the Creative Commons Attribution 3.0 License.
the Creative Commons Attribution 3.0 License.
A regional-scale ecological risk framework for environmental flow evaluations
Gordon C. O'Brien
Chris Dickens
Eleanor Hines
Victor Wepener
Retha Stassen
Leo Quayle
Kelly Fouchy
James MacKenzie
P. Mark Graham
Wayne G. Landis
Environmental flow (E-flow) frameworks advocate holistic, regional-scale, probabilistic E-flow assessments that consider flow and non-flow drivers of change in a socio-ecological context as best practice. Regional-scale ecological risk assessments of multiple stressors to social and ecological endpoints, which address ecosystem dynamism, have been undertaken internationally at different spatial scales using the relative-risk model since the mid-1990s. With the recent incorporation of Bayesian belief networks into the relative-risk model, a robust regional-scale ecological risk assessment approach is available that can contribute to achieving the best practice recommendations of E-flow frameworks. PROBFLO is a holistic E-flow assessment method that incorporates the relative-risk model and Bayesian belief networks (BN-RRM) into a transparent probabilistic modelling tool that addresses uncertainty explicitly. PROBFLO has been developed to evaluate the socio-ecological consequences of historical, current and future water resource use scenarios and generate E-flow requirements on regional spatial scales. The approach has been implemented in two regional-scale case studies in Africa where its flexibility and functionality has been demonstrated. In both case studies the evidence-based outcomes facilitated informed environmental management decision making, with trade-off considerations in the context of social and ecological aspirations. This paper presents the PROBFLO approach as applied to the Senqu River catchment in Lesotho and further developments and application in the Mara River catchment in Kenya and Tanzania. The 10 BN-RRM procedural steps incorporated in PROBFLO are demonstrated with examples from both case studies. PROBFLO can contribute to the adaptive management of water resources and contribute to the allocation of resources for sustainable use of resources and address protection requirements.
- Article
(3474 KB) - Full-text XML
-
Supplement
(267 KB) - BibTeX
- EndNote
The global use of water resources has altered the wellbeing of aquatic ecosystems and the benefits that people derive from them (Acreman and Dunbar, 2004; Dudgeon et al., 2006; Growns, 2008; Vörösmarty, 2010; Isaak et al., 2012; Murray et al., 2012; Grafton et al., 2013; Dudgeon, 2014). Environmental flows (E-flows), according to the Brisbane Declaration (2007), are defined as the “quantity, timing and quality of water flows required to sustain freshwater and estuarine ecosystems and the human livelihoods and wellbeing that depend on these ecosystems”. In an effort to determine E-flows, the international community has developed a plethora of E-flow assessment methods, which have been applied on numerous spatial scales in a wide range of ecosystem types across the globe (Tharme, 2003; Acreman and Dunbar, 2004; Pahl-Wostl et al., 2013; Poff and Matthews, 2013). These methods have evolved during three distinct periods according to Poff and Matthews (2013) including an emergence and synthesis period, consolidation and expansion period and the current globalization period. During this globalization period a range of best practice E-flow management and assessment principles, and associated frameworks to undertake E-flow on multiple spatial scales in multiple political and/or legislative contexts, have been developed (Poff et al., 2010; Pahl-Wostl et al., 2013). These principles promote the use of holistic assessment tools that consider both social and ecological features of ecosystems on regional spatial scales, are adaptive and incorporate risk evaluation and address uncertainty (Poff et al., 2010; Acreman et al., 2014).
Ecological risk assessments have been undertaken internationally at different spatial scales using the relative-risk model (RRM) established since the mid-1990s (Hunsaker et al., 1990; Landis and Weigers, 1997, 2007; Wiegers et al., 1998; Landis, 2004, 2016). The RRM has been applied to evaluate a range of natural and anthropogenic stressors including water pollution, diseases, alien species and a range of altered environmental states (Walker et al., 2001; Moraes et al., 2002; Hayes and Landis, 2004; Colnar and Landis, 2007; Anderson and Landis, 2012; Ayre and Landis, 2012; Bartolo et al., 2012; O'Brien et al., 2012; Hines and Landis, 2014; Ayre et al., 2014). This tool can be used to carry out holistic, probabilistic assessments of the risk to the availability and conditions of ecosystem service and ecological endpoints, and facilitate socio-ecological trade-offs. For more information on the application of the RRM consider Colnar and Landis (2007), Anderson and Landis (2012) or O'Brien and Wepener (2012). Recent developments to the RRM incorporate the use of Bayesian networks (BNs) that have been established as a powerful tool for ecological risk assessment, ecosystem management and E-flow assessment (Pollino et al., 2007; Hart and Pollino, 2008; Shenton et al., 2011; Chan et al., 2012; Pang and Sun, 2014; Liu et al., 2016; McDonald et al., 2016). In 2012, Ayre and Landis combined both approaches and incorporated BNs into RRMs, which was then formalized into a BN-RRM approach (Hines and Landis, 2014; Herring et al., 2015; Landis et al., 2016).
Between 2013 and 2016 a BN-RRM-based holistic E-flow assessment approach was developed that adheres to the principles of best E-flow management practice and can easily be incorporated into regional E-flow frameworks such as the Ecological Limits of Hydrologic Alteration framework (Poff et al., 2010). This BN-RRM approach, which we have called PROBFLO, is a transparent and adaptable, evidence-based probabilistic modelling approach that can also incorporate expert solicitations and explicitly address uncertainty. PROBFLO is a scenario-based E-flow assessment tool that allows for the evaluation of the socio-ecological consequences of altered flows with consideration of the synergistic effects of non-flow drivers of ecosystem impairment. It adheres to the regional-scale ecological risk assessment exposure and effects, or sources of multiple stressors, habitats and ranked ecological impacts relationship (Wiegers et al., 1998). This paper presents the PROBFLO BN-RRM approach that was used to establish E-flows for the Senqu River in Lesotho, and it evaluates the socio-ecological effects of altered flow and non-flow stressors and developments made in the Mara River in the Kenya and Tanzania case study.
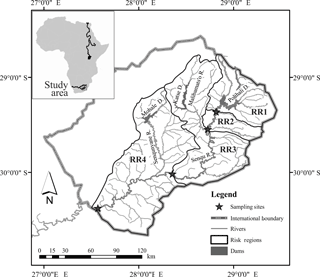
Figure 1The upper Senqu River study area with risk regions (RRs) established for the study including dams associated with Phase I of the Lesotho Highlands Water Project and the location of the new Polihali Dam planned to be built in Phase II.
The Lesotho Highlands Water Project (LHWP) is a USD multi-billion water transfer and hydropower project implemented by the governments of Lesotho and South Africa (LHWP, 1986, 2011). Phase I of the LHWP involved the application of the Downstream Response to Imposed Flow Transformations (DRIFT) approach to establish the E-flows associated with the construction of the Katse and Mohale dams on the Malibamats'o and Senqunyane rivers in Lesotho respectively (Arthington et al., 2003; King et al., 2003). Phase II involves the augmentation of the LHWP by construction of the Polihali Dam to divert water directly from the upper Senqu River to the existing Phase I infrastructure of the LHWP (Fig. 1). For Phase II, the custodians of the project, the Lesotho Highlands Development Authority (LHDA), required the service provider awarded with the E-flow determination project to review and implement current best E-flow practice. This included the requirements to implement a probabilistic, regional-scale modelling approach that is transparent and holistic, addressing socio-ecological components and endpoints, and one that considers uncertainty explicitly. The PROBFLO approach has, as a result, been selected for Senqu River in Lesotho as a part of Phase II of LHWP between the proposed Polihali Dam site (29.289593∘ S; 28.863890∘ E) and the border of South Africa (30.413231∘ S; 27.564090∘ E) (LHWP, 2016).
The entire Mara River in Kenya and Tanzania upstream of the mouth into Lake Victoria (1.518178∘ S; 33.943497∘ E) was considered in this regional-scale PROBFLO case study (NBI, 2016) (Fig. 2). The Mara River and its tributaries are an essential source of water for domestic needs, agriculture, pastoralism and wildlife, including tourism, in Kenya and Tanzania (Mati et al., 2008; Defersha and Melesse, 2012). Although extensive research has been undertaken into the management of the terrestrial ecosystems of the Serengeti National Park and Maasai Mara National Reserve, there are limited studies on the effects of land use threats to the wellbeing of the Mara River, with only site-specific evaluations of the environmental flows of the river (Broten and Said, 1995; Gereta et al., 2002; Onjala, 2002; Karanja, 2003; Lamprey and Reid, 2004; Hoffman, 2007; Mati et al., 2008; Atisa, 2009; GLOWS-FIU, 2012; Majule, 2010; Hoffman et al., 2011; Ogutu et al., 2011; Defersha and Melesse, 2012; Kiambi et al., 2012; Dessu et al., 2014).
The PROBFLO framework is based on 10 procedural RRM steps (Landis, 2004), and it incorporates BN development and evaluation procedures (Marcot et al., 2006; Ayre and Landis, 2012) into a robust E-flow assessment method that gives emphasis to adaptive management for holistic E-flow management (Fig. 3). The PROBFLO approach has been implemented in the Senqu and Mara River case studies to evaluate the socio-ecological consequences of altered flows and determine E-flows, which is demonstrated through application of the following 10 procedural steps.
