the Creative Commons Attribution 4.0 License.
the Creative Commons Attribution 4.0 License.
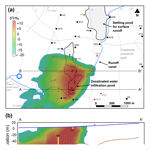
Managed aquifer recharge with reverse-osmosis desalinated seawater: modeling the spreading in groundwater using stable water isotopes
Yonatan Ganot
Ran Holtzman
Noam Weisbrod
Anat Bernstein
Hagar Siebner
Yoram Katz
Daniel Kurtzman
The spreading of reverse-osmosis desalinated seawater (DSW) in the Israeli coastal aquifer was studied using groundwater modeling and stable water isotopes as tracers. The DSW produced at the Hadera seawater reverse-osmosis (SWRO) desalination plant is recharged into the aquifer through an infiltration pond at the managed aquifer recharge (MAR) site of Menashe, Israel. The distinct difference in isotope composition between DSW (δ18O = 1.41 ‰; δ2H = 11.34 ‰) and the natural groundwater (δ18O = −4.48 ‰ to −5.43 ‰; δ2H = −18.41 ‰ to −22.68 ‰) makes the water isotopes preferable for use as a tracer compared to widely used chemical tracers, such as chloride. Moreover, this distinct difference can be used to simplify the system to a binary mixture of two end-members: desalinated seawater and groundwater. This approach is validated through a sensitivity analysis, and it is especially robust when spatial data of stable water isotopes in the aquifer are scarce. A calibrated groundwater flow and transport model was used to predict the DSW plume distribution in the aquifer after 50 years of MAR with DSW. The results suggest that after 50 years, 94 % of the recharged DSW was recovered by the production wells at the Menashe MAR site. The presented methodology is useful for predicting the distribution of reverse-osmosis desalinated seawater in various downstream groundwater systems.
- Article
(5139 KB) - Full-text XML
-
Supplement
(95 KB) - BibTeX
- EndNote
Desalinated seawater global production is projected to double by 2040 while extending its geographical extent (Hanasaki et al., 2016). In some regions, desalinated seawater (DSW) is already the main source for fresh water (Dawoud, 2005). In Israel, for example, DSW reached 66 % of the domestic and industrial fresh water supply in 2017 (Israel Water Authority, 2018). This growing use of DSW affects downstream water systems such as reservoirs (Ronen-Eliraz et al., 2017; Negev et al., 2017; Stuyfzand et al., 2017; Ganot et al., 2017, 2018), wastewater treatment plants (Lahav et al., 2010; Negev et al., 2017) and agricultural irrigation (Lahav et al., 2010; Yermiyahu et al., 2007). One direct way by which DSW use affects the water budget is managed aquifer recharge (MAR). MAR using different water sources has been practiced for over 5 decades as part of the integrated water resource management of Israel (Dreizin et al., 2008; Gvirtzman, 2002) and is becoming a major component of water management in many Mediterranean countries (Rodríguez-Escales et al., 2018). Excess DSW produced in Israel due to operational constraints made it an attractive alternative source for MAR, raising the need to understand its effect. While the relatively rapid hydrological and geochemical processes (timescales of hours to weeks) of this new MAR activity were recently monitored and modeled (Ganot et al., 2017, 2018; Ronen-Eliraz et al., 2017), the potential long-term (months to decades) impact of this process on the natural aquifer is still unknown, lacking observations and quantitative studies.
Stable water isotopes 18O and 2H are excellent tracers for water generated by seawater reverse-osmosis (SWRO) desalination. The lack of fractionation during the reverse-osmosis process, in contrast with various isotope-fractionation processes occurring in natural, fresh water (Al-Basheer et al., 2017; Gat, 1996; Kloppmann et al., 2008a, b), is the cause of the distinct difference in isotope composition between reverse-osmosis DSW and groundwater (GW) originating from natural, fresh water (Ganot et al., 2018; Kloppmann et al., 2018; Negev et al., 2017). For example, the advantage of using 18O and 2H as a quantitative tool for tracing treated wastewater (originating from DSW) that mixes with GW was recently demonstrated by comparing the mixing ratios of chloride, carbamazepine and water isotopes in the soil aquifer treatment (SAT) site at the Shafdan MAR system, Israel (Negev et al., 2017).
Here, we use stable water isotopes to trace the spreading of DSW in the aquifer and the production wells within the MAR site of Menashe, Israel. The DSW is produced at the Hadera SWRO desalination plant, which has operated since 2010 with an annual production capacity of 130 million cubic meters (MCM). It is one of five large SWRO desalination plants (production capacity ≥90 MCM, per year per plant) that were built along the Mediterranean coast of Israel during 2005–2015 (Stanhill et al., 2015). The DSW is regularly supplied directly to consumers through the centralized national water system. Periodically, operational constraints, such as maintenance of the national system, prohibit distribution of the DSW; limited reservoir capacity makes storage of this expensive surplus of DSW in the aquifer through MAR operations the only feasible solution (Ganot et al., 2017, 2018; Ronen-Eliraz et al., 2017).
