the Creative Commons Attribution 4.0 License.
the Creative Commons Attribution 4.0 License.
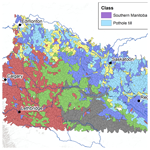
A watershed classification approach that looks beyond hydrology: application to a semi-arid, agricultural region in Canada
Kevin R. Shook
Chris Spence
Colin J. Whitfield
Classification and clustering approaches provide a means to group watersheds according to similar attributes, functions, or behaviours, and can aid in managing natural resources. Although they are widely used, approaches based on hydrological response parameters restrict analyses to regions where well-developed hydrological records exist, and overlook factors contributing to other management concerns, including biogeochemistry and ecology. In the Canadian Prairie, hydrometric gauging is sparse and often seasonal. Moreover, large areas are endorheic and the landscape is highly modified by human activity, complicating classification based solely on hydrological parameters. We compiled climate, geological, topographical, and land-cover data from the Prairie and conducted a classification of watersheds using a hierarchical clustering of principal components. Seven classes were identified based on the clustering of watersheds, including those distinguishing southern Manitoba, the pothole region, river valleys, and grasslands. Important defining variables were climate, elevation, surficial geology, wetland distribution, and land cover. In particular, three classes occur almost exclusively within regions that tend not to contribute to major river systems, and collectively encompass the majority of the study area. The gross difference in key characteristics across the classes suggests that future water management and climate change may carry with them heterogeneous sets of implications for water security across the Prairie. This emphasizes the importance of developing management strategies that target sub-regions expected to behave coherently as current human-induced changes to the landscape will affect how watersheds react to change. The study provides the first classification of watersheds within the Prairie based on climatic and biophysical attributes, with the framework used being applicable to other regions where hydrometric data are sparse. Our findings provide a foundation for addressing questions related to hydrological, biogeochemical, and ecological behaviours at a regional level, enhancing the capacity to address issues of water security.
- Article
(4834 KB) - Full-text XML
-
Supplement
(1459 KB) - BibTeX
- EndNote
Watershed classification methods provide a means of grouping watersheds according to similar attributes, or behaviours, and can identify sub-regions that are expected to exhibit coherent responses. This strategy can identify how catchment characteristics are similar, or dissimilar, among groups of watersheds and thus might influence hydrologic behaviour (McDonnell and Woods, 2004). Classifying watersheds can be useful for developing predictions in ungauged basins (Peters et al., 2012), and moreover, classification can be used to inform how changes to key traits (e.g., climate and land management) may affect system function. Establishing these links between watershed function and biophysical structure, including hydroclimate, is an opportunity of watershed classification (Wagener et al., 2007). Accordingly, the regionalization of hydrological response through watershed classifications has been used to inform natural resource management (Detenbeck et al., 2000; Jones et al., 2014).
Many different approaches to watershed classification have been employed to date, including non-linear dimension reduction techniques (Kanishka and Eldho, 2017), decision trees (Bulley et al. 2008), and independent component analysis (Mwale et al., 2011), among others. Hydrological characteristics (e.g., statistical properties of streamflow regime) are widely used to inform classification owing to their potential linkages between watershed features and hydrologic responses (Brown et al., 2014; Sivakumar et al., 2013; Spence and Saso, 2005). Other classification exercises have included a wider number of characteristics, including biophysical attributes along with streamflow response, to differentiate watershed classes (e.g., Sawicz et al., 2011; Burn, 1990). Ecoregions, which incorporate historical aspects of climate, topography, and vegetation regimes, have also served as a method of differentiation for eco-hydrological studies (Loveland and Merchant, 2004). In select cases, classification is performed independently of streamflow response factors (Knoben et al., 2018). In arid or poorly gauged regions of the world, these types of approaches to classification that are independent of or not strongly dependent on hydrological indices (streamflow response) are needed, although few such classifications have been performed. The need for new approaches to watershed classification can also be true of regions undergoing strong pressures from climate change and land use, where historical streamflow records may not reflect current behaviour, particularly if a regime shift has occurred.
In Canada, watershed classification has been applied in many regions (e.g., Cavadias et al., 2001; Ouarda et al., 2002; Spence and Saso, 2005). To date, most have focused on larger basins, and none have covered in detail the semi-arid Canadian Prairie, which spans nearly 5×105 km2 in western Canada, from the Rocky Mountain foothills in the west to Lake Winnipeg in the east (Fig. 1). This is despite its importance as a major food producing region of the world and one that faces numerous water security challenges (Gober and Wheater, 2014; Spence et al., 2018). Earlier work by Durrant and Blackwell (1959) grouped large Prairie watersheds based on flood regimes. A recent classification that included the Prairie region focused on stream hydrology (e.g., MacCulloch and Whitfield, 2012), but was broader and included watersheds from mountainous and forested regions to the west and north, respectively. In the Canadian Prairie, and similar regions elsewhere, extrapolating catchment-scale field and modelling studies presents challenges. It is inherently difficult to explain or predict different responses among basins, as poorly developed stream networks with intermittent or seasonal flow do not easily lend themselves to classification methods featuring streamflow response. MacCulloch and Whitfield (2012), who found a single streamflow class across the Canadian Prairie, raised the question as to whether a single grouping is appropriate, and suggested the need to expand classifications to include a greater diversity of biological, physical, and chemical properties.
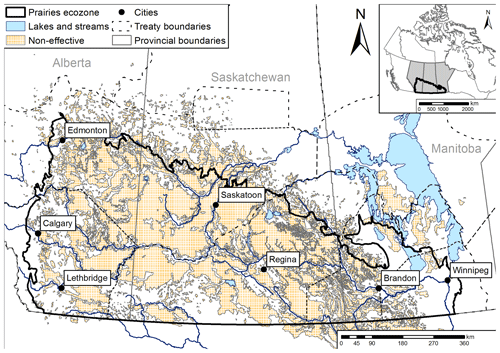
Figure 1Map of the study area spanning the Prairie ecozone in western Canada (inset). Large cities in each of the three provinces are shown for reference, while the region characterized as not contributing runoff (2-year) is also shown. Prairie ecozone based on the region classified by the Ecological Stratification Working Group (1995).
Like many of the world's agricultural regions, the Canadian Prairie has undergone vast environmental change co-incident with the green revolution. Predominant agricultural practices have changed over the decades, and each is known to influence water cycling and storage, including tillage practices, summer fallowing, and cropping type (Awada et al., 2014; Van der Kamp et al., 2003; Shook et al., 2015). Significant warming over the last 70 years, especially in winter (Coles et al., 2017; DeBeer et al., 2016), has resulted in more rain at the expense of snow (Vincent et al., 2015), and multiple-day rainfall events have been increasing in frequency relative to shorter events in some regions (Dumanski et al., 2015; Shook and Pomeroy, 2012). These observed changes in precipitation have reduced the predictability of runoff derived from snowmelt and add uncertainty to water management and agricultural decision-making.
Disentangling the relative impacts of climate and land-use changes on water quantity and quality is complex, particularly as their effects are heterogeneous across spatial extent and scale. For the Prairie and elsewhere, new approaches to classification that can distinguish sub-regional and, importantly, sub-hydrometric station variability, are needed. Further, because land-management decisions in agricultural regions are intrinsically linked to system function, there is a need for classifications that can inform decision-makers at a relevant scale. Indeed, stable isotope-based investigations of runoff from small lake catchments in the Boreal Plains (north of the Prairie) emphasize the need for local-scale characterization of watershed behaviour (Gibson et al., 2010, 2016), while streamflow dynamics for the Prairie and nearby Boreal Plain are linked to local surface geology and land cover (Devito et al., 2005; Mwale et al., 2011), suggesting an opportunity for a new approach to watershed classification in the region. Another potential advantage of a more comprehensive approach is that by de-emphasizing available hydrometric observations for larger and well-studied or monitored basins and including other environmental characteristics, the risk of overlooking other functions (e.g., ecology, biogeochemistry) that may be equally important to the management of a watershed's natural resources can be reduced. A system-based watershed classification for the Prairie that avoids the prejudice of classifying only those watersheds where a reasonably robust understanding of hydrology or streamflow exists can serve as a template for other regions of the world where streamflow-based classification is not viable.
The objective of the present work is to develop a watershed classification system based on hydrologically and ecologically significant traits for the Canadian Prairie. In this region, assessment of localized hydrological response to change is challenged by limited spatial resolution of observed streamflow data and higher-order streamflow being unrepresentative of local response due to a poorly developed drainage network. In establishing such an approach, we seek to advance our understanding of watershed hydrology and broader watershed behaviour within the Prairie whilst also providing a framework for similar classification exercises in other regions where streamflow-based methods are not ideal. Our approach avoids the limitations of classifying according to known hydrologic response and increases the spatial resolution of watershed classification relative to many existing approaches. We compile physiographic characteristics, including geology, wetland distribution, and land cover, of watersheds approximately 100 km2 to achieve the classification. This framework will identify those areas that are climatically and geographically similar, and thus might be expected to respond in a hydrologically coherent manner to climate and land-management changes. Additionally, it provides a foundation on which to base prediction of watershed hydrologic, biogeochemical, and ecological responses to these stressors.
2.1 Region domain and description
The Canadian Prairie (Prairie ecozone) spans the provinces of Alberta, Saskatchewan, and Manitoba, and is part of the Nelson Drainage Basin (Fig. 1). The climate is semi-arid, with mean annual precipitation ranging between 350 and 610 mm (1970–2000), increasing from west to east. The mean annual temperature was 1–6 ∘C over the same period with warmer conditions towards the southwest (Mekis and Vincent, 2011; Vincent et al., 2012; http://climate.weather.gc.ca/climate_normals/index_e.html, last access: 13 November 2017). Much of the region deglaciated during the Late Pleistocene approximately 10 000 years before present, resulting in an often hummocky landscape with numerous depressions. Combined with the dry climate, the relatively short post-glaciation history has prevented maturing of a ubiquitous drainage network, and many headwaters remain disconnected from higher-order streams (Shook et al., 2015). Depressions in the hummocky landscape, and the wetlands that form within them, are important features for Prairie hydrology (Van der Kamp et al., 2016) and often facilitate groundwater recharge (i.e., depression-focused recharge) (Van der Kamp and Hayashi, 2009). The location of wetlands and their size relative to the watershed outlet control hydrologic gate-keeping (e.g., Spence and Woo, 2003) and thus the potential to contribute streamflow to higher-order watersheds (Leibowitz et al., 2016; Shaw et al., 2012; Shook et al., 2013). The size distribution of wetlands within a watershed and their spatial arrangement also dictate biogeochemical function and provide habitat and foraging for biota (Evenson et al., 2018). Terrestrial vegetation is typically open grassland, with aspen parkland ecotone along the northern edges of the ecozone boundary (Ecological Stratification Working Group, 1995).
2.2 Watershed boundaries
The focus of this study was on those watersheds that drain a distinctively prairie landscape, with watersheds defined according to topographic delineation. Thus, we constrained our study to the Canadian Prairie ecozone (4.7×105 km2) and watersheds occurring therein. Delineations of candidate study watersheds were obtained from the HydroSHEDs global dataset (Lehner and Grill, 2013). Watershed boundaries within this dataset were based on a Shuttle Radar Topographic Mission (SRTM) digital elevation model (DEM) calculated at a 15 arcsec resolution. The resolution is equivalent to for example approximately 285 m east–west and 464 m north–south at Saskatoon, SK. As with other SRTM products, the HydroSHEDs dataset may be prone to errors in regions with low relief due to an elevation precision of 1 m. However, the dataset provided watershed delineations over the geographic region of interest and at a fine enough scale (i.e., 100 km2), and thus it was sufficient based on data availability for the purpose of the current study.
Only those watersheds completely within the Canadian Prairie ecozone were extracted (n=4729) from the HydroSHEDs dataset. Those watersheds that were very large (>4000 km2) or small (<5 km2) were removed from analysis (see Table S1 in the Supplement). Because HydroSHEDs includes the basins of larger water bodies, including lakes, watersheds consisting of a majority of water were removed as the study only concerns the uplands of these systems. Finally, highly urbanized areas (i.e., watersheds with cover being >40 % urban) were removed. After considering these criteria, 4175 watersheds remained for use in subsequent analyses, covering a total area of 4.2×105 km2. The mean watershed area for this subset was 99.8±58.7 km2.
