the Creative Commons Attribution 4.0 License.
the Creative Commons Attribution 4.0 License.
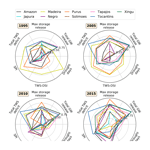
Multi-decadal hydrologic change and variability in the Amazon River basin: understanding terrestrial water storage variations and drought characteristics
Suyog Chaudhari
Emilio Moran
Gonzalo Miguez-Macho
We investigate the interannual and interdecadal hydrological changes in the Amazon River basin and its sub-basins during the 1980–2015 period using GRACE satellite data and a physically based, 2 km grid continental-scale hydrological model (LEAF-Hydro-Flood) that includes a prognostic groundwater scheme and accounts for the effects of land use–land cover (LULC) change. The analyses focus on the dominant mechanisms that modulate terrestrial water storage (TWS) variations and droughts. We find that (1) the model simulates the basin-averaged TWS variations remarkably well; however, disagreements are observed in spatial patterns of temporal trends, especially for the post-2008 period. (2) The 2010s is the driest period since 1980, characterized by a major shift in the decadal mean compared to the 2000s caused by increased drought frequency. (3) Long-term trends in TWS suggest that the Amazon overall is getting wetter (1.13 mm yr−1), but its southern and southeastern sub-basins are undergoing significant negative TWS changes, caused primarily by intensified LULC changes. (4) Increasing divergence between dry-season total water deficit and TWS release suggests a strengthening dry season, especially in the southern and southeastern sub-basins. (5) The sub-surface storage regulates the propagation of meteorological droughts into hydrological droughts by strongly modulating TWS release with respect to its storage preceding the drought condition. Our simulations provide crucial insight into the importance of sub-surface storage in alleviating surface water deficit across Amazon and open pathways for improving prediction and mitigation of extreme droughts under changing climate and increasing hydrologic alterations due to human activities (e.g., LULC change).
- Article
(16335 KB) - Full-text XML
-
Supplement
(23844 KB) - BibTeX
- EndNote
The Amazon River basin is one of the most hydrologically and ecologically diverse regions in the world (Fan and Miguez-Macho, 2010; Latrubesse et al., 2017; Lenton et al., 2009; Lesack, 1993; Malhi et al., 2008; Moran et al., 2018; Timpe and Kaplan, 2017; Tófoli et al., 2017). It is home to the world's largest tropical rainforest and hosts ∼25 % of all terrestrial species on Earth (Malhi et al., 2008). Hydrologically, it contributes to 20 %–30 % of the world's total river discharge into the oceans (Clark et al., 2015; Muller-Karger et al., 1988; Nepstad et al., 2008) and accounts for ∼15 % of global terrestrial evapotranspiration (Field et al., 1998; Malhi et al., 2008). Thus, the Amazon is an important component of global terrestrial ecosystems and the hydrologic cycle (Cox et al., 2004; Nobre et al., 1991); it also plays a major role in global atmospheric circulation through precipitation recycling and atmospheric moisture transport (Malhi et al., 2008; Soares-Filho et al., 2010).
The hydro-ecological systems of the Amazon are dependent on plentiful rainfall (Cook et al., 2012; Espinoza et al., 2015, 2016; Espinoza Villar et al., 2009; Nepstad et al., 2008) and the vast amount of water that flows down through extensive river networks and massive floodplains (Bonnet et al., 2008; Coe et al., 2002; Frappart et al., 2011; Miguez-Macho and Fan, 2012a; Yamazaki et al., 2011; Zulkafli et al., 2016). The spatiotemporal patterns of precipitation are, however, changing due to climate change and variability (Brando et al., 2014; Cook et al., 2012; Lima et al., 2014; Malhi et al., 2008, 2009; Nepstad et al., 2008), large-scale alterations in land use (e.g., deforestation) (Chen et al., 2015; Coe et al., 2009; Davidson et al., 2012; Kalamandeen et al., 2018; Lima et al., 2014; Panday et al., 2015; Tollefson, 2016), and more recently the construction of mega-dams (Finer and Jenkins, 2012; Latrubesse et al., 2017; Moran et al., 2018; Soito and Freitas, 2011; Timpe and Kaplan, 2017; Winemiller et al., 2016), among others. Such changes in precipitation patterns typically manifest themselves in terms of altered magnitude, duration, and timing of streamflow (Marengo, 2005). A prominent streamflow alteration pattern that has been widely observed across the Amazon is the extended dry-season length (Espinoza et al., 2016; Marengo et al., 2011) and an increase in the number of dry events (i.e., droughts) over the longer term (Malhi et al., 2009; Marengo and Espinoza, 2016), which has been suggested to be a result of ongoing climatic and human-induced changes (Cook et al., 2012; Cook and Vizy, 2008; Lee et al., 2011; Malhi et al., 2008; Shukla et al., 1990). However, the cross-scale interactions and feedbacks in the human–water relationship make it difficult to explicitly quantify the causes. These changes have resulted in decreases in runoff (Espinoza et al., 2009; Haddeland et al., 2014; Lima et al., 2014) and loss of terrestrial biodiversity (Barletta et al., 2010; Newbold et al., 2016; Tófoli et al., 2017; Toomey et al., 2011; Winemiller et al., 2016). Increased variability in streamflow has also resulted in the disruption of the food pulse and fishery yields, which the Amazon region thrives upon (Castello et al., 2013, 2015; Forsberg et al., 2017). Moreover, persistent dry events create negative social externalities, such as deterioration of respiratory health due to drought-induced fires (Smith et al., 2014), exhaustion of family savings (Brondizio and Moran, 2008), and isolation of communities that are affected by river navigation and drinking water scarcity (Sena et al., 2012), hence affecting the overall livelihood of the local communities. Thus, it is critical to understand the characteristics of historical droughts to better understand the dominant mechanisms that modulate droughts and their evolution over time.
As often is the case, droughts in the Amazon are driven by El Niño events; however, some droughts are suggested to be caused by climate change and variability (Espinoza et al., 2011; Lewis et al., 2011; Marengo et al., 2008; Marengo and Espinoza, 2016; Phillips et al., 2009; Xu et al., 2011; Zeng et al., 2008) and due to accelerating human activities causing rapid changes in the land use and water cycle (Lima et al., 2014; Malhi et al., 2008). Numerous studies have quantified the impacts and spatial extent of these periodic droughts on the hydrological and ecological systems in the Amazon (Alho et al., 2015; Brando et al., 2014; Castello et al., 2013, 2015, Chen et al., 2009, 2010; da Costa et al., 2010; Davidson et al., 2012; Fernandes et al., 2011; Lewis et al., 2011; Phillips et al., 2009; Saleska et al., 2007, 2016; Satyamurty et al., 2013; Schöngart and Junk, 2007; Xu et al., 2011; Zeng et al., 2008). For example, Lewis et al. (2011) found that the 2010 drought was spatially more extensive than the 2005 drought; the spatial extent was over 3.0 million square kilometers in 2010 and 1.9 million square kilometers in 2005. These catastrophic droughts had major implications on the hydrology of the Amazon River basin; for example, the 2005 hydrological drought led to reduction in streamflow by 32 % from the long-term mean, as reported in Zeng et al. (2008), and in 2010 moisture stress induced persistent declines in vegetation greenness affecting an area of ∼2.4 million square kilometers, which was 4 times greater than the area impacted in 2005 (Xu et al., 2011). Moreover, these extreme drought events, coupled with forest fragmentation, have caused widespread fire-induced tree mortality and forest degradation across Amazonian forests (Aragão et al., 2007; Brando et al., 2014; Davidson et al., 2012; Malhi et al., 2008; Rammig et al., 2010).
Due to the limited availability of observed data (e.g., precipitation, streamflow) for the entire basin, hydrologic characteristics of droughts in the Amazon have been studied primarily by using hydrological models and satellite remote sensing. For example, early studies (Coe et al., 2002; Costa and Foley, 1999; Lesack, 1993; Vorosmarty et al., 1996; Zeng, 1999) examined different components of the Amazon water budget and their trends through relatively simpler models. More recent literature (Dias et al., 2015; Fan et al., 2019; Getirana et al., 2012; Miguez-Macho and Fan, 2012a, b; Paiva et al., 2013a, b; Pokhrel et al., 2012a, b, 2013; Shin et al., 2018; Siqueira et al., 2018; Wang et al., 2019; Yamazaki et al., 2011, 2012) provided further advances in modeling the hydrological dynamics connected with anthropogenic activities in the Amazon and other parts of the world. Methods with varying complexities were used in similar studies, ranging from simple water budget analyses (Betts et al., 2005; Costa and Foley, 1999; Fernandes et al., 2008; Lesack, 1993; Sahoo et al., 2011; Vorosmarty et al., 1996; Zeng, 1999) to state-of-the-art land surface models (Getirana et al., 2012; Miguez-Macho and Fan, 2012a, b; Paiva et al., 2013a, b; Pokhrel et al., 2013; Siqueira et al., 2018; Wongchuig Correa et al., 2017; Yamazaki et al., 2011, 2012), with some targeting the overall development of parameterization and process representation in the model (Coe et al., 2008, 2009; Dias et al., 2015; Getirana et al., 2010, 2012, Miguez-Macho and Fan, 2012a, b; Paiva et al., 2013b; Pokhrel et al., 2013; Yamazaki et al., 2011) and others focusing on the hydrological changes occurring in the basin due to weather variability (Coe et al., 2002; Lima et al., 2014; Wongchuig Correa et al., 2017).
Major drought events in the Amazon, particularly those in recent years, have been detected by satellite remote sensing, and their impacts on terrestrial hydrology have been examined (Chen et al., 2010; Filizola et al., 2014; Xu et al., 2011). In particular, the hydrologic impact of droughts has been revealed by examining the anomalies in terrestrial water storage (TWS) inferred from the Gravity Recovery and Climate Experiment (GRACE) satellites. A significant decrease in TWS over Central Amazon in the summer of 2005, relative to the average of the five other summer months during the 2003–2007 period, was reported by Chen et al. (2009). However, due to the vast latitudinal extent of the Amazon basin, these severe dry conditions were observed only in some regions of the basin. Xavier et al. (2010) and Frappart et al. (2013) used GRACE TWS estimates to identify the signature of these drought events and suggested that the 2005 drought only affected the western and central parts of the basin, whereas very wet conditions peaking in mid-2006 were observed in the eastern, northern, and southern regions of the basin. Although the ramifications of these extreme droughts have been widely studied using remote sensing datasets (e.g., GRACE), the understanding of their time evolution is limited due to data gaps and short study periods, hence hindering their comprehensive categorization. Further, GRACE provides the changes in vertically integrated TWS variations; thus variations in the individual TWS components cannot be estimated solely by GRACE. This shortcoming is overcome by using hydrological models that separate TWS into its individual components and provide simulations for an extended timescale. However, discrepancy between models and GRACE observations has also become a major topic of discussion, as most of the global models show an opposite trend in TWS compared to GRACE in the Amazon and other global river basins (Scanlon et al., 2018); yet, no clear explanation or quantification exists in the published literature, apart from the attribution of the discrepancy to model shortcomings (see Sect. 3.3 for details).
As referenced above, the changing hydroclimatology of the Amazon basin, along with specific drought-related analysis (e.g., 2005, 2010), has been widely reported in a large body of literature published over recent decades. Several studies have used statistical measures to quantify drought severity (Espinoza et al., 2016; Gloor et al., 2013; Joetzjer et al., 2013; Marengo, 2006; Marengo et al., 2008, 2011; Wongchuig Correa et al., 2017; Zeng et al., 2008; Zhao et al., 2017a), concerning common variables, such as streamflow and precipitation, thus limiting the quantification of drought impact on water stores, viz. flood, groundwater, and TWS. Further, even though these studies encompass different aspects of hydrological and climatic changes, most span only a few years to a decade, except for some precipitation-related studies (Marengo, 2004; Marengo et al., 1998). Other studies have used a relatively longer study period (Costa et al., 2003; Espinoza et al., 2016; Zeng, 1999), but the spatial extent is limited. Thus, a comprehensive understanding of the interdecadal hydrologic change and variability across the entire basin and that of changes in drought characteristics is still lacking. Given the number of droughts that have occurred and their widespread impact in the Amazon, it is imperative to have a better understanding of these past events so as to anticipate future hydrological conditions (Phipps et al., 2013). Many aspects of the droughts are yet to be studied, such as, the interdependence between TWS and meteorological (precipitation-related) and hydrological (streamflow-related) droughts. A complete categorization of the drought events with respect to their causes and impacts and the resulting basin response is still coming up short.
