the Creative Commons Attribution 4.0 License.
the Creative Commons Attribution 4.0 License.
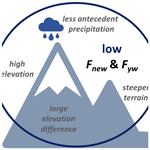
Monthly new water fractions and their relationships with climate and catchment properties across Alpine rivers
Marius G. Floriancic
Michael P. Stockinger
James W. Kirchner
Christine Stumpp
The Alps are a key water resource for central Europe, providing water for drinking, agriculture, and hydropower production. Thus, understanding runoff generation processes of Alpine streams is important for sustainable water management. It is currently unclear how much streamflow is derived from old water stored in the subsurface and how much stems from more recent precipitation that reaches the stream via near-surface quick flow processes. It is also unclear how this partitioning varies across different Alpine catchments in response to hydroclimatic forcing and catchment characteristics. Here, we use stable water isotope time series in precipitation and streamflow to quantify the young water fractions (Fyw; i.e., the fraction of water younger than approximately 2–3 months) and new water fractions (Fnew; here, the fraction of water younger than 1 month) in streamflow from 32 Alpine catchments. We contrast these measures of water age between summer and winter and between wet and dry periods and then correlate them with hydroclimatic variables and physical catchment properties.
New water fractions varied between 3.5 % and 9.6 %, with values of 9.2 % in rainfall-dominated catchments, 9.6 % in hybrid catchments, and 3.5 % in snow-dominated catchments (mean across all catchments of 7.1 %). Young water fractions were approximately twice as large (reflecting their longer timescale) and ranged between 10.1 % and 17.6 %, with values of 17.6 % in rainfall-dominated catchments, 16.6 % in hybrid catchments, and 10.1 % in snow-dominated catchments (mean across all catchments of 14.3 %). New water fractions were negatively correlated with catchment size (Spearman rank correlation, rS, of −0.38), q95 baseflow (), catchment elevation (), total catchment relief (), and the fraction of slopes steeper than 40° (). Large new water fractions, implying faster transmission of precipitation to streamflow, are more prevalent in small catchments, at low elevations, with small elevation differences, and with large fractions of forest cover (rS=0.36). New water fractions averaged 3.3 % following dry antecedent conditions, compared with 9.3 % after wet antecedent conditions. Our results quantify how hydroclimatic and physical drivers shape the partitioning of old and new waters across the Alps, thus indicating which landscapes transmit recent precipitation more readily to streamflow and which landscapes tend to retain water over longer periods. Our results further illustrate how new water fractions may find relationships that remained invisible with young water fractions.
- Article
(5831 KB) - Full-text XML
-
Supplement
(1670 KB) - BibTeX
- EndNote
The Alps are often referred to as the “water tower of Europe”, as they contribute a disproportionally high fraction of the streamflow of European rivers (Weingartner et al., 2007). They provide water for agriculture, domestic use, and hydropower production, not only in the Alpine region but also for approximately 170 million people living in the downstream basins (Mastrotheodoros et al., 2020). Thus, it is important to understand the origins of streamflow in Alpine rivers and how they might change in future climates (Briffa et al., 2009). So far, little is known about the transport and storage of the waters that become Alpine river flow, i.e., the extent to which streamflow (1) originates from old water stored in the subsurface or (2) consists of more recent precipitation reaching the stream via near-surface quick flow processes. Across the Alps, contributions from both slow subsurface flow and fast surface or near-surface flow processes are poorly understood (Hayashi, 2019). It is likewise unclear how these slow and fast flow processes, and thus the partitioning of old and new water in Alpine streams, are related to hydroclimatic forcing and physical catchment characteristics across different Alpine catchments.
Stable water isotopes are essential tools for estimating the contribution of different sources to streamflow and for assessing how this source partitioning varies with precipitation characteristics and catchment wetness conditions (Segura et al., 2012). Stable water isotopes have been measured in many catchments worldwide, and data compilations are available for the globe, e.g., the Global Network of Isotopes in Rivers (GNIR; IAEA, 2022b) and the Global Network of Isotopes in Precipitation (GNIP; IAEA, 2022a), and for some regions and countries, e.g., for Switzerland (Staudinger et al., 2020), as well as for individual intensively studied catchments (e.g., Hubbard Brook, Plynlimon, and Alptal). Although most multi-catchment time series have been sampled at low temporal frequency, they can nonetheless be used to assess the mixture of streamflow sources on timescales similar to their sampling intervals. Thus, they can yield insights into how long it takes until precipitation becomes streamflow or, in turn, how much streamflow is coming from recent precipitation versus from water that has been stored in the catchment over longer timescales (Hrachowitz et al., 2009).
Many previous studies have assessed the fraction of “event” water by hydrograph separation using two-component mixing models (Klaus and McDonnell, 2013). Prior to the widespread use of stable water isotopes and other conservative tracers, it was assumed that storm flow mostly originated from recent rainfall that traveled rapidly to the stream via overland flow or preferential subsurface flow paths (Kirchner, 2003). This conceptual model was overthrown by tracer studies that showed that, although stream discharge responds quickly to rainfall inputs, recent precipitation makes up only a small fraction of storm flow (Sklash and Farvolden, 1979; Neal and Rosier, 1990; McDonnell and Beven, 2014), a phenomenon often referred to as the “old water paradox” (Kirchner, 2003; McDonnell et al., 2010). Instead of flowing rapidly to the stream, most precipitation instead infiltrates and mobilizes older water from subsurface storage (von Freyberg et al., 2017, 2018). Thus, subsurface storage supplies a large fraction of streamflow, not only under baseflow conditions but also during high-flow events (Browne, 1981; Fleckenstein et al., 2006; Floriancic et al., 2022).
Although aquifer waters can be very old (Gleeson et al., 2016; Jasechko et al., 2017), stream waters derived from them are typically much younger (Berghuijs and Kirchner, 2017). The explanation for this apparent paradox is that hydraulic conductivities in aquifers vary by orders of magnitude (Gleeson et al., 2011b), with the faster flow paths transmitting disproportionally more water, which, because it flows faster, is younger than the waters left behind in the slower flow paths (Berghuijs and Kirchner, 2017; Kirchner et al., 2023). In global-scale syntheses, Jasechko et al. (2016, 2017) found that, although most groundwaters are dominated by fossil waters, 25 % of global streamflow is younger than 1.5–3 months. According to Jasechko et al. (2016), rivers draining mountainous regions tend to have smaller fractions of young water than rivers draining flatter terrain. Jasechko et al. (2016) explained this finding by arguing that steeper areas allow for deeper vertical infiltration and thus a greater predominance of deeper, slower flow paths. However, it remains unclear if the association between steep terrain and young water fractions is consistent across the European Alps and the extent to which these results are influenced by other hydroclimatic variables and physical catchment properties.
Recent studies have assessed the impact of catchment properties on the fraction of young water, defined as the fraction of streamflow that is younger than approximately 2–3 months, which can be inferred from the amplitude of seasonal tracer cycles in precipitation and streamflow (Kirchner, 2016a, b) For example, von Freyberg et al. (2018) found that young water fractions tend to be smaller in steeper terrain, and Ceperley et al. (2020) found a decrease in young water fractions above an elevation of 1500 m a.s.l. (meters above sea level) across Swiss and Italian Alpine catchments. In their work, von Freyberg et al. (2018) also found that higher fractions of young water were associated with higher antecedent catchment wetness, as well as with hydroclimatic factors and catchment characteristics that favor faster transmission of waters to the stream (wet climates, low subsurface permeability, and high drainage density). Stockinger et al. (2019) found that young water fractions were not related to climate but were inversely related to the ratio of average discharge to average precipitation. Catchment area has previously been identified as a major control on catchment mean transit time (DeWalle et al., 1995; Soulsby et al., 2000) but has not been found to be significantly related to young water fractions (von Freyberg et al., 2018).
