the Creative Commons Attribution 4.0 License.
the Creative Commons Attribution 4.0 License.
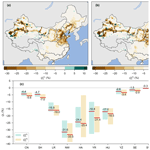
Multimodel assessments of human and climate impacts on mean annual streamflow in China
Xingcai Liu
Wenfeng Liu
Hong Yang
Martina Flörke
Yoshimitsu Masaki
Hannes Müller Schmied
Sebastian Ostberg
Yadu Pokhrel
Yusuke Satoh
Yoshihide Wada
Human activities, as well as climate variability, have had increasing impacts on natural hydrological systems, particularly streamflow. However, quantitative assessments of these impacts are lacking on large scales. In this study, we use the simulations from six global hydrological models driven by three meteorological forcings to investigate direct human impact (DHI) and climate impact on streamflow in China. Results show that, in the sub-periods of 1971–1990 and 1991–2010, one-fifth to one-third of mean annual streamflow (MAF) was reduced due to DHI in northern basins, and much smaller (<4 %) MAF was reduced in southern basins. From 1971–1990 to 1991–2010, total MAF changes range from −13 % to 10 % across basins wherein the relative contributions of DHI change and climate variability show distinct spatial patterns. DHI change caused decreases in MAF in 70 % of river segments, but climate variability dominated the total MAF changes in 88 % of river segments of China. In most northern basins, climate variability results in changes of −9 % to 18 % in MAF, while DHI change results in decreases of 2 % to 8 % in MAF. In contrast with the climate variability that may increase or decrease streamflow, DHI change almost always contributes to decreases in MAF over time, with water withdrawals supposedly being the major impact on streamflow. This quantitative assessment can be a reference for attribution of streamflow changes at large scales, despite remaining uncertainty. We highlight the significant DHI in northern basins and the necessity to modulate DHI through improved water management towards a better adaptation to future climate change.
- Article
(5949 KB) - Full-text XML
-
Supplement
(1204 KB) - BibTeX
- EndNote
Human activities have remarkably intensified and significantly altered hydrological regimes and water resources worldwide (Oki and Kanae, 2006; Döll et al., 2009; Tang and Oki, 2016). They have been reported to have aggravated hydrological drought and impaired hydrological resilience in many regions (Wada et al., 2013; Wada and Heinrich, 2013; Veldkamp et al., 2017). Human impact (here we only consider the direct human impact – DHI, e.g., impact caused by the construction and management of dams and reservoirs, water withdrawal from surface water, groundwater pumping, etc.) on streamflow has been on the rise across the world (Jaramillo and Destouni, 2015), causing the same order of magnitude of hydrologic alterations as that by climate change and variability in some regions (Ian and Reed, 2012; Haddeland et al., 2014; Zhou et al., 2015). As such, there has been increased attention in attributing hydrological impacts from various drivers (Patterson et al., 2013; Tan and Gan, 2015; Bosmans et al., 2017). Understanding the relative contributions of DHI to streamflow changes is of great importance for climate change adaptation and sustainable development (Yin et al., 2017).
In China, the hydrological system is experiencing significant changes induced by both climate and human impacts (Piao et al., 2010; Tang et al., 2013; Liu et al., 2014; Wada et al., 2017). Great efforts have been made to quantify the relative contributions of DHI in China (Liu and Du, 2017). Some studies have shown that DHI outweighed climatic impact on streamflow and runoff in several small catchments in the Hai River (Wang et al., 2009, 2013b) and the Yellow River (Li et al., 2007; Tang et al., 2008; Zhan et al., 2014; Chang et al., 2016). Other studies have reported that the construction and operation of the Three Gorges reservoir resulted in considerable changes in streamflow (Wang et al., 2013a), but DHI contributed to small changes in streamflow in some catchments (Liu et al., 2012; Ye et al., 2013) and slight changes in lake areas in the Yangtze River basin (Wang et al., 2017). Most of these studies attributed human impact by comparing observed streamflow to simulations which were estimated with a climate elasticity approach based on the Budyko framework (Zhang et al., 2001) or with hydrological models (Wang et al., 2009, 2010; Yuan et al., 2018). These assessments largely relied on hydroclimatic observations and were performed on relatively small catchment scales to obtain quantitatively distinguishable attributions. The previous studies assessed DHI on streamflow changes at the outlets of catchments, but the spatial extents of the impacts have not been adequately examined. As mentioned above, many previous studies reported large DHI on streamflow; however, a recent large-scale assessment over the United States and Canada showed that human activities such as water management did not substantially alter the hydrological effects of climate change (Ficklin et al., 2018). In addition, the potential uncertainty associated with DHI and streamflow simulations can hardly be estimated from a single model assessment, as done in previous studies. Therefore, an improved assessment with larger spatial coverage and by employing a multimodel comparison approach is essential for understanding regional difference and the associated uncertainty of the impacts.
The recent development of human impact parameterizations in hydrological models has facilitated the assessment of the DHI on streamflow (Pokhrel et al., 2016; Liu et al., 2017b; Veldkamp et al., 2018). Consequently, several global hydrological modeling initiatives considering human impact have been undertaken, e.g., by the Inter-Sectoral Impact Model Intercomparison Project phase 2a (ISIMIP2a; Gosling et al., 2017). Under the ISIMIP2a framework, retrospective simulations of hydrological changes were performed for both natural conditions and those with human activities by six global hydrological models (GHMs). The simulations provide a basis for quantifying the streamflow changes caused by various drivers in a consistent manner on large scales. Meanwhile, the grid-based simulations allow an attribution at different geographic levels and, therefore, provide more detailed information about regional streamflow changes. The ISIMIP2a simulations have included the most important DHI at large scales, including the operation of reservoirs and dams on rivers as well as sectoral water withdrawals for irrigation, industry, domestic use, and livestock. In this study, using the ISIMIP2a multimodel simulations, we quantify the relative contribution of DHI and climate variability on streamflow changes in the major river basins in China at a decadal timescale during the 1971–2010 period. This is the first study to focus on performing such a quantitative assessment for all rivers of China with comparable modeling experiments. This study can serve as a reference for attribution of streamflow changes at large scales that can facilitate regional water resource management under climate change and growing human impact on freshwater system.
2.1 Simulation data
In this study, we use the simulations of monthly streamflow of China produced by six GHMs, namely DBH (Tang et al., 2007, 2008; Liu et al., 2016), H08 (Hanasaki et al., 2008a, b), LPJmL (Bondeau et al., 2007; Rost et al., 2008; Biemans et al., 2011; Schaphoff et al., 2013), MATSIRO (Takata et al., 2003; Pokhrel et al., 2015), PCR-GLOBWB (Wada et al., 2014), and WaterGAP2 (Flörke et al., 2013; Müller Schmied et al., 2014, 2016). Two experiments, i.e., simulations with (VARSOC) and without (NOSOC) human impact, were performed at a half-degree spatial resolution for the 1971–2010 period by using the six GHMs following the ISIMIP2a simulation protocol (https://www.isimip.org/protocol/\#isimip2a, last access: 18 February 2019). All the model runs used the same river routing map (DDM30; Döll and Lehner, 2002). For both experiments, the GHMs were forced by three global meteorological forcing products (GMFs), i.e., the PGMFD v.2 (Princeton; Sheffield et al., 2006), GSWP3 (http://hydro.iis.u-tokyo.ac.jp/GSWP3/, last access: 18 February 2019), and a combination of WFD (until 1978; Weedon et al., 2011) and WFDEI (from 1979 onwards; Weedon et al., 2014) datasets. Ensembles of annual streamflow are derived from the simulations of NOSOC (referred to as Qn) and VARSOC (referred to as Qv) experiments, respectively, for river segments (here a grid cell is treated as a river segment regardless of the cases where a grid cell contains several small river segments) which are then spatially averaged for individual basins. Long-term mean annual streamflow (MAF) in each river segment is calculated for both NOSOC and VARSOC simulations over a specific period (see Sect. 2.3) and is then spatially averaged over individual basins for each ensemble member. In addition to streamflow, total runoff from NOSOC and VARSOC simulations and water withdrawals from VARSOC simulations are also derived at grid cells and individual basins for associated analyses. The simulations may have large uncertainties over the Tibetan Plateau because long-term meteorological and streamflow observations are sparse in this region (Zhang et al., 2017) and the modeling of glacier melting is absent in most of the models. Therefore, the simulation data in the Tibetan Plateau region are removed and are not included in spatial averages by masking the grid cells with altitudes higher than 4000 m in all analyses.