3.1 Step 1: Vision exercise
The importance of having clear water resource management objectives cannot be overemphasized. Numerous Integrated Water Resource Management strategies, regional management plans and frameworks, national legislation and established E-flow assessment tools advocate the establishment of clear goals or visions to direct the use and protection of water resources (Biswas, 2004; Mitchell, 2005; Dudgeon et al., 2006; Richter et al., 2006; Poff et al., 2010; King and Pienaar, 2011; NBI, 2016). Although many vision development approaches are available, the initial application of PROBFLO involved the application of the Resource Quality Objectives (RQOs) determination procedure (DWA, 2011) to describe and document the water quality, water quantity, habitat and biota objectives for the water resource being evaluated (NBI, 2016; DWA, 2011). The RQO process results in narrative and numerical descriptions of various ecosystem features required to achieve a balance between the use and protection of water resources and hence to achieve a documented vision. As part of the initial development of the RRM approach, multiple social and ecological endpoints were evaluated in a relative manner. Social endpoints were limited to the availability and quality of ecosystem services and ecological endpoints, including the requirements to maintain selected ecological indicators in an acceptable integrity state or wellbeing. In addition, for these environmental flow assessment (EFA) case studies, endpoints associated with socio-ecological impacts of the rivers resulting directly or indirectly with altered flows were considered. Findings resulted in relative risks to endpoints that could be compared and used to consider cost-benefit trade-offs between social and ecological endpoints by adjusting water resource use and protection scenarios.
The treaties for Phase I and Phase II of the LHWP entered into by the Kingdom of Lesotho and the Republic of South Africa provided the requirements for the establishment of a vision for water resource use and protection for the Senqu River case study (LHWP, 1986, 2011). The Treaty gives emphasis to protection of the existing quality of the environment and, in particular, requires maintenance of the wellbeing of persons and communities immediately affected by the project, including those downstream of the dam. Accordingly, the vision states that there should be no change to the existing quality of the downstream environment and that the net effect of the dam should not be negative to the people living downstream of the dam. For the PROBFLO assessment, RQOs describing the desired quality and quantity of water, habitat and biota for the study area were established. The endpoints selected to represent the social and ecological management objectives for the PROBFLO assessment were based on the vision represented by the RQOs in this case study, including the maintenance of the following ecosystem services and ecological objectives affected by the river: (1) the supply of building sand from the Senqu River, (2) water for domestic use, (3) recreation/spiritual use of the river, (4) fish stocks as food for people, (5) edible plants from the riparian zone as food for people, (6) medicinal plants for people, (7) floodplain non-woody plants (for grazing), (8) woody plants for fuel and construction, (9) reeds for construction and (10) fish stocks, (11) aquatic macro-invertebrate and (12) riparian ecosystem integrity or wellbeing.
The vision for the Mara River case study was based on existing regional Mara River management objectives (WRMA, 2014). In 2014, a Catchment Management Strategy (CMS) for the Mara Basin in Kenya was developed to facilitate the management of the water resources, environment and human behaviour in ways that achieve equitable, efficient and sustainable use of water for the benefit of all users (WRMA, 2014). The aims of the Mara River basin as part of the Strategic Environmental Assessment (EAC, 2003) to maintain “the people living in harmony with nature while achieving human wellbeing and sustainable economic development in perpetuity” were also considered. Also considered were the objectives for the Mara River basin as described by the Biodiversity, and Strategy Action Plan, which describes “a region rich in biodiversity which benefits the present and future generations and ecosystem functions” (GLOWS-FIU, 2012). The Kenyan Water Resources Management Authority (WRMA) established a high ecological importance, high livelihoods value and low commercial value vision for the upper Mara River basin (Nyangores and Amala rivers) and a high ecological importance, moderate livelihoods value and moderate commercial value vision for the mainstream Mara River. In this context the endpoints selected for the study included (1) the provision of water for basic human needs according to the national legislation of Kenya and Tanzania, (2) the maintenance of the ecological integrity of the riverine ecosystem (instream and riparian ecosystems), (3) the provision of flows for the commercial production of crops, (4) the maintenance of existing livestock industry, (5) the maintenance and viability of the ecotourism industry and (6) the maintenance of the ecological integrity of the Mara Wetland in the lower reaches of the basin.
3.2 Step 2: Mapping and data analyses
The BN-RRM approach that forms the basis of PROBFLO includes the relative evaluation of multiple sources of stressors to endpoints on a regional scale, which should be spatially and temporally referenced for regional comparisons/evaluations in a PROBFLO assessment (Landis, 2004; Landis and Wiegers, 2007). For this the spatial extent of the study area must be defined and described, and the locations of potential sources, habitats and impacts must be identified and spatially referenced. In addition, source-stressor exposure and habitat/receptor to endpoint pathways/relationships should be spatially referenced where possible (O'Brien and Wepener, 2012; Landis et al., 2016). Available data describing the ecosystem need to be reviewed and spatially referenced and the uncertainties associated with the availability and quality of data used in the assessment must be documented for evaluation in Step 7. O'Brien and Wepener (2012) provide an approach to delineate ecosystem types, the topological features of importance, the catchment and ecoregion boundaries, the land or water resource use scenarios and the pathways of stressors' exposure. This approach is used to direct the selection of risk regions for assessment (Smit et al., 2016). Best practice E-flow frameworks accentuate the importance of ecosystem type classification as part of E-flow assessments to improve our understanding of flow–ecosystem relationships (Poff et al., 2010; Arthington, 2012).
3.3 Step 3: Risk region selection
In this step, combinations of the management objectives, source information and habitat data are used to establish geographical risk regions that can be assessed in a relative manner (Landis, 2004; O'Brien and Wepener, 2012). In the end, the outcomes of the assessment will be available at the spatial scale established during this step for multiple temporal scenarios associated with alternative management options. In this regard it is important to consider the spatial connectivity of multiple variables including flows and other variables within the study area so that risk regions incorporate appropriate sources, stressors, habitats and endpoints (Landis, 2004; O'Brien and Wepener, 2012). The approach can address spatial and temporal relationships of variables between risk regions, such as the downstream effect of a source on multiple risk regions, in the context of the assimilative capacity of the ecosystem or the upstream connectivity requirements of a migratory fish between risk regions. To demonstrate that PROBFLO can conform to the regional E-flow assessment frameworks such as ELOHA (Poff et al., 2010), the selection of RRs should include explicit hydrological and geomorphological classification. The relative risk outcomes of the assessment can later be directly related to the system classification as proposed by ELOHA. With additional E-flow information for a range of hydrological and geomorphological ecosystem types, the outcomes can be used to establish regional E-flows.
The selection of risk regions for the Senqu River E-flow assessment was based on the proposed location of the Polihali Dam and catchment boundaries of the Senqu River and large tributaries (Malibamatso and Senqunyane rivers) for this E-flow assessment. Physical access to sampling sites within Lesotho to conduct biophysical field surveys was extremely difficult and this also contributed to risk region selection. Four broad risk regions were selected for the Senqu River PROBFLO study (Fig. 1).
In the Mara River case study, a review of land use and land cover (Mati et al., 2008; Atisa et al., 2014), hydrology (Mango et al., 2011; McClain et al., 2014), the vision for the case study, current and future land and water resource use options and socio-ecological importance (Karanja, 2002; LVBC and WWF-ESARPO, 2010; Mango et al., 2011; Defersha and Melesse, 2012; GLOWS-FIU, 2012; Dessu et al., 2014; Dutton et al., 2013) was used to select risk regions. Ten risk regions were selected for the Mara River case study which conformed to catchment boundaries, ecoregions, land use practices and the international boundary (Fig. 2).
3.4 Step 4: Conceptual model
In this step conceptual models that describe hypothesized relationships between multiple sources, stressors, habitats and impacts to endpoints selected for the study are generated (Wiegers et al., 1998) (Fig. 4). This includes the holistic (consider flow- and non-flow-related variables in a spatial–temporal context), best practice characterization of flow–ecosystem and flow–ecosystem service relationships in the context of a regional-scale E-flows framework (Poff et al., 2010), with relevant non-flow (water quality and habitat) relationships in the models. Conceptual models should be constructed by expert stakeholders usually including hydrologists, geomorphologists, ecologists and ecosystem services, including social and resource economics scientists. These experts should be familiar with socio-ecological system processes and be able to describe probable cause and effect variables and relationships of sources to stressors to multiple receptors in relation to their impacts on the endpoints, selected for the study. The conceptual models for the case studies presented addressed requirements of the ELOHA and the Nile Basin regional-scale E-flow frameworks to conform to these frameworks (Poff et al., 2010; NBI, 2016). The Nile Basin regional-scale E-flow framework expands on the ELOHA framework to include an initial situation assessment, data review and alignment phase and a governance and Resource Quality Objectives setting phase. The PROBFLO conceptual model thus conforms to the regional-scale E-flow framework procedures in (1) the selection of socio-ecological endpoints, to direct the hydrologic foundations for the study including the selection of hydrological statistics required; (2) the classification of ecosystem types based on geomorphic, water quality, quantity and ecoregion considerations; and with these data, (3) the incorporation of evidence-based flow–ecosystem relationships and flow–ecosystem service relationships, with relevant non-flow variable relationships upon which the assessment is based. Initial conceptual model development considers all relevant sources, stressors, habitat, effects and impact relationships with spatial and temporal considerations.
