the Creative Commons Attribution 4.0 License.
the Creative Commons Attribution 4.0 License.
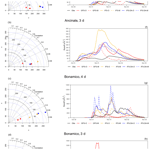
Impact of high-resolution sea surface temperature representation on the forecast of small Mediterranean catchments' hydrological responses to heavy precipitation
Alfonso Senatore
Luca Furnari
Giuseppe Mendicino
Operational meteo-hydrological forecasting chains are affected by many sources of uncertainty. In coastal areas characterized by complex topography, with several medium-to-small size catchments, quantitative precipitation forecast becomes even more challenging due to the interaction of intense air–sea exchanges with coastal orography. For such areas, which are quite common in the Mediterranean Basin, improved representation of sea surface temperature (SST) space–time patterns can be particularly important. The paper focuses on the relative impact of different resolutions of SST representation on regional operational forecasting chains (up to river discharge estimates) over coastal Mediterranean catchments, with respect to two other fundamental options while setting up the system, i.e. the choice of the forcing general circulation model (GCM) and the possible use of a three-dimensional variational assimilation (3D-Var) scheme. Two different kinds of severe hydro-meteorological events that affected the Calabria region (southern Italy) in 2015 are analysed using the WRF-Hydro atmosphere–hydrology modelling system in its uncoupled version. Both of the events are modelled using the 0.25∘ resolution global forecasting system (GFS) and the 16 km resolution integrated forecasting system (IFS) initial and lateral atmospheric boundary conditions, which are from the European Centre for Medium-Range Weather Forecasts (ECMWF), applying the WRF mesoscale model for the dynamical downscaling. For the IFS-driven forecasts, the effects of the 3D-Var scheme are also analysed. Finally, native initial and lower boundary SST data are replaced with data from the Medspiration project by Institut Français de Recherche pour L'Exploitation de la Mer (IFREMER)/Centre European Remote Sensing d'Archivage et de Traitement (CERSAT), which have a 24 h time resolution and a 2.2 km spatial resolution. Precipitation estimates are compared with both ground-based and radar data, as well as discharge estimates with stream gauging stations' data. Overall, the experiments highlight that the added value of high-resolution SST representation can be hidden by other more relevant sources of uncertainty, especially the choice of the general circulation model providing the boundary conditions. Nevertheless, in most cases, high-resolution SST fields show a non-negligible impact on the simulation of the atmospheric boundary layer processes, modifying flow dynamics and/or the amount of precipitated water; thus, this emphasizes the fact that uncertainty in SST representation should be duly taken into account in operational forecasting in coastal areas.
- Article
(17094 KB) -
Supplement
(2889 KB) - BibTeX
- EndNote
Operational river flood forecasting is a highly challenging activity for several reasons that go beyond strictly scientific aspects. Hydrometeorological forecasting requires extremely complex systems, where issues like communication of warning, accessibility of the results, and administrative and/or institutional factors can be as important as monitoring and modelling activities (Pagano et al., 2014; Silvestro et al., 2017). Nevertheless, the cornerstone of such systems, and undoubtedly the most demanding part from a scientific point of view, is still the meteorological–hydrological modelling chain, supported by in situ or remotely sensed measurements.
Increasingly refined modelling chains have been developed in recent years (e.g. UK Environmental Prediction research, Lewis et al., 2019a; Canadian Great Lakes, Gronewold et al., 2011; the US Navy's Coupled Ocean/Atmosphere Mesoscale Prediction System COAMPS®, Hodur, 1997). Despite their complexity, these systems all have to deal with some inherent limitations of the meteorological and hydrological models. The main sources of errors in weather forecasts are connected to both inaccuracy in defining the initial state, due to the lack of available measures or observation/assimilation errors, and approximations of the models, whose structures are not capable of properly representing the phenomena of interest (Allen et al., 2002; Buizza, 2018). These problems are exacerbated by the chaotic nature of the atmosphere. Even though hydrological models are much simpler than meteorological models with respect to their structure (Liu et al., 2012; Pagano et al., 2014), they also have to struggle with different sources of uncertainty that, according to Renard et al. (2010), can be grouped into four categories: (1) input uncertainty, (2) output uncertainty (e.g. runoff estimates are not straightforward), (3) structural model uncertainty, and (4) parametric uncertainty. Furthermore, as catchments are very seldom perfect natural systems, some effects of human disturbances can virtually not be modelled.
The main link between atmospheric and hydrological compartments in a forecasting chain is precipitation forecast, which is an output variable for weather models and constitutes the main input for hydrological models. Quantitative precipitation forecast (QPF) is a major challenge for operational meteorology, because the reliability of precipitation forecasts crucially affects streamflow forecasts' skill (for a review see Cuo et al., 2011; for recent applications see e.g. Davolio et al., 2015, 2017; Tao et al., 2016; Li et al., 2017). Among the various strategies adopted for addressing this issue, in recent years, several studies that were focused on coastal areas have assessed the importance of sea surface temperature (SST) initial and boundary conditions as relevant drivers of QPF, which, as previously stated, is consequently capable of influencing the streamflow forecast. This impact can be particularly strong in topographically complex coastal areas, characterized by several small catchments, such as in the Mediterranean Basin, for which several cooperative research efforts have been activated, including the MEDiterranean EXperiment (MEDEX; Jansa et al., 2014) and the HYdrological cycle in the Mediterranean eXperiment (HyMeX; Drobinski et al., 2018).
Several studies have recently focused on the effects of sea surface–atmosphere interactions over heavy precipitation at midlatitudes, particularly in the Mediterranean area (e.g. Manzato et al., 2015; Romaniello et al., 2015; Rainaud et al., 2016). Some of them showed that large variations in the average values of SST boundary conditions significantly affected the location and intensity of high-impact events (Lebeaupin et al., 2006; Miglietta et al., 2011; Senatore et al., 2014; Meredith et al., 2015; Pastor et al., 2015; Miglietta et al., 2017; Pytharoulis, 2018). Furthermore, using coupled atmosphere–ocean simulations, Berthou et al. (2014, 2015) highlighted the major effects of long-term SST changes in the representation of Mediterranean intense rain events, although features at shorter timescales can also contribute significantly. Lebeaupin et al. (2006) found that higher-resolution SST fields have poor effects on convection in the case study they analysed (southern France). Ivatek-Šahdan et al. (2018), examining several events in the eastern Adriatic, also found that more realistic SST fields did not substantially improve precipitation estimates; furthermore, they showed that the impact of high-resolution SST varied in different cases. Conversely, Katsafados et al. (2011) found noticeable deviations among the forecast skills of simulations with SST boundary conditions at different resolutions in a test case in the eastern Mediterranean, whereas Cassola et al. (2016) verified that high-resolution SST fields can positively impact QPF in the forecasting range of 36–48 h in a study in north-western Italy. Finally, Berthou et al. (2016), in southern France, and Stocchi and Davolio (2017), in the Adriatic Sea, highlighted that SST–atmosphere interactions mainly affect precipitation patterns and intensity via complex (and varying event-by-event) modifications of the stability of the upstream atmospheric boundary layer.
The main objective of this paper is to contribute to the current discussion on the impact of SST representation by extending the analysis over the whole meteo-hydrological forecasting chain, i.e. going beyond precipitation forecasts and evaluating sensitivity on streamflow forecasts. Furthermore, SST sensitivity is assessed in the context of the overall uncertainty linked to initial and boundary conditions in regional modelling, using different forcing GCMs, with and without data assimilation. To this aim, different accuracy levels of SST representation are used in an operational meteorological–hydrological forecasting chain over a coastal Mediterranean area including, in addition to the native SST fields of the general circulation models (GCMs), higher-resolution fields: the Medspiration level 4 ultra-high-resolution foundation SST “SSTfnd” from the Medspiration project by the Centre European Remote Sensing d'Archivage et de Traitement (CERSAT) and the Institut Français de Recherche pour L'Exploitation de la Mer (IFREMER; Merchant et al., 2008; Robinson et al., 2012). Furthermore, two GCM forecasts are used, namely the global forecasting system (GFS) provided by the US National Weather Service (NWS) and the integrated forecasting system (IFS) developed at the European Centre for Medium-Range Weather Forecasts (ECMWF), as well as a three-dimensional variational assimilation (3D-Var) scheme.
The study area, corresponding to the Calabrian Peninsula (southern Italy), due to its particular position in the middle of the Mediterranean Sea and its complex and steep orography, quite regularly experiences severe precipitation events and is particularly prone to significant ground effects (Federico et al., 2003a, b, 2008; Chiaravalloti and Gabriele, 2009; Llasat et al., 2013; Gascòn et al., 2016; Avolio and Federico, 2018), the most recent of these (at the time of publication) being a flash flood event on 20 August 2018 that caused 10 casualties (Avolio et al., 2019). According to Avolio and Federico (2018), severe precipitation events over Calabria can be classified as either short-lived events, which last less than 24 h, or long-lived events, which last more than 24 h. Following this classification, in this paper, two case studies of events that occurred in 2015 are considered, the former is characterized by convective, very localized precipitation (11–12 August; CFM, 2015a) and the latter by more persistent and widespread stratiform precipitation (30 October–2 November; CFM, 2015b).
The meteorological–hydrological forecasting chain is based on the WRF-Hydro modelling system (Gochis et al., 2015). This open-source community model, originally developed as the hydrological extension of the Weather Research and Forecast (WRF; Skamarock et al., 2008) model, provides a coupling architecture that allows the user to connect vertical water fluxes between the Earth surface and the atmosphere, which are simulated at coarse resolution by the atmospheric model, to lateral surface and sub-surface fluxes, simulated at high-resolution by the hydrological model, in both a one-way (i.e. with no feedback from the routing models to the atmosphere) and two-way (with feedback) manner. The WRF-Hydro system has dramatically evolved in recent years (Salas et al., 2018; Lin et al., 2018; Lahmers et al., 2019), and has been operationally adopted into the NOAA National Water Model (NWM, Cohen et al., 2018) across the continental US, as well as being used for research applications (e.g. Yucel et al., 2015; Senatore et al., 2015; Arnault et al., 2016; Verri et al., 2017).
The paper is organized as follows. Section 2 describes the study area; the two events analysed; and the numerical model, including its set-up and details on the space and time resolutions of the boundary conditions. In Sect. 3 the results of the meteorological and hydrological outputs are analysed separately for the two events. Finally, Sect. 4 discusses and summarizes the main findings and outlines future research lines.
2.1 Study area and description of the events
Calabria is a peninsula characterized by a complex orography. Its geographical and morphological features produce a very irregular precipitation distribution (average annual precipitation varies between 600 and 1500 mm; Federico et al., 2010) and foster the occurrence of extreme weather events, which often caused deaths (Petrucci et al., 2018). Among the relatively numerous recent events, this study focuses on two case studies that occurred in 2015 and were characterized by distinctive features.
The first high-impact event (case study 1) was very localized in space and time and hit the north-eastern part of the region on the morning of 12 August 2015. The analysis at the synoptic scale (Fig. 1a, b) shows that a main low-pressure system which originated from the Atlantic moved over the French and Spanish coasts in the early hours of 12 August 2015, while a cut-off low occurred over the central Mediterranean, giving rise to a new low-pressure vortex with reduced dimensions that caused intense local rainfall. The observed precipitation patterns (Fig. 1c) involved only small areas of the mainland, specifically the Corigliano and Rossano municipalities. The data provided by the Italian national radar network (integrated into the same map in Fig. 1c), although underestimating ground observations, show that most of the precipitation occurred over the Ionian Sea. The Corigliano rain gauge measured high rainfall values (Fig. 1d). During the 48 h from 00:00 UTC on 11 August 2015 until 00:00 UTC on 13 August 2015, 255.2 mm of rain was recorded, with a maximum of 246.4 mm in 24 h (from 18:00 UTC on 11 August to 18:00 UTC on 12 August), 223.2 mm in 12 h (from 01:45 UTC on 12 August to 13:45 UTC on 12 August), 167.4 mm in 6 h, 107.2 mm in 3 h, and 51.4 mm in 1 h. The hydrological impact concerned some small/very small coastal catchments, the most important of which was the Citrea Creek (11.4 km2 – catchment boundaries highlighted in Fig. 1e), which overflowed causing several tens of millions of euros in damage.