2.3 Physiographic data collection
The physiographic watershed variables were assembled from Canadian provincial and federal governments and non-governmental agency datasets (see Table S2 for a full list of variables and their sources). Variables were derived from climatic, hydrologic, geological, geographic, and land-cover data, and details are described briefly below. Spatial processing and statistical analyses were conducted in ArcGIS version 10.5 and R version 3.4.3 (R Core Team, 2018), respectively.
2.3.1 Climate
Mean annual precipitation and temperature data were derived from the Canadian Gridded Temperature and Precipitation Anomalies (CANGRD) dataset spanning (ECCC, 2017). CANGRD is the only gridded climate product available for the region that uses adjusted and homogenized station data, and was picked for this reason (Mekis and Vincent, 2011; Vincent et al., 2015). The 1970–2000 period was chosen because the number of stations with adjusted and homogenized data used to derive CANGRD significantly diminished after 2000 (Laudon et al., 2017). Mean annual values over the 30-year period were constructed from 50 km resolution gridded cells (n=626) within and surrounding the Prairie ecozone and interpolated to a higher spatial resolution raster by kriging using a spherical semivariogram. Values were clipped according to the watershed boundaries and averaged over the watersheds to obtain mean annual precipitation and temperature for each watershed. Mean annual potential evapotranspiration (PET) was derived as a measure of dryness across the region. To maintain consistency among climate data and use the same temperature data as described above, options were limited with which to calculate PET. The Thornthwaite equation (Thornthwaite, 1948) was applied using R package SPEI (Vicente-Serrano et al., 2010). A disadvantage of the Thornthwaite approach is that it calculates PET solely as a function of air temperature and latitudinal position, and it assumes a fixed correlation between temperature and radiative forcing. As such, it integrates effects of other factors directly or indirectly influencing radiation or latent heat, like advection, vegetation, and humidity. The calculation adjusts for any lag in this relationship using corrections for latitude and month; however, it likely does not represent the full annual and seasonal variability in PET across a landscape, given regional heterogeneity of the aforementioned factors. Despite the limitations, the simplicity of this method is ideal for application across the wide geographic area of interest with limited data required as input, allowing for approximation of mean annual PET for the study area.
2.3.2 Wetland traits
Large regions within the Canadian Prairie have been designated as being “non-effective” where they do not contribute flow to the stream network at least 1 year in 2 (Godwin and Martin, 1975). The locations of these regions are shown in Fig. 1. This definition stems from work by Agriculture and Agri-Food Canada where Prairie drainage areas were divided into gross and effective drainage areas, whereby the former describes the area within a topographic divide that is expected to contribute under highly wet conditions and the latter is the area that contributes runoff during a mean annual runoff event (Mowchenko and Meid, 1983). Thus, at its simplest, the non-effective area is the difference between the gross and effective drainage area; however, the exact area contributing runoff is dynamic and the controls complex, which include antecedent storage capacity and climatic conditions (Shaw et al., 2012: Shook and Pomeroy, 2011). Briefly, the “non-effective” regions are caused by the intermittent connectivity of runoff among the landscape depressions, which trap runoff and prevent it from contributing to downstream flow when the depressions are not connected. Trapped surface water can form wetlands (hereafter, inclusively referring to water area ponded in these depressions). These depressions can store water and are indicative of water storage of the basin. Thus the non-effective portion of a basin is an index of its lack of contribution and is an important quality when considering the hydrological dynamics of this region (Shook and Pomeroy, 2012).
The Global Surface Water dataset (Pekel et al., 2016) provides a geographically comprehensive layer of any ∼30 m × 30 m pixel that was inundated at least once between 1984 and 2015, as identified from the Landsat constellation of satellites. It was assumed that the dataset was indicative of potential maximum wetland coverage, as this period spanned several wet climate periods. As such, “wetland” in this context can include some seasonal ponds (i.e., prairie potholes) as well as larger or more permanent shallow water bodies (but see Sect. 2.2 and Table S1). Using R package raster (Hijmans, 2017), wetland variables were calculated for each study watershed, including fractional wetland area and the number of wetlands within the watershed per unit area (i.e., wetland density – km−2). The ratio of the area of the largest wetland to total wetland area in the watershed was also used as a metric (i.e., WL). Further, we used the ratio of the linear distance of the largest wetland's centroid to the watershed outlet (LW) to the maximum watershed boundary distance to the outlet (LO) to represent a centroid fraction (LW∕LO, i.e., the relative location of the largest wetland to watershed outlet). The basin outlet was defined as the point of lowest elevation on the watershed boundary. Both WL and LW∕LO can be used to evaluate the relative importance of hydrological gate-keeping; for example, larger wetland depressions located closer to the outlet control the likelihood of the watershed contributing flow downstream and attenuating peak flow (Shook and Pomeroy, 2012; Ameli and Creed, 2019).
To estimate wetland size distribution, it was assumed that they followed a generalized Pareto distribution (GPD) defined according to Shook et al. (2013):
where z is wetland area and μ is the location parameter (i.e., the minimum size for which the distribution was fitted and has units of m2), and the scale (β) and shape (ξ) parameters are determined for each watershed. The β parameter is an index of the dispersion of the distribution, similar to the standard deviation, with the same units as the data being fitted (in this case m2). The ξ parameter is dimensionless and governs the shape of the fitted distribution. Hosking and Wallis (1987) plot the effect of variation in the shape parameter on the GPD. The scale and shape parameters were used to quantify the size distribution of wetlands and thus to describe the wetland frequency distributions for the cluster analyses (see Sect. 3.2). Note that because the sizes of the water bodies were taken from infrequent remote-sensing measurements (i.e., the Landsat data have a minimum revisit time of 8 or 16 d), they also are biased against short-lived water bodies.
2.3.3 Topographical parameters
Topographic variables, including the mean elevation, mean and coefficient of variation of slope, and stream density, were also calculated for each watershed. Because of the hummocky nature of many regions in the domain, it is possible for a basin to have some fraction of its area located at an elevation below that of the outlet. As such, the fraction of area below the basin outlet (ABO) was calculated for each basin. The elevation and slope variables were based on a DEM generated from the SRTM dataset. Stream vectors were obtained from the hydrographic features of the CanVec (1:50 000) series available from Natural Resources Canada (NRC, 2016, https://open.canada.ca/data/en/dataset?q=canvec&sort=&collection=fgp, last access: 22 November 2017). The total length of streams within a watershed was calculated and divided by the watershed area to produce the stream density. Additionally, the dimension shape factor (DSF) was used to describe watershed shape, as it has been found important for hydrological responses in previous Canadian catchment classification exercises (Spence and Saso, 2005). The DSF (km−1) was calculated as follows:
where P (km) and A (km2) are the watershed perimeter and area, respectively, and are derived from the HydroSHEDs global dataset (Lehner and Grill, 2013).
Geographical parameters of surficial geology, local surface landforms, soil particle size classes (sand, silt, clay), and soil zone were included in the analysis. Surficial geology polygons were derived by compiling provincial government data sources for Alberta (Atkinson et al., 2017), Saskatchewan (Simpson, 2008), and Manitoba (Matile and Keller, 2006). Due to the different geological classification schemes for each province, more detailed classes were grouped to broader categories related to depositional environment and surficial materials using those from the Geological Survey of Canada (2014), which provided for comparison across provincial boundaries. Local surface form (i.e., areas categorized by slope, relief, and morphology) and soil zone data were obtained from the Soil Landscape dataset (AAFC, 2013). The soil zones in the Canadian Prairie used in the analyses were black, dark brown, brown, grey, and dark grey. The zones incorporate characteristics of colour and organic content, which are influenced by regional climate and vegetation. Clay, silt, and sand content were collected from the Detailed Soil Survey of Canada (AAFC, 2015). Mean catchment values of surficial geology, local surface landform, soil zone, and particle size class were determined by areal weighting of soil polygons within the watershed boundaries.
2.3.4 Land-cover and cropland practice
Fractional areas of land-use types were derived from Agriculture and Agri-Food Canada's 2016 Annual Crop Inventory (AAFC, 2016). These raster data define land use and land cover. Variables used in our analysis were standardized to watershed area and included unmanaged grasslands, forests (i.e., the sum of coniferous, deciduous, and mixed forest areas), pasture, and cropland (sum of cropped land areas). The predominant cropland practice was defined according to the fractional area of tillage by agricultural region sub-division (e.g., normalized to the area prepared for seed within that division by year). Averaged areas over the years 2011 and 2016 for each practice, including zero till, conservation till (leaving crop residue on soil surface), and conventional till (incorporating residues into soil) (Statistics Canada, 2016), were used to describe these activities and normalized as a fraction of the watershed.
2.3.5 Hydrological variable calculation
The relatively sparse hydrometric stream gauging in the domain, and the resulting paucity of data, present two notable challenges to hydrologic response-based watershed classification. The first is that the basin network is biased to stations on higher-order (and often exotic) streams traversing the region (i.e., larger river basins), and thus there are a limited number of hydrometric gauges on streams draining solely Prairie watersheds, particularly at the spatial resolution of our study watersheds (∼100 km2). Further, only a subset of these are considered reference stations (i.e., gauging unmanaged flows). Second, in the more arid and/or cold regions of the Prairie, some of these hydrometric stations are operated only seasonally, presenting additional challenges in using these records for classification exercises (e.g., MacCulloch and Whitfield, 2012).
As a result, mean annual runoff (Q2) and 1:100-year flood (Q100) magnitudes were estimated for the 4175 watersheds using relationships defined from canonical correlation analysis (CCA) to correlate gauged data with multivariate climatic and physiographic data according to procedures given by Spence and Saso (2005). According to Spence and Saso (2005), expected uncertainty using these methods approached 50 % but exhibited biases of less than 15 % (n=34). Hydrological stations used were those identified in MacCulloch and Whitfield (2012) and within the Prairie region (n=11), and data were obtained from archived databases of the Water Survey of Canada (https://wateroffice.ec.gc.ca/search/historical_e.html, last access: 12 February 2018) between 1990 and 2014. We note that greater uncertainty than that reported by Spence and Saso (2005) may result when using the CCA approach with a smaller sample size. Multivariate geographic data were collected as outlined in the above sections according to the watershed boundaries for the hydrological stations. Due to the fact that many watersheds within the HydroSHEDs dataset are likely to drain internally and do not consistently connect to a higher-order stream network, these streamflow data were interpreted as “runoff”, meaning the amount of water accumulated within the watershed polygon that drains to its lowest point annually.
Briefly, CCA correlates the streamflow record of gauged basins with physico-climatic characteristics of watersheds by representing these variables as a reduced set of canonical variables. The analysis results in two canonical variable sets: one for the physico-climatic variables (i.e., V1 and V2) and another for the hydrological variables (i.e., W1 and W2). These canonical variables are constructed from linear combinations of the variable sets such that the correlations of the canonical variables are maximized. Canonical variables plotting similarly on X–Y plots (W1–W2 and V1–V2) indicate good correlation (Spence and Saso, 2005). Where canonical correlations (λ1, λ2) were above 0.75 (Cavadias et al., 2001), that set of physico-climatic variables was deemed useful for estimating hydrological variables. Those physico-climatic variables passing this threshold were included as variables in a multiple regression to develop a predictive equation for Q2. Analyses were performed using R package vegan (Oksanen et al., 2018).
3.1 Pre-processing compositional datasets
Principal component analysis (PCA) was used as a pre-processing step to reduce the dimensionality associated with compositional datasets (e.g., topographical and land-cover parameters) (Fig. S1 in the Supplement). Using this approach, the principal components (PCs) that could cumulatively explain 80 % of the variation in a subset of compositional data were included in the subsequent cluster analysis. This procedure identified the major data patterns and aided in reducing the number of zero-weighted variables. Where necessary, variables that were not transformed into PCs were log-transformed to reduce data skewness. Variable unit ranges were also scaled during the PCA to reduce the impact of certain variables exhibiting a large range of values on the subsequent cluster analysis.
3.2 Agglomerative hierarchical clustering of principal components and watershed classification
Clustering analysis was performed on the suite of physiographic variables, which included PC variables derived from pre-processing (Tables S2 and S3). Agglomerative hierarchical clustering of principal components (HCPC) was used to define clusters of watersheds using the HCPC function in R package FactoMineR (Lê et al., 2008; Husson et al., 2009) to apply a PCA on the standardized multivariate dataset of watershed attributes and was the basis for clustering. The majority of physiographic variables were included as active variables in the PCA, and thus influenced the arrangements of the PCs. In contrast, watershed area, DSF, latitude, and longitude were used only as supplementary variables, and thus did not explicitly affect the clustering analysis. These variables did, however, aid in watershed class characterization and interpretation. The first set of PCs that together explained 50 % of the variation in the dataset (n=6) was retained for agglomerative clustering. Retaining these first PCs at a threshold of 50 % allowed for clearer focus on main trends in the data and reduced the impact of noise on subsequent analyses, which might occur if subsequent, less influential, PCs were retained.
