the Creative Commons Attribution 4.0 License.
the Creative Commons Attribution 4.0 License.
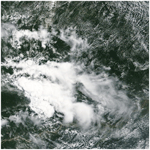
Land–atmosphere interactions in the tropics – a review
Pierre Gentine
Adam Massmann
Benjamin R. Lintner
Sayed Hamed Alemohammad
Rong Fu
Julia K. Green
Daniel Kennedy
Jordi Vilà-Guerau de Arellano
The continental tropics play a leading role in the terrestrial energy, water, and carbon cycles. Land–atmosphere interactions are integral in the regulation of these fluxes across multiple spatial and temporal scales over tropical continents. We review here some of the important characteristics of tropical continental climates and how land–atmosphere interactions regulate them. Along with a wide range of climates, the tropics manifest a diverse array of land–atmosphere interactions. Broadly speaking, in tropical rainforest climates, light and energy are typically more limiting than precipitation and water supply for photosynthesis and evapotranspiration (ET), whereas in savanna and semi-arid climates, water is the critical regulator of surface fluxes and land–atmosphere interactions. We discuss the impact of the land surface, how it affects shallow and deep clouds, and how these clouds in turn can feed back to the surface by modulating surface radiation and precipitation. Some results from recent research suggest that shallow clouds may be especially critical to land–atmosphere interactions. On the other hand, the impact of land-surface conditions on deep convection appears to occur over larger, nonlocal scales and may be a more relevant land–atmosphere feedback mechanism in transitional dry-to-wet regions and climate regimes.
Please read the corrigendum first before continuing.
-
Notice on corrigendum
The requested paper has a corresponding corrigendum published. Please read the corrigendum first before downloading the article.
-
Article
(8027 KB)
-
The requested paper has a corresponding corrigendum published. Please read the corrigendum first before downloading the article.
The tropics play a substantial role in regulating the global hydrologic and carbon cycles. Tropical rainforests are one of the main terrestrial carbon sinks (Nakicenovic and Swart, 2000; Friedlingstein et al., 2006), but their projected responses to a warming climate remain unclear because of uncertainties associated with the representation of abiotic and biotic processes in models as well as confounding factors such as deforestation and changes in land use and land cover (Wang et al., 2009; Davidson et al., 2012; Fu et al., 2013; Saatchi et al., 2013; Hilker et al., 2014; Boisier et al., 2015; Doughty et al., 2015; Gatti et al., 2015; Knox et al., 2015; Saleska et al., 2016). The ecosystems of tropical monsoonal and seasonal wet–dry climates are also important contributors to the global carbon cycle, especially with respect to the interannual variability in the tropical terrestrial carbon sink (Poulter et al., 2014; Jung et al., 2017; Green et al., 2019).
Some regions of the tropics have been further identified as hotspots in which land–atmosphere interactions modify the climate (Dirmeyer, 2011; Koster et al., 2011; Green et al., 2017) locally, i.e., at horizontal scales on the order of a few boundary layer heights, regionally, at scales up to a few hundred kilometers, or at larger scales, over several thousand kilometers, through coupling between the surface and the overlying atmosphere (Lintner and Neelin, 2009). These interactions may in turn dramatically affect the future state of rainforests (Cox et al., 2004).
While tropical land–atmosphere interactions are often examined through the lens of coupling between land-surface states (e.g., soil moisture) and rainfall, other aspects of the coupling are also important. For example, even under nonprecipitating conditions, surface radiation, temperature, and vapor pressure deficit (VPD) may be altered (Lawton et al., 2001; Pielke et al., 2016; Green et al., 2017) through coupling with clouds, aerosols, and shallow (nonprecipitating) convection (Avissar and Nobre, 2002; Medvigy et al., 2011; Seneviratne, 2013; Cook et al., 2014; Guillod et al., 2015; Krakauer et al., 2016; Martin et al., 2016, 2017; Green et al., 2017; Khanna et al., 2017; Thiery et al., 2017; Vogel et al., 2017). In addition, tropical forests can exhibit important variations in canopy photosynthetic capacity with new leaves (Saleska et al., 2003, 2016). These variations can further feed back onto the atmosphere on seasonal timescales (Green et al., 2017). It is clear that the tropical energy, water, and carbon cycles cannot be understood in isolation; rather, the interactions among these cycles are essential. For example, knowledge of such interactions must be taken into account in ascertaining whether the terrestrial tropics will act as a future carbon sink or source (Zhang et al., 2015; Swann et al., 2015).
The two-way interactions that occur between the land surface and overlying atmosphere represent one of the more uncertain aspects of the terrestrial climate system, particularly in the tropics (Betts and Silva Dias, 2010). While the land surface is widely recognized as integral to the occurrence of important tropical climate phenomena such as monsoons (Zeng and Neelin, 1999; Zeng et al., 1999), isolating and quantifying its precise role remains elusive. Indeed, such efforts have frequently been hampered by the paucity of observational data, not to mention the complex and multiple pathways through which land–atmosphere interactions can take place.
Several notable field campaigns have been conducted in the tropics with the purpose of advancing knowledge of land–atmosphere interactions. One of the most well-known campaigns was the Large-Scale Biosphere–Atmosphere Experiment in Amazonia (LBA; Avissar et al., 2002; Keller et al., 2004), which aimed at refining our understanding of climatological, hydrological, biogeochemical, and ecological processes of the Amazon and their linkages in addition to the anthropogenic impacts (e.g., land-use land cover changes and deforestation) on these. Among many other topics, LBA generated fundamental insights on the structure of the tropical atmosphere, processes generating precipitation, and the seasonal variability in surface turbulent fluxes in tropical rainforests (Avissar and Nobre, 2002; Betts et al., 2002; Laurent et al., 2002; Machado and Laurent, 2002; Acevedo et al., 2004; Khairoutdinov and Randall, 2006; Fitzjarrald et al., 1988; Juárez et al., 2007; Restrepo-Coupe et al., 2013). One thrust of LBA research sought to isolate the effect of deforestation on precipitation both in a local context as well as remotely via teleconnections (Avissar et al., 2002; Werth and Avissar, 2002). Such research has pointed to deforestation decreasing precipitation, albeit with uncertain magnitude and dependence on the spatial scales involved. Even now, two decades after the inception of LBA, the relationship between tropical deforestation and precipitation remains uncertain despite progress with respect to key processes such as vegetation access to deep water in the dry season (Oliveira et al., 2005) and modulation of energy availability for photosynthesis via cloud cover (Betts and Silva Dias, 2010).
Another field campaign, the African Monsoon Multidisciplinary Analysis (AMMA) campaign, focused on the West African monsoon system, especially the Sahel transition zone (Redelsperger et al., 2006; Boone et al., 2009b). Building on previous field work in the region (e.g., HAPEX-Sahel; Gourtorbe et al. 1994), AMMA generated fundamental understanding of mesoscale convective systems and surface processes (Lebel et al., 2009; Taylor et al., 2009; Boone et al., 2009a; Lohou et al., 2010; Couvreux et al., 2011). More recently, the 2014–2015 Green Ocean Amazon (GOAmazon) campaign (Martin et al., 2016) sought to quantify the impact of atmospheric composition and aerosols under clean and polluted conditions on cloud formation and radiation over the Amazon Basin as well as on shallow to deep convection development (Anber et al., 2015a; Tang et al., 2016; Giangrande et al., 2017).
The remainder of this review article is organized as follows. In Sects. 2–4, we introduce some fundamental considerations of the climate system components necessary for understanding tropical land–atmosphere interactions, including convection, clouds, and rainfall (Sect. 2); surface turbulent fluxes (Sect. 3); and vegetation and ecosystem processes (Sect. 4). We then synthesize prior work on tropical land–atmosphere interactions from both local and nonlocal perspectives (Sect. 5). We close this review (Sect. 6) by highlighting what we view as the outstanding issues, challenges, and knowledge gaps for tropical land–atmosphere interactions. For example, we argue that shallow cloud feedback and its impact on radiation have received too little attention compared to precipitation feedback, in rainforests especially.
The net radiative heating of the atmosphere in the global tropics – arising from the top-of-the-atmosphere imbalance of net incoming solar (shortwave) radiation exceeding outgoing terrestrial (longwave) radiation – leads to one of the defining hallmarks of the tropics, namely very high rain rates. This is not to say, of course, that rainfall in the tropics is high everywhere or at all times, as climates within the tropics can be both wet and dry. Indeed, many of earth's desert regions are found on the margins of the tropics, and apart from deserts, parts of the tropics may experience very dry conditions seasonally. In this review, we will exclude consideration of deserts and focus on the humid tropics.
2.1 Shallow vs. deep convection
The distinction between shallow and deep convection remains imprecise, as these have been regarded as both fundamentally distinct or as a continuum in both observations and model convection parameterizations (Khairoutdinov and Randall, 2006; Bretherton and Park, 2009; Park and Bretherton, 2009; Rio et al., 2009; Wu et al., 2009; Del Genio and Wu, 2010; Hohenegger and Bretherton, 2011; Böing et al., 2012; D'Andrea et al., 2014; Rochetin et al., 2014b). We will loosely refer to shallow convection as convection confined below the freezing level (typically less than 3 km deep) and comprising nonprecipitating clouds small characteristic motion scales (typically less than a kilometer in the horizontal direction).
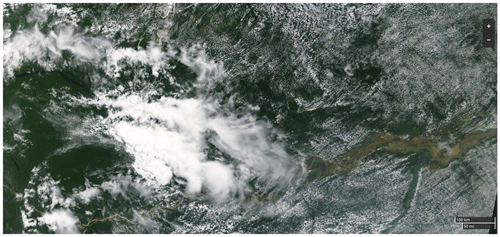
Figure 1Snapshot of cloud cover over the Amazon Basin on 25 September 2018 (courtesy NASA Earth Observatory, MODIS visible bands). Small clouds are shallow convective clouds, highlighting surface Bowen ratio changes between the river and the forest. On the left, the deep convective cells do not follow the surface heterogeneity (and are much larger in scale). Note the cold-pool bow effect on the bottom left corner, with no clouds within the cold pools and clouds on the edge of the cold pool.
An important point is that shallow convection is frequently generated by thermals rooted in the boundary layer and is thus ultimately related to surface sensible heat flux (H) and latent heat flux (LE) and their partitioning (Gentine et al., 2013a, b; Vilà‐Guerau de Arellano et al., 2014; Tawfik et al., 2014, 2015a, b). Within the Amazon, shallow convection and associated clouds frequently occur over the vegetated surface, while over cooler and more humid river basins, shallow clouds are virtually absent (Gentine et al., 2013a; Rieck et al., 2014; 2015; see also Fig. 1). In addition, shallow convection is strongly influenced by the diurnal cycle of surface radiation and surface turbulent heat fluxes (Gentine et al., 2013a, b; Vilà‐Guerau de Arellano et al., 2014).
On the other hand, we use the term deep convection in association with deep, precipitating clouds. Deep convection may be triggered by a suite of thermodynamic or dynamic processes, including boundary layer thermals (D'Andrea et al., 2014; Guillod et al., 2014; Rochetin et al., 2014a; 2014b; Anber et al., 2015a), radiative destabilization (Anber et al., 2015b), cold pools (cold density currents due to rain evaporation that cools the air within precipitating downdrafts; Engerer et al., 2008; Del Genio and Wu, 2010; Böing et al., 2012; Feng et al., 2015; Torri et al., 2015; Gentine et al., 2016; Heever, 2016; Drager and van den Heever, 2017), forced vertical motions such as via mesoscale and large-scale circulations (Werth and Avissar, 2002; Roy et al., 2003), or propagating tropical wave activity (Kuang, 2008, 2010). As such, deep convection may be viewed as being less dependent on the surface state compared to shallow convection.
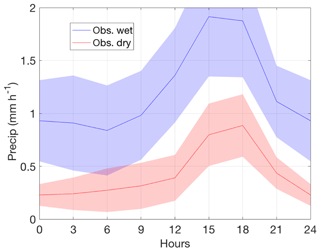
Figure 2Diurnal cycle in local time of dry-season (red) and wet-season (blue) observations of precipitation (Precip) at K34, near Manaus, along with their standard deviation averaged across years 2010–2014.
Over the central Amazon a large fraction of wet-season precipitation occurs during the nighttime (Fig. 2). Moreover, during the daytime in both the dry and the wet seasons, the diurnal cycle reflects not only locally surface-triggered deep convection (Khairoutdinov and Randall, 2006; Ghate and Kollias, 2016) but also propagates mesoscale convective systems and squall lines throughout the Amazon Basin; Ghate and Kollias, 2016; Rickenbach et al., 2002). However, during the dry season, precipitation occurs more frequently with the “popcorn-type” deep convection that is more locally triggered and thus directly related to the state of the land surface (Ghate and Kollias, 2016; see an example at https://youtu.be/c2-iquZziPU, last access: 20 September 2019). While shallow convection does not produce much rainfall, it exerts significant influence on deep convection through its control on surface radiative fluxes and on transport of moisture into the lower troposphere. More discussion will be given in Sect. 4.2.
2.2 Considerations for modeling tropical clouds, convection, and rainfall
Current-generation climate models struggle to represent both shallow and deep convection over continents and their transitions (Guichard et al., 2004; Bechtold et al., 2013; Yin et al., 2013; D'Andrea et al., 2014; Couvreux et al., 2015), especially in the tropics, as they exhibit substantial errors in the phasing and intensity of both the diurnal and seasonal cycles of convection (Bechtold et al., 2013) as well as biases in the climatological distribution of rainfall over land. For example, over the Amazon, many climate models underestimate surface precipitation, evapotranspiration (ET), and specific humidity (Yin et al., 2013), with the dry bias in moisture extending upwards into the lower free troposphere (Lintner et al., 2017). Such biases may reflect deficiencies or errors in how convection is represented in models (Yano and Plant, 2012; Stevens and Bony, 2013; Bechtold et al., 2014). Indeed, in current-generation climate models, cloud processes occur at scales smaller than resolved grid-scale prognostic variables and therefore need to be parameterized, i.e., represented as a function of the resolved-scale variables. This is important, as it means that climate models do not explicitly represent the small-scale convective physics of the climate system.
Cloud-resolving models (CRMs) that include explicit convection at scales of ∼1 km alleviate many of the biases evident in coarser-scale, parameterized convection climate models, especially in terms of the diurnal cycle of convection or the sign and magnitude of the feedbacks between deep convection and surface evaporative fraction (Grabowski and Smolarkiewicz, 1999; Taylor et al., 2013; Anber et al., 2015a). Nonetheless, due to convective wave coupling in the tropics, a simple prescription of lateral boundary conditions in small-domain CRMs may be problematic, as the convective scales ultimately interact with and are coupled to planetary scales. With a sufficiently large domain and fine-enough resolution, coupling between the convective scales and planetary scales may be explicitly resolved, but simulations of this nature are likely to be computationally too expensive for many applications. However, techniques exist to represent the effect of large-scale dynamics on the convective scales, which, when combined with cloud-resolving simulations, yield powerful tools for understanding land–atmosphere interactions in the tropics, as we elaborate further below.
A major component of land–atmosphere interactions considered is related to surface turbulent fluxes and associated momentum, energy, water, and trace gases exchanges between the land surface and atmosphere (Goulden et al., 2004; Fisher et al., 2009; Restrepo-Coupe et al., 2013). Surface turbulent flux measurements are usually obtained from eddy-covariance methods, typically located above the canopy (Baldocchi et al., 2001). Observing turbulent fluxes is challenging in tropical environments given logistics, cost, maintenance, and harsh environmental factors such as intense rainfall, high wind, and relative humidity, which impact sensors (Campos et al., 2009; Da Rocha et al., 2009; Restrepo-Coupe et al., 2013; Zahn et al., 2016; Chor et al., 2017; Gerken et al., 2017). In light of these challenges, it is perhaps not surprising that even the best estimates of surface turbulent fluxes manifest large uncertainties (Mueller et al., 2011).
Apart from site-level measurements, which are limited to a small number of locations around the tropics, remote-sensing observations can provide indirect information about surface turbulent fluxes and other relevant quantities over tropical land regions. Remote-sensing observations are useful for generalizing and comparing fluxes across the tropics even if they are not as direct as site-level measurements. Yet, there are considerable uncertainties in remote sensing and reanalysis estimates of rainfall (Washington et al., 2013; Levy et al., 2017), radiation (Jimenez et al., 2011), and surface turbulent fluxes (Jung et al., 2013; Alemohammad et al., 2017), especially in terms of upscaling point observations to larger areas, as those measurements are fundamentally indirect.
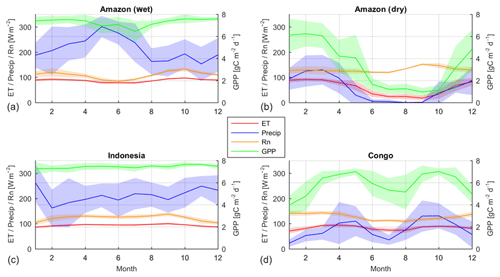
Figure 3Seasonal variations in evapotranspiration (ET) from WECANN, precipitation (Precip) based on GPCP in units of energy (W m−2; by multiplying it by the latent heat of vaporization), net radiation (Rn) from CERES, and gross primary production (GPP) based on WECANN informed by solar-induced fluorescence (SIF) over the wet part of the Amazon (a), over the Savanna region of Brazil (b), over Indonesia (c), and over the Congo Basin (d).
While direct, satellite-based retrievals of carbon (e.g., gross primary production – GPP) and water fluxes would be most suitable for the study of tropical land–atmosphere interactions, such retrievals are beyond current remote-sensing capabilities. However, some recent work demonstrates that existing satellite observations, especially solar-induced fluorescence (SIF), may be leveraged to remotely assess surface turbulent fluxes in the tropics. In contrast to the normalized difference vegetation index (NDVI) or many other vegetation indices which are indirect byproducts of photosynthesis (Morton et al., 2014), SIF (at the leaf scale) is directly related to the ecosystem-scale photosynthesis rate, providing important information on the impact of stressors on photosynthesis, and is available from existing remote-sensing platforms (Frankenberg et al., 2011, 2012, 2014; Joiner et al., 2011, 2013; Guanter et al., 2014; Lee et al., 2015; Duveiller and Cescatti, 2016; Liu et al., 2017; Thum et al., 2017; Norton et al., 2018). SIF is thus an important indicator of the rates of photosynthesis and transpiration through stomatal (small pores at the leaf surface) regulation (Alemohammad et al., 2017; Pagán et al., 2019). Indeed, during photosynthesis, plants take up CO2 from the atmosphere while releasing water to the atmosphere through stomata. We note that recent developments in observations of SIF seem to indicate that the major fraction of the SIF signal might be related to chlorophyll photosynthetically active radiation and that changes in SIF yield (equivalent to light use efficiency) may account for only a small fraction of the observed SIF signal (Du et al., 2017). This is still an open topic for better understanding what is actually observed by SIF remote sensing.
