the Creative Commons Attribution 4.0 License.
the Creative Commons Attribution 4.0 License.
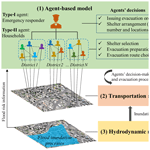
Development of an integrated socio-hydrological modeling framework for assessing the impacts of shelter location arrangement and human behaviors on flood evacuation processes
Feng Wu
Hao Jiang
Naliang Guo
Yong Tian
Chunmiao Zheng
In many flood-prone areas, it is essential for emergency responders to use advanced computer models to assess flood risk and develop informed flood evacuation plans. However, previous studies have had a limited understanding of how evacuation performance is affected by the arrangement of evacuation shelters (with respect to their number and geographical distribution) and human behaviors (with respect to the heterogeneity of household evacuation preparation times and route-searching strategies). In this study, we develop an integrated socio-hydrological modeling framework that couples (1) a hydrodynamic model for flood simulation, (2) an agent-based model for evacuation management policies and human behaviors, and (3) a transportation model for simulating household evacuation processes in a road network. We apply the model to the Xiong'an New Area and examine household evacuation outcomes for various shelter location plans and human behavior scenarios. The results show that household evacuation processes are significantly affected by the number and geographical distribution of evacuation shelters. Surprisingly, we find that establishing more shelters may not improve evacuation results if the shelters are not strategically located. We also find that low heterogeneity in evacuation preparation times can result in heavy traffic congestion and long evacuation clearance times. If each household selects their own shortest route without considering the effects of other evacuees' route choices, traffic congestion will likely occur, thereby reducing system-level evacuation performance. These results demonstrate the unique functionality of our model with respect to supporting flood risk assessment and advancing our understanding of how multiple management and behavioral factors jointly affect evacuation performance.
- Article
(12300 KB) - Full-text XML
- BibTeX
- EndNote
Flooding is one of the most devastating natural disasters and can lead to a significant number of fatalities, social and economic disruptions, property and infrastructure damage, and environmental degradation around the world (Smith and Matthews, 2015; McClymont et al., 2020; Brunner et al., 2020; Tanoue et al., 2016; Kreibich et al., 2014; Wang et al., 2019). The global flood database shows that the global flood inundation land area is approximately 2.23 ×106 km2, with 255–290 million people being directly affected by floods (Tellman et al., 2021). Flood-related economic damage increased globally from USD 94 billion in the 1980s to more than USD 1 trillion in the 2010s (Hino and Nance, 2021). Furthermore, the severity, duration, and frequency of damaging floods are expected to continue to increase in the future due to changes in climate, land use, and infrastructure (Jongman et al., 2012; Moulds et al., 2021; Wedawatta and Ingirige, 2012; Tellman et al., 2021). In many areas facing flood threats, it is essential for emergency responders and decision-makers to use advanced computer models to assess the flood risk and to establish effective disaster-mitigation plans (Simonovic and Ahmad, 2005).
Before an extreme flood occurs, evacuation is a critical emergency preparedness measure and a common practice because it is impractical and/or economically costly to construct the necessary infrastructure to resist floods (Wang et al., 2016; Liu and Lim, 2016; Islam et al., 2020; Kreibich et al., 2015). However, studies have shown that emergency evacuation is a complex and dynamic process that can be affected by factors from a wide range of interdisciplinary domains (Zhuo and Han, 2020; Hasan et al., 2011; Huang et al., 2012; Chen et al., 2021; Sung et al., 2018). These factors include but are not limited to (1) the accuracy, lead time, and sources of flood early warnings as well as the broadcasting channels through which flood information is disseminated to the affected population (Shi et al., 2020; Verkade and Werner, 2011; Alonso Vicario et al., 2020; Palen et al., 2010; Nester et al., 2012; Goodarzi et al., 2019); (2) the infrastructure and engineering facilities needed for emergency evacuation, which are influenced by the accessibility of transportation networks, road capacity, and locations of evacuation zones (Mostafizi et al., 2017; Chen and Zhan, 2008; Saadi et al., 2018; Mostafizi et al., 2019; Koch et al., 2020; Oh et al., 2021; Liu and Lim, 2016); and (3) demographic attributes and household behavioral characteristics, such as residents' beliefs and risk perception, previous knowledge, social networks, and past experience with flood events (Hofflinger et al., 2019; Huang et al., 2017; Lindell et al., 2020; Wang and Jia, 2021; Shahabi and Wilson, 2014; Du et al., 2017). These studies highlight the need to develop comprehensive socio-hydrological modeling tools that can adequately incorporate various factors and processes to support flood management plans in the context of coupled flood–human systems.
Among the many emergency management policies and plans that can be implemented, appropriate shelter location arrangement is essential for massive evacuation operations. City planners and policy-makers need to identify safe areas outside of flood inundation regions as feasible shelter locations for households who live in at-risk areas. Some studies have explored the criteria for shelter location arrangement and evacuation planning (Alçada-Almeida et al., 2009; Nappi and Souza, 2015; Bayram et al., 2015; Li et al., 2012; Alam et al., 2021). For instance, Bayram et al. (2015) developed an optimization model to allocate evacuation sites and assign each evacuee to the nearest shelter, with the objective of minimizing the total evacuation time. However, in their study, each evacuee's travel time was estimated based on a simple traffic volume–travel time function, which was not able to fully represent evacuees' complex interactions in a road network. Liu and Lim (2016) applied spatial analysis methods to assign shelters to households, considering the spatial relationships between households and shelter sites. A limitation of their study was that evacuee's travel time was obtained from a simplified traffic model, and the road network was not well represented in the network analysis. In a recent study, Alam et al. (2021) used a massive traffic simulation model and a multiple-criteria evaluation method to identify candidate evacuation shelters; they considered environmental conditions, structural attributes, emergency services, and transportation factors. However, the aforementioned study focused on obtaining a suitability score for each candidate shelter site with various weighting factors, yet failed to examine the extent to which evacuation performance could be affected by the number of shelters and their geographical distribution in the community. Thus, current studies have left a research gap that warrants efforts to use physically based flood simulation models to identify safe areas as feasible shelter locations and, more importantly, to use transportation models to address the following question: how is evacuation performance affected by the number and geographical distribution of evacuation shelter locations? This is the major research question that we seek to explore in this study.
The second research question to be explored in this study is associated with the role played by human behaviors in evacuation processes, which is an important research direction in disaster management (Aerts et al., 2018; Simonovic and Ahmad, 2005; Urata and Pel, 2018). After receiving flood evacuation warnings, households will make decisions based on flood risk information, spend some time completing a set of preparation tasks, and then evacuate to safe areas. Among these decisions and behaviors, households' evacuation preparation times (i.e., from the time that they receive flood evacuation orders to the time they start to evacuate via a road network) play an important role in evacuation performance. Many empirical studies have examined the geographic, demographic, and behavioral factors that affect households' preparation times (Lindell et al., 2005, 2020; Huang et al., 2012, 2017; Chen et al., 2021). They found that household evacuation preparation times can vary significantly from one household to another, exhibiting a certain degree of behavioral heterogeneity in a community (Lindell et al., 2005, 2020; Rahman et al., 2021). As a result, we hypothesize that the heterogeneity in households' evacuation preparation times affects traffic flow in the corresponding road network and, consequently, influences the final evacuation outcomes. However, few studies have explicitly examined how traffic conditions and evacuation performance are affected by different degrees of heterogeneity in evacuation preparation times (Wang et al., 2016). Hence, this is the second question that we aim to explore in this study.
Furthermore, in this work, we also seek to assess how evacuation processes are affected by households' evacuation-route-searching strategies, a process that involves emergency responders and policy-makers. Previous studies have typically applied the shortest-distance route-searching method to simulate how evacuees find evacuation routes from their original locations to evacuation destinations (He et al., 2021; Bernardini et al., 2017; Du et al., 2016; Li et al., 2022). However, each evacuee's search for the shortest evacuation path may not ensure system-level evacuation outcomes. In this study, we focus on comparing the evacuation scenario in which each household chooses the shortest path for evacuation with the scenario in which system-level global optimal routes are assigned to the evacuees. Such comparative analyses are expected to provide policy implications in terms of evacuees' route selections to improve evacuation performance during natural disasters.
Motivated by the above research questions and knowledge gaps, we develop an integrated socio-hydrological modeling framework in this study that couples (1) a physically based hydrodynamic model (MIKE 21) for flood inundation simulation, (2) an agent-based model (ABM) for simulating flood management plans and human behaviors, and (3) a large-scale traffic simulation model (MATSim) for simulating households' evacuation processes in a road network. Specifically, the hydrological component of the socio-hydrological modeling framework is represented by the MIKE 21 model, which simulates flood inundation processes across space and over time in a flood-prone area for a given storm event. The simulation results of the MIKE 21 model can provide flood risk information and will be used by policy-makers to make flood management plans. The social component of the modeling framework is represented by an ABM and MATSim, which simulate policy-makers' flood management plans, households' responses to flood management plans, and households' collective evacuation activities in the road network. By coupling the three models, our modeling framework is capable of simulating a wide range of components and processes in a coherent manner to support flood evacuation management.
We apply the modeling framework to the Xiong'an New Area, a large residential area with a high risk of flooding in northern China. Using a 100-year flood hazard as an example, a set of scenario analyses are conducted to explore how residents' evacuation processes are jointly affected by management policies (i.e., the number and geographical distribution of evacuation shelter locations) and human behaviors (i.e., the heterogeneity in households' evacuation preparation times and route-searching strategies). This study aims to provide both modeling and policy implications for researchers and emergency responders to develop advanced socio-hydrological modeling tools for flood risk assessment and to improve the overall understanding of how flood evacuation performance is jointly affected by various management and behavioral factors.
The remainder of this paper is organized as follows: Sect. 2 presents the modeling framework; Sect. 3 introduces the case study site, model construction, and scenario design; Sect. 4 presents the modeling results; Sect. 5 discusses the insights, limitations, and future research directions of this study; and conclusions are given in Sect. 6.
This section introduces the integrated modeling framework of this study. As illustrated in Fig. 1, the modeling framework consists of three models: (1) an ABM for simulating household decision-making and emergency responders' flood management policies, (2) a transportation model for simulating residents' evacuation activities in a road network, and (3) a hydrodynamic model for simulating flood inundation processes. A detailed introduction to the three models and their coupling methods are described in turn in the following.
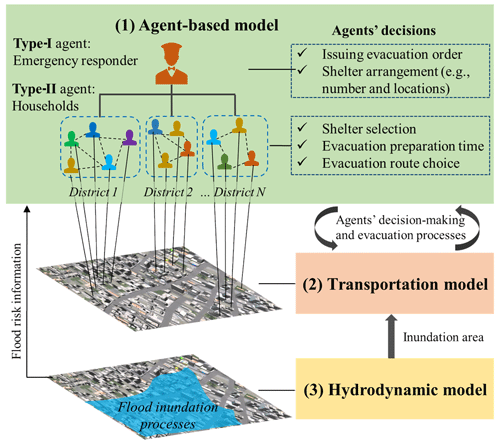
Figure 1Illustration of the integrated modeling framework that couples an ABM for simulating household decision-making and emergency responders' flood management policies, a transportation model for simulating residents' evacuation processes in a road network, and a hydrodynamic model for simulating flood inundation processes.