Gentile et al. (2023) argued that young water fractions should depend on the fraction of catchment area covered by unconsolidated debris deposits and the fraction of baseflow, and von Freyberg et al. (2018) found a significant positive correlation between young water fractions and the fraction of the catchment covered by forests. Previous studies of young water fractions in the Alpine region have primarily focused on small headwater catchments (Gentile et al., 2023; von Freyberg et al., 2018; Ceperley et al., 2020) and have not included larger downstream basins. Whereas young water fractions have been widely used to assess how hydroclimatic and physiographic properties shape catchment transport, new water fractions have thus far remained underexploited for this purpose. To date, no studies have systematically linked new water fractions to hydroclimatic drivers and physical catchment properties, using datasets that include both headwater catchments and larger downstream basins.
For this study, we compiled time series of streamflow, precipitation, and stable water isotopes for 32 catchments across the Austrian and Swiss Alps, spanning a wide range of catchment sizes and elevation gradients. We analyzed these time series using two recently developed methods for assessing the relative proportions of younger and older water in streamflow, young water fractions (Fyw; Kirchner, 2016a, b; von Freyberg et al., 2017) and new water fractions (Fnew; Kirchner, 2019; Knapp et al., 2019; Kirchner and Knapp, 2020b), to address the following research questions:
-
How much new and young water can be found in Alpine catchments of different sizes?
-
How do new water fractions vary among different wetness conditions and seasons?
-
How do new water fractions vary with antecedent precipitation across Alpine rivers?
-
How do new water fractions propagate downstream from headwater catchments to the large basins of the Danube and Rhine catchments?
-
Which hydroclimatic variables (climate and streamflow response) and physical catchment properties (topography, lithology, and land use) are associated with larger or smaller fractions of new water?
2.1 Precipitation and streamflow data
The analysis is based on 32 Austrian and Swiss Alpine catchments for which streamflow isotope data were available (see Fig. 1 and Table 1). Daily discharge time series for 12 of the 20 Swiss sites were obtained from the CH-IRP database (Staudinger et al., 2020). Discharge time series for the remaining 8 Swiss sites and for all 12 Austrian sites were obtained from the Federal Office of the Environment “Hydrological data and forecasts” database (FOEN; 2022a) and the “Hydrographisches Jahrbuch” contained in the WISA database (Umweltbundesamt, 2022a), respectively. Daily catchment averages of precipitation for all 32 sites (12 WISA, 8 FOEN, and 12 CH-IRP) were obtained from the gridded precipitation dataset E-OBS (version 20.0e) at a 0.1° resolution covering the period from 1980 to 2014 (Cornes et al., 2018). For this purpose, the catchment boundaries for all 32 gauging stations were extracted from the Copernicus EU-DEM v1.1 at a 25 m resolution using the ArcMap 10.6 Spatial Analyst toolbox.
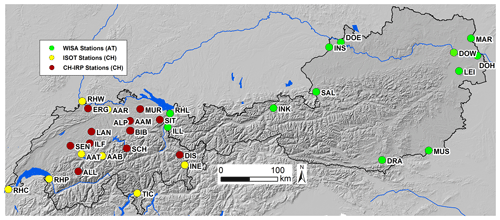
Figure 1Location of the streamflow isotope sampling sites in Austria (WISA database – green markers) and Switzerland (ISOT database – yellow markers; CH-IRP database – red markers). The hillshade in this map is based on the EU-DEM v1.1, available due to funding from the European Union.
Table 1Data summary for study catchments, including the original database ID, site name abbreviation (site code), river and gauge names, database name, latitude and longitude at the catchment outlet, and number of samples for δ18O and δ2H in the publicly available streamflow isotope datasets: WISA (national Austrian isotope database; n=12), ISOT (national Swiss isotope database; n=8), and CH-IRP (Staudinger et al., 2020; n=12).
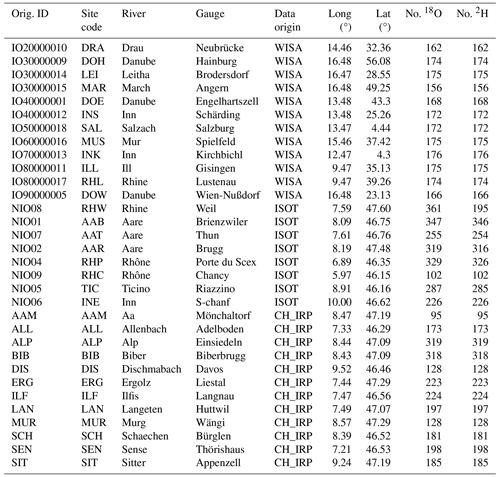
2.2 Isotope data
We compiled streamflow and precipitation isotope data for 32 catchments across the Austrian and Swiss Alps (see Fig. 1 and Table 1). Monthly streamflow isotopes for the 12 Austrian sites were obtained from the WISA “H2O Fachdatenbank” database (Umweltbundesamt, 2022b); for the 8 Swiss stations, this information was sourced from the NAQUA ISOT (Nationalen Grundwasserbeobachtung – Isotopendaten) database (FOEN, 2022b; Schürch et al., 2003). Streamflow isotopes for 12 additional stations across the Swiss Alps were obtained from the CH-IRP database (Staudinger et al., 2020). Many of the study catchments lack direct measurements of precipitation isotopes within the catchment boundaries. In all but the largest catchments where direct measurements are available, they are available only at single sampling locations. Because precipitation isotopes vary with altitude and other factors, we did not interpolate individual station measurements across our study catchments; instead, we relied on the monthly gridded precipitation isotope reanalysis database Piso.AI (Nelson et al., 2021), which we averaged within the boundaries of each of our study catchments.
2.3 Hydroclimatic variables
We assessed the relationship of isotopic signatures with 9 hydroclimatic variables (Table 2) in all 32 catchments. Daily catchment-averaged precipitation from the gridded precipitation dataset E-OBS (version 20.0e) at a 0.1° resolution (Cornes et al., 2018) was used to calculate the mean annual, winter (November–April) and summer (May–October) precipitation for each of the 32 catchments. The catchment boundaries were used to average mean monthly potential evapotranspiration (PET) across each catchment from the Global Aridity Index and Potential Evapotranspiration Climate Database v2 (Trabucco and Zomer, 2019); from these monthly averages, we also calculated annual, winter (November–April), and summer (May–October) PET. The discharge fraction (qP−1) was calculated by dividing the total annual streamflow (in mm) by the total annual precipitation. The discharge that is exceeded 95% of the time (q95 – in mm d−1) was obtained from the streamflow duration curve by calculating the 5th percentile of all streamflow values. The use of q instead of Q indicates that the values are divided by area to obtain an area-normalized discharge quantile (in mm d−1 rather than m3 s−1).