Human impact considered in the VARSOC experiment (see the maps in Fig. S1 and Table S1 in the Supplement for more details) includes the time-varying areas for both irrigated and rain-fed cropland (Fader et al., 2010; Portmann et al., 2010) and reservoirs (dams) from the Global Reservoir and Dam (GRanD) database (Lehner et al., 2011) including their commissioning year (see Fig. S1 and Table S1). Reservoir regulation was considered in the VARSOC experiment, which often reduces high streamflow in high-flow seasons and increases streamflow in dry seasons (Masaki, et al., 2017). Interbasin water transfer was not considered in any of the model runs. The simulations of water withdrawals are different between the GHMs with respect to water use requirements and water withdrawal sources which are shown in Table S1. The sources of water withdrawals, depending on models, may include river channel, reservoirs, groundwater, and lakes, and their fractions may be determined from reported statistics (e.g., Siebert et al., 2010) or estimated in models (Wada et al., 2014). In addition to the irrigation water requirement which is usually estimated by coupling crop models, most GHMs considered the requirements for domestic and industrial water use that were prescribed in H08 (Hanasaki et al., 2008a), LPJmL, and MATSIRO (Pokhrel et al., 2015) or were estimated according to the population; socioeconomic and technological development in PCR-GLOBWB (Wada et al., 2014); and the population, thermal electricity production, gross added value, and technological change in WaterGAP (Flörke et al., 2013). The water use requirement for livestock was also prescribed in the LPJmL model and estimated according to livestock densities in PCR-GLOBWB and WaterGAP2.
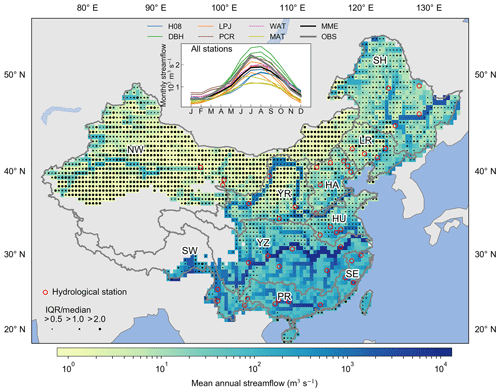
Figure 1Multimodel medians of mean annual streamflow (MAF) in China from the VARSOC experiment. MAF medians are computed across 18 GHM–GMF combinations over the 1971–2000 period. The ensemble spread is represented by the ratio of interquartile range (IQR; 75th percentile minus 25th percentile) to the ensemble median of MAF (median). The hydrological stations used in this study are identified by red circles. The inner plot shows the comparison of the simulated seasonal streamflow (each GHM has three lines for the three GMFs) from the VARSOC experiment against the observations averaged for all the hydrological stations shown on the map over the period 1971–2000. H08, DBH, LPJ, PCR, WAT, and MAT denote the H08, DBH, LPJmL, PCR-GLOBWB, WaterGAP2, and MATSIRO models, respectively. MME denotes the multimodel ensemble median, and OBS denotes observation. The Tibetan Plateau region is masked by removing the grid cells with an altitude higher than 4000 m, and the same applies in subsequent figures. The 10 major basins in China are labeled and are indicated with grey lines. The southern basins include the Yangtze River (YZ), southwestern rivers (SW), southeastern rivers (SE), and Pearl River (PR), the northern basins include the Songhua River (SH), Liao River (LR), northwestern rivers (NW), Hai River (HA), Yellow River (YR), and Huai River (HU).
2.2 Observed monthly streamflow and reported water withdrawals
The ISIMIP2a streamflow simulations have been extensively validated with observations over the world in several studies (Liu et al., 2017b; Veldkamp et al., 2018; Zaherpour et al., 2018) but were not fully evaluated in China due to limited observations, particularly for the water withdrawals. Therefore, before the quantitative attribution, an evaluation of the multimodel simulations is performed, which may add confidence regarding the GHM performance over China. Observations of monthly streamflow from 44 hydrological stations in China (Fig. 1) during 1971–2000 are used for model validation. The observations since 2001 are not available in this study. Some stations are relocated on the map to reconcile the catchment areas of the stations and the accumulative flow areas of corresponding gird cells from the DDM30 river network. After relocation, the differences are mostly less than 10 % (about 50 % at five stations) between the reported catchment areas of stations and the accumulative flow areas from the DDM30 river network. Annual water withdrawals in individual basins for the years 1980, 1985, 1990, 1995, and 1997–2010 were collected from China Water Resources Bulletin from the Ministry of Water Resources (MWR) of China (http://www.mwr.gov.cn/sj/tjgb/szygb/, last access: 18 February 2019).
2.3 Streamflow changes and attribution
The study period is evenly split into two sub-periods (P1 for 1971–1990 and P2 for 1991–2010). The DHI-induced MAF changes over time is calculated as
where and denote MAF changes (%) induced by DHI during the sub-periods P1 and P2, respectively; and denote MAF from the VARSOC experiment for the two sub-periods, respectively; and and denote MAF from the NOSOC experiment for the two sub-periods, respectively.
The contribution of DHI change (corresponding to climate variability) on streamflow changes between the two sub-periods is also examined. MAF difference between the two periods in the VARSOC experiment is defined as the total MAF changes (ΔQa) caused by both climate variability and DHI change from P1 to P2, which is expressed as a percentage of the MAF of the first sub-period P1:
The difference between the two periods in the NOSOC experiment is defined as streamflow changes induced by only climate variability (ΔQc) and expressed as a percentage of in order to be comparable with ΔQa:
The difference between ΔQa and ΔQc then counts as MAF changes induced by DHI change (ΔQh) between the two sub-periods:
Unless otherwise stated, ΔQa, ΔQc, and ΔQh are relative changes (%) with respect to in this paper.
To address the potential uncertainty resulting from the use of sub-periods, similar analyses are performed for three different sub-periods, namely 1981–1990, 1991–2000, and 2001–2010, with comparison to the sub-period 1971–1980. For these analyses, MAF is calculated over each decade.
In addition to streamflow, changes in water withdrawals and total runoff between the two sup-periods are also analyzed to explore their links with MAF changes.
2.4 Multimodel ensemble
Ensemble medians across the 18 GHM-GMF combinations (six GHMs and three GMFs) are used for analyses of streamflow and runoff. But 12 ensemble members are used for water withdrawals because only four GHMs (H08, LPJmL, PCR-GLOBWB, and MATSIRO) provide related output for the ISIMIP2a simulations. The interquartile range (IQR), i.e., the range between 25th and 75th percentiles, is calculated to present the spread across multimodel ensembles. The ratio of IQR to the median is used to measure the uncertainty in multimodel simulations of streamflow, which is comparable across regions.
3.1 Evaluation of multimodel simulations
In this study, the northern basins refer to the Songhua River (SH), Liao River (LR), northwestern rivers (NW), Hai River (HA), Yellow River (YR), and Huai River (HU); the southern basins refer to the Yangtze River (YZ), southeastern rivers (SE), southwestern rivers (SW), and Pearl River (PR; Fig. 1). The ensemble medians of MAF at grid cells over the 1971–2000 period from the VARSOC experiment show distinct spatial pattern of high streamflow in southern basins and relatively low streamflow in northern basins (Fig. 1). The multimodel simulations show larger spreads in the northern basins. The ratios of IQR ∕ median are larger than 1 or 2 in the northwestern basin, the Yellow River basin and Liao River basin. Smaller spread (IQR ∕ median less than 0.5) is found in the middle and lower reaches of the Yangtze River basin, the Pearl River basin, and the southeastern basin.
The inner plot shows the comparison between observed seasonal streamflow averaged across all hydrological stations and the averaged simulations in all river segments identified by stations over the 1971–2000 period. The ensemble medians of seasonal cycle generally coincide with the observations. However, there are large variations across all model ensembles, with some of them deviating from observations. It should be noted that the stations are located at different reaches of individual basins. Thus, the station-averaged estimates are largely dominated by those with large streamflow (e.g., at the lower reaches). Additionally, the coverage of stations used is relatively small (due to data availability), especially in hydrologically variable regions like in the northwestern rivers, leading to an evaluation of the performance of the GHMs in the whole basin that is not necessarily representative. The model spreads in the ensembles of seasonal streamflow in the northern basins are relatively larger than those in the southern basins (see Fig. S2 for each basin). Comparison between the simulated and observed annual streamflow (Fig. S3) shows similar patterns to the seasonal streamflow with respect the discrepancies between northern and southern basins. The Nash–Sutcliffe coefficient was calculated for the multimodel median and observed monthly streamflow at each station (see Table S2), which shows that the multimodel medians have better performance in the southern basins. This evaluation indicates that the multimodel simulations have relatively poor performance in northern basins and most stations with low Nash–Sutcliffe coefficients have smaller streamflow (e.g., in dry areas or upper reaches). The large spreads between models underline the necessity of using ensemble medians rather than individual models for the attribution of streamflow changes.