There are currently only a few places that are practicing MAR with DSW, but this practice is expected to grow due to the increasing use of DSW globally. Practically, most of the known case studies of MAR with DSW involve brackish-water aquifers (mainly in the Persian Gulf countries) and not necessarily reverse-osmosis DSW. In this work we present a unique case study that explores the spreading of a reverse-osmosis DSW plume in a fresh-water aquifer. The use of two isotope-distinguished end-members, in this case, reverse-osmosis DSW and natural, fresh GW, is a prerequisite to implement the analysis presented in this paper.
Predicting the long-term DSW distribution in the aquifer and the production wells is the main objective of this study. We incorporate water isotope data of 18O and 2H in a regional GW flow and transport model (e.g., Boronina et al., 2005; Krabbenhoft et al., 1990; Liu et al., 2014; Reynolds and Marimuthu, 2007; Stichler et al., 2008) in order to predict the DSW distribution in the aquifer. While the methodology for measuring the present mixing of DSW and GW was reported previously (Negev et al., 2017), in the current study our GW modeling approach allows us to predict future mixing trends in the production wells of the Menashe MAR site. Predicting the DSW distribution in the aquifer is of main interest from water quantity (estimating the recovery potential of DSW originating from MAR) and quality perspectives (e.g., Birnhack et al., 2011; Ganot et al., 2018 and references therein).
2.1 Study area
The Menashe MAR site is located on sand dunes 28 m above sea level in the northern part of the Israeli coastal aquifer, an unconfined sandy aquifer stretching over an area of 2000 km2 along the Mediterranean coast (Fig. 1a). The local climate is Mediterranean, with an annual average temperature of 20.2 ∘C, and annual mean precipitation of 566 mm yr−1 (Israel Meteorological Service, 2014). The aquifer thickness varies from 100 m on the coastline (to the west of the Menashe site) to a few meters in the east. It is composed of Pleistocene calcareous sandstone interleaved with discontinuous marine and continental silt and clay lenses. Thick Neogene clay (Saqiye Group), which is highly impermeable, underlies the aquifer (Kurtzman et al., 2012). The regional groundwater level is ∼3 m a.m.s.l. (September 2014, Israel Water Authority, 2014), and the characteristic aquifer properties are a hydraulic conductivity of 10 m d−1, storativity of 0.25 and porosity of 0.4 (Shavit and Furman, 2001).
The Menashe MAR site diverts the natural ephemeral flows from the Menashe Heights streams into a settling pond, and from there to three infiltration ponds. Production wells that encircle the site recover the recharged water from the aquifer (Sellinger and Aberbach, 1973). In the last few years, the southern infiltration pond is dedicated for the infiltration of a surplus of DSW from the Hadera SWRO desalination plant, located 4 km to the west, on the coastline (Fig. 1b).
2.2 Water sampling
Groundwater from 14 wells at the Menashe MAR site were sampled biannually during 2015 to 2017 (n=42). In addition, water was sampled from the infiltration pond DSW inlet pipe (n=3), a few locations inside the pond during MAR events (n=4), shallow observation wells (OA and OB; n=11) and runoff canals (n=1). Stable water isotopes (expressed as δ18O and δ2H in ‰ vs. the VSMOW – Vienna Standard Mean Ocean Water) were measured by a cavity ring-down spectroscopy (CRDS) analyzer (L2130-i, Picarro).
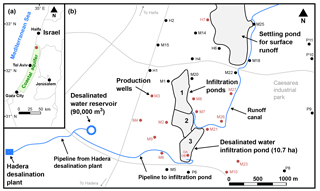
Figure 1Map of the study area. (a) Location of the Israeli coastal aquifer and the Menashe MAR site (red circle). (b) The Menashe MAR site. A surplus of desalinated seawater is delivered from the Hadera SWRO desalination plant (lower left) to the southern infiltration basin (pond 3). The red dots represent wells that were sampled for water isotope analysis.
2.3 Groundwater flow and transport model
A detailed three-dimensional transient water flow and solute transport model was set up in order to estimate DSW spreading in the aquifer at the Menashe site area. The model covers an area of 65 km2, including a western offshore strip of 9 km2 (Fig. 2a). The geological data processed from well logs, geological maps and structural maps served as the basis for the conceptual model, constructed via the GMS software package (version 10.3; https://www.aquaveo.com, last access: 22 November 2018). The variety of rock types was grouped into four hydrogeological units, each characterized by a set of hydrological properties (Table 1). Over 100 well logs were analyzed using the T-PROGS software (Carle, 1999) and provided the spatial distribution of the hydrogeological units. This geostatistically generated unit array, conditioned to the borehole logs, was combined with structural map data of the major marine clay lenses present in the aquifer. The resulting model hence reflects the hydrogeological units' proportions and transition trends as well as the division into sub-aquifers by marine clay within the western part of the aquifer (Fig. 2b, c).
