2022). However, few of these articles discussed power differences between those involved, accounted for those who disengage or who and what are excluded, or were mindful of the influences the model can have on decision-making processes. In the literature review, 24 of the included articles paid specific attention to including people and values in the modelling process. We discern different themes, including (i) engagements with how models can create connections and disconnections from the people and places that are being modelled, (ii) how non-modellers relate to specific world views and policy projects included in the model, (iii) representations of who and what are modelled in just and fair ways, and lastly (iv) how modellers reflect on engaging with who and what are modelled.
4.4.1 Connecting to and disconnecting from people and places
Lane et al. (2011b) experimented with “doing flood risk science differently” to foster connections between academics and local people for whom flooding is a matter of concern, and they used this as a basis for co-producing knowledge in non-hierarchical ways. The project and approach created a way for local knowledge to be taken into account by the responsible institutions in the case of Pickering, UK. By explicitly confronting modelling results and proposed management options with the experiences and opinions of local residents, it became clear that more inclusive and less invasive flood risk management options were possible. Opitz-Stapleton and MacClune (2012) reflected in a book chapter on elements that create disconnects between affected communities and the hydrological and climatological modelling that is used for community-based climate change adaptation and disaster risk reduction. Based on case studies from the edited volume, they identified a number of issues that can create disconnects between the modelling activity and the community for which it is intended. One issue that plays a significant role in community (dis)engagement is the degree of complexity of the model. The authors warned against thinking too much from a modelling and consultant perspective rather than from a community perspective, and they suggested avoiding the selection of a model that is overly complex and maladapted to conditions of data scarcity, working at scales that are beyond the ones a community is generally thinking at (usually under 10 km), overlooking politics at transboundary and national levels, and not speaking the same language of the communities for whom the model is developed. Opitz-Stapleton and MacClune (2012) concluded that organising modelling activities meets their proposed specification needs: “a rare combination of technical skill, cultural sensitivity, political awareness, and above all, the time to continually engage with and build relationships within the community in order to foster resilient change.” (p. 208).
An often-used framework to analyse the uptake of models was provided by Cash et al. (2003). The framework analyses how a model connects with its environment, based on its acceptance by stakeholders in relation to salience (does it fit), legitimacy (is it fair), and credibility (is it believable). We explain it here as the framework is used in 2 of the 48 articles included in this review. Bremer et al. (2020) applied the framework to different case studies on watershed management programmes in the Atlantic Forest of Brazil. Falconi and Palmer (2017) applied it to assess whether participatory computer models for water resource management in the USA, the Solomon Islands, Senegal, and Zimbabwe are indeed effective participatory decision-making tools based on surveys. They also emphasised that a contextual analysis is first required to gain insights into who, when, how, and why questions. Both articles highlight that models cannot meet the expectations of each stakeholder and therefore need to be carefully embedded in decision-making processes. Bremer et al. (2020) also emphasised that it is necessary to take power dynamics into account in this process. They concluded that, as hydrological modelling can influence larger development projects, it is essential to critically reflect on how and by whom these will be used and to what extent they are grounded in local realities.
4.4.2 Stakeholders are confronted with different realities of modelling and measuring
Wardropper et al. (2017) analysed how inherent uncertainty in the application of the Soil and Water Assessment Tool (SWAT) to the Yahara Watershed in Wisconsin (USA) influenced the development and implementation of a water quality management programme. The programme aimed to reduce phosphorus pollution. Modelling was used as a tool to estimate water quality and assign needed pollution reductions to different groups, while monitoring and compliance were based on measurements. An additional challenge in the case study was that results of the policy were not directly visible, as they were most likely to be seen within a 10-year time frame. The authors questioned how the inherent uncertainty in this approach affected people in the watershed. The authors interviewed policy-makers and those who would be subjected to the new policy on how to design such a policy in situations of uncertainty. These deliberations were found to be crucial in designing a policy that was experienced as being both fair and effective, although the risk remained that the resulting actions were not influential enough to reduce the pollution. Kouw (2017) also discussed inherent traits of modelling practices that can create disconnects between models and model users, also emphasising that uncertainty is dealt with differently by engineers, decision-makers, and users. Subsequently, Kouw (2017) called for more integration of social scientists into the practice of developing and using technical tools for decision-making.
Landström et al. (2011b) described in detail a participatory model experiment in which modellers, social scientists, and local residents met on a bi-monthly basis over a period of 1 year to co-produce knowledge about flood risks in Pickering, UK, using a “competency group” approach. This approach asked for all participants to join as individuals, not as representatives of a certain group, and for science to be produced based on questions by the group. What was important for the project was that science was disconnected from institutions that had a role in discussions on flood risks, that scientific questions were not defined in advance, and that scientific questions were open to reframing during the project. Two models were developed as a result of this collaboration: the first was intended to be the final model and ultimately served as a starting point for discussion, and the second was designed based on requests and inputs of the participants and ultimately played a key role in shaping flood management strategy in the area.
4.4.3 Representation and fairness
Haeffner et al. (2018) researched how perceptions and concerns of stakeholders and decision-makers were represented in the management of water systems in urban areas in Utah, USA. First, the authors undertook a review of socio-hydrological frameworks – including models – that sought to unravel the interplay between water and society. Based on this review, they argued that socio-hydrological studies tend to assume that stakeholders have “roughly equal chances of experiencing, perceiving, and responding”, while generally this is not the case (p. 666). Drawing on data collected through semi-structured interviews and surveys from city council employees, public utilities, and residents, they concluded that public officials and residents do not share the same concerns about the water supply system. While residents' main concerns relate to shortages and tariffs, public officials are significantly more focused on the deterioration of water supply infrastructures. They also found that citizens who were most involved in decision-making were also more often likely to agree with the perspectives of water system leaders, Based on these results, they concluded that models assuming that residents were well informed and had a shared understanding of the water supply system might lead to an oversimplification of socio-hydrological dynamics in a given location and that more local involvement could mitigate this.
4.4.4 Intent: building reflection on engaging with the real world from a modeller's perspective
There are several authors who reflected on the impact of the work in their field and who subsequently called for modellers to take an explicitly ethical approach (Abbott and Vojinovic, 2014; Bergström, 1991). Clark (1998, p. 833) also pointed to the responsibility of modellers, and specifically when it comes to improved resolutions in GIS applications, as “seemingly omniscient but insensitive systems”. Although this is an old article, its reflections are still valid as technology and resolutions keep improving. Besides meeting standards for data use and processing, facilitating access for all, and auditing, Clark (1988) also pointed to the responsibility of the modeller: “Have you personally asked whether what you are doing is beneficial to the business, the customer and society? You cannot transfer this responsibility to someone else” (p. 832). Shrader-Frechette (1997) also called for ethical rationality in hydrogeological modelling, meaning that modelling hypotheses have to be considered in the light of their “ethical goodness” or “ethical badness” for the population on site. de Oliveira Ferreira Silva (2022) called for a similar approach to validate models and their hypotheses, especially when it came to the impact of their use on society. Also, Lane (2014) based his suggestions for principles for socio-hydrological modellers on personal experiences with hydrology. Based on a deconstruction of practices of hydrological science, Lane proposed (i) embracing conflict and controversy in science, (ii) looking for extremes to test knowledge while doing this in a way that is sensitive to political and ethical ramifications, (iii) using real-life events to think with and step out of model land, and (iv) co-producing knowledge with affected groups. Lane concluded that hydrologists cannot do this alone but that it requires both social science and hydrology.