Alemohammad et al. (2017) applied a machine-learning algorithm based on remotely sensed SIF, called WECANN (Water, Energy, and Carbon Artificial Neural Networks), to derive surface turbulent fluxes. WECANN exhibits reasonable interannual variability, and its seasonality is constrained by the use of SIF (Alemohammad et al., 2017); yet like any other products it is not a direct observation of the fluxes, which are only available at sparse tower observations. WECANN performs well compared to eddy-covariance observations and has less uncertainty compared to many other retrievals (see Alemohammad et al., 2017).
Given the paucity of flux towers and associated surface flux measurements across the tropics, we use WECANN to calculate surface flux climatologies across the continental tropics. WECANN has been validated against available flux tower data and outperforms other products in terms of reproducing both the seasonality and interannual variability (Alemohammad et al., 2017). While remote-sensing retrievals are not perfect and cannot be considered the truth, they do provide spatially extensive data coverage, including regions with sparse (or no) site-level measurements (e.g., Congo Basin). In what follows, we present climatologies of ET and GPP and compare these against precipitation (based on GPCP 1DD V1.2; Huffman et al., 2001) and net radiation (based on CERES SYN; Kato et al., 2013) in order to understand the typical seasonal cycles of those energy, water, and carbon fluxes across the continental tropics.
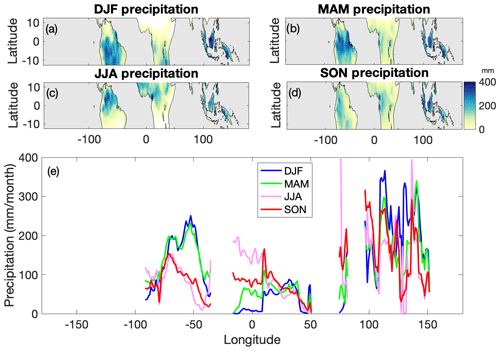
Figure 4Seasonality of precipitation based on GPCP in the tropics in December–January–February (a), March–April–May (b), June–July–August (c), and September–October–November (SON) and its latitudinal average (e).
We first focus on the main tropical rainforests and the northeastern savanna (Cerrado) region of Brazil (Fig. 3). In the wetter part of the Amazon, net radiation, Rn, peaks in the dry season (August to November; Fig. 3) when precipitation (Fig. 4) and cloud cover – especially shallow cloud cover, including fog – are reduced, (Anber et al., 2015a). As a result of reduced dry-season cloud cover, incident surface solar radiation increases, and both GPP (Fig. 5) and ET (Fig. 6) increase in the dry season (Fig. 3). As discussed further in the next section, the forest in the climatologically wetter Amazon is primarily light limited, while water stress there is moderate in the dry season. The seasonal cycle is more pronounced for GPP than for ET (Fig. 3); canopy photosynthetic regeneration (Saleska et al., 2003, 2016) is an important factor affecting the seasonal cycle of GPP in rainforests, potentially increasing the maximum rate of GPP in the dry season. In addition, canopy evaporation (of intercepted rain) comprises a large fraction of total ET in the wet season (Scott et al., 1997; Oleson et al., 2008; Miralles et al., 2010; Sutanto et al., 2012; van Dijk et al., 2015; Andreasen et al., 2017) and partly compensates for reduced transpiration in the wet season. In fact, because of this compensation, the wettest parts of the Amazon exhibit weak ET seasonality. On the other hand, most land-surface models exaggerate water stress in the Amazon (Powell et al., 2013) and typically exhibit much lower rates of ET and GPP in the dry season and simulate opposite seasonality of net ecosystem exchange compared to observations (de Gonçalves et al., 2013; Alemohammad et al., 2017). This exaggerated water stress results from incorrect access to deep soil water, whether due to limited groundwater representation (Maxwell and Condon, 2016) because of the functional relationship of the water stress representation which does not obey physical constraints (such as flow down potential gradients as in plant hydraulics models; Kennedy et al., 2019) or simply because their assumed rooting depth is too shallow (Fan et al., 2017).
In contrast to the ever-wet western and central Amazon, over the Cerrado region of northeastern Brazil, the seasonal cycles of Rn, precipitation, GPP, and ET are much more pronounced, with a marked dry season (Fig. 3). The seasonal cycle of GPP tracks precipitation, and water stress, exhibiting a strong increase during the wet season. Similarly, ET increases sharply in the wet season and then decreases more slowly than precipitation in the dry region (Fig. 3). Conversely, net radiation increases sharply during the dry season. This region clearly exhibits a strong water stress response.
Turning to the Maritime Continent, rainfall is intense throughout the year and seasonality is modest, with a short peak in November to January (Fig. 3). Much of the seasonal cycle is attributable to monsoon circulations, which are strongly influenced by topography and the land- and ocean-surface thermal contrast (Chang et al., 2005). The topography and the distribution of island land masses lead to strong local variability (Fig. 4e), and pronounced diurnal cycles in convection are evident (Nitta, 1987; Hamada et al., 2008). Additionally, the Madden–Julian Oscillation, an important mode of climate variability in the tropical Indo-Pacific with a life cycle of 30–90 d, strongly impacts rainfall on intraseasonal timescales (Hidayat and Kizu, 2009). Convective activity in the region also regulates the East Asian monsoon (Huang and Sun, 1992). The region is also influenced by topographic effects and land–sea-breeze interactions, which may impart considerable regional heterogeneity. Given the relatively constant regionally averaged precipitation with regular convection occurring over course of the annual cycle, ET and GPP remain near steady throughout the entire year in this mostly light-limited environment (Fig. 3).
The Congo Basin exhibits two rainy seasons (Fig. 3), with peaks in March–April–May and September–October–November, related to seasonal changes in moisture convergence associated with the African easterly jet and intertropical convergence zone (ITCZ) over the Atlantic (Washington et al., 2013). Throughout the year, monthly mean precipitation is much less than that observed over the Amazon or Indonesia. The seasonality of GPP and, to a lesser extent, ET, tracks that of precipitation, with substantial decreases during the June to August dry season and even more pronounced reduction during the December to February period. This seasonality in GPP and ET (Fig. 3) suggests that the Congo Basin should exhibit substantially more water stress during dry seasons compared to the Amazon or Indonesian rainforests (Guan and Liang, 2015).
Integrated over the entire tropical latitudinal band, precipitation is highest in DJF and MAM, when the wet season extends over most of the Amazon and adjacent savanna regions (Fig. 4). This seasonal cycle of tropical-mean precipitation largely determines the seasonal cycle of GPP. GPP peaks during the wet season in South America, as GPP is highest in the savanna regions, while GPP over the rainforest exhibits fewer seasonal variations (Fig. 6). The seasonal pattern of ET resembles GPP (Fig. 6). Indeed, the seasonality in ET reflects the combined influences of (1) the seasonality of water availability in drier, water-limited regions, (2) the seasonality of surface radiation in the wetter, more energy-limited portion of the Amazon, and (3) changes in photosynthetic capacity throughout the year. The seasonal cycle of sensible heat flux (Fig. 7) largely follows water stress, especially in the rainforest where radiation remains high throughout the year, with an increase during the dry season. Water stress is further apparent in the evaporative fraction, EF, the ratio of latent heat flux to the sum of latent and sensible heat fluxes (Fig. 8). Tropically averaged EF does not evolve much over the year; the modest seasonality may be understood in terms of variation in the latitudinal peak in radiation and compensation of decreased canopy interception by transpiration (because of increased net surface radiation) in the dry season. However, in transitional and dry regions, EF exhibits much more seasonal variation. The surface moist static energy flux, H + LE, shows some slight variations in SON and JJA but otherwise remains relatively steady across longitudes because of the compensation between the increased H and reduced ET in the dry season. In the dry-to-wet transition, SON, moist static energy flux exhibits an interesting peak at about −60 longitude (Fig. 9) through the combined increase in radiation, due to reduced cloudiness, inducing higher sensible heat flux and maintaining high ET rates.
Over tropical Africa, the precipitation is highest in JJAS during the wet phase of the West African monsoon, with a secondary maximum in DJF corresponding to the southern African monsoon (Fig. 4). Similarly the latitudinally averaged GPP and ET increase during the West African monsoon (Figs. 5 and 6), accompanied by a strong decrease in sensible heat flux (Fig. 7). In DJF the southern African monsoon displays increased water flux (Fig. 6) and photosynthesis tracking of the increased rainfall (Fig. 4). The Congo rainforest clearly exhibits two brief rainy seasons (Figs. 3 and 8), with peaks in March–April–May and September–October–November (Fig. 3), and displays substantial water stress and strong reduction in EF to values below 0.6 during the dry season (Fig. 8).
We cannot understand tropical land–atmosphere interactions, not to mention the basic features of terrestrial tropical climate, without consideration of vegetation and ecosystem processes. Indeed, over land, what is viewed as the tropics has traditionally been defined with vegetation and ecosystems implicitly considered, as in the Köppen climate classification scheme (Köppen, 1884). Under this scheme, the terrestrial tropics are divided into three main groups – tropical rainforest, tropical monsoon, and tropical wet and dry (or savanna) – all of which are characterized by annual mean temperatures exceeding 18 ∘C but which differ in terms of precipitation amount and seasonality.
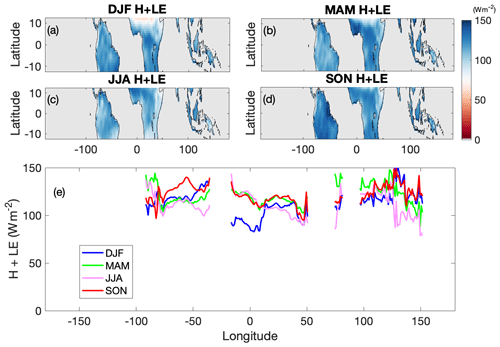
Figure 9Same as Fig. 4 but for sea-level surface moist static energy flux, the sum of sensible heat flux (H) and latent heat flux (LE).
One outstanding challenge in simulating tropical land regions is determining why most contemporary land-surface models incorrectly represent the wettest rainforest GPP and ET rates, their seasonal cycles, and how they relate to water stress. In the wettest tropical forests, such as the western portion of the Amazon or Indonesia, energy and light limit the rates of ET and GPP. It is thus reasonable to conclude that soil moisture and water stress should have minor effects in such regions and, consequently, that precipitation variability should not matter much. In fact, sharp vertical gradients in the canopy exist (as well as at the surface of the soil in the dry season) in terms of light and water availability along with nutrient allocation (Fig. 10). Understory species receive only a small amount of mostly diffuse light. However, water is typically not limiting for low-canopy species, as relative humidity is high and VPD is low, leading to low stress on understory conductance (Leuning, 1995; Leuning et al., 1995; Wang and Leuning, 1998; Heroult et al., 2013).
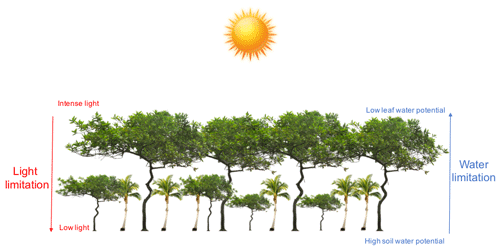
Figure 10Schematic showing the vertical structure of light and water limitations in a tropical forest.
On the other hand, tall-canopy species receive a large amount of radiation, especially in the dry season, causing sunlit leaf warming and higher leaf VPD (e.g., Penman-Monteith equation) that lead to heat and water stress (Jardine et al., 2014). Leaf and xylem water status are regulated by the relative demand of sap from transpiration, which depends on leaf VPD. It also depends on sap supply to the leaves, the rate of which is controlled by the xylem conductivity and which is high for tall tropical rainforest trees (Liu et al., 2019). This conductivity is reduced by cavitation in the xylem (formulation of air bubbles blocking the ascent of sap flow from the roots to the leaves; Martinez-Vilalta et al., 2014; Martinez-Vilalta and Garcia-Forner, 2016). To avoid leaf desiccation and xylem cavitation, stomatal closure is usually observed during peak daytime sunlight hours in rainforest top-canopy species (Brodribb, 2003; Pons and Welschen, 2003; Zhang et al., 2013). This limits the risk of leaf desiccation or xylem cavitation (Fig. 11) in that usually efficient xylem. This type of behavior with strong stomatal regulation appears to be typical in the tropical rainforests (Fisher et al., 2006; Konings and Gentine, 2017) even though this appears to contradict results from isotopic measurements showing that stomata remain relatively open (Ometto et al., 2006). On seasonal timescales, this is modulated by the change in photosynthetic capacity of the canopy due to leaf flush and canopy regeneration (Saleska et al., 2003, 2006). More work is needed to understand stomatal and canopy regulation of tropical rainforests in response to stressors, especially given the importance of rainforests in the global carbon cycle.
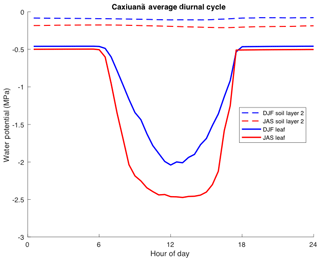
Figure 11Climatology of the diurnal cycle of leaf water potential and topsoil water potential in the dry and wet seasons in Caxiuanã, Brazil, simulated by the Community Land Model (CLM) with plant hydraulics.
In tall-canopy species the flow in the xylem from the roots may be limited, as the xylem hydraulic conductivity is inversely proportional to height, though this is partially compensated by more efficient xylem (higher specific conductivity; Liu et al., 2019). However, higher evaporative demand in the dry season and/or under anomalously dry conditions can only be partially be mitigated by the more efficient xylem and by the plant internal storage; stomatal shutdown may therefore be inevitable in avoiding desiccation and xylem cavitation (Fig. 11; Dawson, 1993; Phillips et al., 1997, 2004, 2008; Lee et al., 2005; Oliveira et al., 2005; Scholz et al., 2011; Zeppel et al., 2014; Konings and Gentine, 2017). In summary, water stress in tropical rainforest canopy species may not primarily be due to soil water stress but rather to atmospheric demand or a combination of soil-moisture stress and atmospheric demand. The reduction of leaf and xylem water potentials increase stress in the soil–plant continuum. Radiation, temperature, and VPD are therefore essential in understanding tropical wet-forest dryness response.
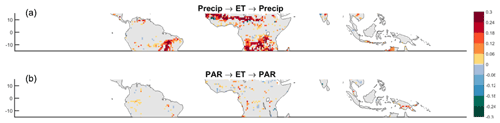
Figure 12Land–atmosphere feedback strength (change in the variance due to the feedback) between precipitation (Precip) and ET (a) and photosynthetically active radiation (PAR) (b) based on recent metric developed by Green et al. (2017) using a multivariate Granger causality approach.
Land-surface and ecosystem models, apart from a few exceptions (Xu et al., 2016; Kennedy et al., 2019), do not represent plant hydraulics and typically only rely on an empirical reduction of stomatal and ecosystem conductance, and therefore transpiration and GPP, as functions of root-averaged soil moisture or water potential (e.g., Noilhan and Planton, 1989; Sellers et al., 1996a, b; Ek, 2003; Boulet et al., 2007; Gentine et al., 2007; Ngo-Duc et al., 2007; Stoeckli et al., 2008; Balsamo et al., 2009; Boone et al., 2009a; Bonan et al., 2011, 2012; Lawrence et al., 2011; Canal et al., 2014; Han et al., 2014; Naudts et al., 2015; De Kauwe et al., 2015; Chaney et al., 2016; Chen et al., 2016; Haverd et al., 2016, among others). The root profile averaging of soil moisture or water potential to define water stress exaggerates the impact of surface drying, as in reality deeper roots may still effectively transport water to the plant xylem even if surface roots experience dry conditions and therefore can maintain overall high rates of GPP and transpiration.
The inclusion of plant hydraulics in tall-canopy species leads to strong differentiation between leaf (and upper xylem) and soil water potential (Fig. 11) during midday, especially in the dry season. Indeed, leaf and xylem water potentials substantially drop because of the large transpiration rates through the stomata and because the xylem cannot be instantaneously refilled due to the large flow drag in the elongated xylem. As a result, plant hydraulics induce a shutdown of stomata during the day, reducing the transpiration rate near peak solar hours, also known as “midday depression”, (Malhi et al., 1998; Williams et al., 1998; Harris et al., 2004), in order to reduce desiccation of the leaf and xylem. In addition, plant hydraulics also induce a natural hydraulic redistribution of water in the root profile, reducing dryness in the upper profile in the dry season (Lee et al., 2005; Oliveira et al., 2005; Domec et al., 2010; Prieto and Ryel, 2014; Kennedy et al., 2019), using deep root moisture rather than surface soil moisture when needed, as the water flows down gradient of water potentials. This is fundamentally different from typical parameterizations using average water stress of the root water profile, which are oversensitive to surface water stress, in typical parameterizations. Both of those effects lead to reduced sensitivity to water stress and help maintain higher rates of transpiration throughout the entire dry season, whereas typical land-surface models overestimate water stress in the dry season (de Gonçalves et al., 2013; Alemohammad et al., 2017).
Having reviewed some of the important components of tropical land–atmosphere interactions, we now turn to the coupling of these components. Often, land–atmosphere interactions are framed in terms of a one-dimensional column, comprising a “point” of interest, although a point here may be understood not only as a site (such as an eddy-covariance flux tower) but also as spatial averages of varying scales. While this local, column view is certainly instructive, we suggest that it is also necessary to consider land–atmosphere interactions through an interplay with remote influences, i.e., a nonlocal perspective. This may be especially true in the tropics given the strong coupling that exists between convective and large scales. We will revisit the seasonality of tropical climate through the lens of these local and nonlocal perspectives of land–atmosphere interactions.