2.1 The ABM for human decision-making during flood events
In this study, an ABM is developed to simulate the government's disaster management plans and residents' flood evacuation behaviors. Therefore, two types of agents are considered in the ABM: (1) an emergency responder (Type-I agent) and (2) the set of households (Type-II agents). These agent types are described in turn below.
An emergency responder agent is a government institution that makes flood management plans. As shown in Fig. 1, we specifically consider two flood management decisions: (1) issuing a flood evacuation order to the residents who live in flood-prone areas and (2) shelter arrangement (i.e., deciding upon the number and location of evacuation zones that should be used to protect evacuees from flood hazards). Note that other management practices (e.g., sandbagging and levee construction) are also important flood management measures that are not explicitly discussed in this work.
In this study, each household is represented by an autonomous decision unit (i.e., an agent), under the assumption that all family members in a household typically evacuate using a shared mode of transportation after communicating with each other and arriving at a final evacuation decision (Du et al., 2016). After receiving evacuation orders, an agent will spend some time completing a set of evacuation preparation tasks and then evacuate from the household location to a chosen evacuation destination. The following three decisions and/or behaviors are explicitly considered during this process.
The first decision is the selection of an evacuation shelter if multiple shelter options are available. During evacuation processes, the agents seek to evacuate to safe areas as soon as possible, aiming to minimize their travel time. However, during an emergency situation, it is unclear and/or quite challenging for the agents to assess which shelter can ensure the shortest traveling time due to, for example, uncertainties in the real-time traffic conditions and traffic load (e.g., the number of evacuating agents on the road). Here, we follow the classic approach to evacuation simulation and assume that an agent focuses on choosing the shortest route from their original location to a safe area, thereby choosing the geographically nearest shelter in the system as their evacuation destination. Based on the above reasons, we assume that an agent will choose the evacuation shelter that is located the shortest geographical distance from their residential location.
The second decision is associated with evacuation preparation activities (e.g., gather family members, pack bags, board up windows, and shut off utilities). These activities are aggregated and represented by a behavioral parameter called “evacuation preparation time”. This parameter measures how long it takes an agent to prepare for evacuation and is indicated by the interval between the time that an agent receives an evacuation order and the time that they start to evacuate via a road network. Previous studies have shown that households' evacuation preparation times are influenced by both psychological and logistical preparation tasks, which may vary among agents, with noticeable behavioral heterogeneity, even at the community scale (Lindell et al., 2020, 2005; Wang et al., 2016). In this study, the heterogeneity in agents' evacuation preparation times is represented by the variation (i.e., standard deviation) in the evacuation preparation times of all households, and we explicitly examine the role of human behavioral heterogeneity in community evacuation outcomes.
The third decision is related to agents' route selection strategies during evacuation processes. In a complex road network, an agent may have multiple route choices from their original location to a destination. We assume that all of the agents have good knowledge of the road network in their community. Thus, two route-searching methods are incorporated into the model: (1) the shortest-distance route-searching method (Mode 1) and (2) the system-level optimization-based route-searching method (Mode 2). In the shortest-distance route-searching method, each agent seeks to reduce their evacuation time without considering the effects of other agents' evacuation route selections. The agents focus on finding the shortest route from their current location to the selected evacuation destination in the road network (Gallo and Pallottino, 1988; Fu et al., 2006; Li et al., 2022). Therefore, an agent's choice of evacuation route in Mode 1 will not be affected by their departure time, as they will always choose the shortest route regardless of the time at which they started to evacuate. The optimization-based route-searching method (Mode 2) adopts a heuristic iterative method to optimize all of the agents' collective evacuation routes so that system-level evacuation efficiency is achieved (Zhu et al., 2018; He et al., 2021). In contrast with Mode 1, an agent's evacuation route in Mode 2 is affected by real-time traffic condition and the evacuation status of other agents. Therefore, an agent's evacuation route in Mode 2 might be different if they begin evacuation at different times.
It is worth noting that agents will typically focus on reducing their own travel time and, therefore, do not necessarily consider system-level evacuation efficiency. With respect to the above two route-searching modes, Mode 1 represents the case in which every agent in the system focuses on achieving individual-level evacuation efficiency (i.e., chooses the shortest route for evacuation), whereas Mode 2 represents the case in which system-level evacuation efficiency is important (i.e., all of the agents' route choices are optimized at the system level). In this regard, Mode 1 is the baseline evacuation scenario and Mode 2 is the benchmark scenario. The results of Mode 2 can be used to assess the extent to which the evacuation outcome of Model 1 can be improved by changing agents' route choices. Policy-makers can compare the results of the two modes to improve evacuation performance by, for example, providing recommended evacuation routes for agents who may encounter and/or cause severe traffic congestion during their evacuation processes. Based on the above three decisions and behaviors, all of the agents' movements and interactions in the road network are incorporated into a transportation model, which is described in the following section.
2.2 Transportation model for large-scale evacuation simulation
As mentioned in Sect. 2.1, after an agent decides to evacuate, they will move from their (household) location to a chosen evacuation destination via the traffic network. During evacuation processes, an agent interacts with other agents and with the environment to adjust their movement in the road network over time. There are a number of modeling platforms and software packages used to model agents' evacuation processes. These include the Network Explorer for Traffic Analysis (NEXTA), the Transportation Analysis and Simulation System (TRANSIMS), the Planung Transport Verkehr (PTV) VISSIM, the City Traffic Simulator (CTS), and the Multi-Agent Transport Simulation (MATSim) model (Mahmud and Town, 2016; Lee et al., 2014; Murray-Tuite and Wolshon, 2013).
This study applies MATSim to simulate agents' evacuation processes. MATSim is a widely used open-source software for large-scale transportation simulation. The model can provide detailed information about each agent's movements in a road network (Horni, 2016; Lämmel et al., 2010; Zhuge et al., 2021). As shown in Fig. 2, MATSim requires a variety of data as model inputs. The plan data include the initial locations, evacuation destinations, and departure times of all agents, and these data can be retrieved from agents' attributes and evacuation decisions in the ABM. The network data describe the attributes of the road network, such as the geographical structure of the road network, the number of lanes of each road, and road segment lengths, and speed limits. These data are available from local or regional government institutions (e.g., the Department of Transportation) or from online data retrieval platforms such as OpenStreetMap or Google Maps (Farkas et al., 2014). Finally, the config input includes a model execution engine that defines a set of global model environments. Three modules, namely, an execution module, a scoring module, and a replanning module, are incorporated into MATSim for transportation simulation. This model has been widely used by researchers and practitioners to support evacuation planning and simulation for various types of natural disasters, such as earthquakes (Koch et al., 2020), hurricanes (Zhu et al., 2018), tsunamis (Muhammad et al., 2021), and floods (Saadi et al., 2018). For more details about MATSim and its applications in transportation simulation, the reader is referred to the studies of Lämmel et al. (2009) and Horni (2016).
2.3 The hydrodynamic model for flood inundation simulation
Information on flood inundation processes (e.g., flood extent and water level) is essential for governments and the public to make flood management and evacuation decisions. Hydrodynamic models are important tools for simulating the timing and duration of flood dynamics by solving a set of mathematical equations that describe fluid motion (Guo et al., 2021). There are many hydrodynamic models available for flood dynamics simulation. These include but are not limited to HEC-RAS, MIKE 11, MIKE 21, JFlow, TRENT, TUFLOW, and Delft3D (Teng et al., 2017).
Following our prior work (Wu et al., 2021), we use the classic MIKE 21 hydrodynamic model to simulate flood inundation processes in a floodplain. MIKE 21 numerically solves the two-dimensional shallow water equations to obtain water levels and flows across space and over time in various watershed environments, such as rivers, lakes, estuaries, bays, and coastal areas. MIKE 21 has been widely used to simulate flood inundation processes on many floodplains across the world and is considered one of the most effective modeling tools for flood risk mapping, flood forecasting, and scenario analysis (Nigussie and Altunkaynak, 2019; Papaioannou et al., 2016). Interested readers may refer to our prior work (Wu et al., 2021) for detailed introductions to the construction, calibration, and validation of the MIKE 21 model in the specific study area.
2.4 Model integration and flowchart of the modeling framework
In the prior sections (Sect. 2.1–2.3), the structures and functionalities of the three models were introduced; this section introduces how they are coupled in an integrated modeling framework. Previous studies have shown that computer models can be coupled in either a loose or a tight manner (Harvey et al., 2019; Bhatt et al., 2014; Murray-Rust et al., 2014; Du et al., 2020; Li et al., 2021). The former refers to models that are linked together by input–output data interfaces – that is, the output of one model is used as the input of another model. In contrast, for the latter, a model uses a common data pool and workload to exchange data among multiple model components, and, as a result, components affect each other during modeling processes.
In this study, both the loose and tight coupling methods are employed to combine the three models. Specifically, MIKE 21 is coupled with the ABM and MATSim in a loose manner, whereas the ABM and MATSim are coupled in a tight manner. The model-coupling process and flowchart of the integrated model are illustrated in Fig. 3. First, MIKE 21 simulates flood inundation processes for a specific flood event (e.g., a 100-year flood). The modeling results of MIKE 21 are then used to assess the inundated area and affected households in the flood zone, which are used as input data for the ABM and MATSim. Next, based on the modeling results of MIKE 21, two types of agents in the ABM are generated. The household agents who are located in the flood zone will receive flood warnings from an emergency responder agent and make evacuation decisions. Finally, all of the agents' movements and evacuation activities are simulated by MATSim. By integrating the three models, the proposed modeling framework is capable of simulating flood inundation processes, flood management practices, and household decision-making and evacuation processes in a coherent manner. In the next sections, we will use a real-world case study to demonstrate how the modeling framework can be used by researchers and practitioners for flood risk assessment and evacuation management.
2.5 Measurement of flood evacuation performance
Agents' evacuation processes reflect their evacuation status and movements across space and over time in a road network. In this study, we use multiple parameters and indicators to represent agents' evacuation processes and evaluate their evacuation performance. For a residential area with n household agents, we first use a categorical variable, , to describe agent j's evacuation status at time step t. denotes that agent j has not started their evacuation process at time t. denotes that agent j has already started evacuation but has not arrived at their evacuation destination at time t. denotes that agent j has arrived at their evacuation destination at time t, which represents a successful evacuation case. Let τ0 denote the time at which the flood evacuation order is issued to the public, and let τj and denote agent j's departure time (i.e., the time at which the agent begins their evacuation via the road network after their evacuation preparation time) and arrival time (i.e., the time at which agent j arrives at their evacuation destination), respectively. The agent's evacuation time ϕj is defined as the time period from their departure time τj to their arrival time (i.e., ).