It is here that Srinivasan et al. (2016, 2018) and Melsen et al. (2018b) engaged in a discussion on how modelling should happen. Melsen et al. (2018b) pointed out that models are not value-neutral and that they have significant power, which raises questions about the responsibility and accountability of those making and using models. This, the authors suggested, calls for a reflexive approach to modelling, which should incorporate questions about the model's (potential) impact, who is included and excluded and why, as well as a conscious effort to include less powerful stakeholders. In line with this idea, Srinivasan et al. (2018) proposed a number of practices to improve socio-hydrological modelling, including reflecting critically on model structure and functional form, teaching people to use models as a hypothesis rather than a truth, developing guidelines on how to make modelling choices explicit, soliciting input from stakeholders, and mobilising knowledge brokers or institutions to mediate between modellers and others involved. They warned that educating scientists from both the social and natural sciences would take time and that currently the academic culture does not value interdisciplinarity.
The literature review provides an overview of the current state of research on the influence of water models. We closely reviewed a total of 61 articles through our methodology, based on the narrative review and query TITLE-ABS-KEY (“water model*” OR “hydr* model*” OR “groundwater model*”) AND TITLE-ABS-KEY (“justice” OR “equit*” OR “politic*” OR “ethic*”). The query embodies a particular way of engaging with the influence of models grounded in the idea that modelling processes are not linear and that they shape and are shaped by society in different ways. The articles that are included in the review represent a broad spectrum of theoretical and practical approaches to the influence of water models as well as a broad range in terms of focus. The four themes used to order the 13 mechanisms models can influence are mental models and policy projects, the influence of modellers' choices, the real-world impact models have, and engagement with non-modellers through models.
We see the list of themes and 13 mechanisms as a starting point for researching the influence of water models as well as an inspiration for the design of modelling processes. Examples from the articles that were reviewed show for instance that modelling with a particular intention in mind, such as environmental justice or gender equality, does impact the way in which a modelling process is carried out (Haeffner et al., 2018; Meenar et al., 2018). This also shows that it is useful to place discussions on the fitness for purpose (Beven, 2019); salience, credibility, and legitimacy (Cash et al., 2003); or post-audits in a broader socio-political context. Attending to the influence of models raises questions such as “whose purpose is served?” and “who decided what a model should do?”.
Our systematic and narrative literature review methodology also posed specific challenges. For example, many of the words commonly used to describe the influence of models (including reflexivity, influence, power, accountability, and responsibility) proved to have multiple meanings that are also used to describe specific – yet different – processes in modelling. This made it necessary to specify the query with the risk of missing relevant articles (low sensitivity). Also, it is known that reflexivity on these political aspects of water modelling comes in many forms and often happens in formal and informal meetings (Babel and Vinck, 2022; Melsen, 2022; Kouw, 2016). This also means that modelling processes may have been informed by reflexive practices, without being mentioned in scientific articles. Increasing the sensitivity (obtaining more relevant publications) by broadening the query for the systematic literature review would decrease the specificity and increase enormously the number of publications to be screened without necessarily providing more papers relevant to the aim of the query.
To complement the systematic literature review, we did an initial literature search with a variety of keywords, and we asked the HESS community to suggest relevant literature. These suggestions were very useful and yielded 34 relevant publications that were not retrieved with the systematic literature research. Of course, the selection of these hand-picked publications depended on the set-up of the initial search and who reacted during the public review process.
Interestingly, we saw that in the articles reviewed there is limited attention paid to the influence of vested interests on the choice of technologies used – including private and academic interests – and limited attention paid to the way in which model outputs are presented. Another observation is that several articles that discuss the impact of models do not specify the modelling software used. It is clear that choices have to be made, within the limited framework of scientific articles, on what information can be conveyed, and it is clear that interactions between specific elements in a model (such as a framework) and specific representations of the world are prioritised over how a model is developed (Cornejo and Niewöhner, 2021; Jackson, 2006; Kroepsch, 2018).
Lastly, and interestingly, the power disparities between those involved in and affected by modelling processes, together with the power of models, are addressed by only a few authors in this literature review (Budds, 2009; Godinez-Madrigal et al., 2019; Haeffner et al., 2021; Harvey and Chrisman, 1998; Holifield, 2009; Connor et al., 2008; Cornejo and Niewöhner, 2021; Meenar et al., 2018). Few of the articles focus on those who disengage from the modelling process or on who and what are excluded, and few are mindful of the influences the model can have on decision-making processes. It is exactly those articles, and especially the case studies that describe knowledge controversies, that provide opportunities to learn and bring up questions and examples of how accountability can look in practice. Hence, we call for a power-sensitive approach to modelling in the water sector. We argue that this is a crucial endeavour since models are not only influenced by power but also have the power to (re)produce particular long-lasting social, cultural, and technical configurations in the world with more or less desirable social and sustainable outcomes.
This review confirms that models shape the world around them and that the world around models shapes them in return. This happens in ways we are aware of or in more covert or unconscious ways. There are different mechanisms at play that define how a model and modelling process influence what is seen as a “natural” or legitimate understanding or solution; who and what are concealed, revealed, and in what ways; and possibly also who gets what, when, and how. These mechanisms play out in four phases of model development: the inception and commissioning; the making of the model itself; the use of the model; and, during these processes, the engagement with non-modellers. We have shown that it matters that a model is made in a specific context in which a problem framework is defined, and this problem framework can be altered. The literature also shows that we have to be aware of the ways in which our world view and expertise influence the problem framing, the choice to use modelling for a specific purpose, and how these are embedded by others in modelling frameworks and databases. The modeller is not the sole responsible person in this process, and funders, commissioners, and model users play important roles.
In Sect. 3 we argued that this is applicable to both models that are developed for practical applications and those that are developed in laboratory settings (King and Kraemer, 1993). Approaching models as neutral tools may conceal opportunities to do modelling in support of more just and equitable water distributions. The review also shows that modelling can be done differently, for instance by exposing black-boxing of decisions; explicitly showcasing the development process of modelling and how modelling decisions affect outcomes; openly questioning modelling decisions and the assumptions behind them; foregrounding power relations; calling for particular ethics; and focusing on the process instead of the tool. We therefore call on water modellers, commissioners, funders, and model users to further understand and engage with the power of water models, from ideation to implementation, in an ethical and accountable way. We have identified a few avenues for power-sensitive water modelling, based on this review, and refined these based on other calls related to the politics of modelling (Chilvers and Kearnes, 2015; Doorn, 2012; Krueger et al., 2016; Lane, 2014; Maeda et al., 2021; Rusca et al., 2023; Saltelli et al., 2020; Turnhout et al., 2007; Puy et al., 2022; Venot et al., 2022; Voinov et al., 2014; Zwarteveen et al., 2017). We refer to the literature reviewed in this article, in which practical examples are given of the points made below.