Table 2Averages of major hydroclimatic variables across the 32 Alpine catchments: mean annual precipitation, winter precipitation, summer precipitation, mean annual potential evapotranspiration, winter potential evapotranspiration, and summer potential evapotranspiration (all in mm); the ratio of annual streamflow to annual precipitation (as fraction, F); and the discharge reached or exceeded 95 % of the time (in mm d−1).
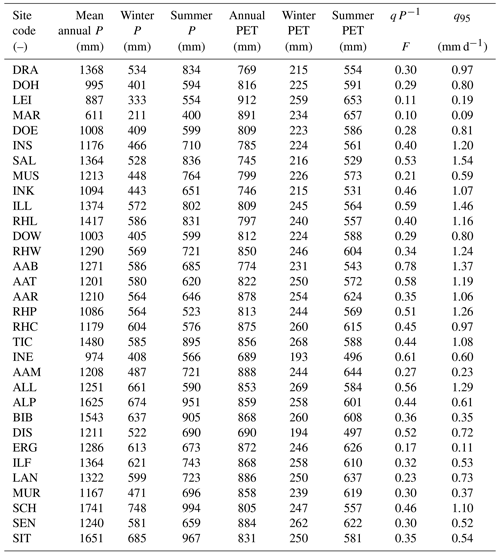
2.4 Physical catchment properties
We assessed the relationship between isotopic signatures and 9 physical catchment properties (Table 3) across all 32 study catchments. We calculated the following six topographic properties: total catchment area, mean catchment elevation, elevation difference (calculated from maximum elevation − minimum elevation), mean catchment slope, the fraction of slope below 10°, and the fraction of slope above 40°. To assess the effect of lithology, we calculated the fraction of (potentially karstified) carbonate sedimentary rocks and the fraction of unconsolidated debris deposits for all catchments. Because plants, such as trees, affect the hydrological cycle by increasing water losses through transpiration, we also calculated the fraction of forest cover for each catchment. From the catchment boundaries, the total catchment area was calculated with the Tabulate Area tool of ArcMap 10.6. The mean, minimum, and maximum catchment elevations were calculated from the Copernicus EU-DEM v1.1 at a 25 m resolution using the ArcMap 10.6 Zonal Statistics tool. The mean slope, fraction of slope below 10°, and fraction of slope above 40° were also calculated from the Copernicus EU-DEM v1.1. From the 0.5° resolution raster map GLiM (Global Lithological Map; Hartmann and Moosdorf, 2012), the fraction of area of potentially karstified carbonate sedimentary rocks (GLiM class SC) and the fraction of area covered with unconsolidated debris deposits (GLiM class SU) were calculated. From the Copernicus CORINE land cover (CLC 2018), the fraction of area covered by forests was calculated by combining the following three CLC classes: broad-leaved forest, coniferous forest, and mixed forest. All geodata mentioned above were extracted using the obtained catchment boundaries and the ArcMap 10.6 Zonal Statistics tool.
Table 3Catchment averages of physical catchment properties across the 32 Alpine catchments: total catchment area, mean catchment elevation, elevation difference (max–min), mean catchment slope, fraction of area with a slope < 10°, fraction of area with a slope > 40°, fraction of area with potentially karstified carbonate sedimentary rocks, fraction of area with unconsolidated debris deposits, and fraction of area covered by forests.
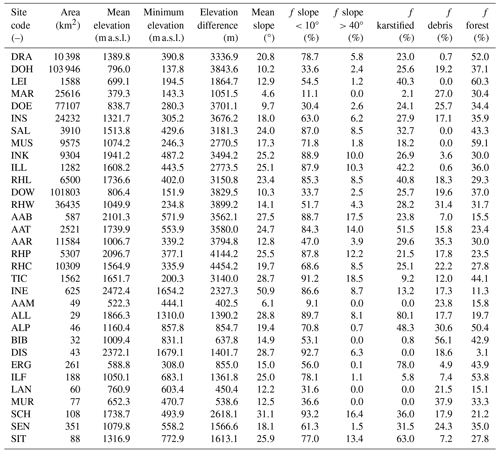
2.5 Calculations of young water fractions (Fyw) and new water fractions (Fnew)
In seasonal climates, summer precipitation is isotopically heavier and winter precipitation is isotopically lighter, resulting in a seasonal cycle of precipitation isotopes. Kirchner (2016a, b) showed that the young water fraction (Fyw; the fraction of streamflow that is younger than 2–3 months) can be estimated from the ratio of the seasonal amplitudes of sinusoidal fits to precipitation and streamflow isotope time series. Fits that are robust against outliers can be obtained using iteratively reweighted least squares (IRLS); an R script for this approach is available in the supplementary material of von Freyberg et al. (2018). We used results for the volume-weighted Fyw, because estimates of Fyw are more reliable when the sinusoidal isotope fits are weighted by the volumes of precipitation and streamflow and when they are derived from longer time series that yield more stable amplitude estimates (Kirchner, 2016a, b; von Freyberg et al., 2017, 2018).
We also calculated new water fractions (Fnew) using the ensemble hydrograph separation approach outlined in Kirchner (2019). A major advantage of Fnew over Fyw is that the timescale over which water is considered “young” (2–3 months) depends on the shape of the catchment transit time distribution, which will typically be unknown, whereas the timescale over which water is “new” is directly linked to the sampling frequency of the isotope time series. That is, because our isotope data were sampled at a monthly resolution, Fnew estimates the fraction of stream water that originated from precipitation in the 1-month period since the previous stream water sample was collected.
The ensemble hydrograph separation approach is based on correlations between isotopic fluctuations in streamflow and precipitation (and potentially also other end-members). It estimates the average contribution of precipitation to streamflow through correlations across an ensemble of precipitation and streamflow isotope samples. This makes it insensitive to unknown or unmeasured end-members and also avoids the spurious results that can arise in traditional hydrograph separation when the “old water” and “new water” isotopic signatures overlap. While traditional hydrograph separation assesses how fractions of new and old water change over successive time steps (e.g., during an individual storm event), ensemble hydrograph separation can quantify the average fractions of new and old water over ensembles of non-successive time steps reflecting different conditions (e.g., antecedent moisture). This is another major advantage of Fnew over Fyw, and a main goal of this study is to calculate new water fractions across all 32 Alpine catchments for the entire dataset and for subsets of the data reflecting different catchment conditions. Here, we report volume-weighted new water fractions of streamflow ( in the notation of Kirchner, 2019) calculated using the R script provided by Kirchner and Knapp (2020a, b). Using ensemble hydrograph separation, we could calculate the fraction of streamflow that is “new” since the last streamflow sampling, which is the fraction of streamflow younger than 1 month for our data.
To assess Fnew for the driest and wettest halves of the dataset, we split our data into two subsets based on monthly precipitation totals recorded prior to sampling and then calculated Fnew separately for the 50 % of sampling dates that received more precipitation and the 50 % of sampling dates that received less precipitation. To assess seasonal differences, we also split the data into the winter (November–April) and summer (May–October) halves of the year and then calculated Fnew separately for the winter and summer subsets. We also calculated the fraction of new water as a function of incoming precipitation (as described in Sect. 3.5 of Kirchner, 2019, and Sect. 5.4 of Knapp et al., 2019). We expect that higher values of Fnew will often be associated with higher precipitation totals in the month immediately preceding sampling.
