the Creative Commons Attribution 4.0 License.
the Creative Commons Attribution 4.0 License.
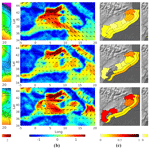
Synoptic weather patterns conducive to compound extreme rainfall–wave events in the NW Mediterranean
Juan C. Peña
Sotiris Assimenidis
José A. Jiménez
The NW Mediterranean coast is highly susceptible to the impacts of extreme rainstorms and coastal storms, which often lead to flash floods, coastal erosion, and flooding across a highly urbanised territory. Often, these storms occur simultaneously, resulting in compound events that intensify local impacts when they happen in the same location or spread impacts across the territory when they occur in different areas. These multivariate and spatially compound events present significant challenges for risk management, potentially overwhelming emergency services. In this study, we analysed the prevailing atmospheric conditions during various types of extreme episodes, aiming to create the first classification of synoptic weather patterns (SWPs) conducive to compound events involving heavy rainfall and storm waves in the Spanish NW Mediterranean. To achieve this, we developed a methodological framework that combines an objective synoptic classification method based on principal component analysis and k-means clustering with a Bayesian network. This methodology was applied to a dataset comprising 562 storm events recorded over 30 years, including 112 compound events. First, we used the framework to determine the optimal combination of domain size, classification variables, and number of clusters based on the synoptic skill to replicate local-scale values of daily rainfall and significant wave height. Subsequently, we identified SWPs associated with extreme compound events, which are often characterised by upper-level lows and trough structures in conjunction with Mediterranean cyclones, resulting in severe to extreme coastal storms combined with convective systems. The obtained classification demonstrated strong skill, with scores exceeding 0.4 when considering factors like seasonality or the North Atlantic Oscillation. These findings contribute to a broader understanding of compound terrestrial–maritime extreme events in the study area and have the potential to aid in the development of effective risk management strategies.
- Article
(12053 KB) - Full-text XML
-
Supplement
(2552 KB) - BibTeX
- EndNote
The NW Mediterranean coast frequently experiences flash floods during heavy rainfall episodes (Llasat and Puigcerver, 1992; Llasat et al., 2014; Gaume et al., 2016) and coastal storms when high waves erode and flood the coastal fringe (Mendoza et al., 2011; Jiménez et al., 2012). When these hazards coincide, they result in compound extreme events that can have a significant impact regionally. These compound events increase the local impact where both hazards concur at the same basin, and they can also distribute the impact widely across the territory when they occur simultaneously in different areas (Zscheischler et al., 2020). For instance, the recent Storm Gloria in January 2020 had substantial repercussions in Catalonia (NW Mediterranean, Spain). This storm led to four casualties, structural damage in different breakwaters along the coast, a railway bridge collapse, and impacts associated with extreme waves, rainfall, and wind action distributed throughout the territory (Canals and Miranda, 2020). The Spanish national reinsurance company Consorcio de Compensación de Seguros paid EUR 143 million for storm-induced damage, which was limited to insured property (Luján-López, 2022). Managing the risk associated with such events is challenging due to the intensity of the observed damage and their extensive spatial coverage, which can overwhelm protection services (e.g. during Storm Gloria, civil protection services received approximately 15 000 calls and performed 2510 services in 4 d, as reported by the Ministry of Home Affairs of the Government of Catalonia).
Compound coastal floods have gained increasing attention in recent years (Wahl et al., 2015; Wu et al., 2018; Bevacqua et al., 2019; Hendry et al., 2019; Camus et al., 2022). However, despite their high relevance in the NW Mediterranean, these events have predominantly been studied within large-scale (continental) analyses of the multivariate type, where different hazards act simultaneously at the same location (Paprotny et al., 2018; Zscheischler et al., 2018, Bevacqua et al., 2019). A recent analysis of compound events in the NW Mediterranean found that they occur relatively frequently, approximately 3.4 times per year, and are often spatially compound, involving the simultaneous occurrence of heavy rainfall and storm waves in different parts of the region, while a few areas show concentrations of multivariate events (Sanuy et al., 2021). Assessing the magnitude of the impact and associated risks involves considering the intensity of the involved hazards and their distribution over the territory, which need to be combined with the level of exposure and vulnerability of affected areas (e.g. Kron, 2013).
Since these hazards are influenced by specific weather conditions, identifying meteorological patterns conducive to extreme events becomes crucial. This knowledge can be used to indirectly estimate their probability of occurrence (Catto and Dowdy, 2021; Camus et al., 2022) and can therefore become a relevant element in risk management. Various methods have been used for weather classification, ranging from manual procedures (Hess and Brezowky, 1969; Lamb, 1972) and correlation-based map typing (Yarnal, 1993; Wu et al., 2018) to more objective approaches such as principal components and clustering (e.g. Huth et al., 2008). The EU COST 733 project (Huth, 2010) has significantly contributed to advancing scalable classification techniques applicable to various European regions. Several classification methodologies were proposed and rigorously compared within this project, which highlighted that different classification approaches demonstrate comparable effectiveness (e.g., Philipp et al., 2010, Beck and Philipp, 2010). Notably, the synoptic skill of weather classifications, i.e. their capacity to accurately replicate the magnitudes of key target variables at the local scale, was identified as being particularly sensitive to various methodological aspects inherent to objective approaches. These encompassed factors such as the predefined number of classification groups, the selection and number of atmospheric variables, and the spatial dimensions of the classification domain (see e.g. Philipp et al., 2016; Beck et al., 2016; Teegavarapu and Goly, 2018; Falkena et al., 2020). In this context, Bayesian networks (BNs) have been employed to assess the dependencies between multiple nonlinearly related variables in natural hazard analysis (Beuzen et al., 2018; Plant et al., 2016; Couasnon et al., 2018), and they can therefore be used to evaluate the synoptic skill of objective weather classification methods in reproducing local-scale variability in target variables. Additionally, BNs provide a framework for assessing conditioned probabilities based on weather patterns, their climatological factors, and their characteristics at the local scale (e.g. Sanuy et al., 2021).
While the dominant weather patterns during individual rainfall and coastal storms in the NW Mediterranean have been comprehensively identified (Martín-Vide et al., 2008; Mendoza et al., 2011; Gilabert and Llasat, 2017; Gil-Guirado et al., 2021), synoptic weather patterns (SWPs) conducive to compound events involving both hazards have not been thoroughly studied in this region. Recent analysis of concurrent intense precipitation and extreme wind events over the Iberian Peninsula identified their link with cyclones and associated atmospheric rivers; however, the results suggested that the climatology of extreme weather events in the NE sectors (the NW Mediterranean coast) is driven by different mechanisms relative to other sectors (Hénin et al., 2021). Mediterranean cyclones have been highlighted as significant contributors to extreme precipitation and coastal storm impacts (Flaounas et al., 2022). Inter-annual variations in wave height during coastal storms are predominantly influenced by seasonality (Morales-Márquez et al., 2020), while those in extreme precipitation are more closely tied to the North Atlantic Oscillation (NAO) (Casanueva et al., 2014; Krichak et al., 2014; Criado-Aldeanueva and Soto-Navarro, 2020). The complex orography of the region influences precipitation and wind patterns, and the coastal storm climate is characterised by the more significant role played by waves in erosion and flooding than surges (Mendoza and Jiménez, 2009; Sanuy et al., 2020).
To investigate the meteorological conditions under which compound events involving heavy rainfall and storm waves occur in the NW Mediterranean and to understand their impact on contributing hazards and how they vary across the territory, we classified the weather conditions during recorded storm events, both univariate and compound, spanning 30 years (1990–2020). We utilised principal component analysis (PCA) in combination with k-means clustering as an objective classification approach, considering multiple combinations of atmospheric variables, domains and number of clusters. The selected atmospheric variables included mean sea level pressure (mslp), 500 hPa geopotential height (z500), 850 hPa temperature (t850), and 10 m wind fields (u,v). Initially, the BN prediction skill is used to identify the most suitable combination of domain, variables, and number of clusters for individual and compound events. Subsequently, we relate the obtained patterns to storm characteristics across the region by calculating BN conditioned probabilities. This study represents the first classification and characterisation of weather patterns conducive to compound events on the NW Mediterranean coast.
2.1 Study area
The study area encompasses the approximately 600 km long coastline of Catalonia in the NW Mediterranean, including its river basins (Fig. 1). The presence of many small torrential catchments combined with the orographic forcing of Mediterranean air masses contribute to convective instability, altering pressure fields and causing intense rainfall and flash floods (Jansà et al., 2014). Hydrological and morphological factors have been shown to significantly influence flash flood occurrences (Llasat and Puigcerver, 1992; Llasat et al., 2014). Coastal storms in the area are dominated by NE–E extreme waves, with secondary impacts from the S–SE (Mendoza et al., 2011). Coastal flooding primarily results from wave-induced run-up, while storm surges play a secondary role in inundation in this part of the NW Mediterranean (Mendoza and Jiménez, 2009; Sanuy et al., 2020). Over recent decades, the region has experienced a shoreline retreat due to reduced sediment supply from rivers and the presence of numerous obstacles disrupting natural littoral dynamics (Jiménez and Valdemoro, 2019). Consequently, wave action on a progressively narrowing coastline has led to a significant increase in coastal damage (Jiménez et al., 2012).