-
Take a holistic approach to modelling. A model is more than the final product or output. The modelling process stretches beyond programming and coding and includes everything that influences model-making and that is influenced by it. For instance, it includes the processes of problematisation, defining the purpose of the model, commissioning, implementation of decisions based on the modelling, and the co-shaping of discussions (Jackson, 2006; Junier, 2017; Kroepsch, 2018; Munk, 2010; Trombley, 2017). This holistic approach to modelling helps to identify where changes can be made. The development of a water model should be based on a thorough understanding of the interactions with the places a model is developed and applied in (Clark, 1998; Lane et al., 2011b).
-
Foster accountability. Modellers, commissioners, and model users have an ethical obligation to take possible real-life consequences of a modelling process or use of a model into account and to change a modelling process accordingly (Bergström, 1991; Lane et al., 2011b; Meenar et al., 2018). This also includes reviewing a modelling process after it is concluded.
-
Work towards just and equitable water distributions. The choice and use of water models happen in a political context and have political consequences in a world where some gain and others are overlooked or lose. A first step is to consciously define ethical and epistemic values that underlie the modelling process (Deitrick et al., 2021; Holifield, 2009; Meenar et al., 2018, Packett et al., 2020). There is a joint responsibility to work towards more just and equitable water distributions for people and nature (Abbot and Vojinovic, 2014, Bergström, 1991; Lane, 2014).
-
Be transparent. Increasing transparency throughout the modelling process is a way forward to make explicit and ultimately examine and attend to the multitude of interests shaping the development and use of models and their socio-economic and ecological consequences. Modellers and commissioners can play a pivotal role in fostering such transparency, for instance by explicitly stating the underlying choices, assumptions, normative commitments, and expectations and by tracking the choices throughout the modelling process, which is potentially facilitated through protocols (Babel et al., 2019; Addor and Melsen, 2019; Krueger and Alba, 2022).
-
Democratise modelling. Give space to multiple bodies of knowledge and multiple stakeholders and incorporate marginalised voices of people and nature at all stages of the modelling. This includes questioning who and what are represented (and how) in the data, problem framing, mental model, and decision-making process (Lane et al., 2011; Godinez-Madrigal et al., 2019; Haeffner et al., 2021; Holifield, 2009; Jackson, 2006; Bremer et al., 2020, Voinov et al., 2016).
We present these five considerations as a starting point for modellers, commissioners, and users to think about the potential power-laden effects of modelling processes and to identify possibilities for altering the design of these processes or identifying alternatives. Our call should not be understood as a suggestion to do away with modelling in the water sector altogether but rather as an exploration of how to improve the practice. Although the proposed approach adds further complexity to the modelling process, it also opens up new possibilities for strengthening modelling processes, models, and their outcomes.
In this article we researched how the academic literature engages with the influence water models have. Driven by a hypothesis that there are few scientific articles that critically unpack or reflexively engage with the socially and ecologically different effects water models and related modelling processes have, we conducted a literature review based on the ROSES method to assess whether our assumption is correct, and secondly we identified which lessons we can draw from the existing literature. To contribute to overcoming disciplinary thinking, we made use of the open peer-review process of Hydrology and Earth System Sciences (HESS) and invited researchers and practitioners from a broad range of disciplines to think with us, share experiences and thoughts, and contribute the articles that we included in the analysis (Appendix A).
Of the 408 articles included in the systematic literature review, 27 were finally included in the critical appraisal. In addition, 30 articles were added to the critical appraisal during the review process and 4 as suggested by the HESS community. The 61 studies reveal how models shape and are shaped by the social and material aspects of the world we live in and how commissioners, modellers, users, and those affected engage with this. Over the years there has indeed been a limited but steady number of studies that have engaged with the influence of water models. The main reason for the exclusion of so many studies from the review is that, while most of them do mention a reflection on the potential impact of the model or an intention or expectation for the model to contribute to a more equitable and just world, these statements are mostly brief, disconnected from a specific context, and do not make explicit how the model did, or could, achieve these goals.
The 61 studies that are included in this review highlight different approaches to unpacking and critically engaging with the influence of water models. The studies show that shaping of models and by models happens in different ways throughout a modelling process, and they show how commissioners, modellers, users, and those affected are involved. The studies highlight the ways in which mental models and policy projects become embedded in a modelling process (including through data and categorisation), how modeller's choices (also impacted by familiarity, habits, standardisation, or institutional interests) have different effects on the models' outputs and the real world, and what impact the models have by legitimising specific understandings of the world and inclusive or exclusive procedures. A large number of studies also showcase how to intentionally and constructively engage with the potential influence of models by mindfully connecting to people and places, understanding the different realities of stakeholders that are modelled and measured, and making explicit how the model and modelling process represent people and places in fair ways.
This has led us to define a call for power-sensitive modelling in which we invite everyone engaged with modelling to work towards just and equitable water distributions, to have a holistic approach to modelling, to contextualise water modelling to engage with impacts, to be transparent, to foster broad accountability, and to democratise modelling. Studying and doing power-sensitive modelling requires a reflexive approach that is grounded and that builds on long-term collaborations and the recognition that modelling is a complex and multi-faceted process. To paraphrase Thompson and Smith (2019), this requires making explicit what happens within model land but also stepping out of it. As such research finds itself at a crossroads, cooperation across disciplinary boundaries is essential for nurturing generative reflexivity and accountability in relation to the power of models (Chilvers and Kearnes, 2015) as well as challenging or enriching modelling results with knowledge from non-modellers and especially those affected by decisions that are related to the modelling exercises (Wardropper et al., 2017; Hasala et al., 2020). Interdisciplinary research, where both certified and non-certified water experts engage with and challenge each other, seems essential (Krueger et al., 2016). This is challenging and is seen as a major obstacle in a professional world that does not value complexity but promotes disciplinary thinking (Melsen et al., 2018b; Srinivasan et al., 2018; Rusca and Di Baldassare, 2019). However, with this interdisciplinary analysis of water models, we hope to inspire others to engage in power-sensitive modelling and to consider how quantitative models may help to foster transformative pathways towards more just and equitable water distributions.
-
30 through a general search and personal collection
-
27 additional articles through the systematic review
-
4 through the HESS community and reviewers
Based on our assessment, “X” indicates that an article explicitly discusses (i) the mental models and policy projects, (ii) the influence of modellers' choices, (iii) the impact models have, and/or (iv) engagement with non-modellers.
“x” indicates that an article discusses one of the above-mentioned elements, but not explicitly.
The data used in this study are available in the Supplement.
The supplement related to this article is available online at: https://doi.org/10.5194/hess-28-4157-2024-supplement.