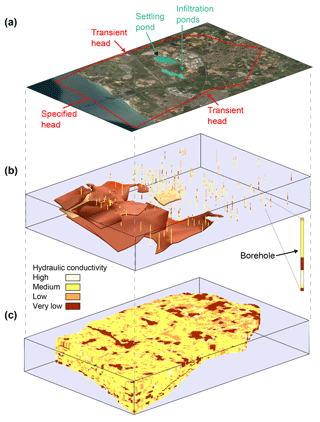
Figure 2The model used in simulations of water flow and solute transport. (a) The modeled area and boundary conditions. (b) The major continuous marine clay lenses and the borehole log. (c) The combined deterministic and geostatistically generated material array representing the aquifer in the model.
The model domain was discretized horizontally into 70×70 m mesh cells. The vertical section of the aquifer, with thickness ranging 50–100 m from east to west, was divided into 24 layers with a vertical spatial resolution of 5 m or smaller. The model's bottom boundary was defined by the impermeable Saqiye Group underlying the aquifer. The model's top boundary was defined by the water table representing an unconfined aquifer. Boundary conditions along the northern, eastern and southern model boundaries were set to be of a transient head type, based on periodic water level measurements. The western boundary was set to a constant head boundary dictated by the sea level. Initial conditions were based on static heads measured at several dozens of production and observation wells included in the model. Sources and sinks in the flow model include recharge by precipitation, MAR (both runoff and DSW recharge) and production wells. Natural recharge from precipitation was based on adjacent rain gauge measurements (Gan Shmuel), using an average recharge coefficient of 0.4 (which is representative of sands). Recharge flux of DSW by MAR activity was calculated by a variably saturated model of the upper 30 m of the sediment under the southern infiltration pond (Ganot et al., 2017). Pumping activity of the production wells was based on a database from the national water company of Israel, Mekorot.
The transport model considers the stable water isotopes 18O and 2H as conservative tracers, i.e., neglecting isotope fractionation (there is strong evidence that local groundwater tends to be isotopically uniform, see for example Krabbenhoft et al., 1990 and references therein). We normalize the tracer concentration as , where δ is the isotope composition of δ18O or δ2H in the aquifer, and δmin and δmax are the minimum and maximum isotope composition, respectively. Since practically δmax=δDSW, the normalized concentration of DSW is CDSW=1, whereas that of GW ranges from CGW=0 (δ18O = −5.43 ‰, δ2H = −22.68 ‰) to CGW=0.13 (δ18O = −4.48 ‰, δ2H = −18.41 ‰). Boundary conditions of the transport model are of specified mass flux (qC, where q is the specific discharge), with zero flux at the bottom boundary (considered impermeable) as well as zero flux at the northern, eastern and southern boundaries, and also with the precipitation and the runoff-pond source terms, due to their GW isotope compositions (CGW=0). Mass flux with the DSW isotope composition (CDSW=1) is given at the western boundary (sea) and the DSW infiltration pond source term. The validity of the use of a single value (CGW=0) for the GW mass-flux boundaries, in light of the range of isotope compositions in the aquifer prior to the MAR of DSW (δ18O = −4.48 ‰ to −5.43 ‰ and δ2H = −18.41 ‰ to −22.68 ‰), is discussed in Sect. 3.2.3. Initial conditions were set by interpolating the water isotope data from several production wells.
The MODFLOW (Harbaugh et al., 2000) and MT3DMS (Zheng and Wang, 1999) codes were used through the GMS user interface to numerically solve the flow and transport models, respectively. Both codes, which use a finite difference scheme, are considered reliable and are therefore widely used for regional aquifer modeling (Zhou and Li, 2011). The flow and transport model was calibrated using a dataset from 2015 to 2017. During 2015, 2016 and 2017 a volume of 2.6, 1.3 and 0.6 MCM of DSW were recharged, respectively, at the Menashe MAR site. In these years the MAR events consisted of a noncontinuous discharge of DSW to pond 3 (Fig. 1b) during January and/or February (Ganot et al., 2017, 2018). In addition, a volume of 3.2 and 1.6 MCM of runoff water were discharged to the settling pond during 2015 and 2017, respectively.
3.1 Water isotopes
The distinct difference between the water isotopes of the production wells and DSW is shown in a δ2H vs. δ18O diagram for the period of 2015 to 2017 (Fig. 3a and Table S1 in the Supplement). During 2016, and more prominently in 2017, few wells showed a progressive change in composition towards higher isotope values – a transition from GW towards DSW on the mixing line (Fig. 3a) indicates mixing with DSW – while most wells retain a constant isotope composition. Note that for all samples in Fig. 3a, there is a strong linear correlation between δ18O and δ2H (R2=0.9991); thus, hereafter we only report δ2H as a tracer.
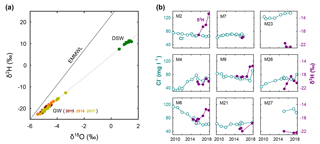
Figure 3(a) Water isotopic composition of the production wells (GW) and reverse-osmosis desalinated seawater (DSW); the eastern Mediterranean meteoric water line (EMMWL) is shown for comparison (Gat and Dansgaard, 1972). (b) Chloride (Cl−) and δ2H sampled in nine production wells at the Menashe MAR site.