5.1 Quantifying land–atmosphere interactions
Green et al. (2017) recently developed a method to define the feedback between the biosphere and atmosphere using multivariate conditional Granger causality (based on lagged autoregressive vectors). We here use a similar framework using ET from WECANN and precipitation from GPCP as well as photosynthetically active radiation from CERES (Fig. 12).
Most of the feedback between surface ET and precipitation occurs in the climatically transitional and/or monsoonal regions, such as the savanna region of northeastern Brazil and the monsoonal regions over South Asia, the Sahel, southern Africa, and northern Australia. In Brazil, these results are consistent with spatial transition inherent in the convective margins concept introduced by Lintner and Neelin (2007; see also Fig. 13) and the impact of soil moisture and evapotranspiration on setting the location of the transition between the dry and wet regions. The Sahelian and southern African monsoon are also located in regions between very dry (deserts) and humid regions, where surface feedback may be crucial for the penetration of the monsoonal flow inland (Lintner and Neelin, 2009; Lintner et al., 2015). Indeed, the biosphere in this region modulates the local climate state: multiple equilibrium states, corresponding to different ecosystem initial conditions, may exist under the same external (e.g., top-of-the-atmosphere solar) forcing (Wang et al., 2000). The effect of vegetation on land–atmosphere coupling manifests itself at multiple timescales. At short timescales after precipitation, evaporation is accelerated with intercepted water in the canopy. However, at longer timescales vegetation acts to delay and prolong evaporation of water stored in the root zone. The magnitude and timescale of these sources of water recycling vary depending on ecosystem structure, including rooting depth and canopy structure, which may coevolve with atmospheric conditions at the interannual timescale (Nicholson, 2000). This represents a clear pathway for two-way feedbacks between the land surface and precipitation.
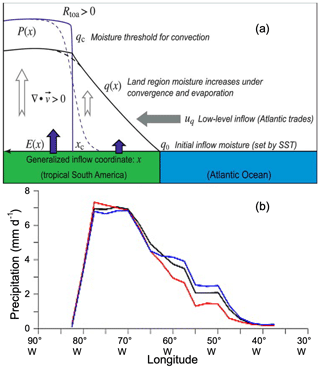
Figure 13(a) Schematic of the key elements of the convective margins framework as applied along an inflow path across northeastern South America. The solid blue and black lines are precipitation and vertically integrated moisture for steady-state conditions, while the dashed blue and black lines correspond to precipitation and vertically integrated moisture “smeared out” in the presence of time-varying, transient behavior. Adapted from Fig. 2 of Lintner and Neelin (2009). (b) Rainfall longitude.
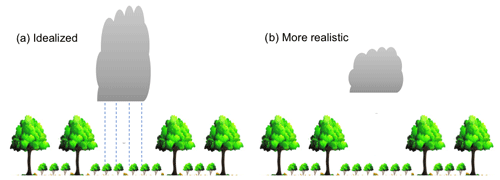
Figure 14Mesoscale heterogeneity impact on cloud generation in the dry season. (a) Typical perspective regarding the impact of deforestation and clearings generating deep convective clouds and (b) more realistic impact, in terms of mostly a modification of shallow convection cloud cover, impacting radiation more than precipitation.
We further emphasize that those feedbacks (Fig. 12) are likely to also be influenced by nonlocal conditions, with regional and large-scale changes in ocean to land flow and the inland distance of penetration influencing local coupling. We note that climate models seem to exhibit feedbacks of soil moisture (and therefore evapotranspiration) to precipitation in similar tropical regions, when averaged across models, even though individual model response varies (Koster et al., 2011; Seneviratne, 2013; 1 ∘ pixel and monthly timescales). We emphasize that the PAR radiation product is very uncertain in the tropics (Jimenez et al., 2011), as it ultimately relies on a model to obtain surface incoming radiation, which might explain the reduced biosphere–precipitation feedback strength in the wet tropics compared to other regions. It is also likely that the bulk of the radiative feedbacks are taking place at smaller timescales such as the ones observed with MODIS (Fig. 15). This shallow cloud cover is relatively steady spatially and in time, especially in the dry season.
5.2 A local view of tropical land–atmosphere interactions
A critical aspect of land–atmosphere interactions in tropical rainforests is related to shallow clouds and fog rather than deep convective clouds. Clearly, much of the focus of tropical land–atmosphere interactions has been on feedbacks involving precipitating deep convection and the impact of surface heterogeneity on convective rainfall. On the other hand, the coupling of the land surface to radiation has been relatively understudied. Shallow clouds lead to reduced productivity and transpiration (Anber et al., 2015a), yet the latter depends on cloud thickness, as cumulus clouds (shallow convection) generate more diffuse light and may consequently boost photosynthesis when they are not too thick (Pedruzo-Bagazgoitia et al., 2017; Fig. 14). Fog, on the other hand, strongly diminishes the amount of incident light for ecosystems. Fog (Anber et al., 2015a) and shallow clouds (Giangrande et al., 2017) appear to be two of the primary differences between the dry and the wet season (in addition to the preferential occurrence of nighttime mesoscale convective systems in the rainy season, which are not directly relevant for land–atmosphere interactions associated with daytime processes). Low-level cloudiness largely affects the surface incoming radiation by reducing shortwave surface incoming radiation in the wet season, especially in the morning (Anber et al., 2015a; Giangrande et al., 2017), which in turn leads to strong reduction in GPP and ET. These clouds are also tightly connected to surface processes and especially the surface energy partitioning. Indeed, nighttime fog, which often persists into the early daylight hours, is due to nighttime longwave cooling in very humid boundary layers due, for instance, to evening rain in the wet season (Anber et al., 2015a). Shallow clouds are themselves directly forced by surface-generated thermals, i.e., boundary layer processes, and they are modified by the sensible and latent heat flux magnitude (Vilà‐Guerau de Arellano et al., 2014, 2019). Shallow convection and low-cloud cover are also tightly connected to ecosystem seasonality and to the diurnal cycle (Anber et al., 2015a; Tang et al., 2016; Giangrande et al., 2017).
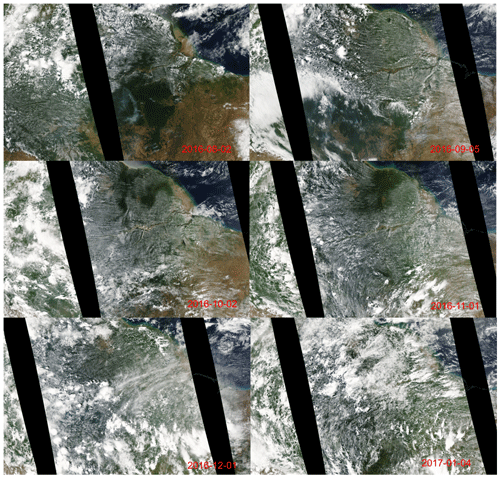
Figure 15MODIS visible image of the northwestern Amazon as the basin transitions into the wet season. In the dry season, surface heterogeneity, whether due to rivers, forest-deforested patches, or land–ocean contrast, is very clear. In the wet season those sharp gradients disappear as cloud cover mostly dominated by deep convection starts organizing at scales independent of the surface heterogeneity.
Historically, the study of land–atmosphere interactions in the tropics, and tropical rainforests in particular, has emphasized effects of heterogeneity, especially due to deforestation, on the generation of deep convection through mesoscale circulations (see Lawrence and Vandecar, 2015, for a complete review as well as Avissar and Pielke, 1989; Pielke and Avissar, 1990; Pielke et al., 1991; Dalu et al., 1996; Avissar and Schmidt, 1998; Taylor et al., 2007, 2009, 2011; Rieck et al., 2015; Khanna et al., 2017). The hypothesis behind this is that deforestation reduces EF and surface roughness (Khanna et al., 2017). The associated increased buoyancy flux over the deforested areas, mostly reflecting a shift toward increased sensible heating, induces mesoscale circulations. These circulations enhance cloudiness through local buoyancy fluxes, turbulent kinetic energy generation, and low-level moisture advection from adjacent forested areas, thus providing all the key ingredients for moist convection generation (Rieck et al., 2014, 2015). It seems unlikely however that momentum roughness plays a major role in this high-radiation environment (Park et al., 2018), where circulations are mostly buoyancy-driven. Instead, the heat and moisture roughness lengths (Park et al., 2018) as well as leaf area index (LAI) and stomatal conductance, which scales the magnitude of the evapotranspiration flux, are the main players, in addition to changes in soil-moisture availability, for the circulation. The impact of the deforestation on surface fluxes and local circulation can change from being driven by buoyancy to being driven by surface roughness as the spatial scale of the deforestation increases (Khanna et al., 2017).
Induced mesoscale circulations and associated deep convection are clearly observable with remote-sensing observations (Khanna et al., 2017) and are more important in the dry season (Khanna et al., 2017), when convection is more locally, and regionally, triggered (Anber et al., 2015a; Ghate and Kollias, 2016). Once precipitation occurs, cold pools, i.e., density currents induced by ice melt and evaporating rain in downdrafts, dominate the surface-induced mesoscale circulation (Rieck et al., 2015) and reduce the surface heterogeneity signal. In the wet season, the relative contribution of local forcing to the total rainfall is small, as the bulk of the precipitation is due to mesoscale convective systems or larger-scale systems propagating throughout the basin, less tightly connected to surface and boundary layer processes (Ghate and Kollias, 2016).
Even during the dry season, a large fraction of the Amazon and Indonesia only experiences minimal water stress (Figs. 8 and 7) so that increased radiation generates higher rates of photosynthesis (Fig. 5) and ET (Fig. 6; Anber et al., 2015a). Indeed, higher transpiration in the dry season (due to the higher demand which is not entirely compensated by the slight water stress) can compensate the effect of reduced rain reevaporation intercepted by the canopy. As a result the feedback loop between precipitation and ET is weakened and the impact of the dry season on ET (and hence clouds) is strongest (Fig. 14). In addition, the feedback of shallower clouds and surface radiation may be more important (Fig. 14) than the feedback of deeper clouds and precipitation, as those shallow clouds are preferentially triggered over drier surfaces. Because shallow clouds have a small life cycle (typically less than 30 min) compared to deep convective and mesoscale systems, they are more directly connected to the underlying surface conditions and interact more with the local conditions.
Fewer studies have investigated changes in shallow clouds (Wang et al., 2000, 2009; Lawton et al., 2001; Chagnon et al., 2004; Ray et al., 2006; Pielke et al., 2011; Rieck et al., 2014; Anber et al., 2015a) even though the impact of changes in the surface energy partitioning and heterogeneity on low-level clouds is clear and spatially systematic (Fig. 15). Given the importance of cloud cover on shortwave radiation and its importance for the differentiation between the dry and wet seasons over wet tropical rainforests, we believe that this low-cloud feedback might be quite critical for rainforest ecosystem functioning. Indeed it was pointed out by Morton et al. (2014, 2016) and Anber et al. (2015a) that light changes between the dry and wet season due to changes in cloud cover were one of the primary reasons for changes in the seasonality of surface fluxes in addition to leaf flush out (Lopes et al., 2016; Saleska et al., 2016). At subdaily scales, the shading due to low clouds reduces surface temperature and ecosystem respiration (Mahecha et al., 2010; Peterhansel and Maurino, 2011; Thornley, 2011; Hadden and Grelle, 2016; Ballantyne et al., 2017). This reduction depends strongly on the cloud cycling and thickness. As a result, cloud-induced reductions in respiration can cancel reductions in photosynthesis such that the net effect of cloud shading on net ecosystem exchange is unclear. Horn et al. (2015) showed that explicitly calculating the surface coupling leads to a change in the length scales of clouds and a reduction of the cloud lifetime. As a result, and although the cloud cover remains almost the same, there are larger populations of smaller shallow cumuli. These responses to vegetation influence also the moisture transport and the cloud characteristics (Sikma et al., 2019).
In addition to regulating radiative energy balance at the surface, Wright et al. (2017) have shown that shallow convection transports moisture, provided by plants' transpiration, from the atmospheric boundary layer to the lower troposphere during the late dry season and early dry-to-wet transition seasons (July–September; Fu and Li, 2004). This mechanism, referred to as the “shallow convective moisture pump”, plays an important role in priming the atmosphere for increasing deep convection (e.g., Schiro et al., 2016;a Zhuang et al., 2017) and wet-season onset over the Amazon (Wright et al., 2017).
The results discussed until now omitted the relation between physical processes and the atmospheric composition and, more specifically, the role of chemical reactions and aerosols. Over rainforests, the pristine and undisturbed conditions of the atmospheric boundary layer described in the seminal study by Garstang and Fitzjarrald (1999) are currently undergoing rapid changes due to atmospheric composition modifications. Their direct impact on the radiative and microphysical properties is due to biomass burning and enhancement of concentrations of secondary organic aerosol precursors. Biomass burning in Amazonia leads to increased aerosol optical depth and to abnormal distributions of the heating rate profile. Analyzing systematic experiments performed by large-eddy simulations, Feingold et al. (2005) studied the processes that lead to the suppression of clouds. Firstly, at the surface there are clear indications that the latent and sensible heat flux are reduced, yielding convective boundary layers characterized by less turbulent intensity and by delays in the morning transition (Barbaro and Vilà-Guerau de Arellano, 2014). Both aspects tend to reduce cloud formations. Secondly, Barbaro and Vilà-Guerau de Arellano (2014) indicated that the vertical location of the smoke layer is crucial in determining the dynamics of the boundary layer which can delay the onset of shallow cumulus. In turn, and as described by Feingold et al. (2005), smoke confined in the well-mixed sub-cloud layer might positively benefit the cloud formation, since it distributes the heat uniformly that contributes to enhancing convection. On the other hand, smoke layers located within the cloud layer tend to stabilize the cloud layer and therefore decrease the possibility of cloud formation. These results are very much dependent on the aerosol optical properties defined by their heating, scattering, and hygroscopic properties. As a first indicative figure, the mentioned large-eddy simulation (LES) study and observations by Koren et al. (2004) stressed that smoke layers with an aerosol optical depth larger than 0.5 might already lead to cloud suppression by 50 %. Yu et al. (2008) have shown observationally that the influence of aerosols on shallow clouds varies with meteorological conditions. When the ambient atmosphere is drier (relative humidity ≤ 60 %), the cloud-burning effect (evaporation of cloud droplets) due to increased absorption of solar radiation by aerosols outweighs the increase in cloud droplets due to the aerosol–cloud microphysical effect. The reduced shallow clouds can further enhance the surface dryness. In contrast, when the ambient atmosphere is relatively humid (relative humidity ≥ 60 %), the aerosol–cloud microphysical effect outweighs the cloud-burning effect, leading to an increase in shallow clouds and relative humidity near surface. In so doing, aerosols can amplify the original moisture anomalies near the surface. Aerosols have also been shown to increase the lifetime of mesoscale convection over the Congo and Amazon basins due to the delay in the precipitation that enhances ice formation and increased lifetime of the mature and decay phase of deep convection (Chakraborty et al., 2016).
These modifications are not only related to the direct emission of aerosol but also to changes in the gas-phase chemistry that act as a precursor for the formation of secondary organic aerosol. Andreae et al. (2002) showed large differences in the NOx and ozone (O3) mixing ratio throughout the Amazon, from rather pristine conditions with NOx and ozone levels below 0.1 and 20 ppb to values above 0.1 ppb and maximum levels of O3 near 50 ppb near Manaus. Recent field experiments within the GoAmazon campaign (Fuentes et al., 2016; Martin et al., 2016) corroborate these levels as well as the high levels of the bio-organic compounds, in particular isoprene and monoterpene. Closely related, these changes are accentuated by anthropogenic emissions from Manaus. The unique distribution of aerosols in Amazonia might explain observed differences in deep convection, in particular lightning frequency, between Amazonia, the Maritime Continent, and the Congo Basin (Williams et al., 2004). To represent these chemistry changes and their effect on convection adequately, the dynamics that drive processes such as the entrainment of pollutants from the free troposphere need to be taken into account (Vilà-Guerau de Arellano et al., 2011). As a result of this interaction between radiation, the land surface, dynamics, and chemical processes, the frequency of the clear convective vs. shallow cloudy conditions may be modified in the future. Current efforts in monitoring them and improving the parameterizations of convection are under way. These efforts should include also, in an integrated manner, the combined role of dynamics and chemistry to quantify relevant processes. Two of them are relevant and difficult to be represented in large-scale models: first, the role of canopy in controlling the emission and deposition of the aerosol precursors on tropical rainforest (Sánchez Gácita et al., 2017) and, second, ventilation of pollutants from the sub-cloud layer into the cloud layer, i.e., mass flux parameterizations, under representative Amazon conditions (Ouwersloot et al., 2013).
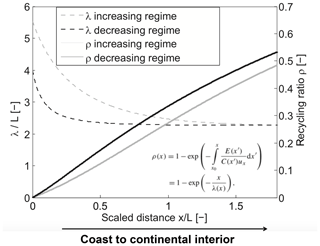
Figure 16Continental recycling ratio, ρ, and recycling length scale, λ, normalized by length scale, L, along the inflow direction x for atmospheric moisture, either increasing or decreasing along the inflow path in the idealized model of Schaefli et al. (2012). ρ represents how much of the rainfall is derived from terrestrial evapotranspiration, while λ represents the length scale over which evapotranspired water is removed.
In addition to affecting cloud microphysics, biomass burning in the tropics significantly affects the global carbon budget. For example, in September and October of 2015, fires on the Maritime Continent released more terrestrial carbon (11.3 Tg C) than the anthropogenic emissions of the EU (8.9 Tg C; Huijnen et al., 2016). The extent of forest fires in this region is tied to El Niño-induced drought conditions, and antecedent sea surface temperature (SST) patterns are closely related to the burned area at the global scale, particularly in hotspots concentrated in the tropics (Chen et al., 2016). Aerosol emissions and biomass burning exert a strong control on land–atmosphere coupling of the carbon and water cycles, and the consequences of this coupling are observable globally.