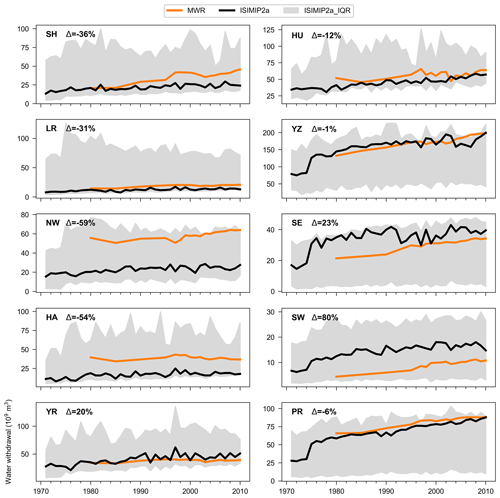
Figure 2Reported and simulated water withdrawals in the 10 basins of China. ISIMIP2a indicates the simulated water withdrawals from the ISIMIP2a VARSOC experiment (see Table S1 for details) during 1971–2010; MWR indicates the water withdrawals reported by the Ministry of Water Resources (MWR) of China for the years 1980, 1985, 1990, 1995, and 1997–2010. Δ indicates the difference between simulations and reported data. Shaded areas denote the IQR of ISIMIP2a simulations. The basin names labeled in each panel are corresponding to the basins in Fig. 1.
Compared to the reported data by the MWR of China, the ensemble medians from ISIMIP2a simulations underestimated water withdrawals in most northern basins except for the Yellow River (Fig. 2). The simulations underestimate water withdrawals by more than 50 % in the northwestern rivers and the Hai River and by more than 30 % in the Songhua River and Liao River. The simulated water withdrawals are 12 % less than reported data in the Huai River. In the Yellow River and the southeastern rivers, water withdrawals are overestimated by 20 % or more. The overestimation of water withdrawals is the largest (80 %) in the southwestern rivers. Small differences between simulations and reported data are found in the Yangtze River (−1 %) and the Pearl River (−6 %). The large deviations in the multimodel simulations of water withdrawals could make the modeling of streamflow more challenging (Döll et al., 2016; Wada et al., 2017).
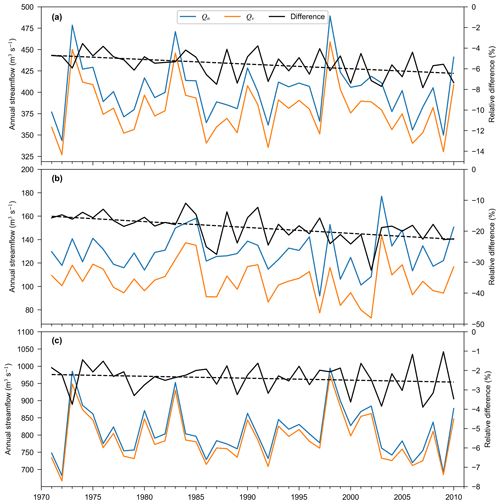
Figure 3Spatially averaged annual streamflows (m3 s−1) from NOSOC and VARSOC experiments and their differences (%) during the 1971–2010 period. (a) Average of ensemble medians of annual streamflow from NOSOC (Qn) and VARSOC (Qv) for China, (b) for the northern basins, and (c) for the southern basins. The northern and southern basins are described in Fig. 1. The dashed lines denote the linear trend of the relative differences.
3.2 Annual streamflow and DHI-induced streamflow change
Figure 3 shows the spatially averaged ensemble medians of Qn and Qv over China, the northern basins and the southern basins, respectively. Qn and Qv show considerable annual variations and no statistically significant trends over the 1971–2010 period. The relative differences between Qn and Qv over China range from −8 % to −4 % and show a statistically significant downward trend over the study period (Fig. 3a). The differences between Qn and Qv over the northern basins are larger than those for the southern basins. The absolute differences (not shown here) are −37 to −14 (m3 s−1) for the northern basins and are −37 to −7 (m3 s−1) for the southern basins. The relative differences for the northern basins (Fig. 3b) are also larger than those for the southern basins (Fig. 3c). The former ranges from −30 % to −10 %, while the latter ranges from −4 % to −1 %. A statistically significant downward trend is found in the relative differences for the northern basins, while a non-significant downward trend is found for the southern basins. The downward trend in the differences indicates that annual streamflow has been increasingly affected by human impact.
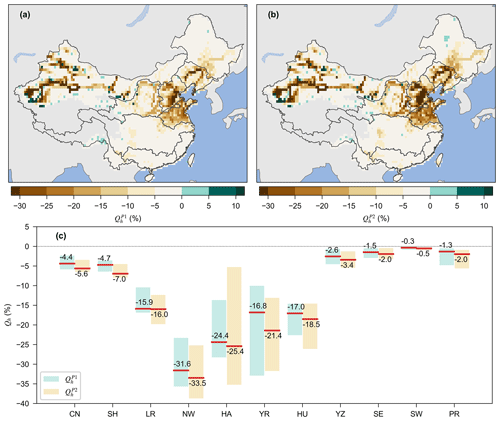
Figure 4Long-term MAF altered by DHI. Ensemble medians of long-term MAF altered by DHI in (a) the sub-period 1971–1990 () and (b) the sub-period 1991–2010 (), and (c) ensemble medians and ranges of averaged long-term MAF altered by DHI for each basin and China (denoted by CN). In plot (c), the range indicates the 25th and 75th values, and the numbers indicate the median values from all ensemble members.
3.3 MAF altered by DHI in the two sub-periods
Considerable decreases in long-term MAF are induced by DHI in the two sub-periods (Fig. 4a and b for and , respectively) in most northern basins. About 3 % and 4 % of total river segments in China show large negative values (i.e., less than −30 %) of and , respectively, which are mostly found in some parts of the northwestern rivers and the North China Plain. and are negative for more than 90 % of the river segments and range from −5 % to 0 in more than 60 % of the river segments of China. The magnitudes of the basin-averaged and are larger than 10 % in northern basins except for the Songhua River (Fig. 4c). The magnitudes of are larger than for all basins, especially in the Yellow River. The northwestern rivers show the largest negative values of and (−31.6 % and −33.5 %, respectively), which is followed by the Hai River (−24 % and −25 %), the Yellow River (−17 % and −21 %), and the Huai River (−17 % and −19 %). DHI induced slight decreases in MAF (−3.4 % to −0.3 %) in the southern basins. Overall, DHI altered MAF by −4.4 % and −5.6 % in China during the sub-periods P1 and P2, respectively.
3.4 MAF changes induced by DHI change and climate variability between the two sub-periods
The MAF changes induced by DHI change and climate variability between the two sub-periods are shown in Fig. 5. In general, total MAF changes (ΔQa; Fig. 5a) are larger in northern basins, except the Songhua River, than in southern basins. Compared to the first sub-period, in the second sub-period MAF increased by more than 30 % in many river segments of the northwestern rivers and increased by more than 5 % in large parts of the Huai River, which may be due to the return flow from water withdrawals. MAF increases are also found in considerable areas of southern basins such as the Yangtze River and the southwestern rivers. MAF decreases are found in most river segments in the Yellow River, the Hai River, and the Liao River. Significant negative values of ΔQa (less than −20 %) are found in some river segments in the upper reaches of the southwestern rivers and some parts of the northwestern rivers. The total MAF decreased by more than 10 % ( %) and increased by more than 10 % (ΔQa>10 %) in about 24 % and 17 % of river segments of China, respectively.
MAF changes induced by climate variability between the two sub-periods (ΔQc; Fig. 5b) have very similar spatial patterns to ΔQa (Fig. 5a). This indicates that climate impact dominates MAF changes during the two sub-periods. The magnitudes of ΔQc are relatively smaller than those of ΔQa in the Hai River and the Yellow River but are larger in the northwestern parts of the northwestern rivers. MAF changes induced by DHI change (ΔQh; Fig. 5c) are generally large and negative in northern basins. A decrease larger than 10 % in the MAF induced by DHI is found in some segments of the northwestern rivers and the lower reaches of the Huai River, the Hai River, and the Liao River. Positive values of ΔQh are small and are mostly found in southern river segments. Climate impact dominated MAF changes in most river segments (88 %) of China (Fig. 5d). Only 12 % of river segments show MAF changes that are mainly caused by DHI change, which are mostly in the northern basins.