2.6 Statistical measures for data analysis
Isotope data are presented in delta notation in per mil (‰) of 18O relative to V-SMOW (Vienna Standard Mean Ocean Water) throughout the paper (the respective 2H plots can be found in the Supplement). Overall, both δ18O and δ2H should yield similar results if nonequilibrium isotope fractionation processes due to evaporation can be neglected (Craig and Gordon, 1965). Some data are presented in box plots, in which the horizontal line indicates the median, the box represents the interquartile range, and the whiskers extend to 1.5 times the interquartile range from the first and third quartiles (or to the maximum and minimum values). The dots indicate outliers. Spearman rank correlations were calculated to obtain the correlation coefficient rS and its associated p value. Differences between groups of samples were tested using Wilcoxon signed-rank tests. Results are presented as statistically significant when p<0.05.
3.1 Hydroclimatic variables and catchment characteristics
Among the 32 study sites, average annual precipitation ranges from 611 to 1741 mm (mean of 1244 mm), winter precipitation ranges from 211 to 748 mm (mean of 534 mm), and summer precipitation ranges from 400 to 994 mm (mean of 710 mm). Mean annual PET ranges from 669 to 912 mm (mean of 825 mm), winter PET ranges from 193 to 269 mm (mean of 240 mm), and summer PET ranges from 496 to 657 mm (mean of 586 mm). The ratio of average discharge to average precipitation (qP−1) ranges from 0.10 to 0.78 (mean of 0.38); the q95 ranges from 0.09 to 1.54 mm d−1 (mean of 0.83 mm d−1).
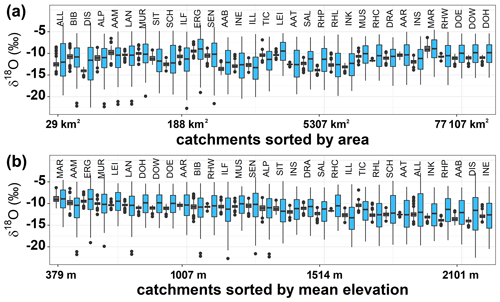
Figure 2Box plots of the δ18O isotopic composition of precipitation (light blue) and streamflow (dark gray) across all 32 Alpine catchments sorted by (a) catchment area (from small to large) and (b) mean catchment elevation (from low to high).
The catchments range in size from 29 to 103 946 km2 (mean of 13 923 km2 and median of 1562 km2). The mean catchment elevation varies from 379 to 2472 m a.s.l. (mean of 1310 m a.s.l.), and the elevation difference varies from 403 to 4454 m (mean of 2477 m). The mean catchment slope ranges from 4.6 to 50.9° (mean of 20.1°), the fraction of catchment area with a slope < 10° ranges from 9.1 % to 93.2 % (mean of 65 %), and the fraction of catchment area with a slope > 40° ranges from 0 % to 18.5 % (mean of 6.1 %). The fraction of catchment area covered by carbonate sedimentary rocks ranges from 0 % to 80.1 % (mean of 27.4 %), and the fraction of area covered by unconsolidated rocks ranges from 0 % to 56.1 % (mean of 17.5 %). The fraction of catchment area covered by forests ranges from 3.1 % to 60.3 % (mean of 32.9 %).
3.2 Isotopic variation in precipitation and streamflow across Alpine catchments
The seasonal variations in isotope ratios in precipitation and streamflow are expected to be related to the size of the catchment (i.e., more damped streamflow isotope ratios in larger catchments) and the mean catchment elevation (i.e., precipitation isotope ratios are lighter at higher elevations). The relationships of precipitation and streamflow δ18O signatures with catchment area and elevation are shown in Fig. 2. Overall, the amplitudes of the seasonal isotope signatures are damped from precipitation to streamflow. While there is only a weak trend between the isotope ratios and catchment area, precipitation and streamflow isotope ratios are lighter in higher-elevation catchments, as expected.
The Spearman rank correlation coefficients (rS) between catchment area and the median δ18O for precipitation and streamflow were 0.28 and 0.02, respectively (not significant). The range of variation (max–min) in δ18O was inversely correlated with catchment area: rS values of −0.71 (p≤0.05) and −0.45 (p≤0.05) for precipitation and streamflow, respectively. This suggests that the median of δ18O precipitation and streamflow isotopes only slightly increases with catchment size, whereas precipitation and streamflow isotopes are significantly less variable over time in larger catchments. Median δ18O decreased with increasing catchment elevation: rS values of −0.85 (p≤0.05) and −0.90 (p≤0.05) for precipitation and streamflow, respectively. Similar results were obtained for δ2H; see the Supplement (Fig. S1).
3.3 New (Fnew) and young (Fyw) water fractions across Alpine catchments
In Fig. 3, the fractions of new water (Fnew) and young water (Fyw) are shown. The catchments are sorted by mean catchment elevation from MAR (March at Angern, 379 m a.s.l.) to INE (Inn at S-chanf, 2472 m a.s.l.). Both Fnew and Fyw tended to be smaller at higher mean catchment elevations (rS of −0.37 and −0.32, respectively). However, the study catchments encompass different precipitation and discharge regimes: MAR to BIB are rainfall dominated (precipitation falls almost exclusively as rain), RHW to DRA are hybrid (both rain and snow can contribute significantly to winter precipitation), and SAL to INE are snow dominated (most winter precipitation falls as snow). Both Fnew and Fyw differed significantly between rainfall-dominated and snow-dominated catchments as well as between hybrid and snow-dominated catchments (p<0.05). Mean Fnew varied from 9.2 % in rainfall-dominated catchments and 9.6 % in hybrid catchments to 3.5 % in snow-dominated catchments, and mean Fyw varied from 17.6 % in rainfall-dominated catchments and 16.6 % in hybrid catchments to 10.1 % in snow-dominated catchments. Fnew and Fyw were strongly correlated (Pearson R of 0.88), with Fyw systematically exceeding Fnew (Fig. 3c). This is expected because Fyw expresses the fraction of water younger than 2–3 months, which should always be greater than the fraction of water younger than 1 month (i.e., Fnew estimated from monthly data).
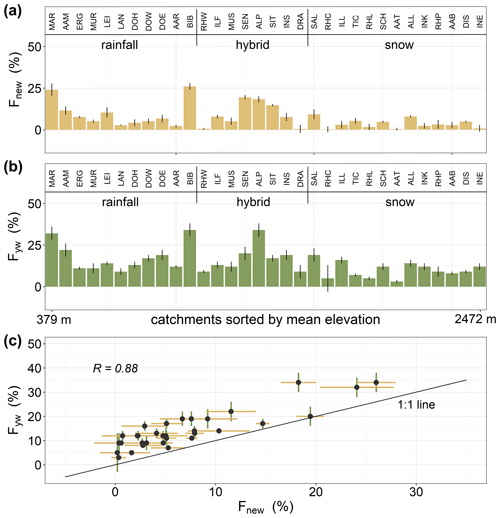
Figure 3The (a) new water fractions and (b) young water fractions for all catchments sorted by elevation. Fnew and Fyw are smaller in catchments with higher mean elevation. Fnew and Fyw are strongly correlated, with Fyw being systematically higher, as indicated by the points lying above the 1:1 line in panel (c).