All the authors contributed to the conceptualisation and to the narrative review. RtH and JPV developed the query for the systematic literature review. RtH and RA developed the methodology. RtH did the data collection and analysis for the systematic literature review and wrote the original draft. JPV, RA, MR, DWW, and TK reviewed and edited the paper closely, and all the authors reviewed it.
Rossella Alba and Tobias Krueger are supported by funding from the Volkswagen Foundation through grant no. 96955 (Water Security for Whom?), which has no influence on the content of this paper.
Publisher's note: Copernicus Publications remains neutral with regard to jurisdictional claims made in the text, published maps, institutional affiliations, or any other geographical representation in this paper. While Copernicus Publications makes every effort to include appropriate place names, the final responsibility lies with the authors.
This article is the follow-up activity of the workshop “Towards a reflexive approach: Connecting critical research on water modelling”, co-organised by the Hydrology and Society group at IRI-THESys, Humboldt University of Berlin, and the Water Resources Management Group of Wageningen University on 19 and 20 January 2022. We thank all the participants for sharing their experiences and reflections on modelling waters. We also thank the reviewers and members of the HESS community for their constructive comments and engagement.
Rossella Alba and Tobias Krueger are supported by funding from the Volkswagen Foundation through grant no. 96955 (Water Security for Whom?).
This paper was edited by Thom Bogaard and reviewed by Stuart Lane, Keith Beven, and Maurits Ertsen.
Abbott, M. B. and Vojinovic, Z.: Towards a hydroinformatics praxis in the service of social justice, J. Hydroinform., 16, 516–530, https://doi.org/10.2166/hydro.2013.198, 2014.
Addor, N. and Melsen, L. A.: Legacy, Rather Than Adequacy, Drives the Selection of Hydrological Models, Water Resour. Res., 55, 378–390, https://doi.org/10.1029/2018WR022958, 2019.
Alam, M. F., McClain, M., Sikka, A., and Pande, S.: Understanding human–water feedbacks of interventions in agricultural systems with agent based models: A review, Environ. Res. Lett., 17, 103003, https://doi.org/10.1088/1748-9326/ac91e1, 2022.
Andersson, L.: Experiences of the use of riverine nutrient models in stakeholder dialogues, Int. J. Water Resour. D., 20, 399–413, https://doi.org/10.1080/0790062042000248547, 2004.
Babel, L. and Vinck, D.: The “sticky air method” in geodynamics: Modellers dealing with the constraints of numerical modelling, Rev. Anthropol. Connaiss., 16, https://doi.org/10.4000/rac.27795, 2022.
Babel, L., Vinck, D., and Karssenberg, D.: Decision-making in model construction: Unveiling habits, Environ. Modell. Softw., 120, 104490, https://doi.org/10.1016/j.envsoft.2019.07.015, 2019.
Beck, M. B.: Coping with ever larger problems, models, and data bases. Water Sci. Technol., 39, 1–11, https://doi.org/10.2166/wst.1999.0183, 1999.
Bergström, S.: Principles and confidence in hydrological modelling, Hydrol. Res., 22, 123–136, https://doi.org/10.2166/nh.1991.0009, 1991.
Beven, K.: Environmental modelling: an uncertain future?, CRC Press, 328 pp., https://doi.org/10.1201/9781482288575, 2009.
Beven, K.: How to make advances in hydrological modelling, Hydrol. Res., 50, 1481–1494, https://doi.org/10.2166/nh.2019.134, 2019.
Bijker, W.: Constructing Worlds: Reflections on Science, Technology and Democracy (and a Plea for Bold Modesty), Engag. Sci. Technol. Soc., 3, 315, https://doi.org/10.17351/ests2017.170, 2017.
Bijker, W. E., Hughes, T. P., and Pinch, T. (Eds.): The Social construction of technological systems: new directions in the sociology and history of technology, MIT Press, Cambridge, Mass, 405 pp., ISBN 0-262-02262-1, 1987.
Bouleau, G.: The co-production of science and waterscapes: The case of the Seine and the Rhône Rivers, France, Geoforum, 57, 248–257, https://doi.org/10.1016/j.geoforum.2013.01.009, 2014.
Bremer, L. L., Hamel, P., Ponette-González, A. G., Pompeu, P. V., Saad, S. I., and Brauman, K. A.: Who Are we Measuring and Modeling for? Supporting Multilevel Decision-Making in Watershed Management, Water Resour. Res., 56, e2019WR026011, https://doi.org/10.1029/2019WR026011, 2020.
Budds, J.: Contested H2O: Science, policy and politics in water resources management in Chile, Geoforum, 40, 418–430, https://doi.org/10.1016/j.geoforum.2008.12.008, 2009.
Cash, D., Clark, W. C., Alcock, F., Dickson, N., Eckley, N., and Jäger, J.: Salience, Credibility, Legitimacy and Boundaries: Linking Research, Assessment and Decision Making, SSRN Electron. J., https://doi.org/10.2139/ssrn.372280, 2003.
Clark, M. J.: Putting water in its place: A perspective on GIS in hydrology and water management, Hydrol. Process., 12, 823–834, https://doi.org/10.1002/(SICI)1099-1085(199805)12:6<823::AID-HYP656>3.0.CO;2-Z, 1998.
Chilvers, J. and Kearnes, M. (Eds.): Remaking participation: towards reflexive engagement, in: Remaking Participation: Science, Environment and Emergent Publics, Routledge, 28, https://doi.org/10.1177/0162243919850885, 2015.
Connor, L., Higginbotham, N., Freeman, S., and Albrecht, G.: Watercourses and Discourses: Coalmining in the Upper Hunter Valley, New South Wales, Oceania, 78, 76–90, https://doi.org/10.1002/j.1834-4461.2008.tb00029.x, 2008.
Constanza, R. and Ruth, M.: Using Dynamic Modeling to Scope Environmental Problems and Build Consensus, Environ. Manage., 22, 183–195, https://doi.org/10.1007/s002679900095, 1998.
Cornejo P., S. M. and Niewöhner, J.: How Central Water Management Impacts Local Livelihoods: An Ethnographic Case Study of Mining Water Extraction in Tarapacá, Chile, Water, 13, 3542, https://doi.org/10.3390/w13243542, 2021.
Cowardin, L., Carter, V., Golet, F. C., and LaRoe, E. T.: Classification of wetlands and deep water habitats in the United States, number FWS/OBS-79/31, US Fish and Wildlife Service, https://pubs.usgs.gov/publication/2000109 (last access: 27 August 2024), 1979.
Cronin, P., Ryan, F., and Coughlan, M.: Undertaking a literature review: a step-by-step approach, B. J. Nursing, 17, 38–43, https://doi.org/10.12968/bjon.2008.17.1.28059, 2008.