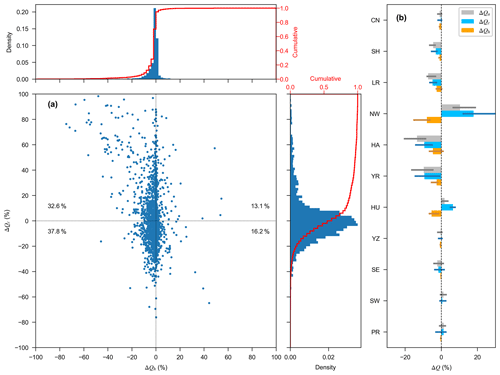
Figure 6Relative MAF changes for river segments and basins. (a) Ensemble medians of MAF changes induced by climate change (ΔQc) versus those induced by DHI change (ΔQh) for river segments of China. Data points in (a) denote the values for individual river segments; the right histogram and the top histogram show the distributions of ΔQc and ΔQh, respectively. The numbers are the proportions of data points in each quadrant. (b) Spatially aggregated ensemble medians of total MAF changes (ΔQa), MAF changes induced by climate change (ΔQc), and MAF changes induced by DHI change (ΔQh) for individual basins and China; the error bars indicate the IQR in each basin.
MAF changes induced by climate variability (ΔQc) versus those induced by DHI change (ΔQh) for all river segments are shown in Fig. 6a. Note that very few river segments with values of ΔQc (0.9 % of total river segments) and ΔQh (0.4 %) beyond [] are not shown in the figure. Magnitudes of ΔQc are much larger than those of ΔQh. The latter ranges −5 % to 5 % in most (∼ 81 %) river segments. ΔQh is less than −10 % in only about 7 % of river segments of China, while even fewer (∼ 3 %) segments show ΔQh values larger than 5 %. The values of ΔQc range from −10 % to 10 % in more than half of river segments and range from −20 % to 20 % in nearly 80 % of river segments (see Table S3 for related numbers). ΔQh is negative in 70 % of river segments, while negative values of ΔQc are found in more than half of the river segments of China (see the percentage numbers in Fig. 6a and Table S3).
The total MAF spatially averaged over China decreased by only 1 % from the first sub-period to second sub-period (Fig. 6b; see also Tables S4–S6 for more details of spatially aggregated ensemble members and medians of ΔQa, ΔQc, and ΔQh in basins). At the basin scale, the magnitudes of MAF changes are usually very small (less than 2 %) in southern basins and are relatively large in northern basins (5 % to 13 %). ΔQa in the Hai River shows the largest decrease of 13 %, which is followed by a nearly 10 % decrease in the Yellow River and a 7 % decrease in the Liao River. Increases in total MAF are found in the northwestern rivers (10 %), the Huai River (1.8 %), the Pearl River (1.3 %), and the southwestern rivers (1.2 %), which are consistent with the spatial patterns shown in Fig. 5a. DHI change causes decreases in MAF (negative ΔQh) in all the basins, resulting in a larger decrease or a smaller increase in ΔQa compared to ΔQc. The largest negative values of ΔQh are found in the northwestern rivers (−8 %), the Huai River (−5.4 %), and the Hai River (−4.6 %; see Tables S4–S6). ΔQh is about −2.6 % for the Liao River and the Yellow River. ΔQh is only about −0.7 % to −0.07 % in southern basins. The increase of MAF induced by climate variability (ΔQc) is the largest in the northwestern rivers (18 %), followed by the Huai River (6 %) and the Pearl River (1 %), and climate variability caused a nearly 9 % decrease in MAF in the Hai River and the Yellow River.
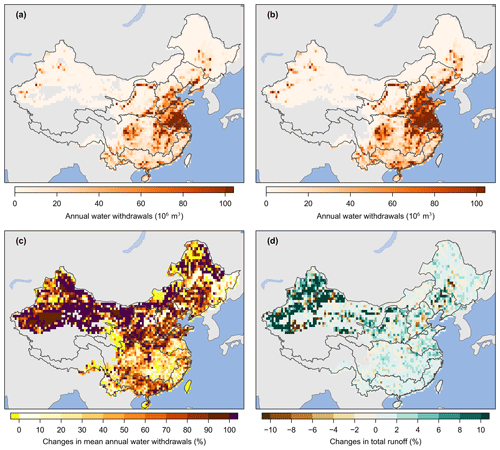
Figure 7Changes in water withdrawals and total runoff between the two sub-periods. (a) Ensemble medians of mean annual water withdrawals over the 1971–1990 period. (b) Ensemble medians of mean annual water withdrawals over 1991–2010 period. (c) Ensemble medians of the changes in mean annual water withdrawals. (d) Ensemble medians of the changes in mean annual total runoff.
3.5 Water withdrawal and its changes between the two sub-periods
For both sub-periods, the estimates of long-term mean annual water withdrawals are large (more than 100 × 106 m3 yr−1) in many areas of the Huai River, the Hai River, and the Yellow River (Fig. 7a). Large water withdrawals are also found in some lower reaches of the Yangtze River. In these regions, mean annual water withdrawals are usually larger in the lower reaches compared to the upper reaches and significantly increased from 1971–1990 to 1991–2010. The relative changes in water withdrawals between the two sub-periods show distinct spatial patterns from northern to southern basins and generally increased at all river segments of China (Fig. 7c). The spatial patterns of changes in water withdrawals resemble those of ΔQh, with large values in the Huai River, the Hai River, and the Yellow River, but they are relatively smaller in the northwestern rivers. Similar analysis is performed for changes in total runoff to examine its linkage with streamflow changes. The spatial patterns of changes in total runoff induced by DHI change between the two sub-periods (Fig. 7d) are different from that of ΔQh (Fig. 5c). Total runoff changes are positive in most areas of China due to increasing irrigation water (from both local and external sources) which partly becomes return flow, especially in the northwestern rivers. Large changes are also found in upper and middle reaches in the Yellow River, the Liao River, and the Hai River. The magnitude of changes is less than the that induced by climate variability (not shown here), which is similar as Fig. 5d. This indicates that the runoff changes are less linked to streamflow changes in the study period.
The simulated streamflow in China from the ISIMIP2a VARSOC experiment (i.e., simulations with consideration to DHI) is validated against observed streamflow from 44 hydrological stations. While the multimodel ensemble medians match well with observations, the evaluation indicates that the individual simulations of streamflow are subject to considerable uncertainties among models which are especially pronounced in northern basins as indicated by the ratio of the interquartile range to the median. The simulations of water withdrawals show large deviations from the reported data in many basins, which partly affects the performance of GHMs in streamflow simulations. It should be noted that the overestimation or underestimation of streamflow at these stations does not necessarily indicate the performance of GHMs in the whole basins because of limited stations used in this study.
Simulated annual streamflow has been increasingly affected by human impact, which is more significant in northern basins. Using the multimodel ensemble medians of streamflow, we quantify the DHI on the long-term MAF during two sub-periods, 1971–1990 and 1991–2010, and the long-term MAF changes induced by changes in DHI and climate between the two sub-periods. DHI often results in decreased streamflow in China, particularly in northern rivers, through water withdrawals, while resulting in increased runoff due to return flow from irrigation. Potential implications of the distinct spatial patterns of DHI and its change on streamflow and the associated uncertainties in current assessment are discussed as follows.
4.1 DHI considerably altered streamflow in northern basins
DHI causes MAF decreases in both of the sub-periods. At the basin level, DHI resulted in decreases by one-fifth to one-third of the long-term MAF based on Qn in northern basins and slightly altered MAF in southern basins of China. The spatial patterns of the MAF altered by DHI (Qh) are generally in accordance with those reported by previous studies (Liu and Du, 2017) and are very close to those of irrigated areas of China (see Fig. S1). The expansion of agriculture and enhanced irrigation and food demands should be the main reason for the large DHI on streamflow in northern basins (Liu et al., 2015; see also Fig. S1c), where agricultural water use accounts for about 70 %–90 % of total water use as reported by China Water Resources Bulletin from 1997 to 2010. Water withdrawal for irrigation is less due to the large streamflow and relatively wetter conditions in southern basins. Limited water resources can further amplify the effects of damming on river segments in northern basins (Yang and Lu, 2014), despite having fewer reservoirs compared to southern basins (see Fig. S1a).
