the Creative Commons Attribution 4.0 License.
the Creative Commons Attribution 4.0 License.
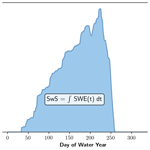
Changing snow water storage in natural snow reservoirs
Christina Marie Aragon
David F. Hill
This work introduces a novel snow metric, snow water storage (SwS), defined as the integrated area under the snow water equivalent (SWE) curve (units: length-time, e.g., m d). Unlike other widely used snow metrics that capture snow variables at a single point in time (e.g., maximum SWE) or describe temporal snow characteristics (e.g., length of snow season), SwS is applicable at numerous spatial and temporal scales. This flexibility in the SwS metric enables us to characterize the inherent reservoir function of snowpacks and quantify how this function has changed in recent decades. In this research, changes in the SwS metric are evaluated at point, gridded and aggregated scales across the conterminous United States (hereafter US), with a particular focus on 16 mountainous Environmental Protection Agency (EPA) Level III Ecoregions (ER3s). These ER3s account for 72 % of the annual SwS (SwSA) in the US, despite these ER3s only covering 16 % of the US land area. Since 1982, spatially variable changes in SwSA have been observed across the US with notable decreasing SwSA trends in the western US and in the 16 mountainous ER3s. All mountainous ER3 (except for the Northeastern Highlands in New England) exhibit decreasing trends in SwSA resulting in a 22 % overall decline in SwSA across mountainous ER3s. The peak monthly SwS (SwSM) occurs in March at all spatial scales, while the greatest percentage loss of SwSM occurs early in the snow season, particularly in November. Unsurprisingly, the highest elevations contribute most to SwSA in all mountain ranges, but the specific elevations that have experienced loss or gain in SwSA over the 39-year study period vary between mountain ranges. Comparisons of SwS with other snow metrics underscore the utility of SwS, providing insights into the natural reservoir function of snowpacks, irrespective of SWE curve variability or type (e.g., ephemeral, mountain, permanent). As we anticipate a future marked by increased climate variability and greater variability in mountain snowpacks, the spatial and temporal flexibility of snow metrics such as SwS may become increasingly valuable for monitoring and predicting snow water resources.
- Article
(5912 KB) - Full-text XML
- BibTeX
- EndNote
Seasonal snow is a critical resource in mountainous regions and at high latitudes across the United States (US), and many other countries, providing an important ecosystem service by functioning as a natural and spatially distributed reservoir (Barnett et al., 2005). These snow reservoirs play a key role in the water cycle by storing water during the cool season and releasing water gradually throughout the warm season when human and ecological demand is the highest (Li et al., 2017). The natural reservoir function of snowpacks is at risk due to anthropogenic climate change, which has been shown to decrease snowpack magnitude and persistence while increasing snowpack variability (Siirila-Woodburn et al., 2021; Scalzitti et al., 2016; Sospedra-Alfonso et al., 2015; Morán-Tejeda et al., 2013). The variability in climatic variables that drive snowpack variability, such as precipitation and temperature, has increased in the recent past and is projected to continue to increase as a result of climate change (Scalzitti et al., 2016; Sospedra-Alfonso et al., 2015; Morán-Tejeda et al., 2013; Pendergrass et al., 2017; Ohmura, 2012). Given the vulnerability of seasonal snow water storage to climate change and the importance of snow-derived water to municipalities, agriculture, ecosystems and hazard forecasters, it is vital to understand how water storage in our natural snow reservoirs is evolving in the context of a changing climate (Immerzeel et al., 2020; Sturm et al., 2017; Barnett et al., 2005; Li et al., 2017; Siirila-Woodburn et al., 2021).
Snow water equivalent (SWE) is a relevant snowpack characteristic for many water resources applications. SWE is the depth of water obtained upon melting a column of snow. Having an estimate of SWE across a watershed is analogous to knowing the stage (water elevation) in a surface reservoir; it quantifies the amount of water being stored for later use. Other directly measurable snow characteristics largely fall into two categories: (1) they can be temporal snapshots that give us information about snow magnitude at a certain point in time or (2) they can provide information about snow timing (Nolin et al., 2021). The 1 April SWE, snow-covered area (SCA) and peak SWE (SWEmax) are examples of temporal snapshot metrics. Snow metrics that give us information about the timing of snow include snow cover duration (SCD), date of snow onset (DSO) and date of snow disappearance (DSD).
Composite snow metrics, such as the snow storage index (SSI) (Hale et al., 2023) and the water tower index (WTI) (Viviroli et al., 2007), are not directly measurable, but they combine information streams in order to relate snowpack to water storage. The SSI indicates the degree to which snowpack delays the timing and magnitude of surface water inputs relative to when it falls as precipitation, whereas the WTI identifies locations where mountain runoff contributes disproportionately to lowland water supplies. Additionally, a global WTI was developed, which ranks all water towers in terms of their water-supplying role and downstream societal and ecological demand (Immerzeel et al., 2020).
A conceptual SWE curve is shown in Fig. 1a. The conceptual SWE curve referenced throughout this paper is for mountain snowpacks and is delineated by three points: the DSO, the peak SWE (SWEmax) and the DSD. SWE accumulation begins at the DSO and continues up to a SWEmax, which may or may not occur on 1 April (Northern Hemisphere). After SWEmax, the ablation phase of the snow season begins and the SWE depth declines until it reaches zero at the DSD. The SCD is captured by the width of the SWE curve. Multiple factors can result in systematic changes in the shape of the SWE curve, including climate change (Lute et al., 2015); natural land cover change, such as wildfire (Gleason et al., 2019) or beetle kill (Pugh and Small, 2012; Boon, 2007; Winkler et al., 2014); and anthropogenic land cover change, such as forest thinning (Krogh et al., 2020; Sun et al., 2022) or logging (Winkler et al., 2005; Troendle and Reuss, 1997).
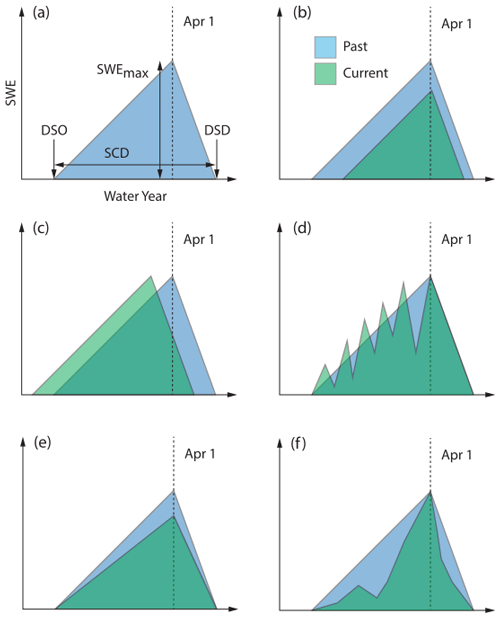
Figure 1Conceptual illustration of a SWE curve and various ways that it could change from past to present.
The shaded regions in the other panels of Fig. 1 provide plausible examples of how the SWE curve may have changed from the past to present day. For example, a current SWE curve could be a scaled (reduced) version of a past SWE curve (Fig. 1b). This would result in a later DSO, a lower SWEmax, an earlier DSD and a shorter SCD. Changes in SWE curves could also result from a temporal shift in the historic curve (Fig. 1c). This would not impact SWEmax or SCD, but the metrics including 1 April SWE, DSO and DSD would be affected. Figure 1d gives yet another example of a theoretical current scenario, compared to a historic one. In this case, the shape of the conceptual SWE curve is changed by repeated accumulation and melt events during the accumulation season. As shown in this graphic, metrics such as DSO, DSD, SCD, SWEmax and 1 April SWE could all remain unchanged, but it is clear that the snowpack is different than in the past. Previous literature has quantified increasing ablation during the accumulation period by defining a “melt fraction” (Musselman et al., 2021), which is the ratio of the melt that occurs during the accumulation phase to the total melt. Their metric helps to identify snowpacks that have considerable variance and vulnerability to warming and rain-on-snow events. Another example of changing snowpack is shown in Fig. 1e. Here, the SCD remains constant due to consistent DSO and DSD, but SWEmax decreases in magnitude, resulting in less snow overall. Finally, Fig. 1f shows a theoretical future in which DSO, DSD, SCD, 1 April SWE and SWEmax all remain constant, but it is clear that there is less snow present throughout the season.
The conceptual SWE curve and the above discussion is focused on a mountain snowpack, a snowpack with a distinct period of steady accumulation up to a SWEmax, followed by a similarly steady ablation season that persists throughout the winter. While mountain snowpacks play a key role in natural water storage, other types of snowpacks also have distinct characteristics and are important to the hydrological cycle. For example, ephemeral snowpacks, snowpacks that tend to be have a lower cold content than mountain snowpacks and come and go throughout the winter (Sturm et al., 1995; Hatchett, 2021), play a role in soil moisture and runoff regimes (Livneh and Badger, 2020; Hamlet and Lettenmaier, 2007). Ephemeral snowpacks tend to experience accumulation and ablation processes nearly in tandem (Liston and Elder, 2006). Alternatively, Greenland and Antarctic ice sheets primarily experience accumulation processes (Liston and Elder, 2006). While metrics such as 1 April SWE, SWEmax and SCD do a good job of characterizing mountain snowpacks, they are not as useful at capturing the transient nature of ephemeral snowpacks or the lack of an ablation season on ice sheets.