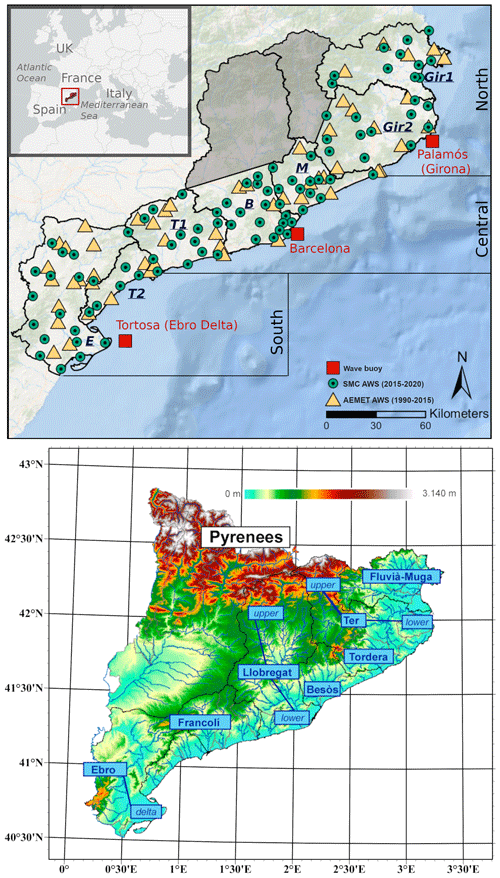
Figure 1Top: location of the study area and a map showing the distribution of dataset nodes in river basins and coastal sectors. Rainfall data were recorded at automatic weather stations (AWSs; circles and squares), while wave data were recorded by three wave buoys (red squares). Bottom: topography and main rivers at the study site. Maps were created with ESRI-ArcGIS base maps and elevation data were provided by Institud Cartogràfic i Geológic de Catalunya.
The terrestrial part of the study area was divided into seven sectors of natural river catchments along the coast (hereafter called basins), based on the literature (Llasat et al., 2016; Sanuy et al., 2021). This subdivision allowed for a focused analysis of direct pluvial hazards occurring in the lower parts of the basins while excluding the upper parts of long and regulated river catchments, such as the Ter in Girona and the Llobregat in Barcelona (Fig. 1). Moreover, the marine part was divided into three large sectors covering the main wave climate areas of the coast: north, central, and south. Each wave sector characterised the corresponding waves in more than one basin (Fig. 1).
2.2 Data
2.2.1 Rain
Rainfall data for the study area were collected by automatic weather stations (AWSs) from two networks distributed throughout the study area and covering the coastal basins of interest (Fig. 1 and Table 1). The datasets included daily cumulative rainfall for 1990–2015 recorded by the Spanish State Meteorology Agency (AEMET) and 30 min data for 2015–2020 from the Meteorological Service of Catalonia (SMC), which were converted to daily cumulative precipitation before merging.
2.2.2 Waves
The wave data consisted of a reconstruction of the significant wave height (Hs) and peak period (Tp) for the Palamós, Barcelona, and Tortosa wave buoys (Fig. 1). The reconstruction process was based on ERA5 data (SMC, 2021) and utilised a multilinear regression technique employing five oceanic variables (significant wave height, total wave mean period, mean wave period based on the first moment, mean zero-crossing wave period, and total wave peak period) and three atmospheric variables (mean sea level pressure, wind speed and wind direction at 10 m) as predictors for the targeted buoy variables (Hs or Tp). To account for the influence of wind and the morphology of the Catalan coast, the data was categorised into four groups based on wind direction (0 to 90∘, 90 to 180∘, 180 to 270∘, and 270 to 360∘), resulting in distinct regression coefficients for each group.
2.2.3 Atmospheric variables
The synoptic classifications were based on ERA5 data (Hersbach et al., 2023a, b) and comprised various combinations of meteorological parameters, including mslp, z500, t850 , and both components of the 10 m wind (u and v). These data span from 1990–2020 and cover a synoptic scale extending from 25∘ W to 30∘ E longitude and 30 to 65∘ N latitude, with a spatial resolution of and a temporal resolution of 3 h.
Monthly mean NAO time series data for the same period were obtained from the NOAA Climate Prediction Center (CPC) website (https://www.cpc.ncep.noaa.gov/products/precip/CWlink/pna/nao.shtml, last access: July 2023).
3.1 General framework
The developed methodology consists of three main steps (Fig. 2):
- a.
Event identification. This step involves identifying and characterising rain and wave storm events as individual or compound events based on the presence of either or both meteo-marine drivers.
- b.
Weather classifications. Here, synoptic weather patterns were identified by using a PCA and k-means approach, utilising multiple atmospheric variables, domains, and varying cluster sizes.
- c.
BN analysis. In this step, a BN is employed to select an appropriate combination of domain, classification variables, and number of clusters (N). The BN was also employed to identify critical SWPs and to characterise the probabilistic spatial distribution of intensity and the local simultaneity of compound events.
To evaluate the synoptic classifications, different domains and variable combinations were defined, as the classification skill is sensitive to these factors (Beck et al., 2016). The largest domain (D0) corresponds to the downloaded synoptic scale. Additional regions were defined (Fig. 3 and Table 2), and the synoptic skill in estimating basin-scale wave heights and daily rainfalls across these domains were compared. The k-means approach involved various combinations of atmospheric variables (Table 2), including the use of each individual variable as a predictor as well as groups of two to four selected from those that individually performed the best. Consequently, Step b (Sect. 3.3) and the BN skill analysis (Sect. 3.4) were conducted for each of the three event types identified in Step a. Furthermore, this analysis was performed for each combination of domain, k-means variable set, and number of clusters (Table 2).
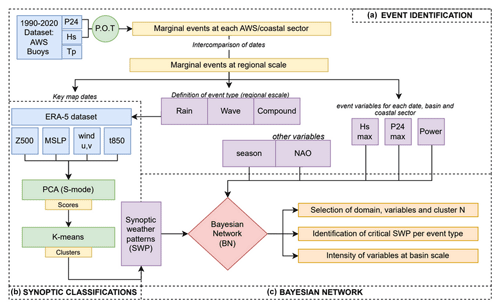
Figure 2General methodological framework. (a) Both compound and individual events are identified and characterised based on their peak conditions. (b) The PCA + k-means approach is applied to both compound and individual events with varying combinations of domain size, classification variables, and number of clusters. (c) The Bayesian network is used to assess the synoptic skill in estimating P24 and Hs at the local scale. Once the appropriate combination is determined, the BN is used to identify critical SWPs based on their intensity and the spatial distribution of extreme conditions at the local scale.
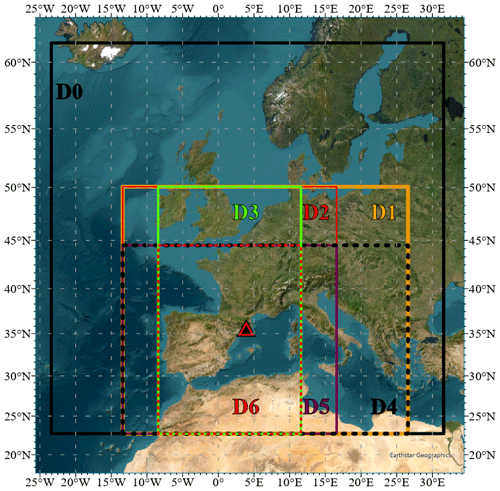
Figure 3Domain sizes tested in the classification analysis. The triangle indicates the location of the study area.
3.2 Event identification
In each basin, rain and coastal storm individual events were identified using the peak over threshold (POT) method for all stations within the basin. Rainstorms were identified with a P24 (daily precipitation) threshold value of 40 mm and a 3 d interval to establish independent events. This threshold has been identified as the lower limit for events that could potentially lead to flash floods if they are locally convective (Barbería et al., 2014; Cortès et al., 2018). For each event in a basin, the maximum P24 at any station and the accumulated P24 at that station were retained, resulting in a single time series of rainstorms per basin. Wave storms were identified using a dual-threshold approach (Sanuy et al., 2020) with a 0.98 quantile, a minimum duration of 6 h, and a 3 d lag to identify independent events and define their duration. The second threshold, set at the 0.995 quantile, was used to retain only extreme episodes. The absolute thresholds for the 0.995 / 0.98 quantiles, aggregating data from the three wave datasets, were 2.9 / 2.2 m, respectively. Each event was characterised by its maximum Hs and power content, approximated as the integration of Hs2 × Tp over the storm duration. Also, the season and the monthly average NAO corresponding to each event was saved to be later used in the BN analysis.
The final dataset of storms included three event types at the regional scale: (i) compound events in which rain and wave events were both present at any of the basins; (ii) rain events, as characterised by a rainstorm of P24≥40 mm in at least one basin without the co-occurrence of a wave storm in any coastal sector; and (iii) wave events, as characterised by the absence of a rainfall event in any basin but with a wave storm in any of the coastal sectors. For the compound events, further classification at the basin scale was done to distinguish between multivariate events (both rain and waves in the same basin), compounding wave events (basin affected only by waves), and compounding rain events (basin affected only by rain). Sanuy et al. (2021) emphasised the importance of this classification proposed by Zscheischler et al. (2020) for risk management during compound events in NW Mediterranean conditions.
Storm events, both individual and compound, underwent basic exploratory analysis to determine their annual and seasonal frequencies and average magnitudes.