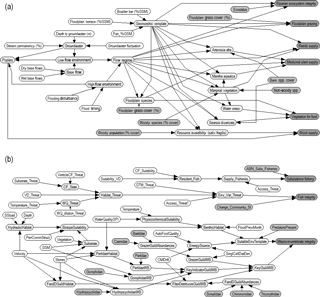
Figure 5Bayesian network models used in the Senqu River case study to evaluate the risk of flow alterations associated with Phase II of the Lesotho Highlands Water Project to riparian ecosystem integrity and ecosystem service endpoints (a) and instream ecological endpoints and fisheries supply endpoints (b). White nodes represent input exposure variables, light grey nodes complete the expose leg of the risk assessment, grey nodes represent effect leg of models and dark grey nodes represent endpoints. Working Netica™ models are provided in the Supplement.
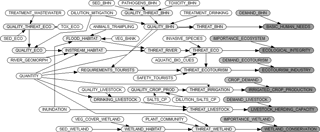
Figure 6Bayesian network models used in the Mara River case study to evaluate the risk of water resource use to a range of socio-ecological endpoints. White nodes represent input exposure variables, light grey nodes complete the expose leg of the risk assessment, grey nodes represent effect leg of models and dark grey nodes represent endpoints. Working Netica™ models are provided in the Supplement.
3.5 Step 5: Ranking scheme
Ranking schemes are used to represent the state of variables, with unique measures and units to be comparable as non-dimensional ranks and combined in BN-RRMs (Landis, 2004; Landis et al., 2016). Four states, designated as zero, low, moderate and high as traditionally used in RRMs (Colnar and Landis, 2007; O'Brien and Wepener, 2012; Hines and Landis, 2014; Landis et al., 2016), have been incorporated into the PROBFLO process. The states represent the range of wellbeing conditions, levels of impacts and management ideals as follows.
-
Zero. This describes a pristine state, with no impact/risk, comparable to the pre-anthropogenic source establishment, baseline or reference state.
-
Low. This is a largely natural state with low impact/risk, and it describes an ideal range for sustainable ecosystem use.
-
Moderate. This state refers to moderate use or a modified state, with moderate impact/risk, representing the threshold of potential concern or alert range.
-
High. This describes a significantly altered or impaired state, with unacceptably high impact/risk.
This ranking scheme selected for PROBFLO represents the full range of potential risk to the ecosystem and ecosystem services with management options. Low risk states usually represent management targets with little impact, and moderate risk states represent partially suitable ecosystem conditions that usually warrant management/mitigation measures to avoid high risk conditions. The incorporation of BN modelling into PROBFLO allows the approach to incorporate the variability between ranks for each model variable, represented as a percentage for each rank. Indicator flow and non-flow variables representing the socio-ecological system being evaluated in a PROBFLO assessment are selected (linked to endpoints – step 1), and unique measures and units of measurement are converted into, and represented by, ranks for integration in BN assessments. For the BN assessment, ranks are assigned scores along a percentage continuum representing the state of the variables using natural breaks of 0.25 (zero), 0.5 (low), 0.75 (moderate) and 1 (high) in the calculation.
3.6 Step 6: Calculate risks
From the general inclusive conceptual models (step 4), with the principle of requisite simplicity (Stirzaker et al., 2010), smaller social and ecological endpoint specific models that represent the system being assessed are unpacked and converted into Bayesian network models (Figs. 5 and 6) for analyses. These models can be analysed individually or integrated using a range of BN modelling tools, using nodes representing variables that share the same indicators and measures. Bayesian networks are probabilistic modelling networks that graphically represent joint probability distributions over a set of statistical values (Pollino et al., 2007; Korb and Nicholson, 2010). They include parent or input nodes and child or conditional nodes with links that represent causal relationships between nodes combined by Conditional Probability Tables (CPTs) (McCann et al., 2006; Landis et al., 2016). Conditional Probability Tables describe conditional probabilities between the occurrence of states in the parent nodes and the resulting probabilities of states in the child nodes (Landis et al., 2016). The two PROBFLO case studies presented here made use of the Netica™ BN software by Norsys Software (http://www.norsys.com/).
The BNs are initially used to evaluate the risk of anthropogenic/natural hazards to endpoints per risk region, in relative manner for comparisons, for multiple temporal periods (high or low flow months and wet or drought phases etc.) which can also be compared relative to each other. Bayesian networks also make use of available data and expert solicitations as evidence to represents risks to current or present scenarios. Present projections of risk to the endpoints can generally be easily validated using available data, knowledge of existing relationships between variables and by carrying out directed field survey campaigns to describe/test risk relationships. Present risk projections are then calibrated by evaluating benchmark or historical scenario risk projections using the established models, which can often be validated with historical data (see data in the Supplement). An example of how a BN can represent a component of the socio-ecological ecosystem being evaluated is presented in Figs. 5 and 6. Within Fig. 5 we for example hypothesize that the ecological integrity of fish in the Senqu can be selected as an indicator of the Senqu River ecosystem as a suitable ecological endpoint. In this example the ecological integrity of fish is hypothesized to be a function of the conditions of the Senqu River environment for fish, representing the exposure leg of the risk assessment and the potential for fish to occur within the reach of the river being considered as an effects component of the study (refer to Table S1 in the Supplement). The conditions of the Senqu River environment itself are hypothesized in this study to be a function of the potential of communities to be a “disturbance to wildlife”. This describes the instream habitat conditions and migration access for fish as source-stressor relationships with local communities, multiple barriers and other source/stressors that affect instream habitat wellbeing, selected indicators representing ecosystem components with associated measures in the study. Water resource use scenarios were used to describe the state of source/stressor and effect nodes as inputs into the model. These variables were integrated using CPTs to represent other system variables, which ultimately result in risk described to endpoints (refer to Table S2). These models are then used to determine E-flow requirements according to acceptable trade-off of risk to endpoints selected for the study, and the consequences of alternative water resource use, management and/or climatic condition scenarios.
To determine E-flow requirements in PROBFLO, trade-offs of acceptable risk to social and ecological endpoints are initially established for each risk region by stakeholders. This usually takes place within a legislative context where regional legislation/policies/agreements are addressed, such as requirements to maintain ecosystem sustainability, which affects trade-off developments. These trade-offs of acceptable risk are represented in the BNs as forced endpoint risk distributions or profiles. These profiles usually range between low and moderate risk, with usually no high risk probabilities. In relation to the definitions of the ranks used in PROBFLO, trade-offs of acceptable risk for E-flow determination should only dominate the “moderate” risk range when there is certainty that the E-flow requirements can be provided, such as in the case of E-flow releases from a dam. In case studies where there is high uncertainty associated with the ability to provide E-flow requirements, such as the management of multiple water resource users to cumulatively maintain E-flows, then a buffer should be provided according to the definition of ranks and the “low” risk range should be selected. After the selection of trade-offs of acceptable risk are established, the calibrated BNs are forced to generate the state (rank distributions) of input flow variables used in the assessments. These flow-related variable state requirements that are spatially and temporally referenced are provided to a hydrologist or geomorphologist, for example, to describe the E-flow requirements which can be presented in various formats, such as daily or monthly water (usually m3 s−1) and sediment (usually kg m−3 s−1) discharge duration percentiles. During E-flow determination procedures, the state of non-flow variable nodes, which contribute to the risk to endpoints, associated with flow variables can either be maintained in their current state, and described as such or amended with available water resource use information. This can include the increased requirement of water for basic human needs, for increases in growths of human populations depending on the resource, for example. Following the establishment of E-flows, the socio-ecological consequences of altered flows, associated with alternative water resource management options or climate change variability, for example, can be evaluated in a relative manner by generating and evaluating a range of future scenarios in PROBFLO.
3.6.1 Senqu River risk calculation
In the Lesotho case study the nine social endpoints and three ecological endpoints were used to represent the socio-ecological endpoints of interest in the study. The 12 BN models established for the study included cause and effect linkages used to estimate risk (refer to BN models provided in the Supplement). These BNs were used to evaluate the risk of multiple sources and stressors with flow-related stressors for base winter (low), summer (high) and drought flows. Where appropriate, CPTs of the BNs for endpoints were adjusted between RRs to represent the subtle changes in ecosystem process dynamics down the length of the Senqu River (refer to Tables S1 and S2).