5.3 Nonlocal view of tropical land–atmosphere interactions
5.3.1 Moisture tracking and source attribution
A fundamental consideration in the study of the hydrologic cycle over tropical continents is where the moisture for precipitation ultimately derives. In fact, many of the seminal studies of tropical land region water cycle in the 1980s and 1990s focused on the concept of recycling, i.e., the contribution of evapotranspiration over a region of interest to precipitation in that region (Brubaker et al., 1993; Eltahir and Bras, 1994; Trenberth, 1999). While these early studies typically estimated recycling using bulk formulas derived under simplifying assumptions, more sophisticated approaches for estimating recycling have emerged (van der Ent et al., 2010), including comprehensive moisture tracking operating on subdaily inputs on models and reanalysis, e.g., the dynamic recycling model (Dominguez et al., 2006), the water accounting model (van der Ent et al., 2010), and Lagrangian approaches using parcel dispersion models such as FLEXPART (Gimeno et al., 2012). Contemporary estimates of recycling ratios for the Amazon Basin range from 25 % to 35 % (Zemp et al., 2014).
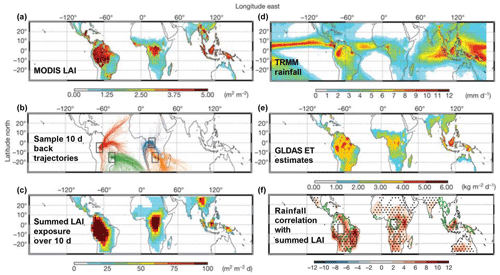
Figure 17Ten-day back-trajectory analysis over several regions of the continental tropics along with LAI, mean Tropical Rainfall Measuring Mission (TRMM) estimated rainfall, and Global Land Data Assimilation System (GLDAS) ET estimates.
These more sophisticated approaches have also enabled identification and quantification of upstream sources of moisture that lead to downstream rainfall over tropical land regions (Dirmeyer and Brubaker, 2007; Drumond et al., 2014; Hoyos et al., 2018; Stohl and James, 2005). For example, by combining a Lagrangian back-trajectory approach with rainfall and leaf area index data, Spracklen et al. (2012) quantified the linkage between downstream rainfall amount and upstream air mass exposure to vegetation (Fig. 17). Over more than half of the tropical land surface, Spracklen et al. estimate a 2-fold increase in downstream rainfall for those air masses passing over extensive vegetation compared with those passing over little upstream vegetation. Based on these estimates and extrapolating current Amazonian deforestation trends into the future, these authors project wet- and dry-season rainfall decreases of 12 % and 21 %, respectively, by the year 2050. In some regions, such attributions underscore the importance of upstream land regions as moisture sources: for example, Drumond et al. (2014) used the FLEXPART model forced with ERA-Interim reanalysis to estimate E−P along trajectories passing over the Río de la Plata basin in subtropical South America to establish that much of the moisture entering this region derives from the Amazon Basin to the north and west.
5.3.2 Large-scale coupling and idealized modeling
Some studies have attempted to frame tropical land–atmosphere interactions on a larger scale, and implicitly nonlocal, way (Zeng and Neelin, 1999; Lintner et al., 2013; Berg et al., 2017). For example, Lintner et al. (2013) developed an idealized prototype for diagnosing large-scale land–atmosphere coupling constructed from coupling the vertically integrated temperature and moisture equations to a simple bucket soil-moisture model. From this model, they derived analytic sensitivity of precipitation to soil-moisture spatial variation along a transect to various process parameters related to convection and radiation, such as the timescale for convective adjustment and the strength of cloud-radiative feedback. Schaefli et al. (2012) developed a conceptually similar model from which an analytic expression for the ratio of evapotranspired moisture integrated along the flow path to precipitation (or recycling ratio) was obtained (Fig. 16). Such idealized model frameworks, which consider tropical land–atmosphere interactions by coupling both water and energy cycles, can be helpful in interpreting and diagnosing linkages between local and nonlocal feedbacks.
5.4 Land–atmosphere interactions and their impact on tropical seasonality
One of the outstanding issues in the study of tropical land region climates involves controls on precipitation seasonality, particularly its regional variability. Generally, the seasonality follows the variation in maximum solar heating, but other factors, such as ocean thermal inertia, topography, dynamics and circulation, moisture transport, and the state of the land surface, can exert considerable influence on the timing and amplitude of tropical land region seasonal evolution. Over the Amazon Basin, seasonality exhibits marked variation in both latitude and longitude: for example, at 5 ∘ S, the dry-to-wet transition proceeds from the central Amazon eastward toward the Atlantic coast (Liebmann and Marengo, 2001). It is also worth noting a pervasive tendency for the dry-to-wet season transition to occur much more rapidly than the wet-to-dry transition, as evident in tropical monsoon systems including South Asia, West Africa, and South America.
Analyzing multiple observational and reanalysis products, Fu and Li (2004) identified a strong influence of surface turbulent fluxes on the dry-to-wet transition and its interannual variability over the Amazon. In particular, their results link earlier wet-season onset to wetter conditions in the antecedent dry season: the higher latent fluxes at the end of a wetter dry season encourage weaker convective inhibition (CIN) and enhance convective available potential energy (CAPE), both of which are more favorable to wet-season rainfall occurrence. However, these authors also underscore the participation of the large-scale circulation and its role in establishing a background environment (e.g., moisture convergence) to support wet-season rainfall. Incursion of cold fronts into the southern Amazon may act as triggers for rapid initiation of wet-season onset once the local thermodynamics become favorable (Li et al., 2006).
Recent research suggests that the land–atmosphere coupling is integral to the earlier occurrence of determining earlier occurrence of wet-season onset over western and southern Amazonia, relative to that of eastern Amazonia. Both in situ and satellite ecological observations have consistently shown that rainforests increase their photosynthesis, and thus evapotranspiration (ET), during the late dry season across Amazonia (e.g., Huete et al., 2006; Lopes et al., 2016; Munger et al., 2016; Wehr et al., 2017). The wet-season onset over the Southern Hemisphere portion of western Amazonia occurs during September to October, about 2 to 3 months before the Atlantic ITCZ (Fu et al., 2016). Using several satellite measurements, including the isotopic signature of deuterium in atmospheric water vapor (HDO) and SIF, Wright et al. (2017) have shown that increasing late dry-season ET is the primary source of increasing water vapor in the lower troposphere that initiates the increase in deep convection and rainfall over southern Amazonia. In particular, the increase in water vapor with enriched HDO in the boundary layer and free troposphere follows the increase in photosynthesis during late dry season prior to wet-season onset. During this period, the water vapor HDO is too high to be explained by transport from Atlantic Ocean and is consistent with that from plant transpiration. Such a moistening of the atmosphere starts in western southern Amazonia, the part of Amazonia that is most remote from the Atlantic Ocean with high biomass. It then progresses towards eastern southern Amazonia. Thus, during the late dry season this appears to contribute to the timing and spatial variation in the initial moistening of the atmosphere, which ultimately lead to wet-season onset over southern Amazonia.
Wet-season onset over southern Amazonia has been increasingly delayed since the late 1970s (Marengo et al., 2011; Fu et al., 2013). In addition to the influence of global circulation change, such a change has been attributed to land use. For example, Butt et al. (2011) have compared long-term rainfall data between deforested and forested areas over part of the southern Amazonia. They observed a significant delay in wet-season onset over the deforested areas, consistent with that implied by Wright et al. (2017). In addition, Zhang et al. (2008, 2009) have shown that biomass-burning aerosols, which peak in late dry season, can also weaken and delay dry-to-wet season transition by stabilizing the atmosphere, reducing clouds and rainfall.
In this review paper, we have discussed some of the important aspects of land–atmosphere interactions pertaining to the tropics. While our review is by no means exhaustive, it illustrates some of the key processes in the coupled tropical land–atmosphere system acting across multiple spatial and temporal scales, especially in rainforest ecosystems.
We have argued that feedbacks between the land surface and precipitation in the tropics are possibly nonlocal in nature (for instance due to the weak temperature gradient) and may mostly impact moisture advection from the ocean and the position of deep convection onset. Local rainfall feedback associated with mesoscale heterogeneities appears to be rather small in magnitude, at least compared to the annual-mean rainfall, and not sufficiently spatially systematic to truly affect ecosystem functioning.
Moreover, we contend that land-surface–cloud feedbacks, especially those involving shallow clouds and fog, are critical in terms of regulating light (direct and diffuse), temperature, and water vapor deficit over tropical forest, but such feedbacks have received relatively less attention. Remote-sensing platforms provide useful information for quantifying such feedbacks, but these need to be complemented by ground measurements. Eddy-covariance measurements may prove difficult to use, as mesoscale circulations alter the homogeneity assumption of eddy-covariance methods.
We have also discussed errors and biases in the representation of tropical continental climates in current-generation climate and earth system models. Multi-model assessments of soil-moisture–precipitation feedback strength in ensembles of earth system models such as those of Koster et al. (2004) manifest strong land–precipitation feedbacks in similar transitional regions to the ones observed (Green et al., 2017), which seems to be mostly related to modification of the moisture-advection penetration distance from the ocean rather than to local feedbacks. These feedbacks appear to be of relatively minor importance in the core of tropical rainforests but are more critical for more marginal rainfall regions (savanna). These regions are of critical importance for the terrestrial global carbon cycle, providing the main terrestrial sink, but might be severely impacted by climate change and droughts in particular (Laan-Luijkx et al., 2015). Whether the interannual variability in surface CO2 flux in those regions is a zero-sum game with wet years compensating dry years still is an open question especially in the context of rising CO2 concentration.
The core of rainforests seems to be more affected by radiation feedbacks at relatively small spatial scales (∼ 1 km), which can be dramatically influenced by land cover and land-use change. Projected rates of future deforestation are poorly constrained, especially regionally, though in recent years, the Congo and Indonesia have experienced increasing deforestation, while the deforestation rate in the Amazon has dropped.
The only data are from WECANN: https://avdc.gsfc.nasa.gov/pub/data/project/WECANN/ (last access: 24 September 2019).
PG designed the work and contributed to all sections, AM contributed to all sections, BR contributed to Sect. 5, SHA contributed the WECANN data, RF contributed to Sect. 5, JKG contributed data analysis, DK contributed to Sect. 4, and JVG contributed the aerosol discussion.
The authors declare that they have no conflict of interest.
We would like to acknowledge high-performance computing support from Yellowstone (ark:/85065/d7wd3xhc) provided by NCAR's Computational and Information Systems Laboratory, sponsored by the National Science Foundation.
This research has been supported by the New Investigator (grant no. NNX14AI36G), DOE Early Career (grant no. DE-SC0014203), and NSF CAREER and GoAmazon (grant no. DE-SC0011094).
This paper was edited by Nunzio Romano and reviewed by three anonymous referees.
Acevedo, O. C., Moraes, O. L. L., da Silva, R., Fitzjarrald, D. R., Sakai, R. K., Staebler, R. M., and Czikowsky, M. J.: Inferring nocturnal surface fluxes from vertical profiles of scalars in an Amazon pasture, Global Change Biol., 10, 886–894, https://doi.org/10.1111/j.1529-8817.2003.00755.x, 2004.
Alemohammad, S. H., Fang, B., Konings, A. G., Aires, F., Green, J. K., Kolassa, J., Miralles, D., Prigent, C., and Gentine, P.: Water, Energy, and Carbon with Artificial Neural Networks (WECANN): a statistically based estimate of global surface turbulent fluxes and gross primary productivity using solar-induced fluorescence, Biogeosciences, 14, 4101–4124, https://doi.org/10.5194/bg-14-4101-2017, 2017.
Anber, U., Gentine, P., Wang, S., and Sobel, A. H.: Fog and rain in the Amazon, P. Natl. Acad. Sci. USA, 112, 11473–11477, https://doi.org/10.1073/pnas.1505077112, 2015a.
Anber, U., Wang, S., and Sobel, A.: Effect of Surface Fluxes versus Radiative Heating on Tropical Deep Convection, J. Atmos. Sci., 72, 3378–3388, https://doi.org/10.1175/JAS-D-14-0253.1, 2015b.
Andreae, M. O., Artaxo, P., Brandao, C., Carswell, F. E., Ciccioli, P., Da Costa, A. L., Culf, A. D., Esteves, J. L., Gash, J. H. C., Grace, J., and Kabat, P.: Biogeochemical cycling of carbon, water, energy, trace gases, and aerosols in Amazonia: The LBA-EUSTACH experiments, J. Geophys. Res.-Atmos., 107, https://doi.org/10.1029/2001JD000524, 2002.
Andreasen, M., Jensen, K. H., Desilets, D., Zreda, M., Bogena, H. R., and Looms, M. C.: Cosmic-ray neutron transport at a forest field site: the sensitivity to various environmental conditions with focus on biomass and canopy interception, Hydrol. Earth Syst. Sci., 21, 1875–1894, https://doi.org/10.5194/hess-21-1875-2017, 2017.
Avissar, R. and Nobre, C.: Preface to special issue on the Large-Scale Biosphere-Atmosphere Experiment in Amazonia (LBA), J. Geophys. Res.-Atmos., 107, https://doi.org/10.1029/2002JD002507, 2002.
Avissar, R. and Pielke, R. A.: A parameterization of heterogeneous land surfaces for atmospheric numerical models and its impact on regional meteorology, Mon. Weather Rev., 117, 2113–2136, 1989.
Avissar, R. and Schmidt, T.: An evaluation of the scale at which ground-surface heat flux patchiness affects the convective boundary layer using large-eddy simulations, J. Atmos. Sci., 55, 2666–2689, 1998.
Avissar, R., Dias, P., Dias, M., and Nobre, C.: The Large-Scale Biosphere-atmosphere Experiment in Amazonia (LBA): Insights and future research needs, J. Geophys. Res.-Atmos., 107, https://doi.org/10.1029/2002JD002704, 2002.
Baldocchi, D., Falge, E., Gu, L., Olson, R., Hollinger, D., Running, S., Anthoni, P., Bernhofer, C., Davis, K., Evans, R., and Fuentes, J.: FLUXNET: A new tool to study the temporal and spatial variability of ecosystem-scale carbon dioxide, water vapor, and energy flux densities, B. Am. Meteorol. Soc., 82, 2415–2434, 2001.
Ballantyne, A., Smith, W., Anderegg, W., Kauppi, P., Sarmiento, J., Tans, P., Shevliakova, E., Pan, Y., Poulter, B., Anav, A., and Friedlingstein, P.: Accelerating net terrestrial carbon uptake during the warming hiatus due to reduced respiration, Nat. Clim. Change, 7, 148–152, https://doi.org/10.1038/nclimate3204, 2017.
Balsamo, G., Beljaars, A., Scipal, K., Viterbo, P., van den Hurk, B., Hirschi, M., and Betts, A. K.: A Revised Hydrology for the ECMWF Model: Verification from Field Site to Terrestrial Water Storage and Impact in the Integrated Forecast System, J. Hydrometeorol., 10, 623–643, https://doi.org/10.1175/2008JHM1068.1, 2009.
Barbaro, E. and Arellano, J.: Aerosols in the convective boundary layer: Shortwave radiation effects on the coupledland-atmosphere system, J. Climate, 119, 5845–5863, https://doi.org/10.1002/(ISSN)2169-8996, 2014.
Bechtold, P., Semane, N., Lopez, P., Chaboureau, J.-P., Beljaars, A., and Bormann, N.: Representing equilibrium and non-equilibrium convection in large-scale models, J. Atmos. Sci., 71, 734–753, https://doi.org/10.1175/JAS-D-13-0163.1, 2013.
Bechtold, P., Semane, N., Lopez, P., Chaboureau, J.-P., Beljaars, A., and Bormann, N.: Representing Equilibrium and Nonequilibrium Convection in Large-Scale Models, J. Atmos. Sci., 71, 734–753, https://doi.org/10.1175/JAS-D-13-0163.1, 2014.
Berg, A. M., Lintner, B. R., Findell, K. L., and Giannini, A.: Soil moisture influence on seasonality and large-scale circulation in simulations of the West African monsoon, J. Climate, 30, 2295–2317, 2017.
Betts, A. K. and Silva Dias, M. A. F.: Progress in understanding land-surface-atmosphere coupling from LBA research, J. Adv. Model. Earth Syst., 2, https://doi.org/10.3894/JAMES.2010.2.6, 2010.
Betts, A. K., Gatti, L. V., and Cordova, A. M.: Transport of ozone to the surface by convective downdrafts at night, J. Climate, , 107, 13.1–13.6, 2002.
Böing, S. J., Jonker, H. J. J., Siebesma, A. P., and Grabowski, W. W.: Influence of the Subcloud Layer on the Development of a Deep Convective Ensemble, J. Atmos. Sci., 69, 2682–2698, https://doi.org/10.1175/JAS-D-11-0317.1, 2012.
Boisier, J. P., Ciais, P., Ducharne, A., and Guimberteau, M.: Projected strengthening of Amazonian dry season by constrained climate model simulations, Nat. Clim. Change, 5, 656–660, https://doi.org/10.1038/nclimate2658, 2015.
Bonan, G. B., Lawrence, P. J., Oleson, K. W., Levis, S., Jung, M., Reichstein, M., Lawrence, D. M., and Swenson, S. C.: Improving canopy processes in the Community Land Model version 4 (CLM4) using global flux fields empirically inferred from FLUXNET data, J. Geophys. Res.-Biogeo., 116, 1–22, https://doi.org/10.1029/2010JG001593, 2011.
Bonan, G. B., Oleson, K. W., Fisher, R. A., Lasslop, G., and Reichstein, M.: Reconciling leaf physiological traits and canopy flux data: Use of the TRY and FLUXNET databases in the Community Land Model version 4, J. Geophys. Res.-Biogeo., 117, 1–19, 2012.
Boone, A., De Rosnay, P., Balsamo, G., Beljaars, A., Chopin, F., Decharme, B., Delire, C., Ducharne, A., Gascoin, S., Grippa, M., and Guichard, F.: The AMMA Land Surface Model Intercomparison Project (ALMIP), B. Am. Meteorol. Soc., 90, 1865–1880, https://doi.org/10.1175/2009BAMS2786.1, 2009a.
Boone, A., Getirana, A., Demarty, J., and Cappelaere, B.: The African Monsoon Multidisciplinary Analyses (AMMA) Land surface Model Intercomparison Project Phase 2 (ALMIP2), GEWEX News, 19, 9–10, 2009b.