3.3 SWP classification
To identify weather patterns, we employed an objective methodology previously used in the area by Aran et al. (2011) and Gil-Guirado et al. (2021) and consisting of two main steps: PCA and cluster analysis (CA). We applied the PCA + CA approach separately to rain, wave, and compound events identified in the previous step using the +00:00 UTC maps corresponding to each event's peak (maximum P24 for the rain events and maximum Hs for wave and compound events). This classification procedure was applied across all seven domains.
PCA was used to reduce the dimensionality of mslp, z500, t850, and wind (u,v) data within each domain (Table 2). Additionally, we applied PCA, separately for each variable, to the standardised anomalies of the maps. The determination of the number of principal components aimed for 99 % explained variance for variables with low variability (mslp, z500, t850) and 90 % for those with high variability (u and v) (Table 2). Subsequently, CA was employed for the PCA score matrix to identify the SWPs. We utilised the non-hierarchical k-means method (McQueen, 1967) and tested various combinations of atmospheric variables and different numbers of clusters (N) as outlined in Table 2. Classifications yielding clusters with fewer than five members (dates) were not considered robust.
3.4 Bayesian network
BNs are tools used to analyse complex systems by assessing the interdependence and conditional probability of variables (Pearl, 1988; Jensen, 1996). They have been effective when used in descriptive and predictive applications for natural hazards which differ in how the variables are connected and discretised (Beuzen et al., 2018). Descriptive BNs were used to see through the nonlinear relationships between variables in order to explore specific relationships between predictors and target variables (Fig. 4).
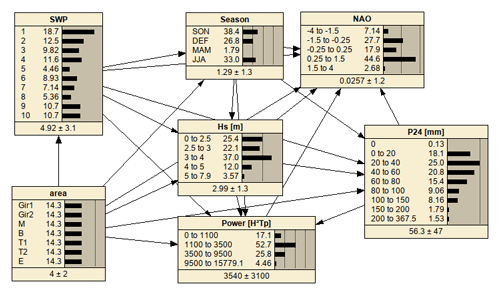
Figure 4Example of a Bayesian network (BN) used to evaluate classifications and analyse SWPs. This example corresponds to the BN for N=10 groups, which was trained with the k-means classification using z500 and u at D0.
The BN variables correspond to the essential elements required for evaluating the performance of classifications (SWPs) in estimating target variables (Hs and P24) and characterising coastal storm intensities (Power) on a basin scale (Area) as well as when they are combined with seasonality or the NAO. The discretisation of the variables was determined based on expert knowledge by identifying threshold combinations representative of the study site.
Regarding hazard intensity, P24=40 mm represents the local threshold for flash flood potential due to convective events (Barbería et al., 2014; Cortès et al., 2018), while P24=100 mm and P24=200 mm are the thresholds for potentially damaging and extreme rainstorms, respectively, according to the warning criteria of the Meteorological Service of Catalonia (as defined at https://www.meteo.cat/wpweb/divulgacio/la-prediccio-meteorologica/situacio-meteorologica-de-perill/, last access: April 2023). The variable P24 was additionally discretised using uniform steps between the thresholds. Wave storms were classified using the local classification system of Mendoza et al. (2011), which uses storm power content (Power). Class II (moderate) events are characterised by a mean Power=3500 m2 hs and peak Hs≥3 m, while class IV (severe) storms exhibit a mean Power=9100 m2 hs and mean peak Hs=5 m. Energy content class values from Mendoza et al. (2011) were translated into power content thresholds by applying a factor of 10, assuming a representative mean value of Tp of 10 s throughout the entire duration of wave events. We also considered the warning criteria established by the Meteorological Service of Catalonia for coastal storms, which relies solely on significant wave height (Hs). According to this criterion, hazardous conditions are categorised as “high level” when Hs reaches or exceeds a threshold of 4 m. In this study, we classified the results into three intensity levels: (i) extreme conditions that comprise individual or compound events with P24≥200 mm, storm power content ≥ IV, and Hs≥4 m; (ii) severe conditions that encompass events that do not meet the extreme criteria but have P24≥100 mm, a classification of ≥ II for storm power content, and Hs≥4 m; and moderate conditions that include events that exceed POT thresholds but fall short of meeting the severe criteria.
We generated five BNs to test different values of N (i.e. 6, 10, 14, 18, 26) and created a training dataset for each combination of event type, domain, and N, resulting in a total of 88 tests. Each training dataset included one vector of variables for each event and basin, totalling 2632 training vectors for rain events and 518 / 784 cases for wave/compound events, respectively. To assess the SWP system's predictive capability for estimating Hs and P24, known as its synoptic skill, we calculated the BN skill measure as , where mrs is the mean square of the residuals of the linear regression between the mean value of the BN-predicted probability distributions and the data, and msd is the mean square of the data. The standard deviation of the prediction can be used as a weight, with less weight given to uncertain predictions (high standard deviation) and more weight given to confident outputs (Plant et al., 2016). Consequently, an incorrect but uncertain prediction is then considered more skilful than a confidently incorrect prediction. Both weighted and unweighted skills were calculated for the BN tests of interest using SWP alone or in combination with seasonality or the NAO as predictors. In meteorological applications, skill values above 0.2 are generally considered “useful”, those surpassing 0.4 are categorised as “good”, and when they achieve or exceed 0.6, they are regarded as “excellent” (Sutherland et al., 2004).
The BN was also used to calculate conditioned probabilities, particularly the probability of SWP occurrence at predefined intensity levels. BN training incorporates the values of the variables for each basin and event, resulting in presentation probabilities that encapsulate both the temporal frequency of SWPs and the number of basins impacted at specified intensity thresholds. Furthermore, we derived probabilities for intensity levels at the basin scale, conditioned by SWP, and calculated the probability of the variable “Area” conditioned by multivariate occurrences of such intensity levels.
4.1 Compound event characterisation
From 1990 to 2020, the study area experienced 112 compound episodes, of which 61 were classified as severe in at least one component (i.e. with P24≥100 mm or Hs≥4 m with storm class ≥ II). Regarding the other two event types, 376 rain and 74 wave episodes were recorded during the same period, of which 37 rain events were severe and only three of the wave events reached a class II storm with Hs≥4 m. On average, the entire territory experienced 3.7 compound events per year, 16.3 rain events per year, and 6.2 wave events per year. The frequency of severe storms in these types averaged at 2, 1.23, and 0.1, respectively. It is worth noting that no statistically significant trend was detected in any event category during the analysed period (Fig. 5).
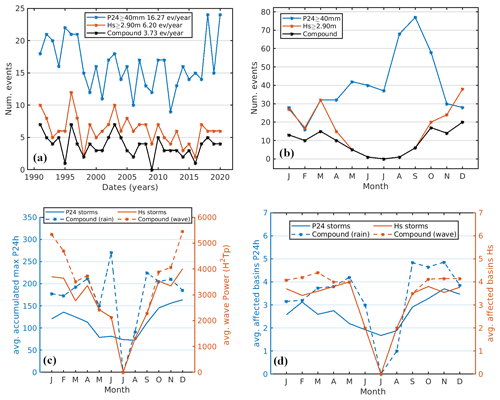
Figure 5(a) Annual number of occurrences of each event type. (b) Monthly number of occurrences of each event type during the period of study. (c) Monthly average magnitudes of cumulative rainfall and wave power during individual and compound events. (d) Monthly average number of affected basins during individual and compound events along the Catalonian coast.
The seasonal distribution of conditions contributing to compound events exhibit significant differences. Rainstorms occur year-round, with two peaks occurring in spring and late summer–autumn. By contrast, wave storms were notably absent during the summer months, with their main season spanning from September to April (Fig. 5). The combination of these distinct temporal patterns conditions the occurrence of compound events year-round, which reflects the temporal pattern of wave storms, which – although less frequent than rainstorms – are the controlling factor. One significant property of these compound events, in contrast to individual storms, is their potential for greater damage. This is evident in the fact that when heavy rain and wave storms coincide, the characteristics that determine their intensity, such as rainfall, wave height, and storm power, are magnified, and the spatial extent increases (as indicated by the number of affected basins) compared to when they occur individually (Fig. 5). During the wave storm season, the maximum accumulated precipitation values at a single station were, on average, 55 % higher during compound events than during standalone rainstorms, while the maximum Hs and wave power were on average 4 % and 20 % higher, respectively, than during individual wave storms.
Despite the relatively small study area, which comprises approximately 600 km of coastline, the occurrence and characteristics of these events display regional variations due to the influence of the orography and coastal configuration conditioning them. Notably, the annual frequency of compound events was highest in the north, averaging 2.6 events per year, compared to 1.2 events per year in the central and southern coastal sectors. It is important to mention that the occurrence of a compound multivariate event that simultaneously affects the entire area is “rare” (Sanuy et al., 2021) and has only happened during some extreme storms (e.g. Gloria). This extreme storm and other four episodes constitute just 0.9 % of the total number of events and 4.5 % of the compound events.
This spatial variability was also reflected in the magnitude of the events. The wave storm energy flux was fairly evenly distributed across the sectors, with the largest Hs observed in the north. Similarly, rainstorms presented higher average P24 values in the northern sector. These results align with findings from the BN analysis of SWP, as discussed in Sect. 4.3.