3.4 New water fractions (Fnew) across Alpine catchments for different subsets of data
In the following section, the potential of the Fnew analysis is further explored, as Fnew can be calculated for different subsets of the data; for example, for different antecedent conditions or different seasons (Fig. 4). For most catchments, Fnew was larger in the wettest (highest-precipitation) 50 % of all months than in the driest half (mean Fnew of 9.3 % and 3.3 %, respectively; p<0.05). Fnew also tended to be larger in summer than in winter (mean Fnew of 12.7 % and 8.9 %, respectively; p<0.05). This is not surprising, as precipitation is typically higher in the summer than the winter across most of the Alps (mean across all catchments of our study was 710 mm in summer and 534 mm in winter; only RHP and ALL receive more precipitation in winter than in summer). Readers should note that we did not consider the delayed timing of snowmelt explicitly as a delayed precipitation input (as suggested in von Freyberg et al., 2018), rather only the timing of precipitation.
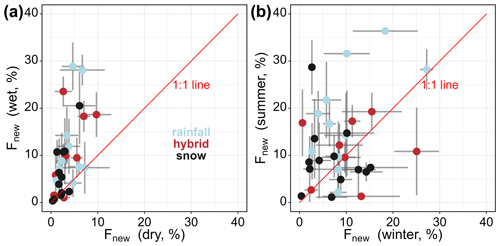
Figure 4New water fractions for (a) the driest and wettest half of the dataset and (b) the winter and summer half of the dataset. The colors indicate the different precipitation regimes (light blue for rainfall, red for hybrid, and black for snow dominated). New water fractions tend to be higher in wet periods with few exceptions. Summer new water fractions tend to be higher in most catchments.
We also calculated the fraction of new water for different ranges of monthly precipitation. It is expected that Fnew is larger for months with more incoming precipitation. As shown in Fig. 5, 18 of our 32 study catchments show increases in Fnew above a certain threshold in monthly precipitation rates (i.e., roughly 70 mm for MAR; roughly 110 mm for AAM; 175 mm for MUS, DOE, DOH, DOW, ERG, MUR, RHL, BIB, and LEI; roughly 200 mm for SEN, ALP, LAN, INK, SIT, and INS; and roughly 225 mm for SAL). For these catchments, it is evident that – after the threshold precipitation inputs are reached – the occurrence of more recent precipitation results in more recent precipitation in streamflow, as indicated by increasing Fnew. For 14 out of 32 catchments, more incoming precipitation does not raise Fnew above ∼10 % (Fig. S2), suggesting that catchment storage is large enough to damp tracer fluctuations, even with high monthly precipitation rates. These different responses to incoming precipitation are associated with differences in catchment elevation and slope. The 18 catchments in which Fnew increased substantially above a precipitation threshold (Fig. 5a) have a mean elevation of 1068 m a.s.l. and a mean slope of 16.1°, whereas the 14 catchments in which Fnew remained small (Fig. S2) are, on average, both higher (mean elevation of 1584 m a.s.l.) and steeper (mean slope of 24.3°); both differences are statistically significant (p<0.05).
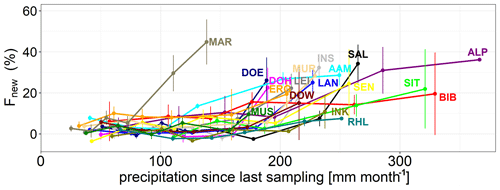
Figure 5The volume-weighted fraction of new water (Fnew) as a function of monthly precipitation totals during the month immediately preceding the sampling date. A total of 18 out of the 32 catchments exhibit a clear increase in Fnew at higher monthly precipitation totals. In these cases, Fnew increases at monthly precipitation rates exceeding ∼175 mm per month. For 14 out of 32 catchments, Fnew remains below 10 %, even at the highest monthly precipitation totals (shown in Fig. S2).
3.5 Downstream propagation of Fnew in the Danube and Rhine
New water fractions (Fnew) were mapped across seven subcatchments of the Danube River basin (Fig. 6a and c). Two headwater basins of the Inn have exceptionally small Fnew values (0.7 % at INE and 2.3 % at INK), potentially due to snowpack storage in these high-elevation catchments. It should also be noted that INE is sampled directly below several large lakes (Saint Moritz and Engadin, Switzerland); thus, the damped isotope signal probably reflects mixing and storage within those lakes. The remaining five Danube catchments exhibit a weak declining trend in Fnew with increasing catchment area, which nonetheless results in a perfect rank correlation () due to the small sample size (if all seven seven subcatchments are considered together, the rank correlation is nonsignificant).
The new water fractions (Fnew) were also mapped across the 18 subcatchments of the Rhine River basin (Fig. 6b and d). The smaller headwater catchments tend to have larger Fnew (i.e., Fnew exceeds 8 % for BIB, ALP, AAM, SIT, and SEN), suggesting that these headwater streams contain relatively large proportions of recent precipitation. These five headwater catchments are at low to intermediate mean elevations (522–1317 m a.s.l.). Across the Rhine Basin, smaller catchments tend to have higher Fnew (, p<0.05; Fig. 6d).
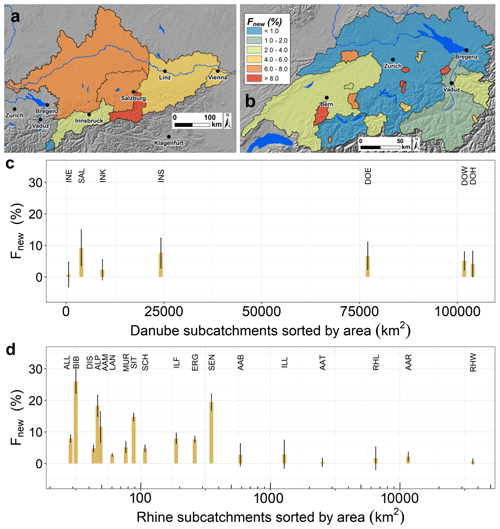
Figure 6New water fractions (Fnew) mapped across the subcatchments of the Danube (a) and Rhine (b) basins (n=7 and n=18, respectively) and Fnew as a function of catchment area for the Danube (c) and Rhine (d) subcatchments. The Inn headwater catchments in the southeast of the Danube Basin (INE and INK) have small Fnew values. For the other subcatchments in the Danube and Rhine basins, the expected decrease in Fnew with increasing catchment size is evident. Note that the x axis in panel (d) is logarithmic. The hillshades in the maps are based on the EU-DEM v1.1, available due to funding from the European Union.
3.6 Relationships between Fnew and hydroclimatic variables
Previous studies have indicated that the (volume-weighted) fractions of new water are related to hydroclimatic variables, e.g., catchments have larger Fnew following wet antecedent conditions (Knapp et al., 2019). Thus, we analyzed the relationships between Fnew and a range of climate variables (annual, summer, and winter precipitation sums and annual, summer and winter evapotranspiration sums) as well as the ratio of total discharge to total precipitation (qP−1) and the discharge that is reached or exceeded 95 % of the year (q95). As Fig. 7 shows, Fnew values were only weakly related to catchment annual, winter, and summer precipitation. Thus, mean annual or seasonal precipitation is a poor predictor of Fnew, despite the fact that Fnew does tend to be higher in wetter months (Fig. 4). Fnew was more strongly correlated with the amount of annual PET and summer PET (rS of 0.41 and 0.40, respectively). Fnew was also negatively correlated with the qP−1 ratio (, p<0.05) or positively correlated with the fraction ( of precipitation that was evaporated and transpired (rS=0.36, p<0.05). Thus, site-to-site variations in Fnew were positively correlated with both potential and actual evapotranspiration. Fnew was also inversely correlated with q95 ( at p<0.05), suggesting higher Fnew in catchments with less baseflow.