Dadson, S., Hall, J. W., Garrick, D., Sadoff, C., Grey, D., and Whittington, D.: Water security, risk, and economic growth: insights from a dynamical systems model, Water Resour. Res. 53, 6425–6438, https://doi.org/10.1002/2017WR020640, 2017.
de Oliveira Ferreira Silva, C.: The Challenge of Model Validation and Its (Hydrogeo)ethical Implications for Water Security, in: Computational Intelligence for Water and Environmental Sciences, edited by: Bozorg-Haddad, H. and Zolghadr-Asli, B., Springer Nature Singapore, 477–489, https://doi.org/10.1007/978-981-19-2519-1, 2022.
Deitrick, A. R., Torhan, S. A., and Grady, C. A.: Investigating the Influence of Ethical and Epistemic Values on Decisions in the Watershed Modeling Process, Water Resour. Res., 57, e2021WR030481, https://doi.org/10.1029/2021WR030481, 2021.
Demeritt, D.: The Construction of Global Warming and the Politics of Science, Ann. Am. Assoc. Geogr., 91, 307–337, https://doi.org/10.1111/0004-5608.00245, 2001.
Demeritt, D.: Science studies, climate change and the prospects for constructivist critique, Econ. Soc. 35, 453–479, https://doi.org/10.1080/03085140600845024, 2006.
Dobson, B., Wagener, T., and Pianosi, F.: How Important Are Model Structural and Contextual Uncertainties when Estimating the Optimized Performance of Water Resource Systems?, Water Resour. Res., 55, 2170–2193, https://doi.org/10.1029/2018WR024249, 2019.
Doorn, N.: Responsibility Ascriptions in Technology Development and Engineering: Three Perspectives, Sci. Eng. Ethics, 18, 69–90, https://doi.org/10.1007/s11948-009-9189-3, 2012.
Étienne, M.: Companion modelling: a participatory approach to support sustainable development, Editions Quae, 368 pp., https://doi.org/10.1007/978-94-017-8557-0, 2011.
Falconi, S. M. and Palmer, R. N.: An interdisciplinary framework for participatory modeling design and evaluation – What makes models effective participatory decision tools?, Water Resour. Res., 53, 1625–1645, https://doi.org/10.1002/2016WR019373, 2017.
Fernandez, S.: Much Ado About Minimum Flows…Unpacking indicators to reveal water politics, Geoforum, 57, 258–271, https://doi.org/10.1016/j.geoforum.2013.04.017, 2014.
Garcia-Cuerva, L., Berglund, E. Z., and Rivers, L.: Exploring Strategies for LID Implementation in Marginalized Communities and Urbanizing Watersheds, in: World Environmental and Water Resources Congress 2016, World Environmental and Water Resources Congress 2016, West Palm Beach, Florida, 22–26 May 2016, 41–50, https://doi.org/10.1061/9780784479889.005, 2016.
Godinez-Madrigal, J., Van Cauwenbergh, N., and van der Zaag, P.: Production of competing water knowledge in the face of water crises: Revisiting the IWRM success story of the Lerma-Chapala Basin, Mexico, Geoforum, 103, 3–15, https://doi.org/10.1016/j.geoforum.2019.02.002, 2019.
Golinski, J.: Making Natural Knowledge: Constructivism and the History of Science, University of Chicago Press, Chicago, 368 pp., https://doi.org/10.7208/chicago/9780226302324.001.0001, 2005.
Haas, P. M.: Introduction: Epistemic Communities and International Policy Coordination, Int. Organ., 46, 1–35, https://doi.org/10.1017/S0020818300001442, 1992.
Haddaway, N. R., Macura, B., Whaley, P., and Pullin, A. S.: ROSES RepOrting standards for Systematic Evidence Syntheses: pro forma, flow-diagram and descriptive summary of the plan and conduct of environmental systematic reviews and systematic maps, Environ. Evid., 7, 1–8, https://doi.org/10.1186/s13750-018-0121-7, 2018.
Haeffner, M., Jackson-Smith, D., and Flint, C. G.: Social Position Influencing the Water Perception Gap Between Local Leaders and Constituents in a Socio-Hydrological System, Water Resour. Res., 54, 663–679, https://doi.org/10.1002/2017WR021456, 2018.
Haeffner, M., Hellman, D., Cantor, A., Ajibade, I., Oyanedel-Craver, V., Kelly, M., Schifman, L., and Weasel, L.: Representation justice as a research agenda for socio-hydrology and water governance, Hydrolog. Sci. J., 66, 1611–1624, https://doi.org/10.1080/02626667.2021.1945609, 2021.
Haines, S.: Reckoning resources: Political lives of anticipation in Belize’s water sector, Sci. Tech. Studies, 32, 97–118, https://doi.org/10.23987/sts.64650, 2019.
Hamilton, S. H., Pollino, C. A., Stratford, D. S., Fu, B., and Jakeman, A. J.: Fit-for-purpose environmental modeling: Targeting the intersection of usability, reliability and feasibility, Environ. Modell. Softw., 148, 105278, https://doi.org/10.1016/j.envsoft.2021.105278, 2022.
Haraway, D.: Situated Knowledges: The Science Question in Feminism and the Privilege of Partial Perspective, Fem. Stud., 14, 575, https://doi.org/10.2307/3178066, 1988.
Harmel, R. D., Smith, P. K., Migliaccio, K. W., Chaubey, I., Douglas-Mankin, K. R., Benham, B., Shukla, S., Muñoz-Carpena, R., and Robson, B. J.: Evaluating, interpreting, and communicating performance of hydrologic/water quality models considering intended use: A review and recommendations, Environ. Modell. Softw., 57, 40–51, 2014.
Harvey, F. and Chrisman, N.: Boundary Objects and the Social Construction of GIS Technology, Environ. Plan. Econ. Space, 30, 1683–1694, https://doi.org/10.1068/a301683, 1998.
Hasala, D., Supak, S., and Rivers, L.: Green infrastructure site selection in the Walnut Creek wetland community: A case study from southeast Raleigh, North Carolina, Landscape Urban Plan., 196, 103743, https://doi.org/10.1016/j.landurbplan.2020.103743, 2020.
Holifield, R.: How to speak for aquifers and people at the same time: Environmental justice and counter-network formation at a hazardous waste site, Geoforum, 40, 363–372, https://doi.org/10.1016/j.geoforum.2008.02.005, 2009.
Holifield, R.: Environmental justice as recognition and participation in risk assessment: negotiating and translating health risk at a superfund site in Indian country, Ann. Assoc. Am. Geogr., 102, 591–613, https://doi.org/10.1080/00045608.2011.641892, 2012.
Holländer, H. M., Bormann, H., Blume, T., Buytaert, W., Chirico, G. B., Exbrayat, J.-F., Gustafsson, D., Hölzel, H., Krauße, T., Kraft, P., Stoll, S., Blöschl, G., and Flühler, H.: Impact of modellers' decisions on hydrological a priori predictions, Hydrol. Earth Syst. Sci., 18, 2065–2085, https://doi.org/10.5194/hess-18-2065-2014, 2014.