The isotope composition of δ2H and the concentration of chloride are shown for comparison in nine wells during the years 2010 to 2018 (Fig. 3b). The chloride concentration of DSW at the Menashe MAR site is always lower than 10 mg L−1 (Ganot et al., 2018), while in the local GW it is found in a wider range of 40 to 140 mg L−1. The large chloride concentration variability in the different wells prior to MAR with DSW (before 2015) suggests that various water sources feed the aquifer (as there is no extensive soluble salt layer in the aquifer according to the recent available geological data). Moreover, the breakthrough of DSW in wells M2, M6 and M9, captured by an increase in δ2H, is not reflected in the chloride concentration (expected to decrease). This implies that chloride – in general a widely used conservative tracer – is less sensitive to reverse-osmosis DSW in natural, fresh GW systems and therefore less useful for its detection.
Finally, we note that the very different DSW signature, in terms of δ2H from the other water sources in the Menashe site, reduces the problem of mixing various water sources to a binary system: (i) DSW and (ii) all other natural sources. This is because the signatures of runoff water (δ18O = −4.77 ‰ and δ2H = −19.5 ‰) and rainwater (δ18O = −5.8 ‰ and δ2H = −19.9 ‰; Gat and Dansgaard, 1972; Goldsmith et al., 2017) are very similar to that of the local GW. Therefore, the binary system approach used in this study, which is based on conservative water isotope tracers is superior to both conservative and nonconservative chemical tracers.
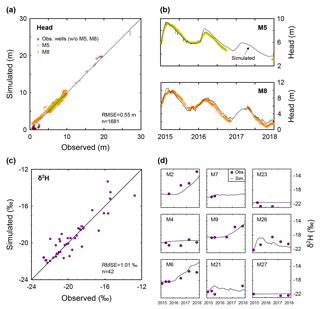
Figure 4Model calibration. (a) Comparison of the simulated and observed hydraulic head. (b) Temporal variations of the simulated and observed hydraulic head in wells M5 and M8. (c) Comparison of the simulated and observed δ2H. (d) Temporal variations of the simulated and observed δ2H in nine selected wells.
3.2 Model
3.2.1 Calibration
The flow model was calibrated against head data from 13 wells (Fig. 4a). We mainly used continuous head data measured at two production wells, M5 and M8 (Fig. 4b). Well M5, situated 400 m SE of pond 3, exploiting aquifer layers bounded between −16 and −54 m MSL, was inactive during 2015–2017, making it ideal for head monitoring. Well M8, situated 1 km north of pond 3, exploiting aquifer layers bounded between −14 and −48 m MSL, was used for production during some of the study period, and thus only selected head data (representing quasi-static heads) were used for calibration.
The transport model was calibrated against isotope data from 12 wells (M2–4, M6–10, M21, M23 and M26–27; Fig. 4c). Specifically, we used data corresponding to the breakthrough of DSW in the down-gradient (western) production wells near the DSW infiltration pond (M2, M6, and M9) since other wells showed smaller δ2H variations (Fig. 4d). The simulated groundwater heads and δ2H for the calibration period are generally in good agreement with the observations. The calibrated hydrological parameters are specified in Table 2.
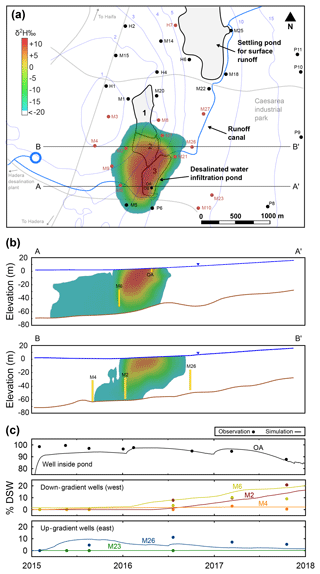
Figure 5Simulation results showing DSW spreading at the end of 2017. (a) Plan view (water table); the colored area shows the DSW plume, the white area indicates natural GW (δ2H < −20 ‰) and the blue contours are the GW head. (b) East–west cross sections through wells OA and M6 (A-A′) and wells M4, M2 and M26 (B-B′); well screens are shown in yellow. (c) Observed and simulated DSW fraction (%) in selected wells along the cross sections A-A′ and B-B′.
3.2.2 DSW spreading in the aquifer
Our simulations show that at the end of 2017 the DSW plume was spreading westwards (in the direction of the natural hydraulic gradient, as expected), approaching the closest western production wells (M2, M6, M9; Fig. 5a). Note that the production wells to the east (up-gradient) show a constant δ2H, indicating no interaction with the DSW recharge. Variability of δ2H along the production well screens (in the vertical direction), implies that the measured δ2H is a mixture of several aquifer layers (Fig. 5b).