4.2 Selection of the domain, classification variables, and number of clusters
As previously mentioned, we employed the BN to guide the selection of the domain used for characterising synoptic weather types, the choice of variables, and the determination of the optimal number of clusters for classifying these weather types, all of which were based on the BN skill assessment (see Sect. 3.4). The results of the BN skill assessment, including the identification of clusters with fewer than five events (referred to as “bad clusters”), are presented in Figs. S1–S3 in the Supplement. A summary of the results is presented in Table 3, where the best-performing classifications in groups containing five or more dates are highlighted.
Table 3Summary of the top skills within acceptable classifications (i.e. excluding classifications yielding clusters with fewer than 5 d) for each event type. The optimal combination, based on BN skill, for each event type is highlighted in bold.
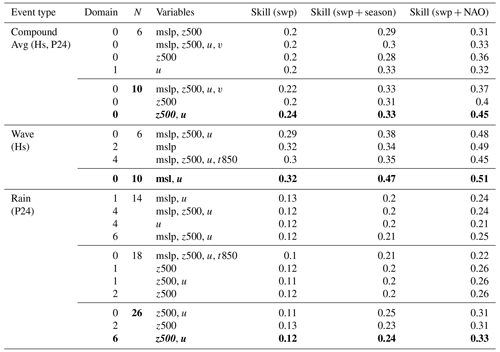
For compound events, skills were calculated as the average of the Hs and P24 skills, while for wave and rain events, individual target variables were used to determine their respective skills. Notably, the most promising classification was observed for N=10, where z500 and u were used as classification variables at domain D0. This configuration yielded skills ranging from 0.24 (SWP only) to 0.45 (SWP and NAO combined). Conversely, all classifications with N=14 resulted in groups containing a limited number of dates, as depicted in Fig. S1.
Regarding wave types, only one instance of N=10 clusters met the requirement of having at least five dates per cluster when utilising mslp and u at D0. This yielded skills ranging from 0.32 (SWP alone) to 0.51 (SWP + NAO). Interestingly, the skills of SWP alone or SWP + NAO were comparable for N=6 and N=10, while SWP + season skills saw the most benefit at N=10, reaching a skill value of 0.47, closely approaching the performance of SWP + NAO for compound events.
In the case of rain events, none of the combinations for N up to 26 achieved a skill level of 0.2 when relying solely on SWP. Acceptable skills were obtained when SWP was combined with season, starting at N=14. In particular, for N=18, the skills were nearly equivalent. The best results were observed within acceptable clusters for N=26 when utilising the combination of z500 and u at D6, which yielded a skill of 0.33 when incorporating SWP with NAO as predictors.
An overarching analysis of the entire test set (see Figs. S1–S3) revealed that, unlike rain and wave events, the highest skills for the compound type were achieved with the largest domain. Furthermore, combining two or more variables consistently led to enhanced skills compared to single-variable models, especially when applied at larger domains and for increasing values of N.
Notably, the weighted skills, which account for BN output uncertainty, spanned a range from 0.5 (using only SWP) to 0.9 (using SWP in conjunction with NAO). This underscores the BN's capacity to effectively capture uncertainty in its estimations. Specifically, when the BN output deviated significantly from the measurements, the BN output distribution exhibited a larger standard deviation. Conversely, instances when the BN output exhibited a low standard deviation closely aligned with the measurements. This analysis highlights the potential for improved prediction accuracy by introducing additional variables into the BN framework.
For illustrative purposes, we conducted an additional test using the best classification of compound events, namely z500 and u at D0 with 10 clusters (Fig. 6). In this test, we incorporated both the NAO and the season predictors together with the SWP. However, to prevent overfitting and manage the complexity of the model, we constrained the NAO variable to three bins: positive NAO(>0.25), negative , and approximately neutral =. The estimation of P24 was carried out in two scenarios: one with the node linked to Hs and Power (Fig. 6a) and another without this link (Fig. 6b). Notably, allowing the BN to account for relationships within the SWP between rain and wave intensity directly impacted the output uncertainty, as illustrated in Fig. 6. The P24 skill improved to 0.49 when the nodes were linked, compared to a skill of 0.43 without the link. Interestingly, the weighted skills reached 0.95 in both cases, indicating that the advantage of noise reduction was captured by the conventional measure of skill. Therefore, the test demonstrates that incorporating knowledge about Hs and storm energy content can enhance the prediction of P24, as these variables exhibit partial correlations.
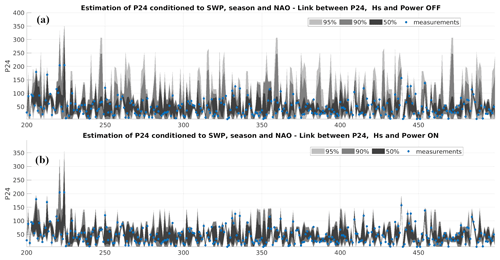
Figure 6Test results for z500 and u at D0 using SWP, NAO, and season as predictors. The BN has to estimate 784 cases, corresponding to 112 events at seven basins. For illustration purposes, cases 200 to 500 are shown (x axis). The blue dots represent the measurements, while the shaded areas depict the BN output distribution. (a) Test without a link between the target variables P24, Hs, and Power. (b) Test with the target variables linked.
4.3 Synoptic weather patterns conducive to extreme compound events
The compound SWPs derived from the combination of z500 and u with N=10 clusters can be classified based on their conditional presentation probabilities concerning the various intensity levels of the key variables P24 and Hs (see Sect. 3.4). The corresponding probabilities, obtained through the trained BN, are presented in Table 4.
Table 4Probability of occurrence of each SWP either unconditioned (in blue) or conditioned (in red) on different intensities of the variables of interest. The colour intensity varies from the maximum to the minimum values within each column.
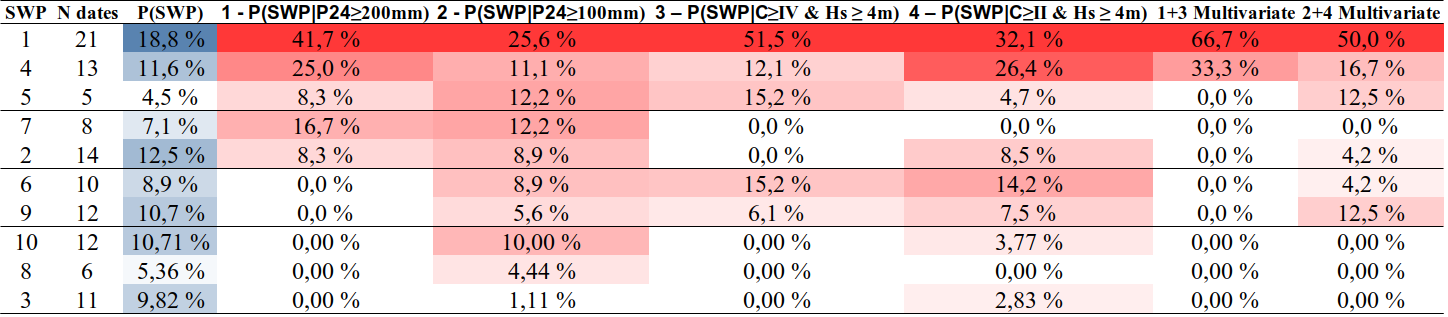
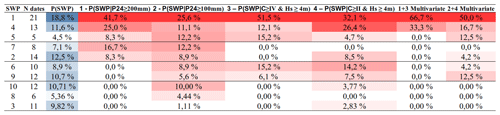
An initial set of patterns corresponds to extreme conditions in both rainfall and coastal storms; specifically, P(SWP mm) and P(SWP and Hs≥4 m). These patterns, denoted as SWP1, SPW4, and SPW5, collectively account for 75 % of the extreme daily rainfall and 78.8 % of the extreme coastal storms during compound events in the study area (Table 4).
SWP1 exhibits the highest probability of occurrence when conditioned on extreme rain and wave intensities, with probabilities reaching 42 % and 52 %, respectively (Table 4). This suggests that approximately half of the instances involving compound events featuring at least one extreme driver in any basin can be attributed to this specific pattern. It is worth noting that SWP1 exclusively corresponds to class IV coastal storms and multivariate severe events across all severe basins (see Fig. 7c and d). Furthermore, the prevalence of P24≥100 mm is most pronounced in the northernmost sectors, followed by Barcelona and the Ebro Delta. Notably, SWP1 accounts for 67 % of the multivariate extreme occurrences and 50 % of the multivariate severe events at the basin scale (as indicated in Table 4). This pattern is characterised by a cut-off structure at the z500 level, accompanied by a high-pressure system centred over the British Isles that extends eastward and southward. Additionally, a Mediterranean cyclone is situated south or southwest of the Iberian Peninsula (see Fig. 7a and b).
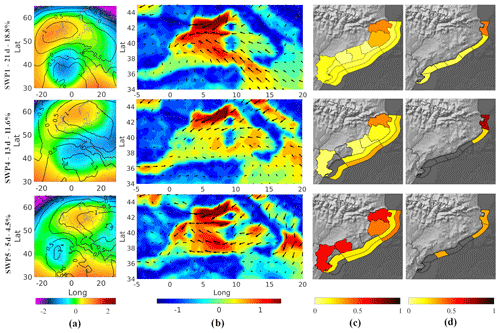
Figure 7Compound SWPs associated with P24>200 mm and coastal storms of C ≥ IV and Hs≥4 m. (a) z500 anomaly (colour map) overlaid with the mslp anomaly (contours). (b) Wind anomaly. (c) Probability of P24≥100 mm (land) at each basin; probability of a storm power content of class ≥ IV (internal coastal band) and probability of Hs≥4 m (external coastal band) at each coastal sector. (d) Spatial distribution of multivariate severe events of P24≥100 mm with a coastal storm of class ≥ II and Hs≥4 m.