Boulet, G., Chehbouni, A., Gentine, P., Duchemin, B., Ezzahar, J., and Hadria, R.: Monitoring water stress using time series of observed to unstressed surface temperature difference, Agr. Forest Meteorol., 146, 159–172, https://doi.org/10.1016/j.agrformet.2007.05.012, 2007.
Bretherton, C. S. and Park, S.: A New Moist Turbulence Parameterization in the Community Atmosphere Model, J. Climate, 22, 3422–3448, https://doi.org/10.1175/2008JCLI2556.1, 2009.
Brodribb, T. J.: Stomatal Closure during Leaf Dehydration, Correlation with Other Leaf Physiological Traits, Plant Physiol., 132, 2166–2173, https://doi.org/10.1104/pp.103.023879, 2003.
Brubaker, K. L., Entekhabi, D., and Eagleson, P. S.: Estimation of continental precipitation recycling, J. Climate, 6, 1077–1089, 1993.
Butt, N., de Oliveira, P. A., and Costa, M. H.: Evidence that deforestation affects the onset of the rainy season in Rondonia, Brazil, J. Geophys. Res.-Atmos., 116, 407, https://doi.org/10.1029/2010JD015174, 2011.
Campos, J. G., Acevedo, O. C., Tota, J., and Manzi, A. O.: On the temporal scale of the turbulent exchange of carbon dioxide and energy above a tropical rain forest in Amazonia, J. Geophys. Res., 114, 1–10, https://doi.org/10.1029/2008JD011240, 2009.
Canal, N., Calvet, J.-C., Decharme, B., Carrer, D., Lafont, S., and Pigeon, G.: Evaluation of root water uptake in the ISBA-A-gs land surface model using agricultural yield statistics over France, Hydrol. Earth Syst. Sci., 18, 4979-4999, https://doi.org/10.5194/hess-18-4979-2014, 2014.
Chagnon, F. J. F., Bras, R. L., and, Wang, J.: Climatic shift in patterns of shallow clouds over the Amazon, Geophys. Res. Lett., 31, L24212, https://doi.org/10.1029/2004GL021188, 2004.
Chakraborty, S., Fu, R., Massie, S. T., and Stephens, G.: Relative influence of meteorological conditions and aerosols on the lifetime of mesoscale convective systems, P. Natl. Acad. Sci. USA, 113, 7426–7431, 2016.
Chaney, N. W., Herman, J. D., Ek, M. B., and Wood, E. F.: Deriving Global Parameter Estimates for the Noah Land Surface Model using FLUXNET and Machine Learning, J. Geophys. Res.-Atmos., 121, 1–41, https://doi.org/10.1002/2016JD024821, 2016.
Chang, C. P., Wang, Z., McBride, J., and Liu, C. H.: Annual cycle of Southeast Asia–Maritime Continent rainfall and the asymmetric monsoon transition, J. Climate, 18, 287–301, 2005.
Chen, Y., Ryder, J., Bastrikov, V., McGrath, M. J., Naudts, K., Otto, J., Ottlé, C., Peylin, P., Polcher, J., Valade, A., Black, A., Elbers, J. A., Moors, E., Foken, T., van Gorsel, E., Haverd, V., Heinesch, B., Tiedemann, F., Knohl, A., Launiainen, S., Loustau, D., Ogée, J., Vessala, T., and Luyssaert, S.: Evaluating the performance of land surface model ORCHIDEE-CAN v1.0 on water and energy flux estimation with a single- and multi-layer energy budget scheme, Geosci. Model Dev., 9, 2951–2972, https://doi.org/10.5194/gmd-9-2951-2016, 2016.
Chor, T. L., Dias, N. L., Araújo, A., Wolff, S., Zahn, E., Manzi, A., Trebs, I., Sá, M. O., Teixeira, P. R., and Sörgel, M.: Flux-variance and flux-gradient relationships in the roughness sublayer over the Amazon forest, Agr. Forest Meteorol., 239, 213–222, https://doi.org/10.1016/j.agrformet.2017.03.009, 2017.
Cook, B. I., Shukla, S. P., Puma, M. J., and Nazarenko, L. S.: Irrigation as an historical climate forcing, Clim. Dynam., 44, 1715–1730, https://doi.org/10.1007/s00382-014-2204-7, 2014.
Couvreux, F., Rio, C., Guichard, F., Lothon, M., Canut, G., Bouniol, D., and Gounou, A.: Initiation of daytime local convection in a semi-arid region analysed with high-resolution simulations and AMMA observations, Q. J. Roy. Meteor. Soc., 138, 56–71, https://doi.org/10.1002/qj.903, 2011a.
Couvreux, F., Roehrig, R., Rio, C., Lefebvre, M. P., Caian, M., Komori, T., Derbyshire, S., Guichard, F., Favot, F., D'Andrea, F., and Bechtold, P.: Representation of daytime moist convection over the semi-arid Tropics by parametrizations used in climate and meteorological models, Q. J. Roy. Meteor. Soc., 141, 2220–2236, https://doi.org/10.1002/qj.2517, 2015.
Cox, P. M., Betts, R. A., Collins, M., Harris, P. P., Huntingford, C., and Jones, C. D.: Amazonian forest dieback under climate-carbon cycle projections for the 21st century, Theor. Appl. Climatol., 78, 137–156, 2004.
Dalu, G. A., Pielke, R. A., Baldi, M., and Zeng, X.: Heat and momentum fluxes induced by thermal inhomogeneities with and without large-scale flow, J. Atmos. Sci., 53, 3286–3302, 1996.
D'Andrea, F., Gentine, P., and Betts, A. K.: Triggering deep convection with a probabilistic plume model, J. Atmos. Sci., 71, 3881–3901, https://doi.org/10.1175/JAS-D-13-0340.1, 2014.
Da Rocha, H. R., Manzi, A. O., Cabral, O. M., Miller, S. D., Goulden, M. L., Saleska, S. R., R.‐Coupe, N., Wofsy, S. C., Borma, L. S., Artaxo, P., and Vourlitis, G: Patterns of water and heat flux across a biome gradient from tropical forest to savanna in Brazil, J. Geophys. Res., 114, G00B12, https://doi.org/10.1029/2007JG000640, 2009.
Davidson, E. A., de Araújo, A. C., Artaxo, P., Balch, J. K., Brown, I. F., Bustamante, M. M., Coe, M. T., DeFries, R. S., Keller, M., Longo, M., and Munger, J. W.: The Amazon basin in transition, Nature, 481, 321–328, https://doi.org/10.1038/nature10717, 2012.
Dawson, T. E.: Hydraulic lift and water use by plants – implications for water balance, performance and plant-plant interactions, Oecologia (Berl), 95, 565–574, 1993.
De Gonçalves, L. G., Borak, J. S., Costa, M. H., Saleska, S. R., Baker, I., Restrepo-Coupe, N., Muza, M. N., Poulter, B., Verbeeck, H., Fisher, J. B., and Arain, M. A.: Overview of the large-scale biosphere–atmosphere experiment in Amazonia Data Model Intercomparison Project (LBA-DMIP), Agr. Forest Meteorol., 182, 111–127, 2013.
De Kauwe, M. G., Kala, J., Lin, Y.-S., Pitman, A. J., Medlyn, B. E., Duursma, R. A., Abramowitz, G., Wang, Y.-P., and Miralles, D. G.: A test of an optimal stomatal conductance scheme within the CABLE land surface model, Geosci. Model Dev., 8, 431–452, https://doi.org/10.5194/gmd-8-431-2015, 2015.
Del Genio, A. D. and Wu, J.: The Role of Entrainment in the Diurnal Cycle of Continental Convection, J. Climate, 23, 2722–2738, https://doi.org/10.1175/2009JCLI3340.1, 2010.
Dirmeyer, P.: The terrestrial segment of soil moisture-climate coupling, Geophys. Res. Lett., 38, L16702, https://doi.org/10.1029/2011GL048268, 2011, L16702, 2011.
Dirmeyer, P. A. and Brubaker, K. L.: Characterization of the global hydrologic cycle from a back-trajectory analysis of atmospheric water vapor, J. Hydrometeorol., 8, 20–37, 2007.
Domec, J.-C., King, J. S., Noormets, A., Treasure, E., Gavazzi, M. J., Sun, G., and McNulty, S. G.: Hydraulic redistribution of soil water by roots affects whole-stand evapotranspiration and net ecosystem carbon exchange, New Phytol., 187, 171–183, https://doi.org/10.1111/j.1469-8137.2010.03245.x, 2010.
Dominguez, F., Kumar, P., Liang, X., and Ting, M.: Impact of atmospheric moisture storage on precipitation recycling, J. Climate, 19, 1513–1530, 2006.
Doughty, C. E., Metcalfe, D. B., Girardin, C. A. J., Amézquita, F. F., Cabrera, D. G., Huasco, W. H., Silva-Espejo, J. E., Araujo-Murakami, A., Da Costa, M. C., Rocha, W., and Feldpausch, T. R.: Drought impact on forest carbon dynamics and fluxes in Amazonia, Nature, 519, 78–82, https://doi.org/10.1038/nature14213, 2015.
Drager, A. J. and van den Heever, S. C.: Characterizing convective cold pools, J. Adv. Model. Earth Syst., 9, 1091–1115, https://doi.org/10.1002/2016MS000788, 2017.
Drumond, A., Marengo, J., Ambrizzi, T., Nieto, R., Moreira, L., and Gimeno, L.: The role of the Amazon Basin moisture in the atmospheric branch of the hydrological cycle: a Lagrangian analysis, Hydrol. Earth Syst. Sci., 18, 2577–2598, https://doi.org/10.5194/hess-18-2577-2014, 2014.
Du, S., Liu, L., Liu, X., and Hu, J.: Response of Canopy Solar-Induced Chlorophyll Fluorescence to the Absorbed Photosynthetically Active Radiation Absorbed by Chlorophyll, Remote Sens., 9, 911–919, https://doi.org/10.3390/rs9090911, 2017.
Duveiller, G. and Cescatti, A.: Spatially downscaling sun-induced chlorophyll fluorescence leads to an improved temporal correlation with gross primary productivity, Remote Sens. Environ., 182, 72–89, https://doi.org/10.1016/j.rse.2016.04.027, 2016.
Ek, M. B.: Implementation of Noah land surface model advances in the National Centers for Environmental Prediction operational mesoscale Eta model, J. Geophys. Res., 108, 8851, https://doi.org/10.1029/2002JD003296, 2003.
Eltahir, E. A. B. and Bras, R. L.: Precipitation Recycling in the Amazon Basin, Q. J. Roy. Meteor. Soc., 120, 861–880, 1994.
Engerer, N. A., Stensrud, D. J., and Coniglio, M. C.: Surface Characteristics of Observed Cold Pools, Mon. Weather Rev., 136, 4839–4849, https://doi.org/10.1175/2008MWR2528.1, 2008.
Fan, Y., Miguez-Macho, G., Jobbágy, E. G., Jackson, R. B., and Otero-Casal, C.: Hydrologic regulation of plant rooting depth. P. Natl. Acad. Sci. USA, 114, 10572–10577, 2017.
Feingold, G., Jiang, H. L., and Harrington, J. Y.: On smoke suppression of clouds in Amazonia, Geophys. Res. Lett., 32, https://doi.org/10.1029/2004GL021369, 2005.
Feng, Z., Hagos, S., Rowe, A. K., Burleyson, C. D., Martini, M. N., and de Szoeke, S. P.: Mechanisms of convective cloud organization by cold pools over tropical warm ocean during the AMIE/DYNAMO field campaign, J. Adv. Model. Earth Syst., 7, 357–381, https://doi.org/10.1002/2014MS000384, 2015.
Fisher, J. B., Malhi, Y., Bonal, D., Da Rocha, H. R., De Araujo, A. C., Gamo, M., Goulden, M. L., Hirano, T., Huete, A. R., Kondo, H., and Kumagai, T. O.: The land-atmosphere water flux in the tropics, Global Change Biol., 15, 2694–2714, https://doi.org/10.1111/j.1365-2486.2008.01813.x, 2009.
Fisher, R. A., Williams, M., Do Vale, R. L., Da Costa, A. L., and Meir, P.: Evidence from Amazonian forests is consistent with isohydric control of leaf water potential, Plant Cell Environ., 29, 151–165, 2006.
Fitzjarrald, D. R., Stormwind, B. L., Fisch, G., and Cabral, O. M.: Turbulent transport observed just above the Amazon forest, J. Geophys. Res.-Atmos., 93, 1551–1563, 1988.
Frankenberg, C., Fisher, J. B., Worden, J., Badgley, G., Saatchi, S. S., Lee, J. E., Toon, G. C., Butz, A., Jung, M., Kuze, A., and Yokota, T.: New global observations of the terrestrial carbon cycle from GOSAT: Patterns of plant fluorescence with gross primary productivity, Geophys. Res. Lett., 38, https://doi.org/10.1029/2011GL048738, 2011.
Frankenberg, C., O'Dell, C., Guanter, L., and McDuffie, J.: Remote sensing of near-infrared chlorophyll fluorescence from space in scattering atmospheres: implications for its retrieval and interferences with atmospheric CO2 retrievals, Atmos. Meas. Tech., 5, 2081–2094, https://doi.org/10.5194/amt-5-2081-2012, 2012.
Frankenberg, C., O'Dell, C., Berry, J., Guanter, L., Joiner, J., Köhler, P., Pollock, R., and Taylor, T. E.: Prospects for chlorophyll fluorescence remote sensing from the Orbiting Carbon Observatory-2, Remote Sens. Environ., 147, 1–12, https://doi.org/10.1016/j.rse.2014.02.007, 2014.
Friedlingstein, P., Cox, P., Betts, R., Bopp, L., von Bloh, W., Brovkin, V., Cadule, P., Doney, S., Eby, M., Fung, I., and Bala, G.: Climate–carbon cycle feedback analysis: results from the C4MIP model intercomparison, J. Climate, 19, 3337–3353, 2006.
Fu, R. and Li, W.: The influence of the land surface on the transition from dry to wet season in Amazonia, Theor. Appl. Climatol., 78, 97–110, https://doi.org/10.1007/s00704-004-0046-7, 2004.
Fu, R., Yin, L., Li, W., Arias, P. A., Dickinson, R. E., Huang, L., Chakraborty, S., Fernandes, K., Liebmann, B., Fisher, R., and Myneni, R. B.: Increased dry-season length over southern Amazonia in recent decades and its implication for future climate projection, P. Natl. Acad. Sci. USA, 110, 18110–18115, 2013.
Fu, R., Arias, P. A., and Wang, H.: The Connection Between the North and South American Monsoons, The Monsoons and Climate Change, Springer, Cham., 2016.
Fuentes, J. D., Chamecki, M., Nascimento dos Santos, R. M., and Von Randow, C.: Linking meteorology, turbulence, and air chemistry in the Amazon rain forest, B. Am. Meteorol. Soc., 97, 2329–2342, 2016.
Garstang, M. and Fitzjarrald, D. R.: Observations of surface to atmosphere interactions in the tropics, Oxford University Press, USA, 1999.
Gatti, L. V., Gloor, M., Miller, J. B., Doughty, C. E., Malhi, Y., Domingues, L. G., Basso, L. S., Martinewski, A., Correia, C. S. C., Borges, V. F., and Freitas, S.: Drought sensitivity of Amazonian carbon balance revealed by atmospheric measurements, Nature, 506, 76–80, https://doi.org/10.1038/nature12957, 2015.
Gentine, P., Entekhabi, D., Chehbouni, A., Boulet, G., and Duchemin, B.: Analysis of evaporative fraction diurnal behaviour, Agr. Forest Meteorol., 143, 13–29, https://doi.org/10.1016/j.agrformet.2006.11.002, 2007.
Gentine, P., Holtslag, A., and D'Andrea, F.: Surface and atmospheric controls on the onset of moist convection over land, J. Hydrometeorol., 14, 1443–1462, https://doi.org/10.1175/JHM-D-12-0137.1, 2013a.
Gentine, P., Ferguson, C. R., and Holtslag, A. A. M.: Diagnosing evaporative fraction over land from boundary-layer clouds, J. Geophys. Res.-Atmos., 118, 8185–8196, https://doi.org/10.1002/jgrd.50416, 2013b.
Gentine, P., Garelli, A., Park, S. B., and Nie, J.: Role of surface heat fluxes underneath cold pools, Geophys. Res. Lett., 43, 874–883, https://doi.org/10.1002/2015GL067262, 2016.
Gerken, T., Ruddell, B. L., Fuentes, J. D., Araújo, A., Brunsell, N. A., Maia, J., Manzi, A., Mercer, J., dos Santos, R. N., von Randow, C., and Stoy, P. C.: Investigating the mechanisms responsible for the lack of surface energy balance closure in a central Amazonian tropical rainforest, Agr. Forest Meteorol., 255, 92–103, https://doi.org/10.1016/j.agrformet.2017.03.023, 2017.
Ghate, V. P. and Kollias, P.: On the controls of daytime precipitation in the Amazonian dry season, J. Hydrometeorol., 17, 3079–3097, https://doi.org/10.1175/JHM-D-16-0101.1, 2016.
Giangrande, S. E., Feng, Z., Jensen, M. P., Comstock, J. M., Johnson, K. L., Toto, T., Wang, M., Burleyson, C., Bharadwaj, N., Mei, F., Machado, L. A. T., Manzi, A. O., Xie, S., Tang, S., Silva Dias, M. A. F., de Souza, R. A. F., Schumacher, C., and Martin, S. T.: Cloud characteristics, thermodynamic controls and radiative impacts during the Observations and Modeling of the Green Ocean Amazon (GoAmazon2014/5) experiment, Atmos. Chem. Phys., 17, 14519–14541, https://doi.org/10.5194/acp-17-14519-2017, 2017.
Gimeno, L., Stohl, A., Trigo, R. M., Dominguez, F., Yoshimura, K., Yu, L., Drumond, A., Durán‐Quesada, A. M., and Nieto, R.: Oceanic and terrestrial sources of continental precipitation, Rev. Geophys., 50, https://doi.org/10.1029/2012RG000389, 2012.