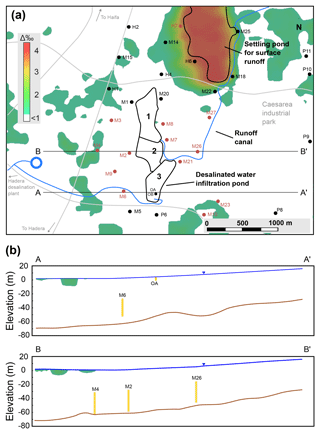
Figure 6Examination of the validity of the assumption of binary isotopic mixing. (a) Plan view (water table) of δ2H difference (Δ‰) between simulation results (2015–2017) with δ2Hmax= −18.41 ‰ (CGW=0.13) and δ2Hmin= −22.68 ‰ (CGW=0) at the end of 2017; the white area indicates Δ‰ < 1. (b) East–west cross sections through wells OA and M6 (A-A′) and wells M4, M2 and M26 (B-B′); well screens are shown in yellow.
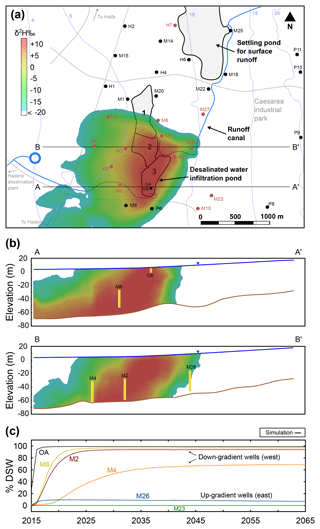
Figure 7Long-term simulations of DSW spreading at the end of 2065 after 50 years of MAR. (a) Plan view (water table); the colored area shows the DSW plume, the white area indicates natural GW (δ2H < −20 ‰) and the blue contours are the GW head. (b) East–west cross sections through wells OA and M6 (A-A′) and wells M4, M2 and M26 (B-B′); well screens are shown in yellow. (c) Simulated DSW fraction (%) in selected wells along the cross sections A-A′ and B-B′.
The δ2H variations shown in Fig. 5a reflect the DSW spreading in the aquifer. The highest δ2H that was measured in the aquifer (prior to MAR of DSW) was δ2H = 18.41 ‰, and therefore any value above it indicates mixing with DSW. However, because the initial measured δ2H values in the aquifer are in the range of δ2H = −18.41 ‰ to −22.68 ‰, the extent of DSW mixing in each well is relative to its specific initial δ2H. This can be calculated by a mixing ratio (MR) approach with MR ∕ (δDSW−δi), where δw is the δ2H in the well, δi is the initial (background) δ2H in the well and δDSW is the δ2H of DSW. The MR value of 0 and 1 implies original aquifer water and pure DSW, respectively. Figure 5c shows the MR (expressed in %DSW) of three down-gradient wells (M2, M4 and M6), two up-gradient wells (M23 and M26) and an observation well (OA) inside the DSW pond. Wells M2 and M6 have up to a 20 % DSW portion, while M23 and OA retain original aquifer water and almost pure DSW, respectively. At the end of 2017, about 7 % of the recharged DSW was recovered by the production wells.
Knowing the water composition of the aquifer and DSW, and assuming a conservative transport of all the major ions, one can estimate the water composition in a specific well based on the calculated mixing ratio, [X]w= MR × × [X]i. Here [X]w is the (calculated) ion concentration in the well, [X]DSW is the ion concentration in the DSW and [X]i is the initial ion concentration (background) in the well. Diversion of the observed concentration from the calculated concentration can give insight to the sediment–water reaction (e.g., Ganot et al., 2018; Ronen-Eliraz et al., 2017; Stuyfzand et al., 2017).
3.2.3 Binary system assumption
The model was based on the assumption that all water types in this system can be described by two end-members sorted by their isotope composition: (1) the “heavy” DSW (δ2H = 11.34 ‰), and (2) the “light” natural water (δ2H = −22.68 ‰) which includes all other water types (rain, runoff and GW). As pointed out before, while DSW isotope composition is constant, that of the local natural water is more variable. To examine the validity of the assumption of binary δ2H values, we ran the simulation again for the same period of 2015 to 2017, but this time with the maximum value of GW δ2H = −18.41 ‰ (in all GW boundaries and also as a rain and runoff source) in order to check the model sensitivity to the natural GW isotope variability. We subtracted the isotope composition results of the two simulations in all model cells to produce an error map (Fig. 6a) of δ2H differences (Δ‰). In terms of δ2H composition in the production wells (Fig. 6b), the results of both simulations were similar (Δ‰ < 1), while some differences (up to Δ‰ = 4.3) were found in the domain boundaries and at the upper layer that was affected by rain and runoff recharge. Specifically, a notable difference is seen in the runoff settling pond, which is a source of natural water recharge. Nevertheless, for the area surrounding the DSW infiltration basin (pond 3), the binary assumption is valid due to the following conditions: (1) the distinct difference between the isotope composition of DSW and GW; (2) the model boundaries are relatively far (> 2 km) from the source of the MAR with DSW; and (3) the screens of the production wells are relatively deep (depth > 50 m). Hence, in this case we can conclude that the initial variability of isotope composition in the aquifer has a negligible impact on the simulation results. Practically, it implies that interpolation efforts of the aquifer isotope composition (prior to MAR with DSW) are unnecessary, as one can use an average isotope value to normalize the tracer concentration in the aquifer. In addition, a major advantage of the binary assumption is that it allows one to estimate the mixing when the spatial data of water isotope is limited. This was exploited in the current study, where isotope data of the model boundaries were unavailable.