This is followed by SWP4 and SWP5, in accordance with intensity (Table 4). SWP4 exhibits a stronger association with P24≥200 mm (25 %), whereas SWP5 is more closely linked with class IV storms featuring an Hs of over 4 m (15.2 %). SWP4 is characterised by an upper-level trough extending from eastern Europe to the Iberian Peninsula, coupled with a high-pressure system over the North Sea. At lower levels, high pressures extend toward eastern Europe, accompanied by a Mediterranean cyclone located near Sardinia (see Fig. 7a and b). SWP4 tends to generate class ≥4 coastal storms in the northern and central coastal sectors, with P24≥100 mm mainly concentrated in the north, where severe multivariate episodes occur. In contrast, central and southern basins tend to experience compounding waves or rainfall, with a higher likelihood of P24≥100 mm occurring in Maresme, Barcelona, and the Ebro Delta. On the other hand, SWP5 is characterised by an upper-level low-pressure system over Portugal, coupled with a Mediterranean low situated near Algiers (see Fig. 7a and b). The distribution of drivers across the territory differs from that of SWP4, with class 4 storms reaching the Ebro Delta and severe rainfalls being more probable in the southern third of the study area. SWP5 also tends to concentrate Hs≥4 m more to the north compared to SWP4.
Moving on, a second set of patterns, represented by SWP7 and SWP2, are linked to extreme levels of rainfall, accounting for 16.7 % and 8.3 % respectively (Table 4). However, these patterns involve moderate to severe coastal storms. Both SWP7 and SWP2 share common characteristics with Mediterranean low-pressure systems located either over the Balearic Islands or to the west. Additionally, they exhibit the presence of a trough originating from the British Isles.
In the case of SWP7, this trough is accompanied by high-pressure systems extending towards eastern Europe (see Fig. 8a and b). Conversely, SWP2 exhibits a similar structure, but it tends towards a cut-off pattern over the Iberian Peninsula and features a broader trough extending eastward. These structural differences influence the distribution of meteorological drivers across the territory. SWP7 leads to severe rainfall events across all basins, with higher probabilities in the southern third of the region. However, it does not result in class II storms with Hs≥4 m, thereby precluding the occurrence of multivariate episodes. On the other hand, SWP2 affects the northern and southern regions, albeit with lower probabilities. In this case, it triggers class II coastal storms with Hs≥4 m, leading to the observation of multivariate events in the northern areas (Fig. 8c and d).
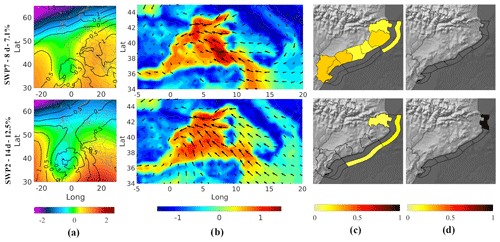
Figure 8Compound SWP associated with P24>200 mm and coastal storms of and Hs≥4 m. (a) z500 anomaly (colour map) overlaid with the mslp anomaly (contours). (b) Wind anomaly. (c) Probability of P24≥100 mm (land) at each basin; probability of a storm power content of class ≥ IV (internal coastal band) and the probability of Hs≥4 m (external coastal band) at each coastal sector. (d) Spatial distribution of multivariate severe events of P24≥100 mm with a coastal storm of class ≥ II and Hs≥4 m.
A third group of patterns exhibits distinctive characteristics involving moderate to severe rainfall coupled with extreme coastal storms. Notably, SWP6 is linked to 15.6 % of the extreme coastal storms across various sectors and contributes to 4.2 % of the severe multivariate events in different basins (Table 4). In the case of SWP6, both the cut-off pattern and the Mediterranean low are centred over the Balearic Islands and are accompanied by a high-pressure system located over northern Europe (see Fig. 9a and b). This atmospheric configuration creates conditions conducive to extreme coastal storms and severe rainfall events spanning from north to south, with Hs≥4 m primarily concentrated in the central coastal sectors. However, multivariate events are observed exclusively in the northernmost basin. Moving on to SWP9, although it has a slightly lower impact on extreme waves (6.1 %), it exhibits a higher association with multivariate severe events (12.5 %). SWP9 is characterised by an Atlantic low situated close to the northwest of the Iberian Peninsula alongside a high-pressure system over Scandinavia. Notably, there is no presence of a Mediterranean low (Fig. 9a and b). This atmospheric configuration results in coastal storms originating from the southern direction that affect the central and northern sectors with class ≥ IV storms, accompanied by Hs≥4 m, severe rainfall, and multivariate episodes in the northern regions (Fig. 9c and d).
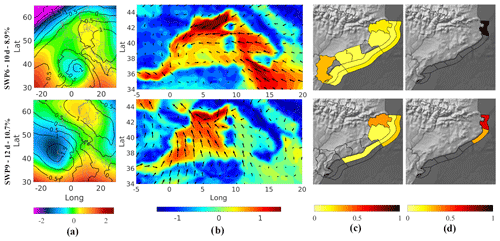
Figure 9Compound SWPs associated with mm and coastal storms of C≥IV and Hs≥4 m. (a) z500 anomaly (colour map) overlaid with the mslp anomaly (contours). (b) Wind anomaly. (c) Probability of P24≥100 mm (land) at each basin; probability of a storm power content of class ≥ IV (internal coastal band) and probability of Hs≥4 m (external coastal band) at each coastal sector. (d) Spatial distribution of multivariate severe events of P24≥100 mm with a coastal storm of class ≥ II and Hs≥4 m.
The remaining patterns, namely SWP10, SWP8, and SWP3 (Fig. 10), correspond to events characterised as moderate to severe in both rain and wave components. Notably, there are no instances of severe multivariate episodes across any of the basins (Table 4). In the case of SWP10, it is marked by a northwestern Atlantic trough with a Mediterranean low positioned over the Balearic Islands, albeit with lesser intensity compared to previous patterns (see Fig. 10a and b). This atmospheric configuration leads to P24≥100 mm across the entire territory, accompanied by waves reaching Hs≥4 m and class II coastal storms primarily in the northern sector (Fig. 10c). SWP8 is characterised by a cut-off pattern over the Iberian Peninsula, with a corresponding low-pressure system located over the Gulf of Cádiz (Fig. 10a and b). While winds in the Mediterranean do not generate severe coastal storms in the study area, severe rainfall events may occur in Barcelona and the northern basins (Fig. 10c). Finally, SWP3 is marked by a Mediterranean low situated between the Gulf of Lyon and the Ligurian Sea, accompanied by a configuration of low-pressure systems at both at the surface and upper levels and extending from the British Isles to the North Sea and Scandinavia (see Fig. 10a and b). This atmospheric setup results in winds blowing from the land toward the Mediterranean Spanish coast, with Hs reaching 4 m at specific locations in the central and northern sectors. Severe rainfall events were recorded exclusively in the northernmost basin Fig. 10c).
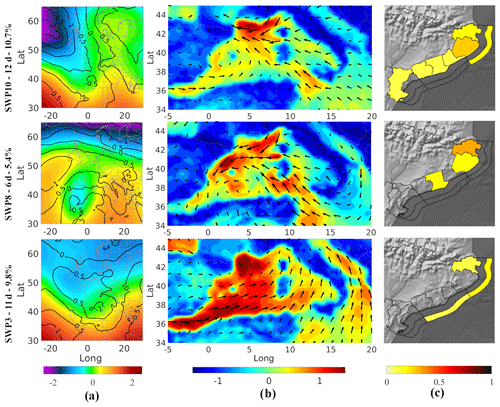
Figure 10Compound SWPs associated with mm coastal storms of with Hs≥4 m. (a) z500 anomaly (colour map) overlaid with the mslp anomaly (contours). (b) Wind anomaly. (c) Probability of P24≥100 mm (land) at each basin; probability of a storm power content of class ≥ IV (internal coastal band) and the probability of Hs≥4 m (external coastal band) at each coastal sector. Please note that none of the SWPs were linked to severe multivariate episodes; therefore, the panels for such cases are not displayed.
Our integrated framework, which combines an objective classification method with Bayesian networks, has proven effective at identifying and characterising extreme compound SWPs. Notably, the majority of SWPs associated with extreme conditions exhibited upper-level lows or trough formations, often in conjunction with Mediterranean lows or cyclones. These atmospheric patterns resulted in severe to extreme coastal storms combined with convective systems. These identified patterns align closely with impactful events previously documented in the literature (see Table 5). The descriptions of their impact closely correspond to the probabilistic characterisations derived from the BN framework. In contrast, none of the synoptic patterns associated with extreme individual rain or wave events featured this specific combination of atmospheric structures (Figs. S4 and S5 in the Supplement). For instance, the strong winds responsible for individual wave events did not originate from Mediterranean lows and were not associated with extreme coastal storms, typically reaching only the severe intensity level (Fig. S5). Similarly, extreme individual rain episodes were linked to cut-off and trough configurations without the presence of a Mediterranean cyclone (Fig. S4).
Table 5Compilation of significant historical events associated with the identified extreme compound SWP, with concise summaries of documented impacts along with corresponding references.