4.2 Hydrological effects from DHI change are limited compared with climate variability
Though MAF changes between the two sub-periods are relatively small, especially in southern basins, the respective contributions of climate variability and DHI change are still distinguishable. In general, streamflow changes are dominated by climate variability between the two sub-periods in most river segments of China. The small portion (12 %) of river segments where DHI change outweighs climate impact on MAF changes are mostly in northern China. The small magnitudes of MAF changes induced by DHI change between the two sub-periods may be partly due to the fact that DHI change is not significant in most areas of China in the VARSOC experiment. Although the irrigated areas in both the northern and southern basins increased by about 20 % in the second sub-period (see Fig. S1c), the changes between the two sub-periods are small (less than 5 %) in many areas except in the Huai River and the Hai River (see Fig. S1b). Furthermore, there are only a few reservoir data from the GRanD database after the year 2000, and most reservoirs in China were built in the first sub-period (see Fig. S1d). The reservoirs lacking construction years were set to be built (and operated) at the beginning of the experiment in the model runs.
It is noted that the absolute MAF changes between the two sub-periods are large in main streams in both southern and northern basins (see Fig. S4); the significant MAF changes induced by DHI change in the Yangtze River are associated with the large reservoir regulations, e.g., the Three Gorges reservoir (Wang et al., 2013a).
4.3 Water withdrawals are identified as the major DHI to streamflow
Overall, the spatial patterns of water withdrawal changes (Fig. 7c) are similar to MAF changes induced by DHI change (ΔQh; Fig. 5c) between the two sub-periods. Though water use partly infiltrates into land surface and eventually increases local runoff (see Fig. 7d), water withdrawals should be the major DHI that contributes to decreases in streamflow in most river segments in China. For example, the significant decreases in MAF are supposed to be largely related to water withdrawals in the northwestern rivers where streamflow is low and only one reservoir was included in the VARSOC simulations. The water withdrawal changes in northwestern rivers are relatively small compared to other northern basins, but they still have significant implications because of the limited water resources. As mentioned above, water withdrawal for agricultural irrigation accounts for the largest proportion of human water use in China, most of which finally evaporates into the atmosphere through both crop and soil because of the low irrigation efficiencies (Zhu et al., 2013), which might be the main source depleting the streamflow and local water resources. Though the return flow might increase runoff over most river segments of China (Fig. 7d), it seems to be only a small proportion of the water withdrawals and does not offset the decreases in streamflow. Unlike water withdrawals, the effects of reservoir regulation on annual streamflow are mixed in current GHMs, as reservoir regulation generally reduces streamflow in flood (and growing) seasons while streamflow increases in dry seasons (Masaki et al., 2017).
4.4 Increasing DHI may impair the adaptive capacity of freshwater system
Though the effects of DHI change on streamflow are smaller compared to those of climate variability in China (see Sect. 4.2), the DHI-induced streamflow changes significantly increased, particularly in the northern basins over the 1971–2010 period (Fig. 3). The northern basins have relatively poor water endowments and have been identified as regions that are highly sensitive to climate change (Piao et al., 2010). The relatively high DHI further increases the pressure on and threats to water management and adaptation to future climate change in these regions. For example, frequent zero flow was observed in some reaches of the Yellow River due to climate variability and human water use in the 1990s (Tang et al., 2013). Most northern regions suffered severe water scarcity during the past decades (Liu et al., 2017a), and the water resources have been increasingly insufficient for human water needs in many areas of northern basins (Liu and Xia, 2004). The unregulated pumping of non-renewable groundwater has resulted in significant depletion and far-reaching effects on both hydrological cycle and human society in these regions (Feng et al., 2013). The DHI change over time further enlarges associated streamflow changes in these basins (see Fig. 4c and S5). The situation could be worse if no adaptation is taken to act under future climate change (Piao et al., 2010; Liu et al., 2015). Thus, in view of the considerable DHI in these regions, there is an urgent need for a structural transformation of the economy towards reducing water use and a sustainable development.
4.5 Uncertainties in the quantitative assessment
The major uncertainty in this quantitative assessment usually originates from input forcings (Müller Schmied et al., 2014) and inter-model differences such as human impact parameterizations (Liu et al., 2017b). That is, the uncertainties in streamflow simulations would spread to the assessment. For example, there are very few meteorological observations in the northwestern rivers, possibly leading to considerable uncertainties in the meteorological forcings used to drive GHMs. Furthermore, the GHMs cannot fully reflect sectoral water withdrawals (Fig. 2; see also Huang et al., 2018) because of a lack of data on water abstractions for human use from surface and groundwater sources (Liu et al., 2017b). Meanwhile, the different water withdrawal requirement and withdrawal sources considered in GHMs (see Table 1) may result in inter-model uncertainty in the estimates of water withdrawals and perhaps enlarge the discrepancy in streamflow simulations. The multimodel ensemble medians seem to be in line with observations averaged across the stations in China, but large discrepancies are found in some basins (Fig. S2). This indicates a large potential for the GHMs to improve streamflow simulations in China. It should be noted that we have relocated some stations on the map to reconcile the catchment areas of the stations and the corresponding grid cells on the DDM30 river network. However, catchment areas still are inconsistent between some stations and their corresponding grid cells, especially for the stations not on the main stream. This may be partly responsible for the deviation between simulated and observed streamflow. More hydrological observations (from large catchment areas) are necessary to perform a comprehensive evaluation of streamflow simulations.
In addition to the uncertainties in multimodel simulations of streamflow, the quantitative assessment depends on the selection of comparison periods. To examine the possible effects of the selection of sub-periods, we perform similar assessments for different sub-periods, i.e., MAF changes in three decades, 1981–1990, 1991–2000, and 2001–2010, compared to the first decade (1971–1980). The assessments show similar patterns of MAF changes as in Fig. 5, with larger relative changes in most northern basins (see Fig. S5 for the analysis at basin scale). Effects of climate variability on streamflow vary over different sub-periods. In contrast, DHI change usually resulted in MAF decrease across all basins, and this impact slightly increases over time (see Table S7 for corresponding numbers), especially in the northern basins such as the Yellow River, the northwestern rivers, the Liao River, and the Hai River. In the Yellow River, MAF changes induced by DHI change outweigh those induced by climate variability in the 2001–2010 period. Human activities may be weaker in China before the year 1971, and the DHI change could be larger if compared to earlier periods (e.g., Müller Schmied et al., 2016). This assessment suggests that the magnitudes of the impacts of both climate variability and DHI change on streamflow are associated with specific sub-periods; however, DHI change decreased streamflow in almost all basins in the study period.
4.6 Comparison with previous studies
Both this study and previous ones (Table S8) show that DHI (change) almost always contributes to decreases in streamflow in China, but the DHI contributions are much more significant in previous assessments compared to this one. Previous studies have shown that DHI contributed to decrease in streamflow by 20 % to 80 % across catchments in the Hai River, Yellow River, and Huai River (see Table S8; it should be noted that the proportions in the table were calculated as ). In four cases the DHI contributions are larger than those of climate impact, and in most cases DHI contributes more than 40 % in these studies (see Table S8 for the results from previous studies), while DHI contributions are mostly smaller than climate variability in this assessment (Fig. 6a). There are several reasons for the large differences between this assessment and previous ones which make their results not comparable directly, such as different methods and data, sub-periods, and study areas (see Table S8 for details). Unlike this study, the previous assessments were usually performed in small catchments that experienced evident human activities and comparison periods were usually chosen using statistical approaches (e.g., abruptly changing point detection for a time series).
One major difference between previous studies (e.g., Li et al., 2007; Bao et al., 2012) and this study is that the former estimates DHI contribution by comparing simulations with observations, while we compare two simulation experiments. The former may be subject to uncertainty in comparing the data from two systems (i.e., the model and the real world). In this study, the two simulation experiments favor the estimation of DHI contribution in a consistent manner that is largely free of uncertainty in the data from different systems. The multimodel simulations also allow profiling the uncertainties among models and input forcings, which is difficult for a single model assessment. However, the deficiency of this approach is that DHI is not real. Therefore, the assessment is inevitably influenced by the extent to which the models can reproduce the real DHI. Considering the complexity of DHI on streamflow and the ability of current hydrological models in reproducing historical hydrological changes, multimodel simulations and different attribution approaches are well worth obtaining more robust assessments (Liu et al., 2017b; Yuan et al., 2018).