By summarizing the evacuation statuses of the agents' over time, the effectiveness of flood evacuation processes in a region can be reflected by a matrix with two indicators at the system level: (1) the agents' average evacuation time Φ and (2) the system-level evacuation clearance time Γ. The agents' average evacuation time Φ is the average value of all of the agents' evacuation times, which is calculated by . In comparison, the system-level evacuation clearance time Γ for a region is the duration from the time at which the flood evacuation warning is issued in a residential area to the time at which the last agent arrives at their evacuation destination (i.e., ).
3.1 Study site
The Xiong'an New Area (XNA) is used as a case study to illustrate the functionality of the proposed modeling framework in flood simulation and evacuation management. The XNA is located in the Baiyangdian River basin, which includes the largest freshwater wetland in North China. This region covers three counties (Xiongxian, Rongcheng, and Anxin), encompassing a total area of 1768 km2 (Fig. 4). The region has a population of 1.1 million, and the gross domestic product is CNY 21.5 billion (Sun and Yang, 2019).
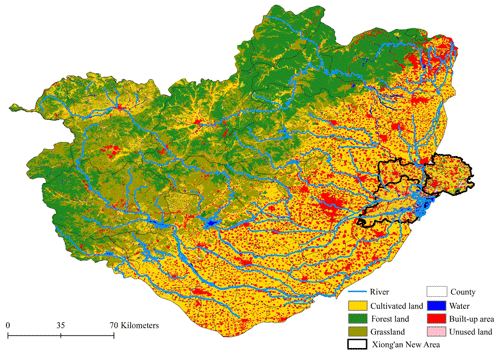
Figure 4Map of the Baiyangdian River basin and the Xiong'an New Area (marked with solid black lines).
The XNA has a typical continental monsoon climate, with annual average precipitation totaling approximately 570 mm. The region is influenced by various natural disasters and environmental problems, such as water pollution, heat waves, and groundwater overexploitation. In particular, the XNA has a high risk of flooding due to frequent extreme rainstorm events (Jiang et al., 2018; Su et al., 2021). Historical climate records show that a total of 139 flood events have occurred in the XNA over the past 300 years (Wang et al., 2020). For example, the heavy storm from 19 to 21 July 2016 affected a total population of approximately 517 000, leading to severe destruction and economic losses. Studies have found that, compared with historical flood conditions, both the frequency and intensity of extreme flood events in the region are expected to increase under future climate change (Zhu et al., 2017; Wang et al., 2020). The flood problems in the XNA and many other flood-prone areas worldwide call for the development of advanced computer models and decision support systems to allow for robust flood risk assessment and informed management practices during extreme flood events.
3.2 Data collection and model construction
Based on the modeling framework, data from various sources were collected and compiled to construct the model, including meteorological, land use, hydrological, transportation, and census data. Among them, land topology was retrieved from a 7 m resolution digital elevation model from the State Bureau of Surveying and Mapping. Meteorological data (e.g., daily precipitation, temperature, solar radiation, and wind speed) from 98 stations in the study area were collected from the China Meteorological Administration. Population and household distribution were based on 30 m resolution census data from the census bureau of the local government. Road network data were retrieved from OpenStreetMap, an open-source global map data repository. Table 1 presents the data used in this study as well as their sources.
Figure 5 illustrates how the data are merged and integrated into the modeling framework. As introduced in Sect. 2, the modeling process starts by running the MIKE 21 model, with meteorological, DEM, land use, soil type, and river network data as the model inputs. For a given storm event, the MIKE 21 model generates flood processes, which can be used to predict the inundated area and the affected population. These data are then used to construct the ABM and the MATSim model to simulate agents' flood management and evacuation behaviors.
3.3 Flood simulation and scenario design
As mentioned above, the case study site has a high risk of flooding due to frequent extreme rainstorm events. Following the precautionary principle in natural disaster management (Etkin et al., 2012), we use the 100-year flood event as an example to evaluate the impacts of extreme flooding on the study area and then examine the impacts of various management policies and human behaviors on household evacuation processes.
We run the hydrodynamic model to simulate flood inundation processes for the flood with a 100-year return period. The modeling results show that the inundated area is 66.5 % of the land area (Fig. 6). The affected population is 508 986 (45.8 % of the total population). These modeling results are consistent with the results that were reported in our prior work and are also empirically similar to the flood hazard experienced in this region in July 2016. For detailed introductions regarding the construction, calibration, and validation of the hydrodynamic model, the reader is referred to Wu et al. (2021). With such a high flood risk, it is essential for emergency responders to understand how flood evacuation performance is affected by various human behavioral factors and evacuation management plans.
A scenario-based analysis is conducted to examine the roles played by the following factors in flood evacuation simulations: (1) evacuation shelter establishment (i.e., the number and geographical distribution of shelter locations), (2) heterogeneity in households' evacuation preparation times, and (3) evacuees' route-searching strategies. Three experiments are designed to assess the joint impacts of the above three factors (Table 2), and these experiments are introduced in turn in the following.
Table 2Scenario design for simulating household evacuation processes.
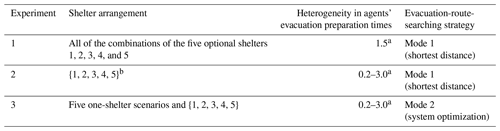
a Residents' behavioral heterogeneity is measured by the variation (i.e., standard deviation) in their evacuation preparation times. In the study area, the average evacuation preparation time of residents is set to 4 h based on our communication with the local flood management authorities. b The set {1, 2, 3, 4, 5} denotes that all five shelters are selected for this scenario.
The first experiment focuses on assessing the impact of the number and geographical distribution of evacuation shelters on agents' evacuation processes. Note that five optional sites for evacuation shelters are identified in the XNA based on the flood inundation area for the 100-year flood (illustrated by the red stars in Fig. 6). Considering all of the possible combinations of these shelters, a total of 31 simulations are performed in this experiment (5 simulations for single-shelter scenarios and 26 simulations for multiple-shelter scenarios). Experiment 2 assesses the impacts of agents' behavioral heterogeneity (i.e., variations in households' evacuation preparation times) on traffic flow and evacuation outcomes. Note that agents apply the shortest-distance route-searching method (Mode 1) to evacuate from their household locations to evacuation destinations in the first and second experiments. Experiment 3, in contrast, simulates evacuation processes in which agents apply the system-level optimization method (Mode 2) for route selection. The simulation results of experiment 3 are compared with those of the first and second experiments to explore the effects of agents' route-searching strategies on evacuation outcomes.
4.1 An example of household evacuation processes
In this study, the results of household evacuation simulations are extracted and analyzed with the Senozon Via data visualization tool (Milevich et al., 2016). Figure 7a presents a snapshot of residents' evacuation schemes for the case in which all five evacuation shelters are used in the study area (note that each household is illustrated by a green dot in Fig. 7a). Figure 7b depicts the change in the ratio of the three groups of the population during the evacuation processes. The percentage of the population in the S=1 group (i.e., the agents that have not started evacuating) displays a consistent decreasing trend, as more agents start their evacuation processes over time. Consequently, the S=3 group (i.e., the agents that have arrived at a safe zone) exhibits a consistent increasing trend. The S=2 group (i.e., the agents that have started evacuating but have not arrived at a safe zone, representing the residents who are moving in the road network) increases at the beginning of the evacuation period, reaching a peak of 43.1 % after approximately 6.5 h, and then decreases until the end of the evacuation period. The entire evacuation process takes approximately 15.5 h (i.e., the evacuation clearance time). In the following sections, the factors that influence the evacuation process will be assessed under different conditions.
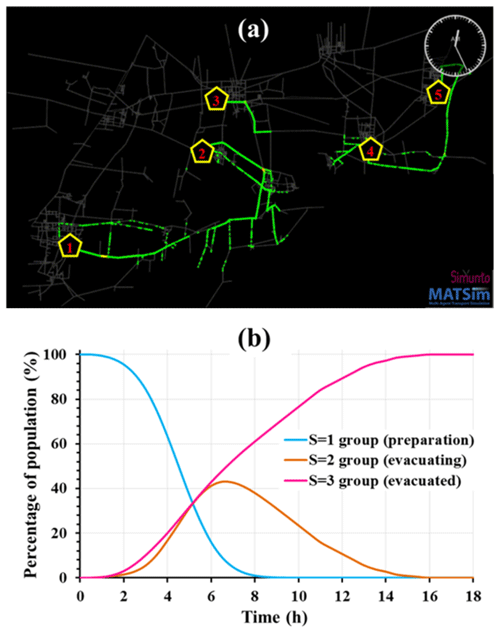
Figure 7(a) A snapshot of residents' evacuation schemes when all five evacuation shelters are established in the study area. (b) The percentages of the population in the three groups of agents. Note that the S=1 group includes agents who have not started evacuating, S=2 includes agents who have started evacuating but have not arrived at an evacuation destination, and S=3 includes agents who have successfully arrived at their destinations.
4.2 Impacts of shelter location arrangement on evacuation processes
We first conduct experiment 1 to examine agents' evacuation processes for the five scenarios in which only one evacuation shelter is established. Figure 8 shows that the geographical location of an evacuation shelter has a fundamentally important influence on residents' flood evacuation performance. Residents' average evacuation time is the shortest for shelter site 1 (20.1 h), followed by sites 2 (23.7 h), 5 (33.3 h), 3 (35.7 h), and 4 (46.8 h). The box plot of all of the agents' evacuation times also shows that the variation in agents' evacuation time is largest for shelter site 4 (32.4 h) and smallest for shelter site 1 (15.4 h). In terms of the system-level evacuation outcomes, shelter sites 1 and 2 are associated with the shortest evacuation clearance time (∼56 h), and shelter site 4 is associated with the longest evacuation clearance time (∼108.9 h) (see the inset in Fig. 8). In this regard, among the five optional shelter locations, sites 1 and 2 are the best locations for shelter establishment, and site 4 is the worst, with the longest evacuation time.
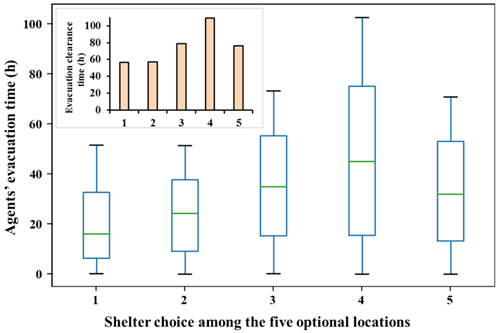
Figure 8Box plot of agents' evacuation times (main figure) and the system-level evacuation clearance times for the five one-shelter scenarios (inset).