In this study, we investigate the interannual and interdecadal variability in TWS and drought events in the Amazon River basin over 1980–2015 period. Our study is driven by the following key science questions: (1) how do interannual and interdecadal changes in drought conditions manifest as long-term variations in TWS at varying spatial and temporal scales in the Amazon River basin? (2) What are the impacts of TWS variations on dry-season water deficit and release? Is the Amazonian dry season getting stronger or more severe? (3) What are the dominant factors driving the evolution of TWS and drought conditions at varying spatial and temporal scales? (4) How does the sub-surface water storage regulate the water deficiency caused by the surface drought conditions? These questions are answered by using hydrological simulations from a continental-scale hydrological model and the TWS data from GRACE satellites; the goal is to provide a comprehensive picture of characteristics and evolution of droughts in the Amazon with respect to their types and spatial impact. Specifically, this study aims to (i) examine the impacts of drought conditions on TWS and other hydrological variables, (ii) understand the hydrological variability and drought evolution in the Amazon at an annual and decadal scale over the past four decades, (iii) quantify the role of sub-surface water storage in alleviating the surface drought conditions, and (iv) summarize each drought year by providing a comprehensive characterization for the major drought events in the Amazon and its sub-basins.
2.1 The LEAF-Hydro-Flood (LHF) model
The model used in this study is LHF (Fan et al., 2013; Miguez-Macho and Fan, 2012a, b; Pokhrel et al., 2013, 2014), a continental-scale land hydrology model that resolves various land surface hydrologic and groundwater processes on a full physical basis. It is derived from the model Land Ecosystem–Atmosphere Feedback (LEAF) (Walko et al., 2000), the land surface component of the Regional Atmosphere Modeling System (RAMS) (Pielke et al., 1992). The original LEAF was extensively improved and enhanced to develop LEAF-Hydro for North America (Fan et al., 2007; Miguez-Macho et al., 2007) by adding a prognostic groundwater storage and allowing (1) the water table to rise and fall or the vadose zone to shrink or grow; (2) the water table, recharged by soil drainage, to relax through streamflow into rivers, and lateral groundwater flow, leading to convergence to low valleys; (3) two-way exchange between groundwater and rivers, representing both losing and gaining streams; (4) river routing to the ocean as kinematic waves; and (5) setting sea level as the groundwater head boundary condition. Miguez-Macho and Fan (2012a) further enhanced the LEAF-Hydro framework by incorporating the river–floodplain routing scheme which solves the full momentum equation of open-channel flow, giving more realistic streamflow estimates by considering the prominent backwater effect observed in the Amazon (Bates et al., 2010; Yamazaki et al., 2011). The LHF model has been extensively validated in the North and South American continents on 5 and 2 km grids, respectively (Fan et al., 2013; Miguez-Macho et al., 2008; Miguez-Macho and Fan, 2012a, b; Pokhrel et al., 2013; Shin et al., 2018) and used to examine the impacts of climate change on the groundwater system in the Amazon (Pokhrel et al., 2014). A complete description of LHF can be found in Miguez-Macho and Fan (2012a).
2.2 Atmospheric forcing
Atmospheric forcing data are taken from WATCH Forcing Data methodology applied to ERA-Interim reanalysis data (WFDEI) (Weedon et al., 2014), available for the 1979–2016 period at 0.5∘ spatial resolution and 3 h time steps. The WFDEI dataset is widely used in both global- and regional-scale studies (Beck et al., 2016; Felfelani et al., 2017; Hanasaki et al., 2018; Müller Schmied et al., 2014) and has been suggested to represent the observations in the Amazon region well (Monteiro et al., 2016). The original WFDEI data at 0.5∘ resolution are spatially interpolated using a bilinear interpolation method to model grid resolution (∼2 km), following our previous studies (Miguez-Macho and Fan, 2012a, b; Pokhrel et al., 2013, 2014; Shin et al., 2018). The more recent European Centre for Medium-Range Weather Forecasts Reanalysis 5th (ERA5) dataset, which provides atmospheric forcing data from 1979 to present day at a spatial resolution of 0.25∘, shows promise by outperforming its predecessors (Towner et al., 2019). However, as no studies existed in the past literature which comprehensively validated the ERA5 dataset over the Amazon region until recently, WFDEI forcing remains a better alternative as a model input.
2.3 Land use–land cover and leaf area index
The land cover data used in this study are obtained from the European Space Agency Climate Change Initiative's Land Cover project (ESA-CCI; http://maps.elie.ucl.ac.be/CCI/, last access: 24 June 2019). The data comprise an annual time series of high-resolution land cover maps for the 1992–2015 period at a 300 m spatial resolution, generated by combining the baseline map from the Medium-spectral Resolution Imaging Spectrometer (MERIS) instrument and the land use–land cover (LULC) changes detected from AVHRR (1992–1999), SPOT-Vegetation (1999–2012), and PROBA-V (2013–2015) instruments. The classification follows the LULC classes defined by the UN Land Cover Classification System (LCCS). Spatiotemporal coverage and resolution of these LULC maps are consistent with the specific LHF model requirements; hence we use annual land cover input, spatially aggregated to 2 km LHF model grids, following the general practice in hydrologic impact studies (Arantes et al., 2016; Panday et al., 2015).
Because the ESA-CCI data did not cover the simulation period prior to year 1992, we derive the time series products for the 1980–1991 period by using the trend in leaf area index (LAI) and the ESA-CCI land cover map for year 1992 as a baseline. A pixel-by-pixel analysis is conducted, and the pixels with mean annual LAI higher than 5 are transitioned into the forest canopy, whereas for other pixels the LULC type is retained from the previous year's LULC map. The threshold of LAI equal to 5 for facilitating the land cover transition into forest is determined based on the LAI classifications provided in past literature (Asner et al., 2003; Myneni et al., 2007; Xu et al., 2018). Reverse prediction of LULC changes was constrained to the forest canopy only, as it is difficult to predict the LULC type based on LAI values less than 5. Also, forest cover is known to be the most prominent land cover in the Amazon; hence it is reasonable to assume that most of the LULC changes occurring in the basin are transitioned from forest cover.
Monthly LAI data are derived by temporally aggregating the 8 d composites from the Global Land Surface Satellite (GLASS) LAI product (Liang and Xiao, 2012; Xiao et al., 2014) to monthly values for the entire model domain. GLASS LAI values for the period of 1982–1999 are derived from AVHRR reflectance, whereas MODIS reflectance values are used for the period 2000–2012. Because of the data constraint, LAI data for the years before 1982 and after 2012 are assumed to be the same as that of the years 1982 and 2012, respectively.
2.4 Validation data
2.4.1 Observed streamflow
We use monthly averaged streamflow data obtained from the Agência Nacional de Águas (ANA) in Brazil (http://hidroweb.ana.gov.br, last access: 24 June 2019). A total of 55 stream gauge stations are selected considering a wide coverage over the Amazonian sub-basins and a good balance between low and high flow values. The major selection criterion is the data length; i.e., we only include gauges with at least 30 years' coverage. In a few cases, such as for the Japura sub-basin, the threshold was overlooked because this criterion resulted in a small number of gauging stations. All the selected stations have observational data for varying time frames with minimal data gaps; the months with missing data are skipped in the statistical analysis.
2.4.2 GRACE data
The TWS products from the GRACE satellite mission are used to validate the TWS simulated by LHF for the 2002–2015 period. Equivalent water height from three processing centers, namely (i) the Jet Propulsion Laboratory (JPL), (ii) the Center for Space Research (CSR), and (iii) the German Research Center for Geoscience (GFZ) (http://grace.jpl.nasa.gov/data/ get-data/, last access: 24 June 2019) (Landerer and Swenson, 2012), is used along with two mascon products from CSR and JPL; mascon products have been suggested to better capture TWS signals in many regions (Scanlon et al., 2016). Basin-averaged data of variation in TWS anomalies are calculated from GRACE by taking an area-weighted arithmetic mean with varying cell area (Felfelani et al., 2017).
2.5 TWS drought severity index
To examine the occurrence and severity of hydrological droughts over the past decades, we employ the drought severity index derived from time-varying TWS change from GRACE, known as the GRACE drought severity index (GRACE-DSI) (Zhao et al., 2017b). We apply the GRACE-DSI framework to the 36-year simulated TWS (hereafter referred to as TWS-DSI) to examine the interannual and interdecadal drought evolution over the entire basin. This index is solely based on the TWS anomalies and has been shown to capture the past droughts with favorable agreement with other drought indices derived from precipitation (e.g., PDSI and SPEI) (Zhao et al., 2017a, b). TWS-DSI is calculated for each grid cell in the model domain as follows:
where TWSi,j is the TWS anomaly from LHF for year i and month j; and and σj are the temporal mean and standard deviation of TWS anomalies for month j, respectively.
2.6 Occurrence and duration of drought
The characteristics of hydrological droughts are identified from the simulated streamflow using the widely used threshold level approach. Different thresholds have been proposed in previous studies: mean flow, minimum and maximum flows (Marengo and Espinoza, 2016; Wongchuig Correa et al., 2017), 80th percentile (Q80) flow (Van Loon et al., 2012; Van Loon and Laaha, 2015; Wanders and Van Lanen, 2015), and 90th percentile (Q90) flow (Wanders et al., 2015; Wanders and Wada, 2015). In this study, we use Q90, which is derived from the flow duration curve, where Q90 is the streamflow that is equaled or exceeded for 90 % of the time. Q90 is used to isolate severe drought events over the simulation period. Monthly threshold values are derived using the 36-year simulated streamflow and are smoothed by a 30 d moving average. Drought condition is identified by determining whether the variable is below the threshold, expressed mathematically as
where Ds(t,x) indicates whether the grid (x) is in a drought state at time (t), Q(t,x) is the streamflow, and Q90(tx) is the threshold for grid (x) at time (t). Consecutive drought states are added to get the drought duration. Events with duration less than 3 d are not considered as droughts. The number of drought days per year is calculated by aggregating the duration of all the drought events in a year.
2.7 Dry-season total water deficit
We define the dry-season total water deficit (TWD) as the cumulative difference between monthly potential evapotranspiration (PET) and precipitation (P) for the period during which P<PET. The corresponding drop in the simulated TWS, during the same period as of TWD, is defined as the TWS release (TWS-R). TWD and TWS-R can be conceptualized as the annual water demand and supply as described in Guan et al. (2015). PET estimated at the daily interval using the Penman–Monteith approach (Monteith, 1965) as in Pokhrel et al. (2014) is aggregated to the monthly scale to calculate TWD; for consistency, we use the WFDEI forcing data that are used for LHF simulations (Sect. 2.2). TWS anomalies required for the estimation of storage release are obtained from the LHF model.
2.8 Simulation setup
LHF is set up for the entire Amazon basin (∼7.1 million square kilometers) including the Tocantins River basin. Simulations are conducted for the 1979–2015 period at a spatial resolution of 1 arcmin (∼2 km). The model time step is 4 min as in previous studies (Miguez-Macho and Fan, 2012a, b; Pokhrel et al., 2013, 2014); however, model output is saved at daily time steps. To stabilize water table depth, the model is spun up for ∼150 years, starting with the equilibrium water table (Fan et al., 2013) for 1979, and results for the 1980–2015 period (36 years) are analyzed. As this study aims to analyze the hydrological changes in the Amazon on a decadal scale, simulations for 1979 are considered as additional spin-up and hence not used. Dynamic monthly LAI and annual LULC maps are used to account for LULC changes (see Sect. 2.3). Moreover, as the model simulates land surface, hydrologic, and groundwater processes on a complete physical basis, no calibration was performed on the model output. The original novelty of the LHF model framework, combined with the incorporated dynamic human role through land cover change, creates a state-of-the-art framework for assessing long-term hydrological changes. The complete LHF framework along with the input data employed in this study are presented in Fig. S1 in the Supplement.
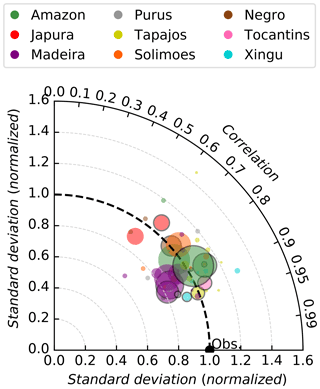
Figure 1Taylor diagram showing the correlation and standard deviation ratio between the simulated and observed streamflow at 55 gauge stations across the Amazon. The locations of the 55 gauge stations are shown in Fig. S2. Highlighted points with a black border are the gauge stations for which time series comparisons are shown in Figs. S3 and S4. The size of the markers indicates the annual mean simulated streamflow at that station, whereas the color indicates the Amazon sub-basin in which the station is located. The linear distance between each marker and the observed data (i.e., OBS; the black dot) is proportional to the root mean square error (RMSE).
3.1 Evaluation of simulated streamflow
Figure 1 presents the Taylor diagram (Taylor, 2001) illustrating the statistics of the simulated streamflow against observations at 55 gauging locations (see Sect. 2.4.1 and Fig. S2) across the entire Amazon basin. The Taylor diagram provides a synthetic view of error in the simulations in terms of the ratio of standard deviation (SD) of the simulated streamflow to the observed as a radial distance and their correlation as an angle in the polar axis. Most of the stations show a high correlation (>0.8) and a SD ratio close to unity, indicating a good model performance overall for varying geographical locations and stream sizes over the Amazon. Low correlation (∼0.6) is seen for some gauging stations situated on streams with smaller annual mean flow and steep slope profile, for example, the smaller streams across the Andes in Japura and Negro sub-basins, along with the streams in the northeastern parts of the Amazon. In these streams with high topographic gradients, precipitated water quickly flows away, causing slightly erratic patterns of seasonal streamflow, which is apparent in both simulated and observed time series (Figs. S3 and S4). However, due to the difficulty in resolving hillslope processes for low-order streams using 2 km grids, the model is unable to fully capture the flow seasonality in the streams with high topographic gradient.