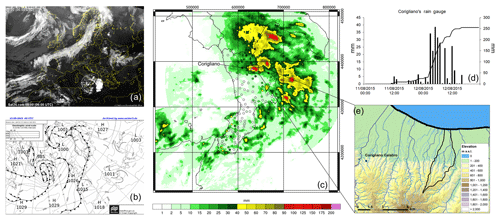
Figure 1(a) Satellite images of the thermal infrared channel (10.8 µm) at 06:00 UTC on 12 August 2015 from http://www.sat24.com (last access: 5 December 2019), © EUMETSAT. (b) Surface pressure and weather fronts at 06:00 UTC on 12 August 2015 from http://www1.wetter3.de/ (last access: 5 December 2019), © Met Office. (c) Cumulative rain (mm) observed between 18:00 UTC 11 on August 2015 and 18:00 UTC on 12 August 2015; the points represent the weather stations, whereas spatially distributed values represent the radar estimation. (d) Cumulative and hourly rainfall (mm) observed at the Corigliano rain gauge. (e) The Citrea Creek catchment, showing the location of the Corigliano rain gauge.
The second event (case study 2) involved a much larger area and developed over 4 d, from 30 October to 2 November 2015. The synoptic analysis (Fig. 2e) shows another cut-off low remaining stationary over Sicily for much of the period and attracting humid and warm air from the Ionian Sea to the south-east (a detailed synoptic description of the event is provided by Avolio and Federico, 2018). The orographic effect in this event turned out to be decisive, with the Calabrian mountain ranges acting as a real barrier; therefore, a large part of the rainfall occurred on the Ionian (eastern) side of the region. While on 30 October 2015 only the northern part of the region was affected (Fig. 2a; about 200 mm in 24 h at the Oriolo station), the highest precipitation during the entire event was recorded on the southern coast (Fig. 2b, c, d), with a maximum of about 740 mm (Chiaravalle Centrale station) and a daily maximum of about 370 mm (Sant'Agata del Bianco). In Fig. 2a–d, rain gauge observations overlap the precipitation fields detected by the weather radars, also extending over the sea. The hydrological impact of the event concerned the whole eastern side of the region. Two catchments are selected for this study, namely the Ancinale River that is closed at the Razzona gauging station (116 km2, Fig. 2f) and the Bonamico Creek that is closed at the Casignana gauging station (138 km2, Fig. 2g). These catchments are chosen because they are two of the biggest with available water level observations (unfortunately no discharge data are available), and they are located to the north and south of the rainiest area, respectively. Specifically, Chiaravalle Centrale station is located at the Ancinale River outlet.
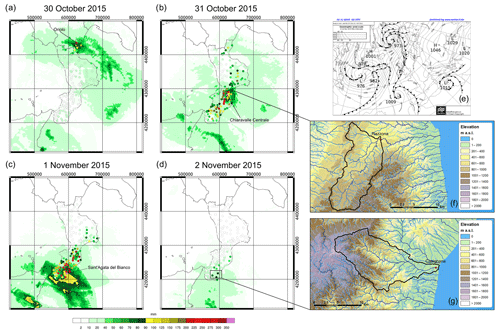
Figure 2Panels (a) to (d) represent the respective daily rainfall (mm) amounts observed from 30 October to 2 November 2015; the points in these panels represent the weather stations, and the spatially distributed values represent the radar estimation. (e) Surface pressure and weather fronts at 00:00 UTC on 1 November 2015 from http://www1.wetter3.de/, © Met Office. (f) Elevation maps of the Ancinale River catchment and (g) the Bonamico Creek catchment.
2.2 Numerical model description and set-up
2.2.1 WRF
The Advanced Research WRF (ARW) model, version 3.7.1, is used in two one-way nested domains (Fig. 3). The external domain, D01, covers a large area of the Mediterranean (33.04–49.85∘ N, 3.59–28.59∘ E) with a 10 km (187×205 grid points) horizontal resolution, whereas the innermost domain, D02, is centred over the Calabrian Peninsula (37.10–40.87∘ N, 13.88–18.71∘ E), with a 2 km (200×200 grid points) horizontal resolution. The model runs on 44 vertical atmospheric layers, up to a 50 hPa pressure top (about 20 000 m), and on 4 soil layers, down to 2 m below the surface. The time step of the model simulation is 60 s in D01 and 12 s in D02.
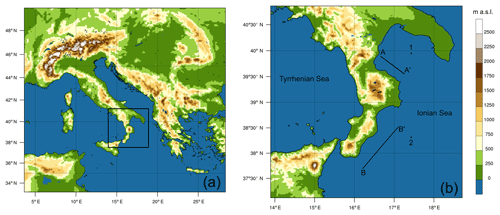
Figure 3(a) The outer domain (D01) with a spatial resolution of 10 km; (b) the inner domain (D02) with a spatial resolution of 2 km. Points 1 and 2 are considered when evaluating SSTSK (skin SST) evolution locally during the events according to different configurations (Figs. 5 and 10 respectively). Vertically integrated water vapour fluxes are calculated across A–A' and B–B' (Figs. 9 and 13 respectively).
Physical parameterization of the model is the same as that used by Senatore et al. (2014) and is reported in Table 1. Boundary and initial conditions are provided by two operational forecast GCMs, namely the GFS in forecast mode with a spatial resolution of 0.25∘ (about 27 km) and the high-resolution (HRES) IFS-ECMWF in forecast mode with a spatial resolution of about 16 km. In both cases, boundary conditions are provided every 6 h. As a further step, both initial and lower boundary SST data are replaced by the Medspiration L4 ultra-high-resolution SSTfnd (obtained as a daily mean with a resolution of 0.022∘). The high-resolution Medspiration SST fields are ingested into the WRF initial and lower boundary condition files of both domains via GIS-based techniques, following Senatore et al. (2014).
Furthermore, two relevant options allowed by the WRF modelling system are always activated for all SST boundary conditions: the sst_update option, allowing dynamical lower boundary (i.e. SST) conditions, and the sst_skin option, based on Zeng and Beljaars (2005), which permits the simulation of SST dynamics. It is noteworthy that the higher resolution of the SST fields does not imply a greater accuracy, which can be objectively assessed via a comparison with in situ observations. For this purpose, a preliminary search is performed in the Copernicus Marine Environment Monitoring Service (CMEMS), in particular in the Coriolis Ocean database for ReAnalysis (CORA; Cabanes et al., 2013), using the latest version (5.2, April 2019). Useful data (i.e. continuous measurements with a sub-daily time step at the sea–surface interface) are only found at the border of the external domain (D01) for both of the case studies (Fig. S1a in the Supplement).
Finally, both as an additional comparison and with the aim of highlighting its relative impact with respect to the effects of different boundary conditions provided by different GCMs and/or more detailed SST fields, a data assimilation technique is also used for both of the test cases. Specifically, a 3D-Var assimilation scheme (Barker et al., 2004; Huang et al., 2009; Barker et al., 2012) is adopted, introducing conventional meteorological observations into the initial conditions and adjusting boundary conditions to improve simulation performance.
A summary of all of the simulations carried out is reported in Table 2.
2.2.2 WRF-Hydro
In this work, WRF-Hydro version 3.0 is used in one-way mode. Therefore, the atmospheric model outputs are used as input of the hydrological model utilizing an hourly time step. According to the WRF parameterization, the land surface model (LSM) is unified NOAH and is used at the same resolution as the D02 domain, whereas an increased horizontal resolution of 200 m is used (2000×2000 grid points) for the lateral routing of surface and subsurface water; this results in an aggregation factor of 1∕10 from the atmospheric to the hydrological model.
No observed discharge or flow depth data are available for case study 1; therefore, model calibration is not performed. In case study 2, model calibration is performed manually with respect to the available water level data for the two selected catchments (Ancinale and Bonamico) with the aim of reproducing the timing of the hydrological responses to heavy precipitation and, primarily, to correctly simulate the peak flow time, which is a paramount variable for civil protection activities.
The humidity and temperature conditions in the four soil layers at the beginning of the analysed event (30 October 2015 at 00:00 UTC) are achieved using offline simulations with a spin-up time of 1 month. The meteorological forcing for this period is basically given by the spatial interpolation of ground-based observations (provided by the monitoring network managed by the Centro Funzionale Multirischi – ARPACAL, Calabria region). The interpolation techniques adopted are the same as those described in Senatore et al. (2015) except that precipitation fields are interpolated via inverse distance weighting (IDW) instead of exponential kriging. Furthermore, during the event (i.e. from 30 October to 2 November 2015) precipitation fields (Fig. 4a, b) are only achieved by merging hourly ground-based rainfall observations to hourly radar data estimates provided by the Italian weather radar network managed by the National Department of Civil Protection. The merging procedure follows Sinclair and Pegram (2005) with the difference that a simpler double IDW interpolation method is used instead of a double kriging interpolation. The merging technique guarantees an increase in the total “observed” rainfall volume of +4.6 % over the Ancinale River and +10.6 % over the Bonamico Creek in comparison with a simple IDW interpolation.
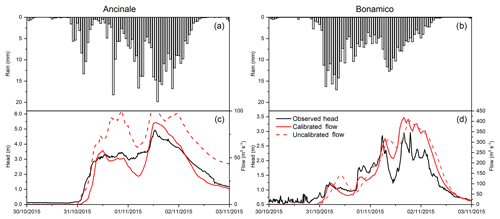
Figure 4(a) Observed hourly rainfall (mm) averaged over the Ancinale River catchment; (b) as in panel (a), but for the Bonamico Creek catchment. (c) A comparison between observed hydrometric levels (m) with respect to uncalibrated and calibrated simulated flow (m3 s−1) over the Ancinale River catchment; (d) as in panel (c), but for the Bonamico Creek catchment.
The parameters involved in the calibration procedure are broadly the same as those used in previous studies with WRF-Hydro (e.g. Yucel et al., 2015; Senatore et al., 2015). Specifically, the LSM parameters calibrated are the infiltration factor (REFKDT), the coefficient governing deep drainage (SLOPE), and the thicknesses of the four soil layers. In addition, two spatially distributed parameters of the hydrological model, namely the overland flow roughness scaling factor (OVROUGHRTFAC) and the initial retention height scaling factor (RETDEPRTFAC), are calibrated along with the Manning roughness coefficients (one value for each stream order).
The calibrated parameters are shown in Table 3, whereas resulting hydrographs and uncalibrated hydrographs are shown in Fig. 4c and d. The more impulsive behaviour of the Bonamico Creek, typical of Calabrian “fiumare”, is simulated using lower values of the infiltration factor and lower soil layer thicknesses. Nevertheless, in order to allow timely simulation of peak flows, a small delay of the initial response is necessary via an increase in the RETDEPRTFAC value, which is compatible with noteworthy initial ponding in the wide alluvial bed and infiltration in the gravelly soil. Conversely, the abundance of organic matter in the soils of the dense forests within the Ancinale River catchment, which (especially in autumn) can store considerable quantities of water, most probably contributes substantially to the smoother response of the Ancinale River. Figure 4c and d highlight that the calibration procedure mainly influences the results for the Ancinale River, especially in terms of total volumes.