Goulden, M. L., Miller, S. D., and da Rocha, H. R.: Diel and seasonal patterns of tropical forest CO2 exchange, Ecological, 14, 42–54, 2004.
Goutorbe, J.-P., Lebel, T., Tinga, A., Bessemoulin, P., Brouwer, J., Dolman, A. J., Engman, E. T., Gash, J. H. C., Hoepffner, M., Kabat, P., Kerr, Y. H., Monteny, B., Prince, S., Said, F., Sellers, P., and Wallace, J. S.: HAPEX-Sahel: a large-scale study of land-atmosphere interactions in the semi-arid tropics, Ann. Geophys., 12, 53–64, https://doi.org/10.1007/s00585-994-0053-0, 1994.
Grabowski, W. W. and Smolarkiewicz, P. K.: CRCP: A cloud resolving convection parameterization for modeling the tropical convecting atmosphere, Physica D: Nonlinear Phenomena, 133, 171–178, 1999.
Green, J. K., Konings, A. G., Alemohammad, S. H., Berry, J., Entekhabi, D., Kolassa, J., Lee, J.-E., and Gentine, P.: Regionally strong feedbacks between the atmosphere and terrestrial biosphere, Nat. Geosci., 48, 1–12, https://doi.org/10.1038/ngeo2957, 2017.
Green, J., Seneviratne, S. I., Berg, A. A., Findell, K. L., Hagemann, S., Lawrence, D. M., and Gentine, P.: Large influence of soil moisture on long-term terrestrial carbon uptake, Nature, 565, 476, 2019.
Guan, K. and Liang, M.: Photosynthetic seasonality of global tropical forests constrained by hydroclimate, Nat. Geosci., 8, 284–289, 2015.
Guanter, L., Zhang, Y., Jung, M., Joiner, J., Voigt, M., Berry, J. A., Frankenberg, C., Huete, A. R., Zarco-Tejada, P., Lee, J. E., and Moran, M. S.: Global and time-resolved monitoring of crop photosynthesis with chlorophyll fluorescence, P. Natl. Acad. Sci. USA, 111, E1327–E1333, https://doi.org/10.1073/pnas.1320008111, 2014.
Guichard, F., Petch, J. C., Redelsperger, J. L., Bechtold, P., Chaboureau, J. P., Cheinet, S., Grabowski, W., Grenier, H., Jones, C. G., Köhler, M., and Piriou, J. M.: Modelling the diurnal cycle of deep precipitating convection over land with cloud-resolving models and single-column models, Q. J. Roy. Meteor. Soc., 130, 3139–3172, https://doi.org/10.1256/qj.03.145, 2004.
Guillod, B. P., Orlowsky, B., Miralles, D., Teuling, A. J., Blanken, P. D., Buchmann, N., Ciais, P., Ek, M., Findell, K. L., Gentine, P., Lintner, B. R., Scott, R. L., Van den Hurk, B., and I. Seneviratne, S.: Land-surface controls on afternoon precipitation diagnosed from observational data: uncertainties and confounding factors, Atmos. Chem. Phys., 14, 8343–8367, https://doi.org/10.5194/acp-14-8343-2014, 2014.
Guillod, B. P., Orlowsky, B., Miralles, D. G., Teuling, A. J., and Seneviratne, S. I.: Reconciling spatial and temporal soil moisture effects on afternoon rainfall, Nat. Commun., 6, 6443, https://doi.org/10.1038/ncomms7443, 2015.
Hadden, D. and Grelle A.: Changing temperature response of respiration turns boreal forest from carbon sink into carbon source, Agr. Forest Meteorol., 223, 30–38, https://doi.org/10.1016/j.agrformet.2016.03.020, 2016.
Hamada, J.-I., Yamanaka, M. D., Mori, S., Tauhid, Y. I., and Sribimawati, T.: Differences of Rainfall Characteristics between Coastal and Interior Areas of Central Western Sumatera, Indonesia, J. Meteorol. Soc. Jpn., 86, 593–611, https://doi.org/10.2151/jmsj.86.593, 2008.
Han, X., Franssen H.-J. H., Montzka, C., and Vereecken, H.: Soil moisture and soil properties estimation in the Community Land Model with synthetic brightness temperature observations, Water Resour. Res., 50, 6081–6105, https://doi.org/10.1002/2013WR014586, 2014.
Harris, P. P., Huntingford, C., Cox, P. M., Gash, J. H. C., and Malhi, Y.: Effect of soil moisture on canopy conductance of Amazonian rainforest, Agr. Forest Meteorol., 122, 215–227, https://doi.org/10.1016/j.agrformet.2003.09.006, 2004.
Haverd, V., Cuntz, M., Nieradzik, L. P., and Harman, I. N.: Improved representations of coupled soil–canopy processes in the CABLE land surface model (Subversion revision 3432), Geosci. Model Dev., 9, 3111–3122, https://doi.org/10.5194/gmd-9-3111-2016, 2016.
Heroult, A., Lin, Y., and Bourne, A.: Optimal stomatal conductance in relation to photosynthesis in climatically contrasting Eucalyptus species under drought, Plant Cell Environ., 36, 262–274, https://doi.org/10.1111/j.1365-3040.2012.02570.x, 2013.
Hidayat, R. and Kizu, S.: Influence of the Madden-Julian Oscillation on Indonesian rainfall variability in austral summer, Int. J. Climatol., 23, https://doi.org/10.1002/joc.2005, 2009.
Hilker, T., Lyapustin, A. I., Tucker, C. J., Hall, F. G., Myneni, R. B., Wang Y., Bi, J., Mendes de Moura, Y., and Sellers, P. J.: Vegetation dynamics and rainfall sensitivity of the Amazon, P. Natl. Acad. Sci. USA, 111, 16041–16046, https://doi.org/10.1073/pnas.1404870111, 2014.
Hohenegger, C. and Bretherton, C. S.: Simulating deep convection with a shallow convection scheme, Atmos. Chem. Phys., 11, 10389–10406, https://doi.org/10.5194/acp-11-10389-2011, 2011.
Horn, G. L., Ouwersloot, H. G., de Arellano, J. V.-G., and Sikma, M.: Cloud Shading Effects on Characteristic Boundary-Layer Length Scales, Bound.-Lay. Meteorol., 157, 237–263, https://doi.org/10.1007/s10546-015-0054-4, 2015.
Hoyos, I., Dominguez, F., Cañón-Barriga, J., Martínez, J. A., Nieto, R., Gimeno, L., and Dirmeyer, P. A.: Moisture origin and transport processes in Colombia, northern South America, Clim. Dynam., 50, 971–990, 2018.
Huang, R. and Sun, F.: Impacts of the Tropical Western Pacific on the East Asian Summer Monsoon, J. Meteor. Soc. Jpn., 70, 243–256, https://doi.org/10.2151/jmsj1965.70.1B_243, 1992.
Huete, A. R., Didan, K., Shimabukuro, Y. E., Ratana, P., Saleska, S. R., Hutyra, L. R., Yang, W., Nemani, R. R., and Myneni, R.: Amazon rainforests green-up with sunlight in dry season, Geophys. Res. Lett., 33, L06405, https://doi.org/10.1029/2005GL025583, 2006.
Huffman, G. J., Adler, R. F., Morrissey, M. M., Bolvin, D. T., Curtis, S., Joyce, R., McGavock, B., and Susskind, J.: Global precipitation at one-degree daily resolution from multisatellite observations, J. Hydrometeorol., 2, 36–50, https://doi.org/10.1175/1525-7541(2001)002<0036:GPAODD>2.0.CO;2, 2001.
Huijnen, V., Wooster, M. J., Kaiser, J. W., Gaveau, D. L. A., Flemming, J., Parrington, M., Inness, A., Murdiyarso, D., Main, B., and van Weele, M.: Fire carbon emissions over maritime southeast Asia in 2015 largest since 1997, Sci. Rep. UK, 6, 1–8, https://doi.org/10.1038/srep26886, 2016.
Jardine, K., Chambers, J., Alves, E. G., Teixeira, A., Garcia, S., Holm, J., Higuchi, N., Manzi, A., Abrell, L., Fuentes, J. D., and Nielsen, L. K.: Dynamic Balancing of Isoprene Carbon Sources Reflects Photosynthetic and Photorespiratory Responses to Temperature Stress, Plant Physiol., 166, 2051–2064, https://doi.org/10.1104/pp.114.247494, 2014.
Jimenez, C., Prigent, C., Mueller, B., Seneviratne, S. I., McCabe, M. F., Wood, E. F., Rossow, W. B., Balsamo, G., Betts, A. K., Dirmeyer, P. A., and Fisher, J. B.: Global intercomparison of 12 land surface heat flux estimates, J. Geophys. Res., 116, 1–27, https://doi.org/10.1029/2010JD014545, 2011.
Joiner, J., Yoshida, Y., Vasilkov, A. P., Yoshida, Y., Corp, L. A., and Middleton, E. M.: First observations of global and seasonal terrestrial chlorophyll fluorescence from space, Biogeosciences, 8, 637–651, https://doi.org/10.5194/bg-8-637-2011, 2011.
Joiner, J., Guanter, L., Lindstrot, R., Voigt, M., Vasilkov, A. P., Middleton, E. M., Huemmrich, K. F., Yoshida, Y., and Frankenberg, C.: Global monitoring of terrestrial chlorophyll fluorescence from moderate-spectral-resolution near-infrared satellite measurements: methodology, simulations, and application to GOME-2, Atmos. Meas. Tech., 6, 2803–2823, https://doi.org/10.5194/amt-6-2803-2013, 2013.
Juárez, R. I. N., Hodnett, M. G., Fu, R., Goulden, M. L., and von Randow, C.: Control of Dry Season Evapotranspiration over the Amazonian Forest as Inferred from Observations at a Southern Amazon Forest Site, J. Climate, 20, 2827–2839, https://doi.org/10.1175/JCLI4184.1, 2007.
Jung, M., Reichstein, M., and Bondeau, A.: Towards global empirical upscaling of FLUXNET eddy covariance observations: validation of a model tree ensemble approach using a biosphere model, Biogeosciences, 6, 2001–2013, https://doi.org/10.5194/bg-6-2001-2009, 2009.
Jung, M., Reichstein, M., Schwalm, C. R., Huntingford, C., Sitch, S., Ahlström, A., Arneth, A., Camps-Valls, G., Ciais, P., Friedlingstein, P., and Gans, F.: Compensatory water effects link yearly global land CO2 sink changes to temperature, 541, 516–520, https://doi.org/10.1038/nature20780, 2017.
Kato, S., Loeb, N. G., Rose, F. G., Doelling, D. R., Rutan, D. A., Caldwell, T. E., Yu, L., and Weller, R. A.: Surface Irradiances Consistent with CERES-Derived Top-of-Atmosphere Shortwave and Longwave Irradiances, J. Climate, 26, 2719–2740, https://doi.org/10.1175/JCLI-D-12-00436.1, 2013.
Keller, M., Alencar, A., Asner, G. P., Braswell, B., Bustamante, M., Davidson, E., Feldpausch, T., Fernandes, E., Goulden, M., Kabat, P., and Kruijt, B.: Ecological Research in the Large-Scale Biosphere–Atmosphere Experiment in Amazonia: Early Results, Ecol. Appl., 14, 3–16, 2004.
Kennedy, D., Swenson, S., Oleson, K. W., Lawrence, D. M., Fisher, R., Lola da Costa, A. C., and Gentine, P.: Implementing plant hydraulics in the Community Land Model, version 5, J. Adv. Model. Earth Sy., 11, 485–513, 2019.
Khairoutdinov, M. and Randall, D.: High-resolution simulation of shallow-to-deep convection transition over land, J. Atmos. Sci., 63, 3421–3436, 2006.
Khanna, J., Medvigy, D., Fueglistaler, S., and Walko, R.: Regional dry-season climate changes due to three decades of Amazonian deforestation, Nat. Clim. Change, 7, 200–204, https://doi.org/10.1038/nclimate3226, 2017.
Knox, R. G., Longo, M., Swann, A. L. S., Zhang, K., Levine, N. M., Moorcroft, P. R., and Bras, R. L.: Hydrometeorological effects of historical land-conversion in an ecosystem-atmosphere model of Northern South America, Hydrol. Earth Syst. Sci., 19, 241–273, https://doi.org/10.5194/hess-19-241-2015, 2015.
Konings, A. G. and Gentine, P.: Global variations in ecosystem-scale isohydricity, Global Change Biol., 23, 891–905, https://doi.org/10.1111/gcb.13389, 2017.
Köppen, W.: The thermal zones of the Earth according to the duration of hot, moderate and cold periods and of the impact of heat on the organic world, Meteorol. Z., 1, 215–226, 1884.
Koren, I., Kaufman, Y. J., Remer, L. A., and Martins, J. V.: Measurement of the effect of Amazon smoke on inhibition of cloud formation, Science, 303, 1342–1345, https://doi.org/10.1126/science.1089424, 2004.
Koster, R. D., Dirmeyer, P. A., Guo, Z., Bonan, G., Chan, E., Cox, P., Gordon, C. T., Kanae, S., Kowalczyk, E., Lawrence, D., and Liu, P.: Regions of strong coupling between soil moisture and precipitation, Science, 305, 1138–1140, 2004.
Koster, R. D., Dirmeyer, P. A., Guo, Z., Bonan, G., Chan, E., Cox, P., Gordon, C. T., Kanae, S., Kowalczyk, E., Lawrence, D., and Liu, P.: The Second Phase of the Global Land–Atmosphere Coupling Experiment: Soil Moisture Contributions to Subseasonal Forecast Skill, J. Hydrometeorol., 12, 805–822, https://doi.org/10.1175/2011JHM1365.1, 2011.
Krakauer, N. Y., Puma, M. J., Cook, B. I., Gentine, P., and Nazarenko, L.: Ocean–atmosphere interactions modulate irrigation's climate impacts, Earth Syst. Dynam., 7, 863–876, https://doi.org/10.5194/esd-7-863-2016, 2016.
Kuang, Z.: A Moisture-Stratiform Instability for Convectively Coupled Waves, J. Atmos. Sci., 65, 834–854, https://doi.org/10.1175/2007JAS2444.1, 2008.
Kuang, Z.: Linear response functions of a cumulus ensemble to temperature and moisture perturbations and implications for the dynamics of convectively coupled waves, J. Atmos. Sci., 67, 941–962, 2010.
Laan-Luijkx, I. T., Velde, I. R., Krol, M. C., Gatti, L. V., Domingues, L. G., Correia, C. S. C., Miller, J. B., Gloor, M., Leeuwen, T. T., Kaiser, J. W., and Wiedinmyer, C.: Response of the Amazon carbon balance to the 2010 drought derived with CarbonTracker South America, Global Biogeochem. Cy., 29, 1092–1108, https://doi.org/10.1002/2014GB005082, 2015.
Laurent, H., Machado, L. A., Morales, C. A., and Durieux, L.: Characteristics of the Amazonian mesoscale convective systems observed from satellite and radar during the WETAMC/LBA experiment, J. Geophys. Res.-Atmos., 107, 1–10, 2002.
Lawrence, D. and Vandecar, K.: Effects of tropical deforestation on climate and agriculture, Nat. Clim. Change, 5, 27–36, https://doi.org/10.1038/nclimate2430, 2015.
Lawrence, D. M., Oleson, K. W., and Flanner, M. G.: Parameterization improvements and functional and structural advances in Version 4 of the Community Land Model, J. Adv. Model. Earth Sy., 3, 1–27, 2011.
Lawton, R. O., Nair, U. S., Pielke Sr., R. A., and Welch, R. M.: Climatic Impact of Tropical Lowland Deforestation on Nearby Montane Cloud Forests, Science, 294, 584–587, 2001.
Lebel, T., Cappelaere, B., Galle, S., Hanan, N., Kergoat, L., Levis, S., Vieux, B., Descroix, L., Gosset, M., Mougin, E., and Peugeot, C.: AMMA-CATCH studies in the Sahelian region of West-Africa: An overview, J. Hydrol., 375, 3–13, https://doi.org/10.1016/j.jhydrol.2009.03.020, 2009.
Lee, J. E., Oliveira, R. S., Dawson, T. E., and Fung, I.: Root functioning modifies seasonal climate, P. Natl. Acad. Sci. USA, 102, 17576–17581, 2005.
Lee, J.-E., Berry, J. A., van der Tol, C., Yang, X., Guanter, L., Damm, A., Baker, I., and Frankenberg, C.: Simulations of chlorophyll fluorescence incorporated into the Community Land Model version 4, Global Change Biol., 21, 3469–3477, https://doi.org/10.1111/gcb.12948, 2015.
Leuning, R.: A critical appraisal of a combined stomatal-photosynthesis model for C3 plants, Plant Cell Environ., 18, 339–355, 1995.
Leuning, R., Kelliher, F. M., De Pury, D. G. G., and Schulze, E. D.: Leaf nitrogen, photosynthesis, conductance and transpiration: scaling from leaves to canopies, Plant Cell Environ., 18, 1183–1200, https://doi.org/10.1111/j.1365-3040.1995.tb00628.x, 1995.
Levy, M. C., Cohn, A., Lopes, A. V., and Thompson, S. E.: Addressing rainfall data selection uncertainty using connections between rainfall and streamflow, Sci. Rep. UK, 7, 1–12, https://doi.org/10.1038/s41598-017-00128-5, 2017.
Li, W., Fu, R., and Dickinson, R. E.: Rainfall and its seasonality over the Amazon in the 21st century as assessed by the coupled models for the IPCC AR4, J. Geophys. Res., 111, 1–12, 2006.
Liebmann, B. and Marengo, J. A.: Interannual variability of the rainy season and rainfall in the Brazilian Amazon basin, J. Climate, 14, 4308–4318, 2001.
Lintner, B. R. and Neelin, J. D.: A prototype for convective margin shifts, Geophys. Res. Lett., 34, L05812, https://doi.org/10.1029/2006GL027305, 2007.
Lintner, B. R. and Neelin, J. D.: Soil Moisture Impacts on Convective Margins, J. Hydrometeorol., 10, 1026–1039, https://doi.org/10.1175/2009JHM1094.1, 2009.