Jackson, S.: Water models and water politics: design, deliberation, and virtual accountability, Proc. Int. Conf. Digit. Gov. Res., San Diego California, USA, 21–24 May 2006, 95–104, https://doi.org/10.1145/1146598.1146632, 2006.
Jasanoff, S. and Kim, S.-H.: Containing the Atom: Sociotechnical Imaginaries and Nuclear Power in the United States and South Korea, Minerva, 47, 119–146, https://doi.org/10.1007/s11024-009-9124-4, 2009.
Jenkins, D. G. and McCauley, L. A.: GIS, SINKS, FILL, and Disappearing Wetlands: Unintended Consequences in Algorithm Development and Use, SAC'06: Proceedings of the 2006 ACM symposium on Applied computing, Dijon, France, 23–27 April 2006, 277–282, https://doi.org/10.1145/1141277.1141342, 2006.
Jensen, C. B.: A flood of models: Mekong ecologies of comparison, Soc. Stud. Sci., 50, 76–93, https://doi.org/10.1177/0306312719871616, 2020.
Junier, S. J.: Modelling expertise: Experts and expertise in the implementation of the Water Framework Directive in the Netherlands, Delft University of Technology, https://doi.org/10.4233/UUID:EEA8A911-F786-4158-A67E-B99663275BF8, 2017.
King, J. L. and Kraemer, K. L.: Models, Facts, and the Policy Process: The Political Ecology of Estimated Truth, Working Paper #URB-006, Center for Research on Information Systems and Organizations, University of California, Irvine, https://escholarship.org/uc/item/1c31s58g (last access: 16 July 2024), 1993.
Knorr-Cetina, K.: Epistemic cultures: how the sciences make knowledge, Harvard University Press, Cambridge, Massachusetts, 329 pp., ISBN 9780674258945, 1999.
Kouw, M.: Standing on the Shoulders of Giants – And Then Looking the Other Way? Epistemic Opacity, Immersion, and Modeling in Hydraulic Engineering, Perspect. Sci., 24, 206–227, https://doi.org/10.1162/POSC_a_00201, 2016.
Kouw, M.: Risks in the Making: The Mediating Role of Models in Water Management and Civil Engineering in the Netherlands, Ber. Wissgesch., 40, 160–174, https://doi.org/10.1002/bewi.201701823, 2017.
Kroepsch, A. C.: Groundwater Modeling and Governance: Contesting and Building (Sub)Surface Worlds in Colorado's Northern San Juan Basin, Engag. Sci. Technol. Soc., 4, 43–66, https://doi.org/10.17351/ests2018.208, 2018.
Krueger, T. and Alba, R.: Ontological and epistemological commitments in interdisciplinary water research: Uncertainty as an entry point for reflexion, Front. Water, 4, 1038322, https://doi.org/10.3389/frwa.2022.1038322, 2022.
Krueger, T., Maynard, C., Carr, G., Bruns, A., Mueller, E. N., and Lane, S.: A transdisciplinary account of water research, WIREs Water, 3, 369–389, https://doi.org/10.1002/wat2.1132, 2016.
Laborde, S.: Environmental Research from Here and There: Numerical Modelling Labs as Heterotopias, Environ. Plann. D, 33, 265–280, https://doi.org/10.1068/d14128p, 2015.
Landström, C., Whatmore, S. J., and Lane, S. N.: Virtual engineering: computer simulation modelling for flood risk management in England, Sci. Technol. Stud., 24, 3–22, 2011a.
Landström, C., Whatmore, S. J., Lane, S. N., Odoni, N., Ward, N., and Bradley, S.: Coproducing flood risk knowledge: redistributing expertise in critical “participatory modelling,” Environ. Plan. Econ. Space, 43, 1616–1633, 2011b.
Lane, S. N.: Making mathematical models perform in geographical space(s), in: Handbook of Geographical Knowledge, Chap. 17, edited by: Agnew, J. and Livingstone, D., Sage, London, https://doi.org/10.4135/9781446201091, 2012.
Lane, S. N.: Acting, predicting and intervening in a socio-hydrological world, Hydrol. Earth Syst. Sci., 18, 927–952, https://doi.org/10.5194/hess-18-927-2014, 2014.
Lane, S. N., Landström, C., and Whatmore, S. J.: Imagining flood futures: risk assessment and management in practice, Philos. T. R. Soc. A, 369, 1784–1806, https://doi.org/10.1098/rsta.2010.0346, 2011a.
Lane, S. N., Odoni, N., Landström, C., Whatmore, S. J., Ward, N., and Bradley, S.: Doing flood risk science differently: an experiment in radical scientific method, T. I. Brit. Geogr., 36, 15–36, 2011b.
Lane, S. N., November, V., Landström, C., and Whatmore, S.: Explaining rapid transitions in the practice of flood risk management, Ann. Assoc. Am. Geogr., 103, 330–342, https://doi.org/10.1080/00045608.2013.754689, 2013.
Lasswell, H. D.: Politics: Who Gets What, When, How, Whittlesey House, Cleveland, New York, 264, https://doi.org/10.1007/978-3-531-90400-9_60, 1936.
Latour, B.: When things strike back: a possible contribution of “science studies” to the social sciences, Brit. J. Sociol., 51, 107–123, 2000.
Latour, B.: The promises of constructivism, in: Chasing Technology: Matrix of Materiality, Indiana Series for the Philosophy of Science, edited by: Ihde, D., Indiana University Press, 27–46, https://sciencespo.hal.science/hal-01027765 (last access: 16 July 2024), 2003.
Latour, B. and Woolgar, S.: Laboratory life: the construction of scientific facts, Princeton University Press, Princeton, NJ, 294 pp., ISBN 9780691028323, 1986.
Law, J.: After method: Mess in social science research, Routledge, Oxon, United Kingdom, ISBN 0-203-48114-3, 2004.
Linton, J. and Budds, J.: The hydrosocial cycle: Defining and mobilizing a relational-dialectical approach to water, Geoforum, 57, 170–180, https://doi.org/10.1016/j.geoforum.2013.10.008, 2014.
Losee, R. M.: A discipline independent definition of information, J. Am. Soc. Inform. Sci., 48, 254–269, https://doi.org/10.1002/(SICI)1097-4571(199703)48:3<254::AID-ASI6>3.0.CO;2-W, 1997.
MacKenzie, D.: An engine, not a camera: How financial models shape markets, The MIT Press, Cambridge, 392 pp., https://doi.org/10.7551/mitpress/9780262134606.001.0001, 2006.
MacKenzie, D. and Wajcman, J. (Eds.): The social shaping of technology, 2nd edn., Open University Press, Buckingham, Philadelphia, 462 pp., ISBN 9780335199143, 1999.
Macnaghten, P.: Governing Science and Technology: From the Linear Model to Responsible Research and Innovation, in: The Cambridge Handbook of Environmental Sociology, edited by: Legun, K., Keller, J., Bell, M., and Carolan, M., Cambridge University Press, 347–361, https://doi.org/10.1017/9781108554510.023, 2020.