Table 3Calibrated parameters of the offline WRF-Hydro model for the Ancinale River and the Bonamico Creek.
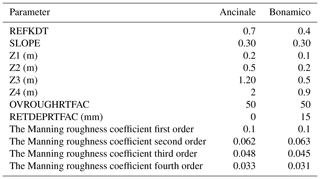
As for the hydrographs, adopting typical stage–discharge power relationships (i.e. , where q is the discharge, h is the water level, and a and b are the two calibration coefficients) the coefficients of determination (R2) between simulated discharge values and observed water levels are equal to 0.942 and 0.831 for the Ancinale River and the Bonamico Creek respectively. Concerning the reliability of the simulated discharge amount, as reference observations are missing, an indirect validation of the peak flows achieved is performed using the Hydrologic Engineering Center's (CEIWR-HEC) River Analysis System (HEC-RAS) (Hydrologic Engineering Center, 2016). Cross-sections for both of the outlets of the catchments and for four upstream and downstream points, approximately 50 m apart, are determined by merging data from an ultra-high-resolution (5 m) digital terrain model provided by the Calabria Region Cartographic Centre with the heights given in very recent official maps (Technical Cartography of Calabria Region) at a scale of 1:5000. Such cross-sections are further validated by field sample measurements. One-dimensional steady flow simulations reaching observed peak heights provide peak discharges broadly comparable to the results achieved with the model.
For the sake of brevity, hereafter the WRF-Hydro hydrographs calibrated using observed precipitation fields (shown in Fig. 4) will be referred to as “observed hydrographs” or simply “observations”.
3.1 Case study 1
The analysis with the CORA database (Fig. S1b–d) shows that Medspiration-derived lower boundary conditions, although originating from a high-resolution dataset, do not provide a clear improvement of skin SST representation compared to the original GCM fields. It is to be noticed, however, that the SST boundary conditions in D01 are aggregated at a 10 km resolution. Panels in Fig. S2 focus on D02 and show the skin SST fields from 11 August at 18:00 UTC to 12 August at 18:00 UTC with a time step of 6 h, for all simulations carried out in this case study. The main features highlighted by the skin SST maps are the strong underestimation of native IFS fields close to the coastline (this is due to a known interpolation problem along coastlines that lowers temperatures to unrealistic values; Linus Magnusson, personal communication, 2019) and the overestimation, especially in the Tyrrhenian Sea (up to more than 2 K), of the native GFS fields. The other skin SST fields mostly differ by less than ±0.5 K from each other. It is noteworthy that skin SST fields in the simulations using the Medspiration product are not identical due to the fact that, with the method of Zeng and Beljaars (2005), skin SST values are influenced by the surface winds and net radiation fluxes modelled by the different simulations.
A comparison of the time evolution of the average skin SST values in the whole D02 domain would be biased by the non-negligible IFS underestimation near the coastline. Instead, an analysis performed on selected significant points could provide more interesting insights. For this reason, focusing on the Ionian Sea, in Fig. 5, point 1, which is closer to Corigliano-Rossano, and point 2, which is off the Calabrian southern coast (the exact location of both points is given in Fig. 3b), are examined. Concerning daily values, a clear but slight (< 0.5 K) overestimation of GFS-O is shown for both days in point 1 and on the second day in point 2. Some hourly differences are more evident: e.g. in point 1 GFS-O values are up to 1.5 K higher than other models on 12 August at around 12:00 UTC, whereas in point 2 peak values of IFS-O and IFS-M on 11 August at around 12:00 UTC are about 1 K higher than other models. Nevertheless, the differences among models during the night between 11 and 12 August (i.e. right before and during the rain event) are generally low. The only noteworthy difference is given (in point 2) by the small underestimation of Medspiration simulations (i.e. GFS-M, IFS-M, and IFS-DA-M) of about 0.3–0.4 K, shown by a sudden reduction of their skin SST values, most probably due to the change of the Medspiration SST field (from 11 to 12 August). This behaviour, which is clearly not realistic, highlights a weakness occurring while directly ingesting such external data in the WRF simulation.
The accumulated precipitation modelled by all simulations for the 24 h period from 11 August at 18:00 UTC to 12 August at 18:00 UTC is shown in Fig. 6. Overall, all models miss the location of the event, moving it further south, off the Ionian coast. GFS-based simulations forecast more rainfall than IFS-based simulations (average respective values in the domain of 10.1 and 8.9 mm with the native SST fields and 10.4 and 9.5 mm with the Medspiration SST fields for GFS and IFS), but are centred more to the south. IFS-based simulations forecast rainfall clusters with more elongated shapes in the south–north direction, allowing more precipitation to reach the central and northern Ionian coasts (namely, the Corigliano-Rossano area). Even though simulations based on the 3D-Var scheme still miss the correct location of the event, they both provide more rainfall in the domain (average values of 11.2 mm with the native SST fields and 11.0 mm with the Medspiration SST fields) and also show a well-defined rainfall cluster close to the central Ionian coast. Both IFS and 3D-Var simulations overestimate land precipitation in that area.
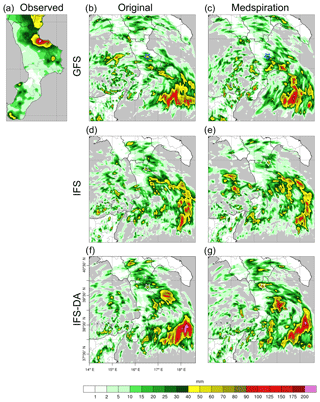
Figure 6The 24 h accumulated precipitation (mm) from 18:00 UTC on 11 August 2015 to 18:00 UTC on 12 August 2015: (a) merged ground measurements and radar observations simulated (b–g) using different configurations. The small blue (b–c) or white (d–g) stars highlight the accumulated rainfall peaks near Corigliano, which are analysed in detail in Fig. 7.
According to the generally small differences identified in the SST fields, Fig. 6 clearly shows that ingesting high-resolution SST information provides (in terms of spatial distribution of accumulated precipitation) much less relevant (and partially chaotic) effects than changing initial and boundary conditions or using data assimilation schemes, and a minor or possibly opposite impact on the accuracy of the simulations. Given the peculiar features of the analysed event, it makes sense to focus on the area surrounding the Corigliano gauge station. For each simulation, the graph in Fig. 7a merges intensity, location, and time correlation information of the closest rainfall peaks (with a threshold of at least 40 mm) to that station, whereas Fig. 7b explicitly shows the time evolution of accumulated rainfall for each of the locations identified (the points are highlighted using small stars in the panels of Fig. 6). Given that all simulations strongly underestimate the observed rainfall value of 246.4 mm (the highest simulated value of about 100 mm is given by IFS-DA-M), there is no configuration clearly outperforming the others. Both GFS peaks are located to the south (about 20 km) and delay the rain event by 8 (GFS-M) to 11 (GFS-O) hours. IFS-O and IFS-DA-O peaks are lower than IFS-M and IFS-DA-M, but they are generally closer to the Corigliano station (about 13 and 22 km respectively). Furthermore, Fig. 7b shows that ingesting Medspiration fields moves the rainfall events up for both IFS-O and IFS-DA-O. This suggests that removing the unrealistic low SST values along the coastline near Corigliano station (i.e. considering IFS-M and IFS-DA-M in place of IFS-O and IFS-DA-O respectively) has the twofold effect of increasing rainfall amounts and accelerating flow dynamics. Such effects are more easily recognizable looking at the 3D-Var simulations, which provide more water vapour and precipitation. Moving from IFS-O to IFS-DA-O to IFS-DA-M, the 850 hPa wind speed on 12 August at 00:00 UTC generally increases in D02 and specifically off the northern Ionian coast of Calabria (Fig. S3). As a result, Fig. 8 shows that when moving from IFS-O to IFS-DA-O to IFS-DA-M, the integrated water vapour (IWV) cluster off the Ionian Sea simulated 3 h later (03:00 UTC) is both larger and closer to the coast.
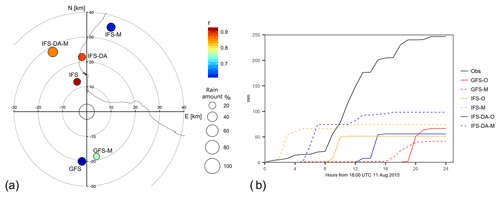
Figure 7Circles in panel (a) are located at the peaks highlighted in Fig. 6 for each of the different configurations; the colours indicate the time correlation, whereas the size refers to the percentage rain amount with respect to Corigliano observations. (b) Temporal accumulated rainfall (mm) observed at Corigliano and simulated by the different peaks.
Differences between IFS-O and IFS-DA-O are due to the assimilation of 14 vertical profiles of pressure, wind speed and direction, absolute and dew point temperature, and relative humidity in D01, with 14 point measurements provided by aircrafts at a fixed pressure level (corresponding to about 12 km). In contrast, differences between IFS-DA-O and IFS-DA-M are mainly due to different skin SST values. Specifically, higher SST values given by ingesting Medspiration fields enhance water vapour concentration in the atmosphere (the average upward moisture flux from the sea surface in domain D01 increases by about 5 %), while they concurrently affect the stability of the atmospheric boundary layer, providing more energy to the system and accelerating the flow dynamics (as reported by e.g. by Stocchi and Davolio, 2017). The early arrival of the moist air mass in the Corigliano-Rossano area using Medspiration SST fields is highlighted by the time series of the hourly averaged water vapour flux through section A–A' (Fig. 9; section A–A' is shown in Fig. 3b). Local flow peaks are moved up from 2 to 4 h in advance using IFS-DA-M compared with IFS-DA-O, and similar behaviour, even though less evident, is observed with IFS-M compared with IFS-O.
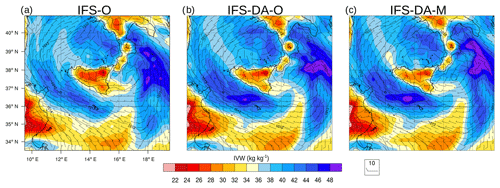
Figure 8Column IWV (integrated water vapour; kg kg−1, colour), sea level pressure (hPa; contours), and wind direction and speed (barbs) at a height of 10 m at 03:00 UTC on 12 August 2015 for (a) IFS-O, (b) IFS-DA-O, and (c) IFS-DA-M respectively.
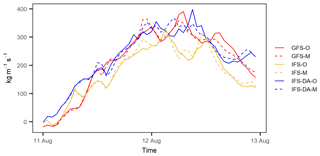
Figure 9Time evolution from 00:00 UTC on 11 August 2015 to 00:00 UTC on 13 August 2015 of the vertically integrated water vapour flux (kg m−1 s−1) crossing section A–A', shown in Fig. 3b.
Concerning the assessment of the hydrological impact of the forecast event, notwithstanding the detailed analysis performed, case study 1 does not provide relevant results (Table 4). The centre of the Citrea catchment is located approximately 8 km south-east of the Corigliano gauge station, has a maximum length of about 7 km in the south–north direction, and a maximum width of only 2.5 km. The level of accuracy achieved by all simulations performed is not yet sufficient to correctly forecast the hydrological impact for such small catchments in areas with very complex topography, such as those areas analysed in this study. The maximum rainfall accumulation value over the catchment is forecast by IFS-O, with 16 mm in 3 h. However, the accuracy of the models is already high enough to make them very useful (it is worthwhile recalling that the starting time of the simulation is more than 24 h before the event). In fact, if model forecasts are used to infer information about wider “warning areas” than single small catchments (as carried out by the Italian Civil Protection system), they provide essential inputs for civil protection activities.
3.2 Case study 2
Case study 2 embraces a longer period than case study 1. In this section, forecasting skills are assessed considering both the whole 4 d length of the event (i.e. from 30 October 2015) and a 3 d forecast starting on 31 October 2015, in order to reduce the uncertainties attributable to the longer lead time forecast.