The results of the error analysis also support the model assumption that isotope fractionation is negligible during GW flow (i.e., isotope composition is conservative) as the isotope composition variability in the aquifer (which originates from fractionation processes) does not impact the simulation results (Fig. 6). Moreover, our measurements at the Menashe MAR site show a similar isotope composition between the DSW source water at the surface, in the variably saturated zone and at the shallow GW (Ganot et al., 2018). Therefore, even if isotope fractionation exists in the aquifer to some extent as a slow process, it should be considered negligible compared to the distinct difference between the isotope end-members.
3.2.4 Predicting long-term DSW spreading in the aquifer (2015–2065)
We test the extent of DSW spreading in the aquifer by performing long-term (50 years) simulation of MAR with DSW, considering 50 repeated annual cycles of the hydraulic conditions recorded in 2015, with a MAR event of 2.6 MCM (Fig. 7a). According to the simulation results, the water in the down-gradient (westwards) wells closest to the DSW pond, M2 and M6, will be fully exchanged with DSW after 10 years of MAR, while the up-gradient wells show little (M26) or no (M23) mixing with DSW (Fig. 7b, c). Interestingly, well M4, located further to the west, reaches a steady DSW mixing of almost 70 % after about 35 years of MAR without being fully exchanged with DSW, while the DSW plume continues to progress further west. By the end of 2065, the total DSW volume of 130 MCM recharged at the infiltration pond will be distributed as follows: 114 MCM (88 %) is recovered by the western pumping wells (M2–9, P6), 8.4 MCM (6 %) by the eastern pumping wells (M21, M26) and only 7.5 MCM (6 %) remains in the aquifer.
We track the fate of reverse-osmosis DSW that was introduced to groundwater by MAR using stable water isotopes. The use of the water isotopes of 18O and 2H is advantageous in this system for two reasons: (1) there is a distinct difference between the isotope composition of DSW and natural, fresh water; and (2) the water isotope composition of all natural water sources – groundwater, rain and runoff – is very similar. The former makes water stable isotopes a more sensitive tracer (compared to other natural conservative tracers such as chloride), whereas the latter reduces the problem to a binary mixture of two end-members: reverse-osmosis DSW and natural GW. We formulate a detailed three-dimensional GW flow and transport model, exploiting these advantages. The model, calibrated using field data (measured during 2015–2017), is used to predict the spreading of DSW in the aquifer during 50 years of MAR with DSW. Our simulation results suggest that most of the recharged DSW (94 %) is recovered by the production wells, indicating the efficacy of the Menashe MAR site.
The advantage of using stable water isotopes for tracing reverse-osmosis DSW in various downstream water systems is already known from previous studies. In this study we used this advantage in a modeling framework to predict future mixing and spreading trends of DSW in an aquifer. Hence, this modeling approach can be used in other MAR sites (e.g., Mazariegos et al., 2017; Negev et al., 2017; Stuyfzand et al., 2017) to predict reverse-osmosis DSW distribution in aquifers. As the production of DSW using reverse-osmosis is projected to increase and the use of MAR systems expands, we believe that the methodology presented in this paper will be highly relevant for more MAR hydrologists.
The underlying research data can be found in the Supplement or by contacting the corresponding author.
The supplement related to this article is available online at: https://doi.org/10.5194/hess-22-6323-2018-supplement.
The authors declare that they have no conflict of interest.
The research leading to these results received funding from the
joint German–Israeli water technology research program BMBF–MOST, project
WT1401. We thank Amos Russak and Raz Studny (Ben-Gurion University) for
sampling and analysis assistance.
Edited by:
Bill X. Hu
Reviewed by: Alex Furman and one anonymous referee
Al-Basheer, W., Al-Jalal, A., and Gasmi, K.: Variations in isotopic composition of desalinated water, Water Environ. J., 31, 209–214, https://doi.org/10.1111/wej.12232, 2017.
Birnhack, L., Voutchkov, N., and Lahav, O.: Fundamental chemistry and engineering aspects of post-treatment processes for desalinated water – A review, Desalination, 273, 6–22, https://doi.org/10.1016/j.desal.2010.11.011, 2011.
Boronina, A., Balderer, W., Renard, P., and Stichler, W.: Study of stable isotopes in the Kouris catchment (Cyprus) for the description of the regional groundwater flow, J. Hydrol., 308, 214–226, https://doi.org/10.1016/j.jhydrol.2004.11.001, 2005.