The spatial distribution of the simulated streamflow across the entire model domain and the time series comparison of simulated vs. observed streamflow at 12 selected stations are presented in the Supplement (Figs. S2 and S3). The simulated seasonal cycle compares well with the observed one for the entire basin (i.e., Obidos station) as well as for most sub-basins; however, discrepancies in the seasonal peaks can be seen in some basins (e.g., Xingu, Tocantins, and Tapajos). Man-made reservoirs generally attenuate streamflow peaks and seasonal variability, reducing the SD, which is reflected in the observed data but not yet accounted for in the model; this could have exaggerated the SD ratio in some cases. For example, the streamflow in the Tocantins River shows higher SD compared to observed streamflow, likely due to the operation of the Tucurui I and II dams. Conversely, the SD ratio is lower than unity at some stations, including those on the Madeira River (Fig. 1), due to the dry bias found in the input precipitation (see Fig. S5 and Sect. 3.2). For sub-basins with higher groundwater contribution to streamflow, such as Xingu, Tapajos, Tocantins, and Madeira, the dry-season flow is overestimated (Fig. S3), which results from possibly exaggerated groundwater buffer in the model for these regions (Miguez-Macho and Fan, 2012a). Given that LHF is a continental-scale model, simulates streamflow on a full physical basis, and is not calibrated with observed streamflow, we consider these results to be satisfactory to study the hydrologic changes and variability.
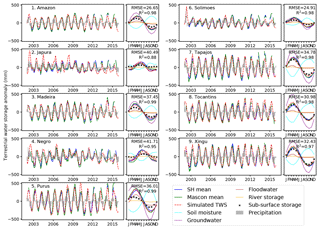
Figure 2Comparison of simulated TWS anomalies from LHF and TWS anomalies obtained from GRACE for the entire Amazon and its eight sub-basins for the 2002–2015 period. Basin-averaged precipitation anomalies obtained from the WFDEI forcing dataset are also shown as grey bars. Seasonal cycles of GRACE and simulated TWS are shown in the right panel of each basin along with the simulated individual TWS components. GRACE results are shown as the mean of the spherical harmonics (SH) solutions from three different processing centers (i.e., CSR, JPL, and GFZ) and mascon solutions from CSR and JPL. Simulated TWS anomalies are calculated with respect to the GRACE anomaly window of 2004–2009 for consistency.
3.2 Evaluation of simulated TWS anomalies with GRACE
Figure 2 presents the comparison of simulated TWS anomalies and GRACE data for the entire Amazon basin and its eight sub-basins; for model results, the individual TWS components are also provided. The model performs very well in simulating the basin-averaged TWS anomalies for the entire Amazon basin and most sub-basins. However, some differences between the simulated and GRACE-based TWS anomaly are evident, especially in sub-basins with a relatively smaller area and elongated shape (e.g., Purus and Japura). Note that the accuracy of GRACE–model agreement is generally low in such small basins due to high bias and leakage correction errors (Chaudhari et al., 2018; Felfelani et al., 2017; Longuevergne et al., 2010), reflected by higher root mean square error (RMSE) values in Fig. 2. Simulated TWS evidently follows precipitation anomalies (shown in grey bars in Fig. 2), implying that any uncertainties in the precipitation forcing could have directly impacted TWS. For example, the simulated TWS peak in 2002 in the Solimoes River basin results from the anomalous high precipitation; however this could not be validated due to a data gap in GRACE. Overall, the model performance is better in the first half of the simulation period (i.e., 2002–2008) compared to the second half, especially in the western sub-basins including the Solimoes and Japura, which could be partially attributed to the decreasing trend in the precipitation forcing noted in Fig. S6.
Figure 2 also shows the seasonal cycle including the contribution of different storage components to TWS. In all the basins, the simulated seasonal cycle matches extremely well with GRACE, adding more confidence to the model results. TWS signal is sturdily modulated by the sub-surface water storage, demonstrating the importance of groundwater in the Amazon, especially in the southwestern sub-basins. The inverse relationship in the seasonal cycle of two sub-surface water stores, viz. soil moisture and groundwater, is readily discernable in Fig. 2, which is caused by the competing use of the sub-surface compartment by the two terms (Felfelani et al., 2017; Pokhrel et al., 2013). However, in some sub-basins, such as the Purus, Solimoes, and Negro, the low-lying areas with large floodplains cause floodwater storage to be equally prominent.
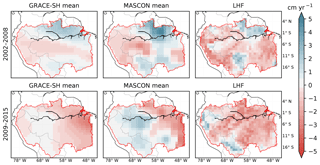
Figure 3Temporal trend of GRACE solutions compared to the trend in simulated TWS from LHF for the Amazon River basin for two different time periods. GRACE-SH trends displayed are mean trends computed from water thickness anomalies obtained from CSR, GFZ, and JPL processing centers, whereas the mascon mean trend is computed from anomalies obtained from CSR and JPL centers.
3.3 Trends in simulated TWS and comparison with GRACE
Here, we present a more detailed examination of the simulated TWS by comparing its spatial variability and trend with GRACE data. Because a shift in agreement between model and GRACE was detected in Figs. 2 and S7, we conduct a trend analysis for two different time windows: 2002–2008 and 2009–2015 (Fig. 3). It is evident from Fig. 3 that the model captures the general spatial pattern of TWS trend in GRACE and its north–south and east–west gradients especially for the first half of the analysis period; however, notable differences are evident in the second half (2009–2015), particularly over the Madeira River basin. This is a noteworthy observation given that the basin-averaged TWS variability matches extremely well with GRACE data (Fig. 2) and thus warrants further investigation. There could be a number of factors contributing to the disagreement, some of which could be model-specific (e.g., wet bias in simulated discharge; Fig. S3); however, this is a general pattern observed in many hydrological models as reported in a recent study (Scanlon et al., 2018).
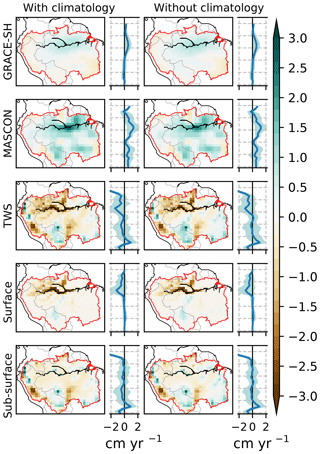
Figure 4Same as in Fig. 3 but for the complete model–GRACE overlap period (i.e., 2002–2015). The latitudinal mean is shown on the right side of each panel.
Scanlon et al. (2018) indicated a low correlation between GRACE and models, which they attributed to the (i) lack of surface water and groundwater storage components in most of the models, (ii) uncertainty in climate forcing, and (iii) poor representation of human intervention in the models (Scanlon et al., 2018; Sun et al., 2019). Here, we shed more light on the disagreement issue by investigating the contributions from the explicitly simulated surface and sub-surface storage components and their latitudinal patterns, addressing the first concern noted above which is the most critical among the three in the Amazon because of the varying contribution of different stores across scales (Pokhrel et al., 2013). Figure 4 shows trends in TWS anomalies from GRACE products and the LHF simulation for the complete model–GRACE overlap period (i.e., 2002–2015) with climatology and with climatology removed; for LHF results, the surface and sub-surface component contributions to the TWS are shown. Also shown in the figure are the zonal means.
Simulated TWS from the LHF model displays a higher correlation with GRACE trends compared to most of the global models discussed in Scanlon et al. (2018). Due to the incorporation of a groundwater scheme and other surface water dynamics, the trend in basin-averaged TWS with climatology removed for the Amazon River basin is found to be −1.64 mm yr−1, much less negative than most of the simulated TWS trends reported in Scanlon et al. (2018). The difference in the sign of trend can partly be explained by the negative trend observed in the WFDEI precipitation (Fig. S6), concentrated over the Andes region which eventually drains into the main stem of the Amazon through the Solimoes River. Due to steep topography, the impact of decreased precipitation over the Andes range is carried over to its foothills in terms of runoff, hence corresponding well with the negative trends in simulated surface water storage over the Central Amazon (Fig. 4). Lower recharge rates in the region with decreasing precipitation trend (Fig. S6) are also very likely, which is supported by the negative trend visible in the sub-surface water storage in Fig. 4, over the northwest region of the Amazon. Hence, it can be concluded that, even though the model shows some bias in TWS compared to GRACE data, the model accurately represents the key hydrologic processes in the Amazon basin; yet, these results should be interpreted with some caution while acknowledging the uncertainty in the forcing dataset. We also emphasize that it is important to evaluate models using spatiotemporal trends, especially with GRACE, instead of just using the basin-averaged time series, a commonly used approach in most previous studies.
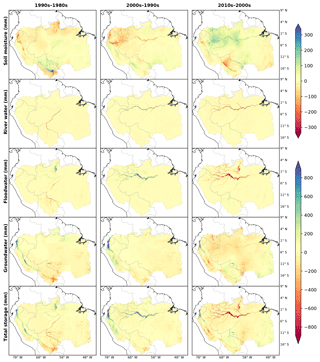
Figure 5Interdecadal difference between individual water store and TWS storage for the period of 1980–2015 at the original ∼2 km model grids. The changes are displayed as the difference between consecutive decadal means for TWS and its components. Decadal windows are 1980–1989 as 1980s, 1990–1999 as 1990s, 2000–2009 as 2000s, and 2010–2015 as 2010s. Note that the 2010s period consists of only six years, and the ranges of color bars differ among the plots.
3.4 Interannual and interdecadal TWS change and variability
Figure 5 show the interdecadal shifts in mean simulated TWS (total and its components) for the simulation period. Several observations can be made from this figure. First, the change between the 2010s and 2000s suggests high negative anomalies in all the water stores, especially over Central Amazon. This is likely a result of increasing drought occurrence and severity in the region, e.g., the 2010 (Lewis et al., 2011; Marengo et al., 2011) and 2015 (Jiménez-Muñoz et al., 2016) Amazonian droughts. Second, although the 2000s encompassed one of the severe Amazonian droughts, viz. 2005 (Marengo et al., 2008; Zeng et al., 2008), its impact was not pronounced in terms of the decadal mean, which could be due to the offset caused by anomalous wet years including 2006 and 2009 (Chen et al., 2010; Filizola et al., 2014). Third, we find an increase in river water storage in the northwestern region and decrease in the southwest of the Amazon on a decadal scale (Fig. 5, column 1, row 2), which is in line with the findings reported in previous studies based on the observed streamflow in 18 sub-basins for the 1974–2004 period (Espinoza et al., 2009; Wongchuig Correa et al., 2017).
The most remarkable feature we observe in Fig. 5 is the exceptional interdecadal shifts between the 2000s and 2010s. The central and northwestern part of the Amazon region, encompassing the Negro and Solimoes, along with some parts of the Madeira in the southwest, experienced a major decadal dry spell compared to the previous decades. Although a major part of this decadal dry condition could be attributed to the decreasing trend in input precipitation discussed in Sect. 3.3 (Fig. S6), the regional hydrologic changes in terms of TWS are also prominent. Another peculiar phenomenon observed at the decadal scale is the start of the negative anomaly in groundwater storage over the Central Amazon. A small but spatially well distributed below-decadal-average water table (dictated by groundwater storage) is evident in the Central Amazon region and the upper stretches of the Madeira basin during the 2010s (Fig. 5, column 3, row 4). Since the water table is shallow, and groundwater is the major contributor of streamflow in this region (Miguez-Macho and Fan, 2012a), some part of the negative anomaly in surface water stores can be attributed to the below-decadal-average groundwater table.
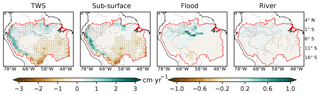
Figure 6Temporal trend in simulated TWS and its components (i.e., sub-surface water, floodwater, and river water stores) for the period of 1980 to 2015 expressed in centimeters per year (cm yr−1). Markers indicate significant trends at the 99 % level. Note that the ranges of color bars differ among the plots.
Significant long-term trends in simulated TWS and its components are evident in sizeable portions of the basin (Fig. 6). While a negative trend is found in the southern and southeastern regions (e.g., Madeira, Tapajos, Xingu and Tocantins), the trend is positive in the northern and western regions (Solimoes and Negro) (see Fig. S9 for basin-averaged trends). Being the major contributor, sub-surface water storage mimics the trend patterns in TWS (see Sect. 3.2). On the contrary, surface water storage trends are mainly dominated by floodwater and are concentrated along the main stem of the Amazon and the upper reaches of the Negro. The positive trends in floodwater can be explained by the corresponding trends in input precipitation (Fig. S5). Excess precipitation in sub-basins, such as the Solimoes and Negro, which are characterized by a high topographic gradient, is directly translated in the surface water storage, in this case floodwater. Although a corresponding increment in river water storage is also expected, its smaller storage makes the trend magnitudes negligible. Nominal negative trends, but significant, in floodwater storage are found in the upper reaches of Madeira as well, corresponding to the negative trends in input precipitation over that region.