This work aims to characterize the extent to which snowpacks serve as natural reservoirs and evaluate spatial and temporal changes in snow water storage in a new, integrated way. As we move into a future of increased climate and snowpack variability, we need snow metrics that can capture diverse and dynamic snowpack regimes. As the majority of natural snow water storage in the US occurs in mountainous regions, it is important to understand how the natural reservoir function of snowpacks is changing across individual mountain ranges. When attempting to quantify snow water storage change, it can be difficult to merge the scale at which most in situ observations are available (at the point/station scale) and the scale at which snowpacks operate as natural reservoirs (at the mountain range scale). This study presents a new spatially and temporally flexible snow metric, snow water storage (SwS), in order to address the reality of changing snowpack regimes and the challenge of spatial variability between snow observations and decision-making. This study examines SwS trends in mountain snowpacks by addressing the following research questions:
-
What are the trends in monthly and annual SwS across the US at discrete point, gridded and aggregated scales?
-
What is the role of mountainous ER3s in US SwS and how has this changed in recent decades?
-
How does SwS relate to other common snow metrics?
2.1 Snow water storage metric (SwS)
SwS quantifies the depth of water stored in snow reservoirs over time and is calculated by integrating the area under the SWE curve:
where SWE has dimensions of length, and integration occurs over a time period (water year, a given month, etc.) of interest. If daily SWE data are used for this calculation at a given point, SwS will have dimensions of meter-days (or md).
As defined above, SwS is a quantity computed at a single point, e.g., a SNOTEL location. However, the SwS metric can also be aggregated across various spatial scales. There are numerous reanalysis products that provide spatially distributed SWE information on a regular grid. In this case, SwS can be computed for a horizontal area (say a particular watershed) of interest, resulting in SwS having dimensions of cubic meter days (m3 d).
SwS can also be computed for various integration periods. If the integration is done over the entire water year, this yields annual SwS (SwSA). In the integration is for a particular month, this yields monthly SwS (SwSM). Integrating daily SWE data over a single day produces the daily value of SwS (SwSD), but this is simply is the same as daily SWE.
SwS is the integrated area under the SWE curve, indicating the cumulative meter-days of water stored as a snowpack. SwS quantifies the degree to which a snowpack functions as a water storage reservoir. Unlike 1 April SWE or SWEmax, SwS can be applied to mountain, ephemeral or permanent snowpacks. Unlike other storage-related metrics such as SSI and WTI, SwS is directly measurable and does not require the combination of multiple data streams to calculate. Ultimately, this integrated metric helps us to understand how much water is held in our snow reservoirs and for how long.
2.2 Data
Daily observations of SWE were obtained from Natural Resources Conservation Service (NRCS) Snow Telemetry (SNOTEL) stations (Serreze et al., 1999) and from Cooperator Snow Sensors (COOP). The SNOTEL network provides data at discrete scattered points across the western US, while the COOP stations used in this study provide data across California. This study used the 465 stations that have a period of record from at least water year 1982 to water year 2020 with less than 10 % of days missing during that period.
This study also uses the University of Arizona SWE (UASWE) dataset (Zeng et al., 2018; Broxton et al., 2019), a daily 4 km gridded dataset that spans the US. The UASWE dataset assimilates SWE and snow depth observations into an empirical temperature index snow model that is forced with PRISM temperature and precipitation data (Daly et al., 2008). The primary value of this dataset is that it provides SWE estimates at locations other than the SNOTEL stations. This allows for the aggregation of SWE information over areas of interest (Zeng et al., 2018). The UASWE product has been shown to outperform (Dawson et al., 2018) other gridded SWE products such as the SWE estimates from the Snow Data Assimilation System (SNODAS; NSIDC, 2004). Additionally, a spatially continuous, gridded product allows us to build a more complete picture of spatial changes in SwS and how changes in SwS are occurring at aggregated scales. Despite the good performance of the UASWE product, there are limitations to using any modeled SWE product. Factors such as imperfect physics, inaccurate boundary conditions and rescaling errors can contribute to inaccuracies in the modeled field, SWE in this case (Sturm, 2015; Zhang et al., 2007).
The Environmental Protection Agency (EPA) Level III Ecoregions (ER3s) (McMahon et al., 2001; Omernik and Griffith, 2014), which are regions with similar ecosystems and environmental resources, were used to identify mountainous regions and to delineate the grid cells in the UASWE dataset that were associated with each ER3 (Fig. 2). Mountainous ER3s were included in this study if at least half of their area resided in the snow-covered mask (described in Sect. 2.3 below). As each ER3 has similarities in biotic, abiotic, terrestrial and aquatic ecosystem components, examining SwS change in any given ecoregion may help us understand ecosystem impacts that are related to changes in SwS. Numerous ER3s correspond to the major mountain regions in the western and eastern US that serve as the largest natural reservoirs in the country.
Finally, NASA Shuttle Radar Topography Mission (SRTM) digital elevation data (Farr et al., 2007) were regridded to create a digital elevation model (DEM) matching the grid of the UASWE product. Elevation data were used to calculate watershed hypsometry in each ER3. The procedure used to calculate a hypsometry grid is described in Sect. 2.4.2.
Although the station and ER3 datasets extend beyond the conterminous US, the UASWE dataset does not. All datasets were spatially constrained to the conterminous US in order to facilitate the comparison of results between spacial scales. A summary of all of these datasets is provided in Table 1.
2.3 Study area
As noted above, this study considers both discrete station data that focus on the western US and spatially continuous gridded data that cover the conterminous US. Regarding the gridded data, many locations have little to no snow. Therefore, the analysis of the gridded product is restricted to locations that have a mean of at least 30 snow-covered days per year based on the 39-year climatology (1982–2021) of the UASWE dataset (Fig. 3). As expected, snow cover duration increases with latitude and elevation, with the longest snow cover duration found along mountain tops in the western US. In the ER3 SwS change analysis, all ER3s are considered that contain grid cells that meet the 30 d snow cover threshold, although the mountainous ER3s are more closely examined because they store the bulk of our winter water.
2.4 Analysis
2.4.1 SwS trends
To evaluate significant trends in SwSA and SwSM across the US, these quantities were computed over a 39-year period of study (water years 1982–2020) at stations and at UASWE grid cells, which have an area of 16 km2. The grid-cell-based SWE from the UASWE product was additionally aggregated for each ER3 in order to assess trends at larger scales. To compute SwS at aggregated ER3 scales, the gridded SWE data within an individual ER3 were simply integrated spatially, resulting in SwS with units of cubic meter days (m3 d).
This study used the Hamed and Rao modified Mann–Kendall (MK) test from the pyMannKendall Python package to compute trends in SwS (Hussain and Mahmud, 2019). The MK test is a rank-based nonparametric test that is used to evaluate monotonic (increasing or decreasing) trends in temporally varying data (Hirsch et al., 1982). Thus, the null hypothesis is that the data are randomly and independently ordered, whereas the alternative hypothesis is that a monotonic trend exists in the data. Although the MK test is widely used in hydrological studies, it does not account for positive autocorrelation, which increases the probability of detecting trends when no trends exist. Because of this, many studies have turned to a modified MK test that does account for autocorrelation (Hamed and Rao, 1998).
2.4.2 Trends by elevation in mountainous ecoregions
This analysis focused on 16 ER3s corresponding to the mountain ranges that receive substantial snowfall relative to surrounding ecoregions. A total of 12 of these ER3s are located in the western US and 4 are located in the eastern US. The relative elevation of SwSA change in each ER3 is examined in this study. In order to make trends in SwSA comparable over the wide range of elevations across the US, the elevations of each ER3 are converted to hypsometry scores. Each ER3 boundary is used to select co-located elevation data from the regridded NASA SRTM digital elevation dataset. ER3 hypsometry is calculated by determining the percentage of the ER3 area that falls below a given elevation within that ER3. Thus, there is 0 % of the ER3 at the lowest elevation of the ER3 and 100 % of the ER3 is below the highest elevation. Each elevation grid cell in the DEM is turned into a value between zero and one based on where that grid cell lies relative to other elevation grid cells within the same ER3. Hypsometry scores in each mountainous ER3 are then binned into 10 % increments, from 0 % of an ER3 below to 100 % of an ER3 below, in order to compute the mean SwSA and the percentage change in each hypsometry band from 1982 to 2020. The percentage change in the interquartile range (IQR) of SWE was also computed for each hypsometry band from 1982 to 2020. To calculate the percent change in the IQR, the IQR in each ER3 is calculated for each year in the study by subtracting the 25th percentile from the 75th percentile of SWE. The trend is evaluated in each hypsometry band following the trend analysis described in Sect. 2.4.1.