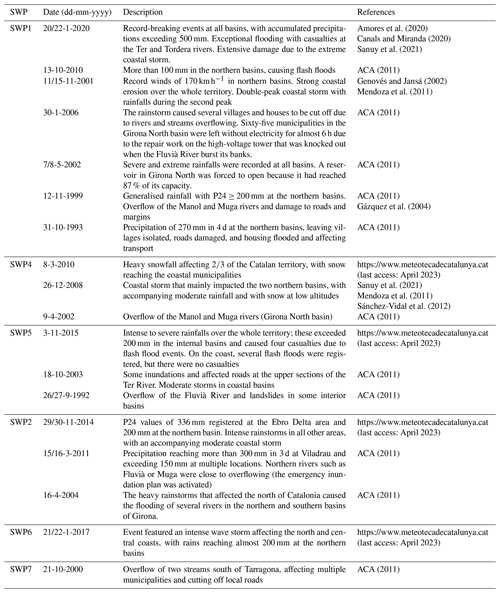
These results are consistent with previous individual SWP classifications of extreme rainfall (Romero et al., 1999; Gázquez et al., 2004), convective rainfall (Barbería et al., 2014; Cortès et al., 2018), floods (Gil-Guirado et al., 2021), coastal storms (Mendoza et al., 2011), and strong winds (Peña et al., 2011) in the study area. For instance, the extreme compound SWP1 (Fig. 7) was identified by Gil-Guirado et al. (2021) as the configuration most associated with terrestrial floods on the Spanish Mediterranean coast. This pattern was also recognised by Peña et al. (2011), who linked the cut-off configuration to the presence of strong winds, heavy showers, and sea-wave erosion in the region. Regarding marine hazards, Mendoza et al. (2011) conducted a preliminary analysis of synoptic situations associated with severe to extreme coastal storms (class III–V). They found that the most extreme storms (class V events) occurred in the presence of a Mediterranean cyclone. Configurations without Mediterranean lows, referred to as southern and eastern advections, were also associated with less severe coastal storms ranging from class II to IV.
The literature on compound coastal flooding has extensively explored the combined effects of surges with river discharges or surges with precipitation (Couasnon et al., 2018; Paprotny et al., 2018; Hendry et al., 2019; Camus et al., 2022; Wahl et al., 2015; Wu et al., 2018; Bevacqua et al., 2019). While precipitation can serve as a proxy for hydrometeorological flooding potential in small river catchments (Bevacqua et al., 2019), especially in the context of NW Mediterranean conditions, it is worth noting that surges play a relatively small role compared to wind waves in contributing to flooding in the area (Mendoza and Jiménez, 2009; Sanuy et al., 2020). Therefore, the analysis of significant Hs and wave power during coastal storms is essential to characterise the hazardous maritime component and “indicate” both coastal erosion and flooding potential.
Most of the research on compound coastal events has primarily focused on multivariate events at large scales due to the possibility of synergistic effects between hazards at sensitive locations (Wahl et al., 2015; Couasnon et al., 2018; Paprotny et al., 2018; Wu et al., 2018; Bevacqua et al., 2019; Hendry et al., 2019; Camus et al., 2022). However, the recent impact of Storm Gloria in January 2020 in the NW Mediterranean (e.g. Amores et al., 2020; Canals and Miranda, 2020) vividly demonstrated the accumulative impact of terrestrial and maritime hazards on various parts of the region. Under such conditions, civil protection services had to prioritise interventions based on urgency because of the simultaneous occurrence of a large number of emergency calls (approximately 15 000) and required interventions (approximately 2500) in all coastal sectors, as reported by the Ministry of Home Affairs of the Government of Catalonia. Understanding the characteristics of compound events, including their regional prevalence in terms of multivariate phenomena and the probabilities of synergistic versus cumulative potential impacts at the local scale, is critical for enhancing event preparedness within the constraint of limited resources. This consideration is particularly relevant for compound-type events where rainfall and coastal extremes occur in different basins. We successfully differentiated the pattern responsible for region-wide multivariate events (SWP1, Fig. 7) from those that exhibit localised multivariate impacts both with and without compounding waves or rain in other areas of the region. This underscores the effectiveness of the final classification in distinguishing impact distributions. Hence, the framework could be tested in the future across broader domains (e.g. the whole Spanish Mediterranean coast), provided that relevant data are accessible.
The prediction skill of the BN played a pivotal role in selecting the optimal combination of atmospheric variables, domain size, and number of clusters needed to effectively replicate rainfall and coastal storm intensity at the local scale. By incorporating seasonality and NAO to account for inter-annual and within-pattern variations, we achieved skill scores exceeding 0.4, signifying strong performance according to the criteria of Sutherland et al. (2004). The relative importance of season and NAO in influencing wave heights and rainfall variations aligns with existing literature (Casanueva et al., 2014; Krichak et al., 2014; Criado-Aldanueva and Soto-Navarro, 2020; Morales-Márquez et al., 2020). Notably, superior performance was consistently observed in smaller domains for rain events, as described by Beck et al. (2016), while this relationship was less clear for wave events. Remarkably, the highest skill levels were consistently attained in the largest domain for compound events.
Our weighted skills, which consider how the BN captures uncertainty, reached excellent values exceeding 0.9. This further validates the derived SWPs as a reliable reference baseline for classifying future dates and exploring shifts in the probabilities of compound severe conditions, along with their regional distribution, under climate change scenarios. Future enhancements may involve exploring additional atmospheric variables such as relative and specific humidity (see e.g. Teegavarapu et al., 2018), additional teleconnections (Casanueva et al., 2014; Taibi et al., 2019), or potentially influencing parameters such as NW Mediterranean Sea moisture and temperature before SWP occurrence (Rainaud et al., 2017). This represents a crucial step towards characterising the impact potential of the hazard drivers under study, making the methodology applicable as a rapid early warning system preceding the use of numerical models based on synoptic forecasting maps.
In the NW Mediterranean, where flash floods and coastal storms often coincide, we have successfully identified and characterised synoptic weather patterns conducive to compound extreme events. To do this, we have developed a methodological framework that combines an objective synoptic classification method with a Bayesian network. The BN played a pivotal role in selecting the optimal atmospheric variables, domain dimensions, and clusters, effectively capturing local-scale variations in key variables (daily rainfall and wave heights) and thereby attaining robust skill scores. Furthermore, the analysis confirmed seasonality and the NAO as influential factors shaping wave heights and rainfall variations. It is noteworthy that, in the case of rainstorms, smaller domains consistently outperformed larger ones, while the largest domain consistently achieved the highest skill levels for compound events.
Identified SWPs conducive to compound events differ from those associated with extreme individual rain or wave events and typically involve upper-level lows and trough structures in combination with Mediterranean cyclones. These atmospheric configurations lead to severe to extreme coastal storms combined with convective systems.
The outstanding weighted skills obtained by our framework indicate its potential as a rapid early warning system for evaluating the likelihood of severe and extreme rain–wave conditions. This has the potential to enhance risk mitigation and emergency preparedness efforts. Moreover, the derived SWPs serve as validated baselines in terms of synoptic skill, enabling the classification of future events and the evaluation of compound events in the context of climate change. Future research directions may encompass the exploration of additional atmospheric variables and parameters, facilitating a deeper understanding of the role of potential drivers for these hazards.
MATLAB scripts were used to automatize the different steps of the analysis and are not publicly available.
Wave data were obtained from SMC and rain data were obtained from AEMET. They are not publicly available (see references in the text). Atmospheric data were obtained from the ERA5 dataset (single levels, https://doi.org/10.24381/cds.adbb2d47, Hersbach et al., 2023b; and pressure levels, https://doi.org/10.24381/cds.bd0915c6, Hersbach et al., 2023a). NAO data was retrieved from NOAA (https://www.cpc.ncep.noaa.gov/products/precip/CWlink/pna/nao.shtml, NOAA Climate Prediction Center, 2024)
The supplement related to this article is available online at: https://doi.org/10.5194/hess-28-283-2024-supplement.
MS conceptualised the research, built the BN, performed the analysis, and co-wrote the manuscript. JCP conceptualised the research, guided the SWP classification, and characterised the obtained types. SA developed the reconstruction of wave observations. JAJ conceptualised the research, co-wrote the manuscript, managed the project, and secured funding. All authors contributed to the interpretation and discussion of the obtained results and to the writing of the final manuscript.
The contact author has declared that none of the authors has any competing interests.
Publisher's note: Copernicus Publications remains neutral with regard to jurisdictional claims made in the text, published maps, institutional affiliations, or any other geographical representation in this paper. While Copernicus Publications makes every effort to include appropriate place names, the final responsibility lies with the authors.
This study was conducted within the framework of the C3RiskMed (PID2020-113638RB-C21) research project funded by the Spanish Ministry of Science and Innovation. The authors express their gratitude to AEMET for supplying rain data and SMC for supplying rain data and wave data.
This research has been supported by the Ministerio de Ciencia e Innovación (PID2020-113638RB-C21) and by the Ministry of Universities (UP2021-034: Margarita Salas postdoc grant).
This paper was edited by Efrat Morin and reviewed by two anonymous referees.
ACA: Avaluació preliminar del risc d'inundació al districte de conca fluvial de Catalunya. Annex 4: Anàlisi de les inundacions històriques, Agència Catalana de l'Aigua, 18 pp., 2011.