Maeda, E. E., Haapasaari, P., Helle, I., Lehikoinen, A., Voinov, A., and Kuikka, S.: Black Boxes and the Role of Modeling in Environmental Policy Making, Front. Environ. Sci., 9, 629336, https://doi.org/10.3389/fenvs.2021.629336, 2021.
Meenar, M., Fromuth, R., and Soro, M.: Planning for watershed-wide flood-mitigation and stormwater management using an environmental justice framework, Environmental Practice, 20, 55–67, 2018.
Melsen, L. A.: It Takes a Village to Run a Model – The Social Practices of Hydrological Modeling, Water Resour. Res., 58, e2021WR030600, https://doi.org/10.1029/2021WR030600, 2022.
Melsen, L. A., Addor, N., Mizukami, N., Newman, A. J., Torfs, P. J. J. F., Clark, M. P., Uijlenhoet, R., and Teuling, A. J.: Mapping (dis)agreement in hydrologic projections, Hydrol. Earth Syst. Sci., 22, 1775–1791, https://doi.org/10.5194/hess-22-1775-2018, 2018a.
Melsen, L. A., Vos, J., and Boelens, R.: What is the role of the model in socio-hydrology? Discussion of “Prediction in a socio-hydrological world,” Hydrolog. Sci. J., 63, 1435–1443, https://doi.org/10.1080/02626667.2018.1499025, 2018b.
Melsen, L. A., Teuling, A. J., Torfs, P. J. J. F., Zappa, M., Mizukami, N., Mendoza, P. A., Clark, M. P., and Uijlenhoet, R.: Subjective modeling decisions can significantly impact the simulation of flood and drought events, J. Hydrol., 568, 1093–1104, https://doi.org/10.1016/j.jhydrol.2018.11.046, 2019.
Mendoza, P. A., Clark, M. P., Mizukami, N., Gutmann, E. D., Arnold, J. R., Brekke, L. D., and Rajagopalan, B.: How do hydrologic modeling decisions affect the portrayal of climate change impacts?, Hydrol. Process., 30, 1071–1095, https://doi.org/10.1002/hyp.10684, 2016.
Morgan, M. S. and Morrison, M. (Eds.): Models as mediators: Perspectives on natural and social science, Cambridge University Press, Cambridge, 401 pp., https://doi.org/10.1017/CBO9780511660108, 1999.
Munk, A. K.: Risking the Flood: Cartographies of Things to Come, University of Oxford, Oxford, 268 pp., uuid:55c2df2e-3506-4a93-8cab-37f133866182, 2010.
Nearing, G. S., Kratzert, F., Sampson, A. K., Pelissier, C. S., Klotz, D., Frame, J. M., Prieto, C., and Gupta, H. V.: What role does hydrological science play in the age of machine learning?, Water Resour. Res., 57, e2020WR028091, https://doi.org/10.1029/2020WR028091, 2021.
Odoni, N. A. and Lane, S. N.: Knowledge-theoretic models in hydrology, Prog. Phys. Geog., 34, 151–171, https://doi.org/10.1177/0309133309359893, 2010.
Opitz-Stapleton, S. and MacClune, K.: Scientific and Social Uncertainties in Climate Change: The Hindu Kush-Himalaya in Regional Perspective, Chap. 11, in: Community, Environment and Disaster Risk Management, vol. 11, edited by: Lamadrid, A. and Kelman, I., Emerald Group Publishing Limited, 207–237, https://doi.org/10.1108/S2040-7262(2012)0000011017, 2012.
Packett, E., Grigg, N. J., Wu, J., Cuddy, S. M., Wallbrink, P. J., and Jakeman, A. J.: Mainstreaming gender into water management modelling processes, Environ. Modell. Softw., 127, 104683, https://doi.org/10.1016/j.envsoft.2020.104683, 2020.
Petticrew, M. and Roberts, H.: Systematic reviews in the social sciences: A practical guide, Blackwell Publishing Ltd, Malden, USA, 352 pp., ISBN 978-1-4051-2110-1, 2006.
Pielke Jr., R. A.: The Role of Models in Prediction for Decision, in: Models in Ecosystem Science, edited by: Canham, C. D., Cole, J. J., and Lauenroth, W. K., Princeton University Press, 113–137, ISBN: 9780691092898, 2003.
Puy, A., Sheikholeslami, R., Gupta, H. V., Hall, J. W., Lankford, B., Lo Piano, S., Meier, J., Pappenberger, F., Porporato, A., Vico, G., and Saltelli, A.: The delusive accuracy of global irrigation water withdrawal estimates, Nat. Commun., 13, 3183, https://doi.org/10.1038/s41467-022-30731-8, 2022.
Rainwater, K., Stovall, J., Frailey, S., and Urban, L.: Transboundary Impacts on Regional Ground Water Modeling in Texas, Groundwater, 43, 706–716, https://doi.org/10.1111/j.1745-6584.2005.00068.x, 2005.
Ramsey, K.: GIS, modeling, and politics: On the tensions of collaborative decision support, J. Environ. Manage., 90, 1972–1980, https://doi.org/10.1016/j.jenvman.2007.08.029, 2009.
Refsgaard, J. C. and Henriksen, H. J.: Modelling Guidelines – Terminology and Guiding Principles, Adv. Water. Resour., 27, 71–82, https://doi.org/10.1016/j.advwatres.2003.08.006, 2004.
Rusca, M. and Di Baldassarre, G.: Interdisciplinary Critical Geographies of Water: Capturing the Mutual Shaping of Society and Hydrological Flows, Water, 11, 1973, https://doi.org/10.3390/w11101973, 2019.
Rusca, M., Mazzoleni, M., Barcena, A., Savelli, E., and Messori, G.: Speculative Political Ecologies: (re)imagining urban futures of climate extremes, J. Polit. Ecol., 30, 581–608, https://doi.org/10.2458/jpe.4827, 2023.
Saltelli, A. and Di Fiore, M. (Eds.): The Politics of Modelling: Numbers Between Science and Policy, Oxford University Press, 231 pp., ISBN 9780198872412, 2023.
Saltelli, A., Bammer, G., Bruno, I., Charters, E., Di Fiore, M., Didier, E., Nelson Espeland, W., Kay, J., Lo Piano, S., Mayo, D., and Pielke Jr., R.: Five ways to ensure that models serve society: a manifesto, Nature, 582, 482–484, https://doi.org/10.1038/d41586-020-01812-9, 2020.
Sanz, D., Vos, J., Rambags, F., Hoogesteger, J., Cassiraga, E., and Gómez-Alday, J. J.: The social construction and consequences of groundwater modelling: insight from the Mancha Oriental aquifer, Spain, Int. J. Water Resour. D., 35, 808–829, https://doi.org/10.1080/07900627.2018.1495619, 2019.
Shapiro, C.: Coordination and integration of wetland data for status and trend and inventory estimates, Technical Report 2, Federal Geographic Data Committee, Wetlands Subcommittee, 210 pp., https://www.govinfo.gov/content/pkg/CZIC-qh545-a1-c88-1995/html/CZIC-qh545-a1-c88-1995.htm (last access: 27 August 2024), 1995.