2.4.3 SwSA compared with other snow metrics
SwSA trends are compared with other commonly used snow metrics including 1 April SWE, SWEmax, day of SWEmax and SCD in order to evaluate what type of information the SwSA metric provides that other metrics do not. This is done in four ways using the station data. First, the percentage of stations with positive, positive significant, negative and negative significant trends in each metric is computed. Second, the trend in the annual number of snow-free periods is calculated at each station from 1982 to 2020 to evaluate whether snowpacks are becoming more ephemeral using the Hamed and Rao modified MK test described in Sect. 2.4.1. The annual number of snow-free periods is defined as the number of times in a water year that there is no snow following a period of snow. Next, the utility of the SwS metric compared with other snow metrics is shown using a case study of SNOTEL station 706 (Quartz Mountain, Oregon), a station that has transitioned from a mountain snowpack to an ephemeral snowpack. Third, a regression is computed between the percentage change in SwSA and each other metric above using empirical data from the stations. Finally, the relationship between the percentage changes in the empirical data is compared with the percentage changes that would be expected based on the conceptual SWE curve. For example, the empirical relationship between the percentage change in SwSA and the percentage change in SWEmax is compared with what it would be if there was a uniform scaling in the conceptual SWE curve as shown in Fig. 1b.
3.1 SwS change trends
3.1.1 SwSA change trends
The average SwSA across all stations in this analysis is 60 md. The lowest SwSA found at a single station was 0 md, whereas the maximum SwSA observed was 510 md. Changes in SwSA range from a decrease of 122 md to an increase of 69 md over the period of study (Fig. 4). Of the 97 SNOTEL and COOP stations with increasing trends in SwSA, only 10 had significant (p<0.1) increases (Fig. 4). Significant decreasing SwSA trends were found at 123 of the 367 stations. Losses in SwSA ranged from 2 to 122 md. Spatially, there are widespread decreasing SwSA trends across most of the 11 western states that contain snow stations, with declines ranging from 17 % to 87 %. The 10 stations with significant increases in SwSA range from a 6 % increase to a 78 % increase. The stations with increasing SwSA trends are mostly located in the Northern and Middle Rockies and also include a few station in the Southern Rockies and in the Cascades.
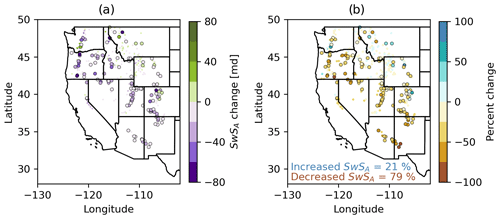
Figure 4Change in SwSA (md) (a) and percentage change in SwSA (b) across US stations from water years 1982 to 2020. Large outlined circles indicate stations with p<0.1.
Moving from discrete station data to the spatially continuous gridded UASWE data, there is a mean SwSA of 1.8×108 m3 d across grid cells (Fig. 5). Mountainous ER3s in the western US have an average SwSA of over 1.4×109 m3 d and the maximum SwSA is 2.6×1010 m3 d. The average SwSA in much of New England and the Upper Peninsula of Michigan ranges from 3.2×108 to 6.4×108 m3 d.
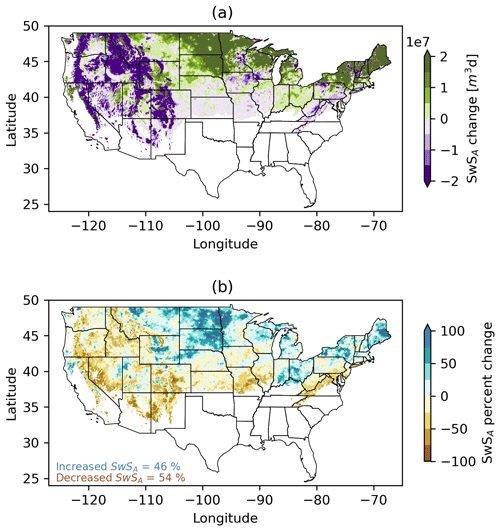
Figure 5Change in SwSA (md) (a) and percentage change in SwSA (b) across the UASWE dataset. Stippling indicates locations with p<0.1.
The grid-cell-scale change analysis yields similar geographic patterns of significant changes in SwSA in the western US to the station-scale analysis (Fig. 5). This is not surprising given that the UASWE product assimilates SNOTEL (and other) station data. The benefit of including a spatially distributed product such as UASWE in this analysis is that it adds detail and insight as to where changes in SwSA are occurring beyond the western US and in between the locations where discrete stations are located. Significant increases in grid-cell SwSA are primarily found in the north central and northeastern US. Only 5 % of US grid cells have significant increasing trends with a mean increase of 84 %. From 1986 to 2015, the north central and northeastern US experienced an increase in annual precipitation, particularly in spring and fall, although these regions also show spatially variable increases in precipitation during the winter (Easterling et al., 2017). These precipitation changes may partially explain the increases in SwSA, although these regions have also experienced increases in winter temperatures over the same time period. Significant decreases in SwSA are more widespread and are found across the western US, the Appalachian Mountains, the Blue Ridge Mountains and in the Ozarks. Of the 54 % of US grid cells that have decreasing trends in SwSA, 11 % have significant decreasing trends. The mean decline in SwSA for the grid cells with significant trends is 44 %.
Figure 6 indicates the raw change and percentage change in SwSA across ER3s. Aggregating UASWE SwSA at ER3 scales spatially filters (and thus mutes) some of the grid-cell-scale trends in SwSA, as can be seen when comparing Figs. 4 and 5. Of the 51 ER3s that are evaluated in this study, 19 have increasing trends and 32 have decreasing trends. Only one ER3, the non-mountainous Lake Agassiz Plain, has a significant positive trend in SwSA (86 % increase). None of the non-mountainous ER3s have significant decreasing SwSA trends. The specific ways in which SwSA has changed across the 16 mountainous ER3s and how these changes relate to other snow metrics will be discussed in Sect. 3.3.
3.1.2 SwSM change trends
The highest monthly mean SwSM occurs in March at stations (12.8 md), grid cells (2.7×107 m3 d) and in mountainous ER3s (7.4 m3 d). Figures 7–9 summarize trends in SwSM change evaluated at stations, UASWE grid cells and ER3s, respectively. Significant decreases in SwSM occur across all months at all spatial scales examined. November experienced the highest number of stations, grid cells and ER3s with significant SwSM losses compared with any other month. The greatest monthly median percentage loss of SwSM occurred in November at stations (56 %) and grid cells (44 %), while it occurred in March at mountainous ER3s (61 %). Looking at raw change values, the months with the greatest decrease in SwSM are not the same as the months with the largest percentage changes. March, December and January are the months with largest decrease in median SwSM at stations (4.6 md), grid cells (6.4×106 m3 d) and mountainous ER3s (1.1×1011 m3 d), respectively.
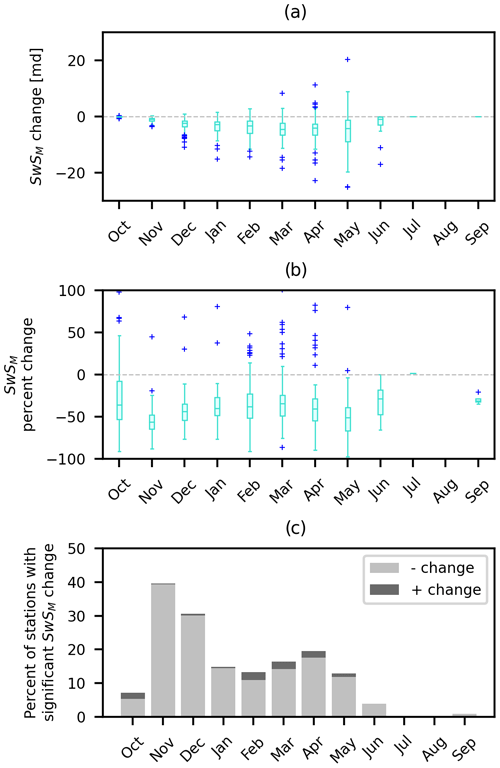
Figure 7Changes (significance p<0.1) in SwSM across US stations, in dimensional units (a) and in terms of percentage change (b). The rectangle indicates the interquartile range, with the middle bar indicating the median. The blue pluses are outlier points. Panel (c) shows the fraction of stations that had significant increases or decreases in SwS.
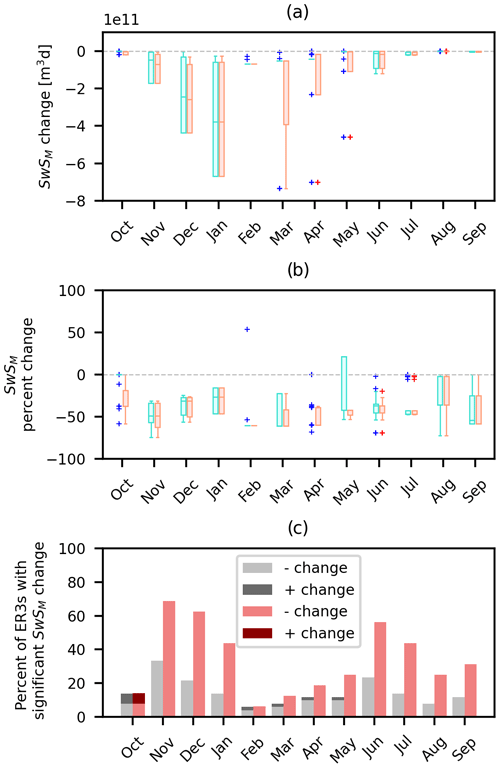
Figure 9As in Fig. 7 but for changes in SwSM aggregated across ER3s from water years 1982 to 2020. Red components of this figure indicate the results only considering mountainous ER3s.