Burnett R. D. and Frind E. O.: Simulation of contaminant transport in three dimensions: 2. Dimensionality effects, Water Resour. Res., 23, 695–705, https://doi.org/10.1029/WR023i004p00695, 1987.
Carle, S. F.: T-PROGS: Transition probability geostatistical software, University of California, Davis, CA, 84, available at: http://gmsdocs.aquaveo.com/t-progs.pdf (last access: 15 August 2017), 1999.
Dawoud, M. A.: The role of desalination in augmentation of water supply in GCC countries, Desalination, 186, 187–198, https://doi.org/10.1016/j.desal.2005.03.094, 2005.
Dreizin, Y., Tenne, A., and Hoffman, D.: Integrating large scale seawater desalination plants within Israel's water supply system, Desalination, 220, 132–149, https://doi.org/10.1016/j.desal.2007.01.028, 2008.
Ganot, Y., Holtzman, R., Weisbrod, N., Nitzan, I., Katz, Y., and Kurtzman, D.: Monitoring and modeling infiltration–recharge dynamics of managed aquifer recharge with desalinated seawater, Hydrol. Earth Syst. Sci., 21, 4479–4493, https://doi.org/10.5194/hess-21-4479-2017, 2017.
Ganot, Y., Holtzman, R., Weisbrod, N., Russak, A., Katz, Y., and Kurtzman, D.: Geochemical Processes During Managed Aquifer Recharge With Desalinated Seawater, Water Resour. Res., 54, 978–994, https://doi.org/10.1002/2017WR021798, 2018.
Gat, J. R.: Oxygen and hydrogen isotopes in the hydrologic cycle, Annu. Rev. Earth Pl. Sc., 24, 225–262, 1996.
Gat, J. R. and Dansgaard, W.: Stable isotope survey of the fresh water occurrences in Israel and the Northern Jordan Rift Valley, J. Hydrol., 16, 177–211, https://doi.org/10.1016/0022-1694(72)90052-2, 1972.
Goldsmith, Y., Polissar, P. J., Ayalon, A., Bar-Matthews, M., deMenocal, P. B., and Broecker, W. S.: The modern and Last Glacial Maximum hydrological cycles of the Eastern Mediterranean and the Levant from a water isotope perspective, Earth Planet. Sc. Lett., 457, 302–312, https://doi.org/10.1016/j.epsl.2016.10.017, 2017.
Gvirtzman, H.: Israel Water Resources, Chapters in Hydrology and Environmental Sciences, Yad Ben-Zvi Press, Jerusalem, 2002.
Hanasaki, N., Yoshikawa, S., Kakinuma, K., and Kanae, S.: A seawater desalination scheme for global hydrological models, Hydrol. Earth Syst. Sci., 20, 4143–4157, https://doi.org/10.5194/hess-20-4143-2016, 2016.
Harbaugh, A. W., Banta, E. R., Hill, M. C., and McDonald, M. G.: MODFLOW-2000, The US Geological Survey Modular Ground-Water Model – User Guide to Modularization Concepts and the Ground-Water Flow Process, Report, available at: http://pubs.er.usgs.gov/publication/ofr200092 (last access: 30 November 2018), 2000.
Israel Meteorological Service: Climate atlas of Israel, http://www.ims.gov.il/IMS/CLIMATE/ClimaticAtlas/ (last access: 4 June 2018), 2014.
Israel Water Authority: National groundwater levels report, http://www.water.gov.il/Hebrew/ProfessionalInfoAndData/Data-Hidrologeime/DocLib2/hydrological-report-sep14.pdf (last access: 4 June 2018), 2014.
Israel Water Authority: Water consumption report, http://www.water.gov.il/Hebrew/ProfessionalInfoAndData/Allocation-Consumption-and-production/20173/intro.pdf, last access: 19 November 2018.
Kloppmann, W., Vengosh, A., Guerrot, C., Millot, R., and Pankratov, I.: Isotope and Ion Selectivity in Reverse Osmosis Desalination: Geochemical Tracers for Man-made Freshwater, Environ. Sci. Technol., 42, 4723–4731, https://doi.org/10.1021/es7028894, 2008a.
Kloppmann, W., Van Houtte, E., Picot, G., Vandenbohede, A., Lebbe, L., Guerrot, C., Millot, R., Gaus, I., and Wintgens, T.: Monitoring Reverse Osmosis Treated Wastewater Recharge into a Coastal Aquifer by Environmental Isotopes (B, Li, O, H), Environ. Sci. Technol., 42, 8759–8765, https://doi.org/10.1021/es8011222, 2008b.
Kloppmann, W., Negev, I., Guttman, J., Goren, O., Gavrieli, I., Guerrot, C., Flehoc, C., Pettenati, M., and Burg, A.: Massive arrival of desalinated seawater in a regional urban water cycle: A multi-isotope study (B, S, O, H), Sci. Total Environ., 619/620, 272–280, https://doi.org/10.1016/j.scitotenv.2017.10.181, 2018.