Next, we compare the average evacuation time of agents for simulations in which all 31 combinations of the five optional evacuation shelter locations are considered. As shown in Fig. 9, when there is a small number of evacuation shelters, establishing more shelters in the system can notably reduce agents' evacuation times, and this effect is more noticeable for the worst-shelter-allocation scenario (illustrated by the blue line) than for the best-shelter-allocation scenario (illustrated by the red line). For example, as the number of shelters increases from two to three, the average evacuation time is reduced from 44.7 h (shelter set {4, 5}) to 29.7 h (shelter set {3, 4, 5}) for the worst-shelter-allocation scenario (a total reduction of 15 h). In contrast, the reduction in evacuation time is only 5 h for the best-shelter-allocation scenario (from 13.1 h for set {2, 3} to 8.1 h for set {1, 2, 3}). These results can yield policy implications in terms of the number and geographical locations of evacuation shelters needed to meet a particular flood management goal. For example, if the management goal is to evacuate all of the residents to a single safe zone, shelter 1 would be the best choice, among the five optional locations, in terms of minimizing the evacuation clearance time. However, for the case of establishing two shelters in the region, shelter set {2, 3} is a better choice compared with the other shelter site combinations.
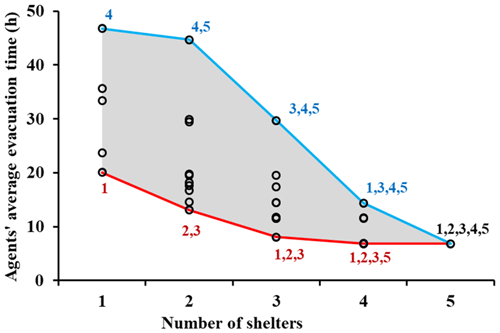
Figure 9The average evacuation time of residents under the scenarios that consider all of the possible combinations of the five optional evacuation shelters.
Notably, the modeling results show that agents' evacuation time decreases if shelters are located closer to denser residential areas. This is because a shelter located closer to denser areas can reduce agents' travel distances. Furthermore, the modeling results show that the reduction in residents' evacuation times, due to the increase in the number of evacuation shelters, is significantly affected by the existing number of evacuation shelters and, in particular, their geographical distribution in the region. After a certain number of evacuation shelters are established (more than three in this case), including more shelters in the system has a marginal effect on reducing evacuation times. Taking the best-shelter-allocation scenario as an example (the red line in Fig. 9), when there are only two evacuation shelters ({ 2, 3} ), adding one more evacuation shelter (1) in the system can reduce the evacuation time by 5 h (from 13.1 h for set { 2, 3} to 8.1 h for set {1, 2, 3}). In contrast, the reduction in evacuation time is only 1.3 h when shelter 5 is added to shelter set {1, 2, 3}. In particular, the average evacuation time is 6.8 h for shelter sets {1, 2, 3, 5} and {1, 2, 3, 4, 5}, which indicates that adding one more shelter in the system did not reduce the average evacuation time. This phenomenon is supported by the Braess paradox phenomena in the field of transportation research (Braess et al., 2005; Pas and Principio, 1997; Murchland, 1970), which suggests that including a new link in a traffic network could possibly result in heavier traffic congestion and longer travel times. This phenomenon and its policy implications will be further discussed in Sect. 5.
4.3 Impacts of residents' behavioral heterogeneity on evacuation processes
Previous studies have shown that the evacuation preparation time of households plays an important role in their emergency evacuation outcomes during natural disasters (Lindell et al., 2005, 2020). However, the heterogeneity in human behaviors has not been explicitly examined in flood evacuation processes. In this section, we conduct experiment 2 to assess the impacts of human behavioral heterogeneity (measured by the variance in agents' evacuation preparation times) on evacuation processes. Figure 10 shows that human behavioral heterogeneity has a nonlinear effect on agents' evacuation outcomes. Increasing the heterogeneity in households' evacuation preparation times will result in reductions in the average evacuation time and the system-level evacuation clearance time, and this effect is more significant when the variation in the evacuation preparation time is small (< 1.5 h). In particular, when the variation in preparation time is large (> 2 h), the change in the heterogeneity of preparation times will not notably affect the average evacuation time or the system-level evacuation clearance time. These results are consistent with the modeling results obtained from our prior work, which examined the role of heterogeneity in residents' tolerance to flood risk during evacuation processes (Du et al., 2016).
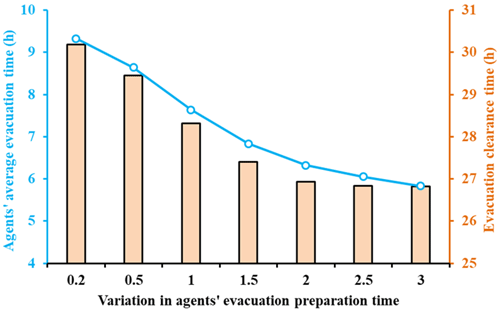
Figure 10The impacts of human behavioral heterogeneity (i.e., the variation in agents' evacuation preparation times) on their average evacuation time (left y axis) and the system-level evacuation clearance time (right y axis).
Next, we assess the impacts of human behavioral heterogeneity on the traffic flow conditions in the road network. Figure 11 plots the percentage of the three groups of the population during evacuation processes, and the S=2 group (illustrated by the two brown lines) includes the agents who are evacuating in the road network. The modeling results show that the peak traffic time (i.e., the time at which the number of agents in the road network reaches a maximum during the evacuation period) is delayed as the level of agent behavioral heterogeneity increases. In addition, the percentage of agents in the road network at the peak traffic time is significantly lower in the high-behavioral-heterogeneity scenario than in other scenarios. For example, the traffic peak time can be delayed from 6.0 to 8.5 h as the variation in the evacuation preparation times increases from 1.0 to 3.0 h. At the time of the traffic peak, the percentage of agents in the road network is reduced from 67.9 % (the low-heterogeneity scenario) to 46.6 % (the high-heterogeneity scenario), and the system-level evacuation clearance time is reduced from 28.5 h (the low-heterogeneity scenario) to 27 h (the high-heterogeneity scenario). Figure 12 compares the peak traffic time and the percentage of evacuating agents at the peak time under various levels of heterogeneity in agents' evacuation preparation times. The modeling results show that flood evacuation outcomes can be improved (i.e., the traffic congestion problem is alleviated, the peak traffic time is delayed, and the evacuation clearance time is reduced) as agents' behavioral heterogeneity increases.
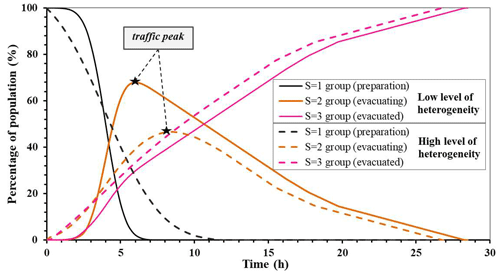
Figure 11Comparison of the evacuation processes for low (solid lines) and high (dotted lines) levels of human behavioral heterogeneity. Note that agents' behavioral heterogeneity is measured by the standard deviation of their evacuation preparation time, and the low and high levels of heterogeneity are 1.0 and 3.0 h, respectively.
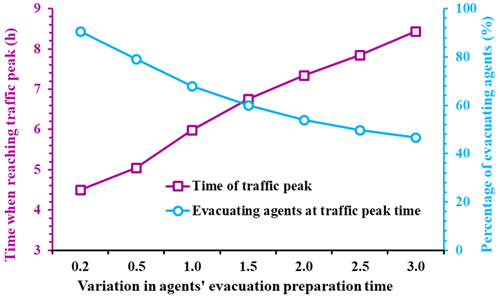
Figure 12Peak traffic time (left y axis) and the percentage of evacuating agents (i.e., S=2 group) at the peak traffic time (right y axis) for various levels of human behavioral heterogeneity.
These modeling results highlight the importance for policy-makers to pay explicit attention to households' behavioral heterogeneity during flood evacuation processes. For example, the modeling results show that the variation in agents' departure times can significantly affect the traffic load in the road network and the evacuation clearance time. Traffic congestion conditions can be alleviated if the variation in agents' departure times is larger. Thus, to improve evacuation efficiency, emergency responders may need to divide all of the households in the community into a number of groups and guide them to evacuate in batches, rather than allowing them to begin their evacuation in a chaotic manner without appropriate coordination.
4.4 Impacts of households' evacuation route choices on evacuation processes
In the above sections, the modeling results for scenarios in which the agents apply the shortest-distance route-searching method to travel from their original locations to destinations (Mode 1) during evacuation processes were presented. In this section, we conduct experiment 3, in which agents' evacuation routes are obtained based on a system-level optimization approach (Mode 2). We then compare the three experiments to explore the joint impacts of the route-searching method and the behavioral heterogeneity of residents on evacuation processes.
Figure 13 compares agents' average evacuation times for the two travel modes. Two implications are obtained from the modeling results. First, the results show that the average evacuation time is consistently smaller for Mode 2 than for Mode 1. This result agrees with the common belief in transportation research that traffic congestion will likely occur in the road network if each agent selects their shortest evacuation route without considering the effects of other agents' route choices. In contrast, traffic flow conditions can be improved if agents' evacuation route choices are optimized from the system level, leading to a noticeable reduction in traffic congestion and shorter evacuation times. Second, one can observe that the variation in evacuation time across different shelter establishment scenarios is significantly higher for Mode 1 than for Mode 2. For example, among the five one-shelter scenarios, the agents' average evacuation time ranges from 46.7 to 20.1 h (a difference of 26.6 h) for Mode 1. In contrast, this value ranges from 16.5 to 9.2 h (a difference of 7.3 h) for Mode 2. This result implies that shelter establishment plays a more important role when residents only seek to minimize their individual evacuation times. In comparison, if agents' evacuation routes are optimized from the system level, shelter establishment will become a less significant factor affecting evacuation performance.
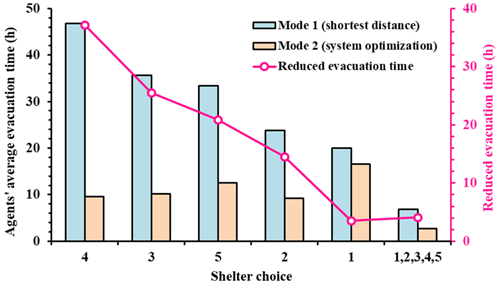
Figure 13Comparison of the average evacuation time of agents for the two evacuation-route-searching strategies.
Figure 14 presents the percentages of the three groups of agents during the evacuation process in order to explicitly examine the impacts of different route-searching strategies. Compared with the shortest-distance route-searching strategy (Mode 1), the system-level optimization route-searching strategy (Mode 2) can reduce the evacuation clearance time by 12 h (from 27.5 h for Mode 1 to 15.5 h for Mode 2). In addition, the percentage of agents in the road network at the peak traffic time is reduced from 60.4 % for Mode 1 to 43.1 % for Mode 2, indicative of a significant improvement in traffic congestion during the evacuation period. However, the peak traffic time is similar in the two scenarios, suggesting that changing agents' route-searching strategies does not considerably affect the peak time of traffic flow.