Although there is an overall negative median percentage change in SwSM in all winter months at the station and grid-cell scale, February and March have higher occurrences of significant SwSM increase than any other months. At the grid-cell scale, October, March–May and July–August all have a 0 % median change in SwSM because most grid cells within the snow-covered mask are snow-free during these times. At the ER3 scale, the median percentage change in SwSM is negative in all months. Most data points that indicate significant positive increases in monthly storage are considered outliers at all spatial scales.
3.2 SwSA change trends in mountainous ER3s
Analysis of mountainous ecoregions illuminates the large role that mountains play in storing winter snow water resources as snowpack, particularly in the western US. An average of 72 % of the annual SwSA in the US (3.5×1013 m3 d) is held in the 16 mountainous ER3s, despite these ER3s only covering 16 % of the US land area (Figure 6). Western mountainous ecoregions cover 12 % of the US land surface and store an average of 65 % of the annual SwSA. Across all mountainous ER3s, there has been a 22 % decline (6.1×1012 m3 d) in SwSA over the 39-year period of study. Over the same time span, there has been a 24 % decline in SwSA in western mountainous ER3s, indicating that western snow reservoirs are shrinking faster than eastern snow reservoirs.
Of the 16 mountainous ER3s (outlined in red), only the Northeastern Highlands has a (nonsignificant) increasing SwSA trend, whereas the other 15 mountainous ER3s have decreasing SwSA trends. This means that the snow water storage in 94 % of mountainous ER3s has declined from 1982 to 2020. Five of the mountainous ER3s, the Cascades, the Eastern Cascade Slopes and Foothills, the Southern Rockies, the Idaho Batholith, and the Arizona/New Mexico Mountains, have significant decreasing SwSA trends, with a mean decrease of 38 %.
Table 2 summarizes the fraction of US SwSA in each mountainous ER3, the percentage change in SwSA from water years 1982 to 2020 and the p value associated with the change. In the western US, the Middle Rockies are responsible for the greatest fraction (12 %) of SwSA in the country, followed by the Southern Rockies (10 %) and the Idaho Batholith (8 %). SwSA has declined in all mountainous ER3s in the western US over the last 39 years. The greatest declines in western SwS were in the Arizona/New Mexico Mountains (56 % decline), the Eastern Cascade Slopes and Foothills (40 % decline), and the Cascades (39 % decline). All eastern mountainous ER3s showed declines in SwSA over the last 39 years with the exception of one, the Northeastern Highlands. The Northeastern Highlands are responsible for the greatest fraction (4 %) of SwSA in the eastern US; SwSA has increased 13 % in this region over the last 39 years. The greatest decline in SwSA in the eastern US was in the Ridge and Valley (11 % decline), which holds an average of 0.2 % of US SwSA.
The greatest SwSA is found in the highest 10 % of mountainous ER3s elevations (Fig. 10). Most mountainous ER3s have decreasing trends in SwSA across all hypsometry bins, although the Sierra Nevada (5), the Wasatch and Uinta Mountains (19), the Southern Rockies (21), the North Central Appalachians (62), and the North Cascades (77) show increasing trends in SwSA at low elevations and decreasing trends at higher elevations. The Northeastern Highlands is the only ER3 that shows increasing trends in SwSA at all elevations. Increasing trends in SwSA at low elevations in some ER3s may partially be a result of very low SwSA to begin with; thus, small changes in SwSA may suggest large percentage changes.
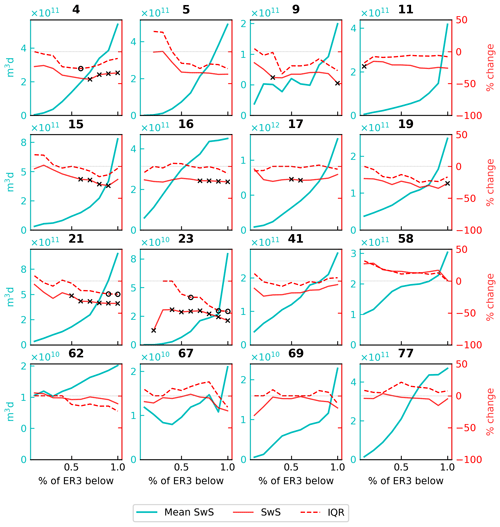
Figure 10SwSA in each mountainous ER3 as a function of ER3 hypsometry. The teal line indicates the average SwSA in each hypsometry bin from water years 1982 to 2020 (left axis). The solid red line indicates the percentage change in subscript A as a function of hypsometry for each mountainous ER3 over the time period of study (right axis). Black “×” symbols indicate where the percentage change in SwSA is significant (p<0.1). The dashed red line indicates the percentage change in the IQR of daily SWE as a function of hypsometry in each mountainous ER3 over the time period of study (right axis). Black “o” symbols indicate where the percentage change in the IQR is significant (p<0.1). Refer to Table 2 for ER3 names.
By looking at the percentage change trends in the IQR of SWE, this study gives an idea of how interannual SWE variability has changed from 1982 to 2020 (Fig. 10). Several ER3s have increases in the SWE IQR of the lowest hypsometry bands, which correspond to the lowest parts of ER3s. This could be a result of increasing snow variability as freezing levels move to higher elevations, resulting in increased irregularity in precipitation form. In the middle and upper hypsometry bands of most ER3s, there is largely a decrease in the IQR. This may be a result of declining snowpacks, which would allow for less variability in the range of SWE values overall. The Northeastern Highlands, Ridge and Valley, Central Appalachians, and North Cascades stand out in that they have increasing tends in IQRs across most hypsometry bands.
3.3 SwSA compared with other snow metrics
Figure 11 demonstrates how the conceptual SWE curve is changing in each of the ER3s based on the trend analysis of four common snow metrics: DSO, SWEmax, SWEmax day of water year (Dmax) and DSD. Trends in DSO, SWEmax, Dmax and DSD were evaluated because these metrics serve as anchor points that define the boundaries of the conceptual SWE curve. These conceptual SWE curves are superimposed on the observed mean SWE curves for the first and last 20 years of study in each ER3. With the exception of the Northeastern Highlands and the North Cascades, the 2020 SWE curve (solid red line) delineates a smaller conceptual SWE curve than in 1982 (dotted red line). The specific anchor points that cause the shrinkage of the conceptual SWE curve are variable across ER3s. Of the ER3s that experienced significant (p<0.1) decreases in SwSA, the Southern Rockies and the Arizona/New Mexico mountains also had significant changes in the DSO, DSD and SWEmax. The Cascades, the Eastern Cascade Slopes and Foothills, and the Idaho Batholith also experienced significant declines in SwSA. These three ER3s had significantly earlier DSDs. Although evaluating change at the anchor points tells us how the conceptual SWE curve is changing, the actual SWE curve is not a triangle and is subject to complex patterns of change including notable accumulation- and ablation-season SWE variability. A comparison of the mean SWE curves from the beginning and end of the study period yields a smaller SWE curve in the last decade of study in all western ER3s, although the eastern ER3s (Northeastern Highlands, North Central Appalachians, Ridge and Valley, and Central Appalachians) have more nuanced change in the SWE curve. These subtler shifts involve certain aspects of the snow season having higher SWE values in the first decade of study, while other parts of the snow season have higher SWE values in the later period of study. The SwS metric accounts for these complex patterns and is able to quantify natural reservoir storage and change.
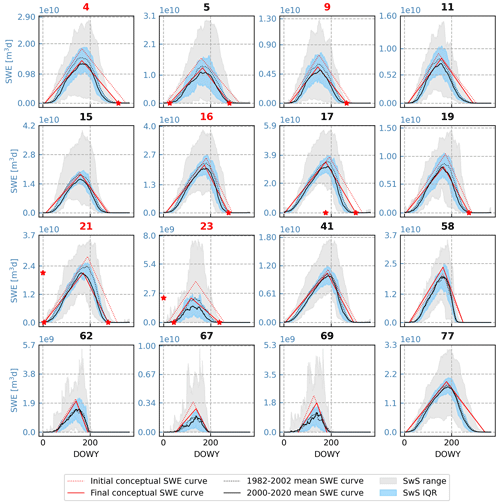
Figure 11Observed SWE curves for mountainous ER3s shown with historic and current conceptual SWE curves. The black dotted line indicates the mean SWE curve from the first 20 years of our study period in each ER3 (1982–2002). The solid black line indicates the mean SWE curve from the last 20 years of our study period for each ER3 (2000–2020). Light blue shading indicates the IQR of observed daily SWE and gray shading indicates the minimum and maximum range of observed daily SWE. Red ER3 labels signify ER3s that had a significant decrease in SwSA over the period of study. The red dotted line indicates the conceptual SWE curve for each ER3 at the start of our study period (1982) based on the trend analysis of DSO, SWEmax, Dmax and DSD. The solid red line indicates the conceptual SWE curve for each ER3 at the end of our study period (2020). Red stars on the x axis indicate significant decreases in DSO, Dmax and DSD. Red stars on the y axis indicate significant decreases in SWEmax. There were no significant increases in any of the snow metrics. Refer to Table 2 for ER3 names.