As new and young water fractions are strongly correlated with each other (rS=0.88, p<0.05), Fyw exhibited similar correlations with hydroclimatic variables to those that were found for Fnew (see Fig. S3).
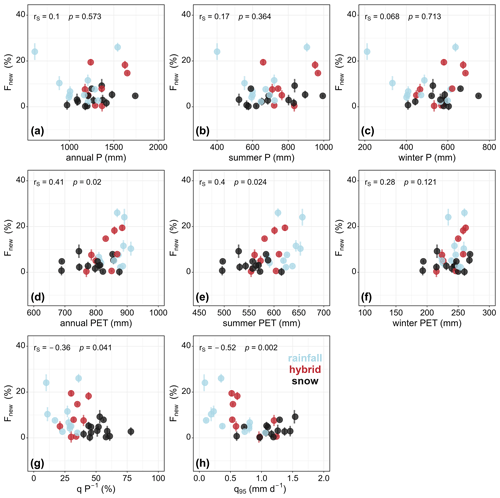
Figure 7Relationships between volume-weighted new water fractions (Fnew) and (a) annual precipitation, (b) summer (May–October) precipitation, (c) winter (November–April) precipitation, (d) annual potential evapotranspiration, (e) summer (May–October) potential evapotranspiration, (f) winter (November–April) potential evapotranspiration, (g) the fraction of annual discharge in relation to annual precipitation (qP−1), and (h) q95 (the discharge reached or exceeded 95 % of the year). The colors indicate the different precipitation regimes (light blue for rainfall, red for hybrid, and black for snow dominated). While Fnew is not strongly related to precipitation, Fnew is related to PET and the streamflow variables.
3.7 Relations of Fnew with physical catchment properties
Previous studies have indicated that the fractions of new (and young) water may be related to physical catchment properties. Therefore, across all 32 study catchments, we compared the isotopically inferred Fnew values with the catchment area, mean catchment elevation, elevation difference, mean catchment slope, and the fraction of slope below 10 and above 40°. To assess the effect of lithology, the fraction of (potentially karstified) carbonate sedimentary rocks and the fraction of unconsolidated debris deposits for all catchments were calculated. To assess the possible role of transpiration, the fraction of forest cover for each catchment was also calculated. Figure 8 shows a systematic assessment of the relation of Fnew and physical catchment properties. Fnew was inversely related to catchment area, mean elevation, and elevation difference (rS values of −0.38, −0.37, and −0.59, respectively, all at p<0.05). Thus, Fnew was smaller in larger catchments, higher catchments, and catchments with greater total relief. However, these three drivers are themselves strongly correlated with one another, so it is unclear which one could be considered to be the primary control on Fnew. Although the average catchment slope was not a good predictor of Fnew (, not significant), there was a much stronger correlation between Fnew and the fraction of slope larger than 40° (, p<0.05). This suggests that catchments with larger fractions of steep slopes have smaller Fnew; however, it should be noted that the fraction of slope larger than 40° was also correlated with mean catchment elevation (rS=0.84). The fractions of potentially karstified carbonate sedimentary rocks and unconsolidated debris deposits were not related to Fnew. Statistically significant correlations between the fraction of catchment area covered by forests and Fnew (rS=0.36 at p<0.05) suggest a possible role of forest vegetation in shaping flow paths and thus water ages.
Young water fractions exhibited similar relationships to catchment properties (see Fig. S4) compared to those observed for Fnew.
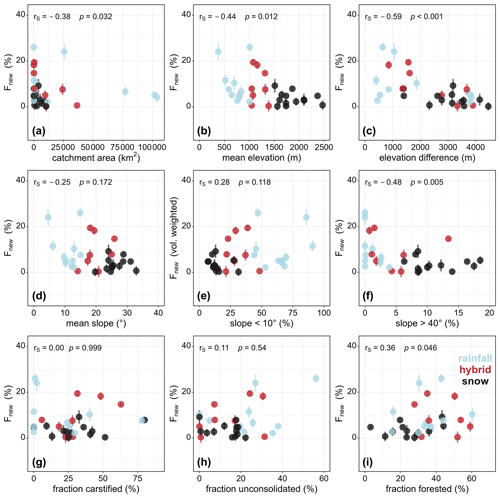
Figure 8Relation of volume-weighted new water fractions (Fnew) and (a) catchment area, (b) mean elevation, (c) elevation difference, (d) mean slope, (e) fraction of slope shallower than 10°, (f) fraction of slope steeper than 40°, (g) fraction of the catchment consisting of karstified rocks, (h) fraction of the catchment covered by unconsolidated rocks, and (i) fraction of the catchment covered by forests. The colors indicate the different precipitation regimes (light blue for rainfall, red for hybrid, and black for snow dominated). Fnew is strongly related to catchment area, elevation difference, fraction of slope steeper than 40°, and fraction of the catchment covered by forest.
4.1 Limitations with respect to data availability and study design
One of the main limitations of our study (and many larger-scale isotope studies in general) is the limited availability of isotope data on streamflow and precipitation. For the presented study, we harvested all streamflow data that were available for longer time periods across the European Alps; however, most of these data only exist at a monthly (i.e., WISA and ISOT) or 14 d (CH-IRP) resolution. While these streamflow data are sufficient to provide reasonable information on average behaviors across longer timescales (and their linkage to potential physical drivers of streamflow), it is important to remind readers that we can not infer any short-term behavior or response to single precipitation events. For this reason, further research based on isotope data with shorter sampling intervals would enable investigations of short-term catchment drainage processes (i.e., as shown in Knapp et al., 2019, or Floriancic et al., 2024).
Even more critical is the use of monthly aggregates of precipitation isotopes. Precipitation isotopologues can be very heterogeneous, even within single precipitation events (e.g., Pinos et al., 2022; Allen et al., 2017). Overall, the number of stations with available precipitation isotope data is limited, and single-station data collections are often not a reliable source for large-basin studies that span large elevation gradients. For this reason, we calculated the monthly volume-weighed precipitation basin averages from the Piso.AI database (Nelson et al., 2021), which, to our understanding, is the most reliable data source for our intended application. We are well aware that other isotope interpolation methods exist (i.e., Seeger and Weiler, 2014; Allen et al., 2018), but comparisons of the precipitation isotope data from Piso.AI and these methods yield similar results (see Figs. S5 and S6).
4.2 New (Fnew) and young (Fyw) water fractions across Alpine catchments
One of the main objectives of the study was to compare the physical drivers of new and young water fractions across a set of catchments with different sizes. Across our 32 study catchments, Fnew and Fyw decreased with increasing catchment elevation (Figs. 3, 8b, and S4). This is consistent with the previous findings of Ceperley et al. (2020), who observed a decrease in young water fractions above an elevation of 1500 m a.s.l. across Swiss Alpine catchments. Conversely, von Freyberg et al. (2018) found weak positive correlations with catchment elevation for a subset of our study catchments (12 of our catchments overlap with their set of 22 catchments). The relative importance of rain versus snow had a clear effect on Fnew and Fyw, which were higher in hybrid catchments and rainfall-dominated catchments but lower in snow-dominated catchments (Fig. 3). Conversely, Gentile et al. (2023) found the highest Fyw in hybrid catchments but similarly low Fyw in rainfall- and snow-dominated catchments. The reason for this discrepancy might be that our dataset also covers much larger catchments (catchment areas in our dataset ranged from 29 to 103 946 km2 versus 0.14 to 351 km2 in Gentile et al., 2023).