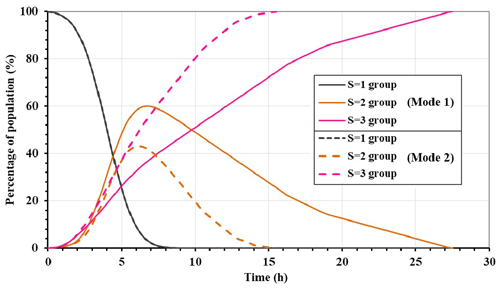
Figure 14Comparison of residents' evacuation processes for the two route-searching strategies (note that all five evacuation shelters are selected for the two scenarios, and the variation in residents' evacuation preparation times is 1.5 h).
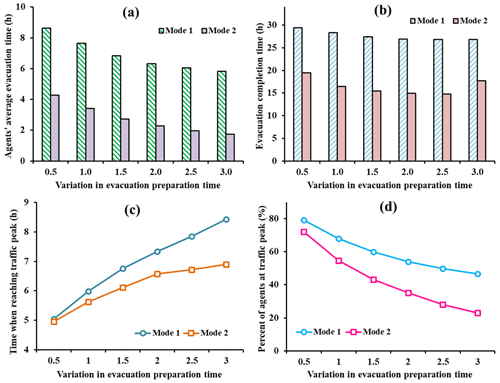
Figure 15The joint impacts of evacuation-route-searching strategies and the variations in agents' evacuation preparation times on (a) the average evacuation time, (b) the system-level evacuation clearance time, (c) the time at which the traffic peak is reached during evacuation processes, and (d) the percentage of evacuating agents at the peak traffic time.
The above analyses focused on assessing the impacts of a single factor (agents' behavioral heterogeneity or evacuation-route-searching strategies). Figure 15 examines how the two factors jointly affect evacuation processes. Notably, in general, the average evacuation time of agents and the system-level evacuation clearance time are small when the variation in the evacuation preparation time is low and/or when agents use Mode 2 to determine their evacuation routes. Interestingly, when the variation in agents' evacuation preparation times is low (< 1.0 h), the difference between Mode 1 and Mode 2 is not significant in terms of the peak traffic time nor the percentage of evacuating agents at the peak traffic time. This result indicates that changing agents' route-searching strategies will not considerably affect the peak traffic time nor the maximum traffic flow if all of the agents start their evacuation activities within a short time window. In contrast, as the variation in the evacuation preparation time of agents increases, the evacuation-route-searching strategy used can significantly affect the peak traffic time and the maximum traffic flow (Fig. 15c, d). However, the variation in agents' evacuation preparation times does not notably affect the changes in the average evacuation time or system-level evacuation clearance time between the two route-searching strategies.
The comparisons of the two route-searching methods, as have been presented in the above sections, show that households' route choices play an important role in their evacuation processes. Evacuation clearance time and traffic congestion will be significantly alleviated and become more robust against the change in shelter location arrangement if evacuation routes are optimized. In this regard, policy-makers may improve flood management by providing clear guidance to all of the households in terms of where they should evacuate to (i.e., shelter choice), when they should evacuate (i.e., departure time), and, in particular, via which route they should evacuate (i.e., route selection). On the other hand, households need to follow the evacuation guidance and take the recommended routes to improve evacuation efficiency.
5.1 Implications for flood risk assessment and evacuation management
In this study, we employ an interdisciplinary socio-hydrological approach that incorporates a physically based hydrodynamic model, an agent-based human behavior model, and a large-scale transportation model into an integrated modeling framework. The proposed modeling framework is motivated by previous socio-hydrological studies that called for the incorporation of various factors in the context of coupled human–flood systems to support flood management. These factors may be associated with a wide range of interdisciplinary domains, such as hydrogeological conditions, flood inundation process, information dissemination platforms, risk perception and awareness, social preparedness, public policy, and urban infrastructure development (Barendrecht et al., 2019; Di Baldassarre et al., 2013; Yu et al., 2022; Pande and Sivapalan, 2017; Troy et al., 2015; Fuchs et al., 2017; Viglione et al., 2014). We apply the model to the XNA in China to assess the inundated areas of an extreme flood event and to examine household evacuation outcomes under various management policies and human behaviors. Several modeling and policy implications can be obtained based on the model construction and simulation results.
First, the simulation results of this study show that the flood risk and flood damage to an area are affected not only by the hydrological characteristics of flood events but also by infrastructural, socioeconomic, and human behavioral factors. In particular, the results show that household evacuation outcomes are significantly affected by shelter location arrangement, route selection strategies, and evacuation preparation times. Therefore, it is essential for researchers and policy-makers to incorporate various social, hydrological, and human behavioral factors into an integrated framework to obtain more robust estimations of flood risk and to design informed policies to support holistic flood management.
Second, the modeling results show that the number of evacuation shelters and, in particular, their geographical distributions have important effects on flood evacuation processes. For example, by comparing the evacuation outcomes obtained for the five optional shelter sites in the case study area, we find that the average evacuation time of residents varies from 20.1 h (shelter site 1) to 46.8 h (shelter site 4) (Fig. 8). In this regard, if there are limited available resources and only one evacuation site can be established in the area, shelter 1 would be a better site than shelter 4 if the management goal is to minimize the average evacuation time of residents. Another implication associated with shelter choice is that establishing more shelters in the area does not necessarily lead to improvements in a community's evacuation processes if there is already a sufficient number of evacuation shelters or if the shelters are not well distributed in the region. For example, in the case in which there are three shelters (e.g., { 1, 2, 3}), including more shelters in the system (e.g., 4, 5, or both) will not effectively reduce the average evacuation time of households (Fig. 8). This finding, although somewhat contrary to what one would intuitively expect, is in line with the classic Braess paradox in the field of transportation research; notably, adding a new link in a traffic network may not improve the operation of the traffic system (Frank, 1981; Murchland, 1970). Some studies have shown that the occurrence of Braess paradox phenomena may be affected by the road network configuration, travel demand, and travelers' route-searching behaviors (Pas and Principio, 1997; Braess et al., 2005). Therefore, regarding emergency management policies, such as where to establish new shelters, policy-makers need to assess the relationships among these factors to determine the number and geographic distribution of shelters in the system.
Third, flood evacuation is a complex process in which residents' evacuation activities can be affected by various social, economic, environmental, and infrastructural factors. Thus, in a particular flood-prone area, residents' decisions and evacuation behaviors could be highly heterogeneous, varying from family to family, from community to community, and from time to time (Paul, 2012; Huang et al., 2017). This study shows that human behavioral heterogeneity can significantly affect flood evacuation outcomes in a given region. For example, the modeling results show that variations in residents' evacuation preparation times could result in noticeable differences in traffic congestion conditions and the time required for evacuees to complete their evacuation processes (Figs. 10, 11, 12). Therefore, in flood management practice, emergency responders need to explicitly consider the heterogeneity in residents' behaviors and determine how to promote behavioral changes by providing the needed resources to vulnerable groups who are not able to take effective flood mitigation actions to improve the overall disaster management performance of the community (Nakanishi et al., 2019; Hino and Nance, 2021).
5.2 Limitations and future research directions
Our modeling framework and the simulations in this study have a number of limitations that warrant future research to make improvements and extend the current approach. First, similar to other studies on emergency evacuation simulation (Wood et al., 2020; Zhu et al., 2018; Koch et al., 2020; Saadi et al., 2018), this work focuses on car-based traffic simulation without considering other transportation modes (e.g., motorcycles). In real-world evacuation cases, residents may use various types of transportation modes to evacuate, including automobiles, motorcycles, buses, or by foot (Melnikov et al., 2016). Residents may also change their travel modes during evacuation processes, for example, due to a change in the available transportation facilities. Recent studies have attempted to improve emergency evacuation simulations by considering more factors in evacuation simulation, such as multiple transportation facilities, changes in traffic network accessibility, variations in travel demand, pedestrian–vehicle interactions, and speed adjustments (Dias et al., 2021; Takabatake et al., 2020; Wang and Jia, 2021; Sun et al., 2020; Chen et al., 2022). Future study could also improve the transportation model to consider more complex agent–agent and agent–environment interactions during evacuation processes. For instance, besides the two route-searching methods that have been analyzed in this study, future work may consider another type of route-searching method in which agents have full access to real-time information on traffic conditions and may decide to change their evacuation routes over time (referred to as mode 3). The three travel modes can be systematically compared in order to achieve a better understanding of how agents' route-searching strategies may affect their evacuation results. This extension will enhance the functionality of the MATSim transportation model and improve the simulation of agent behaviors during community evacuation processes.
Second, regarding the analyses of shelter establishment, we primarily focus on the number and geographical distribution of evacuation shelters without considering other important shelter characteristics, such as shelter capacity. However, it is sometimes necessary to consider the constraint of shelter capacity in evacuation management, especially in large-scale evacuation scenarios. Recently, studies have analyzed the impacts of shelter capacities and their geographic distribution on evacuation outcomes (Alam et al., 2021; Khalilpourazari and Pasandideh, 2021; Oh et al., 2021; Liu and Lim, 2016). Future studies should consider more shelter properties to improve the current modeling framework.
Third, in this work, the hydrodynamic model is coupled with the ABM and the transportation model in a one-way coupling manner. Therefore, the hydrodynamic model generates flood inundation results as the input for the ABM and the transportation model, but the modeling results of the ABM and the transportation model do not affect the hydrodynamic modeling process. Such a one-way model-coupling method is suitable for simulating residents' evacuation activities before a flood occurs, but it is not suitable for cases in which evacuation processes and flood inundation processes have an overlapping time period. In particular, the model is not capable of simulating how human behaviors affect river channel and flood inundation processes (Chen et al., 2016; Witkowski, 2021). This is another limitation that needs to be addressed in future work.
Finally, it is worth noting that this study is still subject to many simplifications and assumptions due to data incompleteness and the specific research scope of the current work. Future work could incorporate more psychological and social factors to describe agents' decisions during evacuation processes. For example, future study can conduct surveys and questionnaires to quantify households' evacuation preparation times after receiving flood evacuation orders (Lindell et al., 2020). Moreover, future studies could consider other factors that may affect human flood risk perception and risk awareness, such as social memories, social interactions, and observations of neighbors' actions (Du et al., 2017; Girons Lopez et al., 2017). These extensions and improvements can make the model capable of simulating more realistic decision-making processes and more complex human–flood interactions to support emergency management during floods.
A fundamental aspect of societal security is natural disaster management. Computational models are needed to assess the flood risk in flood-prone areas and to design holistic management policies for flood warning and damage mitigation. In this study, we propose an integrated socio-hydrological modeling framework that couples a hydrodynamic model for simulating flood inundation processes, an ABM for simulating the flood management practices of emergency responders and human behaviors, and a large-scale transportation model for simulating household evacuation processes in a road network. Using a case study of the XNA in China, we demonstrate the effectiveness of the modeling framework with respect to assessing flood inundation processes for a 100-year flood event and examining households' evacuation outcomes considering various evacuation management policies and human behaviors. A number of scenario analyses are performed to explore the impacts of shelter location arrangement, evacuation preparation times, and route-searching strategies on evacuation performance.