Trends in snow metrics (SwSA, 1 April SWE, SWEmax and SCD) are not changing in the same direction at all stations (Fig. 12). SNOTEL and COOP stations were placed to capture mountain snowpack regimes where snow increases up to a maximum value throughout the accumulation season and then disappears across the ablation season. The 1 April SWE, SWEmax, SwS and SCD measures are snow metrics that are useful for characterizing and monitoring change in mountain snowpack regimes and describe points relevant to a conceptual SWE curve. While trends in these metrics have changed in the same direction at 286 of the 465 SNOTEL and COOP stations, 38.5 % of stations have nonuniform inter-metric trend directions. This means that if trends in only one snow metric were to be examined, it could paint an incomplete picture of change.
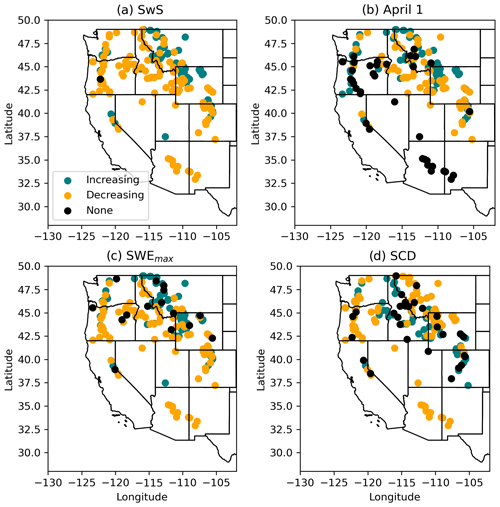
Figure 12Trend directions in SwSA (a), 1 April SWE (b), SWEmax (c) and SCD (d) across stations that do not have the same trend directions across all metrics.
The inability of common one-dimensional snow metrics to reflect snow storage change is particularly apparent when snowpacks transition from one snow regime to another, such as a permanent snowpack transitioning to a mountain snowpack or a mountain snowpack transitioning to an ephemeral snowpack. In our observational record, this study finds that snow regimes are becoming increasingly ephemeral at many station locations. This study found the number of annual snow-free periods has significantly increased at 23 % of stations over the last 38 years. The Quartz Mountain (OR) SNOTEL station (706) provides an example of where a snowpack has increased in ephemerality (Fig. 13). This station went from an average of 1.7 snow-free periods per water year over the first decade of study to an average of 6.3 snow-free periods per water year over the last decade of study. A side-by-side comparison of trends in SwSA, 1 April SWE, SWEmax and SCD illustrates how the SwS metric is able to capture complex change, such as that associated with an ephemeral snowpack. At the Quartz Mountain station, there are significant negative trends in SwS and SWEmax, no trend in 1 April SWE, and a positive trend in SCD (Fig. 13). In this example, 1 April SWE is largely not relevant as a snow monitoring metric because the majority of years are snow-free on 1 April at this location. This station is interesting because it has opposite significant trends in SWEmax, which is decreasing, and SCDs, which are increasing. As SwSA is the integral of the SWE curve, both the magnitude and duration of snow cover are incorporated into its calculation. This allows the SwS metric to provide a robust picture of the degree to which the snowpack is serving as a reservoir for water storage and how that reservoir function may be changing.
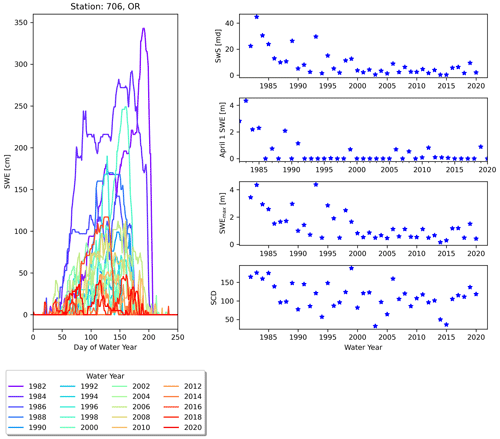
Figure 13SWE curves from every second water year from 1982 to 2020 at the Quartz Mountain SNOTEL station.
There are other ways to demonstrate the utility of SwS as an additional tool for gaining insight into our changing snowpack. The 1 April SWE is overwhelmingly the metric cited by water resource managers as the singular measure of the season's snow. However, how do changes in that measure correspond to changes in others? First, Fig. 14 shows the relationships between percentage changes in various snow metrics of interest. It can next be useful to return to the idea of a conceptual SWE curve (Fig. 1a). Based on this simple geometry, the SwSA is given by
If the geometry of the conceptual SWE curve were to be preserved over time, the SWE curve would be uniformly scaled (Fig. 1b). In this scenario, one would expect the percentage change in SWEmax and SCD to each be half the percentage change in SwS. However, the regression plots in Fig. 14 reveal that the change in SCD is roughly 26 % that of the change in SwS. Furthermore, it is found that the change in SWEmax is 86 % that of the change in SwSA. What this means is that the conceptual SWE curve has been flattening over the period of study of the data at stations. Therefore, relying on a single metric like 1 April SWE gives an incomplete assessment of the storage of snow throughout a full season, and a more holistic metric like SwS may be more informative when considering a full snow season.
SwS is a unique snow metric because it essentially has unlimited degrees of freedom – any change in the SWE curve (changes in SWE magnitude, timing, variability, etc.) will be captured in its calculation. Thus, regardless of how the SWE curve changes, the SwS metric is able to provide information about water storage in natural reservoirs at a given location. SwS is different from the other common snow metrics discussed in this paper (1 April SWE, SCD and SWEmax) because the other metrics have 1 degree of freedom: they provide data on one dimension of the SWE curve. The flexibility of the SwS metric is particularly useful when attempting to quantify storage in snowpacks that have fundamental shifts in their SWE curve. As demonstrated above, the SwS metric can still quantify natural reservoir storage when a snowpack has transitioned from a mountain-type snowpack to an ephemeral type. If a snowpack were to transition from a permanent snowpack to a mountain-type snowpack, the SwS metric would also be able to provide information on the storage.
Of the snow metrics discussed in this paper, SwS is uniquely positioned to capture storage change at aggregated scales, across the full SWE curve. Metrics such as DSO, SWEmax, DSD and SCD are essentially anchor points for the conceptual SWE curve. Although it can be beneficial to note changes at any of these points, change can also happen in between these anchor points, such as an increase in accumulation-season ablation or an increase in ablation-season accumulation, as is seen across mountainous ER3s in Fig. 11. This type of change has already been documented as changing melt fractions (Musselman et al., 2021). At aggregated scales, such as across a watershed, snowpack variability (due to landscape features such as aspect or elevation) influences the SWE curve between the anchor point metrics. Change that occurs between the anchor point metrics is not inherently captured by these metrics. As the SwS metric can accommodate various spatial scales, it is able to capture natural reservoir storage regardless of variability in snow change or snowpack fluctuations that occur in between anchor point metrics.
The widespread losses of SwSA over the last 39 years reported in this study are consistent with the broader narrative of snowpack change literature, which has established declines in snow-covered area, snow cover duration, 1 April SWE and SWEmax, among others (Rupp et al., 2013; Mote et al., 2018; Notarnicola, 2020; Marshall et al., 2019; Bormann et al., 2018; Choi et al., 2010; Huning and AghaKouchak, 2018). Losses of winter snowpack are largely attributed to increasing global temperatures (Hamlet et al., 2005), which have resulted from a combination of natural variability and anthropogenic climate warming (Rupp et al., 2013; Pederson et al., 2013). The declining trends in SwSA are a reflection of declining trends in SwSM in nearly every month, at every scale. The greatest percentage losses in SwSM occur early in the snow season, particularly in November. The loss of early-season SwS is consistent with previous work that used satellite imagery and reported that DSO is occurring later (Notarnicola, 2020). While future work could explore the exact mechanistic drivers of predominantly decreasing SwSA trends, these findings are reasonable in the context of mechanistic drivers explored in other snow change literature. From an energy budget standpoint, snow falling at warmer temperatures (as a result of climate warming) and overall shallower snowpacks (due to reduced snowfall fractions) contribute to reduced cold content and more readily ripening snowpacks (Jennings and Molotch, 2020). Additionally, shallower snowpacks are susceptible to enhanced snowmelt from the albedo feedback as vegetation and soil are exposed (Kapnick and Hall, 2010). Although the majority of SwSA trends are declining, the North Central Plains and the Northeastern Highlands show increasing SwSA trends. Snowmelt and rain-on-snow are known to be flood-generating mechanisms in New England, Minnesota, and along the Mississippi and Missouri rivers (Collins, 2009; Novotny and Stefan, 2007; Wiel et al., 2018; Olsen et al., 1999). The increasing SwS trends in these regions may, therefore, have implications for flood hazards.
Spatial scale has long been a topic of conversation in snow hydrology, as certain processes that occur at very small scales contribute to considerable within-grid-cell heterogeneity as one scales up from point to grid cell to regional scales (Blöschl and Sivapalan, 1995; Molotch and Bales, 2005). This works finds differences in the magnitude and timing of significant changes in SwSA and SwSM when different spatial scales are compared. For example, less of the US landscape shows significant changes in SwSA in the ER3 analysis compared with the grid-cell analysis. Thus, the aggregation of SwSA into ER3s filters some of the grid-cell-scale spatial SwSA trends. Temporally, there is a higher fraction of sites with a significant positive increase in SwSM from October to March in the grid-cell analysis compared with the ER3 analysis. This indicates that local significant increases in SwSM at grid-cell scales are offset by smaller-magnitude increases in SwSM or decreases in SwSM at many locations once the SwSM is aggregated to ER3 scales. From a water resources perspective, these findings underscore the importance of choosing an appropriate aggregation scale in order to accomplish management goals.