Such as in the previous case study, the first analysis is devoted to skin SST fields. The comparison with the SST measurements available from the CORA database shows much better behaviour of the Medspiration SST fields in the analysed region of the external domain (Fig. S1e–g). Focusing on the innermost domain, Fig. S4 highlights (besides the above-mentioned IFS-related problem along coastlines) that, in this case, Medspiration fields for the whole period overestimate both GFS and IFS native SST fields. Specifically, average differences with respect to GFS SST vary from about 0.6 to 0.8 K, whereas differences with respect to IFS SST fields are higher than 0.8 K (the average difference increases to about 1.5 K if the values along coastlines are also considered). It is noteworthy that GFS also underestimates skin SST particularly near coastlines, whereas there is an overestimation off the Tyrrhenian Sea, such as that seen in the previous test case. Focusing on points 1 and 2 (Fig. 10), the following is shown: (1) both points replicate similar general behaviour, with Medspiration fields' values being higher than values from GFS, which, in turn, are higher than values from IFS; (2) differences are more marked in point 1 (average values of +1.0 and +0.6 K for IFS and GFS respectively) than in point 2 (+0.9 and +0.3 K for IFS and GFS respectively); (3) as in case study 1, a sudden reduction of about 0.5 K can also be observed for Medspiration in the graph related to point 1, moving from 1 to 2 November (Fig. 10a). Nevertheless, a similar abrupt change, although less marked (about 0.2 K), is observed also for GFS on 31 October at 06:00 UTC. In summary, this case study shows an evident skin SST increase from IFS to GFS to Medspiration.
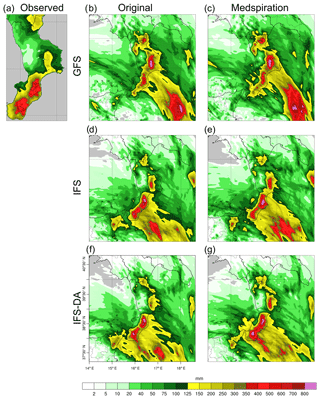
Figure 11Accumulated precipitation (mm) over the whole period of 96 h starting from 00:00 UTC on 30 October 2015: (a) merged ground measurements and radar observations; (b–g) simulated fields with the different configurations.
Figure 11 shows the accumulated rainfall fields in the 4 d simulation period from the six WRF configurations compared with a rainfall map of Calabria achieved by merging ground measurements with radar observations (the merging procedure followed Sinclair and Pegram, 2005; distinct rain gauge and radar data are available in Fig. 2a–d). It clearly highlights, in agreement with the previous case study, that the main impact on rainfall output is given by the choice of the GCM providing the boundary conditions. Average accumulated precipitation in D02 is equal to 80 mm with GFS-O, 71 mm with IFS-O, and 68 mm with IFS-DA-O. Interestingly, the introduction of the 3D-Var scheme this time leads to reduced precipitation (in D01, 22 vertical profiles and 16 point measurements are assimilated). Higher skin SST values with Medspiration result in increased average precipitation in D02 for all three cases, from +8 % (IFS-DA) to +11 % (GFS). Concerning the precipitation patterns, for the aims of this study, it is interesting to focus on the biggest cluster in the south-east corner of the domain (i.e. the direction from which the humid air mass originates). Moving from GFS to IFS to IFS-DA, quite independently of the SST fields' change, a shift of this cluster can be observed from north-east to south-west.
The main change produced by the 3 d forecast compared with the 4 d forecast is the higher correspondence of the GFS-based simulations to the IFS-based simulations (Fig. S5). The GFS-based rainfall footprints located in the south-east of D02 meet the Calabrian Ionian coast further south with respect to the 4 d simulation, which is in agreement with the IFS-based simulations. Overall, the simulated rainfall fields are rather similar to each other and seem to reproduce the observations in the southern part of the region reasonably well (i.e. the area most affected by the event), while the over-forecast found in the 4 d simulation in the central zone is confirmed. 3D-Var forecasts starting on 31 October assimilate 15 vertical profiles and 12 point measurements. Although the IFS-based simulations forecast higher rainfall peaks off the southern Ionian coast (up to 1000 mm), the average accumulated precipitation in D02 is almost identical for all simulations (51 mm with GFS-O, 52 mm with IFS-O, and 53 mm with IFS-DA-O). Precipitation increase caused by the higher skin SST Medspiration fields varies from +9 % (IFS-DA) to +12 % (GFS), which is in agreement with the upward moisture flux increase in D01 (+7 % with GFS, and +8 % with IFS and IFS-DA).
With the aim of objectively assessing the performance of each WRF configuration, a detailed analysis using categorical scores is carried out considering ground-based observations in the civil protection warning areas more affected by the event (grey areas in the reproduction of the Calabria region in Fig. 12). Specifically, 30, 19, and 22 rain gauges are considered for the Cala4, Cala7, and Cala8 zones respectively. Among the numerous scores available in the literature (for a review see e.g. Wilks, 2006), for each zone Fig. 12 shows the results with respect to the frequency bias index (FBI),
and the equitable threat score (ETS),
Here
In the previous equations, the terms hits, misses, false alarms, and correct negatives refer to a typical 2×2 contingency table. The FBI indicates if the forecast system has a tendency to underestimate (FBI < 1) or overestimate (FBI > 1) the event frequency, whereas ETS measures the fraction of correctly predicted events, adjusted for hits associated with random forecasts, and ranges from to 1 (perfect score). Both scores are used for consecutive 6 h time intervals for the analysed rainy period, utilizing precipitation thresholds with a step of 0.2 mm from 0.2 to 1 mm, a step of 1 mm up to 10 mm, a step of 2 mm up to 20 mm, and a step of 5 mm for higher rates.
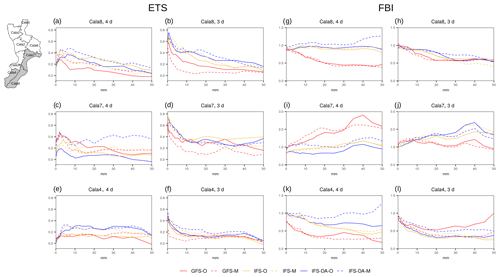
Figure 12Categorical scores ETS (equitable threat score) (a–f) and FBI (frequency bias index) (g–l) calculated on the rain gauges located in the three civil protection warning areas more affected by the event from case study 2 (highlighted as grey areas on the map in the top left) for both the 4 and the 3 d period.
Focusing on the 4 d simulations, ETS graphs show the generally better performance of IFS-DA-M, especially for higher thresholds. Other models have conflicting levels of accuracy: e.g. IFS-DA-O is the best in Cala4, but the worst in Cala7. Nevertheless, ingesting high-resolution SST generally provides better scores in all cases. Complementary information provided by FBI highlights a significant under-forecast of GFS-based simulations in both Cala4 and Cala8 and an over-forecast in Cala7. Other simulations behave better, but FBI also points out that the 3D-Var scheme alone does not necessarily improve IFS-based forecasts (e.g. in Cala7 IFS-O is more accurate than IFS-DA-O), unless a high-resolution SST representation is also considered (IFS-DA-M always shows FBI values around 1). The ETS values of the 3 d simulations are generally higher, but, in this case, the GFS-based simulations are the worst, and introducing the Medspiration fields further reduces their performance. Conversely, a more detailed SST resolution increases the ETS values of the IFS-based simulations in zones Cala4 and Cala8 (but not in zone Cala7). Concerning bias, FBI graphs show substantial under-forecasts in the Cala4 and Cala8 zones and an over-forecast in the Cala7 zone. However, in this case, the GFS-based simulations provide better results, especially in Cala7 and for high thresholds. Results achieved with ETS and FBI are generally also confirmed by other scores (not shown), such as the probability of detection (POD) score or the false alarm rate (FAR).
As previously stated, higher skin SST Medspiration values affect precipitation magnitude. This outcome agrees with the average increase of upward moisture flux from the sea surface in D01 (+8 % with GFS, +13 % with IFS, and IFS-DA in the 4 d time period). Vice versa (and contrary to what was found in the previous case study), the simulations do not show relevant differences in the timing of the event. If the accumulated values of average precipitation in each of the warning areas are considered, all simulations are very highly correlated (≥0.98, graph not shown) with observations. Figure 13, showing the time series of hourly averaged water vapour flux through section B–B', highlights that there are no relevant forward nor backward time deviations between the simulations with original skin SST fields and the corresponding simulations with Medspiration fields. The main effect observed in Fig. 13 is the lower flux of the GFS-based simulations because the main flow of soil moisture is shifted towards the north-east with respect to section B–B' (in agreement with the precipitation maps in Fig. 11). The average flux increase with IFS-M and IFS-DA-M is about 3 %–4 % compared with IFS-O and IFS-DA-O respectively. Figure 14, showing a snapshot of the IWV distribution in D01 during the event (31 October at 21:00 UTC), confirms the similar timing of the simulations. Moving from IFS-O to IFS-DA-O to IFS-DA-M, the size of the cluster of humid air south of Calabria increases, but its position is basically the same. Similar conclusions are inferred from Fig. S6, which provides additional information about 850 hPa wind fields in D02 at the same time as in Fig. 14.
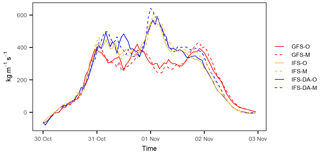
Figure 13Time evolution of the vertically integrated water vapour flux (kg m−1 s−1) crossing section B–B' (shown in Fig. 3b) from 00:00 UTC on 30 October 2015 to 00:00 UTC on 3 November 2015.
All simulations performed for this case study show that the greater energy supplied to the system by the higher skin SST Medspiration fields affects lower layers' flow dynamics, allowing more transport but not accelerating it. This behaviour can be attributed to the long-lasting characteristics of the event that, developing at a wider scale than case study 1 and providing humid air continuously, smooths potential differences in terms of timing.
Assessing the hydrological impact in the two selected catchments is more interesting in this case study, because all simulations forecast heavy rain over the catchment areas of the Ancinale River and Bonamico Creek, yet it is still challenging because reliable hydrological forecasts require accurate QPFs at the catchment scale. A QPF performance analysis is carried out for the catchment areas, considering the average values of the interpolated precipitation fields. The simulated average precipitation over the Ancinale River catchment is strongly overestimated by all of the IFS-based simulations in the 4 d forecasts (from +53 % to +72 %). Such over-forecasts are only partially reduced to about +40 % (except for an increase to +80 % with IFS-O) in the 3 d forecasts. GFS-based simulations provide much more reasonable biases in the 4 d forecasts (+12 % and −1 % for GFS-O and GFS-M respectively), which are only partially confirmed in the 3 d forecasts, where GFS-M provides a nearly unbiased estimate (−3 %) but the GFS-O over-forecast worsens to +44 %. Concerning the Bonamico Creek catchment, in the 4 d forecasts the IFS-based biases are smaller in general (from −13 % to +22 %, but +54 % with IFS-DA-M), whereas GFS-based simulations strongly under-forecast (−56 % and −43 % with GFS-O and GFS-M respectively). The 3 d bias is reduced for IFS-DA-based forecasts and slightly increased for IFS-based forecasts, with values of around −20 % in all cases. GFS-based forecasts are much improved (+10 % and −16 % for GFS-O and GFS-M respectively). The Taylor diagrams in Fig. 15a–d, based on the comparison of the average rainfall time series, generally show better performances of the GFS-based simulations in the Ancinale River and of the IFS-based simulations in the Bonamico Creek.