To provide an in-depth understanding of the interdecadal changes occurring in the Amazon region and to determine whether the changes observed in Fig. 5 are significant, we applied a t test methodology to the long-term TWS anomalies at basin and sub-basin levels. The spatial changes observed in Fig. 5 are summarized with their interdecadal significance in Table S1 (Supplement), along with the decadal means and standard deviations. Significant change at 99 % level is found in the Negro River basin throughout the study period, followed by the Solimoes River basin exhibiting significant change in the last three decades. These changes can be attributed to the corresponding changes in precipitation (Fig. S5), which follow a similar change in respective basins. However, the significant hydrologic changes in the Tocantins and Madeira can be primarily attributed to LULC changes, as the corresponding changes in precipitation were relatively negligible. For example, the Tocantins River basin underwent major LULC changes in response to heavy deforestation caused by dam construction and cattle farming (Costa et al., 2003) until policies were imposed in 2004 by the Brazilian government (captured in the ESA dataset; Fig. S8). Similarly, the Madeira River basin also endured major LULC changes in the late 1990s, which were dominated by agricultural expansion (Dórea and Barbosa, 2007).
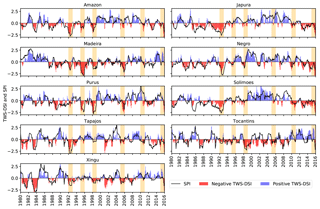
Figure 7TWS drought severity index (TWS-DSI) calculated using the simulated TWS from LHF for Amazon and its sub-basins. TWS-DSI is calculated using basin-averaged TWS anomalies on a monthly scale. Shaded areas indicate the severe drought years reported in the past literature. The black line is the 12-month Standardized Precipitation Index (SPI) calculated by using basin-averaged precipitation data from the WFDEI forcing dataset.
3.5 Interannual and interdecadal drought evolution
3.5.1 Severity of TWS drought
In this section, we examine the time evolution of droughts and quantify their impacts on TWS variability by using TWS-DSI. The use of TWS-DSI enables the depiction of a “bigger picture” encompassing all water stores that represent the vertically integrated total water availability during droughts and dictate the streamflow. Figure 7 shows the TWS-DSI for individual Amazonian sub-basins and the 12-month Standardized Precipitation Index (SPI) (Mckee et al., 1993) calculated from the basin-averaged precipitation time series. As expected TWS-DSI follows a similar pattern of the SPI, but differences in the index peaks can be noted for the drought years. For example, the 2005 drought was prominent in terms of TWS in the southwest region, comprising Purus and Madeira rivers, with TWS-DSI going as high as −3, whereas the corresponding SPI values were −1.78 and −2.2, respectively. Similarly, severe TWS drought (e.g., 2001) is detected in the southeastern basins of the Amazon (Madeira, Xingu, and Tocantins); however, the corresponding SPI values are negligible. The sub-surface storage (major contributor of TWS in these sub-basins) characteristic can be noted in these cases which has a delayed response from the preceding series of low precipitation events due to a slow residence time.
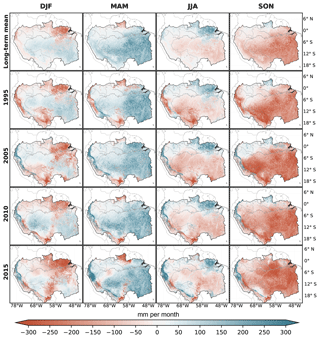
Figure 8Seasonal dynamics of simulated sub-surface water storage from LHF in the Amazon River basin for extreme droughts during the simulation period. Long term mean is the mean seasonal anomaly for the 1980–2015 period, where DJF is December to February, MAM is March to May, JJA is June to August, and SON is September to November.
The impact of drought conditions on TWS is quantified by examining the seasonal dynamics in the simulated sub-surface water storage for the four most extreme historical drought years during the simulation period (Fig. 8). Although no clear trend can be seen in terms of the evolution of the drought impact on sub-surface water storage, the spatial variability between different drought years is readily discernible. For example, the 1995 and 2010 droughts more or less had a similar magnitude and spatial impact on the sub-surface storage; however, the 2005 drought was more intense and dramatic in the Solimoes River basin, findings also noted in previous studies (Marengo et al., 2008; Phillips et al., 2009; Zeng et al., 2008). Similarly, the more recent drought in 2015 had a more pronounced impact in the eastern and northeastern region and average impact on the other parts of the basin. Due to the shallow water table in the Amazonian lowlands, sub-surface storage acts as a buffer during the low precipitation events, hence facing higher anomalies during drought conditions compared to the long-term mean. As the Negro River (i.e. northern region of the Amazon) basin experiences an opposite seasonal phase compared to the rest of the Amazon region, the drought conditions in this basin are observed during the period of December to March. The opposite seasonal cycle of precipitation and flooding in the north and south banks of the Amazon mitigates the number of floods and droughts in the basin as a whole while resulting in more dramatic floods or droughts in particular sub-basins (e.g., Tocantins, Tapajos and Madeira).
3.5.2 Time evolution of dry-season total deficit and TWS release
The dry-season TWS variability is examined by using the cumulative difference between PET and P, termed as the TWD (see Sect. 2.7). Further, to examine the response from TWS against TWD, we quantify the TWS-R, hence creating a supply–demand relationship between them. Figure S10 shows TWD, the corresponding TWS-R, and the total contribution of the surface water storage to TWS-R for the extreme drought years during 1980–2015 compared to their respective long-term means. Spatial patterns in TWD and TWS-R are analogous to the patterns in the simulated sub-surface storage during the months of September to November (SON) as seen in Fig. 8. We find that TWS-R receives a fairly equal contribution from surface (along the rivers) and sub-surface (soil moisture and groundwater) water stores (rest of the region); however, the latter is more dominant during drought years. A clear positive trend in drought years is visible in Fig. S10, indicating an increase in TWS-R, with a significant sub-surface contribution, especially in the southeastern part of the Amazon. This change can be directly attributed to the major LULC changes occurring in the basin, causing loss of TWS to evapotranspiration through agricultural expansion, especially in the Tocantins, Xingu, Tapajos, and Madeira river basins (Chen et al., 2015; Costa et al., 2003; Dórea and Barbosa, 2007).
3.5.3 Hydrological drought trends in Amazonian sub-catchments
The hydrological drought behavior of each sub-basin is characterized by quantifying the drought days per year at the Level-5 HydroBASINS scale (Lehner and Grill, 2013), referred here to as “sub-catchments”. Based on the streamflow simulated at the most downstream grid in the sub-catchments, temporal trends for the 1980–2015 period are calculated and presented in Fig. 9. Significant trends in drought durations are discernible in the Tapajos and Madeira sub-basin along with the southeastern portions of the Amazon, congruent with the heavy deforestation activities found in these sub-basins (Chen et al., 2015; Costa et al., 2003; Dórea and Barbosa, 2007). Although LULC changes, such as deforestation activities, generally increase streamflow and are also known to offset the impact on streamflow caused by a decrease in precipitation over the Amazon (Panday et al., 2015), this mechanism is dominant mostly during the wet season. In the dry season, however, the streams in the Amazon are fed primarily by the sub-surface water storage (see Sect. 3.2), which is negatively impacted by deforestation activities (e.g., increased regional evapotranspiration).
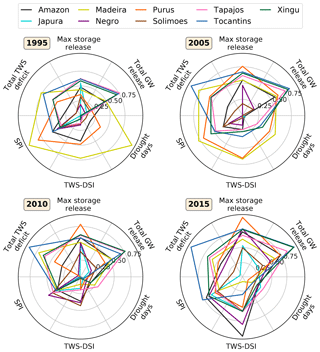
Figure 10Intercomparison and comprehensive characterization of the severe drought events during the study period in the Amazon River basin and its sub-basins. Color coding in each subplot represents individual river basins. Note that all variables are basin averages normalized (0–1) for each variable over all drought years. The bottom half of the variables in the figure are drought indices representing different types of droughts: TWS-DSI denotes TWS drought severity index (Sect. 2.7), SPI (Standardized Precipitation Index) represents meteorological drought severity, and “drought days” represents hydrological drought severity in the basin (Sect. 2.6). The top half of the variables quantify the water deficit in terms of total TWS deficit (cumulative PET-P), water supply as the TWS release (max storage release), and the groundwater contribution of TWS release (total GW release).
3.6 Comprehensive characterization of Amazonian droughts
As a first attempt to comprehensively characterize the Amazonian droughts, we present a summary of all the drought characteristics discussed in the previous sections on a spider plot (Fig. 10). Each spider plot is a representation of a drought year with respect to the (i) causes of drought and their type in terms of common indices; (ii) response of different water stores, such as TWS, to the drought event; (iii) role of groundwater storage in alleviating the dry conditions on the surface; and (iv) the spatial impact of the drought in different sub-basins of the Amazon. Although no significant trend in the combined drought characteristic is apparent, Fig. 10 provides important insights into the variability of Amazonian droughts. It is evident from the figure that the drought variability over the years was significant in terms of both magnitude and spatial impact. The most notable feature in Fig. 10 is the distinct relationship between the SPI and drought duration. For example, during the 1995 drought, most of the river basins (e.g., Tocantins, Tapajos, Xingu, and Negro) experienced significant meteorological and TWS droughts; however, the severity of hydrological droughts was relatively negligible in those basins. Groundwater–surface water exchange is the key mechanism behind this unique behavior, causing groundwater to fulfill the drought deficit in streamflow over the basin. Due to shallow water tables at the downstream end of these basins, a significant quantity of groundwater is fed to the rivers, which manifests as high peaks in total groundwater release, evident in Fig. 10. Similarly, a high number of drought days are found corresponding to less groundwater release, such as during the 1995 drought in Madeira. Conversely, TWS-DSI generally follows the same pattern as that of the SPI but with a lesser magnitude, which can be attributed to the delayed response from groundwater.
Further, the behavior of the Amazonian sub-basins can be characterized by the shape of the polygon formed by the comparison of different aspects of past droughts. The convex and concave characteristic in the plots mainly depends on the interrelation between meteorological and hydrological drought indices, which is further controlled by the sub-surface water storage. A convex polygon indicates a lower groundwater contribution to streamflow in the sub-basin, such as in Purus during 1995 and 2005, whereas a concave polygon suggests higher groundwater release to streamflow in that particular year.
3.7 Intensification of the Amazonian dry season
Results suggest an increasing trend in TWD with significant decadal variability over the Amazon and its sub-basins, indicating an increase in dry-season length over the past 36 years (Fig. 11). Further, the increasing gap between TWD and TWS-R suggests an intensified terrestrial hydrologic system over the dry season during the study period. As the LULC impact is partly accounted for in the PET calculations (i.e., through changing surface albedo), the river basins with substantial LULC change, such as Madeira, Tapajos, Tocantins, and Xingu, portray higher TWD trend magnitudes (significance >95 %). The peaks in the TWD correspond well with drought years; for example, the peaks in the TWD for Madeira are analogous to the drought years (e.g., 1988, 1995, 2005, and 2010). Due to this definitive response to drought conditions, TWD is also used to characterize historical drought events in earlier sections. We note that the trends in the total deficit should be interpreted with caution as the uncertainty in the forcing could have affected TWD and TWS-R trend estimates.
We find that the river basins that contain high altitudinal areas (Purus, Solimoes, and Negro) have a fairly balanced relationship between TWD and TWS-R, but southern and southeastern sub-basins exhibit a higher water deficiency (Fig. S11), with approximately 2- to 3-fold differences between TWD and TWS-R during regular years. For drought years, however, the difference between TWD and TWS-R is even higher, creating highly anomalous dry conditions in the sub-basins. Consistent higher values of TWD in southern and southeastern sub-basins of the Amazon further highlight the intensification of the dry season, with increasing water deficiency corresponding to an almost constant water supply from TWS-R. This phenomenon is also highlighted in Espinoza et al. (2016), which showed an significant increase in dry day frequency in the central and southern parts of the Amazon. Results from this study combined with the reported increasing trend in the wet season (Gloor et al., 2013) imply an overall intensification of the Amazonian hydrological cycle.
In this study, we examine the interannual and interdecadal trends and variability in the terrestrial hydrological system in the Amazon basin and its sub-basins, with a focus on droughts and their time evolution during the 1980–2015 period by using a continental-scale hydrological model LEAF-Hydro-Flood (LHF) and terrestrial water storage (TWS) data from the GRACE satellite mission. For the first time, we provide a comprehensive characterization of extreme drought events in the Amazon basin during the past four decades, while categorizing them with respect to their (i) cause, (ii) type, (iii) spatial extent, and (iv) impacts on different water stores. We also provide an in-depth understanding of the interrelation between different drought types and the corresponding response of the sub-surface storage to surface drought conditions. Our key findings are summarized below.