In the western US, where snowmelt is vital to supplement warm-season water supplies, about 70 % of runoff in mountainous regions originates as snow (Li et al., 2017). Snowpacks also play an important role in climate, ecological processes and recreation in both eastern and western mountains. Across all mountainous ER3s, there has been a 22 % decline in SwSA over the 39-year period of study. The ER3 mountain ranges considered in this work include the headwaters of 13 of the 18 water basins located in the US, underscoring the importance of these natural reservoirs to water resources. The loss of SwS in these regions is of further concern, as the warm season is projected to increase in length due to anthropogenic climate warming (Mallakpour et al., 2018; Padrón et al., 2020; Siler et al., 2019). Siler et al. (2019) also suggest that declining snow trends may accelerate once the current natural climate mode changes because natural variability has slowed the decline in western snowpacks since the 1980s. The capacity of natural snow reservoirs is declining in most of the western US and across most mountain ranges in the US. As declining trends are expected to continue into the future, monitoring our natural snow reservoirs is essential. Metrics that are highly flexible in space and time (like SwS) can be used to monitor change and evaluate future projections.
SwSA, the changes in SwSA and the variability in SWE are all influenced by elevation. The greatest amount of snow water storage occurs on a disproportionately small fraction of our landscape – at the highest elevations of mountainous ER3s. Almost all mountainous ER3s are losing SwSA at all elevations. In the majority of mountainous ER3s, the highest elevations have experienced the greatest SwSA losses over the last 39 years. The elevation-dependent changes in our natural snow reservoirs are likely associated with documented elevation-dependent changes in temperature and precipitation (Wang et al., 2014; Harpold et al., 2012; Pepin et al., 2015, 2022; Qixiang et al., 2018). Winter temperatures have increased significantly in the recent past (Vose et al., 2017), which increases the vapor pressure deficit in the atmosphere and may enhance sublimation and vapor fluxes (Harpold et al., 2012). Higher elevations have also warmed at faster rates than their low-elevation counterparts, where there have been increasing trends in precipitation (Wang et al., 2014; Pepin et al., 2022). Wang et al. (2014) suggest that elevational warming amplification is likely associated with effective moisture convection. These mechanistic drivers are a plausible explanation for finding the greatest SwSA loss at the highest elevations.
This work also finds elevation-dependent changes in SWE variability. SWE variability has likely increased as a result of winter freezing levels moving to higher elevations (Catalano et al., 2019), an increased fraction of precipitation falling as rain instead of snow and more rain falling on snow (McCabe et al., 2007), all of which are related to increasing winter temperatures. Decreases in SWE variability at higher elevations, where there are declining trends in SwSA, may be a result of shallower snowpacks overall.
A new snow metric, SwS, is defined and used to identify where and to what extent water storage in natural snow reservoirs has already changed in the observational record. Mountains, especially western mountains, play a disproportionate role in natural water storage relative to the surrounding landscape. High-elevation natural snow reservoirs are responsible for the greatest SwSA and have generally experienced the greatest declines in SwSA. Declines in SwSA are associated with a fundamental shift in the shape of the conceptual SWE curve, as it appears to be flattening across stations. As we move into a future of increased snow variability and diminished snowpacks and as more of the winter snow landscape transitions to ephemeral regimes, temporally static metrics such as 1 April SWE and SWEmax may become less representative of our snowpacks. Concurrently, it may be useful to have metrics such as SwS that can adapt to a wide range of circumstances. Spatially and temporally flexible metrics such as SwS may become increasingly valuable, particularly when it comes to monitoring change.
Declining storage in our natural snow reservoirs has broad implications for human and ecological systems. Natural snow reservoirs help to increase water storage far beyond the capacity of artificial reservoirs in the western US, supporting their roll in linking cool-season precipitation to warm-season water demand. As one of the most robust projected impacts of climate change is a continued increase in air temperatures, it is likely that declining trends in SwSA will continue. Water managers, planners and decision-makers will need to account for these declines in natural snow water storage as they relate to streamflows for fish migration and recreation, municipal and agricultural water supplies, and flood hazards. Although this paper does not focus on future predictions of snowpack, SwS could be a useful tool for understanding how our natural snow reservoirs change in the future. Change in our natural snow reservoirs is multidimensional and already happening. Metrics are needed that can capture this complexity of change.
The following is an enumerated list providing links to the publicly available datasets used in this study:
-
SNOTEL (https://wcc.sc.egov.usda.gov/reportGenerator/, United States Department of Agriculture Natural Resources Conservation Service, 2023);
-
UASWE (https://doi.org/10.5067/0GGPB220EX6A, Broxton et al., 2019);
-
NASA SRTM (https://doi.org/10.5067/MEaSUREs/SRTM/, NASA JPL, 2013);
-
USGS Watershed Boundary Dataset (WBD) (https://datagateway.nrcs.usda.gov, US Geological Survey, 2023);
-
EPA Ecoregions (https://www.epa.gov/eco-research/level-iii-and-iv-ecoregions-continental-united-states, US Geological Survey, 2023).
CMA and DFH designed the research questions. CMA determined the methodology and conducted the analysis. DFH conceptualized the SwS metric. CMA prepared the paper with guidance and feedback from DFH.
The contact author has declared that neither of the authors has any competing interests.
Publisher's note: Copernicus Publications remains neutral with regard to jurisdictional claims made in the text, published maps, institutional affiliations, or any other geographical representation in this paper. While Copernicus Publications makes every effort to include appropriate place names, the final responsibility lies with the authors.
This work was supported by funding from the Graduate School Oregon Lottery Award for Academic Excellence from Oregon State University and the Alumni Award from the Water Resources Graduate Program at Oregon State University. We thank Paul C. Loikith and Linnia R. Hawkins for their contributions to this research.
This paper was edited by Xing Yuan and reviewed by two anonymous referees.
Barnett, T. P., Adam, J. C., and Lettenmaier, D. P.: Potential impacts of a warming climate on water availability in snow-dominated regions, Nature, 438, 303–309, https://doi.org/10.1038/nature04141, 2005. a, b
Blöschl, G. and Sivapalan, M.: Scale issues in hydrological modelling: A review, Hydrol. Process., 9, 251–290, https://doi.org/10.1002/hyp.3360090305, 1995. a
Boon, S.: Snow accumulation and ablation in a beetle-killed pine stand in Northern Interior British Columbia, J. Ecosyst. Manage., 8, 1–13, https://doi.org/10.22230/jem.2007v8n3a369, 2007. a
Bormann, K. J., Brown, R. D., Derksen, C., and Painter, T. H.: Estimating snow-cover trends from space, Nat. Clim. Change, 8, 924–928, https://doi.org/10.1038/s41558-018-0318-3, 2018. a
Broxton, P., Zeng, X., and Dawson, N.: Daily 4 km Gridded SWE and Snow Depth from Assimilated In-Situ and Modeled Data over the Conterminous US, Version 1, NASA National Snow and Ice Data Center Distributed Active Archive Center [data set], https://doi.org/10.5067/0GGPB220EX6A, 2019. a, b
Catalano, A. J., Loikith, P. C., and Aragon, C. M.: Spatiotemporal Variability of Twenty-First-Century Changes in Site-Specific Snowfall Frequency Over the Northwest United States, Geophys. Res. Lett., 46, 10122–10131, https://doi.org/10.1029/2019GL084401, 2019. a