Via a set of scenario analyses, the modeling results show that, for a 100-year flood event, approximately 66.5 % of the land area will be flooded, affecting 0.5 million people. Household evacuation processes can be significantly affected by the number and geographical distribution of evacuation shelters. For the five optional evacuation shelter sites, the average evacuation time of residents ranges from 20.1 to 46.8 h, depending on where the evacuation shelters are located. Counterintuitively, yet in line with the Braess paradox in the transportation field, we find that including more shelters in the system may not improve evacuation performance in a region if the number of shelters or the shelter distribution is already optimal or near optimal. In addition, the simulation results show that residents' flood evacuation outcomes are significantly affected by human decision-making processes, such as the selection of evacuation-route-searching strategies. Compared with the system-level route optimization method, the shortest-distance route-searching method is associated with a longer evacuation travel time because evacuees seeking to minimize their own travel time may experience traffic congestion. We also find that a low level of heterogeneity in agents' evacuation preparation times can result in heavy traffic congestion and long evacuation clearance times. These modeling results indicate that the flood risk of, and the ultimate damage to, an area is affected not only by the magnitude of the flood itself but also by flood management practices and household behavioral factors. Therefore, this study is in line with some previous studies that have highlighted the significance of using socio-hydrological methods for hydrological science and watershed management (Di Baldassarre et al., 2013; Sivapalan et al., 2012; Abebe et al., 2019).
This work still has a number of limitations that need to be addressed. Recommended future work includes the incorporation of more types of transportation facilities and route selection methods in the transportation simulation model, the consideration of more psychological and behavioral factors in human decision-making, and the improvement of the model-coupling method by employing a two-way coupling approach to simulate the impacts of human behaviors on flood inundation processes. We envision that these extensions will improve the functionality of the proposed modeling framework and that the simulation results with these improvements can provide more useful modeling and policy implications to support flood risk assessment and emergency evacuation management.
The code used in this study is available from the corresponding author upon request.
The data used in this study can be freely accessed from the following data repository: https://doi.org/10.5281/zenodo.7797361 (Du et al., 2023).
ED and CZ designed this study. HJ, ED, NG, and FW developed the model and wrote the code. HJ, ED, and YT performed the experiments. ED analyzed the results and wrote the original draft. CZ, FW, and YT edited the paper.
The contact author has declared that none of the authors has any competing interests.
Publisher's note: Copernicus Publications remains neutral with regard to jurisdictional claims in published maps and institutional affiliations.
The authors are grateful for the insightful comments and suggestions from the editor, Jianshi Zhao, and one anonymous referee that significantly improved this paper.
This research has been supported by the National Natural Science Foundation of China (grant nos. 41971233, 51909118, and 41861124003).
This paper was edited by Fuqiang Tian and reviewed by Jianshi Zhao and one anonymous referee.
Abebe, Y. A., Ghorbani, A., Nikolic, I., Vojinovic, Z., and Sanchez, A.: A coupled flood-agent-institution modelling (CLAIM) framework for urban flood risk management, Environ. Modell. Softw., 111, 483–492, https://doi.org/10.1016/j.envsoft.2018.10.015, 2019.
Aerts, J. C. J. H., Botzen, W. J., Clarke, K. C., Cutter, S. L., Hall, J. W., Merz, B., Michel-Kerjan, E., Mysiak, J., Surminski, S., and Kunreuther, H.: Integrating human behaviour dynamics into flood disaster risk assessment, Nat. Clim. Change, 8, 193–199, https://doi.org/10.1038/s41558-018-0085-1, 2018.
Alam, M. J., Habib, M. A., and Pothier, E.: Shelter locations in evacuation: A Multiple Criteria Evaluation combined with flood risk and traffic microsimulation modeling, Int. J. Disaster Risk R., 53, 102016, https://doi.org/10.1016/j.ijdrr.2020.102016, 2021.
Alçada-Almeida, L., Tralhão, L., Santos, L., and Coutinho-Rodrigues, J.: A multiobjective approach to locate emergency shelters and identify evacuation routes in urban areas, Geogr. Anal., 41, 9–29, https://doi.org/10.1111/j.1538-4632.2009.00745.x, 2009.
Alonso Vicario, S., Mazzoleni, M., Bhamidipati, S., Gharesifard, M., Ridolfi, E., Pandolfo, C., and Alfonso, L.: Unravelling the influence of human behaviour on reducing casualties during flood evacuation, Hydrolog. Sci. J., 65, 2359–2375, https://doi.org/10.1080/02626667.2020.1810254, 2020.
Barendrecht, M. H., Viglione, A., Kreibich, H., Merz, B., Vorogushyn, S., and Blöschl, G.: The Value of Empirical Data for Estimating the Parameters of a Sociohydrological Flood Risk Model, Water Resour. Res., 55, 1312–1336, https://doi.org/10.1029/2018WR024128, 2019.
Bayram, V., Tansel, B. T., and Yaman, H.: Compromising system and user interests in shelter location and evacuation planning, Transp. Res. B, 72, 146–163, https://doi.org/10.1016/j.trb.2014.11.010, 2015.
Bernardini, G., Santarelli, S., Quagliarini, E., and Orazio, M. D.: Dynamic guidance tool for a safer earthquake pedestrian evacuation in urban systems, Comput. Environ. Urban Syst., 65, 150–161, https://doi.org/10.1016/j.compenvurbsys.2017.07.001, 2017.
Bhatt, G., Kumar, M., and Duffy, C. J.: A tightly coupled GIS and distributed hydrologic modeling framework, Environ. Modell. Softw., 62, 70–84, https://doi.org/10.1016/j.envsoft.2014.08.003, 2014.
Braess, D., Nagurney, A., and Wakolbinger, T.: On a Paradox of Traffic Planning, Transp. Sci., 39, 446–450, https://doi.org/10.1287/trsc.1050.0127, 2005.
Brunner, M. I., Papalexiou, S., Clark, M. P., and Gilleland, E.: How Probable Is Widespread Flooding in the United States?, Water Resour. Res., 56, 1–16, https://doi.org/10.1029/2020WR028096, 2020.
Chen, C., Lindell, M. K., and Wang, H.: Tsunami preparedness and resilience in the Cascadia Subduction Zone: A multistage model of expected evacuation decisions and mode choice, Int. J. Disaster Risk Re., 59, 102244, https://doi.org/10.1016/j.ijdrr.2021.102244, 2021.
Chen, C., Mostafizi, A., Wang, H., Cox, D., and Chand, C.: An integrative agent-based vertical evacuation risk assessment model for near-field tsunami hazards, Risk Anal., 1, 1–15, https://doi.org/10.1111/risa.13881, 2022.
Chen, X. and Zhan, F. B.: Agent-based modelling and simulation of urban evacuation: Relative effectiveness of simultaneous and staged evacuation strategies, J. Oper. Res. Soc., 59, 25–33, https://doi.org/10.1057/palgrave.jors.2602321, 2008.
Chen, X., Wang, D., Tian, F., and Sivapalan, M.: From channelization to restoration: Sociohydrologic modeling with changing community preferences in the Kissimmee River Basin, Florida, Water Resour. Res., 52, 1227–1244, https://doi.org/10.1002/2015WR018194, 2016.
Dias, C., Rahman, N. A., and Zaiter, A.: Evacuation under flooded conditions: Experimental investigation of the influence of water depth on walking behaviors, Int. J. Disaster Risk Re., 58, 102192, https://doi.org/10.1016/j.ijdrr.2021.102192, 2021.
Di Baldassarre, G., Viglione, A., Carr, G., Kuil, L., Salinas, J. L., and Blöschl, G.: Socio-hydrology: conceptualising human-flood interactions, Hydrol. Earth Syst. Sci., 17, 3295–3303, https://doi.org/10.5194/hess-17-3295-2013, 2013.
Du, E., Rivera, S., Cai, X., Myers, L., Ernest, A., and Minsker, B.: Impacts of human behavioral heterogeneity on the benefits of probabilistic flood warnings: An agent-based modeling framework, J. Am. Water Resour. As., 53, 316–332, https://doi.org/10.1111/1752-1688.12475, 2016.
Du, E., Cai, X., Sun, Z., and Minsker, B.: Exploring the Role of Social Media and Individual Behaviors in Flood Evacuation Processes: An Agent-Based Modeling Approach, Water Resour. Res., 53, 9164–9180, https://doi.org/10.1002/2017WR021192, 2017.
Du, E., Tian, Y., Cai, X., Zheng, Y., Li, X., and Zheng, C.: Exploring spatial heterogeneity and temporal dynamics of human-hydrological interactions in large river basins with intensive agriculture: A tightly coupled, fully integrated modeling approach, J. Hydrol., 591, 125313, https://doi.org/10.1016/j.jhydrol.2020.125313, 2020.
Du, E., Wu, F., Jiang, H., Guo, N., Tian, Y., and Zheng, C.: Dataset for the article “Development of an integrated socio-hydrological modeling framework for assessing the impacts of shelter location arrangement and human behaviors on flood evacuation processes” (Version 1.0), Zenodo [data set], https://doi.org/10.5281/zenodo.7797361, 2023.
Etkin, D., Medalye, J., and Higuchi, K.: Climate warming and natural disaster management: An exploration of the issues, Clim. Change, 112, 585–599, https://doi.org/10.1007/s10584-011-0259-6, 2012.
Farkas, K., Nagy, A., Tomas, T., and Szabo, R.: Participatory sensing based real-time public transport information service, in: IEEE International Conference on Pervasive Computing and Communication Workshops, Budapest, Hungary, 24–28 March 2014, 141–144, https://doi.org/10.1109/PerComW.2014.6815181, 2014.
Frank, M.: The Braess paradox, Math. Program., 20, 283–302, https://doi.org/10.1007/BF01589354, 1981.
Fu, L., Sun, D., and Rilett, L. R.: Heuristic shortest path algorithms for transportation applications: State of the art, Comput. Oper. Res., 33, 3324–3343, https://doi.org/10.1016/j.cor.2005.03.027, 2006.
Fuchs, S., Karagiorgos, K., Kitikidou, K., Maris, F., Paparrizos, S., and Thaler, T.: Flood risk perception and adaptation capacity: a contribution to the socio-hydrology debate, Hydrol. Earth Syst. Sci., 21, 3183–3198, https://doi.org/10.5194/hess-21-3183-2017, 2017.
Gallo, G. and Pallottino, S.: Shortest path algorithms, Ann. Oper. Res., 13, 1–79, 1988.
Girons Lopez, M., Di Baldassarre, G., and Seibert, J.: Impact of social preparedness on flood early warning systems, Water Resour. Res., 53, 522–534, https://doi.org/10.1002/2016WR019387, 2017.