Shrader-Frechette, K.: Hydrogeology and framing questions having policy consequences, Philos. Sci., 64, S149–S160, https://doi.org/10.1086/392595, 1997.
Sismondo, S.: Models, Simulations, and Their Objects, Sci. Context, 12, 247–260, https://doi.org/10.1017/S0269889700003409, 1999.
Sivapalan, M., Savenije, H. H. G., and Blöschl, G.: Socio-hydrology: A new science of people and water, Hydrol. Process., 26, 1270–1276, https://doi.org/10.1002/hyp.8426, 2011.
Srinivasan, V., Sanderson, M., Garcia, M., Konar, M., Blöschl, G., and Sivapalan, M.: Prediction in a socio-hydrological world, Hydrolog. Sci. J., 62, 1–8, https://doi.org/10.1080/02626667.2016.1253844, 2016.
Srinivasan, V., Sanderson, M., Garcia, M., Konar, M., Blöschl, G., and Sivapalan, M.: Moving socio-hydrologic modelling forward: unpacking hidden assumptions, values and model structure by engaging with stakeholders: reply to “What is the role of the model in socio-hydrology?,” Hydrolog. Sci. J., 63, 1444–1446, https://doi.org/10.1080/02626667.2018.1499026, 2018.
Stengers, I.: Another science is possible: A manifesto for slow science, John Wiley & Sons, ISBN 978-1-509-52181-4, 2018.
Thompson, E. L. and Smith, L. A.: Escape from model-land, Economics, 13, 20190040, https://doi.org/10.5018/economics-ejournal.ja.2019-40, 2019.
Trombley, J. M.: An Environmental Anthropology of Modeling and Management on the Chesapeake Bay Watershed, PhD thesis, University of Maryland, College Park, https://doi.org/10.13016/M2CV4BS14, 2017.
Turner, M. D.: Production of environmental knowledge: Scientists, complex natures, and the question of agency, in: Knowing Nature: Conversations at the Intersection of Political Ecology and Science Studies, edited by: Goldman, M. J., Nadasdy, P., and Turner, M. D., University of Chicago Press, Chicago, 25–29, ISBN 9780226301402, 2011.
Turnhout, E., Hisschemöller, M., and Eijsackers, H.: Ecological indicators: between the two fires of science and policy, Ecol. Indic., 7, 215–228, https://doi.org/10.1016/j.ecolind.2005.12.003, 2007.
Venot, J.-P., Vos, J., Molle, F., Zwarteveen, M., Veldwisch, G. J., Kuper, M., Mdee, A., Ertsen, M., Boelens, R., Cleaver, F., Lankford, B., Swatuk, L., Linton, J., Harris, L. M., Kemerink-Seyoum, J., Kooy, M., and Schwartz, K.: A bridge over troubled waters, Nat. Sustain., 5, 92, https://doi.org/10.1038/s41893-021-00835-y, 2022.
Voinov, A., Seppelt, R., Reis, S., Nabel, J. E., and Shokravi, S.: Values in socio-environmental modelling: Persuasion for action or excuse for inaction. Environ. Modell. Softw., 53, 207–212, https://doi.org/10.1016/j.envsoft.2013.12.005, 2014.
Voinov, A., Kolagani, N., McCall, M. K., Glynn, P. D., Kragt, M. E., Ostermann, F. O., Pierce, S. A., and Ramu, P.: Modelling with stakeholders – Next generation, Environ. Modell. Softw., 77, 196–220, https://doi.org/10.1016/j.envsoft.2015.11.016, 2016.
Wardropper, C. B., Gillon, S., and Rissman, A. R.: Uncertain monitoring and modeling in a watershed nonpoint pollution program, Land Use Policy, 67, 690–701, https://doi.org/10.1016/j.landusepol.2017.07.016, 2017.
Wesselink, A., de Vriend, H., Barneveld, H., Krol, M., and Bijker, W.: Hydrology and hydraulics expertise in participatory processes for climate change adaptation in the Dutch Meuse, Water Sci. Technol., 60, 583–595, 2009.
Wesselink, A., Kooy, M., and Warner, J.: Socio-hydrology and hydrosocial analysis: toward dialogues across disciplines, WIREs Water, 4, e1196, https://doi.org/10.1002/wat2.1196, 2017.
Whatmore, S. J. and Landström, C.: Manning's N: Putting roughness to work, in: How well do facts travel?: The dissemination of reliable knowledge, edited by: Howlett, P. and Morgan, M. S., Cambridge University Press, 111–135, ISBN 9780521159586, 2010.
Wheeler, K. G., Hall, J. W., Abdo, G. M., Dadson, S. J., Kasprzyk, J. R., Smith, R., and Zagona, E. A.: Exploring Cooperative Transboundary River Management Strategies for the Eastern Nile Basin, Water Resour. Res., 54, 9224–9254, https://doi.org/10.1029/2017WR022149, 2018a.
Wheeler, K. G., Robinson, C. J., and Bark, R. H.: Modelling to bridge many boundaries: the Colorado and Murray-Darling River basins, Reg. Environ. Change, 18, 1607–1619, https://doi.org/10.1007/s10113-018-1304-z, 2018b.
Woolgar, S. and Cooper, G.: Do artefacts have ambivalence: Moses' bridges, Winner's bridges and other urban legends in S&TS, Soc. Stud. Sci., 29, 433–449, https://doi.org/10.1177/030631299029003005, 1999.
Zwarteveen, M., Kemerink-Seyoum, J. S., Kooy, M., Evers, J., Guerrero, T. A., Batubara, B., Biza, A., Boakye-Ansah, A., Faber, S., Cabrera Flamini, A., Cuadrado-Quesada, G., Fantini, E., Gupta, J., Hasan, S., ter Horst, R., Jamali, H., Jaspers, F., Obani, P., Schwartz, K., Shubber, Z., Smit, H., Torio, P., Tutusaus, M., and Wesselink, A.: Engaging with the politics of water governance, WIREs Water, 4, e01245, https://doi.org/10.1002/wat2.1245, 2017.
- Abstract
- Introduction
- Defining models, modelling, and their influence
- Methodology
- Results: narrative synthesis
- Discussion
- Towards power-sensitive modelling
- Conclusion
- Appendix A: Final list of the 61 articles included in the review that explicitly engage with and reflect on the power of water models
- Data availability
- Author contributions
- Competing interests
- Disclaimer
- Acknowledgements
- Financial support
- Review statement
- References
- Supplement
- Abstract
- Introduction
- Defining models, modelling, and their influence
- Methodology
- Results: narrative synthesis
- Discussion
- Towards power-sensitive modelling
- Conclusion
- Appendix A: Final list of the 61 articles included in the review that explicitly engage with and reflect on the power of water models
- Data availability
- Author contributions
- Competing interests
- Disclaimer
- Acknowledgements
- Financial support
- Review statement
- References
- Supplement