Krabbenhoft, D. P., Anderson, M. P., and Bowser, C. J.: Estimating groundwater exchange with lakes: 2. Calibration of a three-dimensional, solute transport model to a stable isotope plume, Water Resour. Res., 26, 2455–2462, https://doi.org/10.1029/WR026i010p02455, 1990.
Kurtzman, D., Netzer, L., Weisbrod, N., Nasser, A., Graber, E. R., and Ronen, D.: Characterization of deep aquifer dynamics using principal component analysis of sequential multilevel data, Hydrol. Earth Syst. Sci., 16, 761–771, https://doi.org/10.5194/hess-16-761-2012, 2012.
Lahav, O., Kochva, M., and Tarchitzky, J.: Potential drawbacks associated with agricultural irrigation with treated wastewaters from desalinated water origin and possible remedies, Water Sci. Technol., 61, 2451, https://doi.org/10.2166/wst.2010.157, 2010.
Liu, Y., Yamanaka, T., Zhou, X., Tian, F. and Ma, W.: Combined use of tracer approach and numerical simulation to estimate groundwater recharge in an alluvial aquifer system: A case study of Nasunogahara area, central Japan, J. Hydrol., 519, 833–847, https://doi.org/10.1016/j.jhydrol.2014.08.017, 2014.
Mazariegos, J. G., Walker, J. C., Xu, X., and Czimczik, C. I.: Tracing Artificially Recharged Groundwater using Water and Carbon Isotopes, Radiocarb. Tucson, 59, 407–421, https://doi.org/10.1017/RDC.2016.51, 2017.
Negev, I., Guttman, J. and Kloppmann, W.: The Use of Stable Water Isotopes as Tracers in Soil Aquifer Treatment (SAT) and in Regional Water Systems, Water, 9, 73, https://doi.org/10.3390/w9020073, 2017.
Reynolds, D. A. and Marimuthu, S.: Deuterium composition and flow path analysis as additional calibration targets to calibrate groundwater flow simulation in a coastal wetlands system, Hydrogeol. J., 15, 515–535, https://doi.org/10.1007/s10040-006-0113-5, 2007.
Rodríguez-Escales, P., Canelles, A., Sanchez-Vila, X., Folch, A., Kurtzman, D., Rossetto, R., Fernández-Escalante, E., Lobo-Ferreira, J.-P., Sapiano, M., San-Sebastián, J., and Schüth, C.: A risk assessment methodology to evaluate the risk failure of managed aquifer recharge in the Mediterranean Basin, Hydrol. Earth Syst. Sci., 22, 3213–3227, https://doi.org/10.5194/hess-22-3213-2018, 2018.
Ronen-Eliraz, G., Russak, A., Nitzan, I., Guttman, J., and Kurtzman, D.: Investigating geochemical aspects of managed aquifer recharge by column experiments with alternating desalinated water and groundwater, Sci. Total Environ., 574, 1174–1181, https://doi.org/10.1016/j.scitotenv.2016.09.075, 2017.
Sellinger, A. and Aberbach, S.: Artificial recharge of coastal-plain aquifer in Israel, Underground Waste Management and Artificial Recharge, 2, 701–714, 1973.
Shavit, U. and Furman, A.: The location of deep salinity sources in the Israeli Coastal aquifer, J. Hydrol., 250, 63–77, https://doi.org/10.1016/S0022-1694(01)00406-1, 2001.
Stanhill, G., Kurtzman, D., and Rosa, R.: Estimating desalination requirements in semi-arid climates: A Mediterranean case study, Desalination, 355, 118–123, https://doi.org/10.1016/j.desal.2014.10.035, 2015.
Stichler, W., Maloszewski, P., Bertleff, B., and Watzel, R.: Use of environmental isotopes to define the capture zone of a drinking water supply situated near a dredge lake, J. Hydrol., 362, 220–233, https://doi.org/10.1016/j.jhydrol.2008.08.024, 2008.
Stuyfzand, P., Smidt, E., Zuurbier, K., Hartog, N., and Dawoud, M.: Observations and Prediction of Recovered Quality of Desalinated Seawater in the Strategic ASR Project in Liwa, Abu Dhabi, Water, 9, 177, https://doi.org/10.3390/w9030177, 2017.
Yermiyahu, U., Tal, A., Ben-Gal, A., Bar-Tal, A., Tarchitzky, J., and Lahav, O.: Rethinking Desalinated Water Quality and Agriculture, Science, 318, 920–921, https://doi.org/10.1126/science.1146339, 2007.
Zheng, C. and Wang, P. P.: MT3DMS: A modular three-dimensional multi-species transport model for simulation of advection, dispersion, and chemical reactions of contaminants in ground-water systems. Documentation and user's guide, in Contract Report SERDP-99-1, US Army Engineer Research and Development, 1999.
Zhou, Y. and Li, W.: A review of regional groundwater flow modeling, Geosci. Front., 2, 205–214, https://doi.org/10.1016/j.gsf.2011.03.003, 2011.