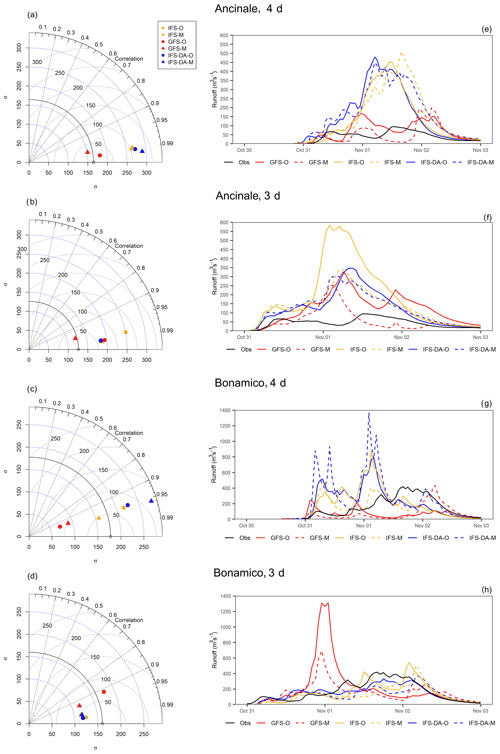
Figure 15(a–d) Taylor diagrams related to the averaged hourly precipitation series over the Ancinale River catchment and the Bonamico Creek catchment simulated by the different configurations forecasting both 4 and 3 d, compared with observations. (e–h) The resulting hydrographs (m s−3) obtained by the different WRF-Hydro simulations compared with observations.
QPF analysis only partially reflects the main outcomes of the hydrological simulations, performed with the offline WRF-Hydro model forced with the WRF downscaled forecasts. According to precipitation over-forecast of the IFS-based simulations, both Fig. 15e and g (4 d simulations) show a very relevant overestimation of all IFS-based hydrographs (except IFS-M for Bonamico Creek). Nevertheless, in the case of the Ancinale River, IFS-O, IFS-M, and IFS-DA-M hydrographs are reasonably correlated with observations (correlation coefficient, r, values of 0.62, 0.77, and 0.70 respectively). Furthermore, simulated peak flow times of IFS-O and IFS-M hydrographs are very close to the observed values, occurring on 1 November at 12:00 UTC (1 h before and 4 h after the observed peak flow respectively). The hydrographs resulting from GFS simulations are closer to observations in terms of volumes; nevertheless, peak times are brought significantly forward in time or are delayed. Concerning the Bonamico Creek, IFS-based hydrographs are not well correlated and forecast peak flows are more than 12 h in advance compared with observations, whereas GFS-based hydrographs considerably underestimate flows.
The 3 d forecasts do not provide a substantial improvement. The hydrological simulations over the Ancinale River (Fig. 15f) are still affected by precipitation over-forecasts. Furthermore, all simulations forecast the peak flows in advance compared with observations. Nevertheless, IFS-M, IFS-DA-O, and IFS-DA-M show r values of around 0.6. In particular, IFS-DA-O (highest r value of 0.65) forecasts the peak flow only 4 h in advance. Despite the good performance with precipitation forecasts, the GFS-M hydrograph is not well correlated with observations and simulates the observed peak flow about 9 h in advance. For Bonamico Creek, IFS-DA-O results are even better (Fig. 15h). The simulated peak flow, according to precipitation forecasts, underestimates the observed peak flow by about 20 %, but the correlation between the simulated and observed hydrographs is high (0.89) and the observed peak flow time (1 November at 16:00 UTC) is delayed by only 2 h. Generally, all of the IFS-based simulations are well correlated (r values always higher than 0.6) even though the peak flow time is always delayed (by up to 12 h). GFS-based simulations are poorly correlated and show significant overestimation and early forecast of the peak flow.
Table 5 aims to support the discussion summarizing the main outcomes concerning (1) the representation of the skin SST fields; (2) the accumulated precipitation values in the internal domain and the related spatial distribution; (3) the time distribution of precipitation; and (4) hydrological impact (hydrograph shape, total discharge, peak flow times), depending on the GCM choice for determining the boundary conditions, the use of the 3D-Var scheme, and the use of the high-resolution Medspiration fields.
Table 5Synoptic table summarizing the main findings for the different case studies. “Medsp” refers to Medspiration, “BC” refers to boundary conditions, “CP” refers to civil protection, and “DA” refers to data assimilation.
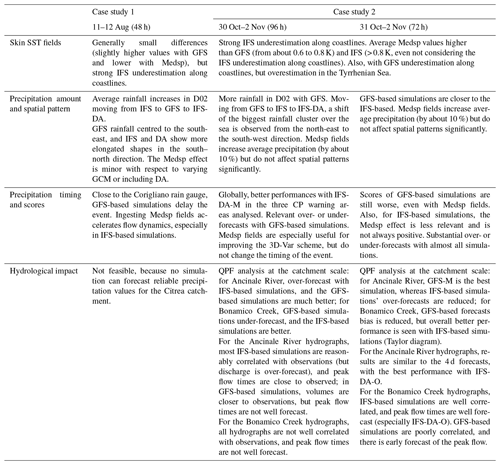
The most evident outcome across the case studies, yet far from surprising, is that the choice of the GCM providing boundary conditions is, comparatively, the most relevant factor affecting the simulations. Specifically, for the case studies analysed, GFS-based simulations generally do not perform as well as IFS-based simulations (this difference is emphasized if the forecast time window is increased, as demonstrated in case study 2). Of course, this is not a generalizable result given the few events involved and the lack of further analyses (e.g. the evaluation of different parameterizations). For example, for case study 2, Avolio and Federico (2018) found (via detailed sensitivity tests) that simulations forced by GFS have better performance than those forced by ECMWF data. Nevertheless, for the purpose of this study it is shown that the various features differentiating the two GCMs (including the spatial resolution, which is improved with IFS) can considerably affect the precipitation fields calculated via dynamical downscaling, comparatively more than using 3D-Var data assimilation methods or imposing specific (high-resolution) skin SST boundary conditions.
The use of the 3D-Var scheme in this study has to be primarily considered as a strategy for improving initial conditions. Several studies have adopted data assimilation approaches to achieve improvements for forecast periods shorter than the 48 to 96 h used in this study (e.g. Sun et al., 2016; Gustafsson et al., 2018; Thiruvengadam et al., 2019), unless specific strategies were used (e.g. cycling 3D-Var runs; Liu et al., 2018). Here, we focus on 2 to 4 d periods (depending on the case studies) for the sake of simplicity and clarity, testing the capability of different model configurations to reproduce the overall development of the hydrometeorological events, from their beginning to their end, and also checking their usefulness with respect to providing a proper warning lead time. Therefore, extensively testing the 3D-Var scheme in order to get the highest benefit goes beyond the scope of this study.
Even though they are used with the outlined limitations, 3D-Var simulations provide some worthy outcomes. For case study 1, applying the 3D-Var scheme with IFS boundary conditions results in a substantial increase in the average rainfall in the innermost domain (up to 25 %), but this change does not provide clear advances in forecasting skill. This is consistent with previous studies which have demonstrated that the effects of data assimilation do not lead to an effective improvement for highly convective events (Liu et al., 2013). In case study 2, it is noteworthy that IFS-O is capable of providing better ETS values than IFS-DA-O in some warning areas, especially in the 4 d forecasts, meaning that sources of uncertainty other than initial conditions can strongly affect forecasting skill. Among those uncertainties, representation of SST conditions can be important, given that, in general, IFS-DA-M (i.e. the simulation including both data assimilation and high-resolution SST representation) provides better performance.
Unlike the 3D-Var scheme, the effects of high-resolution SST representation on forecasts are emphasized to the maximum in this study; this is due to the fact that observed rather than forecast SST fields are replaced as lower boundary conditions in the simulations, which provides a kind of “upper limit” to the effects provided by well forecast SST fields. The foundation SST fields used (defined as the temperature of the water column, free of diurnal temperature variability; Donlon et al., 2007) are produced by the Medspiration project once every 24 h, but the diurnal cycles are ensured by the sst_skin option. They especially improve the SST fields provided by IFS boundary conditions that, although they allow better forecasts than GFS, show very evident problems along the coastlines. The forecast periods analysed in this study allow users to largely overcome the problem highlighted by Cassola et al. (2016), who found that the forced ingestion of high-resolution SST fields can be counterproductive for forecasting ranges shorter than 36–48 h, due to the relatively slow adjustment of initial atmospheric fields.
High-resolution SST fields often provide (but not always, and not always significantly) enhanced forecast performance with respect to the corresponding simulations with native SST fields. Especially in case study 1, the effects close to the Corigliano rain gauge seem to be somehow linked to generally chaotic behaviour. As discussed in the case of improved initial conditions (i.e. the 3D-Var scheme), these outcomes are related to the fact that sources of uncertainties other than SST representation hinder enhanced forecast skill. Furthermore, the average impact of high-resolution SST on the simulated precipitation in D02 is lower than expected with comprehensive approaches used to represent simulation uncertainty (e.g. ensemble forecast systems applied at convection-permitting resolutions; Evans et al., 2014). A preliminary analysis performed by the authors on case study 1 (not shown) using a convection-permitting ensemble system based on the ECMWF ensemble prediction system (EPS) highlights a mean absolute percentage deviation of about 24 % for the perturbed simulations (against that found here, related to the SST fields resolution, of 4 %).
Nevertheless, using more realistic SST fields leads to enough clear changes in the simulation of the atmospheric boundary layer dynamics in both case studies, especially with respect to the configurations with clearly unrealistic fields (i.e. IFS lower boundary conditions). Specifically, in the (shorter, convective, highly localized) summer event higher SST values along the coastlines accelerate flow dynamics, moving faster humid air towards the coast and moving precipitation up (thus agreeing with the results achieved by Stocchi and Davolio, 2017). Conversely, in the (longer, caused by a frontal system, widespread) autumn event the higher energy supplied to the system by a continuously warmer sea surface leads to a generalized increase of the precipitation amount; however, this does not substantially change either the spatial pattern or the timing of the event. The missing change in timing is most probably due to the fact that the stability of the atmospheric boundary layer and the related flow dynamics in case study 2 depend more on large-scale (synoptic) conditions than on local factors (which is also possibly the same reason that the 3D-Var scheme is capable of influencing case study 2 more than case study 1). Such large-scale conditions are capable of leading to much stronger winds than seen in case study 1 (which is evident when comparing Figs. S3 and S6).
Exploring the hydrological impact of case study 2 in detail, the analysis must be related to the resolution of the small-scale catchments, where the experiments show that results achieved on larger scales (i.e. at the resolution of the warning areas) can be “doubly” reversed. For example, both bias analyses and Taylor diagrams related to the Ancinale River basin (Fig. 15a, b) highlight better QPF performance for GFS-based simulations, which is not as obvious (or even not found) in the analysis of models skills on a larger scale. Nevertheless, IFS-based hydrographs are better correlated with those calculated using observed rainfall and peak flow times that are closer to observed values (it is worth recalling that a quantitative discharge analysis is less significant in this case, given that only water level observations are available). Contrary to what was found by Yucel et al. (2015), streamflow simulations are not particularly improved by initial data assimilation. This result is most likely due to the relatively long forecast periods (from 72 to 96 h). Indeed, in the 3 d forecasts, the benefits of the improved initial conditions partially come to light in both catchments even though, interestingly, the best simulation (even if only slightly) is IFS-DA-O, i.e. that using the 3D-Var scheme but not the Medspiration SST fields. Overall, the impact of a reduced lead time from 4 to 3 d provides only slight enhancements (e.g. better performance of the GFS-based simulations or slightly higher ETS values); however, these enhancements do not considerably affect the performance level of the hydrological forecasts.