Goodarzi, L., Banihabib, M. E., and Roozbahani, A.: A decision-making model for flood warning system based on ensemble forecasts, J. Hydrol., 573, 207–219, https://doi.org/10.1016/j.jhydrol.2019.03.040, 2019.
Guo, K., Guan, M., and Yu, D.: Urban surface water flood modelling – a comprehensive review of current models and future challenges, Hydrol. Earth Syst. Sci., 25, 2843–2860, https://doi.org/10.5194/hess-25-2843-2021, 2021.
Harvey, E. P., Cardwell, R. C., McDonald, G. W., van Delden, H., Vanhout, R., Smith, N. J., Kim, J. hwan, Forgie, V. E., and van den Belt, M.: Developing integrated models by coupling together existing models; land use, economics, demographics and transport in Wellington, New Zealand, Comput. Environ. Urban, 74, 100–113, https://doi.org/10.1016/j.compenvurbsys.2018.07.004, 2019.
Hasan, S., Ukkusuri, S., Gladwin, H., and Murray-Tuite, P.: Behavioral model to understand household-level hurricane evacuation decision making, J. Transp. Eng., 137, 341–348, https://doi.org/10.1061/(ASCE)TE.1943-5436.0000223, 2011.
He, M., Chen, C., Zheng, F., Chen, Q., Zhang, J., Yan, H., and Lin, Y.: An efficient dynamic route optimization for urban flooding evacuation based on Cellular Automata, Comput. Environ. Urban, 87, 101622, https://doi.org/10.1016/j.compenvurbsys.2021.101622, 2021.
Hino, M. and Nance, E.: Five ways to ensure flood-risk research helps the most vulnerable, Nature, 595, 27–29, https://doi.org/10.1038/d41586-021-01750-0, 2021.
Hofflinger, A., Somos-Valenzuela, M. A., and Vallejos-Romero, A.: Response time to flood events using a social vulnerability index (ReTSVI), Nat. Hazards Earth Syst. Sci., 19, 251–267, https://doi.org/10.5194/nhess-19-251-2019, 2019.
Horni, A.: Multi-agent Transport Simulation Matsim, Ubiquity Press, London, https://doi.org/10.5334/baw, 2016.
Huang, S., Lindell, M. K., Prater, C. S., Wu, H., and Siebeneck, L. K.: Household evacuation decision making in response to Hurricane Ike, Nat. Hazards Rev., 13, 283–296, https://doi.org/10.1061/(ASCE)NH.1527-6996.0000074, 2012.
Huang, S.-K., Lindell, M. K., and Prater, C. S.: Multistage Model of Hurricane Evacuation Decision: Empirical Study of Hurricanes Katrina and Rita, Nat. Hazards Rev., 18, 05016008, https://doi.org/10.1061/(asce)nh.1527-6996.0000237, 2017.
Islam, K. A., Marathe, M., Mortveit, H., Swarup, S., and Vullikanti, A.: A Simulation-based Approach for Large-scale Evacuation Planning, in: IEEE International Conference on Big Data, 1338–1345, https://doi.org/10.1109/BigData50022.2020.9377794, 2020.
Jiang, R., Yu, X., Xie, J., Zhao, Y., Li, F., and Yang, M.: Recent changes in daily climate extremes in a serious water shortage metropolitan region, a case study in Jing-Jin-Ji of China, Theor. Appl. Climatol., 134, 565–584, https://doi.org/10.1007/s00704-017-2293-4, 2018.
Jongman, B., Ward, P. J., and Aerts, J. C. J. H.: Global exposure to river and coastal flooding: Long term trends and changes, Glob. Environ. Chang., 22, 823–835, https://doi.org/10.1016/j.gloenvcha.2012.07.004, 2012.
Khalilpourazari, S. and Pasandideh, S. H. R.: Designing emergency flood evacuation plans using robust optimization and artificial intelligence, J. Comb. Optim., 41, 640–677, https://doi.org/10.1007/s10878-021-00699-0, 2021.
Koch, Z., Yuan, M., and Bristow, E.: Emergency Response after Disaster Strikes: Agent-Based Simulation of Ambulances in New Windsor, NY, J. Infrastruct. Syst., 26, 06020001, https://doi.org/10.1061/(asce)is.1943-555x.0000565, 2020.
Kreibich, H., van den Bergh, J. C. J. M., Bouwer, L. M., Bubeck, P., Ciavola, P., Green, C., Hallegatte, S., Logar, I., Meyer, V., Schwarze, R., and Thieken, A. H.: Costing natural hazards, Nat. Clim. Chang., 4, 303–306, https://doi.org/10.1038/nclimate2182, 2014.
Kreibich, H., Bubeck, P., Van Vliet, M., and De Moel, H.: A review of damage-reducing measures to manage fluvial flood risks in a changing climate, Mitig. Adapt. Strat. Gl., 20, 967–989, https://doi.org/10.1007/s11027-014-9629-5, 2015.
Lämmel, G., Klüpfel, H., and Nagel, K.: The MATSim Network Flow Model for Traffic Simulation Adapted to Large-Scale Emergency Egress and an Application to the Evacuation of the Indonesian City of Padang in Case of a Tsunami Warning, in: Pedestrian Behavior, edited by: Timmermans, H., Emerald Group Publishing Limited, 245–265, https://doi.org/10.1108/9781848557512-011, 2009.
Lämmel, G., Grether, D., and Nagel, K.: The representation and implementation of time-dependent inundation in large-scale microscopic evacuation simulations, Transp. Res. Part C, 18, 84–98, https://doi.org/10.1016/j.trc.2009.04.020, 2010.
Lee, K. S., Eom, J. K., and Moon, D.: Applications of TRANSIMS in transportation: A literature review, Procedia Comput. Sci., 32, 769–773, https://doi.org/10.1016/j.procs.2014.05.489, 2014.
Li, A. C. Y., Nozick, L., Xu, N., and Davidson, R.: Shelter location and transportation planning under hurricane conditions, Transp. Res. Part E, 48, 715–729, https://doi.org/10.1016/j.tre.2011.12.004, 2012.
Li, B., Hou, J., Ma, Y., Bai, G., Wang, T., Xu, G., Wu, B., and Jiao, Y.: A coupled high-resolution hydrodynamic and cellular automata-based evacuation route planning model for pedestrians in flooding scenarios, Nat. Hazards, 110, 607–628, https://doi.org/10.1007/s11069-021-04960-x, 2022.
Li, X., Zhang, L., Zheng, Y., Yang, D., Wu, F., Tian, Y., Han, F., Gao, B., Li, H., Zhang, Y., Ge, Y., Cheng, G., Fu, B., Xia, J., Song, C., and Zheng, C.: Novel hybrid coupling of ecohydrology and socioeconomy at river basin scale: A watershed system model for the Heihe River basin, Environ. Modell. Softw., 141, 105058, https://doi.org/10.1016/j.envsoft.2021.105058, 2021.
Lindell, M., Sorensen, J., Baker, E., and Lehman, W.: Community response to hurricane threat: Estimates of household evacuation preparation time distributions, Transp. Res. Part D, 85, 102457, https://doi.org/10.1016/j.trd.2020.102457, 2020.
Lindell, M. K., Lu, J.-C., and Prater, C. S.: Household decision making and evacuation in response to hurricane Lili, Nat. Hazards Rev., 6, 171–179, https://doi.org/10.1061/(ASCE)1527-6988(2005)6:4(171), 2005.
Liu, X. and Lim, S.: Integration of spatial analysis and an agent-based model into evacuation management for shelter assignment and routing, J. Spat. Sci., 61, 283–298, https://doi.org/10.1080/14498596.2016.1147393, 2016.
Mahmud, K. and Town, G. E.: A review of computer tools for modeling electric vehicle energy requirements and their impact on power distribution networks, Appl. Energ., 172, 337–359, https://doi.org/10.1016/j.apenergy.2016.03.100, 2016.
McClymont, K., Morrison, D., Beevers, L., and Carmen, E.: Flood resilience: a systematic review, J. Environ. Plann. Man., 63, 1151–1176, https://doi.org/10.1080/09640568.2019.1641474, 2020.
Melnikov, V. R., Krzhizhanovskaya, V. V, Lees, M. H., and Boukhanovsky, A. V: Data-driven Travel Demand Modelling and Agent-based Traffic Simulation in Amsterdam Urban Area, Procedia Comput. Sci., 80, 2030–2041, https://doi.org/10.1016/j.procs.2016.05.523, 2016.
Milevich, D., Melnikov, V., Karbovskii, V., and Krzhizhanovskaya, V.: Simulating an impact of road network improvements on the performance of transportation systems under critical load: Agent-based Approach, Procedia Comput. Sci., 101, 253–261, https://doi.org/10.1016/j.procs.2016.11.030, 2016.
Mostafizi, A., Wang, H., Cox, D., Cramer, L., and Dong, S.: Agent-based tsunami evacuation modeling of unplanned network disruptions for evidence-driven resource allocation and retrofitting strategies, Nat. Hazards, 88, 1347–1372, https://doi.org/10.1007/s11069-017-2927-y, 2017.
Mostafizi, A., Wang, H., Cox, D., and Dong, S.: An agent-based vertical evacuation model for a near-field tsunami: Choice behavior, logical shelter locations, and life safety, Int. J. Disaster Risk Re., 34, 467–479, https://doi.org/10.1016/j.ijdrr.2018.12.018, 2019.
Moulds, S., Buytaert, W., Templeton, M. R., and Kanu, I.: Modeling the Impacts of Urban Flood Risk Management on Social Inequality, Water Resour. Res., 57, e2020WR029024, https://doi.org/10.1029/2020WR029024, 2021.
Muhammad, A., De Risi, R., De Luca, F., Mori, N., Yasuda, T., and Goda, K.: Are current tsunami evacuation approaches safe enough?, Stoch. Environ. Res. Risk Assess., 35, 759–779, https://doi.org/10.1007/s00477-021-02000-5, 2021.
Murchland, J. D.: Braess's paradox of traffic flow, Transp. Res., 4, 391–394, https://doi.org/10.1016/0041-1647(70)90196-6, 1970.
Murray-Tuite, P. and Wolshon, B.: Evacuation transportation modeling: An overview of research, development, and practice, Transp. Res. Part C, 27, 25–45, https://doi.org/10.1016/j.trc.2012.11.005, 2013.
Murray-Rust, D., Robinson, D. T., Guillem, E., Karali, E., and Rounsevell, M.: An open framework for agent based modelling of agricultural land use change, Environ. Modell. Softw., 61, 19–38, https://doi.org/10.1016/j.envsoft.2014.06.027, 2014.
Nakanishi, H., Black, J., and Suenaga, Y.: Investigating the flood evacuation behaviour of older people: A case study of a rural town in Japan, Res. Transp. Bus. Manag., 30, 100376, https://doi.org/10.1016/j.rtbm.2019.100376, 2019.