A quantitative assessment of the contributions of DHI (direct human impact) and climate impact on streamflow changes is performed in the 10 major river basins in China during the 1971–2010 period. The ISIMIP2a multimodel simulations are evaluated against hydrological observations in China and are used for the assessment. The results show that DHI caused decreases of one-fifth to one-third in the long-term MAF in the sub-periods of 1971–1990 and 1991–2010 in most northern basins. MAF changes between the two sub-periods are small in southern basins but are relatively large in northern basins where MAF decreases by 10 % or more. It is found that DHI change between the two sub-periods resulted in MAF decreases in 70 % of the river segments. However, total MAF changes are dominated by climate variability in 88 % of the river segments of China. The respective contributions of climate and DHI changes to streamflow changes are more pronounced in northern basins. The relative contribution of DHI change shows significant regional difference, with relatively larger values in northern basins (−3 % to −8 % of MAF) and smaller values in southern basins (−0.7 % to −0.07 %). The contribution of climate variability to streamflow changes varies between basins, ranging from −9 % to 18 % of MAF in northern basins and from −1.6 % to 1.3 % in southern basins. The same analyses for different sub-periods, i.e., the 1980s, 1990s, and 2000s compared with the 1970s, show similar spatial patterns of the contribution of DHI change. It indicates that human intervention is high in northern basins with an increasing trend over time, which likely impairs the adaptive capacity of the freshwater system under future climate change. This assessment also shows that water withdrawals are the major factor that directly affects streamflow in China. It should be noted that this assessment is subject to uncertainties arising from the uncertainties in multimodel simulations and the choice of study periods. Nevertheless, it can serve as a reference, from a sociohydrological perspective, for the attribution of changes in streamflow at large scales under a changing environment. We highlight the importance of reducing DHI on streamflow for a sustainable development in northern basins of China and expect the assessment to favor China's strategy on adaptation to future climate change.
All model data used in this study can be accessed by the public following the instructions on the website of the Inter-Sectoral Impact Model Intercomparison Project (https://www.isimip.org/, last access: 18 February 2019).
The supplement related to this article is available online at: https://doi.org/10.5194/hess-23-1245-2019-supplement.
XL, QT, WL, and HY designed the research; XL, MF, YM, HMS, SO, YP, YS, and YW prepared the model data; XL performed the analyses and wrote the draft, and all authors wrote the paper.
The authors declare that they have no conflict of interest.
We thank the Inter-Sectoral Impact Model Intercomparison Project
coordinating team for providing the simulated data. This research is
supported by the National Natural Science Foundation of China (41730645,
41425002, 41790424, and 41877164), the Key Research Program of the Chinese
Academy of Sciences (KGFZD-135-17-009-3, ZDRW-ZS-2017-4), and the
International Partnership Program of the Chinese Academy of Sciences
(131A11KYSB20170113). Wenfeng Liu acknowledges the support received from the Early
Postdoctoral Mobility Fellowship awarded by the Swiss National Science
Foundation (P2EZP2_175096). Yadu Pokhrel acknowledges the support
from the Asian Studies Center at Michigan State University.
Edited by: Xing Yuan
Reviewed by: two anonymous referees
Biemans, H., Haddeland, I., Kabat, P., Ludwig, F., Hutjes, R. W. A., Heinke, J., von Bloh, W., and Gerten, D.: Impact of reservoirs on river discharge and irrigation water supply during the 20th century, Water Resour. Res., 47, W03509, https://doi.org/10.1029/2009WR008929, 2011.
Bondeau, A., Smith, P. C., Zaehle, S., Schaphoff, S., Lucht, W., Cramer, W., Gerten, D., Lotze-Campen, H., Müller, C., Reichstein, M., and Smith, B.: Modelling the role of agriculture for the 20th century global terrestrial carbon balance, Glob. Change Biol., 13, 679–706, https://doi.org/10.1111/j.1365-2486.2006.01305.x, 2007.
Bosmans, J. H. C., van Beek, L. P. H., Sutanudjaja, E. H., and Bierkens, M. F. P.: Hydrological impacts of global land cover change and human water use, Hydrol. Earth Syst. Sci., 21, 5603–5626, https://doi.org/10.5194/hess-21-5603-2017, 2017.
Chang, J., Zhang, H., Wang, Y., and Zhu, Y.: Assessing the impact of climate variability and human activities on streamflow variation, Hydrol. Earth Syst. Sci., 20, 1547–1560, https://doi.org/10.5194/hess-20-1547-2016, 2016.
Döll, P. and Lehner, B.: Validation of a new global 30-min drainage direction map, J. Hydrol., 258, 214–231, https://doi.org/10.1016/S0022-1694(01)00565-0, 2002.
Döll, P., Fiedler, K., and Zhang, J.: Global-scale analysis of river flow alterations due to water withdrawals and reservoirs, Hydrol. Earth Syst. Sci., 13, 2413–2432, https://doi.org/10.5194/hess-13-2413-2009, 2009.
Döll, P., Douville, H., Güntner, A., Müller Schmied, H., and Wada, Y.: Modelling Freshwater Resources at the Global Scale: Challenges and Prospects, Surv. Geophys., 37, 195–221, https://doi.org/10.1007/s10712-015-9343-1, 2016.
Fader, M., Rost, S., Müller, C., Bondeau, A., and Gerten, D.: Virtual water content of temperate cereals and maize: Present and potential future patterns, J. Hydrol., 384, 218–231, https://doi.org/10.1016/j.jhydrol.2009.12.011, 2010.
Feng, W., Zhong, M., Lemoine, J.-M., Biancale, R., Hsu, H.-T., and Xia, J.: Evaluation of groundwater depletion in North China using the Gravity Recovery and Climate Experiment (GRACE) data and ground-based measurements, Water Resour. Res., 49, 2110–2118, https://doi.org/10.1002/wrcr.20192, 2013.
Ficklin, D. L., Abatzoglou, J. T., Robeson, S. M., Null, S. E., and Knouft, J. H.: Natural and managed watersheds show similar responses to recent climate change, P. Natl. Acad. Sci. USA, 115, 8553–8557, https://doi.org/10.1073/pnas.1801026115, 2018.
Flörke, M., Kynast, E., Bärlund, I., Eisner, S., Wimmer, F., and Alcamo, J.: Domestic and industrial water uses of the past 60 years as a mirror of socio-economic development: A global simulation study, Global Environ. Change, 23, 144–156, https://doi.org/10.1016/j.gloenvcha.2012.10.018, 2013.
Gosling, S., Müller Schmied, H., Betts, R., Chang, J., Ciais, P., Dankers, R., Döll, P., Eisner, S., Flörke, M., Gerten, D., Grillakis, M., Hanasaki, N., Hagemann, S., Huang, M., Huang, Z., Jerez, S., Kim, H., Koutroulis, A., Leng, G., Liu, X., Masaki, Y., Montavez, P., Morfopoulos, C., Oki, T., Papadimitriou, L., Pokhrel, Y., Portmann, F. T., Orth, R., Ostberg, S., Satoh, Y., Seneviratne, S., Sommer, P., Stacke, T., Tang, Q., Tsanis, I., Wada, Y., Zhou, T., Büchner, M., Schewe, J., and Zhao, F.: ISIMIP2a simulation data from water (global) sector, available at: http://doi.org/10.5880/PIK.2017.010, GFZ Data Services, 2017.
Haddeland, I., Heinke, J., Biemans, H., Eisner, S., Flörke, M., Hanasaki, N., Konzmann, M., Ludwig, F., Masaki, Y., Schewe, J., Stacke, T., Tessler, Z. D., Wada, Y., and Wisser, D.: Global water resources affected by human interventions and climate change, P. Natl. Acad. Sci. USA, 111, 3251–3256, https://doi.org/10.1073/pnas.1222475110, 2014.
Hanasaki, N., Kanae, S., Oki, T., Masuda, K., Motoya, K., Shirakawa, N., Shen, Y., and Tanaka, K.: An integrated model for the assessment of global water resources – Part 1: Model description and input meteorological forcing, Hydrol. Earth Syst. Sci., 12, 1007–1025, https://doi.org/10.5194/hess-12-1007-2008, 2008a.
Hanasaki, N., Kanae, S., Oki, T., Masuda, K., Motoya, K., Shirakawa, N., Shen, Y., and Tanaka, K.: An integrated model for the assessment of global water resources – Part 2: Applications and assessments, Hydrol. Earth Syst. Sci., 12, 1027–1037, https://doi.org/10.5194/hess-12-1027-2008, 2008b.