The agglomerative hierarchical clustering was performed using the Euclidean distances (from the PCA) and Ward's criterion for aggregating clusters. Ward's criterion decomposes the total inertia of clusters into between- and within-group variance, and this method dictates merging for clusters (or watersheds) such that the growth in within-group inertia is minimal (Husson et al., 2009). The total inertia is partitioned into within- and between-group inertias. Within-group inertia represented the homogeneity, or similarity, of watersheds within a cluster. Consequently, watersheds located close to each other in PC space were deemed to be similar in their attributes. Watersheds are grouped according to pairs that minimize within-group inertia (Begou et al., 2015) and are differentiated based on between-group inertia gained by adding clusters. The variables contributing to cluster characteristics were determined by v tests (Husson et al., 2009), which assessed whether the cluster mean for a given variable was significantly (p<0.05) greater or smaller than the overall mean.
3.3 Comparing class-specific observed and simulated wetland depression data
To compare how well the GPD parameters predicted the observed wetland area distributions from the Global Surface Water (GSW) dataset, wetland size distributions were simulated for each class. Wetland area for select watershed class-specific percentiles (i.e., 25th, 50th, and 75th percentiles) derived from the simulated data were then compared to the wetland areas for corresponding watershed class-specific percentiles of the observed watershed data to assess the potential usefulness of using these parameters in representing wetland size distribution.
For this comparison, the fitted wetland area distributions were constrained in their minimum and maximum values by the Global Surface Water dataset spatial resolution (i.e., the 30 m pixel size) and the median area of the largest wetland observed for each watershed class, respectively. The median area of the distribution of the largest wetlands for each watershed class gave an indication of the maximum sizes of the water bodies in those watersheds, and thus provided a maximum value for simulating wetland areas using the GPD. Wetland areas were simulated using R package SpatialExtremes (Ribatet, 2018).
3.4 Resampling and re-classifying procedure
The robustness of the HCPC procedure in characterizing Prairie watersheds was tested using additional hierarchical clustering on 10 subsets of the entire set of 4175. For each iteration, 10 % of watersheds were removed from the original dataset (n=4175) without replacement, and the remaining watersheds (n=3757) were then re-analyzed according to the HCPC outlined above (Fig. S1). The number of potential classes allowed was set at seven (k=7), for consistency with the complete analysis. The resulting classifications were then compared to the classification performed on the complete dataset, with the watersheds being assessed on the percentage of iterations in which they were assigned to the same class as the complete classification. The proportion of membership agreement was calculated and visualized to assess the likelihood of classing watersheds consistently.
4.1 Geographical data processing
4.1.1 Dimension reduction: compositional datasets and principal components analysis
Variation in geology and soil was best explained by two or three principal components (Table 1; Fig. S2). Two PCs captured over 80 % of the variation in surficial geology, with PC1 (proportion explained: 73 %) positively relating to glacial till deposits and negatively with glaciolacustrine deposits, and PC2 (14 %) positively related to riverine or erosive deposits, such as glaciofluvial, alluvial, and eolian deposits. Particle size class data were explained by the first two PCs, where PC1 (75 %) was positively associated with sand and negatively associated with silt and clay, while PC2 (14 %) was related negatively to silt. Positive PC1 (55 %) scores defined the dominance of black soils, and PC2 (43 %) described dominance of brown or dark brown soils on positive or negative scores, respectively. Three PCs described the local surface form dataset. PC1 (55 %) captured the change from a greater portion of hummocky forms to undulating forms, and PC2 (24 %) was negatively associated with higher river-incised landscape fraction. The portion of level surface form was negatively related to PC3 (12 %).
Table 1Pre-processing of compositional data PCA results. Shown are the respective subsets, the number of initial fractional area variables before dimensional reduction, the number of principal components retained to reach over 80 % of subset variation (except for tillage practice), and the proportion of variation explained by each component.
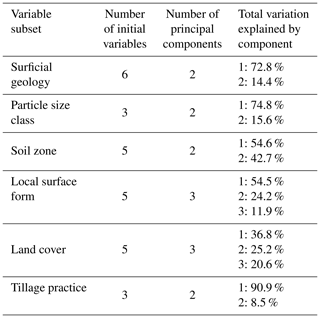
Three PCs were needed to explain over 80 % of the variation in land cover (Table 1; Fig. S2). Land-cover PC1 (37 %) was positively associated with higher cropland and negatively with unmanaged grassland, whereas PC2 (25 %) was negatively associated with higher pasture and forest cover. PC3 was associated with greater fallow and pasture areal proportion (21 %). Cropland practice was described by PC1 (90 %), with zero-till practices being negatively associated with this component. Although it only explained 9 %, PC2 was also retained to describe the change between conventional and conservation till practices, with the practices exhibiting positive and negative relationships, respectively.
4.1.2 Canonical correlation analysis
The canonical coefficients from the CCA were acceptably high at λ1 0.97 and λ2 0.77, respectively, indicating that the physico-climatic variables exhibited influence on the hydrological variables (Cavadias et al., 2001; Spence and Saso, 2005). Canonical correlation values between the hydrological variables and W2 were greater than those with W1 (Table 2); thus, the physico-climatic variables strongly associated with second canonical correlation (i.e., V2) were used in the multiple regressions. These variables were watershed area, DSF, areal fraction of rock, and areal fraction of natural area. Plots of observed and predicted runoff Q2 (R2=0.45) and Q100 (R2=0.48) show moderate agreement at lower flow values (Fig. 2). There is a negative bias estimated between 26 % and 29 %, which is greater than that documented by Spence and Saso (2005) using comparable extrapolation methods, but this is not unexpected because of the smaller sample size in the current study. As Q2 and Q100 exhibited high collinearity, only Q2 was included in subsequent cluster analyses to
where A was the watershed area, N was the natural area fraction and the sum of grasslands and forest, R was the rock fraction area, and DSF was the dimensional shape factor of the watershed. The equation was then used to calculate Q2 for each watershed included in the clustering analysis.
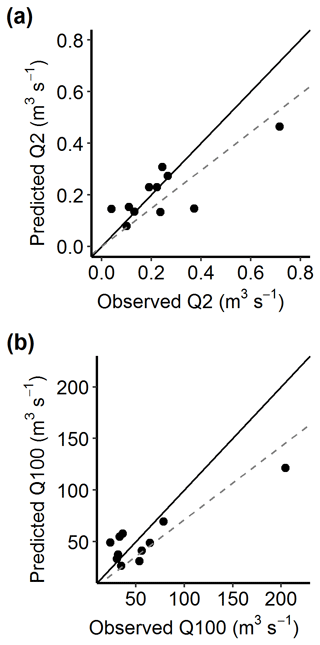
Figure 2Observed versus predicted estimates for (a) Q2 and (b) Q100. The dashed grey line depicts the linear regression between observed and predicted flow values, and the black, solid line shows a 1:1 relationship.
4.2 Watershed classification
4.2.1 Principal component analysis
In total, 29 watershed attributes, including the PCs from compositional datasets (see Table 1), were used in the clustering analysis as active variables, and four were included as supplementary variables (Table 3). In the pre-clustering PCA, the first six PCs explained 54.3 % of data variation and were retained for the HCPC analysis (Fig. 3). The influence of subsequent PCs declined dramatically, and 11 PCs were required to explain >80 %. Variable importance in the classification was not related to the log-transformed range exhibited by that variable (data not shown), and impact was mitigated by scaling the ranges of input variables in the PCA.
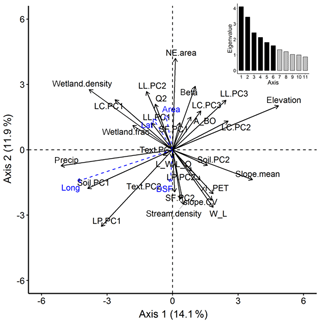
Figure 3Principal component analysis for candidate variables for classification. Active and supplementary variables are shown as solid black and dashed blue arrows, respectively. Eigenvalues for PC axes are provided (inset), with black bars denoting the six PCs used in the hierarchical clustering analysis.
Table 3Correlation of study watershed attributes with principal components (PCs). The values for the six PCs used in the cluster analysis are shown.
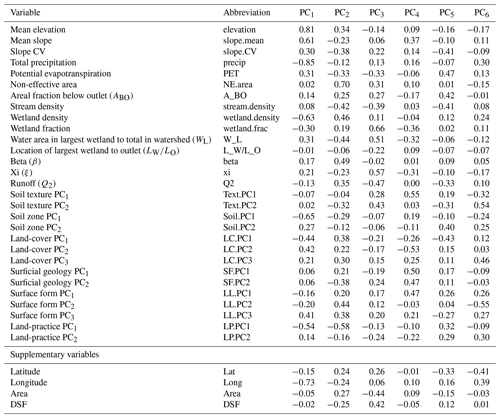
Principal components 1 and 2 captured changes in physical, land-cover, and wetland characteristics (Fig. 3). PC1 was strongly associated with physical and land-cover characteristics, such as elevation, wetland density, and the land-cover PCs. PC2 was strongly related to metrics characterizing the hydrological landscape, including river and wetland density, non-effective area fraction, landscape surface form, and size of the largest wetland (WL). Subsequent PCs explained less variation and were more specialized in the variables associated with them. Generally, these PCs were associated with differences in soil zone and texture class, surficial geology, and varying surface landform. A more detailed account of associations of the variables with the PCs is provided below.
PC1 was positively associated with elevation, mean slope, land-cover PC2, and surface form PC3, and negatively with total annual precipitation, soil zone PC1, wetland density, land-practice PC1, land-cover PC1, and longitude (Table 3; Fig. 3). PC2 was associated with non-effective area fraction, wetland density, β, and surface form PC2, and negatively related to land-practice PC1, WL, and river density. PC3 was positively related to wetland fraction, WL, ξ, soil texture PC2, and DSF. Watershed area and runoff were negatively associated with PC3.
Variable correlations were weaker for the remaining three PCs (Table 3). PC4 was mainly associated with soil texture PC1, surficial geology PC1, and surface landform PC1, characteristic of sandier soil areas featuring glacial till deposits and higher hummocky surface forms, as well as higher mean slope. PC4 was negatively related to land-cover PC2. PC5 was related positively to PET, fraction below outlet, and soil zone PC2, and negatively to land-cover PC1, river density, and slope CV. Finally, PC6 was mainly associated with soil texture PC2 and land-cover PC3, and negatively with surface landform PC2.
4.2.2 Agglomerative hierarchical cluster analysis
Seven clusters were identified from the hierarchical cluster analysis based on the between-group inertia gained by increasing cluster number (k). The HCPC analysis suggested three clusters resulted in the greatest reduction of within-group inertia while minimally increasing k (Fig. 4). Further increasing k refined the separation and differentiation of clusters up to seven (k=7). Minimal added separation was observed up to k=9, and increasing k>9 resulted in little inertia gained between clusters. Thus, seven clusters, or classes, were manually selected based on these observations (Fig. 4).
4.2.3 Class characteristics and interpretation
Our methodology yields sub-regional watershed classes according to climatic, physiographic, wetland, and land-cover variables. The seven classes (Fig. 5) are defined by multivariate sets of attributes (Table 4). Influential classifying variables in all classes were mean elevation, total annual precipitation, land practice, surface forms, and wetland density. Other variables influential to class differentiation included fraction of non-effective area, land cover, and soil variables. Climate and elevation gradients are likely responsible for the west-to-east watershed clustering pattern. Moreover, we observe strong spatial concordance among some classes (Fig. 5), which is likely due to the hierarchical nature of the analysis. For simplicity, we interpret classes based on the variables where large, significant differences in class mean versus the overall mean of the dataset were observed. The classes can be assigned as follows: Southern Manitoba (C1); a Prairie Pothole region (C2 and C3); Major River Valleys (C4); and Grasslands (C5–C7).