Fnew was larger in wet periods compared with dry periods (with means of 9.3 % and 3.3 %, respectively), and it was larger in the summer half of the year compared with the winter half of the year (means of 12.7 % and 8.9 %, respectively). Similar analyses were performed on the Plynlimon dataset by Knapp et al. (2019), who found that wetter antecedent conditions led to higher Fnew. At Plynlimon, Fnew was smaller in summer (which is the drier season there), whereas Fnew was smaller in winter (when precipitation rates are lower and more precipitation falls as snow) in our 32 Alpine catchments. Knapp et al. (2019) observed strong increases in 7 h and weekly Fnew above a precipitation threshold of roughly 5 mm d−1, whereas we found that 18 of our 32 catchments showed strong increases in monthly Fnew above a comparable precipitation threshold of roughly 175 mm per month. Readers should note that the Plynlimon subcatchments studied by Knapp et al. (2019) are substantially smaller (<3.6 km2) than ours (>29 km2) and that the temporal resolution of their data was much higher (7 h to weekly) than ours (monthly).
Overall, Fnew tended to decrease downstream, from smaller headwaters to larger river basins (Sect. 3.5), which is consistent with the larger mean residence times that are typically estimated in larger catchments (DeWalle et al., 1995; Soulsby et al., 2000). Another important factor when moving from the headwaters downstream is the impact of water storage in lakes and reservoirs as well as the potential effects of anthropogenic flow regulation. While the CH-IRP headwater catchments (Staudinger et al., 2020) were carefully selected to avoid major impoundments or diversions, such complications are unavoidable in the larger basins contained in our dataset. Within the Danube Basin, for example, INE has several large lakes just upstream of the sampling location; moreover, within the Rhine Basin, AAT (immediately below Lake Thun) and RHW (approximately 100 km downstream of Lake Constance) are significantly impacted by lakes. Big lakes can substantially dampen Fnew by storing months or years of flow. Such storage yields exceptionally low Fnew values (e.g., Fnew is 0.7 %, 0.4 %, and 0.6 % at INE, AAT, and RHW, respectively). Fnew can also be altered by dams and their accompanying reservoirs; for example, multiple large dams lie upstream of DOE, DOW, and DOH, which have respective Fnew values of 6.7 %, 5.1 %, and 4.1 % (for comparison, the mean of all rainfall-dominated catchments is 9.6 %).
4.3 Conceptualization of physical drivers of Fnew and Fyw across the Alps
When looking at the effects of hydroclimatic variables and physical catchment properties on Fnew, single-variable correlations with Fnew should be interpreted with caution, as they may be confounded by cross-correlations between many potential drivers (Fig. 9). For example, annual and summer PET, discharge fraction, q95, mean slope, fraction of catchment area with a slope > 40°, and fraction of catchment area with a slope < 10° are all strongly related to mean catchment elevation. Thus, it remains unclear which of these variables may be a first-order control on new water fractions.
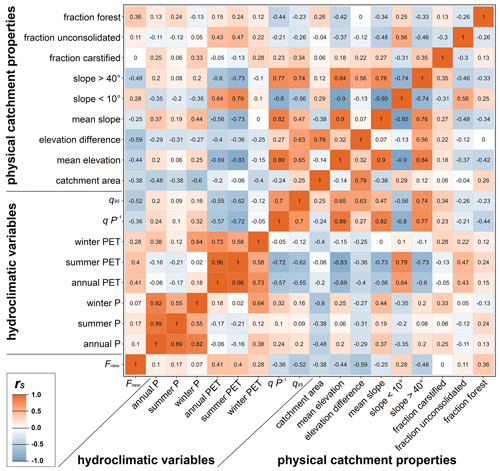
Figure 9Spearman rank correlations between all of the selected hydroclimatic variables and physical catchment characteristics across the 32 study catchments. Red colors indicate strong to intermediate positive correlations; blue colors indicate strong to intermediate inverse correlations.
Overall, we found that high fractions of new water (Fnew) were more likely in small catchments, at low elevations, with small total relief and larger forest cover, and following months with high precipitation, whereas low Fnew values were more likely in large catchments, at high elevations, with large total relief, and following months with low precipitation (Fig. 10).
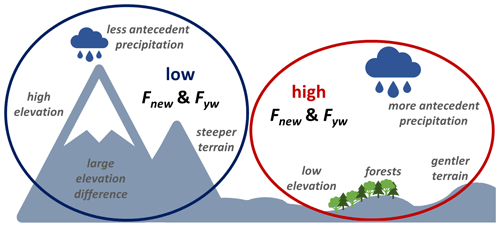
Figure 10Conceptual scheme of the significant relations of hydroclimatic variables and physical catchment properties with volume-weighted new water fractions (Fnew) across the 32 Alpine catchments.
In their work, von Freyberg et al. (2018) found significant correlations between Fyw and monthly precipitation. Conversely, site-to-site differences in average Fnew and Fyw were only weakly correlated with precipitation (annual, summer, and winter) across our 32 sites. Nevertheless, higher precipitation in the month preceding sampling typically led to larger Fnew, which is in line with previous findings in Knapp et al. (2019). Higher Fnew values were typically found during the summer period, which receives more precipitation than the winter period (see Sect. 3.3).
Across the Alps, elevation is correlated with Fnew as well as with many other variables (e.g., temperature, precipitation, PET, and slope) that arguably should have stronger mechanistic connections with new water fractions. Site-to-site differences in Fnew were positively correlated with both annual PET and summer PET as well as with long-term actual evapotranspiration, estimated as (see Sect. 3.6). This is somewhat counterintuitive, as one might expect that if more precipitation leaves the catchment via evapotranspiration, the smaller resulting discharge flux would imply longer retention times in the catchment and, thus, smaller new water fractions. However, PET in our set of catchments is negatively correlated with elevation (Fig. 9); thus, it is likely that high Fnew values in catchments with high PET are confounded by elevation effects rather than being solely explained by PET.
Across our 32 sites, Fnew exhibited a strong inverse correlation with the low-flow variable q95, suggesting higher Fnew in catchments with smaller low flows. Similarly, Gentile et al. (2023) found correlations between baseflow and Fyw. These results and ours are also consistent with the findings of von Freyberg et al. (2018), who showed that the quick-flow index (QFI) is positively correlated with Fyw. Although QFI and q95 are not the same, they are systematically related, as high q95 is more likely in catchments with low QFI. However, q95 across the European Alps is also positively related to elevation (Fig. 9; but see also Floriancic et al., 2022); thus large Fnew in catchments with high q95 might also be affected by elevation rather than q95 being the sole explanation for high Fnew values.
We observed modest (but statistically significant) negative correlations between Fnew and catchment area, and von Freyberg et al. (2018) also found weak (but nonsignificant) correlations between catchment area and Fyw. However, these relationships might be confounded by differences in the dominant precipitation regime; the correlation between Fnew and catchment area is stronger among our hybrid catchments (, p<0.05) than among our rainfall-dominated or snow-dominated catchments (rS of −0.27 and −0.39, respectively). Thus, Fnew (and Fyw) values appear to be inversely related to catchment area, although not so much in catchments with seasonal snow cover or glaciers (Gentile et al., 2023; Ceperley et al., 2020), which generally have small Fnew and Fyw values independent of their catchment area.