Lintner, B. R., Gentine, P., Findell, K. L., D'Andrea, F., Sobel, A. H., and Salvucci, G. D.: An idealized prototype for large-scale land-atmosphere coupling, J. Climate, 26, 2379–2389, https://doi.org/10.1175/JCLI-D-11-00561.1, 2013.
Lintner, B. R., Gentine, P., Findell, K. L., and Salvucci, G. D.: The Budyko and complementary relationships in an idealized model of large-scale land–atmosphere coupling, Hydrol. Earth Syst. Sci., 19, 2119–2131, https://doi.org/10.5194/hess-19-2119-2015, 2015.
Lintner, B. R., Adams, D. K., Schiro, K. A., Stansfield, A. M., Amorim Rocha, A. A., and Neelin, J. D.: Relationships among climatological vertical moisture structure, column water vapor, and precipitation over the central Amazon in observations and CMIP5 models, Geophys. Res. Lett., 44, 1981–1989, 2017.
Liu, H., Gleason, S. M., Hao, G., Hua, L., He, P., Goldstein, G., and Ye, Q.: Hydraulic traits are coordinated with maximum plant height at the global scale, Science Advances, 5, eaav1332, https://doi.org/10.1126/sciadv.aav1332, 2019.
Liu, L., Guan, L., and Liu, X.: Directly estimating diurnal changes in GPP for C3 and C4 crops using far-red sun-induced chlorophyll fluorescence, Agr. Forest Meteorol., 232, 1–9, https://doi.org/10.1016/j.agrformet.2016.06.014, 2017.
Lohou, F., Said, F., Lothon, M., Durand, P., and Serça, D.: Impact of Boundary-Layer Processes on Near-Surface Turbulence Within the West African Monsoon, Bound.-Lay. Meteorol., 136, 1–23, https://doi.org/10.1007/s10546-010-9493-0, 2010.
Lopes, A. P., Nelson, B. W., Wu, J., de Alencastro Graça, P. M. L., Tavares, J. V., Prohaska, N., Martins, G. A., and Saleska, S. R.: Leaf flush drives dry season green-up of the Central Amazon, Remote Sens. Env., 182, 90–98, https://doi.org/10.1016/j.rse.2016.05.009, 2016.
Machado, L. and Laurent, H.: Diurnal march of the convection observed during TRMM-WETAMC/LBA, J. Geophys. Res.-Atmos., 107, 1–13, 2002.
Mahecha, M. D., Reichstein, M., Carvalhais, N., Lasslop, G., Lange, H., Seneviratne, S. I., Vargas, R., Ammann, C., Arain, M. A., Cescatti, A., and Janssens, I. A.: Global convergence in the temperature sensitivity of respiration at ecosystem level, Science, 329, 838–840, https://doi.org/10.1126/science.1189587, 2010.
Malhi, Y., Nobre, A. D., Grace, J., Kruijt, B., Pereira, M. G. P., Culf, A., and Scott, S.: Carbon dioxide transfer over a Central Amazonian rain forest, J. Geophys. Res.-Atmos., 103, 31593–31612, https://doi.org/10.1029/98jd02647, 1998.
Marengo, J. A., Tomasella, J., Alves, L. M., Soares, W. R., and Rodriguez, D. A.: The drought of 2010 in the context of historical droughts in the Amazon region, Geophys. Res. Lett., 38, https://doi.org/10.1029/2011GL047436, 2011.
Martin, S. T., Artaxo, P., Machado, L. A. T., Manzi, A. O., Souza, R. A. F., Schumacher, C., Wang, J., Andreae, M. O., Barbosa, H. M. J., Fan, J., Fisch, G., Goldstein, A. H., Guenther, A., Jimenez, J. L., Pöschl, U., Silva Dias, M. A., Smith, J. N., and Wendisch, M.: Introduction: Observations and Modeling of the Green Ocean Amazon (GoAmazon2014/5), Atmos. Chem. Phys., 16, 4785–4797, https://doi.org/10.5194/acp-16-4785-2016, 2016.
Martin, S. T., Artaxo, P., Machado, L., Manzi, A. O., Souza, R. A. F., Schumacher, C., Wang, J., Biscaro, T., Brito, J., Calheiros, A., and Jardine, K.: The Green Ocean Amazon Experiment (GoAmazon2014/5) Observes Pollution Affecting Gases, Aerosols, Clouds, and Rainfall over the Rain Forest, B. Am. Meteorol. Soc., 98, 981–997, https://doi.org/10.1175/BAMS-D-15-00221.1, 2017.
Martinez-Vilalta, J. and Garcia-Forner, N.: Water potential regulation, stomatal behaviour and hydraulic transport under drought: deconstructing the iso/anisohydric concept, Plant Cell Environ., 40, 962–976, https://doi.org/10.1111/pce.12846, 2016.
Martinez-Vilalta, J., Poyatos, R., Aguadé, D., Retana, J., and Mencuccini, M.: A new look at water transport regulation in plants, New Phytol., 204, 105–115, https://doi.org/10.1111/nph.12912, 2014.
Maxwell, R. M. and Condon, L. E.: Connections between groundwater flow and transpiration partitioning, Science, 353, 377–380, 2016.
Medlyn, B. E., Duursma, R. A., Eamus, D., Ellsworth, D. S., Prentice, I. C., Barton, C. V. M., Crous, K. Y., De Angelis, P., Freeman, M., and Wingate, L.: Reconciling the optimal and empirical approaches to modelling stomatal conductance, Global Change Biol., 17, 2134–2144, https://doi.org/10.1111/j.1365-2486.2010.02375.x, 2011.
Medvigy, D., Walko, R. L., and Avissar, R.: Effects of Deforestation on Spatiotemporal Distributions of Precipitation in South America, J. Climate, 24, 2147–2163, 2011.
Miralles, D. G., Gash, J. H., Holmes, T. R. H., de Jeu, R. A. M., and Dolman, A. J.: Global canopy interception from satellite observations, J. Geophys. Res., 115, 237, https://doi.org/10.1029/2009JD013530, 2010.
Morton, D. C., Nagol, J., Carabajal, C. C., Rosette, J., Palace, M., Cook, B. D., Vermote, E. F., Harding, D. J., and North, P. R. J.: Amazon forests maintain consistent canopy structure and greenness during the dry season, Nature, 506, 221–224, https://doi.org/10.1038/nature13006, 2014.
Morton, D. C., Rubio, J., Cook, B. D., Gastellu-Etchegorry, J.-P., Longo, M., Choi, H., Hunter, M., and Keller, M.: Amazon forest structure generates diurnal and seasonal variability in light utilization, Biogeosciences, 13, 2195–2206, https://doi.org/10.5194/bg-13-2195-2016, 2016.
Mueller, B., Seneviratne, S. I., Jimenez, C., Corti, T., Hirschi, M., Balsamo, G., Ciais, P., Dirmeyer, P., Fisher, J. B., Guo, Z., and Jung, M.: Evaluation of global observations-based evapotranspiration datasets and IPCC AR4 simulations, Geophys. Res. Lett., 38, https://doi.org/10.1029/2010GL046230, 2011.
Munger, J. W., McManus, J. B., Nelson, D. D., Zahniser, M. S., Davidson, E. A., Wofsy, S. C., Wehr, R., and Saleska, S. R.: Seasonality of temperate forest photosynthesis and daytime respiration, Nature, 534, 680–683, https://doi.org/10.1038/nature17966, 2016.
Nakicenovic, N. and Swart, R.: Emissions scenarios, Special report of the Intergovernmental panel on climate change, 2000.
Naudts, K., Ryder, J., McGrath, M. J., Otto, J., Chen, Y., Valade, A., Bellasen, V., Berhongaray, G., Bönisch, G., Campioli, M., Ghattas, J., De Groote, T., Haverd, V., Kattge, J., MacBean, N., Maignan, F., Merilä, P., Penuelas, J., Peylin, P., Pinty, B., Pretzsch, H., Schulze, E. D., Solyga, D., Vuichard, N., Yan, Y., and Luyssaert, S.: A vertically discretised canopy description for ORCHIDEE (SVN r2290) and the modifications to the energy, water and carbon fluxes, Geosci. Model Dev., 8, 2035–2065, https://doi.org/10.5194/gmd-8-2035-2015, 2015.
Ngo-Duc, T., Laval, K., Ramillien, G., Polcher, J., and Cazenave, A.: Validation of the land water storage simulated by Organising Carbon and Hydrology in Dynamic Ecosystems (ORCHIDEE) with Gravity Recovery and Climate Experiment (GRACE) data, Water Resour. Res., 43, https://doi.org/10.1029/2006WR004941, 2007.
Nicholson, S.: Land surface processes and Sahel climate, Rev. Geophys., 38, 117–139, 2000.
Nitta, T.: Convective Activities in the Tropical Western Pacific and Their Impact on the Northern Hemisphere Summer Circulation, J. Meteorol. Soc. Jpn., 65, 373–390, https://doi.org/10.2151/jmsj1965.65.3_373, 1987.
Noilhan, J. and Planton, S.: A simple parameterization of land surface processes for meteorological models, Mon. Weather Rev., 117, 536–549, 1989.
Norton, A. J., Rayner, P. J., Koffi, E. N., and Scholze, M.: Assimilating solar-induced chlorophyll fluorescence into the terrestrial biosphere model BETHY-SCOPE v1.0: model description and information content, Geosci. Model Dev., 11, 1517–1536, https://doi.org/10.5194/gmd-11-1517-2018, 2018.
Oleson, K. W., Niu, G. Y., Yang, Z. L., Lawrence, D. M., Thornton, P. E., Lawrence, P. J., Stöckli, R., Dickinson, R. E., Bonan, G. B., Levis, S., and Dai, A.: Improvements to the Community Land Model and their impact on the hydrological cycle, J. Geophys. Res.-Biogeo., 113, https://doi.org/10.1029/2007JG000563, 2008.
Oliveira, R. S., Dawson, T. E., Burgess, S. S. O., and Nepstad, D. C.: Hydraulic redistribution in three Amazonian trees, Oecologia, 145, 354–363, https://doi.org/10.1007/s00442-005-0108-2, 2005.
Ometto, J. P. H. B., Ehleringer, J. R., Domingues, T. F., Berry, J. A., Ishida, F. Y., Mazzi, E., Higuchi, N., Flanagan, L. B., Nardoto, G. B., and Martinelli, L. A.: The stable carbon and nitrogen isotopic composition of vegetation in tropical forests of the Amazon Basin, Brazil, Biogeochemistry, 79, 251–274, https://doi.org/10.1007/s10533-006-9008-8, 2006.
Ouwersloot, H. G., de Arellano, J. V.-G., van Stratum, B. J. H., Krol, M. C., and Lelieveld, J.: Quantifying the transport of sub-cloud layer reactants by shallow cumulus clouds over the Amazon, J. Geophys. Res.-Atmos., 118, 13–41, https://doi.org/10.1002/2013JD020431, 2013.
Pagán, B. R., Maes, W. H., Gentine, P., Martens, B., and Miralles, D. G.: Exploring the Potential of Satellite Solar-Induced Fluorescence to Constrain Global Transpiration Estimates, Remote Sens., 11, 413, https://doi.org/10.3390/rs11040413, 2019.
Park, S. and Bretherton, C. S.: The University of Washington Shallow Convection and Moist Turbulence Schemes and Their Impact on Climate Simulations with the Community Atmosphere Model, J. Climate, 22, 3449–3469, https://doi.org/10.1175/2008JCLI2557.1, 2009.
Park, S.-B., Boeing, S., and Gentine, P.: Role of shear on shallow convection J. Atmos. Sci., 75, 163–178, 2018.
Pedruzo-Bagazgoitia, X., Ouwersloot, H. G., Sikma, M., van Heerwaarden, C. C., Jacobs, C. M., and Vilà-Guerau de Arellano, J.: Direct and diffuse radiation in the shallow cumulus-vegetation system: enhanced and decreased evapotranspiration regimes, J. Hydrometeorol., 18, 1731–1748, 2017.
Peterhansel, C. and Maurino, V. G.: Photorespiration Redesigned, Plant Physiol., 155, 49–55, https://doi.org/10.1104/pp.110.165019, 2011.
Phillips, N., Nagchaudhuri, A., Oren, R., and Katul, G.: Time constant for water transport in loblolly pine trees estimated from time series of evaporative demand and stem sapflow, Trees, 11, 412–419, 1997.
Phillips, N. G., Oren, R., Licata, J., and Linder, S.: Time series diagnosis of tree hydraulic characteristics, Tree Physiol., 24, 879–890, 2004.
Phillips, N. G., Buckley, T. N., and Tissue, D. T.: Capacity of Old Trees to Respond to Environmental Change, J. Integr. Plant Biol., 50, 1355–1364, https://doi.org/10.1111/j.1744-7909.2008.00746.x, 2008.
Pielke, R. and Avissar, R.: Influence of landscape structure on local and regional climate, Landscape Ecol., 4, 133–155, 1990.
Pielke, R., Dalu, G. Snook, J., Lee, T., and Kittel, T.: Nonlinear Influence of Mesoscale Land-Use on Weather and Climate, J. Climate, 4, 1053–1069, 1991.
Pielke Sr., R. A., Pitman, A., Niyogi, D., Mahmood, R., McAlpine, C., Hossain, F., Goldewijk, K. K., Nair, U., Betts, R., Fall, S., and Reichstein, M.: Land use/land cover changes and climate: modeling analysis and observational evidence, Wires Clim. Change, 2, 828–850, https://doi.org/10.1002/wcc.144, 2011.
Pielke Sr., R. A., Mahmood, R., and McAlpine, C.: Land's complex role in climate change, Phys. Today, 69, 40–46, https://doi.org/10.1063/PT.3.3364, 2016.
Pons, T. L. and Welschen, R.: Midday depression of net photosynthesis in the tropical rainforest tree Eperua grandiflora: contributions of stomatal and internal conductances, respiration and Rubisco functioning, Tree Physiol., 23, 937–947, 2003.
Poulter, B., Frank, D., Ciais, P., Myneni, R. B., Andela, N., Bi, J., Broquet, G., Canadell, J. G., Chevallier, F., Liu, Y. Y., and Running, S. W.: Contribution of semi-arid ecosystems to interannual variability of the global carbon cycle, Nature, 509, 600–603, https://doi.org/10.1038/nature13376, 2014.
Powell, T. L., Galbraith, D. R., Christoffersen, B. O., Harper, A., Imbuzeiro, H. M., Rowland, L., Almeida, S., Brando, P. M., da Costa, A. C. L., Costa, M. H., and Levine, N. M.: Confronting model predictions of carbon fluxes with measurements of Amazon forests subjected to experimental drought, New Phytol., 200, 350–365, https://doi.org/10.1111/nph.12390, 2013.
Prieto, I. and Ryel, R. J.: Internal hydraulic redistribution prevents the loss of root conductivity during drought, Tree Physiol., 34, 39–48, https://doi.org/10.1093/treephys/tpt115, 2014.
Ray, D. K., Nair, U. S., Lawton, R. O., Welch, R. M., and Pielke Sr., R. A.: Impact of land use on Costa Rican tropical montane cloud forests: Sensitivity of orographic cloud formation to deforestation in the plains, J. Geophys. Res., 111, D02108, https://doi.org/10.1029/2005JD006096, 2006.
Redelsperger, J.-L., Thorncroft, C. D., Diedhiou, A., Lebel, T., Parker, D. J., and Polcher, J.: African monsoon multidisciplinary analysis – An international research project and field campaign, B. Am. Meteorol. Soc., 87, 1739–1746, https://doi.org/10.1175/BAMS-87-12-1739, 2006.
Restrepo-Coupe, N., da Rocha, H. R., Hutyra, L. R., da Araujo, A. C., Borma, L. S., Christoffersen, B., Cabral, O. M., de Camargo, P. B., Cardoso, F. L., da Costa, A. C. L., and Fitzjarrald, D. R.: What drives the seasonality of photosynthesis across the Amazon basin? A cross-site analysis of eddy flux tower measurements from the Brasil flux network, Agr. Forest Meteorol., 182–183, 128–144, https://doi.org/10.1016/j.agrformet.2013.04.031, 2013.
Rickenbach, T. M., Ferreira, R. N., Halverson, J. B., Herdies, D. L., and Dias, M. A. S.: Modulation of convection in the southwestern Amazon basin by extratropical stationary fronts, J. Geophys. Res.-Atmos., 107, 1–10, 2002.
Rieck, M., Hohenegger, C., and van Heerwaarden, C. C.: The influence of land surface heterogeneities on cloud size development, Mon. Weather Rev., 142, 3830–3846, https://doi.org/10.1175/MWR-D-13-00354.1, 2014.
Rieck, M., Hohenegger, C., and Gentine, P.: The effect of moist convection on thermally induced mesoscale circulations, Q. J. Roy. Meteor. Soc., 141, 2418–2428, https://doi.org/10.1002/qj.2532, 2015.
Rio, C., Hourdin, F., Grandpeix, J. Y., and Lafore, J. P.: Shifting the diurnal cycle of parameterized deep convection over land, Geophys. Res. Lett., 36, https://doi.org/10.1029/2008GL036779, 2009.
Rochetin, N., Couvreux, F., Grandpeix, J.-Y., and Rio, C.: Deep Convection Triggering by Boundary Layer Thermals. Part I: LES Analysis and Stochastic Triggering Formulation, J. Atmos. Sci., 71, 496–514, https://doi.org/10.1175/JAS-D-12-0336.1, 2014a.
Rochetin, N., Grandpeix, J.-Y., Rio, C., and Couvreux, F.: Deep Convection Triggering by Boundary Layer Thermals. Part II: Stochastic Triggering Parameterization for the LMDZ GCM, J. Atmos. Sci., 71, 515–538, https://doi.org/10.1175/JAS-D-12-0337.1, 2014b.
Roy, S., Weaver, C., Nolan, D., and Avissar, R.: A preferred scale for landscape forced mesoscale circulations? J. Geophys. Res.-Atmos., 108, 8854, https://doi.org/10.1029/2002JD003097, 2003.
Saatchi, S., Asefi-Najafabady, S., Malhi, Y. Aragao, L. E. O. C., Anderson, L. O., Myneni, R. B., and Nemani, R.: Persistent effects of a severe drought on Amazonian forest canopy, P. Natl. Acad. Sci. USA, 110, 565–570, https://doi.org/10.1073/pnas.1204651110, 2013.