Evidence used includes the historical understanding of flow–ecosystem and flow–ecosystem service relationships established during Phase I of the LHWP and data derived from a series of biophysical surveys of the study area (refer to Tables S1 and S2). The surveys included hydrology, hydraulic, water quality and geomorphology components grouped as ecosystem driver components, and fish, macro-invertebrates and riparian vegetation grouped as ecosystem responder components. Hydrological data used in the case study included recently updated basin-wide Integrated Water Resources Management Plan outcomes (ORASECOM, 2014). This database was updated in the study with latest available rainfall records, obtained from the Lesotho Meteorological Services, and regional evaporation information. Observed monthly flow data with actual discharge measurements determined in the study were used to calibrate the monthly WRSM2000 model against rainfall to obtain catchment-specific rainfall-runoff parameters (Pitman et al., 2006). A range of hydrological statistics were used in the RRM-BN model. Findings were used to identify a range of indicators to represent hypothesized causal relationships of the socio-ecological system being evaluated and to identify measures for indicators, with units of measurement and node rank thresholds and relationships between variables in the form of CPTs (refer to Table S2). Netica™ was used to carry out the assessment (Ayre et al., 2014, for example). The tool is versatile and incorporates a range of features used to optimize the assessment. This includes equation features to weight the relative importance of parent variables and generate initial CPTs that were easily refined and applied to the daughter nodes for the assessment. The tool includes case file generation options, which allow the BNs to be linked to Microsoft® Excel, where data can be rapidly analysed and used to populate BNs for the analyses. Risk outcome distributions were also linked to Excel, where scenarios and social and ecological endpoints could be integrated using Monte Carlo randomization approaches that are part of the Oracle Crystal Ball software (Landis, 2004). After establishing BN models for each RR, input parameters were changed using RR-specific data for a range of scenarios including the following.
-
Scenario 1 represents the present-day scenario based on present state hydrology and associated source to endpoint variable state relationships that represent observable conditions. This scenario is based on existing data and additional data collected during the field surveys.
-
Scenario 2 represents a pre-anthropogenic water resource development scenario, considered to represent “natural” hydrology, which was modelled using historical and modelled hydrology and rainfall data, and hypothesized state distributions for non-flow variables. This scenario was selected to calibrate the PROBFLO model for the study.
-
Scenario 3 includes the presence of the new proposed Polihali Dam with full modelled interbasin transfer (IBT) supply. Only large floods overtopping the dam have been considered to be available downstream of the dam, with the existing E-Flows from the downstream lateral tributaries bringing water from Katse and Mohale dams available in RR3 and 4. Non-flow source/stressor catchment conditions were based on the present-day scenario.
-
Scenario 4 is based on scenario 3 but includes E-Flow releases established as 36 % of the natural mean annual runoff (MAR) from the Polihali Dam, with suitable freshet and flood flows. The range of percentages of the MAR considered in these scenarios were selected by stakeholders to evaluate different levels of water resource use for the development.
-
Scenario 5 is based on scenario 3, but with only 25 % of the natural MAR available to contribute towards E-flows, with all floods retained in the Polihali Dam for transfer into the IBT.
-
Scenario 6 is based on scenario 5 with one additional 40 m3 s−1 freshet (small spring flood) released from the dam in addition to the 25 % of the natural MAR to contribute towards E-flows.
-
Scenario 7 is based on scenario 3 with only 18 % of the natural MAR available to contribute towards E-flows with one single 40 m3 s−1 freshet (small spring flood).
-
Scenario 8 is based on scenario 6 but with additional stress imposed by further reduction of available flows to 12 % of the natural MAR, released for maintenance but including the single 40 m3 s−1 freshet (small spring flood).
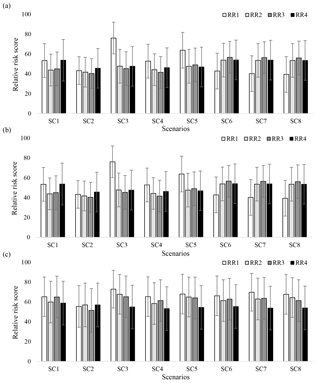
Figure 7Senqu River case study mean relative risk scores (with SD) considered in the assessment including relative risk to: riparian vegetation (a), macro-invertebrates (b) and fish (c) wellbeing endpoints for the four risk regions per scenario (SC).
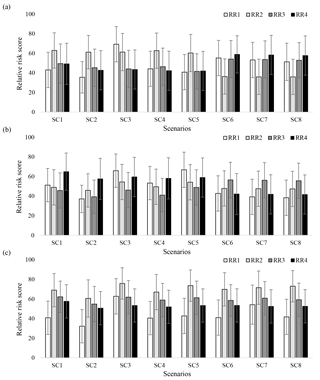
Figure 8Senqu River mean relative risk scores (with SD) for wood for fuel (a), marginal vegetation for livestock grazing (b) and fish for food (c) social endpoints for the four risk regions per scenario (SC) considered in the study.
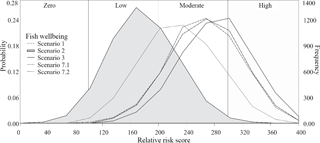
Figure 9Probability profiles generated during a PROBFLO assessment, overlaid onto risk rank range (Zero, Low, Moderate and High) to describe the relative risk of the multiple sources and stressors, including altered flows, associated with alternative management scenarios considered in the Lesotho case study to the fish wellbeing endpoint.
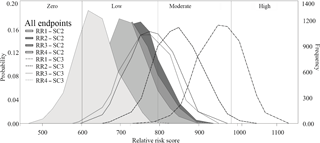
Figure 10Probability profiles generated during a PROBFLO assessment, overlaid onto risk rank range (Zero, Low, Moderate and High) to describe the relative risk of the multiple sources and stressors, including altered flows, associated with alternative management scenarios considered in the Lesotho case study to all of the endpoints integrated in the assessment.
In this assessment, risk was calculated for 12 endpoints, for three temporal periods and for eight scenarios, thus representing 312 BN models that were relatively comparable. The results include the mean relative risk rank scores with associated standard deviation for each endpoint including the maintenance of riparian vegetation, macro-invertebrates and fish wellbeing as ecological endpoints and the maintenance of wood for fuel, marginal vegetation for livestock grazing and fish for food as social endpoints (Figs. 7 and 8). These initial relative mean risk scores allow for the comparison of alternative spatial and temporal socio-ecological risk projections to the endpoints used in the assessment. Initial risk to ecological endpoints compared between the natural (SC2) and present (SC1) scenarios demonstrates that the number of sources and stressors with associated risk to endpoints has increased in the study area, particularly in RR2 to RR4. These changes can largely be attributed to the consequences of Phase I of the LHWP (Fig. 7). These findings include the synergistic effect of non-flow stressors (such as water quality and habitat condition) to the wellbeing of the Senqu River ecosystem in the study area. Effects of the altered hydrology between natural and present-day scenarios to the social endpoints were less obvious (Fig. 8). Spatial trends in the risk results associated with SC3 to SC8 generally include elevated risk to RR1, directly downstream of the proposed dam in particular. These results demonstrate that the impact on socio-ecological endpoints considered will be highest directly below the dam. Thereafter scenarios that exclude floods and freshets (SC3 and SC5) resulted in excessive risk, demonstrating the importance of flood and freshet flows to the socio-ecological endpoints. Outcomes for scenarios 6 to 7 for riparian vegetation and invertebrates include consistent increases in risk spatially from the proposed new dam towards the lower reaches of the study area, which is ascribed to accumulative effects of the existing Phase I dams on the lateral tributaries. The relative risk to the fish community endpoint includes an opposite trend where a reduction in risk from RR1 to RR4 was observed for all scenarios. These results are indicative of the increased relative resilience of the resident and seasonal migratory fish communities to flow alterations in the Senqu River associated with dam developments, due to the increasing size of the river and associated increases in habitat diversity towards the lower reaches of the study area. In addition, reductions in river connectivity (barrier formation) associated with existing impacts from Phase I and the synergistic new stressors associated with Phase II of the LHWP were also shown to contribute to the increase in risk from the lower reaches of the study area in RR4 for fish migrating upstream to RR1. Interestingly the outcomes included improvements to the condition of, or reductions in risk to the wellbeing of social endpoints for scenarios 6 to 8. This included potential improvements in the availability of and/or condition of wood for fuel in RR2 and grazing for livestock for RR1 and RR4 in particular. These projections allow for trade-off considerations where the wellbeing of some social endpoints will decrease in some parts of the study area and increase in others. From our current understanding of the socio-ecological system represented in this study, the results evidence some additional sustainable development opportunities that may be available for stakeholders to consider (Fig. 8). These opportunities may improve the viability of the Phase 2 development and contribute to the establishment of a suitable balance between the use and protection of the ecosystem being evaluated. These relative risk projections to the multiple socio-ecological endpoints considered are based on flow alterations associated with development scenarios, in the context of the exacerbating of non-flow variable determinants on regional scales.