Amores, A., Marcos, M., Carrió, D. S., and Gómez-Pujol, L.: Coastal impacts of Storm Gloria (January 2020) over the north-western Mediterranean, Nat. Hazards Earth Syst. Sci., 20, 1955–1968, https://doi.org/10.5194/nhess-20-1955-2020, 2020.
Aran, M., Peña, J. C., and Torà, M.: Atmospheric circulation patterns associated with hail events in Lleida (Catalonia), Atmos. Res., 100, 428–438, https://doi.org/10.1016/j.atmosres.2010.10.029, 2011.
Barbería, L., Amaro, J., Aran, M., and Llasat, M. C.: The role of different factors related to social impact of heavy rain events: considerations about the intensity thresholds in densely populated areas, Nat. Hazards Earth Syst. Sci., 14, 1843–1852, https://doi.org/10.5194/nhess-14-1843-2014, 2014.
Beck, C. and Philipp, A.: Evaluation and comparison of circulation type classifications for the European domain, Phys. Chem. Earth, 35, 374–387, 2010.
Beck, C., Philipp, A., and Streicher, F.: The effect of domain size on the relationship between circulation type classifications and surface climate, Int. J. Climatol., 36, 2692–2709, 2016.
Beuzen, T., Splinter, K. D., Marshall, L. A., Turner, I. L., Harley, M. D., and Palmsten, M. L.: Bayesian Networks in coastal engineering: Distinguishing descriptive and predictive applications, Coast. Eng., 135, 16–30, https://doi.org/10.1016/j.coastaleng.2018.01.005, 2018.
Bevacqua, E., Maraun, D., Vousdoukas, M. I., Voukouvalas, E., Vrac, M., Mentaschi, L., and Widmann, M.: Higher probability of compound flooding from precipitation and storm surge in Europe under anthropogenic climate change, Science Advances, 5, eaaw5531, https://doi.org/10.1126/sciadv.aaw5531, 2019.
Camus, P., Haigh, I. D., Wahl, T., Nasr, A. A., Méndez, F. J., Darby, S. E., and Nicholls, R. J.: Daily synoptic conditions associated with occurrences of compound events in estuaries along North Atlantic coastlines, Int. J. Climatol., 42, 5694–5713, 2022.
Canals, M. and Miranda, J. (Eds.): Sobre el temporal Gloria (19–23.01.20), els seus efectes sobre el país i el que se'n deriva: Report de Resposta Ràpida (R3), Institut d'Estudis Catalans, Secció de Ciències i Tecnologia, ISBN 978-84-9965-552-9, 2020.
Casanueva, A., Rodríguez-Puebla, C., Frías, M. D., and González-Reviriego, N.: Variability of extreme precipitation over Europe and its relationships with teleconnection patterns, Hydrol. Earth Syst. Sci., 18, 709–725, https://doi.org/10.5194/hess-18-709-2014, 2014.
Catto, J. L. and Dowdy, A.: Understanding compound hazards from a weather system perspective, Weather and Climate Extremes, 32, 100313, https://doi.org/10.1016/j.wace.2021.100313, 2021.
Cortès, M., Turco, M., Llasat-Botija, M., and Llasat, M. C.: The relationship between precipitation and insurance data for floods in a Mediterranean region (northeast Spain), Nat. Hazards Earth Syst. Sci., 18, 857–868, https://doi.org/10.5194/nhess-18-857-2018, 2018.
Couasnon, A., Sebastian, A., and Morales-Nápoles, O.: A copula-based Bayesian network for modeling compound flood hazard from riverine and coastal interactions at the catchment scale: An application to the Houston Ship Channel, Texas, Water, 10, 1190, https://doi.org/10.3390/w10091190, 2018.
Criado-Aldeanueva, F. and Soto-Navarro, J.: Climatic Indices over the Mediterranean Sea: A Review, Appl. Sci.-Basel, 10, 5790, https://doi.org/10.3390/app10175790, 2020.
Falkena, S. K., de Wiljes, J., Weisheimer, A., and Shepherd, T. G.: Revisiting the identification of wintertime atmospheric circulation regimes in the Euro-Atlantic sector, Q. J. Roy. Meteor. Soc., 146, 2801–2814, https://doi.org/10.1002/qj.3818, 2020.
Flaounas, E., Davolio, S., Raveh-Rubin, S., Pantillon, F., Miglietta, M. M., Gaertner, M. A., Hatzaki, M., Homar, V., Khodayar, S., Korres, G., Kotroni, V., Kushta, J., Reale, M., and Ricard, D.: Mediterranean cyclones: current knowledge and open questions on dynamics, prediction, climatology and impacts, Weather Clim. Dynam., 3, 173–208, https://doi.org/10.5194/wcd-3-173-2022, 2022.
Gaume, E., Borga, M., Llasat, M. C., Maouche, S., Lang, M., and Diakakis, M.: Mediterranean extreme floods and flash floods. Into Hydro-meteorological extremes, Chap. 3, The Mediterranean Region under Climate Change. A Scientific Update (coordinated byAllEnvi), IRD Éditions Institut de Recherche pour le Développement, Marseille, 133–144, ISBN 978-2-7099-2219-7, 2016.
Gázquez, A., Perpinyà i Romeu, M., Llasat, M. D. C., and Peña Rabadán, J. C.: Episodios pluviométricos superiores a los 200 mm en 24 horas en Catalunya. Catalogación y configuraciones meteorológicas asociadas, Treballs de la Societat Catalana de Geografia, 56, 139–156, 2004.
Genovés, A. and Jansà, A.: Diabatic processes contribution to the november 2001 storm, Mediterranean Storms, in: Proceedings of the 4th EGS Plinius Conference, 2–4 October 2002, Bay of Pollentia, Mallorca, Spain, 2002.
Gilabert, J. and Llasat, M. C.: Circulation weather types associated with extreme flood events in Northwestern Mediterranean, Int. J. Climatol, 38, 1864–1876, https://doi.org/10.1002/joc.5301, 2017.
Gil-Guirado, S., Pérez-Morales, A., Pino, D., Peña, J. C., and Martínez, F. L.: Flood impact on the Spanish Mediterranean coast since 1960 based on the prevailing synoptic patterns, Sci. Total Environ., 807, 150777, https://doi.org/10.1016/j.scitotenv.2021.150777, 2021.
Hendry, A., Haigh, I. D., Nicholls, R. J., Winter, H., Neal, R., Wahl, T., Joly-Laugel, A., and Darby, S. E.: Assessing the characteristics and drivers of compound flooding events around the UK coast, Hydrol. Earth Syst. Sci., 23, 3117–3139, https://doi.org/10.5194/hess-23-3117-2019, 2019.
Hénin, R., Ramos, A. M., Pinto, J. G., and Liberato, M. L.: A ranking of concurrent precipitation and wind events for the Iberian Peninsula, Int. J. Climatol., 41, 1421–1437, 2021.
Hersbach, H., Bell, B., Berrisford, P., Biavati, G., Horányi, A., Muñoz Sabater, J., Nicolas, J., Peubey, C., Radu, R., Rozum, I., Schepers, D., Simmons, A., Soci, C., Dee, D., and Thépaut, J.-N.: ERA5 hourly data on pressure levels from 1940 to present, Copernicus Climate Change Service (C3S) Climate Data Store (CDS), https://doi.org/10.24381/cds.bd0915c6, 2023a.
Hersbach, H., Bell, B., Berrisford, P., Biavati, G., Horányi, A., Muñoz Sabater, J., Nicolas, J., Peubey, C., Radu, R., Rozum, I., Schepers, D., Simmons, A., Soci, C., Dee, D., and Thépaut, J.-N.: ERA5 hourly data on single levels from 1940 to present, Copernicus Climate Change Service (C3S) Climate Data Store (CDS), https://doi.org/10.24381/cds.adbb2d47, 2023b.
Hess, P. and Brezowsky, H.: Katalog der Grosswetterlagen Europas (1881–1965), Gebrüder Borntraeger, Ber. Dt. Wetterd. 15, 1969.
Huth, R.: Synoptic-climatological applicability of circulation classifications from the COST733 collection: first results, Phys. Chem. Earth, 35, 388–394, 2010.
Huth, R., Beck, C., Philipp, A., Demuzere, M., Ustrnul, Z., Cahynová, M., Kyselý, J., and Tveito, O. E.: Classifications of atmospheric circulation patterns: recent advances and applications, Ann. NY Acad. Sci., 1146, 105–152, 2008.
Jansa, A., Alpert, P., Arbogast, P., Buzzi, A., Ivancan-Picek, B., Kotroni, V., Llasat, M. C., Ramis, C., Richard, E., Romero, R., and Speranza, A.: MEDEX: a general overview, Nat. Hazards Earth Syst. Sci., 14, 1965–1984, https://doi.org/10.5194/nhess-14-1965-2014, 2014.
Jensen, F. V.: An introduction to Bayesian networks, UCL Press, London, UK, ISBN 1857283225, 1996.
Jiménez, J. A. and Valdemoro, H. I.: Shoreline evolution and its management implications in the NW Mediterranean, J. Coastal Res., 86, 1–6, https://doi.org/10.1007/978-3-319-93169-2_32, 2019.
Jiménez, J. A., Sancho, A., Bosom, E., Valdemoro, H. I., and Guillén, J.: Storm-induced damages along the Catalan coast (NW Mediterranean) during the period 1958–2008, Geomorphology, 143–144, 24–33, https://doi.org/10.1016/j.geomorph.2011.07.034, 2012.