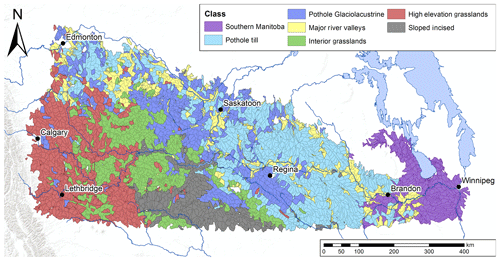
Figure 5Classification of Prairie ecozone watersheds. Watershed delineations are from Lehner and Grill (2013), available at https://www.hydrosheds.org/ (last access: 11 August 2018). See text for detailed interpretation of the seven clusters.
Southern Manitoba (C1)
The majority of Class 1 (C1; n=365) watersheds occurred in the eastern Prairie south of Lake Winnipeg (Fig. 5), and thus “Southern Manitoba” is used as the class name. Distinguishing characteristics associated with this class included soil zone PC1 (predominantly black soils) and cropland practice PC1 (predominantly conventional till) (Table 4). Southern Manitoba had a high incidence of glaciolacustrine and alluvial deposits, as indicated by moderately negative and positive relationships with surficial geology PC1 and PC2, respectively, and the class also had low mean elevation. Topography tended to be level, with mild slopes and strong association with land surface form PC3 (Table 4). Notably, these watersheds exhibited both high annual precipitation and PET compared to other classes, and this class was the only one to have no mean moisture deficit (i.e., precipitation – PET > 0) (Fig. 6). Southern Manitoba watersheds also exhibited smaller fractions of non-effective areas and grasslands than other classes (Fig. 7).
Table 4Classes and distinguishing variables of Prairie watersheds. The v-test statistics, based on Ward's criterion, are shown. Variables with v-test values greater or less than 10 and −10, respectively, are bolded to emphasize defining features of each class. All variables are significant to p<0.001. Classes: Southern Manitoba (1), Pothole Till (2), Pothole Glaciolacustrine (3), Major River Valleys (4), Interior Grasslands (5), High Elevation Grasslands (6), Sloped Incised (7).
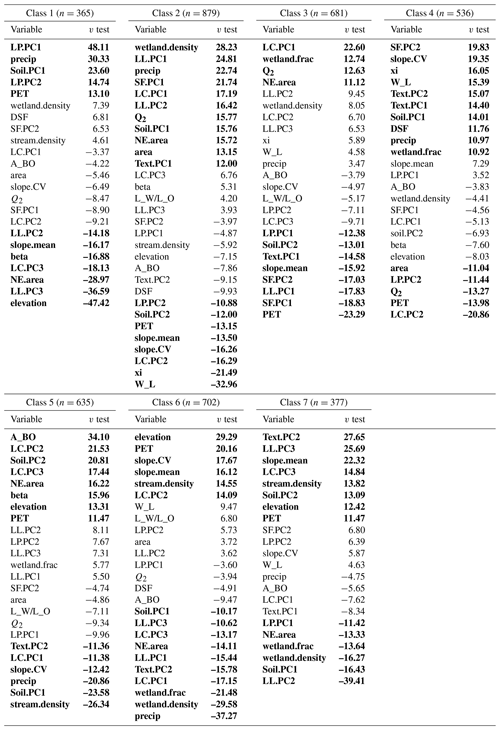
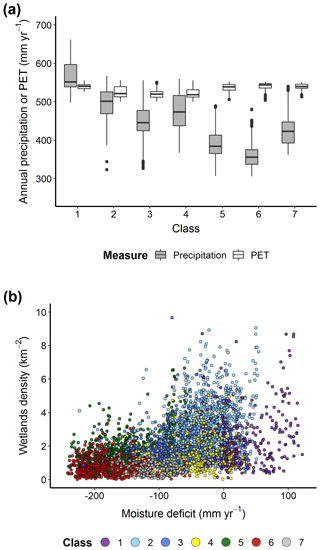
Figure 6Climatic variation among watershed classes. (a) Boxplots of total annual precipitation (grey) and potential evapotranspiration (PET) (white) for each watershed cluster. Lower, middle, and upper limits of boxes show the 25th, 50th, and 75th quantiles, respectively. (b) Wetland density to moisture deficit (precipitation−PET). Classes: Southern Manitoba (1), Pothole Till (2), Pothole Glaciolacustrine (3), Major River Valleys (4), Interior Grasslands (5), High Elevation Grasslands (6), Sloped Incised (7).
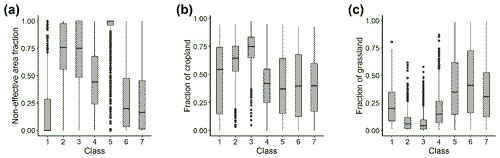
Figure 7Boxplots of select variables by watershed class: (a) fraction of non-effective area; (b) fraction of cropland; and (c) fraction of grassland. Classes: (1) Southern Manitoba, (2) Pothole Till, (3) Pothole Glaciolacustrine, (4) Major River Valleys, (5) Interior Grassland, (6) High Elevation Grasslands, and (7) Sloped Incised.
Prairie Potholes (C2 and C3)
The Prairie Pothole group, consisting of Class 2 (C2; n=879), or Pothole Till, and Class 3 (C3; n=681), Pothole Glaciolacustrine, represent the largest class of watersheds spatially, spanning the northern part of the Alberta Prairie to the southeastern part of Saskatchewan (Fig. 5). Mean annual precipitation was relatively high for the study area, contributing to a slightly negative moisture deficit (Fig. 6). These watersheds contained large fractions of non-effective area (∼75 %) (Fig. 7a), and they exhibited positive scores on land-cover PC1 (Table 4) indicating high cropland cover (∼70 %), whereas unmanaged grassland cover was typically very low (<20 %) (Fig. 7b and c). On average, Pothole watersheds had high wetland densities (wetlands km−2), with C2 exhibiting the greatest density of all classes (Fig. 8a).
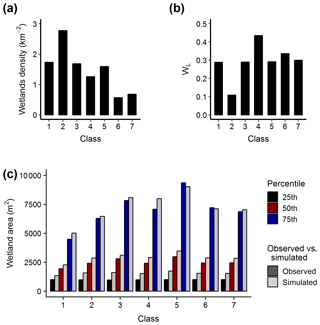
Figure 8Wetland variables and simulated size distributions. Median (a) density of wetlands and (b) fraction of total watershed water area in the largest wetland (WL) are depicted by class. (c) shows observed (dark) and simulated (light) percentiles of wetland areas. Predicted values are based on a generalized Pareto distribution and using median parameters of β and ζ for each cluster. Simulated data were restricted to the raster pixel resolution of observed data from the Global Surface Water dataset. Classes: Southern Manitoba (1), Pothole Till (2), Pothole Glaciolacustrine (3), Major River Valleys (4), Interior Grasslands (5), High Elevation Grasslands (6), Sloped Incised (7).
Surficial geology differentiated classes C2 and C3. Overall, glacial till and hummocky landforms dominated the pothole region; however, C2 was more associated with these characteristics, scoring greater mean values on PC1 of local surface form and surficial geology. In contrast, glaciolacustrine deposits were more common in C3, and soils had a higher incidence of clay and silt, where C2 watersheds were sandier (Table 4). Although both classes contain many wetlands, C2 watersheds had the smallest values of WL, indicating a lower areal water extent was contained in the largest wetland (Fig. 8b).
Major River Valleys (C4)
Class 4 (C4; n=536) watersheds were associated with river valleys, and as such, extend across the Prairie region (Fig. 5) and generally coincide with major rivers (e.g., North and South Saskatchewan, Qu'Appelle) and large lakes. These watersheds had the greatest value of the fraction of water area in the largest depression (WL) (Fig. 8b), as well as high slope CV, wetland fraction, and fractions of black soil (i.e., higher soil zone PC1 scores) (Table 4). These watersheds were also associated with soil texture PC1 and surficial geology PC2, suggestive of higher incidence of sandy riverine deposits (e.g., alluvial and glaciofluvial deposits). The Major River Valleys class tended to have a large “wetland” area, which is interpreted as the area of water of these rivers.
Taken together, these watersheds were related to parameters typical of fluvial environments, including glaciofluvial or alluvial deposits, and sandier soils. Large values of the mean and CV of slopes were also typical of river valley watersheds. About half the basin area tends to be non-effective in these watersheds, compared to the much greater fractions in the Pothole regions (Fig. 7a) that surround many of the Major River Valleys watersheds. Being river valleys, C4 watersheds were generally narrow and small in area. Higher DSF (i.e., narrower watersheds) and smaller areas were generally associated with lower Q2 values (Table 2). Thus, although these watersheds have a high likelihood of contributing to streamflow of major rivers, the watershed Q2 contributions were predicted to be small (Table 4).
Grasslands (C5–C7)
The southwestern Canadian Prairie, which includes the majority of southern Alberta and western Saskatchewan between the South Saskatchewan River and the Cypress Hills, was occupied by classes C5–C7. These watersheds tended to have large factions of unmanaged grasslands (negative land-cover PC1) and mean elevation (Table 4). Compared to the rest of the Prairie, this sub-region tended to be arid, with a strong moisture deficit (Fig. 6). As a result, these classes exhibited relatively low wetland density (Fig. 8a).
Classes 5 (C5; n=635), Interior Grasslands, and 6 (C6; n=702), High-Elevation Grasslands, were characteristic of the grasslands in southeastern Alberta. These watersheds had the greatest values of mean fractional grassland area, with cropland and grassland fractions being comparable (35 %–40 %) (Fig. 7). Distinguishing features of Interior Grasslands were greater values of the fraction of area below the basin outlet, ABO, and a notably large non-effective area fraction (Fig. 7a). High scores on land-cover PC2 and PC3 indicate large fractions of fallow and pasture. These watersheds also scored higher on soil zone PC2, suggesting more common occurrences of brown soils. Small magnitudes of mean slope and stream densities were observed, suggesting that the wetlands within the Interior Grasslands are relatively disconnected from the drainage network. This characteristic might explain why these watersheds have relatively large wetlands (Fig. 8c). In contrast, High Elevation Grasslands were characterized by greater mean elevation and slope values, and smaller non-effective fractions (Table 4; Fig. 7). These watersheds also had greater stream densities and smaller wetland densities.
Class 7 (C7; n=377), Sloped Incised, watersheds are characterized by dissected, river-incised landscapes, as indicated by positive associations with local surface form PC3 (Table 4). Like High Elevation Grasslands (C6), Sloped Incised watersheds followed the Bow, Red Deer, as well as Milk River valleys, suggesting a similar function to those of the Major River Valleys class. Wetland density is smallest in Sloped Incised watersheds, owing to their steepness, resulting in surface water reaching stream networks rather than collecting on the landscape (Fig. 8).
4.3 Predicting wetland size distributions from class parameters
Simulated wetland area distributions by class were compared to observed size distributions from study watersheds to evaluate the concordance of the approximate class-specific distribution to that of the observed distributions of watersheds, collectively. The median wetland density was greatest in C2, followed by C3, C1, and C5 (Fig. 8a). The median wetland densities in C6 and C7 were less than 1 km−2. C4 had the greatest areal fraction of water in the largest wetland (WL), which was over 40 % (Fig. 8b), while C2 had the smallest value at ∼10 %. For the rest of the classes, this value was between 28 % and 34 %. The simulated wetland area distributions slightly overestimated those of the observed values, especially at the 25th percentile. However, the patterns of wetland area in the quartiles was generally consistent among all classes (Fig. 8c). The area of the smallest 25 % of the wetlands appears quite consistent across the classes, with more variation occurring at higher percentiles. The largest difference among classes in wetland size was in the 75th percentile, with the greatest range being in C5 and the smallest in C1.
4.4 Resampling and re-classifying procedure
The HCPC and watershed classification was repeated with 10 random subsets of 3757 watersheds. The majority of watersheds were removed from at least one iteration, with only 50 watersheds being removed a total of 4–6 times (Fig. S3). This resulted in 10 unique watershed subsets to test clustering and agreement with the seven classes, outlined above.
Percent membership agreement of a watershed varied by class, with the majority of classes exhibiting high agreement even after resampling. Classes exhibiting high membership agreement were Pothole Till (C2), Interior Grasslands (C5), High Elevation Grasslands (C6), and Sloped Incised (C7), with a large proportion having more than 90 % agreement with the seven classes from the complete classification (Fig. 9; Table S4). Although a large mean agreement was observed overall, a few watershed classes exhibited low agreement and inconsistent classification. Southern Manitoba (C1) exhibited a bimodal distribution, where most were generally classed as C1 over 75 % of the time and a second set only ∼60 % agreement (Fig. 9). This was due to a new class appearing (Fig. 10). Hereafter, this class is referred to as “Eastern Manitoba”. Briefly, Eastern Manitoba was associated with a large fraction of conventional tillage practice (i.e., positive association with land-practice PC1 and land-practice PC2) and large fractional effective areas (data not shown). The Major River Valleys class was the only one that did not include a watershed that achieved 100 % agreement across the 10 iterations; this class exhibited a peak of total agreement at approximately 60 % (Fig. 9). Where Major River Valleys watersheds were classified inconsistently, the most common alternative classification were Pothole Glaciolacustrine (C3) or secondarily High Elevation Grasslands (C6) (Fig. 10). The loss of Major River Valleys occurred for iterations when the Eastern Manitoba class (C8) became apparent.