The cumulative risk of all ecological and social endpoints for each RR, for each temporal period, per scenario, were evaluated using Monte Carlo simulations (5000 trials, Oracle Crystal Ball software, Oregon) (Ayre et al., 2014). The outcomes included relative risk projections, displayed as relative profiles to single endpoints from multiple RRs, and multiple social and ecological endpoints or all endpoints per RR in the study for comparisons and evaluation. These profiles were generated for multiple scenarios to evaluate the potential social and ecological consequences of alternative water resource development scenarios. This is demonstrated by considering the cumulative risk projections to the fish wellbeing endpoint, which demonstrates that relative to the “natural” hydrology scenario (Scenario 2), in which there is a 83 % probability that risk to the fish endpoint occurred in a zero to low risk range, for the present scenario (Scenario 1), Phase II with the dam and no E-flows scenario (Scenario 3) and Scenario 7 (Phase II with the dam, 18 % release of natural MAR and 40 m3 s−1 freshets) all range between the moderate and high risk range (Fig. 9). The risk outcomes of all future management options suggest that objectives of the stakeholders to maintain the existing wellbeing of the ecosystem could not be achieved because flow scenarios considered would not satisfactorily mitigate the effects of fish migration barriers, which themselves reduce ecosystem wellbeing. To address this, an additional, amended scenario (Scenario 7) was then modelled, which included successful mitigation measures for the existing man-made barriers in the Senqu River as amendments. The outcomes included a reduction in risk in the low to moderate risk ranges, demonstrating that scenarios that promote moderate to high use of the water resources, with barrier mitigation measures (such as construction of fishways) could result in the achievement of the fish wellbeing endpoints in the study. This approach established for this case study allows for the relative comparison of the integrated social and/or ecological consequences of altered flows in the context of non-flow variables for each scenario for each endpoint used to represent the use and protection management objectives of the study as shown in Fig. 10. In Fig. 10 the integrated risk probability profiles to all endpoints for each RR are shown, which compares Scenario 2 (reference scenario) to the high use Scenario 3. These results include elevated risk probabilities for RR1 (84 % moderate and 15 % high rank range) and RR2 (81 % moderate), while existing E-flows from Phase I dams reduce the risk posed for this scenario in RR3 and RR4. The relative risk results to endpoints and integrated risk profiles were presented to stakeholders who used these outcomes to select E-flows and associated water resource use mitigation measures (such as barrier mitigation measures) to be implemented for Phase II of the LHWP. In this case study E-flows were established by initially evaluating the individual flow tolerance exceedance thresholds for numerous social and ecological indicators used in the BNs. This information resulted in the development of a hydrological scenario matching the shape of the natural hydrology that was evaluated in the BNs. Thereafter the BNs themselves were used to generate the hydrological statistic variable conditions required to achieve a suitable risk profile for each social and ecological endpoint that represents the E-flows for the study in the context of the vision for RRs in the study. With this information, a range of alterative water resource use scenarios were generated and considered to explore risk trade-offs between social and ecological endpoints.
3.6.2 Mara River risk calculation
In the Mara River case study, the relative risk of stressors and the E-flows were established according to the four social and two ecological endpoints considered in the assessment. The Mara River case study (refer to Table S1) was based on existing data from historical surveys (Mati et al., 2008; McCartney, 2010; Majule, 2010; LVBC and WWF-ESARPO, 2010; Mango et al., 2011; Kanga et al., 2011; Defersha and Melesse, 2012; Defersha et al., 2012; GLOWS-FIU, 2012; Dutton et al., 2013; Atisa et al., 2014; Gichana et al., 2014; Kilonzo et al., 2014; McClain et al., 2014) and a single site visit to refine the CPTs (NBI, 2016). During this survey seven sites were selected to represent the variability of all of the RRs in the study area. After establishing BN models for each RR (refer to BN models provided in the Supplement), input parameters were changed using RR-specific data for two scenarios, including the present condition and alternately the E-flow requirement to achieve the basic human needs and ecological wellbeing of the Mara River known as the Ecological Reserve (United Republic of Tanzania, 2009; Government of Kenya, 2002).
In this case study relative risk results were used to generate E-flow requirements that would not pose excessive risk to the wellbeing of ecological endpoints and social endpoints as described by the RQOs. The assessment hypothesizes that sufficient flows currently exist to maintain the endpoints in an acceptable condition. In addition, in the context of the precautionary principle, additional flows can be allocated before risk to the endpoints exceeds acceptable, sustainable thresholds. Results further demonstrate that sustainable water allocations would reduce risk to selected social endpoints selected in the study and meet the desired balance between the use and protection of the resource (Fig. 11). The approach highlighted the probable effect of non-flow-related stressors that are affecting the ecological wellbeing of Mara River, including water physiochemical impacts and habitat alteration stressors associated with urban and rural communities, livestock grazing and watering and the effect of the recent exponential increase in local Hippopotamus amphibius populations in the tributaries of the Mara River in particular that are affecting water quality in the system (Kanga et al., 2011; Subalusky et al., 2015). These results were used to demonstrate the relative risk of sources or water resource activities that affect flows relative to other sources at the risk of ecosystem wellbeing (Fig. 11). The approach successfully demonstrated how the BN-RRM approach in PROBFLO can be used to generate acceptable risk profiles for endpoints to evaluate the socio-ecological consequences of altered flows, and it is also demonstrated how these models can be used to determine E-flows and associated information for water resource use.
3.7 Step 7: Uncertainty evaluation
Best ecological risk assessment practice requires the explicit evaluation of uncertainty, or confidence assessment, (O'Brien and Wepener, 2012; Landis, 2004), which has been incorporated into the PROBFLO approach. Any and all aspects of uncertainty associated with the entire BN-RRM process, including objectives and endpoint selection for the assessment, availability and use of evidence, expert solicitations and model uncertainty, for example, must be addressed. In an effort to reduce uncertainty, the BN-RRM approach adopted by PROBFLO inherently considers uncertainty associated with cause and effect relationships and the use of real data with expert solicitations (Uusitalo, 2006; Landis et al., 2016). The additional incorporation of entropy reduction analysis in relative risk calculations using Monte Carlo simulations also contributes to uncertainty reduction in PROBFLO. Additional analyses of the sensitivity of the BN-RRM should be addressed within the uncertainty evaluation section (Pollino et al., 2007; Hines and Landis, 2014), where the relative influence of input nodes on the endpoints can be evaluated as part of the PROBFLO assessment. The results of the uncertainty assessment are used to provide context to the stakeholders of a PROBFLO assessment and contribute to the decision making process in E-flow assessment studies.
For all of the BNs created in the PROBFLO assessments of the Senqu and Mara River case studies, the sensitivity of the input variables was evaluated in Netica™ using the “Sensitivity to Findings” tool (Marcot, 2012). This approach allows for the relative contribution of each variable to be evaluated. These assessments are used to evaluate model structure and interpret risk result outcomes with the stakeholders of the assessment (Marcot, 2012; Landis et al., 2016). This test demonstrates to both PROBFLO operators and stakeholders where models and associated assessments are sensitive to input data. Evidence to justify these sensitive determinants are imperative to a robust assessment. Additional sources of uncertainty include the comparative availability of evidence and expert knowledge pertaining to the socio-ecological systems considered in the assessments. The Senqu River case study addressed the second phase of a water resource use development that already has two substantial flow-altering developments with more than 15 years of pre- and post-development E-flow assessment (using holistic EFA methods; Arthington et al., 2003) monitoring and evaluations. Additional field surveys of the study area were carried out to generate additional information and to test existing hypotheses for the assessment. The Mara River case study was based largely on available historical information and existing EFA results for parts of the study area (McClain and Kashaigili, 2013; Dessu et al., 2014).
3.8 Step 8: Hypotheses establishment
In the hypotheses establishment step of PROBFLO, suitable hypotheses for field and laboratory experiments are established to test flow–ecosystem and flow–ecosystem service relationships (Landis, 2004; O'Brien and Wepener, 2012). In PROBFLO the fundamental adaptive management approach to improving our understanding of socio-ecological risk relationships, while revisiting outcomes and re-evaluating approaches, is formalized in the hypotheses establishment and testing phase. This process is based on a similar process in the RRM approach, established to reduce uncertainties and to confirm the risk rankings in risk assessments (Landis, 2004). In PROBFLO these adaptive management principles acknowledge that socio-ecological systems are dynamic and that our limited understanding of these processes necessitates the incorporation of many assumptions. In many case studies, uncertainties associated with the outcomes need to be mitigated before they can be used to inform decision making. To reduce uncertainty, assumptions can be tested rigorously and early. The adaptive management processes should (1) be informed by iterative learning about the flow–ecosystem and flow–ecosystem service relationships, (2) consider and respond to earlier management successes and failures and (3) increase present-day socio-ecological system resilience that can improve the ability of E-flows management to respond to the threats of increasing resource use (Lee, 2004).
In the Senqu River case study, many hypotheses associated with the flow–ecosystem and flow–ecosystem service relationships, largely established on data associated with Phase I of the LHWP, were established and tested during the field surveys. These hypotheses included the following. (1) Woody vegetation communities, sustainably harvested by local communities for fuel, respond to reduced average flows by increasing in abundance due to reduced flow variability, reduced stream power and through the colonization of new lower marginal zones. (2) Migratory cyprinid fish respond to ecological cue flows, which include increased discharges associated with reduced salinity, and which initiate fish migration. (3) Grazing for livestock of local communities depends on freshet flows lifting water onto the river banks and floodplains to stimulate vegetation growth. Data were collected from the study area to address these hypotheses and improve the understanding of the flow–ecosystem and flow–ecosystem service relationships considered in the study. In the Mara River case study available flow–ecosystem and flow–ecosystem service information was used in the PROBFLO assessment. A range of hypotheses associated with our understanding of the relationships were generated to refine and improve E-flow assessments of the study area.