Summarizing, the results achieved in this study show that none of the different versions of the forecasting chain adopted are capable of achieving quantitative precipitation and (consequently) streamflow forecast in all of the cases analysed, yet several interesting clues are provided. Specifically, similar to past studies, it is shown that the high-resolution representation of SST fields can significantly change the simulation of the atmospheric boundary layer processes, modifying flow dynamics and/or the amount of precipitated water. Nevertheless, the potentially positive impact of high-resolution SST fields can be easily hidden by several other sources of uncertainty (mainly, the relevance of the choice of the GCM providing boundary conditions). Further improvements in both GCMs (e.g. the higher-resolution IFS cycle since March 2016) and RCMs will reduce uncertainties, which clearly highlights the need for high-resolution SST representation in regional modelling. The topic of higher temporal frequency updating of lateral boundary conditions is also being actively investigated (Termonia et al., 2009; Matte et al., 2017; Keresturi et al., 2019). Furthermore, emerging approaches like regional-scale fully coupled ocean–atmospheric (e.g. within the Baltic Sea Experiment – BALTEX, Gustafsson et al., 1998; Pullen et al., 2003; Ren et al., 2004; Loglisci et al., 2004; Ricchi et al., 2019; Lewis et al., 2019b) or ocean–atmospheric–hydrologic (Ruti et al.; 2016; Somot et al., 2018) modelling aim to directly calculate SST fields dynamics. Meanwhile, with the current generation of operational models, a reasonable (yet computationally demanding) solution is to adequately take the uncertainty of SST in forecasting chains into account by also adopting ensemble approaches for this variable.
Rainfall data are available upon request from the Centro Funzionale Multirischi – ARPACAL (2019; http://www.cfd.calabria.it/index.php/dati-stazioni/dati-storici). Radar data are available upon request from the Italian National Civil Protection “Centro Funzionale Centrale Rischio Meteo-idrogeologico e Idraulico – Italian Government” (2019; http://www.protezionecivile.gov.it/home). Instructions for acquiring Medspiration L4 ultra-high-resolution SSTfnd data are provided at http://cersat.ifremer.fr/thematic-portals/projects/medspiration/access-to-data-and-services (Medspiration Service, 2019). Observations used to perform data assimilation are available at https://doi.org/10.5065/39C5-Z211 (NCEP, 2004). Simulation data are available from the corresponding author upon request.
The supplement related to this article is available online at: https://doi.org/10.5194/hess-24-269-2020-supplement.
AS and GM conceptualized the study. AS and LF developed the methodology with support from GM. AS and LF wrote the original draft of the paper. LF carried out the simulations and performed the statistical analyses with support from AS. LF prepared the figures with support from AS. GM supervised the research activity and reviewed the original draft of the paper.
The authors declare that they have no conflict of interest.
This article is part of the special issue “Hydrological cycle in the Mediterranean (ACP/AMT/GMD/HESS/NHESS/OS inter-journal SI)”. It is not associated with a conference.
We thank the “Centro Funzionale Multirischi” of the Calabrian Regional Agency for the Protection of the Environment for providing the observed precipitation data and Italian National Civil Protection “Centro Funzionale Centrale Rischio Meteo-idrogeologico e Idraulico” for providing radar data. Luca Furnari acknowledges support from the “POR Calabria FSE/FESR 2014/2020 – Mobilità internazionale di Dottorandi e Assegnisti di Ricerca/Ricercatori di Tipo A” programme (action nos. 10.5.6 and 10.5.12).
This research has been supported by the POR Calabria FSE/FESR 2014/2020 (grant nos. 10.5.6 and 10.5.12).
This paper was edited by Giuseppe Tito Aronica and reviewed by two anonymous referees.
Allen, M., Frame, D., Kettleborough, J., and Stainforth, D.: Model error in weather and climate forecasting, in: Palmer, T. and Hagedorn, R., Predictability of Weather and Climate (391–427), Cambridge University Press, Cambridge, https://doi.org/10.1017/CBO9780511617652.016, 2002.
Arnault, J., Wagner, S., Rummler, T., Fersch, B., Bliefernicht, J., Andresen, S., and Kunstmann, H.: Role of Runoff–Infiltration Partitioning and Resolved Overland Flow on Land–Atmosphere Feedbacks: A Case Study with the WRF-Hydro Coupled Modelling System for West Africa, J. Hydrometeorol., 17, 1489–1516, https://doi.org/10.1175/JHM-D-15-0089.1, 2016.
Avolio, E. and Federico, S.: WRF simulations for a heavy rainfall event in southern Italy: Verification and sensitivity tests, Atmos. Res., 209, 14–35, https://doi.org/10.1016/j.atmosres.2018.03.009, 2018.
Avolio, E., Cavalcanti, O., Furnari, L., Senatore, A., and Mendicino, G.: Brief communication: Preliminary hydro-meteorological analysis of the flash flood of 20 August 2018 in Raganello Gorge, southern Italy, Nat. Hazards Earth Syst. Sci., 19, 1619–1627, https://doi.org/10.5194/nhess-19-1619-2019, 2019.
Barker, D., Huang, X.-Y, Liu, Z., Auligné, T., Zhang, X., Rugg, S., Ajjaji, R., Bourgeois, A., Bray, J., Chen, Y., Demirtas, M., Guo, Y.-R, Henderson, T., Huang, W., Lin, H.-C., Michalakes, J., Rizvi, S., and Zhang, X.: The Weather Research and Forecasting Model's Community Variational/Ensemble Data Assimilation System: WRFDA, B. Am. Meteorol. Soc., 93, 831–843, https://doi.org/10.1175/BAMS-D-11-00167.1, 2012.
Barker, D. M., Huang, W., Guo, Y. R., Bourgeois, A. J., and Xiao, Q. N.: A Three-Dimensional (3DVAR) Data Assimilation System For Use With MM5: Implementation and Initial Results, Mon. Weather Rev., 132, 897–914, https://doi.org/10.1175/1520-0493(2004)132<0897:ATVDAS>2.0.CO;2, 2004.
Berthou, S., Mailler, S., Drobinski, P., Arsouze, T., Bastin, S., Béranger, K., and Lebeaupin-Brossier, C.: Prior history of Mistral and Tramontane winds modulates heavy precipitation events in southern France, Tellus A, 66, 24064, https://doi.org/10.3402/tellusa.v66.24064, 2014.
Berthou, S., Mailler, S., Drobinski, P., Arsouze, T., Bastin, S., Béranger, K., and Lebeaupin-Brossier, C.: Sensitivity of an intense rain event between atmosphere-only and atmosphere–ocean regional coupled models: 19 September 1996, Q. J. Roy. Meteor. Soc., 141, 258–271, https://doi.org/10.1002/qj.2355, 2015.
Berthou, S., Mailler, S., Drobinski, P., Arsouze, T., Bastin, S., Béranger, K., Flaounas, E., Lebeaupin Brossier, C., Somot, S., and Stéfanon, M.: Influence of submonthly air–sea coupling on heavy precipitation events in the Western Mediterranean basin, Q. J. Roy. Meteor. Soc., 142, 453–471, https://doi.org/10.1002/qj.2717, 2016.
Buizza, R.: Chapter 2 – Ensemble Forecasting and the Need for Calibration, in: Statistical Postprocessing of Ensemble Forecasts, edited by: Vannitsem, S., Wilks, D. S., and Messner, J. W., Elsevier, 15–48, ISBN 9780128123720, https://doi.org/10.1016/B978-0-12-812372-0.00002-9, 2018.
Cabanes, C., Grouazel, A., von Schuckmann, K., Hamon, M., Turpin, V., Coatanoan, C., Paris, F., Guinehut, S., Boone, C., Ferry, N., de Boyer Montégut, C., Carval, T., Reverdin, G., Pouliquen, S., and Le Traon, P.-Y.: The CORA dataset: validation and diagnostics of in-situ ocean temperature and salinity measurements, Ocean Sci., 9, 1–18, https://doi.org/10.5194/os-9-1-2013, 2013.
Cassola, F., Ferrari, F., Mazzino, A., and Miglietta, M. M.: The role of the sea on the flash floods events over Liguria (northwestern Italy), Geophys. Res. Lett., 43, 3534–3542, https://doi.org/10.1002/2016GL068265, 2016.
Centro Funzionale Centrale Rischio Meteo-idrogeologico e Idraulico – Italian Government, Home page: available at: http://www.protezionecivile.gov.it/home, last access: 5 December 2019.
Centro Funzionale Multirischi – ARPACAL: Historical dataset, available at: http://www.cfd.calabria.it/index.php/dati-stazioni/dati-storici, last access: 5 December 2019.
CFM (Centro Funzionale Multirischi della Calabria): Technical report, Rapporto speditivo di evento metopluviometrico del 12 agosto 2015, available at: http://www.cfd.calabria.it/DatiVari/Pubblicazioni/rapporto di evento 12 agosto.pdf (last access: 4 November 2019), 2015a (in Italian).
CFM (Centro Funzionale Multirischi della Calabria): Technical report, Rapporto di evento metopluviometrico del 30 ottobre 2 novembre 2015, available at: http://www.cfd.calabria.it/DatiVari/Pubblicazioni/rapporto di evento 30 ottobre-2 novembre2015.pdf (last access: 4 November 2019), 2015b (in Italian).
Chen, S. H. and Sun, W.-Y.: A One-dimensional Time Dependent Cloud Model, J. Meteorol. Soc. Jpn., 80, 99–118, https://doi.org/10.2151/jmsj.80.99, 2002.
Chiaravalloti, F. and Gabriele, S.: Vibo Valentia flood and MSG rainfall evaluation, Atmos. Res., 93, 286–294, https://doi.org/10.1016/j.atmosres.2008.10.027, 2009.
Cohen, S., Praskievicz, S., and Maidment, D. R.: Featured Collection Introduction: National Water Model, J. Am. Water Resour. As., 54, 767–769, https://doi.org/10.1111/1752-1688.12664, 2018.
Cuo, L., Pagano, T. C., and Wang, Q. J.: A Review of Quantitative Precipitation Forecasts and Their Use in Short- to Medium-Range Streamflow Forecasting, J. Hydrometeorol., 12, 713–728, https://doi.org/10.1175/2011JHM1347.1, 2011.
Davolio, S., Silvestro, F., and Malguzzi, P.: Effects of Increasing Horizontal Resolution in a Convection-Permitting Model on Flood Forecasting: The 2011 Dramatic Events in Liguria, Italy, J. Hydrometeorol., 16, 1843–1856, https://doi.org/10.1175/JHM-D-14-0094.1, 2015.
Davolio, S., Silvestro, F., and Gastaldo, T.: Impact of Rainfall Assimilation on High-Resolution Hydrometeorological Forecasts over Liguria, Italy, J. Hydrometeorol., 18, 2659–2680, https://doi.org/10.1175/JHM-D-17-0073.1, 2017.
Donlon, C., Robinson, I., Casey, K. S., Vazquez-Cuervo, J., Armstrong, E., Arino, O., Gentemann, C., May, D., LeBorgne, P., Piollé, J., Barton, I., Beggs, H., Poulter, D. J., Merchant, C. J., Bingham, A., Heinz, S., Harris, A., Wick, G., Emery, B., Minnett, P., Evans, R., Llewellyn-Jones, D., Mutlow, C., Reynolds, R. W., Kawamura, H., and Rayner, N.: The Global Ocean Data Assimilation Experiment High-resolution Sea Surface Temperature Pilot Project, B. Am. Meteorol. Soc., 88, 1197–1214, https://doi.org/10.1175/BAMS-88-8-1197, 2007.