First, the LHF model simulates the basin-averaged TWS variations and seasonal cycle remarkably well for most of the sub-basins compared to GRACE data; however, some differences are observed in the spatial distribution of temporal trends for the post-2008 period. We find that this discrepancy is caused primarily by the uncertainty in surface water storage simulations along the main stem of the Negro and Amazon, whereas uncertainty in sub-surface storage prevails over the Andes. Second, the 2010–2015 period was found to be the driest in the past four decades due to an increase in the frequency and severity of droughts. A t test conducted on the TWS time series also indicated significant changes at the 99 % level in the decadal mean TWS in the Negro and Solimoes sub-basins. Third, high negative long-term trends in TWS and increasing divergence between dry-season total water deficit (TWD) and corresponding TWS release (TWS-R) indicate significant drying in sub-basins such as Madeira, Tapajos, Xingu, and Tocantins. Basin-averaged trends indicate that the Amazon is getting wetter (1.13 mm yr−1); however, its southern and southeastern portions are getting drier. TWD is also found to be higher than TWS-R in these sub-basins, with approximately a 3-fold difference between the two during some drought years, indicating a strengthening dry season in the region. Fourth, most of the extreme meteorological droughts do not propagate to hydrological droughts significantly, as the deficit is absorbed by the sub-surface water storage, further reducing TWS drought severity compared to that of a meteorological drought in the Amazonian sub-basins.
Altogether, these results provide important insights into the interannual and interdecadal hydrological changes and the key mechanisms that govern drought events in the Amazon, along with a novel way of categorizing basin behavior during drought occurrence (Fig. 10). This framework can be applied to better predict future hydrological conditions and their corresponding socioeconomic impacts toward taking measures to mitigate the drought impacts and facilitate a relatively facile transition of the local population through a future drought event. Basin drying trends reported in this study can also provide key leverage by applying them toward anticipation of future hydrological conditions for the sustainable management of water resources. We also highlight the importance of using spatiotemporal trend estimates for model validation, especially with GRACE, instead of the commonly employed approach of time series comparison. Improvement in the correlation between the temporal trends in simulated TWS and the GRACE anomaly through the inclusion of a prognostic groundwater scheme, which allows dynamic groundwater–surface water interactions in the model framework, is also highlighted. Further, the need to investigate the effects of uncertainties in model forcing to TWS simulations is noted because we find that the trends in precipitation are strongly propagated to TWS simulations.
A limitation of the present study is that the effects of irrigation and man-made reservoirs are not yet incorporated in the model. The basin-wide effects of the existing dams in the Amazon are small (Pokhrel et al., 2012a); however, as more dams are added across the basin, it will become critical to account for such effects. Model improvement is underway (Pokhrel et al., 2018; Shin et al., 2018), and these issues will be addressed in our forthcoming publications. Despite some limitations, this study significantly advances the understanding of changing Amazonian hydrology, and our results have important implications for predicting and monitoring extreme droughts in the region; the research framework can also be applied to other global regions undergoing similar hydrological changes.
Observed river discharge data, land use–land cover maps, and GRACE terrestrial water storage anomalies are available for free download from respective web addresses provided in the Acknowledgements. The simulation results and other findings of this study are available from the corresponding author upon reasonable request.
The supplement related to this article is available online at: https://doi.org/10.5194/hess-23-2841-2019-supplement.
SC, YP, and EM designed the research; YP, SC, and GM set up the model; SC performed simulations, analyzed results, and prepared the draft; all authors discussed the results and wrote the manuscript.
The authors declare that they have no conflict of interest.
Observed river discharge data are obtained from the Agência Nacional de Águas (ANA) in Brazil (http://hidroweb.ana.gov.br, last access: 24 June 2019). We obtained the annual land cover maps from the European Space Agency Climate Change Initiative Land Cover project (http://maps.elie.ucl.ac.be/CCI/viewer/index.php, last access: 24 June 2019). Simulations were conducted at Cheyenne (https://doi.org/10.5065/D6RX99HX) provided by NCAR's Computational and Information Systems Laboratory sponsored by the National Science Foundation. None of these funding sources or agencies should be held responsible for the views herein. They are the sole responsibility of the authors.
This research has been supported by the National Science Foundation (grant no. 1639115).
This paper was edited by Nadia Ursino and reviewed by two anonymous referees.
Alho, C. J. R., Reis, R. E., and Aquino, P. P. U.: Amazonian freshwater habitats experiencing environmental and socioeconomic threats affecting subsistence fisheries, Ambio, 44, 412–425, https://doi.org/10.1007/s13280-014-0610-z, 2015.
Aragão, L. E. O. C., Malhi, Y., Roman-Cuesta, R. M., Saatchi, S., Anderson, L. O., and Shimabukuro, Y. E.: Spatial patterns and fire response of recent Amazonian droughts, Geophys. Res. Lett., 34, L07701, https://doi.org/10.1029/2006GL028946, 2007.
Arantes, A. E., Ferreira, L. G., and Coe, M. T.: The seasonal carbon and water balances of the Cerrado environment of Brazil: Past, present, and future influences of land cover and land use, ISPRS J. Photogramm. Remote Sens., 117, 66–78, https://doi.org/10.1016/j.isprsjprs.2016.02.008, 2016.
Asner, G. P., Scurlock, J. M. O., and A. Hicke, J.: Global synthesis of leaf area index observations: implications for ecological and remote sensing studies, Glob. Ecol. Biogeogr., 12, 191–205, https://doi.org/10.1046/j.1466-822X.2003.00026.x, 2003.
Barletta, M., Jaureguizar, A. J., Baigun, C., Fontoura, N. F., Agostinho, A. A., Almeida-Val, V. M. F., Val, A. L., Torres, R. A., Jimenes-Segura, L. F., Giarrizzo, T., Fabré, N. N., Batista, V. S., Lasso, C., Taphorn, D. C., Costa, M. F., Chaves, P. T., Vieira, J. P., and Corrêa, M. F. M.: Fish and aquatic habitat conservation in South America: a continental overview with emphasis on neotropical systems, J. Fish Biol., 76, 2118–2176, https://doi.org/10.1111/j.1095-8649.2010.02684.x, 2010.
Bates, P. D., Horritt, M. S., and Fewtrell, T. J.: A simple inertial formulation of the shallow water equations for efficient two-dimensional flood inundation modelling, J. Hydrol., 387, 33–45, https://doi.org/10.1016/j.jhydrol.2010.03.027, 2010.
Beck, H. E., van Dijk, A. I. J. M., de Roo, A., Dutra, E., Fink, G., Orth, R., and Schellekens, J.: Global evaluation of runoff from 10 state-of-the-art hydrological models, Hydrol. Earth Syst. Sci., 21, 2881–2903, https://doi.org/10.5194/hess-21-2881-2017, 2017.
Betts, A. K., Ball, J. H., Viterbo, P., Dai, A., and Marengo, J.: Hydrometeorology of the Amazon in ERA-40, J. Hydrometeorol., 6, 764–774, https://doi.org/10.1175/JHM441.1, 2005.
Bonnet, M. P., Barroux, G., Martinez, J. M., Seyler, F., Moreira-Turcq, P., Cochonneau, G., Melack, J. M., Boaventura, G., Maurice-Bourgoin, L., León, J. G., Roux, E., Calmant, S., Kosuth, P., Guyot, J. L., and Seyler, P.: Floodplain hydrology in an Amazon floodplain lake (Lago Grande de Curuaí), J. Hydrol., 349, 18–30, https://doi.org/10.1016/j.jhydrol.2007.10.055, 2008.
Brando, P. M., Balch, J. K., Nepstad, D. C., Morton, D. C., Putz, F. E., Coe, M. T., Silverio, D., Macedo, M. N., Davidson, E. A., Nobrega, C. C., Alencar, A., and Soares-Filho, B. S.: Abrupt increases in Amazonian tree mortality due to drought-fire interactions, P. Natl. Acad. Sci. USA, 111, 6347–6352, https://doi.org/10.1073/pnas.1305499111, 2014.
Brondizio, E. S. and Moran, E. F.: Human dimensions of climate change: The vulnerability of small farmers in the Amazon, Philos. T. Roy. Soc. A, 363, 1803–1809, https://doi.org/10.1098/rstb.2007.0025, 2008.
Castello, L., Mcgrath, D. G., Hess, L. L., Coe, M. T., Lefebvre, P. A., Petry, P., Macedo, M. N., Renó, V. F., and Arantes, C. C.: The vulnerability of Amazon freshwater ecosystems, Conserv. Lett., 6, 217–229, https://doi.org/10.1111/conl.12008, 2013.
Castello, L., Isaac, V. J., and Thapa, R.: Flood pulse effects on multispecies fishery yields in the Lower Amazon, R. Soc. Open Sci., 2, 150299, doi:10.1098/rsos.150299, 2015.
Chaudhari, S., Felfelani, F., Shin, S., and Pokhrel, Y.: Climate and Anthropogenic Contributions to the Desiccation of the Second Largest Saline Lake in the Twentieth Century, J. Hydrol., 560, 342–353, https://doi.org/10.1016/j.jhydrol.2018.03.034, 2018.
Chen, G., Powers, R. P., de Carvalho, L. M. T., and Mora, B.: Spatiotemporal patterns of tropical deforestation and forest degradation in response to the operation of the Tucuruí hydroelectric dam in the Amazon basin, Appl. Geogr., 63, 1–8, https://doi.org/10.1016/j.apgeog.2015.06.001, 2015.
Chen, J. L., Wilson, C. R., Tapley, B. D., Yang, Z. L., and Niu, G. Y.: 2005 drought event in the Amazon River basin as measured by GRACE and estimated by climate models, J. Geophys. Res.-Sol. Ea., 114, 1–9, https://doi.org/10.1029/2008JB006056, 2009.
Chen, J. L., Wilson, C. R., and Tapley, B. D.: The 2009 exceptional Amazon flood and interannual terrestrial water storage change observed by GRACE, Water Resour. Res., 46, 1–10, https://doi.org/10.1029/2010WR009383, 2010.
Clark, E. A., Sheffield, J., van Vliet, M. T. H., Nijssen, B., and Lettenmaier, D. P.: Continental Runoff into the Oceans (1950–2008), J. Hydrometeorol., 16, 1502–1520, https://doi.org/10.1175/JHM-D-14-0183.1, 2015.
Coe, M. T., Costa, M. H., Botta, A., and Birkett, C.: Long-term simulations of discharge and floods in the Amazon Basin, J. Geophys. Res.-Atmos., 107, 1–17, https://doi.org/10.1029/2001JD000740, 2002.
Coe, M. T., Costa, M. H., and Howard, E. A.: Simulating the surface waters of the Amazon River basin: impacts of new river geomorphic and flow parameterizations, Hydrol. Process., 22, 2542–2553, https://doi.org/10.1002/hyp.6850, 2008.
Coe, M. T., Costa, M. H., and Soares-Filho, B. S.: The influence of historical and potential future deforestation on the stream flow of the Amazon River – Land surface processes and atmospheric feedbacks, J. Hydrol., 369, 165–174, https://doi.org/10.1016/j.jhydrol.2009.02.043, 2009.
Cook, B., Zeng, N., and Yoon, J. H.: Will Amazonia dry out?, Magnitude and causes of change from IPCC climate model projections, Earth Interact., 16, https://doi.org/10.1175/2011EI398.1, 2012.
Cook, K. H. and Vizy, E. K.: Effects of twenty-first-century climate change on the Amazon rain forest, J. Climate, 21, 542–560, https://doi.org/10.1175/2007JCLI1838.1, 2008.
Costa, M. H. and Foley, J. A.: Trends in the hydrologic cycle of the Amazon Basin, J. Geophys. Res.-Atmos., 104, 14189–14198, https://doi.org/10.1029/1998JD200126, 1999.
Costa, M. H., Botta, A., and Cardille, J. A.: Effects of large-scale changes in land cover on the discharge of the Tocantins River, Southeastern Amazonia, J. Hydrol., 283, 206–217, https://doi.org/10.1016/S0022-1694(03)00267-1, 2003.
Cox, P. M., Betts, R. A., Collins, M., Harris, P. P., Huntingford, C., and Jones, C. D.: Amazonian forest dieback under climate-carbon cycle projections for the 21st century, Theor. Appl. Climatol., 78, 137–156, https://doi.org/10.1007/s00704-004-0049-4, 2004.
da Costa, C. L., Galbraith, D., Almeida, S., Tanaka Portela, B. T., da Costa, M., de Athaydes Silva Junior, J., Braga, A. P., de Gonçalves, P. H. L., de Oliveira, A. A., Fisher, R., Phillips, O., Metcalfe, D. B., Levy, P., and Meir, P.: Effect of seven years of experimental drought on the aboveground biomass storage of an eastern Amazonian rainforest, New Phytol., 187, 579–591, https://doi.org/10.1111/j.1469-8137.2010.03309.x, 2010.
Davidson, E. A., de Araújo, A. C., Artaxo, P., Balch, J. K., Brown, I. F., C. Bustamante, M. M., Coe, M. T., DeFries, R. S., Keller, M., Longo, M., Munger, J. W., Schroeder, W., Soares-Filho, B. S., Souza, C. M., and Wofsy, S. C.: The Amazon basin in transition, Nature, 481, 321–328, https://doi.org/10.1038/nature10717, 2012.