3.9 Step 9: Test hypotheses
The two PROBFLO case studies included the design of long-term monitoring programmes to test the accuracy of risk projections and improve the understanding of the flow–ecosystem and flow–ecosystem service relationships. In the Senqu River case study a data management system (DMS) with automated data evaluation components was established. In the Mara River case study a range of hypotheses were established and used to design a monitoring plan and associated research programme to confirm the flow–ecosystem and flow–ecosystem service relationships considered in the study.
3.10 Step 10: Communicate outcomes
Regional-scale ecological risk assessments of water resources are carried out on behalf of stakeholders of the use and/or protection of those resources. Stakeholders need information generated with robust, best scientific practice methodologies in a transparent, clear and concise format, to evaluate the socio-ecological consequences of water resource use options. The PROBFLO approach highlights the importance of communicating the outcomes of assessments in the context of the uncertainty identified in an assessment (Hayes and Landis, 2004). A variety of techniques and tools are available to assist in the communication of the E-flow outcomes and associated socio-ecological consequences of altered flows and careful attention must be paid to ensure that the relevant stakeholders of any case study are presented with information that can easily be understood (O'Brien and Wepener, 2012). In the Senqu River case study, the LHDA with South African and Lesotho governmental delegates participated in a project outcomes workshop in 2014. During this workshop the PROBFLO approach adopted for the study, results and outcomes were discussed. Risk results of sources and stressors to social and ecological endpoints were compared in a relative manner, facilitating water resource use and protection trade-off considerations for the LHWP Phase II. In the Mara River case study the PROBFLO assessment successfully formed a part of the Nile E-flows framework development (NBI, 2016) and the ongoing Mau Mara Serengeti (MaMaSe) Sustainable Water Initiative (http://mamase.org). The application of the PROBFLO and associated uncertainty assessment was used to establish a monitoring plan that should be implemented with water resource use scenarios selected from the case studies. These plans were designed to validate the model by testing the response of the receiving environment to observed ecosystem driver conditions, associated with implemented scenarios, and to improve the understanding of the causal relationships hypothesized in the original assessment with real data.
The regional-scale ecological risk assessment approach was established in 1997 in response to the need to apply ecological risk assessments that consider multiple sources, stressors and receptors in the context of spatial and temporal ecosystem dynamics, on multiple spatial scales (Landis and Wiegers, 1997, 2007). The approach, which includes the RRM, has been widely implemented, reviewed and proven to be a robust probabilistic modelling tool to contribute to the sustainable management of ecological resources (Landis and Wiegers, 2007). Recent developments in E-flow frameworks (Poff et al., 2010; NBI, 2016) now also call for holistic, regional-scale, probabilistic E-flow assessments that consider flow and non-flow drivers of change in socio-ecological contexts. We have established a regional-scale ecological risk assessment method, incorporating the BN-RRM approach, to evaluate the socio-ecological consequences of altered flows and establish E-flows called PROBFLO. PROBFLO is a robust method for E-flow assessments that meets current standards of best scientific practice and can make a positive contribution to the sustainable management of water resources. The approach provides true transparency and adaptability options for holistic E-flow management. PROBFLO has already been successfully implemented in two major case studies where its flexibility and functionality has been demonstrated. In both case studies the evidence-based outcomes facilitated informed environmental management decision making, in the context of social and ecological aspirations. From these outcomes, stakeholders have, in addition, been able to consider sustainable social and ecological trade-offs in an attempt to balance the use and protection of water resources. The PROBFLO outcomes used to direct the sustainable use of water resources in the case studies are probabilistic and need to be validated with monitoring data during implementation phases. PROBFLO is an adaptable tool that allows for the rapid incorporation of new information, which will inform adaptive management and reduce uncertainty associated with the accuracy of the projections. In the case studies, stakeholders were presented with evidence-based probabilistic projections of PROBFLO and used the risk projections to consider water resource use trade-off options. Both of these case studies are being used by stakeholders to make water resource use decisions that are currently being undertaken. PROBFLO is a holistic, evidence-based probability modelling E-flow assessment tool that is transparent and adaptable, and it is suitable for application on multiple spatial scales. PROBFLO has the potential to contribute to the sustainable management of water resources for the benefit of social and ecological components of these systems.
The code used in this paper is available in the Supplement.
The Supplement includes justification tables for the exposure and effects input nodes, and the conditional probability justification tables for the daughter nodes use in the Bayesian Network models in the study. In addition two Netica™ working Bayesian Network models used in the risk assessment for the Mara River and Senqu River case study is provided.
The supplement related to this article is available online at: https://doi.org/10.5194/hess-22-957-2018-supplement.
The BN-RRM approach established for use in E-flow assessments was co-developed by GCO'B, CD and VW. The approach was implemented by this team including LQ, KF, JM, MG and RS. The paper was written by GCO'B and CD and edited by WGL, VW, EH and RS.
The authors declare that they have no conflict of interest.
The PROBFLO E-flow assessment approach was established through the Institute
of Natural Resources NPC (Pietermaritzburg, South Africa) as part of the
LHWP Phase II study funded by LHDA. We acknowledge the contributions made to
the initial Senqu River case study by the extended team of ecological and
social scientists and engineers who worked on the project. Contributions
were also made through the NBI Guidance Document on Environmental Flows
study, prepared by HYDROC GmbH in collaboration with Mau Mara Serengeti
Sustainable Water Initiative study on behalf of the Nile Basin Initiative
and Deutsche Gesellschaft für Internationale Zusammenarbeit (GIZ). In
particular, the contributions made by Michael McClain and John Conallin are
acknowledged. The authors also acknowledge the advice provided by fellow
E-flow assessment scientists that have contributed to the development of
PROBFLO including Jacqueline King, Cate Brown, Rebecca Tharme and Jay O'Keeffe.
Finally, we appreciate the comments on the manuscript provided
during the discussion document phase of the HESS publication process and the
highly valuable comments and suggestions provided by the reviewers of the
manuscript.
Edited by: Stacey Archfield
Reviewed by: two anonymous referees
Acreman, M. C. and Dunbar, M. J.: Defining environmental river flow requirements – a review, Hydrol. Earth Syst. Sci., 8, 861–876, https://doi.org/10.5194/hess-8-861-2004, 2004.
Acreman, M. C., Arthington, A. H., Colloff, M. J., Couch, C., Crossman, N. D., Dyer, F., Overton, I., Pollino, C. A., Stewardson, M. J., and Young, W.: Environmental flows for natural, hybrid, and novel riverine ecosystems in a changing world In a nutshell?, Front. Ecol. Environ., 12, 466–473, https://doi.org/10.1890/130134, 2014.
Anderson, S. and Landis, W.: A pilot application of regional scale risk assessment to the forestry management of the Upper Grande Ronde watershed, Oregon, Hum. Ecol. Risk Assess., 18, 705–732, https://doi.org/10.1080/10807039.2012.688696, 2012.
Arthington, A.: “Environmental Flows: Saving Rivers in the Third Millennium”, University of California Press, Berkeley, CA, 406 pp., 2012.
Arthington, A., Rall, J., and Kennard, M.: Environmental flow requirements of fish in Lesotho Rivers using the DRIFT methodology, River Res, available at: http://onlinelibrary.wiley.com/doi/10.1002/rra.728/abstract (last access: 19 August 2016), 2003.
Atisa, G.: Economic Assessment of Best Management Practices in the Mara River Basin: Toward Implementing Payment forWatershed Services. MSc Thesis Florida International University, Miami, Florida, 135 pp., 2009.
Atisa, G., Bhat, M., and McClain, M.: Economic assessment of best management practices in the Mara River Basin: toward implementing payment for watershed services, Water Resour. Manag., 28, 1751–1766, https://doi.org/10.1007/s11269-014-0585-3, 2014.
Ayre, K. and Landis, W.: A Bayesian approach to landscape ecological risk assessment applied to the Upper Grande Ronde Watershed, Oregon, Hum. Ecol. Risk Assess., 18, 946–970, https://doi.org/10.1080/10807039.2012.707925, 2012.
Ayre, K., Caldwell, C., Stinson, J., and Landis, W.: Analysis of regional scale risk of whirling disease in populations of colorado and rio grande cutthroat trout using a bayesian belief network model, Risk Anal., 34, 1589–1605, 2014.
Bartolo, R., van Dam, R. A., and Bayliss, P.: Regional ecological risk assessment for Australia's tropical rivers: Application of the relative risk model, Hum. Ecol. Risk Assess., 18, 16–46, https://doi.org/10.1080/10807039.2012.631467, 2012.
Biswas, A.: Integrated water resources management: a reassessment: a water forum contribution, Water Int., 29, 248–256, https://doi.org/10.1080/02508060408691775, 2004.