Huang, Z., Hejazi, M., Li, X., Tang, Q., Vernon, C., Leng, G., Liu, Y., Döll, P., Eisner, S., Gerten, D., Hanasaki, N., and Wada, Y.: Reconstruction of global gridded monthly sectoral water withdrawals for 1971–2010 and analysis of their spatiotemporal patterns, Hydrol. Earth Syst. Sci., 22, 2117–2133, https://doi.org/10.5194/hess-22-2117-2018, 2018.
Ian, M. F. and Reed, M. M.: Human impacts on terrestrial hydrology: climate change versus pumping and irrigation, Environ. Res. Lett., 7, 044022, https://doi.org/10.1088/1748-9326/7/4/044022, 2012.
Jaramillo, F. and Destouni, G.: Local flow regulation and irrigation raise global human water consumption and footprint, Science, 350, 1248–1251, https://doi.org/10.1126/science.aad1010, 2015.
Lehner, B., Liermann, C. R., Revenga, C., Vörösmarty, C., Fekete, B., Crouzet, P., Döll, P., Endejan, M., Frenken, K., Magome, J., Nilsson, C., Robertson, J. C., Rödel, R., Sindorf, N., and Wisser, D.: High-resolution mapping of the world's reservoirs and dams for sustainable river-flow management, Front. Ecol. Environ., 9, 494–502, https://doi.org/10.1890/100125, 2011.
Li, L., Zhang, L., Wang, H., Wang, J., Yang, J., Jiang, D., Li, J., and Qin, D.: Assessing the impact of climate variability and human activities on streamflow from the Wuding River basin in China, Hydrol. Process., 21, 3485–3491, https://doi.org/10.1002/hyp.6485, 2007.
Liu, C. and Xia, J.: Water problems and hydrological research in the Yellow River and the Huai and Hai River basins of China, Hydrol. Process., 18, 2197–2210, 2004.
Liu, X., Liu, C., Luo, Y., Zhang, M., and Xia, J.: Dramatic decrease in streamflow from the headwater source in the central route of China's water diversion project: Climatic variation or human influence?, J. Geophys. Res.-Atmos, 117, D06113, https://doi.org/10.1029/2011JD016879, 2012.
Liu, X., Zhang, X.-J., Tang, Q., and Zhang, X.-Z.: Effects of surface wind speed decline on modeled hydrological conditions in China, Hydrol. Earth Syst. Sci., 18, 2803–2813, https://doi.org/10.5194/hess-18-2803-2014, 2014.
Liu, Y., Pan, Z., Zhuang, Q., Miralles, D. G., Teuling, A. J., Zhang, T., An, P., Dong, Z., Zhang, J., He, D., Wang, L., Pan, X., Bai, W., and Niyogi, D.: Agriculture intensifies soil moisture decline in Northern China, Sci. Rep., 5, 11261, https://doi.org/10.1038/srep11261, 2015.
Liu, X., Tang, Q., Zhang, X., and Leng, G.: Modeling the role of vegetation in hydrological responses to climate change, in: Terrestrial water cycle and climate change: Natural and human-induced impacts, edited by: Tang, Q. and Oki, T., John Wiley & Sons, Inc., Hoboken, New Jersey, 193–208, 2016.
Liu, L. and Du, J.: Documented changes in annual runoff and attribution since the 1950s within selected rivers in China, Adv. Clim. Change Res., 8, 37–47, https://doi.org/10.1016/j.accre.2017.03.005, 2017.
Liu, J., Yang, H., Gosling, S. N., Kummu, M., Flörke, M., Pfister, S., Hanasaki, N., Wada, Y., Zhang, X., Zheng, C., Alcamo, J., and Oki, T.: Water scarcity assessments in the past, present, and future, Earths Future, 5, 545–559, https://doi.org/10.1002/2016EF000518, 2017a.
Liu, X., Tang, Q., Cui, H., Mengfei, M., Gerten, D., Gosling, S., Masaki, Y., Satoh, Y., and Wada, Y.: Multimodel uncertainty changes in simulated river flows induced by human impact parameterizations, Environ. Res. Lett., 12, 025009, https://doi.org/10.1088/1748-9326/aa5a3a, 2017b.
Masaki, Y., Hanasaki, N., Biemans, H., Müller Schmied, H., Tang, Q., Wada, Y., Gosling, S. N., Takahashi, K., and Hijioka, Y.: Intercomparison of global river discharge simulations focusing on dam operation-multiple models analysis in two case-study river basins, Missouri-Mississippi and Green-Colorado, Environ. Res. Lett., 12, 055002, https://doi.org/10.1088/1748-9326/aa57a8, 2017.
Müller Schmied, H., Eisner, S., Franz, D., Wattenbach, M., Portmann, F. T., Flörke, M., and Döll, P.: Sensitivity of simulated global-scale freshwater fluxes and storages to input data, hydrological model structure, human water use and calibration, Hydrol. Earth Syst. Sci., 18, 3511–3538, https://doi.org/10.5194/hess-18-3511-2014, 2014.
Müller Schmied, H., Adam, L., Eisner, S., Fink, G., Flörke, M., Kim, H., Oki, T., Portmann, F. T., Reinecke, R., Riedel, C., Song, Q., Zhang, J., and Döll, P.: Variations of global and continental water balance components as impacted by climate forcing uncertainty and human water use, Hydrol. Earth Syst. Sci., 20, 2877–2898, https://doi.org/10.5194/hess-20-2877-2016, 2016.
Oki, T. and Kanae, S.: Global hydrological cycles and world water resources, Science, 313, 1068–1072, https://doi.org/10.1126/science.1128845, 2006.
Patterson, L. A., Lutz, B., and Doyle, M. W.: Climate and direct human contributions to changes in mean annual streamflow in the South Atlantic, USA, Water Resour. Res., 49, 7278–7291, https://doi.org/10.1002/2013WR014618, 2013.
Piao, S., Ciais, P., Huang, Y., Shen, Z., Peng, S., Li, J., Zhou, L., Liu, H., Ma, Y., Ding, Y., Friedlingstein, P., Liu, C., Tan, K., Yu, Y., Zhang, T., and Fang, J.: The impacts of climate change on water resources and agriculture in China, Nature, 467, 43–51, https://doi.org/10.1038/nature09364, 2010.
Pokhrel, Y. N., Koirala, S., Yeh, P. J. F., Hanasaki, N., Longuevergne, L., Kanae, S., and Oki, T.: Incorporation of groundwater pumping in a global Land Surface Model with the representation of human impacts, Water Resour. Res., 51, 78–96, https://doi.org/10.1002/2014WR015602, 2015.
Pokhrel, Y. N., Hanasaki, N., Wada, Y., and Kim, H.: Recent progresses in incorporating human land-water management into global land surface models toward their integration into Earth system models, WIREs Water, 3, 548–574, https://doi.org/10.1002/wat2.1150, 2016.
Portmann, F. T., Siebert, S., and Döll, P.: MIRCA2000-Global monthly irrigated and rainfed crop areas around the year 2000: A new high-resolution data set for agricultural and hydrological modeling, Global Biogeochem. Cy., 24, GB1011, https://doi.org/10.1029/2008GB003435, 2010.
Rost, S., Gerten, D., Bondeau, A., Lucht, W., Rohwer, J., and Schaphoff, S.: Agricultural green and blue water consumption and its influence on the global water system, Water Resour. Res., 44, W09405, https://doi.org/10.1029/2007WR006331, 2008.
Schaphoff, S., Heyder, U., Ostberg, S., Gerten, D., Heinke, J., and Lucht, W.: Contribution of permafrost soils to the global carbon budget, Environ. Res. Lett., 8, 014026, https://doi.org/10.1088/1748-9326/8/1/014026, 2013.
Sheffield, J., Goteti, G., and Wood, E. F.: Development of a 50-year high-resolution global dataset of meteorological forcings for land surface modeling, J. Climate, 19, 3088–3111, https://doi.org/10.1175/jcli3790.1, 2006.
Takata, K., Emori, S., and Watanabe, T.: Development of the minimal advanced treatments of surface interaction and runoff, Global Planet. Change, 38, 209–222, https://doi.org/10.1016/S0921-8181(03)00030-4, 2003.
Tan, X. and Gan, T. Y.: Contribution of human and climate change impacts to changes in streamflow of Canada, Sci. Rep., 5, 17767, https://doi.org/10.1038/srep17767, 2015.
Tang, Q. and Oki, T.: Terrestrial Water Cycle and Climate Change: Natural and Human-Induced Impacts, AGU Geophysical Monograph Series, John Wiley & Sons, Hoboken, New Jersey, 246 pp., 2016.