Choi, G., Robinson, D. A., and Kang, S.: Changing Northern Hemisphere Snow Seasons, J. Climate, 23, 5305–5310, https://doi.org/10.1175/2010JCLI3644.1, 2010. a
Collins, M. J.: Evidence for Changing Flood Risk in New England Since the Late 20th Century1, J. Am. Water Resour. Assoc., 45, 279–290, https://doi.org/10.1111/j.1752-1688.2008.00277.x, 2009. a
Daly, C., Halbleib, M., Smith, J. I., Gibson, W. P., Doggett, M. K., Taylor, G. H., Curtis, J., and Pasteris, P. P.: Physiographically sensitive mapping of climatological temperature and precipitation across the conterminous United States, Int. J. Climatol., 28, 2031–2064, https://doi.org/10.1002/joc.1688, 2008. a
Dawson, N., Broxton, P., and Zeng, X.: Evaluation of Remotely Sensed Snow Water Equivalent and Snow Cover Extent over the Contiguous United States, J. Hydrometeorol., 19, 1777–1791, https://doi.org/10.1175/JHM-D-18-0007.1, 2018. a
Easterling, D., Arnold, J., Knutson, T., Kunkel, K., LeGrande, A., Leung, L., Vose, R., Waliser, D., and Wehner, M.: Ch. 7: Precipitation Change in the United States. Climate Science Special Report: Fourth National Climate Assessment, Volume I, Tech. rep., US Global Change Research Program, https://doi.org/10.7930/J0H993CC, 2017. a
Farr, T. G., Rosen, P. A., Caro, E., Crippen, R., Duren, R., Hensley, S., Kobrick, M., Paller, M., Rodriguez, E., Roth, L., Seal, D., Shaffer, S., Shimada, J., Umland, J., Werner, M., Oskin, M., Burbank, D., and Alsdorf, D.: The Shuttle Radar Topography Mission, Rev. Geophys., 45, RG2004, https://doi.org/10.1029/2005RG000183, 2007. a
Gleason, K. E., McConnell, J. R., Arienzo, M. M., Chellman, N., and Calvin, W. M.: Four-fold increase in solar forcing on snow in western U.S. burned forests since 1999, Nat. Commun., 10, 2026, https://doi.org/10.1038/s41467-019-09935-y, 2019. a
Hale, K. E., Jennings, K. S., Musselman, K. N., Livneh, B., and Molotch, N. P.: Recent decreases in snow water storage in western North America, Commun. Earth Environ., 4, 1–11, https://doi.org/10.1038/s43247-023-00751-3, 2023. a
Hamed, K. and Rao, A.: A modified Mann-Kendall trend test for autocorrelated data, J. Hydrol., 204, 182–196, https://doi.org/10.1016/S0022-1694(97)00125-X, 1998. a
Hamlet, A. F. and Lettenmaier, D. P.: Effects of 20th century warming and climate variability on flood risk in the western U.S., Water Resour. Res., 43, W06427, https://doi.org/10.1029/2006WR005099, 2007. a
Hamlet, A. F., Mote, P. W., Clark, M. P., and Lettenmaier, D. P.: Effects of Temperature and Precipitation Variability on Snowpack Trends in the Western United States, J. Climate, 18, 4545–4561, https://doi.org/10.1175/JCLI3538.1, 2005. a
Harpold, A., Brooks, P., Rajagopal, S., Heidbuchel, I., Jardine, A., and Stielstra, C.: Changes in snowpack accumulation and ablation in the intermountain west, Water Resour. Res., 48, W11501, https://doi.org/10.1029/2012WR011949, 2012. a, b
Hatchett, B. J.: Seasonal and Ephemeral Snowpacks of the Conterminous United States, Hydrology, 8, 32, https://doi.org/10.3390/hydrology8010032, 2021. a
Hirsch, R. M., Slack, J. R., and Smith, R. A.: Techniques of trend analysis for monthly water quality data, Water Resour. Res., 18, 107–121, https://doi.org/10.1029/WR018i001p00107, 1982. a
Huning, L. S. and AghaKouchak, A.: Mountain snowpack response to different levels of warming, P. Natl. Acad. Sci. USA, 115, 10932–10937, https://doi.org/10.1073/pnas.1805953115, 2018. a
Hussain, M. and Mahmud, I.: pyMannKendall: a python package for non parametric Mann Kendall family of trend tests, J. Open Source Softw., 4, 1556, https://doi.org/10.21105/joss.01556, 2019. a
Immerzeel, W. W., Lutz, A. F., Andrade, M., Bahl, A., Biemans, H., Bolch, T., Hyde, S., Brumby, S., Davies, B. J., Elmore, A. C., Emmer, A., Feng, M., Fernández, A., Haritashya, U., Kargel, J. S., Koppes, M., Kraaijenbrink, P. D. A., Kulkarni, A. V., Mayewski, P. A., Nepal, S., Pacheco, P., Painter, T. H., Pellicciotti, F., Rajaram, H., Rupper, S., Sinisalo, A., Shrestha, A. B., Viviroli, D., Wada, Y., Xiao, C., Yao, T., and Baillie, J. E. M.: Importance and vulnerability of the world's water towers, Nature, 577, 364–369, https://doi.org/10.1038/s41586-019-1822-y, 2020. a, b
Jennings, K. S. and Molotch, N. P.: Snowfall Fraction, Cold Content, and Energy Balance Changes Drive Differential Response to Simulated Warming in an Alpine and Subalpine Snowpack, Front. Earth Sci., 8, 186, https://doi.org/10.3389/feart.2020.00186, 2020. a
Kapnick, S. and Hall, A.: Observed Climate–Snowpack Relationships in California and their Implications for the Future, J. Climate, 23, 3446–3456, https://doi.org/10.1175/2010JCLI2903.1, 2010. a
Krogh, S. A., Broxton, P. D., Manley, P. N., and Harpold, A. A.: Using Process Based Snow Modeling and Lidar to Predict the Effects of Forest Thinning on the Northern Sierra Nevada Snowpack, Front. Forests Global Change, 3, 21, https://doi.org/10.3389/ffgc.2020.00021, 2020. a
Li, D., Wrzesien, M. L., Durand, M., Adam, J., and Lettenmaier, D. P.: How much runoff originates as snow in the western United States, and how will that change in the future?, Geophys. Res. Lett., 44, 6163–6172, https://doi.org/10.1002/2017GL073551, 2017. a, b, c
Liston, G. E. and Elder, K.: A Distributed Snow-Evolution Modeling System (SnowModel), J. Hydrometeorol., 7, 1259–1276, https://doi.org/10.1175/JHM548.1, 2006. a, b
Livneh, B. and Badger, A. M.: Drought less predictable under declining future snowpack, Nat. Clim. Change, 10, 452–458, https://doi.org/10.1038/s41558-020-0754-8, 2020. a
Lute, A. C., Abatzoglou, J. T., and Hegewisch, K. C.: Projected changes in snowfall extremes and interannual variability of snowfall in the western United States, Water Resour. Res., 51, 960–972, https://doi.org/10.1002/2014WR016267, 2015. a
Mallakpour, I., Sadegh, M., and AghaKouchak, A.: A new normal for streamflow in California in a warming climate: Wetter wet seasons and drier dry seasons, J. Hydrol., 567, 203–211, https://doi.org/10.1016/j.jhydrol.2018.10.023, 2018. a
Marshall, A. M., Abatzoglou, J. T., Link, T. E., and Tennant, C. J.: Projected Changes in Interannual Variability of Peak Snowpack Amount and Timing in the Western United States, Geophys. Res. Lett., 46, 8882–8892, https://doi.org/10.1029/2019GL083770, 2019. a
McCabe, G. J., Clark, M. P., and Hay, L. E.: Rain-on-Snow Events in the Western United States, B. Am. Meteorol. Soc., 88, 319–328, https://doi.org/10.1175/BAMS-88-3-319, 2007. a
McMahon, G., Gregonis, S. M., Waltman, S. W., Omernik, J. M., Thorson T. D., Freeouf, J. A., Rorick, A. H., and Keys, J. E.: Developing a Spatial Framework of Common Ecological Regions for the Conterminous United States, Environ. Manage., 28, 293–316, https://doi.org/10.1007/s0026702429, 2001. a
Molotch, N. P. and Bales, R. C.: Scaling snow observations from the point to the grid element: Implications for observation network design, Water Resour. Res., 41, W11421, https://doi.org/10.1029/2005WR004229, 2005. a
Morán-Tejeda, E., López-Moreno, J. I., and Beniston, M.: The changing roles of temperature and precipitation on snowpack variability in Switzerland as a function of altitude, Geophys. Res. Lett., 40, 2131–2136, https://doi.org/10.1002/grl.50463, 2013. a, b
Mote, P. W., Li, S., Lettenmaier, D. P., Xiao, M., and Engel, R.: Dramatic declines in snowpack in the western US, npj Clim. Atmos. Sci., 1, 1–6, https://doi.org/10.1038/s41612-018-0012-1, 2018. a
Musselman, K. N., Addor, N., Vano, J. A., and Molotch, N. P.: Winter melt trends portend widespread declines in snow water resources, Nat. Clim. Change, 11, 418–424, https://doi.org/10.1038/s41558-021-01014-9, 2021. a, b
NASA JPL: NASA Shuttle Radar Topography Mission Global 1 arc second [Data set], NASA EOSDIS Land Processes Distributed Active Archive Center [data set], https://doi.org/10.5067/MEaSUREs/SRTM/SRTMGL1.003, 2013. a
Nolin, A. W., Sproles, E. A., Rupp, D. E., Crumley, R. L., Webb, M. J., Palomaki, R. T., and Mar, E.: New snow metrics for a warming world, Hydrol. Process., 35, e14262, https://doi.org/10.1002/hyp.14262, 2021. a
Notarnicola, C.: Hotspots of snow cover changes in global mountain regions over 2000–2018, Remote Sens. Environ., 243, 111781, https://doi.org/10.1016/j.rse.2020.111781, 2020. a, b