Colnar, A. M. and Landis, W. G.: Conceptual model development for invasive species and a regional risk assessment case study: the European green crab, Carcinus maenas, at Cherry Point, Hum. Ecol. Risk Assess., 13, 120–155, https://doi.org/10.1080/10807030601105076, 2007.
Defersha, M. and Melesse, A. M.: Field-scale investigation of the effect of land use on sediment yield and runoff using runoff plot data and models in the Mara River basin, Kenya, Catena, 89, 54–64, 2012.
Defersha, M., Melesse, A. M., and McClain, M. E.: Watershed scale application of WEPP and EROSION 3D models for assessment of potential sediment source areas and runoff flux in the Mara River Basin, Kenya, Catena, 95, 63–72, https://doi.org/10.1016/j.catena.2012.03.004, 2012.
Department of Water Affairs (DWA): Procedures to Develop and Implement Resource Quality Objectives, Report, available at: http://inr.org.za/wp-content/uploads/2014/09/dwa-rqo-report_march-2011.pdf, 2011.
Dessu, S., Melesse, A., Bhat, M., and McClain, M.: Assessment of water resources availability and demand in the Mara River Basin, Catena, 115, 104–114, https://doi.org/10.1016/j.catena.2013.11.017, 2014.
Dudgeon, D.: Threats to freshwater biodiversity in a changing world, Global Environmental Change, 1, 243–253, https://doi.org/10.1007/978-94-007-5784-4_108, 2014.
Dudgeon, D., Arthington, A. H., Gessner, M. O., Kawabata, Z.-I., Knowler, D. J., Lévêque, C., Naiman, R. J., Prieur-Richard, A.-H., Soto, D., Stiassny, M. L. J., and Sullivan, C. A.: Freshwater biodiversity: importance, threats, status and conservation challenges, Biol. Rev. Camb. Philos. Soc., 81, 163–82, https://doi.org/10.1017/S1464793105006950, 2006.
Dutton, C., Anisfeld, S., and Ernstberger, H.: A novel sediment fingerprinting method using filtration: application to the Mara River, East Africa, J. Soils Sediments, 13, 1708–1723, 2013.
East African Community (EAC): Memorandum of Understanding (MoU) with the World Wide Fund for Nature Eastern Africa Regional Programme Office (WWF EARPO) for WWF-EARPO to support the EAC in programmes contributing to the vision of the Lake Victoria Development Programme and Established Lake Victoria Basin Commission, Kampala, Uganda, 2003.
Gichana, Z., Njiru, M., Raburu, P., and Masese, F.: Effects Of Human Activities On Microbial Water Quality In Nyangores Stream, Mara River Basin, Int. J. Sci. Technol. Res., 3, 153–157, 2014.
GLOWS-FIU: Environmental Flow Recommendations for the Mara River, Kenya and Tanzania, Global Water for Sustainability Program (GLOWS), Miami, FL, 2012.
Government of Kenya: Kenya Water Act No. 8 of 2002, Government of Kenya, 2002.
Grafton, R., Pittock, J., Davis, R., Williams, J., and Fu, G.: Global insights into water resources, climate change and governance, Nat. Clim. Change, 3, 315–321, https://doi.org/10.1038/nclimate1746, 2013.
Growns, I.: The influence of changes to river hydrology on freshwater fish in regulated rivers of the Murray–Darling basin, Hydrobiologia, 596, 203–211, https://doi.org/10.1007/s10750-007-9097-y, 2008.
Hart, B. and Pollino, C.: Increased use of Bayesian network models will improve ecological risk assessments, Hum. Ecol. Risk Assess., 14, 851–853, https://doi.org/10.1080/10807030802235037, 2008.
Hayes, E. and Landis, W.: Regional ecological risk assessment of a near shore marine environment: Cherry Point, WA, Hum. Ecol. Risk Assess., 10, 299–325, https://doi.org/10.1080/10807030490438256, 2004.
Herring, C. E., Stinson, J., and Landis, W. G.: Evaluating non-indigenous species management in a Bayesian networks derived relative risk framework for Padilla Bay, Washington, Integr. Environ. Assess. Manag., 11, 640–652, 2015.
Hines, E. and Landis, W.: Regional risk assessment of the Puyallup River Watershed and the evaluation of low impact development in meeting management goals, Integr. Environ. Assess., 10, 269–278, https://doi.org/10.1002/ieam.1509, 2014.
Hoffman, C. M.: Geospatial Mapping and Analysis of Water Availability-Demand-Use Within the Mara River Basin, Florida International University, Miami, 2007.
Hoffman, C. M., Melesse, A. M., and McClain, M. E.: Geospatial mapping and analysis of water availability-demand-use within the Mara River Basin, in: Melesse, A. M., Nile River Basin: Hydrology, Climate and Water Use, Springer, Dordrecht, New York, 359–382, 2011.
Hunsaker, C. T., Graham, R. L., Suter II, G. W., O'Neill, R. V., Barnthouse, L. W., and Gardner, R. H.: Assessing ecological risk on a regional scale, Environ. Manage., 14, 325–332, 1990.
Isaak, D., Wollrab, S., Horan, D., and Chandler, G.: Climate change effects on stream and river temperatures across the northwest US from 1980–2009 and implications for salmonid fishes, Clim. Change, 113, 499–524, 2012.
Kanga, E., Ogutu, J., Olff, H., and Santema, P.: Population trend and distribution of the Vulnerable common hippopotamus Hippopotamus amphibius in the Mara Region of Kenya, Oryx, 45, 20–27, 2011.
Karanja, G.: Tourist Impacts in Masai Mara National Reserve, University of Kent at Canterbury, ISNI: 0000 0001 3594 9345, 2002.
Karanja, G.: Tourism impacts in Masai Mara National Reserve, in: Walpole, edited by: Karanja, M. J., Sitati, G., and Leader-Williams, N., Wildlife and People: Conflict and Conservation in Masai Mara, IIED, London, 5–16, 2003.
Kiambi, S., Kuloba, B., Kenana, L., Muteti, D., and Mwenda, E.: Wet Season Aerial Count of Large Herbivores inMasai Mara National Reserve and the Adjacent Community Areas (2010), Mara Research Station, Kenya Wildlife Service, Narok, Kenya, 2012.
Kilonzo, F., Masese, F. O., Van Griensven, A., Bauwens, W., Obando, J., and Lens, P. N. L.: Spatial–temporal variability in water quality and macro-invertebrate assemblages in the Upper Mara River basin, Kenya, Phys. Chem. Earth, 67–69, 93–104, 2014.
King, J. and Pienaar, H. (Eds.): Sustainable use of South Africa's inland waters, Water Research Commission, Technical report, available at: http://www.wrc.org.za/Knowledge Hub Documents/Research Reports/TT 491-11.pdf, 2011.
King, J., Brown, C., and Sabet, H. A scenario-based holistic approach to environmental flow assessments for rivers, River Res. Appl., 19, 619–639, 2003.
Korb, K. and Nicholson, A.: Bayesian artificial intelligence, 2nd edn., CRC Press, Boca Raton, FL, USA, 451 pp., 2010.
Lamprey, R. H. and Reid, R. S.: Expansion of human settlement in Kenya's Maasai Mara: what future for pastoralism and wildlife?, J. Biogeogr., 31, 997–1032, 2004.
Landis, W. (Ed.): Regional scale ecological risk assessment: using the relative risk model, CRC Press, Boca Raton, FL, USA, 309 pp., 2004.
Landis, W.: Comment on ET&C perspectives, November 2015 – A holistic view, Environ. Toxicol. Chem., 35, 1337–1339, https://doi.org/10.1002/etc.3378, 2016.
Landis, W. and Wiegers, J.: Design considerations and a suggested approach for regional and comparative ecological risk assessment, Hum. Ecol. Risk Assess., 3, 287–297, 1997.
Landis, W. and Wiegers, J.: Ten years of the relative risk model and regional scale ecological risk assessment, Hum. Ecol. Risk Assess., 13, 25–38, 2007.
Landis, W. G., Ayre, K. K., Johns, A. F., Summers, H. M., Stinson, J., Harris, M. J., Herring, C. E., and Markiewicz, A. J.: The multiple stressor ecological risk assessment for the mercury contaminated South River and upper Shenandoah River using the Bayesian Network-Relative Risk Model, Integr. Environ. Assess. Manag., 13, 85–99, https://doi.org/10.1002/ieam.1758, 2016.
Lee, K. N.: Appraising Adaptive Management, Conserv. Ecol., 3, 1–18, 2004.
Lesotho Highlands Water Project (LHWP): Treaty on the Lesotho highlands water project between the government of the Kingdom of Lesotho and the government of the Republic of South Africa signed at Maseru, 24 October 1986.
Lesotho Highlands Water Project (LHWP): Agreement on Phase II of the Lesotho highlands water project between the government of the Kingdom of Lesotho and the government of the Republic of South Africa signed at Maseru, 11 August 2011.