Krichak, S. O., Breitgand, J. S., Gualdi, S., and Feldstein, S. B.: Teleconnection–extreme precipitation relationships over the Mediterranean region, Theor. Appl. Climatol., 117, 679–692, 2014.
Kron, W.: Coasts: the high-risk areas of the world, Nat. Hazards, 66, 1363–1382, https://doi.org/10.1007/s11069-012-0215-4, 2013.
Lamb, H. H.: British Isles weather types and a register of the daily sequence of circulation patterns, 1861–1971, H. M. Stationery Off, 1972.
Llasat, M. C. and Puigcerver, M.: Pluies extremes en Catalogne: influence orographique et caractéristiques synoptiques. Hydrologie Continentale, Ed. Orstom, Paris, Francia, 2, 99–115, ISSN 0246-1528, 1992.
Llasat, M. C., Marcos, R., Llasat-Botija, M., Gilabert, J., Turco, M., Quintana-Seguí, P.: Flash flood evolution in North-Western Mediterranean, Atmos. Res., 149, 230–243, 2014.
Llasat, M. C., Marcos, R., Turco, M., Gilabert, J., and Llasat-Botija, M.: Trends in flash flood events versus convective precipitation in the mediterranean region: the case of catalonia, J. Hydrol., 541, 24–37, 2016.
Luján López, A.: Las siniestralidades del Gloria, Consorseguros digital, 16, 14 pp., https://www.consorsegurosdigital.com/es/numero-16/portada/las-siniestralidades-de-gloria (last access: 11 January 2024), 2022.
Martin-Vide, J., Sanchez-Lorenzo, A., Lopez-Bustins, J. A., Cordobilla, M. J., Garcia-Manuel, A., and Raso, J. M.: Torrential rainfall in northeast of the Iberian Peninsula: synoptic patterns and WeMO influence, Adv. Sci. Res., 2, 99–105, https://doi.org/10.5194/asr-2-99-2008, 2008.
McQueen, J.: Some methods for classification and analysis of multivariate observations, in: Proceedings of the Fifth Berkeley Symposium on Mathematical Statistics and Probability, Vol. 1 Statistics, University of California Press, 21 June–18 July 1965 and 27 December 1965–7 January 1966, Berkeley, California 281–297, 1967.
Mendoza, E. T. and Jiménez, J. A.: Regional vulnerability analysis of Catalan beaches to storms, P. I. Civil Eng.-Mar. En., 162, 127–135, https://doi.org/10.1680/maen.2009.162.3.127, 2009.
Mendoza, E. T., Jimenez, J. A., and Mateo, J.: A coastal storms intensity scale for the Catalan sea (NW Mediterranean), Nat. Hazards Earth Syst. Sci., 11, 2453–2462, https://doi.org/10.5194/nhess-11-2453-2011, 2011.
Morales-Márquez, V., Orfila, A., Simarro, G., and Marcos, M.: Extreme waves and climatic patterns of variability in the eastern North Atlantic and Mediterranean basins, Ocean Sci., 16, 1385–1398, https://doi.org/10.5194/os-16-1385-2020, 2020.
NOAA Climate Prediction Center (CPC): https://www.cpc.ncep.noaa.gov/products/precip/CWlink/pna/nao.shtml, last access: 17 January 2024.
Paprotny, D., Vousdoukas, M. I., Morales-Nápoles, O., Jonkman, S. N., and Feyen, L.: Compound flood potential in Europe, Hydrol. Earth Syst. Sci. Discuss. [preprint], https://doi.org/10.5194/hess-2018-132, 2018.
Pearl, J.: Probabilistic reasoning in intelligent systems: networks of plausible inference, Morgan Kaufmann, San Francisco, California, USA, ISBN 9781558604797, 1988.
Peña, J. C., Aran, M., Cunillera, J., and Amaro, J.: Atmospheric circulation patterns associated with strong wind events in Catalonia, Nat. Hazards Earth Syst. Sci., 11, 145–155, https://doi.org/10.5194/nhess-11-145-2011, 2011.
Philipp, A., Bartholy, J., Beck, C., Erpicum, M., Esteban, P., Fettweis, X., Huth, R., James, P., Jourdain, S., Kreienkamp, F., Krennert, T., Lykoudis, S., Michalides, S., Pianko-Kluczynska, K., Post, P., Rassilla Alvarez, D., Schiemann, R., Spekat, A., and Tymvios, F. S.: COST733CAT – a2709 database of weather and circulation type classifications, Phys. Chem. Earth, 35, 360–373, 2010.
Philipp, A., Beck, C., Huth, R., and Jacobeit, J.: Development and comparison of circulation type classifications using the COST 733 dataset and software, Int. J. Climatol., 36, 2673–2691, 2016.
Plant, N. G., Robert Thieler, E., and Passeri, D. L.: Coupling centennial-scale shoreline change to sea-level rise and coastal morphology in the Gulf of Mexico using a Bayesian network, Earths Future, 4, 143–158, 2016.
Rainaud, R., Brossier, C. L., Ducrocq, V., and Giordani, H.: High-resolution air–sea coupling impact on two heavy precipitation events in the Western Mediterranean, Q. J. Roy. Meteor. Soc., 143, 2448–2462, 2017.
Romero, R., Sumner, G., Ramis, C., and Genovés, A.: A classification of the atmospheric circulation patterns producing significant daily rainfall in the Spanish Mediterranean area, Int. J. Climatol., 19, 765–785, 1999.
Sanchez-Vidal, A., Canals, M., Calafat, A. M., Lastras, G., Pedrosa-Pàmies, R., Menéndez, M., Medina, R., Company, J. B., Hereu, B., Romero, J., and Acoverro, T.: Impacts on the deep-sea ecosystem by a severe coastal storm, PloS one, 7, e30395, https://doi.org/10.1371/journal.pone.0030395, 2012.
Sanuy, M., Jiménez, J. A., Ortego, M. I., and Toimil, A.: Differences in assigning probabilities to coastal inundation hazard estimators: Event versus response approaches, J. Flood Risk Manag., 13, e12557, https://doi.org/10.1111/jfr3.12557, 2020.
Sanuy, M., Rigo, T., Jiménez, J. A., and Llasat, M. C.: Classifying compound coastal storm and heavy rainfall events in the north-western Spanish Mediterranean, Hydrol. Earth Syst. Sci., 25, 3759–3781, https://doi.org/10.5194/hess-25-3759-2021, 2021.
SMC: Estudio de la evolución de temporales de mar históricos en la costa catalana a partir de las observaciones y las simulaciones marítimas, Report from Meteorological Service of Catalonia (SMC), PIMA project, https://canviclimatic.gencat.cat/web/.content/02_OFICINA/publicacions/publicacions_de_canvi_climatic/Estudis_i_docs_adaptacio/PIMA_Adapta_Costas/PIMA-Informe-temporals-martims-jul2021_v2_correccions_OCCC.pdf (last access: April 2022), 2021.
Sutherland, J., Peet, A. H., and Soulsby, R. L.: Evaluating the performance of morphological models, Coast. Eng., 51, 917–939, 2004.
Taibi, S., Messelmi, I., Meddi, M., and Feddal, M. A.: Recent Rainfall Variability in the South-West Mediterranean Region and Links with Teleconnection Patterns, in: Patterns and Mechanisms of Climate, Paleoclimate and Paleoenvironmental Changes from Low-Latitude Regions: Proceedings of the 1st Springer Conference of the Arabian Journal of Geosciences (CAJG-1), 12–15 November 2018, Yasmine Hammamet, Tunisia, Springer International Publishing, 111–114, https://doi.org/10.1007/978-3-030-01599-2_26, 2019.
Teegavarapu, R. S. and Goly, A.: Optimal selection of predictor variables in statistical downscaling models of precipitation, Water Resour. Manag., 32, 1969–1992, 2018.
Wahl, T., Jain, S., Bender, J., Meyers, S. D., and Luther, M. E.: Increasing risk of compound flooding from storm surge and rainfall for major US cities, Nat. Clim. Change, 5, 1093–1097, https://doi.org/10.1038/nclimate2736, 2015.
Wu, W., McInnes, K., O'grady, J., Hoeke, R., Leonard, M., and Westra, S.: Mapping dependence between extreme rainfall and storm surge, J. Geophys. Res.-Oceans, 123, 2461–2474, 2018.
Yarnal, B.: Synoptic climatology in environmental analysis, Belhaven Press, London, UK, 1993.
Zscheischler, J., Westra, S., van den Hurk, B. J. J. M., Seneviratne, S.I., Ward, P. J., Pitman, A., AghaKouchak A., Bresch D. N., Leonard M., Wahl T., and Zhang, X.: Future climate risk from compound events, Nat. Clim. Change, 8, 469–477, https://doi.org/10.1038/s41558-018-0156-3, 2018.
Zscheischler, J., Martius, O., Westra, S., Bevacqua, E., Raymond, C., Horton, R. M., van den Hurk, B., Aghakouchak, A., Jézéquel, A., Mahecha, M. D., Maraun, D., Ramos, A. M., Ridder, N. N., Thiery, W., and Vignotto, E.: A typology of compound weather and climate events, Nat. Rev. Earth Environ., 1, 333–347, https://doi.org/10.1038/s43017-020-0060-z, 2020.