Drobinski, P., Silva, N.D., Panthou, G., Bastin, S., Muller, C., Ahrens, B., Borga, B., Conte, D., Fosser, G., Giorgi, F., Güttler, I., Kotroni, V., Li, L., Morin, E., Önol, B., Quintana-Segui, P., Romera, R., and Torma, C. S.: Scaling precipitation extremes with temperature in the Mediterranean: past climate assessment and projection in anthropogenic scenarios, Clim. Dynam., 51, 1237–1257, https://doi.org/10.1007/s00382-016-3083-x, 2018.
Dudhia, J.: Numerical Study of Convection Observed during the Winter Monsoon Experiment Using a Mesoscale Two-Dimensional Model, J. Atmos. Sci., 46, 3077–3107, https://doi.org/10.1175/1520-0469(1989)046<3077:NSOCOD>2.0.CO;2, 1989.
Evans, C., Dyke, D., and Lericos, T.: How Do Forecasters Utilize Output from a Convection-Permitting Ensemble Forecast System? Case Study of a High-Impact Precipitation Event, Weather Forecast., 29, 466–486, https://doi.org/10.1175/WAF-D-13-00064.1, 2014.
Federico, S., Bellecci, C., and Colacino, M.: Numerical simulation of Crotone flood: storm evolution, Il Nuovo Cimento C., 26, 357–371, 2003a.
Federico, S., Bellecci, C., and Colacino, M.: Quantitative precipitation of the Soverato flood: the role of orography and surface fluxes, Il Nuovo Cimento C., 26, 7–22, 2003b.
Federico, S., Avolio, E., Bellecci, C., Lavagnini, A., Colacino, M., and Walko, R. L.: Numerical analysis of an intense rainstorm occurred in southern Italy, Nat. Hazards Earth Syst. Sci., 8, 19–35, https://doi.org/10.5194/nhess-8-19-2008, 2008.
Federico, S., Pasqualoni, L., Avolio, E., and Bellecci, C.: Brief communication “Calabria daily rainfall from 1970 to 2006”, Nat. Hazards Earth Syst. Sci., 10, 717–722, https://doi.org/10.5194/nhess-10-717-2010, 2010.
Gascón, E., Laviola, S., Merino, A., and Miglietta, M. M.: Analysis of a localized flash-flood event over the central Mediterranean, Atmos. Res., 182, 256–268, https://doi.org/10.1016/j.atmosres.2016.08.007, 2016.
Gochis, D. J., Yu, W., and Yates, D.: The WRF-Hydro model technical description and user's guide, version 3.0, NCAR Tech. Doc. 120, https://doi.org/10.5065/D6DN43TQ, 2015.
Gronewold, A. D., Clites, A. H., Hunter, T. S., and Stow, C. G.: An appraisal of the Great Lakes advanced hydrologic prediction system, J. Great Lakes Res., 37, 577–583, https://doi.org/10.1016/j.jglr.2011.06.010, 2011.
Gustafsson, N., Nyberg, L., and Omstedt, A.: Coupling of a High-Resolution Atmospheric Model and an Ocean Model for the Baltic Sea, Mon. Weather Rev., 126, 2822–2846, https://doi.org/10.1175/1520-0493(1998)126<2822:COAHRA>2.0.CO;2, 1998.
Gustafsson, N, Janjić, T, Schraff, C, Leuenberger, D., Weissmann, M., Reich, H., Brousseau, P., Montmerle, T., Wattrelot, E., Bučánek, A., Mile, M., Hamdi, R., Lindskog, M., Barkmeijer, J., Dahlbom, M., Macpherson, B., Ballard, S., Inverarity, G., Carley, J., Alexander, C., Dowell, D., Liu, S., Ikuta, Y., and Fujita, T.: Survey of data assimilation methods for convective-scale numerical weather prediction at operational centres, Q. J. Roy. Meteor. Soc., 144, 1218–1256, https://doi.org/10.1002/qj.3179, 2018.
Hodur, R. M.: The Naval Research Laboratory's Coupled Ocean/Atmosphere Mesoscale Prediction System (COAMPS), Mon. Weather Rev., 125, 1414–1430, https://doi.org/10.1175/1520-0493(1997)125<1414:TNRLSC>2.0.CO;2, 1997.
Huang, X. Y., Xiao, Q., Barker, D. M., Zhang, X., Michalakes, J., Huang, W., Henderson, T., Bray, J., Chen, Y., Ma, Z., Dudhia, J., Guo, Y., Zhang, X., Won, D. J., Lin, H. C., and Kuo, Y. H.: Four-Dimensional Variational Data Assimilation for WRF: Formulation and Preliminary Results, Mon. Weather Rev., 137, 299–314, https://doi.org/10.1175/2008MWR2577.1, 2009.
Hydrologic Engineering Center: HEC-RAS, River Analysis System, Hydraulic Reference Manual, Version 5.0, U.S. Army Corps of Engineers, Davis, California, 2016.
Ivatek-Šahdan, Stanešić, S. A., Tudor, M., Plenković, I. O., and Janeković, I.: Impact of SST on heavy rainfall events on eastern Adriatic during SOP1 of HyMeX, Atmos. Res., 200, 36–59, https://doi.org/10.1016/j.atmosres.2017.09.019, 2018.
Janjić, Z. I.: The Step-Mountain Eta Coordinate Model: Further Developments of the Convection, Viscous Sublayer, and Turbulence Closure Schemes, Mon. Weather Rev., 122, 927–945, https://doi.org/10.1175/1520-0493(1994)122< 0927:TSMECM>2.0.CO;2, 1994.
Jansa, A., Alpert, P., Arbogast, P., Buzzi, A., Ivancan-Picek, B., Kotroni, V., Llasat, M. C., Ramis, C., Richard, E., Romero, R., and Speranza, A.: MEDEX: a general overview, Nat. Hazards Earth Syst. Sci., 14, 1965–1984, https://doi.org/10.5194/nhess-14-1965-2014, 2014.
Kain, J. S.: The Kain–Fritsch Convective Parameterization: An Update, J. Appl. Meteorol., 43, 170–181, https://doi.org/10.1175/1520-0450(2004)043<0170:TKCPAU>2.0.CO;2, 2004.
Katsafados, P., Mavromatidis, E., Papadopoulos, A., and Pytharoulis, I.: Numerical simulation of a deep Mediterranean storm and its sensitivity on sea surface temperature, Nat. Hazards Earth Syst. Sci., 11, 1233–1246, https://doi.org/10.5194/nhess-11-1233-2011, 2011.
Keresturi, E., Wang, Y., Meier, F., Weidle, F., and Wittman, C.: Improving initial condition perturbations in a convection-permitting ensemble prediction system, Q. J. Roy. Meteor. Soc., 145, 993–1012, https://doi.org/10.1002/qj.3473, 2019.
Lahmers, T. M., Gupta, H., Castro, C. L., Gochis, D. J., Yates, D., Dugger, A., Goodrich, D., and Hazenberg, P.: Enhancing the Structure of the WRF-Hydro Hydrologic Model for Semiarid Environments, J. Hydrometeorol., 20, 691–714, https://doi.org/10.1175/JHM-D-18-0064.1, 2019.
Lebeaupin, C., Ducrocq, V., and Giordani, H.: Sensitivity of torrential rain events to the sea surface temperature based on high-resolution numerical forecasts, J. Geophys. Res., 111, D12110, https://doi.org/10.1029/2005JD006541, 2006.
Lewis, H. W., Castillo Sanchez, J. M., Arnold, A., Fallmann, J., Saulter, A., Graham, J., Bush, M., Siddorn, J., Palmer, T., Lock, A., Edwards, J., Bricheno, L., Martínez-de la Torre, A., and Clark, J.: The UKC3 regional coupled environmental prediction system, Geosci. Model Dev., 12, 2357–2400, https://doi.org/10.5194/gmd-12-2357-2019, 2019a.
Lewis, H. W., Siddorn, J., Castillo Sanchez, J. M., Petch, J., Edwards, J. M., and Smyth, T.: Evaluating the impact of atmospheric forcing and air–sea coupling on near-coastal regional ocean prediction, Ocean Sci., 15, 761–778, https://doi.org/10.5194/os-15-761-2019, 2019b.
Li, J., Chen, Y., Wang, H., Qin, J., Li, J., and Chiao, S.: Extending flood forecasting lead time in a large watershed by coupling WRF QPF with a distributed hydrological model, Hydrol. Earth Syst. Sci., 21, 1279–1294, https://doi.org/10.5194/hess-21-1279-2017, 2017.
Lin, P., Yang, Z.-L. Gochis, D. J., Yu, W., Maidment, D. R., Somos-Valenzuela, M. A., and David, C. H.: Implementation of a vector-based river network routing scheme in the community WRF-Hydro modelling framework for flood discharge simulation, Environ. Modell. Softw., 107, 1–11, https://doi.org/10.1016/j.envsoft.2018.05.018, 2018.
Liu, J., Bray, M., and Han, D.: Exploring the effect of data assimilation by WRF-3DVar for numerical rainfall prediction with different types of storm events, Hydrol. Process., 27, 3627–3640, https://doi.org/10.1002/hyp.9488, 2013.
Liu, J., Tian, J., Yan, D., Li, C., Yu, F., and Shen, F.: Evaluation of Doppler radar and GTS data assimilation for NWP rainfall prediction of an extreme summer storm in northern China: from the hydrological perspective, Hydrol. Earth Syst. Sci., 22, 4329–4348, https://doi.org/10.5194/hess-22-4329-2018, 2018.
Liu, Y., Weerts, A. H., Clark, M., Hendricks Franssen, H.-J., Kumar, S., Moradkhani, H., Seo, D.-J., Schwanenberg, D., Smith, P., van Dijk, A. I. J. M., van Velzen, N., He, M., Lee, H., Noh, S. J., Rakovec, O., and Restrepo, P.: Advancing data assimilation in operational hydrologic forecasting: progresses, challenges, and emerging opportunities, Hydrol. Earth Syst. Sci., 16, 3863–3887, https://doi.org/10.5194/hess-16-3863-2012, 2012.
Llasat, M. C., Llasat-Botija, M., Petrucci, O., Pasqua, A. A., Rosselló, J., Vinet, F., and Boissier, L.: Towards a database on societal impact of Mediterranean floods within the framework of the HYMEX project, Nat. Hazards Earth Syst. Sci., 13, 1337–1350, https://doi.org/10.5194/nhess-13-1337-2013, 2013.
Loglisci, N., Qian, M. W., Rachev, N., Cassardo, C., Longhetto, A., Purini, R., Trivero, P., Ferrarese, S., and Giraud, C.: Development of an atmosphere-ocean coupled model and its application over the Adriatic Sea during a severe weather event of Bora wind, J. Geophys. Res., 109, D01102, https://doi.org/10.1029/2003JD003956, 2004.
Manzato, A., Davolio, S., Miglietta, M. M., Pucillo, A., and Setvák, M.: 12 September 2012: A supercell outbreak in NE Italy?, Atmos. Res., 153, 98–118, https://doi.org/10.1016/j.atmosres.2014.07.019, 2015.
Matte, D., Laprise, R., Thériault, J. M., and Lucs-Picher, P.: Spatial spin-up of fine scales in a regional climate model simulation driven by low-resolution boundary conditions, Clim. Dynam., 49, 563–574, https://doi.org/10.1007/s00382-016-3358-2, 2017.
Medspiration Service: Access to data and services available at: http://cersat.ifremer.fr/thematic-portals/projects/medspiration/access-to-data-and-services, last access: 5 December 2019.
Mellor, G. L. and Yamada, T.: Development of a turbulence closure model for geophysical fluid problems, Rev. Geophys., 20, 851–875, https://doi.org/10.1029/RG020i004p00851, 1982.
Merchant, C. J., Filipiak, M. J., Le Borgne, P., Roquet, H., Autret, E., Piolle, J. F., and Lavender, S.: Diurnal warm-layer events in the western Mediterranean and European shelf seas, Geophys. Res. Lett., 35, L04601, https://doi.org/10.1029/2007GL033071, 2008.