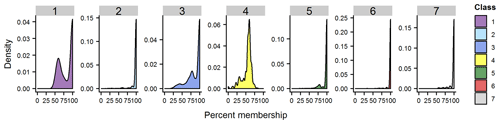
Figure 9Density distributions of percent agreement of watersheds with the classification in Fig. 5 by watershed class. Classes: Southern Manitoba (1), Pothole Till (2), Pothole Glaciolacustrine (3), Major River Valleys (4), Interior Grasslands (5), High Elevation Grasslands (6), Sloped Incised (7).
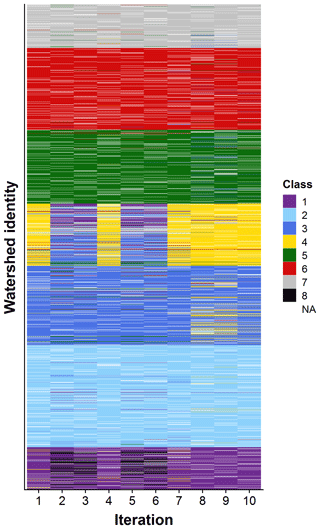
Figure 10Agreement of assigned watershed classification from the (original) complete analysis, with class assignments from the iterative approach using re-sampling. Classes are coloured according to that shown in Fig. 5, with those identified under a new class (C8) depicted in black. Watersheds that were removed from the subsets analyzed are in white. Classes: Southern Manitoba (1), Pothole Till (2), Pothole Glaciolacustrine (3), Major River Valleys (4), Interior Grasslands (5), High Elevation Grasslands (6), Sloped Incised (7).
5.1 Classifying Prairie watersheds
5.1.1 Hydrological approaches
Our classification procedure grouped watersheds of approximately 100 km2 into seven classes. Few studies anywhere have classified watersheds at this granularity, and our investigation gives particular attention to characteristics that influence hydrological and ecological behaviour. Many previous studies in the region spanned larger areas, and this often results in the Prairie being identified as a homogenous region due to relatively low streamflow and atypical geology and surface topography (MacCulloch and Whitfield, 2012; Mwale et al., 2011). Our results are novel in that they characterize in greater detail, and at small watershed scales, the potential for different hydrological behaviour of watersheds within the region. The only similar example that was found in the literature was by Durrant and Blackwell (1959), whose findings parallel those of this study, but at a larger watershed scale. Durrant and Blackwell (1959) described broad regions of Saskatchewan and Manitoba based on mean annual flood, distinguishing five sub-regions, including southwestern Saskatchewan, northern and central Saskatchewan, and southern Manitoba near the Red River and Assiniboine River confluence. In the current study, surficial geology and land surface form strongly influenced how grasslands were separated into three classes, which reinforces the role of local topography in hydrological response, as seen elsewhere (Mwale et al., 2011). Likewise, surficial geology was particularly important for distinguishing the Pothole (Till and Glaciolacustrine) classes. Similarities to the work of Durrant and Blackwell (1959) based on streamflow in larger basins suggest that our approach, with consideration of factors important to watershed behaviour, can yield classification with relevance to hydrologic function, despite the use of few hydrologic indices in our analysis (Fig. 5). This approach holds potential for use in other regions of the world that are dry or ungauged or feature low effective areas, and thus cannot rely on streamflow characteristics as a primary means of classification according to functional behaviour.
Our classification grouped Prairie watersheds using geological, biophysical, and hydroclimatic attributes. In their review of classification approaches, Sivakumar et al. (2013) indicate that solely using geographic data is advantageous when there are limited hydrological data; however, the relationship between physical attributes and hydrologic behaviour is not necessarily definitive in all regions. For these reasons, it was important to include traits indicative of structural hydrological connectivity, such as Q2 estimates and wetland parameters. It is important to note that while Q2 emerged as a defining feature for several of the classes, it was consistently one of many variables important for characterization of that class (Table 4), suggesting that while it provides value added, it does not stand out as a major driving factor in the classification. In particular, the immature drainage network and relatively high depressional water storage capacity make Prairie hydrology relatively distinct (Jones et al., 2014; Shook et al., 2013, 2015). Notably, three classes (i.e., Pothole Till, Pothole Glaciolacustrine, and Interior Grasslands) occur almost exclusively within regions that tend not to contribute to major river systems and collectively encompass the majority of the study area (Table 4; Fig. 5). It is therefore expected that hydrological response will be very different between classes that exhibit higher hydrological connectivity (i.e., potentially lower wetland to stream densities and non-effective area fractions), such as the Major River Valleys or Sloped Incised watersheds, than those that do not, such as Pothole classes.
5.1.2 Ecoregions and human impacts
Ecoregions are commonly used to characterize landscapes according to geographical or ecological similarity (Omernik and Griffith, 2014). Similar to our approach, ecoregion classifications are often hierarchical in nature, allowing for differing levels of detail and spatial extent and thus defining characteristics depending on the scale of interest (Loveland and Merchant, 2004). Ecoregion classifications used in the United States (Omernik and Griffith, 2014) and Canada (Ecological Stratification Working Group, 1995) employ a “top-down” approach, where broad categories are partitioned into smaller, more specialized units. In contrast, our approach provides a bottom-up, agglomerative approach where similar watersheds are merged. Assumptions are inherent in either approach; however, the latter was applicable to the current study to allow for grouping of watersheds given similarities in physiographic characteristics. This approach does not limit class membership to the geographic extent of a higher level class, allowing for membership to potentially span the geographic extent of the Canadian Prairie domain (Fig. 5).
Despite the differing methods for distinguishing similarities (or differences), arrangements of watershed classes in some cases exhibited similar ranges to ecoregion boundaries. The boundaries of Lake Manitoba Plain and Mixed Grassland ecoregions (Ecological Stratification Working Group, 1995) correspond roughly to those of the broader Southern Manitoba (C1) and Grasslands (C5–C7) classes, respectively (Fig. S4). Mwale et al. (2011) also found that annual hydrological regimes based on data from 200 stations and physical attributes in Alberta linked closely with provincial ecoregions. Our emphasis on inclusion of hydrologically relevant characteristics, such as wetland traits and effective areas that are likely important contributors to function, has proven useful for further distinguishing among the Grassland classes as well as the Pothole classes (C2 and C3) (Figs. 5 and S4). Due to the fundamental differences in effective areas and in wetland versus river-dominated systems (Table 4; Fig. 8), we expect different hydrological behaviour between these classes. This is an advantage of the HCPC classification approach in that it allows for identifying the potential similarity at relatively fine spatial scales and does not require similar watersheds to be physically adjacent to one another. This confers the opportunity to further investigate these systems, such as through hydrological modelling and contrasting resulting responses under climate and land-use scenarios.
The highly managed Prairie landscape reinforces the importance of considering anthropogenic alteration in hydrological understanding. Crop rotation and the ways in which fields are managed for winter affect the accumulation and redistribution of snow (Fang et al., 2010; Harder et al., 2018; Van der Kamp et al., 2003). Spring snowmelt and consequent runoff are imperative to summer surface water availability (Dumanski et al., 2015; Shook et al., 2015), and depression-focused recharge of snowmelt into groundwater facilitates storage and mitigates flood impacts (Hayashi et al., 2016). Thus, classifying procedures in the Prairie must consider the human influence on the water cycle.
An example of the complexities introduced by human land-management activities can be shown by the C1 (Southern Manitoba) watersheds, where the land-practice variable was a strong class descriptor. Agricultural activity is high everywhere in the Prairie; however, only C1 was associated with low zero-till practices, instead favouring conventional tillage (Table 4). Manitoba has seen less coherent adoption of zero-till practices since the early 1990s compared to Alberta and Saskatchewan, with conventional or other conservation till practices remaining common in Manitoba (reviewed in Awada et al., 2014). Sustained use of conventional tillage practice within this region may increase the risk of soil erosion, which can negatively affect downstream water bodies (Cade-Menun et al., 2013). This practice, combined with landscape modifications, such as artificial drainage networks, serves to facilitate removal of water and may contribute to concurrent nutrient export from agricultural lands (Weber et al., 2017).
These management practices can be viewed as a trade-off, where high numbers of wetlands and level topography can pose flood risk during wet periods as wetlands fill and merge (Leibowitz et al., 2016), inundating tracts of adjacent land. Conversely, where landscape modification to enhance water export occurs, local, field-scale flood risk may be reduced while heightening the risk of downstream flooding. Land use and land management are important factors in understanding the connectivity and chemical transport in prairie landscapes (Leibowitz et al., 2018). In southern Manitoba, where artificial drainage has been used to increase the area of arable land, beneficial management practices in the form of agricultural reservoirs have been implemented as a means of reducing nutrient export and improving downstream water quality while also mitigating the risk of downstream flooding (Gooding and Baulch, 2017). These factors illustrate the complexities when classifying and understanding the hydrological response of watershed embedded in highly managed landscapes and underscore the necessity of considering the human influence on the water cycle in such approaches.
5.2 HCPC as a clustering and classification framework
5.2.1 Using the HCPC approach and limitations
The HCPC method provides a procedure for integrating multiple physiographic attributes and describes resulting clusters by sets of significant variables (Husson et al., 2009). As discussed above, an advantage of the method is that it groups individual watersheds based on similarities. Therefore, it lends itself well as a foundation for investigating hydrological behaviour through modelling efforts. In the case of the current study, modelling efforts can be applied at a 100 km2 scale to evaluate responses to environmental changes. An additional advantage is that one may select variables or sets of variables of interest to inform the clustering of watersheds, such as those based only on topographic parameters or those dictating local hydrology. For example, climate variables may be excluded if the goal of the classification is parameterizing a hydrological model, as these variables could instead be described by local climate forcing. The relative ease with which different sets of variables can be added to or excluded from the analysis to consider different permutations of the classification is a real strength of the approach. Although this may result in differing cluster results, assessment of how these classes change with addition or removal of certain datasets can identify the variables that control class definition as well as elucidate spatial patterning of classes.
There are a few considerations when using this method. First, the linear restrictions of this method are challenging when working with environmental data, which often do not conform to assumptions of normality. Non-linear PCA methods and self-organizing maps have been applied successfully to classify watersheds in Ontario and to regionalize streamflow metrics (Razavi and Coulibaly, 2013, 2017). Although these methods might be logical next steps for the current study, we chose to focus on conventional PCA due to its smaller computational cost when classifying the large number of watersheds in our study.
Second, the current analysis weighs all variables equally. This can bias the analysis towards attributes that exhibit greater variability, as these can overshadow other more constrained variables. For example, the location of the largest pond relative to the watershed outlet (coded as LW∕LO) is important for controlling local prairie hydrology and hydrological gate-keeping potential (i.e., the likelihood of releasing surface water to the next-order watershed) (Shook et al., 2013, 2015) and water quality (Hansen et al., 2018). Despite its hydrological importance, this variable had little influence on the clustering procedure overall and was only a minor descriptor in certain classes, such as C5 and C6 (Table 4).
The original set of watersheds in the clustering analysis can affect the final classification; however, there was a high degree of agreement between classified subsets of the original dataset and the classification generated using the complete set of watersheds (n=4175) (Fig. 9). Overall, watersheds designated as part of the Pothole and Grassland classes were classified consistently, with most exhibiting over 90 % agreement. Major River Valleys exhibited the weakest agreement (Fig. 9), due to the appearance of a unique (new) class consistent with the Lake Manitoba Plain ecoregion (Fig. S4) for some of the subsets. In these cases, those watersheds previously classified as Major River Valleys were re-distributed to mainly High Elevation Grasslands or Pothole classes depending on dominant watershed features (Fig. 10). Although we do not include a detailed account of the new Eastern Manitoba class that emerged during this exercise, defining characteristics included a high fraction of effective area (i.e., the easternmost portion of the Prairie in Fig. 1), low relief, and lower use of zero-till agriculture (as reviewed in Awada et al., 2014), since this new class would not be expected to translate to notable differences in management outcomes. Moreover, previous reviews of the usefulness of ecoregion classifications agree that strict geographic boundaries are unlikely and are instead more likely “fuzzy” (Loveland and Merchant, 2004; Omernik and Griffiths, 2014).