Dias, L. C. P., Macedo, M. N., Costa, M. H., Coe, M. T., and Neill, C.: Effects of land cover change on evapotranspiration and streamflow of small catchments in the Upper Xingu River Basin, Central Brazil, J. Hydrol. Reg. Stud., 4, 108–122, https://doi.org/10.1016/j.ejrh.2015.05.010, 2015.
Dórea, J. G. and Barbosa, A. C.: Anthropogenic impact of mercury accumulation in fish from the Rio Madeira and Rio Negro Rivers (Amazonia), Biol. Trace Elem. Res., 115, 243–254, https://doi.org/10.1007/BF02685999, 2007.
Espinoza, J. C., Guyot, J. L., Ronchail, J., Cochonneau, G., Filizola, N., Fraizy, P., Labat, D., de Oliveira, E., Ordoñez, J. J., and Vauchel, P.: Contrasting regional discharge evolutions in the Amazon basin (1974–2004), J. Hydrol., 375, 297–311, https://doi.org/10.1016/j.jhydrol.2009.03.004, 2009.
Espinoza, J. C., Ronchail, J., Guyot, J. L., Junquas, C., Vauchel, P., Lavado, W., Drapeau, G., and Pombosa, R.: Climate variability and extreme drought in the upper Solimões River (western Amazon Basin): Understanding the exceptional 2010 drought, Geophys. Res. Lett., 38, 1–6, https://doi.org/10.1029/2011GL047862, 2011.
Espinoza, J. C., Chavez, S., Ronchail, J., Junquas, C., Takahashi, K., and Lavado, W.: Rainfall hotspots over the southern tropical Andes: Spatial distribution, rainfall intensity, and relations with large-scale atmospheric circulation, Water Resour. Res., 51, 3459–3475, https://doi.org/10.1002/2014WR016273, 2015.
Espinoza, J. C., Segura, H., Ronchail, J., Drapeau, G., and Gutierrez-Cori, O.: Evolution of wet-day and dry-day frequency in the western Amazon basin: Relationship with atmospheric circulation and impacts on vegetation, Water Resour. Res., 52, 8546–8560, https://doi.org/10.1002/2016WR019305, 2016.
Espinoza Villar, J. C., Ronchail, J., Guyot, J. L., Cochonneau, G., Naziano, F., Lavado, W., De Oliveira, E., Pombosa, R., and Vauchel, P.: Spatio-temporal rainfall variability in the Amazon basin countries (Brazil, Peru, Bolivia, Colombia, and Ecuador), Int. J. Climatol., 29, 1574–1594, https://doi.org/10.1002/joc.1791, 2009.
Fan, Y. and Miguez-Macho, G.: Potential groundwater contribution to Amazon evapotranspiration, Hydrol. Earth Syst. Sci., 14, 2039–2056, https://doi.org/10.5194/hess-14-2039-2010, 2010.
Fan, Y., Li, H., and Miguez-Macho, G.: Global Patterns of Groundwater Table Depth, Science, 339, 940–943, https://doi.org/10.1126/science.1229881, 2013.
Fan, Y., Clark, M., Lawrence, D. M., Swenson, S., Band, L. E., Brantley, S. L., Brooks, P. D., Dietrich, W. E., Flores, A., Grant, G., Kirchner, J. W., Mackay, D. S., McDonnell, J. J., Milly, P. C. D., Sullivan, P. L., Tague, C., Ajami, H., Chaney, N., Hartmann, A., Hazenberg, P., McNamara, J., Pelletier, J., Perket, J., Rouholahnejad-Freund, E., Wagener, T., Zeng, X., Beighley, E., Buzan, J., Huang, M., Livneh, B., Mohanty, B. P., Nijssen, B., Safeeq, M., Shen, C., van Verseveld, W., Volk, J., and Yamazaki, D.: Hillslope Hydrology in Global Change Research and Earth System Modeling, Water Resour. Res., 55, 1737–1772, https://doi.org/10.1029/2018WR023903, 2019.
Felfelani, F., Wada, Y., Longuevergne, L., and Pokhrel, Y. N.: Natural and human-induced terrestrial water storage change: A global analysis using hydrological models and GRACE, J. Hydrol., 553, 105–118, https://doi.org/10.1016/j.jhydrol.2017.07.048, 2017.
Fernandes, K., Fu, R., and Betts, A. K.: How well does the ERA40 surface water budget compare to observations in the Amazon River basin?, J. Geophys. Res.-Atmos., 113, 1–9, https://doi.org/10.1029/2007JD009220, 2008.
Fernandes, K., Baethgen, W., Bernardes, S., Defries, R., Dewitt, D. G., Goddard, L., Lavado, W., Lee, D. E., Padoch, C., Pinedo-Vasquez, M., and Uriarte, M.: North Tropical Atlantic influence on western Amazon fire season variability, Geophys. Res. Lett., 38, 1–5, https://doi.org/10.1029/2011GL047392, 2011.
Field, C. B., Behrenfeld, M. J., Randerson, J. T., and Falkowski, P.: Primary Production of the Biosphere: Integrating Terrestrial and Oceanic Components, Science, 281, 237–240, https://doi.org/10.1126/science.281.5374.237, 1998.
Filizola, N., Latrubesse, E. M., Fraizy, P., Souza, R., Guimarães, V., and Guyot, J. L.: Was the 2009 flood the most hazardous or the largest ever recorded in the Amazon?, Geomorphology, 215, 99–105, https://doi.org/10.1016/j.geomorph.2013.05.028, 2014.
Finer, M. and Jenkins, C. N.: Proliferation of hydroelectric dams in the andean amazon and implications for andes-amazon connectivity, PLoS One, 7, 1–9, https://doi.org/10.1371/journal.pone.0035126, 2012.
Forsberg, B. R., Melack, J. M., Dunne, T., Barthem, R. B., Goulding, M., Paiva, R. C. D., Sorribas, M. V., Silva, U. L. and Weisser, S.: The potential impact of new Andean dams on Amazon fluvial ecosystems., PLoS One, 12, 1–35, https://doi.org/10.1371/journal.pone.0182254, 2017.
Frappart, F., Papa, F., Güntner, A., Werth, S., Santos da Silva, J., Tomasella, J., Seyler, F., Prigent, C., Rossow, W. B., Calmant, S., and Bonnet, M. P.: Satellite-based estimates of groundwater storage variations in large drainage basins with extensive floodplains, Remote Sens. Environ., 115, 1588–1594, https://doi.org/10.1016/j.rse.2011.02.003, 2011.
Frappart, F., Ramillien, G., and Ronchail, J.: Changes in terrestrial water storage versus rainfall and discharges in the Amazon basin, Int. J. Climatol., 33, 3029–3046, https://doi.org/10.1002/joc.3647, 2013.
Getirana, A. C. V., Bonnet, M.-P., Rotunno Filho, O. C., Collischonn, W., Guyot, J.-L., Seyler, F., and Mansur, W. J.: Hydrological modelling and water balance of the Negro River basin: evaluation based on in situ and spatial altimetry data, Hydrol. Process., 24, 3219–3236, https://doi.org/10.1002/hyp.7747, 2010.
Getirana, A. C. V., Boone, A., Yamazaki, D., Decharme, B., Papa, F., and Mognard, N.: The Hydrological Modeling and Analysis Platform (HyMAP): Evaluation in the Amazon Basin, J. Hydrometeorol., 13, 1641–1665, https://doi.org/10.1175/JHM-D-12-021.1, 2012.
Gloor, M., Brienen, R. J. W., Galbraith, D., Feldpausch, T. R., Schöngart, J., Guyot, J. L., Espinoza, J. C., Lloyd, J., and Phillips, O. L.: Intensification of the Amazon hydrological cycle over the last two decades, Geophys. Res. Lett., 40, 1729–1733, https://doi.org/10.1002/grl.50377, 2013.
Guan, K., Pan, M., Li, H., Wolf, A., Wu, J., Medvigy, D., Caylor, K. K., Sheffield, J., Wood, E. F., Malhi, Y., Liang, M., Kimball, J. S., Saleska, S. R., Berry, J., Joiner, J., and Lyapustin, A. I.: Photosynthetic seasonality of global tropical forests constrained by hydroclimate, Nat. Geosci., 8, 284–289, https://doi.org/10.1038/ngeo2382, 2015.
Haddeland, I., Heinke, J., Biemans, H., Eisner, S., Flörke, M., Hanasaki, N., Konzmann, M., Ludwig, F., Masaki, Y., Schewe, J., Stacke, T., Tessler, Z. D., Wada, Y., and Wisser, D.: Global water resources affected by human interventions and climate change, P. Natl. Acad. Sci. USA, 111, 3251–3256, https://doi.org/10.1073/pnas.1222475110, 2014.
Hanasaki, N., Yoshikawa, S., Pokhrel, Y., and Kanae, S.: A global hydrological simulation to specify the sources of water used by humans, Hydrol. Earth Syst. Sci., 22, 789–817, https://doi.org/10.5194/hess-22-789-2018, 2018.
Jiménez-Muñoz, J. C., Mattar, C., Barichivich, J., Santamaría-Artigas, A., Takahashi, K., Malhi, Y., Sobrino, J. A., and Schrier, G. Van Der: Record-breaking warming and extreme drought in the Amazon rainforest during the course of El Niño 2015–2016, Sci. Rep., 6, 1–7, https://doi.org/10.1038/srep33130, 2016.
Joetzjer, E., Douville, H., Delire, C., Ciais, P., Decharme, B., and Tyteca, S.: Hydrologic benchmarking of meteorological drought indices at interannual to climate change timescales: a case study over the Amazon and Mississippi river basins, Hydrol. Earth Syst. Sci., 17, 4885–4895, https://doi.org/10.5194/hess-17-4885-2013, 2013.
Kalamandeen, M., Gloor, E., Mitchard, E., Quincey, D., Ziv, G., Spracklen, D., Spracklen, B., Adami, M., Aragão, L. E. O. C., and Galbraith, D.: Pervasive Rise of Small-scale Deforestation in Amazonia, Nature, 8, 1600, https://doi.org/10.1038/s41598-018-19358-2, 2018.
Landerer, F. W. and Swenson, S. C.: Accuracy of scaled GRACE terrestrial water storage estimates, Water Resour. Res., 48, 1–11, https://doi.org/10.1029/2011WR011453, 2012.
Latrubesse, E. M., Arima, E. Y., Dunne, T., Park, E., Baker, V. R., d'Horta, F. M., Wight, C., Wittmann, F., Zuanon, J., Baker, P. A., Ribas, C. C., Norgaard, R. B., Filizola, N., Ansar, A., Flyvbjerg, B., and Stevaux, J. C.: Damming the rivers of the Amazon basin, Nature, 546, 363–369, https://doi.org/10.1038/nature22333, 2017.
Lee, J.-E., Lintner, B. R., Boyce, C. K., and Lawrence, P. J.: Land use change exacerbates tropical South American drought by sea surface temperature variability, Geophys. Res. Lett., 38, L19706, doi:10.1029/2011GL049066, 2011.
Lehner, B. and Grill, G.: Global river hydrography, and network routing: baseline data, and new approaches to study the world's large river systems, Hydrol. Process., 27, 2171–2186, https://doi.org/10.1002/hyp.9740, 2013.
Lenton, T. M., Held, H., Kriegler, E., Hall, J. W., Lucht, W., Rahmstorf, S. and Schellnhuber, H. J.: Tipping elements in the Earth System, P. Natl. Acad. Sci. USA, 106, 20561–20563, https://doi.org/10.1073/pnas.0911106106, 2009.
Lesack, L. F. W.: Water Balance and Hydrologic Characteristics of a Rain Forest Catchment in the Central Amazon Basin, Water Resour. Res., 29, 759–773, 1993.
Lewis, S. L., Brando, P. M., Phillips, O. L., van der Heijden, G. M. F., and Nepstad, D.: The 2010 Amazon Drought, Science, 331, LP-554, https://doi.org/10.1126/science.1200807, 2011.
Liang, S. and Xiao, Z.: Global Land Surface Products: Leaf Area Index Product Data Collection (1985–2010), Beijing Normal University, doi:10.6050/glass863.3004.db, 2012.
Lima, L. S., Coe, M. T., Soares Filho, B. S., Cuadra, S. V., Dias, L. C. P., Costa, M. H., Lima, L. S., and Rodrigues, H. O.: Feedbacks between deforestation, climate, and hydrology in the Southwestern Amazon: Implications for the provision of ecosystem services, Landsc. Ecol., 29, 261–274, https://doi.org/10.1007/s10980-013-9962-1, 2014.
Longuevergne, L., Scanlon, B. R., and Wilson, C. R.: GRACE hydrological estimates for small basins: Evaluating processing approaches on the High Plains aquifer, USA, Water Resour. Res., 46, 1–15, https://doi.org/10.1029/2009WR008564, 2010.