Meredith, E. P., Maraun, D., Semenov, V. A., and Park, W.: Evidence for added value of convection-permitting models for studying changes in extreme precipitation, J. Geophys. Res.-Atmos., 120, 12500–12513, https://doi.org/10.1002/2015JD024238, 2015.
Miglietta, M. M., Moscatello, A., Conte, D., Mannarini, G., Lacorata, G., and Rotunno R.: Numerical analysis of a Mediterranean “hurricane” over south-eastern Italy: Sensitivity experiments to sea surface temperature, Atmos. Res., 101, 412–426, https://doi.org/10.1016/j.atmosres.2011.04.006, 2011.
Miglietta, M. M., Bueso, J., Motola, V., and Pasini, A.: Effect of a positive Sea Surface Temperature anomaly on a Mediterranean tornadic supercell, Sci. Rep.-UK, 7, 12828, https://doi.org/10.1038/s41598-017-13170-0, 2017.
Mlawer, E. J., Taubman, S. J., Brown, P. D., Iacono, M. J., and Clough, S. A.: Radiative transfer for inhomogeneous atmospheres: RRTM, a validated correlated-k model for the longwave, J. Geophys. Res., 102, 16663–16682, https://doi.org/10.1029/97JD00237, 1997.
NCEP: NCEP ADP Global Upper Air Observational Weather Data, October 1999–continuing, https://doi.org/10.5065/39C5-Z211, 2004.
Pagano, T. C., Wood, A. W., Ramos, M. H., Cloke, H. L., Pappenberger, F., Clark, M. P., Cranston, M., Kavetski, D., Mathevet, T., Sorooshian, S., and Verkade, J. S.: Challenges of operational river forecasting, J. Hydrometeorol., 15, 1692–1707, https://doi.org/10.1175/JHM-D-13-0188.1, 2014.
Pastor, F., Valiente, J. A., and Estrela, M. J.: Sea surface temperature and torrential rains in the Valencia region: modelling the role of recharge areas, Nat. Hazards Earth Syst. Sci., 15, 1677–1693, https://doi.org/10.5194/nhess-15-1677-2015, 2015.
Petrucci, O., Salvati, P., Aceto, L., Bianchi, C., Pasqua, A. A., Rossi, M., and Guzzetti, F.: The Vulnerability of People to Damaging Hydrogeological Events in the Calabria Region (Southern Italy), Int. J. Environ. Res. Pu., 15, 48, https://doi.org/10.3390/ijerph15010048, 2018.
Pullen, J., Doyle, J. D., Hodur, R., Ogston, A., Book, J. W., Perkins, H., and Signell, R.: Coupled ocean-atmosphere nested modelling of the Adriatic Sea during winter and spring 2001, J. Geophys. Res., 108, 3320, https://doi.org/10.1029/2003JC001780, 2003.
Pytharoulis, I.: Analysis of a Mediterranean tropical-like cyclone and its sensitivity to the sea surface temperatures, Atmos. Res., 208, 167–179, https://doi.org/10.1016/j.atmosres.2017.08.009, 2018.
Rainaud, R., Lebeaupin Brossier, C., Ducrocq, V., Giordani, H., Nuret, M., Fourrié, N., Bouin, M., Taupier-Letage, I., and Legain, D.: Characterization of air–sea exchanges over the Western Mediterranean Sea during HyMeX SOP1 using the AROME–WMED model, Q. J. Roy. Meteor. Soc., 142, 173–187, https://doi.org/10.1002/qj.2480, 2016.
Ren, X., Perrie, W., Long, Z., and Gyakum, J.: Atmosphere–Ocean Coupled Dynamics of Cyclones in the Midlatitudes, Mon. Weather Rev., 132, 2432–2451, https://doi.org/10.1175/1520-0493(2004)132<2432:ACDOCI>2.0.CO;2, 2004.
Renard, B., Kavetski, D., Kuczera, G., Thyer, M., and Franks, S. W.: Understanding predictive uncertainty in hydrologic modelling: The challenge of identifying input and structural errors, Water Resour. Res., 46, W05521, https://doi.org/10.1029/2009WR008328, 2010.
Ricchi, A., Miglietta, M. M., Bonaldo, D., Cioni, G., Rizza, U., and Carniel, S.: Multi-Physics Ensemble versus Atmosphere–Ocean Coupled Model Simulations for a Tropical-Like Cyclone in the Mediterranean Sea, Atmosphere, 10, 202, https://doi.org/10.3390/atmos10040202, 2019.
Robinson, I., Piolle, J. F., Leborgne, P., Poulter, D., Donlon, C., and Arino, O.: Widening the application of AATSR SST data to operational tasks through the Medspiration Service, Remote Sens. Environ., 116, 126–139, https://doi.org/10.1016/j.rse.2010.12.019, 2012.
Romaniello, V., Oddo, P., Tonani, M., Torrisi, L., Grandi, A., and Pinardi, N.: Impact of Sea Surface Temperature on COSMO Forecasts of a Medicane over the Western Mediterranean Sea, Journal of earth science and engineering, 5, 338–348, https://doi.org/10.17265/2159-581X/2015.06.002, 2015.
Ruti, P. M., Somot, S., Giorgi, F., Dubois, C., Flaounas, E., Obermann, A., Dell'Aquila, A., Pisacane, G., Harzallah, A., Lombardi, E., Ahrens, B., Akhtar, N., Alias, A., Arsouze, T., Aznar, R., Bastin, S., Bartholy, J., Béranger, K., Beuvier, J., Bouffies-Cloché, S., Brauch, J., Cabos, W., Calmanti, S., Calvet, J., Carillo, A., Conte, D., Coppola, E., Djurdjevic, V., Drobinski, P., Elizalde-Arellano, A., Gaertner, M., Galàn, P., Gallardo, C., Gualdi, S., Goncalves, M., Jorba, O., Jordà, G., L'Heveder, B., Lebeaupin-Brossier, C., Li, L., Liguori, G., Lionello, P., Maciàs, D., Nabat, P., Önol, B., Raikovic, B., Ramage, K., Sevault, F., Sannino, G., Struglia, M. V., Sanna, A., Torma, C., and Vervatis, V.: Med-CORDEX Initiative for Mediterranean Climate Studies, B. Am. Meteorol. Soc., 97, 1187–1208, https://doi.org/10.1175/BAMS-D-14-00176.1, 2016.
Salas, F. R., Somos-Valenzuela, M. A., Dugger, A., Maidment, D. R., Gochis, D. J., David, C. H., Yu, W., Ding, D., Clark, E. P., and Noman, N.: Towards Real-Time Continental Scale Streamflow Simulation in Continuous and Discrete Space, J. Am. Water Resour. As., 54, 7–27, https://doi.org/10.1111/1752-1688.12586, 2018.
Senatore, A., Mendicino, G., Knoche, H. R., and Kunstmann, H.: Sensitivity of Modelled Precipitation to Sea Surface Temperature in Regions with Complex Topography and Coastlines: A Case Study for the Mediterranean, J. Hydrometeorol., 15, 2370–2396, https://doi.org/10.1175/JHM-D-13-089.1, 2014.
Senatore, A., Mendicino, G., Gochis, D. J., Yu, W., Yates, D. N., and Kunstmann, H.: Fully coupled atmosphere-hydrology simulations for the central Mediterranean: Impact of enhanced hydrological parameterization for short and long time scales, J. Adv. Model. Earth Sy., 7, 1693–1715, https://doi.org/10.1002/2015MS000510, 2015.
Silvestro, F., Rebora, N., Cummings, G., and Ferraris, L.: Dealing with flash floods using a hydrological nowcasting chain, J. Flood Risk Manag., 10, 446–462, https://doi.org/10.1111/jfr3.12161, 2017.
Sinclair, S. and Pegram, G.: Combining radar and rain gauge rainfall estimates using conditional merging, Atmos. Sci. Lett., 6, 19–22, https://doi.org/10.1002/asl.85, 2005.
Skamarock, W. C., Klemp, J. B., Dudhia, J., Gill, D. O., Barker, D. M., Duda, M. G., Huang, X.-Y., Wang, W., and Powers, J. G.: A Description of the Advanced Research WRF Version 3, NCAR Tech. Note NCAR/TN-475+STR, 113 pp., https://doi.org/10.5065/D68S4MVH, 2008.
Somot, S., Ruti, P., Ahrens, B. Coppola, E., Jordà G., Sannino, G., and Solmon F.: Editorial for the Med-CORDEX special issue, Clim. Dynam., 51, 771–777, https://doi.org/10.1007/s00382-018-4325-x, 2018.
Stocchi, P. and Davolio, S.: Intense air-sea exchanges and heavy orographic precipitation over Italy: The role of Adriatic sea surface temperature uncertainty, Atmos. Res., 196, 62–82, https://doi.org/10.1016/j.atmosres.2017.06.004, 2017.
Sun, J., Wang, H., Tong, W., Zhang, Y., Lin, C., and Xu, D.: Comparison of the Impacts of Momentum Control Variables on High-Resolution Variational Data Assimilation and Precipitation Forecasting, Mon. Weather Rev., 144, 149–169, https://doi.org/10.1175/MWR-D-14-00205.1, 2016.
Tao, J., Wu, D., Gourley, J., Zhang, S. Q., Crow, W., Peters-Lidard, C., and Barros, A. P.: Operational hydrological forecasting during the IPHEx-IOP campaign – Meet the challenge, J. Hydrol., 541, 434–456, https://doi.org/10.1016/j.jhydrol.2016.02.019, 2016.
Termonia, P., Deckmyn, A., and Hamdi, R.: Study of the lateral boundary condition temporal resolution problem and a proposed solution by means of boundary error restarts, Mon. Weather Rev., 137, 3551–3566, https://doi.org/10.1175/2009MWR2964.1, 2009.
Tewari, M., Chen, F., Wang, W., Dudhia, J., LeMone, M. A., Mitchell, K., Ek, M., Gayno, G., Wegiel, J., and Cuenca, R. H.: Implementation and verification of the unified NOAH land surface model in the WRF model. 20th conference on weather analysis and forecasting/16th conference on numerical weather prediction, 11–15, 2004.
Thiruvengadam, P., Indu, J., and Ghosh, S.: Assimilation of Doppler Weather Radar data with a regional WRF-3DVAR system: Impact of control variables on forecasts of a heavy rainfall case, Adv. Water Resour., 126, 24–39, https://doi.org/10.1016/j.advwatres.2019.02.004, 2019.
Verri, G., Pinardi, N., Gochis, D., Tribbia, J., Navarra, A., Coppini, G., and Vukicevic, T.: A meteo-hydrological modelling system for the reconstruction of river runoff: the case of the Ofanto river catchment, Nat. Hazards Earth Syst. Sci., 17, 1741–1761, https://doi.org/10.5194/nhess-17-1741-2017, 2017.
Wilks, S. D.: Statistical Methods in the Atmospheric Sciences, International Geophysics Series, 59, chap. XI, https://doi.org/10.1016/S0074-6142(06)80036-7, 2006.
Yucel, I., Onen, A., Yilmaz, K., and Gochis, D. J.: Calibration and Evaluation of a Flood Forecasting System: Utility of Numerical Weather Prediction Model, Data Assimilation and Satellite-Based Rainfall, J. Hydrol., 523, 49–66, https://doi.org/10.1016/j.jhydrol.2015.01.042, 2015.
Zeng, X. and Beljaars, A.: A prognostic scheme of sea surface skin temperature for modelling and data assimilation, Geophys. Res. Lett., 32, L14605, https://doi.org/10.1029/2005GL023030, 2005.