Class membership in our approach is also determinate. In reality, there can be large variability in attributes within a class (e.g., Fig. 7), and membership is determined by the collective similarity of watershed attributes. Previous studies have used fuzzy c-means and Bayesian approaches that can assign a likelihood of membership to classes (Jones et al., 2014; Rao and Srinivas, 2006; Sawicz et al., 2011). An advantage to this approach is that it allows for fuzzy boundaries between classes where a gradient of features likely exists (Loveland and Merchant, 2004). Our re-classifying analysis supports the proposition that boundaries among classified regions are fuzzy and some watershed might flicker among class memberships (Fig. 10). Such approaches are also unsupervised and probabilistic in nature and will eliminate the subjectivity due to the researcher pre-defining the number of classes. Future work thus should consider these fuzzy boundaries and potential for watersheds to exhibit partial membership to multiple classes.
5.2.2 Data quality and availability
The classes resulting from the HCPC are also ultimately dependent on the types of data included. The availability of data and their geographic coverage determined the environmental parameters included in our analyses. Ideally, a more detailed estimate of runoff for each watershed would be a valuable contribution. In the current study, we used the CCA and 11 reference stations to approximate runoff values for the clustering watersheds. Given the number of watersheds included in the analyses, the diversity of physical characteristics and potential hydrological behaviour is likely not completely represented in the small sample size of available hydrometric stations and is a limitation of our approach. Soil moisture would be important to consider in future studies given its role in influencing vegetation community composition, PET, and overall water balance (Hayashi et al., 2003; Shook et al., 2015). Where data are available, future work should consider variables related to snow formation and melt, as well as the proportion of annual precipitation as snowfall. These variables are likely influential when describing hydrological behaviour of the watersheds and classes in the current study and other cold regions (Knoben et al., 2018; Shook and Pomeroy, 2012). Furthermore, a comprehensive wetland inventory or an index of wetland drainage activity that is comparable across the three provinces does not currently exist. These would be valuable additions to future efforts to classify Prairie watersheds given the important role of land modification in watershed functions.
One consideration with the Global Surface Water dataset is that the pixel size (30 m) is quite coarse and will miss numerous smaller wetlands, underestimating the number of wetlands observed. Consequently, it is likely that the analysis omitted some ephemeral wetlands for which persistence is short and size is small. Despite their known important ecological functions (Calhoun et al., 2017; Van Meter and Basu, 2015), their size and transient nature are a challenge to their inclusion in comprehensive datasets spanning large geographic areas. This may inadvertently result in the role of smaller wetlands being under-represented in our analysis or others that rely on this dataset.
Use of the ξ and β parameters as indices of the wetland area frequency distributions was shown to estimate class area distributions reasonably well (Fig. 8c). Although for consistency, we restricted our simulated dataset to the spatial resolution of the surface water raster, one could use these parameters to estimate the frequencies of smaller wetlands in watersheds, which would otherwise be missed by satellite measurements, assuming conformity to a generalized Pareto distribution (Shook et al., 2013). Our analysis supports this application as simulated wetland areas generally approximated those seen across the observed data (Fig. 8c). Nonetheless, in regions where wetland drainage has been undertaken, it is expected that wetland area distribution has been altered via preferential loss of smaller water bodies (Evenson et al., 2018; Van Meter and Basu, 2015). This is exacerbated by the fact that remotely sensed satellite data tend to omit smaller, ephemeral ponds. A more robust characterization of the size and permanence of wetlands in our study watersheds would be expected to improve the current dataset and enhance the clustering and classification analyses.
5.3 Management implications
Classification frameworks help to define sub-regions with potentially similar characteristics or behaviours. For example, climatic zones can be delineated, specifically the dry Grassland watersheds in the southwest and the wet Potholes in the northeast and in Manitoba (Fig. 5). In some cases, this may be related to local wetland densities, with large densities observed corresponding to low moisture deficits (Fig. 6b) (Liu and Schwartz, 2012). Climate variation may divide watersheds with seemingly similar geography into differing classes, as is the case with Major River Valleys and Sloped Incised watersheds. Both sets of watersheds tended to follow river valleys, but the former exhibit greater precipitation and smaller PET (Table 4). These divisions can be used to give context to regions we might expect to behave similarly, whether hydrologically or ecologically, based solely on physical attributes, and echoes other methods, such as ecodistricts (Ecological Stratification Working Group, 1995) to classify landscapes. For example, areas that are geologically similar may differ in terrestrial or aquatic community assemblages, which should influence how each area might be managed (Jones et al., 2014; Wagner et al., 2007). If classifications are used to inform management, the resulting decisions for a given location will depend on the strength of the delineation, the scale at which management is applied, relationships among management practices and the attributes used to define that area, and the relationship of those attributes with the response variable of concern (Wagner et al., 2007).
This set of analyses was unique among watershed classification exercises in Canada in that it considered a suite of wetland variables. The arrangement of wetlands or landscape depressions and their size distribution define the hydrological behaviour of Prairie watersheds (Shook et al., 2015; Shook and Pomeroy, 2011). The storage capacity and subsequent spilling or merging control wetland connectivity and thus the quantity of water available to move from one watershed to another (Leibowitz et al., 2016; Shaw et al., 2012; Shook et al., 2015). In turn, a wetland or depression's hydrological gate-keeping potential, or its likelihood to prevent connectivity to the downstream watershed, is a function of both its storage capacity and landscape position. Large wetlands near an outlet have a great gate-keeping potential, as they block much of the watershed from connecting, and it takes a great deal of water to fill them before permitting flow to the next-order watershed (Shook and Pomeroy, 2011). Simulated frequency distributions of wetland areas indicate that the depressional storages of the classes are very different (Fig. 8). It may be that wetland management practices will have different influences between each pothole class, and possibly among all the classes. This has implications for managing salinizing soils (Goldhaber et al., 2014), biodiversity (Balas et al., 2012), and flooding potential (Evenson et al., 2018; Golden et al., 2017).
Wetland drainage and wetland consolidation change hydrological connectivity and therefore the transport of nutrients and their loading into receiving water bodies (Brown et al., 2017; Vanderhoof et al., 2017). More positive values of the moisture deficit (i.e., where P >= PET) were associated with greater wetland densities (Fig. 6b) (Liu and Schwartz, 2012), and these areas were generally associated with greater fractions of cropland, such as the Pothole Till, Pothole Glaciolacustrine, and Southern Manitoba watersheds. In these regions wetland drainage is widely practised, historically or at present, and conflict over available arable land and wetland conservation is high (Breen et al., 2018).
Extensive drainage in combination with agricultural activity is known to increase the risk of agricultural nutrient mobility (Kerr, 2017) from the landscape to receiving water bodies. Increased connectivity also reduces water residence time and thus tends to decrease wetland nutrient retention (Marton et al., 2015). Over time, zero-till practices can promote nutrient stratification in soils, where concentrations (especially phosphorus) accumulate at the surface, which can increase nutrient loading when surface runoff is generated (Cade-Menun et al., 2013). The cropland–wetland interface might also have important implications for pesticide mobility in Pothole Till and northern Pothole Glaciolacustrine watersheds. These areas coincide with extensive use of canola, which has been linked to high application rates of neonicotinoid pesticides which are known to have high persistence in small, temporary wetlands (Main et al., 2014). Watersheds in the Pothole Till class appear to have more hummocky landscapes than the Pothole Glaciolacustrine classification and smaller, more numerous wetlands (Fig. 8). Moreover, the water area fraction occupied by the largest wetland differs between the classes. The landscape biogeochemical functionality of pothole wetlands is known to vary considerably according to pothole character (Evenson et al., 2018; Van Meter and Basu, 2015). As such, our classification may highlight contrasting biogeochemical functioning, including nutrient retention, between these classes. Thus, although water quality risks are common within the region, the classes may respond very differently to environmental and land-management stresses.
This study provides an overview of a classification framework that can be applied in regions with limited understanding of or data describing streamflow. The HCPC procedure offers a flexible analysis to elucidate the spatial arrangement of watershed classes given a large number of units to classify and a diverse set of attributes to inform the classification. In contrast to classifications based solely on hydrological function, using physiographic data allows for classifying small basins, which are unlikely to be gauged, and confers advantages over alternate procedures that rely heavily on observations of hydrological parameters, namely statistics describing streamflow.
Use of the classification approach for small Canadian Prairie watersheds identified regions of similar climatic and geographic features and, potentially, of hydrological response (Fig. 5). This yielded watershed classes that consider not only drainage patterns, but also land cover, land use, and the underlying geology. In the Prairie region, wetland variables incorporate the hydrologic gate-keeping potential of wetlands as well as parameters indicative of wetland size distributions. With the classification based on a large and diverse set of attributes, a diversity of behaviours is captured. This represents a major step forward for classification of Prairie watersheds that have to date offered only a much more homogenized depiction of watershed function in the region. The watershed classification framework presented promises to be useful in other dry or semi-arid regions, and those that are poorly gauged. Given the inclusive nature of the classification approach, which incorporates landscape controls on hydrology as well as those influencing biogeochemistry and ecology, it also provides a foundation to evaluate the efficacy of land and watershed management practices in the context of a changing climate.
All datasets used in our analysis are publicly available and can be accessed via the references and links we have provided. Please see Sect. 2: Data Availability and Compilation as well as Table S2 in the Supplement for relevant links and references for datasets.
The supplement related to this article is available online at: https://doi.org/10.5194/hess-23-3945-2019-supplement.
JDW, CJW, and CS conceived the study, and JDW collected data and performed analyses. KRS wrote code to analyze basin and wetland data. JDW wrote the manuscript with input from all the co-authors.
The authors declare that they have no conflict of interest.
This article is part of the special issue “Understanding and predicting Earth system and hydrological change in cold regions”. It is not associated with a conference.
This work was pursued under the Prairie Water project and funded by the Global Water Futures program, which was supported by the Canada First Research Excellence Fund. The authors would like to thank John Pomeroy for his valuable input on the scoping and approach to the study. We would also like to thank Wouter Knoben and two anonymous reviewers for their insightful comments on the manuscript. Finally, we would like to thank the Prairie Water team and the Global Institute for Water Security for ongoing support.
This research has been supported by the Canada First Research Excellence Fund (grant no. 418474).
This paper was edited by Chris DeBeer and reviewed by Masaki Hayashi, Wouter Knoben, and one anonymous referee.
AAFC: Soils of Canada, Derived, Soil Landscapes of Canada and Detailed Soil Surveys, version 3.2, Canadian Soil Information Service, Agriculture and Agri-Food Canada, Government of Canada, available at: https://open.canada.ca/data/en/dataset/8f496e3f-1e54-4dbb-a501-a91eccf616b8 (last access: 16 March 2018), 2013.
AAFC: Detailed Soil Surveys, Agriculture and Agri-Food Canada, Government of Canada, available at: https://open.canada.ca/data/en/dataset/7ed13bbe-fbac-417c-a942-ea2b3add1748 (last access: 16 March 2018), 2015.
AAFC: Annual Crop Inventory, Agriculture and Agri-Food Canada, Government of Canada, available at: https://open.canada.ca/data/en/dataset/ba2645d5-4458-414d-b196-6303ac06c1c9 (last access: 30 November 2017), 2016.
Ameli, A. A. and Creed, I. F.: Does Wetland Location Matter When Managing Wetlands for Watershed-Scale Flood and Drought Resilience?, J. Am. Water Resour. Assoc., 55, 529–542, https://doi.org/10.1111/1752-1688.12737, 2019.
Atkinson, N., Utting, D. J., and Pawley, S. M.: Surficial geology of west-central Alberta (GIS data, polygon features); Alberta Energy Regulator, AER/AGS Digital Data 2017-0031, available at: http://ags.aer.ca/publications/DIG_2017_0031.html, last access: 6 December 2017.
Awada, L., Lindwall, C. W., and Sonntag, B.: The development and adoption of conservation tillage systems on the Canadian Prairies, Int. Soil Water Conserv. Res., 2, 47–65, https://doi.org/10.1016/S2095-6339(15)30013-7, 2014.