Malhi, Y., Roberts, J. T., Betts, R. A., Killeen, T. J., Li, W., and Nobre, C. a: Climate Change, Deforestation, and the Fate of the Amazon, Science, 319, 169–172, https://doi.org/10.1126/science.1146961, 2008.
Malhi, Y., Aragão, L. E. O. C., Galbraith, D., Huntingford, C., Fisher, R., Zelazowski, P., Sitch, S., McSweeney, C., and Meir, P.: Exploring the likelihood and mechanism of a climate-change-induced dieback of the Amazon rainforest, P. Natl. Acad. Sci. USA, 106, 20610–20615, https://doi.org/10.1073/pnas.0804619106, 2009.
Marengo, J. A.: Interdecadal variability and trends of rainfall across the Amazon basin, Theor. Appl. Climatol., 78, 79–96, https://doi.org/10.1007/s00704-004-0045-8, 2004.
Marengo, J. A.: Characteristics and spatio-temporal variability of the Amazon river basin water budget, Clim. Dynam., 24, 11–22, https://doi.org/10.1007/s00382-004-0461-6, 2005.
Marengo, J. A.: On the Hydrological Cycle of the Amazon Basin: A Historical Review and Current State-of-the-art, Rev. Bras. Meteorol., 21, 1–19, 2006.
Marengo, J. A. and Espinoza, J. C.: Extreme seasonal droughts and floods in Amazonia: Causes, trends and impacts, Int. J. Climatol., 36, 1033–1050, https://doi.org/10.1002/joc.4420, 2016.
Marengo, J. A., Tomasella, J., and Uvo, C. R.: Trends in streamflow and rainfall in tropical South America: Amazonia, eastern Brazil, and northwestern Peru, J. Geophys. Res.-Atmos., 103, 1775–1783, https://doi.org/10.1029/97JD02551, 1998.
Marengo, J. A., Nobre, C. A., Tomasella, J., Oyama, M. D., Sampaio de Oliveira, G., de Oliveira, R., Camargo, H., Alves, L. M., and Brown, I. F.: The Drought of Amazonia in 2005, J. Climate, 21, 495–516, https://doi.org/10.1175/2007JCLI1600.1, 2008.
Marengo, J. A., Tomasella, J., Alves, L. M., Soares, W. R., and Rodriguez, D. A.: The drought of 2010 in the context of historical droughts in the Amazon region, Geophys. Res. Lett., 38, 1–5, https://doi.org/10.1029/2011GL047436, 2011.
Mckee, T. B., Doesken, N. J., and Kleist, J.: The relationship of drought frequency and duration to time scales, AMS 8th Conf. Appl. Climatol., (January), 179–184, 1993.
Miguez-Macho, G. and Fan, Y.: The role of groundwater in the Amazon water cycle: 1. Influence on seasonal streamflow, flooding and wetlands, J. Geophys. Res.-Atmos., 117, 1–30, https://doi.org/10.1029/2012JD017539, 2012a.
Miguez-Macho, G., and Fan, Y.: The role of groundwater in the Amazon water cycle: 2. Influence on seasonal soil moisture and evapotranspiration, J. Geophys. Res.-Atmos., 117, https://doi.org/10.1029/2012JD017540, 2012b.
Miguez-Macho, G., Li, H., and Fan, Y.: Simulated water table and soil moisture climatology over North America, B. Am. Meteorol. Soc., 89, 663–672, https://doi.org/10.1175/BAMS-89-5-663, 2008.
Monteiro, J. A. F., Strauch, M., Srinivasan, R., Abbaspour, K., and Gücker, B.: Accuracy of grid precipitation data for Brazil: Application in river discharge modelling of the Tocantins catchment, Hydrol. Process., 30, 1419–1430, https://doi.org/10.1002/hyp.10708, 2016.
Monteith, J. L.: Evaporation and environment. The state and movement of water in living organisms. Symposium of the society of experimental biology, 19, 205–234, 1965.
Moran, E. F., Lopez, M. C., Moore, N., Müller, N., and Hyndman, D. W.: Sustainable hydropower in the 21st century, P. Natl. Acad. Sci. USA, 115, 201809426, https://doi.org/10.1073/pnas.1809426115, 2018.
Muller-Karger, F. E., McClain, C. R., and Richardson, P. L.: The dispersal of the Amazon's water, Nature, 333, 56–59, https://doi.org/10.1038/332141a0, 1988.
Müller Schmied, H., Eisner, S., Franz, D., Wattenbach, M., Portmann, F. T., Flörke, M., and Döll, P.: Sensitivity of simulated global-scale freshwater fluxes and storages to input data, hydrological model structure, human water use and calibration, Hydrol. Earth Syst. Sci., 18, 3511–3538, https://doi.org/10.5194/hess-18-3511-2014, 2014.
Myneni, R. B., Yang, W., Nemani, R. R., Huete, A. R., Dickinson, R. E., Knyazikhin, Y., Didan, K., Fu, R., Negron Juarez, R. I., Saatchi, S. S., Hashimoto, H., Ichii, K., Shabanov, N. V., Tan, B., Ratana, P., Privette, J. L., Morisette, J. T., Vermote, E. F., Roy, D. P., Wolfe, R. E., Friedl, M. A., Running, S. W., Votava, P., El-Saleous, N., Devadiga, S., Su, Y., and Salomonson, V. V.: Large seasonal swings in leaf area of Amazon rainforests, P. Natl. Acad. Sci. USA, 104, 4820–4823, https://doi.org/10.1073/pnas.0611338104, 2007.
Nepstad, D. C., Stickler, C. M., Filho, B. S., and Merry, F.: Interactions among Amazon land use, forests and climate: prospects for a near-term forest tipping point, Philos. T. Roy. Soc. B, 363, 1737–1746, https://doi.org/10.1098/rstb.2007.0036, 2008.
Newbold, T., Hudson, L. N., Arnell, A. P., Contu, S., De Palma, A., Ferrier, S., Hill, S. L. L., Hoskins, A. J., Lysenko, I., Phillips, H. R. P., Burton, V. J., Chng, C. W. T., Emerson, S., Gao, D., Pask-Hale, G., Hutton, J., Jung, M., Sanchez-Ortiz, K., Simmons, B. I., Whitmee, S., Zhang, H., Scharlemann, J. P. W., and Purvis, A.: Has land use pushed terrestrial biodiversity beyond the planetary boundary?, A global assessment, Science, 353, LP-291, https://doi.org/10.1126/science.aaf2201, 2016.
Nobre, C. A., Sellers, P. J., and Shukla, J.: Amazonian Deforestation and Regional Climate Change, J. Climate, 4, 957–988, https://doi.org/10.1175/1520-0442(1991)004<0957:ADARCC>2.0.CO;2, 1991.
Paiva, R. C. D., Collischonn, W., Bonnet, M.-P., de Gonçalves, L. G. G., Calmant, S., Getirana, A., and Santos da Silva, J.: Assimilating in situ and radar altimetry data into a large-scale hydrologic-hydrodynamic model for streamflow forecast in the Amazon, Hydrol. Earth Syst. Sci., 17, 2929–2946, https://doi.org/10.5194/hess-17-2929-2013, 2013a.
Paiva, R. C. D., Buarque, D. C., Collischonn, W., Bonnet, M. P., Frappart, F., Calmant, S., and Bulhões Mendes, C. A.: Large-scale hydrologic and hydrodynamic modeling of the Amazon River basin, Water Resour. Res., 49, 1226–1243, https://doi.org/10.1002/wrcr.20067, 2013b.
Panday, P. K., Coe, M. T., Macedo, M. N., Lefebvre, P., and Castanho, A. D. de A.: Deforestation offsets water balance changes due to climate variability in the Xingu River in eastern Amazonia, J. Hydrol., 523, 822–829, https://doi.org/10.1016/j.jhydrol.2015.02.018, 2015.
Phillips, O. L., Aragão, L. E. O. C., Lewis, S. L., Fisher, J. B., Lloyd, J., López-González, G., Malhi, Y., Monteagudo, A., Peacock, J., Quesada, C. a, van der Heijden, G., Almeida, S., Amaral, I., Arroyo, L., Aymard, G., Baker, T. R., Bánki, O., Blanc, L., Bonal, D., Brando, P., Chave, J., de Oliveira, A. C. A., Cardozo, N. D., Czimczik, C. I., Feldpausch, T. R., Freitas, M. A., Gloor, E., Higuchi, N., Jiménez, E., Lloyd, G., Meir, P., Mendoza, C., Morel, A., Neill, D. a, Nepstad, D., Patiño, S., Peñuela, M. C., Prieto, A., Ramírez, F., Schwarz, M., Silva, J., Silveira, M., Thomas, A. S., Steege, H. Ter, Stropp, J., Vásquez, R., Zelazowski, P., Alvarez Dávila, E., Andelman, S., Andrade, A., Chao, K., Erwin, T., Di Fiore, A., Honorio C, E., Keeling, H., Killeen, T. J., Laurance, W. F., Peña Cruz, A., Pitman, N. C. a, Núñez Vargas, P., Ramírez-Angulo, H., Rudas, A., Salamão, R., Silva, N., Terborgh, J., Torres-Lezama, A., Heijden, G. Van Der, Cristina, Á., Oliveira, A. De, Dávila, E. A., Fiore, A. Di, C, E. H., Cruz, A. P., and Vargas, P. N.: Drought sensitivity of the Amazon rainforest, Science, 323, 1344–1347, https://doi.org/10.1126/science.1164033, 2009.
Phipps, S. J., Mcgregor, H. V., Gergis, J., Gallant, A. J. E., Neukom, R., Stevenson, S., Ackerley, D., Brown, J. R., Fischer, M. J., and Van Ommen, T. D.: Paleoclimate data-model comparison and the role of climate forcings over the past 1500 years, J. Climate, 26, 6915–6936, https://doi.org/10.1175/JCLI-D-12-00108.1, 2013.
Pielke, R. A., Cotton, W. R., Walko, R. L., Tremback, C. J., Lyons, W. A., Grasso, L. D., Nicholls, M. E., Moran, M. D., Wesley, D. A., Lee, T. J., and Copeland, J. H.: A comprehensive meteorological modeling system-RAMS, Meteorol. Atmos. Phys., 49, 69–91, https://doi.org/10.1007/BF01025401, 1992.
Pokhrel, Y., Hanasaki, N., Koirala, S., Cho, J., Yeh, P. J.-F., Kim, H., Kanae, S., and Oki, T.: Incorporating Anthropogenic Water Regulation Modules into a Land Surface Model, J. Hydrometeorol., 13, 255–269, https://doi.org/10.1175/JHM-D-11-013.1, 2012a.
Pokhrel, Y., Hanasaki, N., Yeh, P. J.-F., Yamada, T. J., Kanae, S., and Oki, T.: Model estimates of sea-level change due to anthropogenic impacts on terrestrial water storage, Nat. Geosci, 5, 389–392, https://doi.org/10.1038/ngeo1476, 2012b.
Pokhrel, Y., Shin, S., Lin, Z., Yamazaki, D., and Qi, J.: Potential Disruption of Flood Dynamics in the Lower Mekong River Basin Due to Upstream Flow Regulation, Sci. Rep., 8, 17767, https://doi.org/10.1038/s41598-018-35823-4, 2018.
Pokhrel, Y. N., Fan, Y., Miguez-Macho, G., Yeh, P. J. F., and Han, S. C.: The role of groundwater in the Amazon water cycle: 3. Influence on terrestrial water storage computations and comparison with GRACE, J. Geophys. Res.-Atmos., 118, 3233–3244, https://doi.org/10.1002/jgrd.50335, 2013.
Pokhrel, Y. N., Fan, Y., and Miguez-Macho, G.: Potential hydrologic changes in the Amazon by the end of the 21st century and the groundwater buffer, Environ. Res. Lett., 9, 084004, https://doi.org/10.1088/1748-9326/9/8/084004, 2014.
Rammig, A., Jupp, T., Thonicke, K., Tietjen, B., Heinke, J., Lucht, W., Cramer, W., Cox, P., and Jupp, T.: Estimating the risk of Amazonian forest dieback Estimating, New Phytol., 187, 694–706, https://doi.org/10.1111/j.1469-8137.2010.03318.x, 2010.
Sahoo, A. K., Pan, M., Troy, T. J., Vinukollu, R. K., Sheffield, J., and Wood, E. F.: Reconciling the global terrestrial water budget using satellite remote sensing, Remote Sens. Environ., 115, 1850–1865, https://doi.org/10.1016/j.rse.2011.03.009, 2011.
Saleska, S. R., Didan, K., Huete, A. R., and Da Rocha, H. R.: Amazon forests green-up during 2005 drought, Science, 318, 612, https://doi.org/10.1126/science.1146663, 2007.
Saleska, S. R., Wu, J., Guan, K., Araujo, A. C., Huete, A., Nobre, A. D., and Restrepo-Coupe, N.: Dry-season greening of Amazon forests, Nature, 531, 4–5, https://doi.org/10.1038/nature16457, 2016.