Saleska, S. R., Miller, S. D., Matross, D. M., Goulden, M. L., Wofsy, S. C., Da Rocha, H. R., De Camargo, P. B., Crill, P., Daube, B. C., De Freitas, H. C., and Hutyra, L.: Carbon in Amazon forests: unexpected seasonal fluxes and disturbance-induced losses, Science, 302, 1554–1557, 2003.
Saleska, S. R., Wu, J., Guan, K., Araujo, A. C., and Huete, A.: Dry-season greening of Amazon forests, Nature, 531, 7594, https://doi.org/10.1038/nature16457, 2016.
Sánchez Gácita, M., Longo, K. M., Freire, J. L. M., Freitas, S. R., and Martin, S. T.: Impact of mixing state and hygroscopicity on CCN activity of biomass burning aerosol in Amazonia, Atmos. Chem. Phys., 17, 2373–2392, https://doi.org/10.5194/acp-17-2373-2017, 2017.
Schaefli, B., van der Ent, R. J., Woods, R., and Savenije, H. H. G.: An analytical model for soil-atmosphere feedback, Hydrol. Earth Syst. Sci., 16, 1863–1878, https://doi.org/10.5194/hess-16-1863-2012, 2012.
Schiro, K. A., Neelin, J. D., Adams, D. K., and Lintner, B. R.: Deep Convection and Column Water Vapor over Tropical Land versus Tropical Ocean: A Comparison between the Amazon and the Tropical Western Pacific, J. Atmos. Sci., 73, 4043–4063, https://doi.org/10.1175/JAS-D-16-0119.1, 2016.
Scholz, F., Phillips, N., and Bucci, S.: Hydraulic capacitance: biophysics and functional significance of internal water sources in relation to tree size, Size- and Age-Related Changes in Tree Structure, Springer, Dordrecht, 341–361, 2011.
Scott, R., Entekhabi, D., Koster, R. D., and Suarez, M.: Timescales of land surface evapotranspiration response, J. Climate, 10, 559–566, 1997.
Sellers, P. J., Tucker, C. J., Collatz, G. J., Los, S. O., Justice, C. O., Dazlich, D. A., and Randall, D. A.: A Revised Land Surface Parameterization (SiB2) for Atmospheric GCMS. Part II: The Generation of Global Fields of Terrestrial Biophysical Parameters from Satellite Data, J. Climate, 9, 706–737, https://doi.org/10.1175/1520-0442(1996)009<0706:ARLSPF>2.0.CO;2, 1996a.
Sellers, P., Randall, D. A., Collatz, G., Berry, J., Field, C., Dazlich, D., Zhang, C., Collelo, G., and Bounoua, L.: A Revised Land Surface Parameterization (SiB2) for Atmospheric GCMS. Part I: Model Formulation, J. Climate, 9, 676–705, 1996b.
Seneviratne, S.: Impact of soil moisture-climate feedbacks on CMIP5 projections: First results from the GLACE-CMIP5 experiment, Geophys. Res. Lett., 40, 5212–5217, https://doi.org/10.1002/grl.50956, 2013.
Sikma, M. and Vilà‐Guerau de Arellano, J.: Substantial reductions in cloud cover and moisture transport by dynamic plant responses, Geophys. Res. Lett., 46, 1870–1878, 2019.
Stohl, A. and James, P.: A Lagrangian analysis of the atmospheric branch of the global water cycle. Part II: Moisture transports between earth's ocean basins and river catchments, J. Hydrometeorol., 6, 961–984, 2005.
Spracklen, D. V., Arnold, S. R., and Taylor, C. M.: Observations of increased tropical rainfall preceded by air passage over forests, Nature, 489, 282–285, https://doi.org/10.1038/nature11390, 2012.
Stevens, B. and Bony, S.: What Are Climate Models Missing?, Science, 340, 1053–1054, https://doi.org/10.1126/science.1237554, 2013.
Stoeckli, R., Lawrence, D. M., Niu, G. Y., Oleson, K. W., Thornton, P. E., Yang, Z. L., Bonan, G. B., Denning, A. S., and Running, S. W.: Use of FLUXNET in the Community Land Model development, J. Geophys. Res., 113, G01025, https://doi.org/10.1029/2007JG000562, 2008.
Sutanto, S. J., Wenninger, J., Coenders-Gerrits, A. M. J., and Uhlenbrook, S.: Partitioning of evaporation into transpiration, soil evaporation and interception: a comparison between isotope measurements and a HYDRUS-1D model, Hydrol. Earth Syst. Sci., 16, 2605–2616, https://doi.org/10.5194/hess-16-2605-2012, 2012.
Swann, A. L. S., Longo, M., Knox, R. G., Lee, E., and Moorcroft, P. R.: Future deforestation in the Amazon and consequences for South American climate, Agr. Forest Meteorol., 214–215, 12–24, https://doi.org/10.1016/j.agrformet.2015.07.006, 2015.
Tang, S., Xie, S., Zhang, Y., Zhang, M., Schumacher, C., Upton, H., Jensen, M. P., Johnson, K. L., Wang, M., Ahlgrimm, M., Feng, Z., Minnis, P., and Thieman, M.: Large-scale vertical velocity, diabatic heating and drying profiles associated with seasonal and diurnal variations of convective systems observed in the GoAmazon2014/5 experiment, Atmos. Chem. Phys., 16, 14249–14264, https://doi.org/10.5194/acp-16-14249-2016, 2016.
Tawfik, A. B. and Dirmeyer, P. A.: A process-based framework for quantifying the atmospheric preconditioning of surface-triggered convection, Geophys. Res. Lett., 41, 173–178, 2014.
Tawfik, A. B., Dirmeyer, P. A., and Santanello Jr., J. A.: The heated condensation framework. Part I: Description and Southern Great Plains case study, J. Hydrometeorol., 16, 1929–1945, 2015a.
Tawfik, A. B., Dirmeyer, P. A., and Santanello Jr., J. A.: The heated condensation framework. Part II: Climatological behavior of convective initiation and land–atmosphere coupling over the conterminous United States, J. Hydrometeorol., 16, 1946–1961, 2015b.
Taylor, C. M., Parker, D. J., and Harris, P. P.: An observational case study of mesoscale atmospheric circulations induced by soil moisture, Geophys. Res. Lett., 34, L15801, https://doi.org/10.1029/2007GL030572, 2007.
Taylor, C. M., Harris, P. P., and Parker, D. J.: Impact of soil moisture on the development of a Sahelian mesoscale convective system: a case-study from the AMMA Special Observing Period, Q. J. Roy. Meteor. Soc., 136, 456–470, https://doi.org/10.1002/qj.465, 2009.
Taylor, C. M., Birch, C. E., Parker, D. J., Dixon, N., Guichard, F., Nikulin, G., and Lister, G. M. S.: New perspectives on land-atmosphere feedbacks from the African Monsoon Multidisciplinary Analysis, Atmos. Sci. Lett., 12, 38–44, https://doi.org/10.1002/asl.336, 2011.
Taylor, C. M., Birch, C. E., Parker, D. J., Dixon, N., Guichard, F., Nikulin, G., and Lister, G. M. S.: Modelling soil moisture – precipitation feedbacks in the Sahel: importance of spatial scale versus convective parameterization, Geophys. Res. Lett., 40, 6213–6218, https://doi.org/10.1002/2013GL058511, 2013.
Thiery, W., Davin, E. L., Lawrence, D. M., Hirsch, A. L., Hauser, M., and Seneviratne, S. I.: Present-day irrigation mitigates heat extremes, J. Geophys. Res.-Atmos., 122, 1403–1422, https://doi.org/10.1002/2016JD025740, 2017.
Thornley, J. H. M.: Plant growth and respiration re-visited: maintenance respiration defined – it is an emergent property of, not a separate process within, the system – and why the respiration: photosynthesis ratio is conservative, Ann. Bot.-London, 108, 1365–1380, https://doi.org/10.1093/aob/mcr238, 2011.
Thum, T., Zaehle, S., Köhler, P., Aalto, T., Aurela, M., Guanter, L., Kolari, P., Laurila, T., Lohila, A., Magnani, F., Van Der Tol, C., and Markkanen, T.: Modelling sun-induced fluorescence and photosynthesis with a land surface model at local and regional scales in northern Europe, Biogeosciences, 14, 1969–1987, https://doi.org/10.5194/bg-14-1969-2017, 2017.
Torri, G., Kuang, Z., and Tian, Y.: Mechanisms for convection triggering by cold pools, Geophys. Res. Lett., 42, 1943–1950, https://doi.org/10.1002/2015GL063227, 2015.
Trenberth, K. E.: Atmospheric Moisture Recycling: Role of Advection and Local Evaporation, J. Climate, 12, 1368–1381, https://doi.org/10.1175/1520-0442(1999)012<1368:amrroa>2.0.co;2, 1999.
van der Ent, R. J., Savenije, H. H. G., Schaefli, B., and Steele-Dunne, S. C.: Origin and fate of atmospheric moisture over continents, Water Resour. Res., 46, https://doi.org/10.1029/2010WR009127, 2010.
van Dijk, A. I., Gash, J. H., van Gorsel, E., Blanken, P. D., Cescatti, A., Emmel, C., Gielen, B., Harman, I. N., Kiely, G., Merbold, L., and Montagnani, L.: Rainfall interception and the coupled surface water and energy balance, Agr. Forest Meteorol., 214–215, 402–415, https://doi.org/10.1016/j.agrformet.2015.09.006, 2015.
Vilà‐Guerau de Arellano, J., Patton, E. G., Karl, T., van den Dries, K., Barth, M. C., and Orlando, J. J.: The role of boundary layer dynamics on the diurnal evolution of isoprene and the hydroxyl radical over tropical forests, J. Geophys. Res.-Atmos., 116, 8032, https://doi.org/10.1029/2010JD014857, 2011.
Vilà‐Guerau de Arellano, J., Ouwersloot, H. G., Baldocchi, D., and Jacobs, C. M. J.: Shallow cumulus rooted in photosynthesis, Geophys. Res. Lett., 41, 1796–1802, https://doi.org/10.1002/2014GL059279, 2014.
Vogel, M. M., Orth, R., Cheruy, F., Hagemann, S., Lorenz, R., van den Hurk, B. J. J. M., and Seneviratne, S. I.: Regional amplification of projected changes in extreme temperatures strongly controlled by soil moisture-temperature feedbacks, Geophys. Res. Lett., 44, 1511–1519, https://doi.org/10.1002/2016GL071235, 2017.
Wang, J., Bras, R. L., and Eltahir, E.: The impact of observed deforestation on the mesoscale distribution of rainfall and clouds in Amazonia, J. Hydrometeorol., 1, 267–286, 2000.
Wang, J., Chagnon, J. F., Williams, E. R., Betts, A. K., Renno, N. O., Machado, L. A. T., Bisht, G., Knox, R., and Bras, R. L.: Impact of deforestation in the Amazon basin on cloud climatology, P. Natl. Acad. Sci. USA, 106, 3670–3674, https://doi.org/10.1073/pnas.0810156106, 2009.
Wang, Y. P. and Leuning, R.: A two-leaf model for canopy conductance, photosynthesis and partitioning of available energy I, Agr. Forest Meteorol., 91, 89–111, https://doi.org/10.1016/S0168-1923(98)00061-6, 1998.
Washington, R., James, R., Pearce, H., Pokam, W. M., and Moufouma-Okia, W.: Congo Basin rainfall climatology: can we believe the climate models? Philos. T. Roy. Soc. B, 368, https://doi.org/10.1098/rstb.2012.0296, 2013.
Wehr, R., Commane, R., Munger, J. W., McManus, J. B., Nelson, D. D., Zahniser, M. S., Saleska, S. R., and Wofsy, S. C.: Dynamics of canopy stomatal conductance, transpiration, and evaporation in a temperate deciduous forest, validated by carbonyl sulfide uptake, Biogeosciences, 14, 389–401, https://doi.org/10.5194/bg-14-389-2017, 2017.
Werth, D. and Avissar, R.: The local and global effects of Amazon deforestation, J. Geophys. Res.-Atmos., 107, 8087, https://doi.org/10.1029/2001JD000717, 2002.
Williams, M., Malhi, Y., Nobre, A. D., Rastetter, E. B., Grace, J., and Pereira, M. G. P.: Seasonal variation in net carbon exchange and evapotranspiration in a Brazilian rain forest: a modelling analysis, Plant Cell Environ., 21, 953–968, https://doi.org/10.1046/j.1365-3040.1998.00339.x, 1998.
Williams, E., Chan, T., and Boccippio, D.: Islands as miniature continents: Another look at the land‐ocean lightning contrast, J. Geophys. Res.-Atmos., 109, 1–5, 2004.
Wright, J. S., Fu, R., Worden, J. R., Chakraborty, S., Clinton, N. E., Risi, C., Sun, Y., and Yin, L.: Rainforest-initiated wet season onset over the southern Amazon, P. Natl. Acad. Sci. USA, 114, 8481–8486, https://doi.org/10.1073/pnas.1621516114, 2017.
Wu, C.-M., Stevens, B., and Arakawa, A.: What Controls the Transition from Shallow to Deep Convection?, J. Atmos. Sci., 66, 1793–1806, https://doi.org/10.1175/2008JAS2945.1, 2009.
Xu, X., Medvigy, D., Powers, J. S., Becknell, J. M., and Guan, K.: Diversity in plant hydraulic traits explains seasonal and inter-annual variations of vegetation dynamics in seasonally dry tropical forests, New Phytol., 212, 80–95, https://doi.org/10.1111/nph.14009, 2016.
Yano, J. I. and Plant, R. S.: Convective quasi-equilibrium, Rev. Geophys., 50, RG4004, https://doi.org/10.1029/2011RG000378, 2012.
Yin, L., Fu, R., Shevliakova, E., and Dickinson, R. E.: How well can CMIP5 simulate precipitation and its controlling processes over tropical South America?, Clim. Dynam., 41, 3127–3143, https://doi.org/10.1007/s00382-012-1582-y, 2013.
Yu, H., Remer, L. A., Chin, M., Bian, H., Kleidman, R. G., and Diehl, T.: A satellite-based assessment of transpacific transport of pollution aerosol, J. Geophys. Res.-Atmos., 113, https://doi.org/10.1029/2007JD009349, 2008.
Zahn, E., Dias, N. L., Araújo, A., Sá, L. D. A., Sörgel, M., Trebs, I., Wolff, S., and Manzi, A.: Scalar turbulent behavior in the roughness sublayer of an Amazonian forest, Atmos. Chem. Phys., 16, 11349–11366, https://doi.org/10.5194/acp-16-11349-2016, 2016.
Zemp, D. C., Schleussner, C.-F., Barbosa, H. M. J., van der Ent, R. J., Donges, J. F., Heinke, J., Sampaio, G., and Rammig, A.: On the importance of cascading moisture recycling in South America, Atmos. Chem. Phys., 14, 13337–13359, https://doi.org/10.5194/acp-14-13337-2014, 2014.
Zeng, N. and Neelin, J. D.: A land-atmosphere interaction theory for the tropical deforestation problem, J. Climate, 12, 857–872, 1999.
Zeng, N., Neelin, J., Lau, K., and Tucker, C.: Enhancement of Interdecadal Climate Variability in the Sahel by Vegetation Interaction, Science, 286, 1537–1540, 1999.
Zeppel, M. J. B., Lewis, J. D., Phillips, N. G., and Tissue, D. T.: Consequences of nocturnal water loss: a synthesis of regulating factors and implications for capacitance, embolism and use in models, Tree Physiol., 34, 1047–1055, https://doi.org/10.1093/treephys/tpu089, 2014.
Zhang, K., de Almeida Castanho, A. D., Galbraith, D. R., Moghim, S., Levine, N. M., Bras, R. L., Coe, M. T., Costa, M. H., Malhi, Y., Longo, M., and Knox, R. G.: The fate of Amazonian ecosystems over the coming century arising from changes in climate, atmospheric CO2, and land use, Global Change Biol., 21, 2569–2587, https://doi.org/10.1111/gcb.12903, 2015.
Zhang, Y., Fu, R., Yu, H., Dickinson, R. E., Juarez, R. N., Chin, M., and Wang, H.: A regional climate model study of how biomass burning aerosol impacts land-atmosphere interactions over the Amazon, J. Geophys. Res.-Atmos., 113, 1042, https://doi.org/10.1029/2007JD009449, 2008.
Zhang, Y., Fu, R., Yu, H., Qian, Y., Dickinson, R., Silva Dias, M. A. F., da Silva Dias, P. L., and Fernandes, K.: Impact of biomass burning aerosol on the monsoon circulation transition over Amazonia, Geophys. Res. Lett., 36, 1509, https://doi.org/10.1029/2009GL037180, 2009.
Zhang, Y. J., Meinzer, F. C., and Qi, J.: Midday stomatal conductance is more related to stem rather than leaf water status in subtropical deciduous and evergreen broadleaf trees, Plant Cell Environ., 36, 149–158, https://doi.org/10.1111/j.1365-3040.2012.02563.x, 2013.
Zhuang, Y., Fu, R., and Marengo, J. A.: Seasonal variation of shallow-to-deep convection transition and its link to the environmental conditions over the Central Amazon, J. Geophys. Res.-Atmos., 122, 2649–2666, https://doi.org/10.1002/(ISSN)2169-8996, 2017.
- Abstract
- Introduction
- Convection, clouds, and rainfall in the tropics
- Surface turbulent fluxes in the tropics
- Vegetation and ecosystem processes in the tropics
- Tropical land–atmosphere interactions: local and nonlocal perspectives
- Discussion – conclusions
- Data availability
- Author contributions
- Competing interests
- Acknowledgements
- Financial support
- Review statement
- References
The requested paper has a corresponding corrigendum published. Please read the corrigendum first before downloading the article.
- Article
(8027 KB) - Full-text XML
- Abstract
- Introduction
- Convection, clouds, and rainfall in the tropics
- Surface turbulent fluxes in the tropics
- Vegetation and ecosystem processes in the tropics
- Tropical land–atmosphere interactions: local and nonlocal perspectives
- Discussion – conclusions
- Data availability
- Author contributions
- Competing interests
- Acknowledgements
- Financial support
- Review statement
- References