Balas, C. J., Euliss, N. H., and Mushet, D. M.: Influence of conservation programs on amphibians using seasonal wetlands in the prairie pothole region, Wetlands, 32, 333–345, https://doi.org/10.1007/s13157-012-0269-9, 2012.
Begou, J., Bazie, P., and Afouda, A.: Catchment classification: multivariate statistical analyses for physiographic similarity in the Upper Niger Basin, J. Eng. Res. Appl., 5, 60–68, 2015.
Breen, S.-P. W., Loring, P. A., and Baulch, H.: When a Water Problem Is More Than a Water Problem: Fragmentation, Framing, and the Case of Agricultural Wetland Drainage, Front. Environ. Sci., 6, 1–8, https://doi.org/10.3389/fenvs.2018.00129, 2018.
Brown, R., Zhang, Z., Comeau, L. P., and Bedard-Haughn, A.: Effects of drainage duration on mineral wetland soils in a Prairie Pothole agroecosystem, Soil Till. Res., 168, 187–197, https://doi.org/10.1016/j.still.2016.12.015, 2017.
Brown, S. C., Lester, R. E., Versace, V. L., Fawcett, J., and Laurenson, L.: Hydrologic landscape regionalisation using deductive classification and random forests, PLoS One, 9, e112856, https://doi.org/10.1371/journal.pone.0112856, 2014.
Bulley, H. N. N., Marx, D. B., Merchant, J. W., Holz, J. C., and Holz, A. A.: A comparison of Nebraska reservoir classes estimated from watershed-based classification models and ecoregions, J. Environ. Inform., 11, 90–102, https://doi.org/10.3808/jei.200800114, 2008.
Burn, D.: Cluster analysis as applied to regional flood frequency, J. Water Resour. Pl.-Manage., 115, 567–582, 1990.
Cade-Menun, B. J., Bell, G., Baker-Ismail, S., Fouli, Y., Hodder, K., McMartin, D. W., Perez-Valdivia, C., and Wu, K.: Nutrient loss from Saskatchewan cropland and pasture in spring snowmelt runoff, Can. J. Soil Sci., 93, 445–458, https://doi.org/10.4141/cjss2012-042, 2013.
Calhoun, A. J. K., Mushet, D. M., Bell, K. P., Boix, D., Fitzsimons, J. A., and Isselin-Nondedeu, F.: Temporary wetlands: challenges and solutions to conserving a “disappearing” ecosystem, Biol. Conserv., 211, 3–11, https://doi.org/10.1016/j.biocon.2016.11.024, 2017.
Cavadias, G. S., Ouarda, T. B. M. J., Bobee, B., and Girard, C.: A canonical correlation approach to the determination of homogeneous regions for regional flood estimation of ungauged basins, Hydrol. Sci. J., 46, 499–512, https://doi.org/10.1080/02626660109492846, 2001.
Coles, A. E., McConkey, B. G., and McDonnell, J. J.: Climate change impacts on hillslope runoff on the northern Great Plains, 1962–2013, J. Hydrol., 550, 538–548, https://doi.org/10.1016/j.jhydrol.2017.05.023, 2017.
DeBeer, C. M., Wheater, H. S., Carey, S. K., and Chun, K. P.: Recent climatic, cryospheric, and hydrological changes over the interior of western Canada: A review and synthesis, Hydrol. Earth Syst. Sci., 20, 1573–1598, https://doi.org/10.5194/hess-20-1573-2016, 2016.
Detenbeck, N. E., Batterman, S. L., Brady, V. J., Brazner, J. C., Snarski, V. M., Taylor, D. L., Thompson, J. A., and Arthur, J. W.: A Test of Watershed Classification Systems for Ecological Risk Assessment, Environ. Toxicol. Chem., 19, 1174, https://doi.org/10.1897/1551-5028(2000)019<1174:ATOWCS>2.3.CO;2, 2000.
Devito, K., Creed, I., Gan, T., Mendoza, C., Petrone, R., Silins, U., and Smerdon, B.: A framework for broad-scale classification of hydrologic response units on the Boreal Plain: Is topography the last thing to consider?, Hydrol. Process., 19, 1705–1714, https://doi.org/10.1002/hyp.5881, 2005.
Dumanski, S., Pomeroy, J. W., and Westbrook, C. J.: Hydrological regime changes in a Canadian Prairie basin, Hydrol. Process., 29, 3893–3904, https://doi.org/10.1002/hyp.10567, 2015.
Durrant, E. F. and Blackwell, S. R.: The magnitude and frequency of floods in the Canadian Prairies, Proc. Of Symposium No. 1, Spillway Design Floods, sub-committee on hydrology, National Research Council Associate Committee on Geodesy and Geophysics, The Queens Printer, Ottawa, 1959.
ECCC: Canadian Gridded Temperature and Precipitation Anomalies, Environment and Climate Change Canada, Government of Canada, available at: https://open.canada.ca/data/dataset/3d4b68a5-13bc-48bb-ad10-801128aa6604, last access: November 2017.
Ecological Stratification Working Group: A National Ecological Framework for Canada, Agriculture and Agri-Food Canada, Report and national map at 1:7 500 000 scale, Research Branch, Centre for Land and Biological Resources Research and Environment Canada, State of the Environment Directorate, Ecozone Analysis Branch, Ottawa/Hull., 1995
Evenson, G. R., Golden, H. E., Lane, C. R., McLaughlin, D. L., and D'Amico, E.: Depressional wetlands affect watershed hydrological, biogeochemical, and ecological functions, Ecol. Appl., 28, 953–966, https://doi.org/10.1002/eap.1701, 2018.
Fang, X., Pomeroy, J. W., Westbrook, C. J., Guo, X., Minke, A. G., and Brown, T.: Prediction of snowmelt derived streamflow in a wetland dominated prairie basin, Hydrol. Earth Syst. Sci., 14, 991–1006, https://doi.org/10.5194/hess-14-991-2010, 2010.
Geological Survey of Canada: Surficial geology of Canada/Géologie des formations superficielles du Canada, Canadian Geoscience Map, Geological Survey of Canada, Government of Canada, Natural Resources Canada, 195 pp., https://doi.org/10.4095/295462, 2014.
Gibson, J. J., Birks, S. J., Jeffries, D. S., Kumar, S., Scott, K. A., Aherne, J., and Shaw, D. P.: Site-specific estimates of water yield applied in regional acid sensitivity surveys across western Canada, J. Limnol., 69, 67–76, https://doi.org/10.3274/JL10-69-S1-08, 2010.
Gibson, J. J., Yi, Y., and Birks, S. J.: Isotope-based partitioning of streamflow in the oil sands region, northern Alberta: Towards a monitoring strategy for assessing flow sources and water quality controls, J. Hydrol. Reg. Stud., 5, 131–148, https://doi.org/10.1016/j.ejrh.2015.12.062, 2016.
Gober, P. and Wheater, H. S.: Socio-hydrology and the science-policy interface: a case study of the Saskatchewan River basin, Hydrol. Earth Syst. Sci., 18, 1413–1422, https://doi.org/10.5194/hess-18-1413-2014, 2014.
Godwin, R. B. and Martin, F. R. J.: Calculation of gross and effective drainage areas for the Prairie Provinces, in: Canadian Hydrology Symposium – 1975 Proceedings, 11–14 August 1975, Winnipeg, Manitoba, Associate Committee on Hydrology, National Research Council of Canada, 219–223, 1975.
Golden, H. E., Creed, I. F., Ali, G., Basu, N. B., Neff, B. P., Rains, M. C., McLaughlin, D. L., Alexander, L. C., Ameli, A. A., Christensen, J. R., Evenson, G. R., Jones, C. N., Lane, C. R., and Lang, M.: Integrating geographically isolated wetlands into land management decisions, Front. Ecol. Environ., 15, 319–327, https://doi.org/10.1002/fee.1504, 2017.
Goldhaber, M. B., Mills, C. T., Morrison, J. M., Stricker, C. A., Mushet, D. M., and LaBaugh, J. W.: Hydrogeochemistry of prairie pothole region wetlands: Role of long-term critical zone processes, Chem. Geol., 387, 170–183, https://doi.org/10.1016/j.chemgeo.2014.08.023, 2014.
Gooding, R. M. and Baulch, H. M.: Small reservoirs as a beneficial management practice for nitrogen removal, J. Environ. Qual., 46, 96–104, https://doi.org/10.2134/jeq2016.07.0252, 2017.
Hansen, A. T., Dolph, C. L., Foufoula-Georgiou, E., and Finlay, J. C.: Contribution of wetlands to nitrate removal at the watershed scale, Nat. Geosci., 11, 127–132, https://doi.org/10.1038/s41561-017-0056-6, 2018.
Harder, P., Helgason, W. D., and Pomeroy, J. W.: Modeling the Snowpack Energy Balance during Melt under Exposed Crop Stubble, J. Hydrometeorol., 19, 1191–1214, https://doi.org/10.1175/JHM-D-18-0039.1, 2018.
Hayashi, M., Van Der Kamp, G., and Schmidt, R.: Focused infiltration of snowmelt water in partially frozen soil under small depressions, J. Hydrol., 270, 214–229, https://doi.org/10.1016/S0022-1694(02)00287-1, 2003.
Hayashi, M., Van der Kamp, G., and Rosenberry, D. O.: Hydrology of Prairie Wetlands: Understanding the Integrated Surface-Water and Groundwater Processes, Wetlands, 36, 1–18, https://doi.org/10.1007/s13157-016-0797-9, 2016.
Hijmans, R. J.: raster: Geographic data analysis and modeling, R package version 2.6-7, available at: https://CRAN.R-project.org/package=raster (last access: 27 April 2019), 2017.
Hosking, J. R. and Wallis, J. R.: Parameter and quantile estimation for the generalized Pareto distribution, Technometrics, 29, 339–349, 1987.
Husson, F., Josse, J., Lê, S., and Mazet, J.: FactoMineR: Multivariate Exploratory Data Analysis and Data Mining with R, R package version 1.12, available at: http://factominer.free (last access: 24 April 2019), 2009.
Jones, N. E., Schmidt, B. J., Melles, S. J., and Brickman, D.: Characteristics and distribution of natural flow regimes in Canada: a habitat template approach, Can. J. Fish. Aquat. Sci., 71, 1616–1624, https://doi.org/10.1139/cjfas-2014-0040, 2014.
Kanishka, G. and Eldho, T. I.: Watershed classification using isomap technique and hydrometeorological attributes, J. Hydrol. Eng., 22, 04017040, https://doi.org/10.1061/(ASCE)HE.1943-5584.0001562, 2017.
Kerr, J. G.: Multiple land use activities drive riverine salinization in a large, semi-arid river basin in western Canada, Limnol. Oceanogr., 62, 1331–1345, https://doi.org/10.1002/lno.10498, 2017.
Knoben, W. J. M., Woods, R. A., and Freer, J. E.: A quantitative hydrological climate classification evaluated with independent streamflow data, Water Resour. Res., 54, 5088–5109, https://doi.org/10.1029/2018WR022913, 2018.
Laudon, H., Spence, C., Buttle, J., Carey, S. K., McDonnell, J. J., McNamara, J. P., Soulsby, C., and Tetzlaff, D.: Save northern high-latitude catchments, Nat. Geosci., 10, 324–325, https://doi.org/10.1038/ngeo2947, 2017.
Lê, S., Josse, J., and Husson, F.: FactoMineR: An R Package for Multivariate Analysis, J. Stat. Softw., 25, 1–18, 2008.
Lehner, B. and Grill, G.: Global river hydrography and network routing: baseline data and new approaches to study the world's large river systems, Hydrol. Process., 27, 2171–2186, 2013.
Leibowitz, S. G., Mushet, D. M., and Newton, W. E.: Intermittent Surface Water Connectivity: Fill and Spill Vs. Fill and Merge Dynamics, Wetlands, 36, 323–342, https://doi.org/10.1007/s13157-016-0830-z, 2016.
Leibowitz, S. G., Wigington, P. J., Schofield, K. A., Alexander, L. C., Vanderhoof, M. K., and Golden, H. E.: Connectivity of Streams and Wetlands to Downstream Waters: An Integrated Systems Framework, J. Am. Water Resour. Assoc., 54, 298–322, https://doi.org/10.1111/1752-1688.12631, 2018.
Liu, G. and Schwartz, F. W.: Climate-driven variability in lake and wetland distribution across the Prairie Pothole Region: From modern observations to long-term reconstructions with space-for-time substitution, Water Resour. Res., 48, 1–11, https://doi.org/10.1029/2011WR011539, 2012.