Novotny, E. V. and Stefan, H. G.: Stream flow in Minnesota: Indicator of climate change, J. Hydrol., 334, 319–333, https://doi.org/10.1016/j.jhydrol.2006.10.011, 2007. a
NSIDC – National Snow and Ice Data Center: Snow Data Assimilation System (SNODAS) Data Products at NSIDC, Version 1, NSIDC – National Snow and Ice Data Center [data set], https://doi.org/10.7265/N5TB14TC, 2004. a
Ohmura, A.: Enhanced temperature variability in high-altitude climate change, Theor. Appl. Climatol., 110, 499–508, https://doi.org/10.1007/s00704-012-0687-x, 2012. a
Olsen, J. R., Stedinger, J. R., Matalas, N. C., and Stakhiv, E. Z.: Climate Variability and Flood Frequency Estimation for the Upper Mississippi and Lower Missouri Rivers1, J. Am. Water Resour. Assoc., 35, 1509–1523, https://doi.org/10.1111/j.1752-1688.1999.tb04234.x, 1999. a
Omernik, J. M. and Griffith, G. E.: Ecoregions of the Conterminous United States: Evolution of a Hierarchical Spatial Framework, Environ. Manage., 54, 1249–1266, https://doi.org/10.1007/s00267-014-0364-1, 2014. a
Padrón, R. S., Gudmundsson, L., Decharme, B., Ducharne, A., Lawrence, D. M., Mao, J., Peano, D., Krinner, G., Kim, H., and Seneviratne, S. I.: Observed changes in dry-season water availability attributed to human-induced climate change, Nat. Geosc., 13, 477–481, https://doi.org/10.1038/s41561-020-0594-1, 2020. a
Pederson, G. T., Betancourt, J. L., and McCabe, G. J.: Regional patterns and proximal causes of the recent snowpack decline in the Rocky Mountains, U.S., Geophys. Res. Lett., 40, 1811–1816, https://doi.org/10.1002/grl.50424, 2013. a
Pendergrass, A. G., Knutti, R., Lehner, F., Deser, C., and Sanderson, B. M.: Precipitation variability increases in a warmer climate, Sci. Rep., 7, 17966, https://doi.org/10.1038/s41598-017-17966-y, 2017. a
Pepin, N., Bradley, R. S., Diaz, H. F., Baraer, M., Caceres, E. B., Forsythe, N., Fowler, H., Greenwood, G., Hashmi, M. Z., Liu, X. D., Miller, J. R., Ning, L., Ohmura, A., Palazzi, E., Rangwala, I., Schöner, W., Severskiy, I., Shahgedanova, M., Wang, M. B., Williamson, S. N., Yang, D. Q., and Mountain Research Initiative EDW Working Group: Elevation-dependent warming in mountain regions of the world, Nat. Clim. Change, 5, 424–430, https://doi.org/10.1038/nclimate2563, 2015. a
Pepin, N. C., Arnone, E., Gobiet, A., Haslinger, K., Kotlarski, S., Notarnicola, C., Palazzi, E., Seibert, P., Serafin, S., Schöner, W., Terzago, S., Thornton, J. M., Vuille, M., and Adler, C.: Climate Changes and Their Elevational Patterns in the Mountains of the World, Rev. Geophys., 60, e2020RG000730, https://doi.org/10.1029/2020RG000730, 2022. a, b
Pugh, E. and Small, E.: The impact of pine beetle infestation on snow accumulation and melt in the headwaters of the Colorado River, Ecohydrology, 5, 467–477, https://doi.org/10.1002/eco.239, 2012. a
Qixiang, W., Wang, M., and Fan, X.: Seasonal patterns of warming amplification of high-elevation stations across the globe, Int. J. Climatol., 38, 3466–3473, https://doi.org/10.1002/joc.5509, 2018. a
Rupp, D. E., Mote, P. W., Bindoff, N. L., Stott, P. A., and Robinson, D. A.: Detection and Attribution of Observed Changes in Northern Hemisphere Spring Snow Cover, J. Climate, 26, 6904–6914, https://doi.org/10.1175/JCLI-D-12-00563.1, 2013. a, b
Scalzitti, J., Strong, C., and Kochanski, A.: Climate change impact on the roles of temperature and precipitation in western U.S. snowpack variability, Geophys. Res. Lett., 43, 5361–5369, https://doi.org/10.1002/2016GL068798, 2016. a, b
Serreze, M. C., Clark, M. P., Armstrong, R. L., McGinnis, D. A., and Pulwarty, R. S.: Characteristics of the western United States snowpack from snowpack telemetry (SNOTEL) data, Water Resour. Res., 35, 2145–2160, https://doi.org/10.1029/1999WR900090, 1999. a
Siirila-Woodburn, E. R., Rhoades, A. M., Hatchett, B. J., Huning, L. S., Szinai, J., Tague, C., Nico, P. S., Feldman, D. R., Jones, A. D., Collins, W. D., and Kaatz, L.: A low-to-no snow future and its impacts on water resources in the western United States, Nat. Rev. Earth Environ., 2, 800–819, https://doi.org/10.1038/s43017-021-00219-y, 2021. a, b
Siler, N., Proistosescu, C., and Po-Chedley, S.: Natural Variability Has Slowed the Decline in Western U.S. Snowpack Since the 1980s, Geophys. Res. Lett., 46, 346–355, https://doi.org/10.1029/2018GL081080, 2019. a, b
Sospedra-Alfonso, R., Melton, J. R., and Merryfield, W. J.: Effects of temperature and precipitation on snowpack variability in the Central Rocky Mountains as a function of elevation, Geophys. Res. Lett., 42, 4429–4438, https://doi.org/10.1002/2015GL063898, 2015. a, b
Sturm, M.: White water: Fifty years of snow research in WRR and the outlook for the future, Water Resour. Res., 51, 4948–4965, https://doi.org/10.1002/2015WR017242, 2015. a
Sturm, M., Holmgren, J., and Liston, G. E.: A Seasonal Snow Cover Classification System for Local to Global Applications, J. Climate, 8, 1261–1283, https://doi.org/10.1175/1520-0442(1995)008<1261:ASSCCS>2.0.CO;2, 1995. a
Sturm, M., Goldstein, M. A., and Parr, C.: Water and life from snow: A trillion dollar science question, Water Resour. Res., 53, 3534–3544, https://doi.org/10.1002/2017WR020840, 2017. a
Sun, N., Yan, H., Wigmosta, M. S., Lundquist, J., Dickerson-Lange, S., and Zhou, T.: Forest Canopy Density Effects on Snowpack Across the Climate Gradients of the Western United States Mountain Ranges, Water Resour. Res., 58, e2020WR029194, https://doi.org/10.1029/2020WR029194, 2022. a
Troendle, C. A. and Reuss, J. O.: Effect of clear cutting on snow accumulation and water outflow at Fraser, Colorado, Hydrol. Earth Syst. Sci., 1, 325–332, https://doi.org/10.5194/hess-1-325-1997, 1997. a
United States Department of Agriculture Natural Resources Conservation Service: Air & Water Database Report Generato, https://wcc.sc.egov.usda.gov/reportGenerator/ (last access: 3 January 2023), 2023. a
United States Environmental Protection Agency: Level III and IV Ecoregions of the Continental United States, https://www.epa.gov/eco-research/level-iii-and-iv-ecoregions-continental-united-states (last access: 3 January 2023), 2023. a
US Geological Survey: Watershed Boundary Dataset (WBD) – USGS National Map Downloadable Data Collection, US Geological Survey, https://datagateway.nrcs.usda.gov (last access: 3 January 2023), 2023. a
Viviroli, D., Dürr, H. H., Messerli, B., Meybeck, M., and Weingartner, R.: Mountains of the world, water towers for humanity: Typology, mapping, and global significance, Water Resour. Res., 43, W07447, https://doi.org/10.1029/2006WR005653, 2007. a
Vose, R. S., Easterling, D. R., Kunkel, K. E., LeGrande, A. N., and Wehner, M. F.: Temperature changes in the United States, in: Climate Science Special Report: Fourth National Climate Assessment, Volume I, edited by: Wuebbles, D. J., Fahey, D. W., Hibbard, K. A., Dokken, D. J., Stewart, B. C., and Maycock, T. K., US Global Change Research Program, 185–206, https://doi.org/10.7930/J0N29V45, 2017. a
Wang, Q., Fan, X., and Wang, M.: Recent warming amplification over high elevation regions across the globe, Clim. Dynam., 43, 87–101, https://doi.org/10.1007/s00382-013-1889-3, 2014. a, b, c
Wiel, K. v. d., Kapnick, S. B., Vecchi, G. A., Smith, J. A., Milly, P. C. D., and Jia, L.: 100-Year Lower Mississippi Floods in a Global Climate Model: Characteristics and Future Changes, J. Hydrometeorol., 19, 1547–1563, https://doi.org/10.1175/JHM-D-18-0018.1, 2018. a
Winkler, R., Boon, S., Zimonick, B., and Spittlehouse, D.: Snow accumulation and ablation response to changes in forest structure and snow surface albedo after attack by mountain pine beetle, Hydrol. Process., 28, 197–209, https://doi.org/10.1002/hyp.9574, 2014. a
Winkler, R. D., Spittlehouse, D. L., and Golding, D. L.: Measured differences in snow accumulation and melt among clearcut, juvenile, and mature forests in southern British Columbia, Hydrol. Process., 19, 51–62, https://doi.org/10.1002/hyp.5757, 2005. a
Zeng, X., Broxton, P., and Dawson, N.: Snowpack Change From 1982 to 2016 Over Conterminous United States, Geophys. Res. Lett., 45, 12940–12947, https://doi.org/10.1029/2018GL079621, 2018. a, b
Zhang, X., Zwiers, F. W., Hegerl, G. C., Lambert, F. H., Gillett, N. P., Solomon, S., Stott, P. A., and Nozawa, T.: Detection of human influence on twentieth-century precipitation trends, Nature, 448, 461–465, https://doi.org/10.1038/nature06025, 2007. a