Tang, Q., Oki, T., Kanae, S., and Hu, H.: The influence of precipitation variability and partial irrigation within grid cells on a hydrological simulation, J. Hydrometeorol., 8, 499–512, https://doi.org/10.1175/jhm589.1, 2007.
Tang, Q., Oki, T., Kanae, S., and Hu, H.: Hydrological Cycles Change in the Yellow River Basin during the Last Half of the Twentieth Century, J. Climate, 21, 1790–1806, https://doi.org/10.1175/2007JCLI1854.1, 2008.
Tang, Y., Tang, Q., Tian, F., Zhang, Z., and Liu, G.: Responses of natural runoff to recent climatic variations in the Yellow River basin, China, Hydrol. Earth Syst. Sci., 17, 4471–4480, https://doi.org/10.5194/hess-17-4471-2013, 2013.
Veldkamp, T. I. E., Wada, Y., Aerts, J. C. J. H., Doll, P., Gosling, S. N., Liu, J., Masaki, Y., Oki, T., Ostberg, S., Pokhrel, Y., Satoh, Y., Kim, H., and Ward, P. J.: Water scarcity hotspots travel downstream due to human interventions in the 20th and 21st century, Nat. Commun., 8, 15697, https://doi.org/10.1038/ncomms15697, 2017.
Veldkamp, T. I. E., Zhao, F., Ward, P. J., Moel, H. d., Aerts, J. C. J. H., Schmied, H. M., Portmann, F. T., Masaki, Y., Pokhrel, Y., Liu, X., Satoh, Y., Gerten, D., Gosling, S. N., Zaherpour, J., and Wada, Y.: Human impact parameterizations in global hydrological models improve estimates of monthly discharges and hydrological extremes: a multi-model validation study, Environ. Res. Lett., 13, 055008, https://doi.org/10.1088/1748-9326/aab96f, 2018.
Wada, Y. and Heinrich, L.: Assessment of transboundary aquifers of the world-vulnerability arising from human water use, Environ. Res. Lett., 8, 024003, https://doi.org/10.1088/1748-9326/8/2/024003, 2013.
Wada, Y., van Beek, L. P. H., Wanders, N., and Bierkens, M. F. P.: Human water consumption intensifies hydrological drought worldwide, Environ. Res. Lett., 8, 034036, https://doi.org/10.1088/1748-9326/8/3/034036, 2013.
Wada, Y., Wisser, D., and Bierkens, M. F. P.: Global modeling of withdrawal, allocation and consumptive use of surface water and groundwater resources, Earth Syst. Dynam., 5, 15–40, https://doi.org/10.5194/esd-5-15-2014, 2014.
Wada, Y., Bierkens, M. F. P., de Roo, A., Dirmeyer, P. A., Famiglietti, J. S., Hanasaki, N., Konar, M., Liu, J., Müller Schmied, H., Oki, T., Pokhrel, Y., Sivapalan, M., Troy, T. J., van Dijk, A. I. J. M., van Emmerik, T., Van Huijgevoort, M. H. J., Van Lanen, H. A. J., Vörösmarty, C. J., Wanders, N., and Wheater, H.: Human-water interface in hydrological modelling: current status and future directions, Hydrol. Earth Syst. Sci., 21, 4169–4193, https://doi.org/10.5194/hess-21-4169-2017, 2017.
Wang, G., Xia, J., and Chen, J.: Quantification of effects of climate variations and human activities on runoff by a monthly water balance model: A case study of the Chaobai River basin in northern China, Water Resour. Res., 45, W00A11, https://doi.org/10.1029/2007WR006768, 2009.
Wang, J., Hong, Y., Gourley, J., Adhikari, P., Li, L., and Su, F.: Quantitative assessment of climate change and human impacts on long-term hydrologic response: a case study in a sub-basin of the Yellow River, China, Int. J. Climatol., 30, 2130–2137, https://doi.org/10.1002/joc.2023, 2010.
Wang, J., Sheng, Y., Gleason, C. J., and Wada, Y.: Downstream Yangtze River levels impacted by Three Gorges Dam, Environ. Res. Lett., 8, 044012, https://doi.org/10.1088/1748-9326/8/4/044012, 2013a.
Wang, W., Shao, Q., Yang, T., Peng, S., Xing, W., Sun, F., and Luo, Y.: Quantitative assessment of the impact of climate variability and human activities on runoff changes: a case study in four catchments of the Haihe River basin, China, Hydrol. Process., 27, 1158–1174, https://doi.org/10.1002/hyp.9299, 2013b.
Wang, J., Sheng, Y., and Wada, Y.: Little impact of the Three Gorges Dam on recent decadal lake decline across China's Yangtze Plain, Water Resour. Res., 53, 3854–3877, https://doi.org/10.1002/2016WR019817, 2017.
Weedon, G. P., Gomes, S., Viterbo, P., Shuttleworth, W. J., Blyth, E., Österle, H., Adam, J. C., Bellouin, N., Boucher, O., and Best, M.: Creation of the WATCH Forcing Data and its use to assess global and regional reference crop evaporation over land during the twentieth century, J. Hydrometeorol., 12, 823–848, https://doi.org/10.1175/2011jhm1369.1, 2011.
Weedon, G. P., Balsamo, G., Bellouin, N., Gomes, S., Best, M. J., and Viterbo, P.: The WFDEI meteorological forcing data set: WATCH Forcing Data methodology applied to ERA-Interim reanalysis data, Water Resour. Res., 50, 7505–7514, https://doi.org/10.1002/2014WR015638, 2014.
Yang, X. and Lu, X.: Drastic change in China's lakes and reservoirs over the past decades, Sci. Rep., 4, 6041, https://doi.org/10.1038/srep06041, 2014.
Ye, X., Zhang, Q., Liu, J., Li, X., and Xu, C.-Y.: Distinguishing the relative impacts of climate change and human activities on variation of streamflow in the Poyang Lake catchment, China, J. Hydrol., 494, 83–95, https://doi.org/10.1016/j.jhydrol.2013.04.036, 2013.
Yin, Y., Tang, Q., Liu, X., and Zhang, X.: Water scarcity under various socio-economic pathways and its potential effects on food production in the Yellow River basin, Hydrol. Earth Syst. Sci., 21, 791–804, https://doi.org/10.5194/hess-21-791-2017, 2017.
Yuan, X., Jiao, Y., Yang, D., and Lei, H.: Reconciling the attribution of changes in streamflow extremes from a hydroclimate perspective, Water Resour. Res., 54, 3886–3895, https://doi.org/10.1029/2018WR022714, 2018.
Zaherpour, J., Gosling, S. N., Mount, N., Schmied, H. M., Veldkamp, T. I. E., Dankers, R., Eisner, S., Gerten, D., Gudmundsson, L., Haddeland, I., Hanasaki, N., Kim, H., Leng, G., Liu, J., Masaki, Y., Oki, T., Pokhrel, Y., Satoh, Y., Schewe, J., and Wada, Y.: Worldwide evaluation of mean and extreme runoff from six global-scale hydrological models that account for human impacts, Environ. Res. Lett., 13, 065015, https://doi.org/10.1088/1748-9326/aac547, 2018.
Zhan, C. S., Jiang, S. S., Sun, F. B., Jia, Y. W., Niu, C. W., and Yue, W. F.: Quantitative contribution of climate change and human activities to runoff changes in the Wei River basin, China, Hydrol. Earth Syst. Sci., 18, 3069–3077, https://doi.org/10.5194/hess-18-3069-2014, 2014.
Zhang, L., Dawes, W. R., and Walker, G. R.: Response of mean annual evapotranspiration to vegetation changes at catchment scale, Water Resour. Res., 37, 701–708, https://doi.org/10.1029/2000wr900325, 2001.
Zhang, C., Tang, Q., and Chen, D.: Recent changes in the moisture source of precipitation over the Tibetan Plateau, J. Climate, 30, 1807–1819, https://doi.org/10.1175/jcli-d-15-0842.1, 2017.
Zhou, T., Nijssen, B., Gao, H., and Lettenmaier, D. P.: The contribution of reservoirs to global land surface water storage variations, J. Hydrometeorol., 17, 309–325, https://doi.org/10.1175/JHM-D-15-0002.1, 2015.
Zhu, X., Li, Y., Li, M., Pan, Y., and Shi, P.: Agricultural irrigation in China, J. Soil Water Conserv., 68, 147–154, https://doi.org/10.2489/jswc.68.6.147A, 2013.