Nappi, M. M. L. and Souza, J. C.: Disaster management: hierarchical structuring criteria for selection and location of temporary shelters, Nat. Hazards, 75, 2421–2436, https://doi.org/10.1007/s11069-014-1437-4, 2015.
Nester, T., Komma, J., Viglione, A., and Blöschl, G.: Flood forecast errors and ensemble spread-A case study, Water Resour. Res., 48, 1–19, https://doi.org/10.1029/2011WR011649, 2012.
Nigussie, T. A. and Altunkaynak, A.: Modeling the effect of urbanization on flood risk in Ayamama Watershed, Istanbul, Turkey, using the MIKE 21 FM model, Nat. Hazards, 99, 1031–1047, https://doi.org/10.1007/s11069-019-03794-y, 2019.
Oh, W. S., Yu, D. J., and Muneepeerakul, R.: Efficiency-fairness trade-offs in evacuation management of urban floods: The effects of the shelter capacity and zone prioritization, PLoS One, 16, e0253395, https://doi.org/10.1371/journal.pone.0253395, 2021.
Palen, L., Starbird, K., Vieweg, S., and Hughes, A.: Twitter-based information distribution during the 2009 Red River Valley flood threat, Bull. Am. Soc. Inf. Sci. Technol., 36, 13–17, https://doi.org/10.1002/bult.2010.1720360505, 2010.
Pande, S. and Sivapalan, M.: Progress in socio-hydrology: a meta-analysis of challenges and opportunities, WIREs Water, 4, e1193, https://doi.org/10.1002/wat2.1193, 2017.
Papaioannou, G., Loukas, A., Vasiliades, L., and Aronica, G. T.: Flood inundation mapping sensitivity to riverine spatial resolution and modelling approach, Nat. Hazards, 83, S117–S132, https://doi.org/10.1007/s11069-016-2382-1, 2016.
Pas, E. I. and Principio, S. L.: Braess' paradox: Some new insights, Transport Res. B-Meth., 31, 265–276, https://doi.org/10.1016/S0191-2615(96)00024-0, 1997.
Paul, B. K.: Factors Affecting Evacuation Behavior: The Case of 2007 Cyclone Sidr, Bangladesh, Prof. Geogr., 64, 401–414, https://doi.org/10.1080/00330124.2011.609780, 2012.
Rahman, A., Hokugo, A., Ohtsu, N., and Chakma, S.: Evacuation Preparation Scenarios of Households during Early and Emergency Evacuation: A Case Study of Cyclone Bulbul in Southwestern Coastal Bangladesh, J. Integr. Disaster Risk Manag., 11, 108–137, https://doi.org/10.5595/001c.29128, 2021.
Saadi, I., Mustafa, A., Teller, J., and Cools, M.: Investigating the impact of river floods on travel demand based on an agent-based modeling approach: The case of Liège, Belgium, Transp. Policy, 67, 102–110, https://doi.org/10.1016/j.tranpol.2017.09.009, 2018.
Shahabi, K. and Wilson, J. P.: CASPER: Intelligent capacity-aware evacuation routing, Comput. Environ. Urban, 46, 12–24, https://doi.org/10.1016/j.compenvurbsys.2014.03.004, 2014.
Shi, H., Du, E., Liu, S., and Chau, K.: Advances in Flood Early Warning: Ensemble Forecast, Information Dissemination and Decision-Support Systems, Hydrology, 7, 56, https://doi.org/10.3390/hydrology7030056, 2020.
Simonovic, S. P. and Ahmad, S.: Computer-based model for flood evacuation emergency planning, Nat. Hazards, 34, 25–51, https://doi.org/10.1007/s11069-004-0785-x, 2005.
Sivapalan, M., Savenije, H., and Blöschl, G.: Socio-hydrology: A new science of people and water, Hydrol. Process., 26, 1270–1276, https://doi.org/10.1002/hyp.8426, 2012.
Smith, A. B. and Matthews, J. L.: Quantifying uncertainty and variable sensitivity within the US billion-dollar weather and climate disaster cost estimates, Nat. Hazards, 77, 1829–1851, https://doi.org/10.1007/s11069-015-1678-x, 2015.
Su, H., Wang, W., Jia, Y., Han, S. C., Gao, H., Niu, C., and Ni, G.: Impact of urbanization on precipitation and temperature over a lake-marsh wetland: A case study in Xiong'an New Area, China, Agr. Water Manage., 243, 106503, https://doi.org/10.1016/j.agwat.2020.106503, 2021.
Sun, B. and Yang, X.: Simulation of water resources carrying capacity in Xiong'an New Area based on system dynamics model, Water, 11, 1085, https://doi.org/10.3390/w11051085, 2019.
Sun, J., Chow, A. C. H., and Madanat, S. M.: Multimodal transportation system protection against sea level rise, Transp. Res. Part D, 88, 102568, https://doi.org/10.1016/j.trd.2020.102568, 2020.
Sung, K., Jeong, H., Sangwan, N., and Yu, D. J.: Effects of Flood Control Strategies on Flood Resilience Under Sociohydrological Disturbances, Water Resour. Res., 54, 2661–2680, https://doi.org/10.1002/2017WR021440, 2018.
Takabatake, T., Fujisawa, K., Esteban, M., and Shibayama, T.: Simulated effectiveness of a car evacuation from a tsunami, Int. J. Disaster Risk Re., 47, 101532, https://doi.org/10.1016/j.ijdrr.2020.101532, 2020.
Tanoue, M., Hirabayashi, Y., and Ikeuchi, H.: Global-scale river flood vulnerability in the last 50 years, Sci. Rep., 6, 36021, https://doi.org/10.1038/srep36021, 2016.
Tellman, B., Sullivan, J. A., Kuhn, C., Kettner, A. J., Doyle, C. S., Brakenridge, G. R., Erickson, T. A., and Slayback, D. A.: Satellite imaging reveals increased proportion of population exposed to floods, Nature, 596, 80–86, https://doi.org/10.1038/s41586-021-03695-w, 2021.
Teng, J., Jakeman, A., Vaze, J., Croke, B., Dutta, D., and Kim, S.: Flood inundation modelling: A review of methods, recent advances and uncertainty analysis, Environ. Modell. Softw., 90, 201–216, https://doi.org/10.1016/j.envsoft.2017.01.006, 2017.
Troy, T. J., Konar, M., Srinivasan, V., and Thompson, S.: Moving sociohydrology forward: a synthesis across studies, Hydrol. Earth Syst. Sci., 19, 3667–3679, https://doi.org/10.5194/hess-19-3667-2015, 2015.
Urata, J. and Pel, A. J.: People's Risk Recognition Preceding Evacuation and Its Role in Demand Modeling and Planning, Risk Anal., 38, 889–905, https://doi.org/10.1111/risa.12931, 2018.
Verkade, J. S. and Werner, M. G. F.: Estimating the benefits of single value and probability forecasting for flood warning, Hydrol. Earth Syst. Sci., 15, 3751–3765, https://doi.org/10.5194/hess-15-3751-2011, 2011.
Viglione, A., Di Baldassarre, G., Brandimarte, L., Kuil, L., Carr, G., Salinas, J. L., Scolobig, A., and Blöschl, G.: Insights from socio-hydrology modelling on dealing with flood risk – Roles of collective memory, risk-taking attitude and trust, J. Hydrol., 518, 71–82, https://doi.org/10.1016/j.jhydrol.2014.01.018, 2014.
Wang, H., Mostafizi, A., Cramer, L. A., Cox, D., and Park, H.: An agent-based model of a multimodal near-field tsunami evacuation: Decision-making and life safety, Transport Res. C-Emerg., 64, 86–100, https://doi.org/10.1016/j.trc.2015.11.010, 2016.
Wang, W., Yang, S., Stanley, H. E., and Gao, J.: Local floods induce large-scale abrupt failures of road networks, Nat. Commun., 10, 2114, https://doi.org/10.1038/s41467-019-10063-w, 2019.
Wang, Y., Song, L., Han, Z., Liao, Y., Xu, H., Zhai, J., and Zhu, R.: Climate-related risks in the construction of Xiongan New Area, China, Theor. Appl. Climatol., 141, 1301–1311, https://doi.org/10.1007/s00704-020-03277-2, 2020.
Wang, Z. and Jia, G.: A novel agent-based model for tsunami evacuation simulation and risk assessment, Nat. Hazards, 105, 2045–2071, https://doi.org/10.1007/s11069-020-04389-8, 2021.
Wedawatta, G. and Ingirige, B.: Resilience and adaptation of small and medium-sized enterprises to flood risk, Disaster Prev. Manag., 21, 474–488, https://doi.org/10.1108/09653561211256170, 2012.
Witkowski, K.: Man's impact on the transformation of channel patterns (the Skawa River, southern Poland), River Res. Appl., 37, 150–162, https://doi.org/10.1002/rra.3702, 2021.
Wood, N., Henry, K., and Peters, J.: Influence of demand and capacity in transportation simulations of short-notice, distant-tsunami evacuations, Transp. Res. Interdiscip. Perspect., 7, 100211, https://doi.org/10.1016/j.trip.2020.100211, 2020.
Wu, F., Guo, N., Kumar, P., and Niu, L.: Scenario-based extreme flood risk analysis of Xiong'an New Area in northern China, J. Flood Risk Manag., 14, e12707, https://doi.org/10.1111/jfr3.12707, 2021.
Yu, D. J., Haeffner, M., Jeong, H., Pande, S., Dame, J., Di Baldassarre, G., Garcia-Santos, G., Hermans, L., Muneepeerakul, R., Nardi, F., Sanderson, M. R., Tian, F., Wei, Y., Wessels, J., and Sivapalan, M.: On capturing human agency and methodological interdisciplinarity in socio-hydrology research, Hydrolog. Sci. J., 67, 1905–1916, https://doi.org/10.1080/02626667.2022.2114836, 2022.
Zhu, J., Ma, Z., Yan, Z., Yuan, X., and Fu, C.: Problems Faced by Construction of Xiongan New Area under Climate Change, Bull. Chinese Acad. Sci., 32, 1231–1236, https://doi.org/10.16418/j.issn.1000-3045.2017.11.008, 2017.
Zhu, Y., Xie, K., Ozbay, K., and Yang, H.: Hurricane Evacuation Modeling Using Behavior Models and Scenario-Driven Agent-based Simulations, Procedia Comput. Sci., 130, 836–843, https://doi.org/10.1016/j.procs.2018.04.074, 2018.
Zhuge, C., Bithell, M., Shao, C., Li, X., and Gao, J.: An improvement in MATSim computing time for large-scale travel behaviour microsimulation, Transportation, 48, 193–214, https://doi.org/10.1007/s11116-019-10048-0, 2021.
Zhuo, L. and Han, D.: Agent-based modelling and flood risk management: A compendious literature review, J. Hydrol., 591, 125600, https://doi.org/10.1016/j.jhydrol.2020.125600, 2020.