Loveland, T. R. and Merchant, J. M.: Ecoregions and Ecoregionalization: Geographical and Ecological Perspectives, Environ. Manage., 34, S1–S13, https://doi.org/10.1007/s00267-003-5181-x, 2004.
MacCulloch, G. and Whitfield, P. H. H.: Towards a Stream Classification System for the Canadian Prairie Provinces, Can. Water Resour. J., 37, 311–332, https://doi.org/10.4296/cwrj2011-905, 2012.
Main, A. R., Headley, J. V., Peru, K. M., Michel, N. L., Cessna, A. J., and Morrissey, C. A.: Widespread use and frequent detection of neonicotinoid insecticides in wetlands of Canada's prairie pothole region, PLoS One, 9, e92821, https://doi.org/10.1371/journal.pone.0092821, 2014.
Marton, J. M., Creed, I. F., Lewis, D. B., Lane, C. R., Basu, N. B., Cohen, M. J., and Craft, C. B.: Geographically isolated wetlands are important biogeochemical reactors on the landscape, Bioscience, 65, 408–418, https://doi.org/10.1093/biosci/biv009, 2015.
Matile, G. L. D. and Keller, G. R.: Surficial geology of the Norway House map sheet (NTS 63H), Manitoba, Surficial Geology Compilation Map Series SG-63H, scale 1:250 000, Manitoba Science, Technology, Energy and Mines, Manitoba Geological Survey, Manitoba, available at: https://www.gov.mb.ca/iem/info/libmin/SG-63H.zip (last access: 6 December 2017), 2006.
McDonnell, J. J. and Woods, R.: On the need for catchment classification, J. Hydrol., 299, 2–3, https://doi.org/10.1016/j.jhydrol.2004.09.003, 2004.
Mekis, É. and Vincent, L. A.: An overview of the second generation adjusted daily precipitation dataset for trend analysis in Canada, Atmos.-Ocean, 49, 163–177, https://doi.org/10.1080/07055900.2011.583910, 2011.
Mowchenko, M. and Meid, P. O.: The Determination of Gross and Effective Drainage Areas in the Prairie Provinces, edited by: Regina, S. K., Agriculture – Prairie Farm Rehabilitation – Engineering Branch, Agriculture Canada, Prairie Farm Rehabilitation Administration, Engineering Branch, Regina, SK, Canada, 22 pp., 1983.
Mwale, D., Gan, T. Y., Devito, K. J., Silins, U., Mendoza, C., and Petrone, R.: Regionalization of Runoff Variability of Alberta, Canada, by Wavelet, Independent Component, Empirical Orthogonal Function, and Geographical Information System Analyses, J. Hydrol. Eng., 16, 93–107, https://doi.org/10.1061/(ASCE)HE.1943-5584.0000284, 2011.
NRC: Hydro features (1:50 000), National Hydro Network, CanVec series, Earth Sciences Sector, Natural Resources Canada, Government of Canada, available at: http://open.canada.ca/en (last access: 22 November 2017), 2016.
Oksanen, J., Blanchet, F. G., Friendly, M., Kindt, R., Legendre, P., McGlinn, D., Minchin, P. R., O'Hara, R. B., Simpson, G. L., Solymos, P., Stevens, M. H. H., Szoecs, E., and Wagner, H.: vegan: Community Ecology Package, R package version 2.5-2, available at: https://CRAN.R-project.org/package=vegan, last access: 23 September 2018.
Omernik, J. M. and Griffith, G. E.: Ecoregions of the Conterminous United States: Evolution of a Hierarchical Spatial Framework, Environ. Manage., 54, 1249–1266, https://doi.org/10.1007/s00267-014-0364-1, 2014.
Ouarda, T. B., Hache, M., Bruneau, P., and Bobee, B.: Regional Flood Peak and Volume Estimation in Northern Canadian Basin, J. Cold Reg. Eng., 14, 176–191, 2002.
Pekel, J.-F., Cottam, A., Gorelick, N., and Belward, A. S.: High-resolution mapping of global surface water and its long-term changes, Nature, 540, 418–422, https://doi.org/10.1038/nature20584, 2016.
Peters, D. L., Boon, S., Huxter, E. H., Spence, C., van Meerveld, H. J., and Whitfield, P. H.: Zero Flow: A PUB (Prediction in Ungauged Basins) Workshop on Temporary Streams: Summary of workshop discussions and future directions, Can. Water Resour. J., 37, 425–431, 2012.
Rao, A. R. and Srinivas, V. V.: Regionalization of watersheds by fuzzy cluster analysis, J. Hydrol., 318, 57–79, https://doi.org/10.1016/j.jhydrol.2005.06.004, 2006.
Razavi, T. and Coulibaly, P.: Classification of Ontario watersheds based on physical attributes and streamflow series, J. Hydrol., 493, 81–94, https://doi.org/10.1016/j.jhydrol.2013.04.013, 2013.
Razavi, T. and Coulibaly, P.: An evaluation of regionalization and watershed classification schemes for continuous daily streamflow prediction in ungauged watersheds, Can. Water Resour. J., 42, 2–20, https://doi.org/10.1080/07011784.2016.1184590, 2017.
R Core Team: R: A language and environment for statistical computing, R Foundation for Statistical Computing, Vienna, Austria, available at: https://www.R-project.org/ (last access: 27 April 2019), 2018.
Ribatet, M.: SpatialExtremes: Modelling Spatial Extremes, R package version 2.0-7, available at: https://CRAN.R-project.org/package=SpatialExtremes (last access: 24 April 2019), 2018.
Sawicz, K., Wagener, T., Sivapalan, M., Troch, P. A., and Carrillo, G.: Catchment classification: Empirical analysis of hydrologic similarity based on catchment function in the eastern USA, Hydrol. Earth Syst. Sci., 15, 2895–2911, https://doi.org/10.5194/hess-15-2895-2011, 2011.
Shaw, D. A., van der Kamp, G., Conly, F. M., Pietroniro, A., and Martz, L.: The Fill-Spill Hydrology of Prairie Wetland Complexes during Drought and Deluge, Hydrol. Process., 26, 3147–3156, https://doi.org/10.1002/hyp.8390, 2012.
Shook, K. R. and Pomeroy, J. W.: Memory effects of depressional storage in Northern Prairie hydrology, Hydrol. Process., 25, 3890–3898, https://doi.org/10.1002/hyp.8381, 2011.
Shook, K. and Pomeroy, J.: Changes in the hydrological character of rainfall on the Canadian prairies, Hydrol. Process., 26, 1752–1766, https://doi.org/10.1002/hyp.9383, 2012.
Shook, K., Pomeroy, J. W., Spence, C., and Boychuk, L.: Storage dynamics simulations in prairie wetland hydrology models: Evaluation and parameterization, Hydrol. Process., 27, 1875–1889, https://doi.org/10.1002/hyp.9867, 2013.
Shook, K., Pomeroy, J., and van der Kamp, G.: The transformation of frequency distributions of winter precipitation to spring streamflow probabilities in cold regions; case studies from the Canadian Prairies, J. Hydrol., 521, 394–409, https://doi.org/10.1016/j.jhydrol.2014.12.014, 2015.
Simpson, M. A.: Surficial Geology Map of Saskatchewan (250k_surficial, vector digital data), Original maps published 1984 to 1988; merged digital version made available 2008, compiled by: Simpson, M. A., Environment Branch, Saskatchewan Research Council and Saskatchewan Ministry of the Economy, available at: https://gisappl.saskatchewan.ca/Html5Ext/index.html?viewer=GeoAtlas (last access: 6 December 2017), 2008.
Sivakumar, B., Singh, V. P., Berndtsson, R., and Khan, S. K.: Catchment Classification Framework in Hydrology: Challenges and Directions, J. Hydrol. Eng., 20, A4014002, https://doi.org/10.1061/(ASCE)HE.1943-5584.0000837, 2013.
Spence, C. and Saso, P.: A hydrological neighbourhood approach to predicting streamflow in the Mackenzie valley, Prediction in Ungauged Basins: Approaches for Canada's Cold Regions, 21–44, 2005.
Spence, C. and Woo, M. K.: Hydrology of subarctic Canadian shield: Soil-filled valleys, J. Hydrol., 279, 151–166, https://doi.org/10.1016/S0022-1694(03)00175-6, 2003.
Spence, C., Wolfe, J. D., Whitfield, C. J., Baulch, H. M., Basu, N. B., Bedard-Haughn, A. K., Belcher, K. W., Clark, R. G., Ferguson, G. A., Hayashi, M., Liber, K., McDonnell, J. J., Morrissey, C. A., Pomeroy, J. W., Reed, M. G., and Strickert, G.: Prairie water: a global water futures project to enhance the resilience of prairie communities through sustainable water management, Can. Water Resour. J., 44, 115–126, https://doi.org/10.1080/07011784.2018.1527256, 2018.
Statistics Canada: Table 32-10-0162-01, Selected land management practices and tillage practices used to prepare land for seeding, historical data, Census of Agriculture, Statistics Canada, Government of Canada, available at: https://www150.statcan.gc.ca/t1/tbl1/en/cv.action?pid=3210016201 (last access: 23 March 2018), 2016.
Thornthwaite, C.: An Approach toward a Rational Classification of Climate, Geogr. Rev., 38, 55–94, 1948.
Vanderhoof, M. K., Christensen, J. R., and Alexander, L. C.: Patterns and drivers for wetland connections in the Prairie Pothole Region, United States, Wetl. Ecol. Manage., 25, 275–297, https://doi.org/10.1007/s11273-016-9516-9, 2017.
Van der Kamp, G. and Hayashi, M.: Groundwater-wetland ecosystem interaction in the semiarid glaciated plains of North America, Hydrogeol. J., 17, 203–214, https://doi.org/10.1007/s10040-008-0367-1, 2009.
Van der Kamp, G., Hayashi, M., and Gallén, D.: Comparing the hydrology of grassed and cultivated catchments in the semi-arid Canadian prairies, Hydrol. Process., 17, 559–575, https://doi.org/10.1002/hyp.1157, 2003.
Van der Kamp, G., Hayashi, M., Bedard-Haughn, A., and Pennock, D.: Prairie Pothole Wetlands – Suggestions for Practical and Objective Definitions and Terminology, Wetlands, 36, 229–235, https://doi.org/10.1007/s13157-016-0809-9, 2016.
Van Meter, K. J. and Basu, N. B.: Signatures of human impact: size distributions and spatial organization of wetlands in the Prairie Pothole landscape, Ecol. Appl., 25, 451–465, https://doi.org/10.1890/14-0662.1, 2015.
Vincent, L. A., Wang, X. L., Milewska, E. J., Wan, H., Yang, F., and Swail, V.: A second generation of homogenized Canadian monthly surface air temperature for climate trend analysis, J. Geophys. Res.-Atmos., 117, 1–13, https://doi.org/10.1029/2012JD017859, 2012.
Vincent, L. A., Zhang, X., Brown, R. D., Feng, Y., Mekis, E., Milewska, E. J., Wan, H., and Wang, X. L.: Observed trends in Canada's climate and influence of low-frequency variability modes, J. Climate, 28, 4545–4560, https://doi.org/10.1175/JCLI-D-14-00697.1, 2015.
Vicente-Serrano, S. M., Beguería, S., and López-Moreno, J. I.: A Multi-scalar drought index sensitive to global warming: The Standardized Precipitation Evapotranspiration Index – SPEI, J. Climate, 23, 1696–1718, https://doi.org/10.1175/2009JCLI2909.1, 2010.
Wagener, T., Sivapalan, M., Troch, P., and Woods, R.: Catchment classification and hydrologic similarity, Geogr. Compass, 1, 901–931, https://doi.org/10.1111/j.1749-8198.2007.00039.x, 2007.
Wagner, T., Bremigan, M. T., Cheruvelil, K. S., Soranno, P. A., Nate, N. A., and Breck, J. E.: A multilevel modeling approach to assessing regional and local landscape features for lake classification and assessment of fish growth rates, Environ. Monit. Assess., 130, 437–454, https://doi.org/10.1007/s10661-006-9434-z, 2007.
Weber, D., Sadeghian, A., Luo, B., Waiser, M. J., and Lindenschmidt, K. E.: Modelling Scenarios to Estimate the Potential Impact of Hydrological Standards on Nutrient Retention in the Tobacco Creek Watershed, Manitoba, Canada, Water Resour. Manage., 31, 1305–1321, https://doi.org/10.1007/s11269-017-1578-9, 2017.