Satyamurty, P., Da Costa, C. P. W., Manzi, A. O., and Candido, L. A.: A quick look at the 2012 record flood in the Amazon Basin, Geophys. Res. Lett., 40, 1396–1401, https://doi.org/10.1002/grl.50245, 2013.
Scanlon, B. R., Zhang, Z., Save, H., Wiese, D. N., Landerer, F. W., Long, D., Laurent, L., and Chen, J.: Global evaluation of new GRACEmascon products for hydrologic applications, Water Resour. Res., 9412–9429, https://doi.org/10.1002/2016WR019494, 2016.
Scanlon, B. R., Zhang, Z., Save, H., Sun, A. Y., Müller Schmied, H., van Beek, L. P. H., Wiese, D. N., Wada, Y., Long, D., Reedy, R. C., Longuevergne, L., Döll, P., and Bierkens, M. F. P.: Global models underestimate large decadal declining and rising water storage trends relative to GRACE satellite data, P. Natl. Acad. Sci. USA, 115, 1080–1089, https://doi.org/10.1073/pnas.1704665115, 2018.
Schöngart, J. and Junk, W. J.: Forecasting the flood-pulse in Central Amazonia by ENSO-indices, J. Hydrol., 335, 124–132, https://doi.org/10.1016/j.jhydrol.2006.11.005, 2007.
Sena, J. A., de Deus, L. A. B., Freitas, M. A. V., and Costa, L.: Extreme Events of Droughts and Floods in Amazonia: 2005 and 2009, Water Resour. Manag., 26, 1665–1676, https://doi.org/10.1007/s11269-012-9978-3, 2012.
Shin, S., Pokhrel, Y., and Miguez-Macho, G.: High Resolution Modeling of Reservoir Release and Storage Dynamics at the Continental Scale, Water Resour. Res., 55, 787–810, doi:10.1029/2018WR023025, 2018.
Shukla, J., Nobre, C., and Sellers, P.: Amazon Deforestation and Climate Change, Science, 247, LP-1325, https://doi.org/10.1126/science.247.4948.1322, 1990.
Siqueira, V. A., Paiva, R. C. D., Fleischmann, A. S., Fan, F. M., Ruhoff, A. L., Pontes, P. R. M., Paris, A., Calmant, S., and Collischonn, W.: Toward continental hydrologic–hydrodynamic modeling in South America, Hydrol. Earth Syst. Sci., 22, 4815–4842, https://doi.org/10.5194/hess-22-4815-2018, 2018.
Smith, L. T., Aragão, L. E. O. C., Sabel, C. E., and Nakaya, T.: Drought impacts on children's respiratory health in the Brazilian Amazon, Sci. Rep., 4, 1–8, https://doi.org/10.1038/srep03726, 2014.
Soares-Filho, B., Moutinho, P., Nepstad, D., Anderson, A., Rodrigues, H., Garcia, R., Dietzsch, L., Merry, F., Bowman, M., Hissa, L., Silvestrini, R., and Maretti, C.: Role of Brazilian Amazon protected areas in climate change mitigation, P. Natl. Acad. Sci. USA, 107, 10821–10826, https://doi.org/10.1073/pnas.0913048107, 2010.
Soito, J. L. D. S., and Freitas, M. A. V.: Amazon and the expansion of hydropower in Brazil: Vulnerability, impacts and possibilities for adaptation to global climate change, Renew. Sustain. Energy Rev., 15, 3165–3177, https://doi.org/10.1016/j.rser.2011.04.006, 2011.
Sun, A. Y., Scanlon, B. R., Zhang, Z., Walling, D., Bhanja, S. N., Mukherjee, A., and Zhong, Z.: Combining Physically Based Modeling and Deep Learning for Fusing GRACE Satellite Data: Can We Learn From Mismatch?, Water Resour. Res., 55, 1179–1195, doi:10.1029/2018WR023333, 2019.
Taylor, K. E.: Summarizing multiple aspects of model performance in a single diagram, J. Geophys. Res.-Atmos., 106, 7183–7192, https://doi.org/10.1029/2000JD900719, 2001.
Timpe, K. and Kaplan, D.: The changing hydrology of a dammed Amazon, Sci. Adv., 3, 1–14, https://doi.org/10.1126/sciadv.1700611, 2017.
Tófoli, R. M., Dias, R. M., Zaia Alves, G. H., Hoeinghaus, D. J., Gomes, L. C., Baumgartner, M. T., and Agostinho, A. A.: Gold at what cost?, Another megaproject threatens biodiversity in the Amazon, Perspect. Ecol. Conserv., 15, 129–131, https://doi.org/10.1016/j.pecon.2017.06.003, 2017.
Tollefson, J.: Deforestation rates spike in Brazil, Nature, 540, 182–183, https://doi.org/10.1038/nature.2016.21083, 2016.
Toomey, M., Roberts, D. A., Still, C., Goulden, M. L., and McFadden, J. P.: Remotely sensed heat anomalies linked with Amazonian forest biomass declines, Geophys. Res. Lett., 38, L19704, doi:10.1029/2011GL049041.
Towner, J., Cloke, H. L., Zsoter, E., Flamig, Z., Hoch, J. M., Bazo, J., Coughlan de Perez, E., and Stephens, E. M.: Assessing the performance of global hydrological models for capturing peak river flows in the Amazon Basin, Hydrol. Earth Syst. Sci. Discuss., https://doi.org/10.5194/hess-2019-44, in review, 2019.
Van Loon, A. F. and Laaha, G.: Hydrological drought severity explained by climate and catchment characteristics, J. Hydrol., 526, 3–14, https://doi.org/10.1016/j.jhydrol.2014.10.059, 2015.
Van Loon, A. F., Van Huijgevoort, M. H. J., and Van Lanen, H. A. J.: Evaluation of drought propagation in an ensemble mean of large-scale hydrological models, Hydrol. Earth Syst. Sci., 16, 4057–4078, https://doi.org/10.5194/hess-16-4057-2012, 2012.
Vorosmarty, C. J., Willmott, C. J., Choudhury, B. J., Schloss, A. L., Stearns, T. K., Robeson, S. M., and Dorman, T. J.: Analyzing the discharge regime of a large tropical river through remote sensing , ground-based climatic data , and modeling differences (HVPTD) from the 37-GHz scanning multichannel microwave radiometer model (WBM/WTM), Monthly, Water Resour. Res., 32, 3137–3150, https://doi.org/10.1029/96WR01333, 1996.
Walko, R. L., Band, L. E., Baron, J., Kittel, T. G. F., Lammers, R., Lee, T. J., Ojima, D., Pielke, R. A., Taylor, C., Tague, C., Tremback, C. J., and Vidale, P. L.: Coupled atmosphere, biophysics and hydrology models for environmental modeling, J. Appl. Meteorol., 39, 931–944, https://doi.org/10.1175/1520-0450(2000)039<0931:CABHMF>2.0.CO;2, 2000.
Wanders, N. and Van Lanen, H. A. J.: Future discharge drought across climate regions around the world modelled with a synthetic hydrological modelling approach forced by three general circulation models, Nat. Hazards Earth Syst. Sci., 15, 487–504, https://doi.org/10.5194/nhess-15-487-2015, 2015.
Wanders, N. and Wada, Y.: Human and climate impacts on the 21st century hydrological drought, J. Hydrol., 526, 208–220, https://doi.org/10.1016/j.jhydrol.2014.10.047, 2015.
Wanders, N., Wada, Y., and Van Lanen, H. A. J.: Global hydrological droughts in the 21st century under a changing hydrological regime, Earth Syst. Dynam., 6, 1–15, https://doi.org/10.5194/esd-6-1-2015, 2015.
Wang, K., Shi, H., Chen, J., and Li, T.: An improved operation-based reservoir scheme integrated with Variable Infiltration Capacity model for multiyear and multipurpose reservoirs, J. Hydrol., 571, 365–375, https://doi.org/10.1016/j.jhydrol.2019.02.006, 2019.
Weedon, G. P., Balsamo, G., Bellouin, N., Gomes, S., Best, M. J., and Viterbo, P.: Data methodology applied to ERA-Interim reanalysis data, Water Resour. Res., 50, 7505–7514, https://doi.org/10.1002/2014WR015638, 2014.
Winemiller, K. O., McIntyre, P. B., Castello, L., Fluet-Chouinard, E., Giarrizzo, T., Nam, S., Baird, I. G., Darwall, W., Lujan, N. K., Harrison, I., Stiassny, M. L. J. J., Silvano, R. A. M. M., Fitzgerald, D. B., Pelicice, F. M., Agostinho, A. A., Gomes, L. C., Albert, J. S., Baran, E., Petrere, M., Zarfl, C., Mulligan, M., Sullivan, J. P., Arantes, C. C., Sousa, L. M., Koning, A. A., Hoeinghaus, D. J., Sabaj, M., Lundberg, J. G., Armbruster, J., Thieme, M. L., Petry, P., Zuanon, J., Vilara, G. T., Snoeks, J., Ou, C., Rainboth, W., Pavanelli, C. S., Akama, A., Soesbergen, A. v., Saenz, L., Torrente Vilara, G., Snoeks, J., Ou, C., Rainboth, W., Pavanelli, C. S., Akama, A., Van Soesbergen, A., and Sáenz, L.: Balancing hydropower and biodiversity in the Amazon, Congo, and Mekong, Science,, 351, 128–129, https://doi.org/10.1126/science.aac7082, 2016.
Wongchuig Correa, S., Paiva, R. C. D., de Espinoza, J. C., and Collischonn, W.: Multi-decadal Hydrological Retrospective: Case study of Amazon floods and droughts, J. Hydrol., 549, 667–684, https://doi.org/10.1016/j.jhydrol.2017.04.019, 2017.
Xavier, L., Becker, M., Cazenave, A., Longuevergne, L., Llovel, W., and Filho, O. C. R.: Interannual variability in water storage over 2003-2008 in the Amazon Basin from GRACE space gravimetry, in situ river level and precipitation data, Remote Sens. Environ., 114, 1629–1637, https://doi.org/10.1016/j.rse.2010.02.005, 2010.
Xiao, Z., Liang, S., Wang, J., Chen, P., Yin, X., Zhang, L., and Song, J.: Use of general regression neural networks for generating the GLASS leaf area index product from time-series MODIS surface reflectance, IEEE Trans. Geosci. Remote Sens., 52, 209–223, https://doi.org/10.1109/TGRS.2013.2237780, 2014.
Xu, B., Park, T., Yan, K., Chen, C., Zeng, Y., Song, W., Yin, G., Li, J., Liu, Q., Knyazikhin, Y., and Myneni, B. R.: Analysis of Global LAI/FPAR Products from VIIRS and MODIS Sensors for Spatio-Temporal Consistency and Uncertainty from 2012–-2016, For., 9, 73, doi:10.3390/f9020073, 2018.
Xu, L., Samanta, A., Costa, M. H., Ganguly, S., Nemani, R. R., and Myneni, R. B.: Widespread decline in greenness of Amazonian vegetation due to the 2010 drought, Geophys. Res. Lett., 38, 2–5, https://doi.org/10.1029/2011GL046824, 2011.
Yamazaki, D., Kanae, S., Kim, H., and Oki, T.: A physically based description of floodplain inundation dynamics in a global river routing model, Water Resour. Res., 47, 1–21, https://doi.org/10.1029/2010WR009726, 2011.
Yamazaki, D., Baugh, C. A., Bates, P. D., Kanae, S., Alsdorf, D. E., and Oki, T.: Adjustment of a spaceborne DEM for use in floodplain hydrodynamic modeling, J. Hydrol., 436, 81–91, https://doi.org/10.1016/j.jhydrol.2012.02.045, 2012.
Zeng, N.: Seasonal cycle and interannual variability in the Amazon hydrologic cycle, J. Geophys. Res.-Atmos., 104, 9097–9106, https://doi.org/10.1029/1998JD200088, 1999.
Zeng, N., Yoon, J.-H., Marengo, J. A., Subramaniam, A., Nobre, C. A., Mariotti, A. and Neelin, J. D.: Causes and impacts of the 2005 Amazon drought, Environ. Res. Lett., 3, 14002, doi:10.1088/1748-9326/3/1/014002, 2008.
Zhao, M., A, G., Velicogna, I., and Kimball, J. S.: A Global Gridded Dataset of GRACE Drought Severity Index for 2002–14: Comparison with PDSI and SPEI and a Case Study of the Australia Millennium Drought, J. Hydrometeorol., 18, 2117–2129, https://doi.org/10.1175/JHM-D-16-0182.1, 2017a.
Zhao, M., Geruo, A., Velicogna, I., and Kimball, J. S.: Satellite observations of regional drought severity in the continental United States using GRACE-based terrestrial water storage changes, J. Climate, 30, 6297–6308, https://doi.org/10.1175/JCLI-D-16-0458.1, 2017b.
Zulkafli, Z., Buytaert, W., Manz, B., Rosas, C. V., Willems, P., Lavado-Casimiro, W., Guyot, J.-L., and Santini, W.: Projected increases in the annual flood pulse of the Western Amazon, Environ. Res. Lett., 11, 14013, doi:10.1088/1748-9326/11/1/014013, 2016.