Lesotho Highlands Water Project (LHWP): Specialist Consultants to Undertake Baseline Studies (Flow, Water Quality and Geomorphology) and Instream Flow Requirement (IFR) Assessment for Phase 2: Instream Flow Requirements for the Senqu River – Final report No. 6001/2/e, Lesotho Highlands Development Authority, Maseru, 2016.
LVBC & WWF-ESARPO: Biodiversity Strategy and Action Plan for Sustainable Management of the Mara River Basin, Nairobi and Kisumu, Kenya, 2010 Lake Victoria Basin Commission and WWF-ESARPO, 2010.
Majule, A.: Towards sustainable management of natural resources in the Mara river basin in Northeast Tanzania, J. Ecol. Nat. Environ., 2, 213–224, 2010.
Mango, L. M., Melesse, A. M., McClain, M. E., Gann, D., and Setegn, S. G.: Land use and climate change impacts on the hydrology of the upper Mara River Basin, Kenya: results of a modeling study to support better resource management, Hydrol. Earth Syst. Sci., 15, 2245–2258, https://doi.org/10.5194/hess-15-2245-2011, 2011.
Marcot, B.: Metrics for evaluating performance and uncertainty of Bayesian network models, Ecol. Modell., 230, 50–62, 2012.
Marcot, B. G., Steventon, J. D., Sutherland, G. D., and Mccann, R. K.: Guidelines for developing and updating Bayesian belief networks applied to ecological modeling and conservation, Can. J. For. Res., 36, 3063–3074, 2006.
Mati, B., Mutie, S., Gadain, H., and Home, P.: Impacts of land-use/cover changes on the hydrology of the transboundary Mara River, Kenya/Tanzania, Lakes & Reservoirs: Research & Management, 13, 169–177, 2008.
McCann, R. K., Marcot, B. G., and Ellis, R.: Bayesian belief networks: applications in ecology and natural resource management, 36, 3053–3062, 2006.
McCartney, B.: Evaluation of Water Quality and Aquatic Ecosystem Health in the Mara River Basin East Africa, Masters thesis, Florida International University, available at: http://dpanther.fiu.edu/sobek/content/FI/MA/00/00/17/00001/FIMA000017.pdf, 2010.
McClain, M. and Kashaigili, J.: Environmental flow assessment as a tool for achieving environmental objectives of African water policy, with examples from East Africa, Int. J. Water Resour. D, 29, 650–665, 2013.
McClain, M. E., Subalusky, A. L., Anderson, E. P., Dessu, B., Melesse, A. M., Ndomba, P. M., Joseph, O. D., Tamatamah, R. A., Mligo, C., Mcclain, M. E., Subalusky, A. L., Anderson, E. P., Dessu, B., Melesse, A. M., Ndomba, P. M., Mtamba, J. O. D., and Rashid, A.: Comparing flow regime, channel hydraulics, and biological communities to infer flow–ecology relationships in the Mara River of Kenya and Tanzania, Hydrol. Sci. J., 59, 801–819, https://doi.org/10.1080/02626667.2013.853121, 2014.
Mitchell, B.: Integrated water resource management, institutional arrangements, and land-use planning, Environ. Plann. A, 37, 1335-1352, https://doi.org/10.1068/a37224, 2005.
Moraes, R., Landis, W., and Molander, S.: Regional risk assessment of a Brazilian rain forest reserve, Hum. Ecol. Risk Assess., 8, 1779–1803, 2002.
Murray, S., Foster, P., and Prentice, I.: Future global water resources with respect to climate change and water withdrawals as estimated by a dynamic global vegetation model, J. Hydrol., 448–449, 14–29, https://doi.org/10.1016/j.jhydrol.2012.02.044, 2012.
NBI: Preparation of NBI Guidance Document on Environmental Flows: Nile E-flows Framework Technical Implementation Manual, HYDROC GmbH on behalf of the Nile Basin Initiative and Deutsche Gesellschaft für Internationale Zusammenarbeit, 2016.
O'Brien, G. and Wepener, V.: Regional-scale risk assessment methodology using the Relative Risk Model (RRM) for surface freshwater aquatic ecosystems in South Africa, Water SA, 38, 153–166, https://doi.org/10.4314/wsa.v38i2.1, 2012.
Ogutu, J. O., Owen-Smith, N., Piepho, H. P., and Said, M. Y.: Continuing wildlife population declines and range contraction in the Mara region of Kenya during 1977–2009, J. Zool., 285, 99–109, 2011.
Onjala, J. O.: Managing Water Scarcity in Kenya: Industrial Response to Tariffs and Regulatory Enforcement, PhD Dissertation Thesis Roskilde University, Copenhagen, Denmark, 329 pp., 2002.
Orange-Senqu River Commission (ORASECOM): Integrated Water Resources Management Plan For The Orange-Senqu River Basin: Main Report, Report No. ORASECOM 019/2014, Orange-Senqu River Basin Commission, 2014.
Pahl-Wostl, C., Arthington, A., Bogardi, J., Bunn, S. E., Hoff, H., Lebel, L., Nikitina, E., Palmer, M., Poff, L. N., Richards, K., Schlüter, M., Schulze, R., St-Hilaire, A., Tharme, R., Tockner, K., and Tsegai, D.: Environmental flows and water governance: Managing sustainable water uses, Curr. Opin. Environ. Sustain., 5, 341–351, https://doi.org/10.1016/j.cosust.2013.06.009, 2013.
Pitman, W. V., Bailiey, A. K., and Kakebeeke, J. P.: WRSM2000 Water Resources Simulation Model for Windows: Users Guide, Department of Water Affairs and the Water Research Commission, 2006.
Poff, N. and Matthews, J.: Environmental flows in the Anthropocence: past progress and future prospects, Curr. Opin. Environ. Sustain., 5, 667–675, 2013.
Poff, N. L., Richter, B. D., Arthington, A. H., Bunn, S. E., Naiman, R. J., Kendy, E., Acreman, M., Apse, C., Bledsoe, B. P., Freeman, M. C., Henriksen, J., Jacobson, R. B., Kennen, J. G., Merritt, D. M., O'Keeffe, J. H., Olden, J. D., Rogers, K., Tharme, R. E., and Warner, A.: The ecological limits of hydrologic alteration (ELOHA): A new framework for developing regional environmental flow standards, Freshw. Biol., 55, 147–170, https://doi.org/10.1111/j.1365-2427.2009.02204.x, 2010.
Pollino, C. A., Woodberry, O., Nicholson, A., Korb, K., and Hart, B. T.: Parameterisation and evaluation of a Bayesian network for use in an ecological risk assessment, Environ. Modell. Softw., 22, 1140–1152, https://doi.org/10.1016/j.envsoft.2006.03.006, 2007.
Richter, B. D., Warner, A. T., Meyer, J. L., and Lutz, K.: A collaborative and adaptive process for developing environmental flow recommendations, River Res. Appl., 22, 297–318, https://doi.org/10.1002/rra.892, 2006.
Smit, N. J., Vlok, W., van Vuren, J. H. J., Du Preez, L. H., Van Eeden, E., O'Brien, G. C., and Wepener, V.: Socio-ecological System Management of the Lower Phongolo River and Floodplain Using Relative Risk Methodology, Water Resarch Commission, ISBN: 978-1-4312-0811-1, 2016.
Stirzaker, R., Biggs, H., Roux, D., and Cilliers, P.: Requisite simplicities to help negotiate complex problems, Ambio, 39, 600–607, 2010.
Subalusky, A. L., Dutton, C. L., Rosi-Marshall, E. J., and Post, D. M.: The hippopotamus conveyor belt: vectors of carbon and nutrients from terrestrial grasslands to aquatic systems in sub-Saharan Africa, Freshw. Biol., 60, 512–525, 2015.
Tharme, R.: A global perspective on environmental flow assessment: emerging trends in the development and application of environmental flow methodologies for rivers, River Res. Appl., 19, 397–441, 2003.
United Republic of Tanzania: Water Resources Management Act, ISSN: 0856-033IX, 73 pp., 2009.
Uusitalo, L.: Advantages and challenges of Bayesian networks in environmental modelling, Ecol. Model., 203, 312–318, https://doi.org/10.1016/j.ecolmodel.2006.11.033, 2006.
Vörösmarty, C. J., McIntyre, P. B., Gessner, M. O., Dudgeon, D., Prusevich, A., Green, P., Glidden, S., Bunn, S. E., Sullivan, C. A., Reidy Liermann, C., and Davies, P. M.: Global Threats to Human Water Security and River Biodiversity, Nature, 467, 555–561, 2010.
Walker, R., Landis, W., and Brown, P.: Developing a regional ecological risk assessment: A case study of a Tasmanian agricultural catchment, Hum. Ecol. Risk Assess., 7, 417–439, doi:10.1080/20018091094439, 2001.
Wiegers, J., Feder, H., and Mortensen, L.: A Regional Multiple-Stressor Rank-Based Ecological Risk Assessment for the Fjord of Port Valdez, Alaska, Hum. Ecol. Risk Assess., 4, 1125–1173, 1998.
WRMA: Catchment Management Strategy: Lake Victoria South Catchment Area, Kenya, Water Resources Management Authority of Kenya, 2014.