We found only a weak and nonsignificant inverse correlation between Fnew and mean catchment slope (); however, we noted strong inverse correlations between Fnew and both total relief (elevation difference; ) and the fraction of catchment area with slopes steeper than 40° (). These results, while not conclusive, are broadly consistent with the observation of a significant negative correlation between Fyw and average catchment slope by Jasechko et al. (2016) in their survey of 254 global rivers. The aforementioned study argued that this decrease in young water in steep terrain may be related to subsurface storage and transport. Deep vertical infiltration is prevalent in steeper landscapes; this is also consistent with conceptual models of groundwater flow (Gleeson and Manning, 2008) showing that larger topographic gradients lead to longer subsurface flow paths. Geotechnical stresses in steep terrain also promote fracture opening and, thus, increase hydraulic bedrock permeability (Jasechko et al., 2016; Gleeson et al., 2011a), thereby facilitating deep percolation. On the other hand, catchments with slopes steeper than 40° are mainly found at higher elevations and, thus, tend to be snow dominated. Here, smaller fractions of Fnew and Fyw are expected due to snow storage.
High-elevation catchments tend to have smaller Fyw and Fnew (e.g., Gentile et al., 2023; Ceperley et al., 2020; and Fig. 8 in our study), and their streamflow isotopic composition may be affected by snow and glacier melt (Schmieder et al., 2016, 2018). The smaller Fyw and Fnew observed at high elevations in the European Alps may result from snowpack and glacier storage leading to delayed runoff of winter precipitation. However, these high-elevation catchments also have larger elevation gradients and, thus, potentially greater subsurface storage, leading to longer transit times (Jasechko et al., 2016; Schmieder et al., 2018). A weak indication of the importance of snow processes can be found in Fig. 4, where we show that Fnew values in the summer half of the year are generally larger in most of the catchments. However, with our sample of catchments (as in several previous studies), we cannot test these hypotheses independently, as catchments with large topographic gradients are also dominated by snow across the European Alps. Nevertheless, this important question should be considered in further research based on a more targeted dataset that allows for the testing of both hypotheses independently.
We found no significant correlations between Fnew and either the fraction of area with potentially karstified carbonate sedimentary rocks or the fraction of area with unconsolidated debris deposits. This result is somewhat surprising, as one would expect that karstified rocks would increase Fnew by providing quick flow paths that allow bedrock storages to drain quickly (Hartmann et al., 2021). Furthermore, multiple previous studies have highlighted the importance of unconsolidated debris deposits in streamflow generation processes (e.g., Hood and Hayashi, 2015; Hayashi, 2019; Cochand et al., 2019; Floriancic et al., 2018; Gentile et al., 2023). Our analysis is based on the global GLiM database, which uses lithological classes that may be too coarse or may be mapped at an insufficient resolution to be hydrologically informative at such small scales. Previous studies have also reported that weathered bedrock or regolith is important for storing water and delaying runoff response (Grant and Dietrich, 2017; McCormick et al., 2021) by enhancing storage capacity, especially in steeper catchments where precipitation would otherwise drain to river networks directly. Further analyses must await more comprehensive data on subsurface geology and near-surface geomorphological features (Gentile et al., 2023; Floriancic et al., 2022).
We also found a weak (but marginally significant) positive correlation between Fnew and the fraction of catchment area covered by forests. The work of von Freyberg et al. (2018) found similar correlations with Fyw across Swiss headwater catchments, but Hrachowitz et al. (2021) found the opposite relationship for a forest removal experiment. It is somewhat surprising that the prevalence of forests is linked to higher values of Fnew. A possible explanation might be tree roots facilitating preferential pathways through macropores (Brantley et al., 2017; von Freyberg et al., 2018). The formation of preferential pathways can be argued to lead to either more or less recent precipitation ending up in streamflow. Preferential pathways may transport precipitation directly to streams, thus increasing Fnew and Fyw (Brantley et al., 2017; von Freyberg et al., 2018). However, previous studies have also argued that preferential pathways tend to increase deep percolation and mixing in deeper storages, thereby decreasing Fnew and Fyw (Hrachowitz et al., 2021; Weiler et al., 2006). Overall, it remains to be tested whether the positive correlations between Fnew and the fraction of catchment area covered by forests are an artifact of cross-correlations with other variables, as the fraction of catchment area covered by forests is also correlated with mean elevation and the fraction of catchment area with slopes steeper than 40° (R of −0.42 and −0.33, respectively). Thus, it is likely that high Fnew in catchments with more forest cover might be affected by cross-correlations rather than forest cover being the dominant explanation for variations in Fnew.
The Alps are an important water source for Europe, and assessing the partitioning of new (or young) versus old waters in Alpine rivers may provide important insights for sustainable management. For this study, isotope time series from 32 catchments across the Austrian and Swiss Alps were evaluated using two recently developed methods: (1) estimation of new water fractions (Fnew – in this study, the fraction of water younger than about 1 month) using ensemble hydrograph separation and (2) estimation of young water fractions (Fyw – the fraction of water younger than 2–3 months) using seasonal isotope cycles. Mean Fnew and Fyw decreased with mean catchment elevation and varied across precipitation regimes (Fig. 3). Overall, Fnew was higher in the summer months and higher following months with higher precipitation (Fig. 4). However, strong increases in Fnew were only observed in 8 of our 32 catchments and only above a precipitation threshold of roughly 175 mm per month. For most catchments, Fnew remained below 10 %, even when precipitation inputs were large (Fig. 5). Fnew decreased from the headwater streams to the large downstream basins of the Danube and Rhine, potentially as a result of natural dampening by larger source areas and lakes as well as due to anthropogenic influences from dams and reservoirs (Fig. 6). High Fnew values are more likely in small catchments, at low elevations, with small elevation gradients, with larger fractions of forest cover, and when precipitation is high (Figs. 4 and 8), whereas low Fnew values are more likely in large catchments, at high elevations, with large total relief, high baseflow (Figs. 7 and 8), and low antecedent precipitation (Fig. 4). The obtained results reveal which Alpine areas transmit recent precipitation more rapidly to runoff. However, the interpretation of the obtained results needs to be done with caution, as cross-correlations exist (Fig. 9) and the extent to which the reported correlations indicate direct controls on water storage processes is not always clear. The analysis also highlights the importance of further research on the effect of snow processes on the partitioning of new (or young) and old waters as well as the need for higher-resolution lithological information.
Daily discharge time series for 12 of the 20 Swiss sites were obtained from the CH-IRP database (Staudinger et al., 2020). Discharge time series for the remaining 8 Swiss sites and for all 12 Austrian sites were obtained from the Federal Office of the Environment “Hydrological data and forecasts” database (FOEN, 2022a) and the “Hydrographisches Jahrbuch” contained in the WISA database (Umweltbundesamt, 2022a), respectively. Daily catchment-averaged precipitation was obtained from the gridded precipitation dataset E-OBS (version 20.0e) at a 0.1° resolution (Cornes et al., 2018). PET was calculated from the Global Aridity Index and Potential Evapotranspiration Climate Database v2 (https://doi.org/10.6084/m9.figshare.7504448.v3, Trabucco and Zomer, 2019). Monthly gridded precipitation isotopes were obtained from the reanalysis database Piso.AI (Nelson et al., 2021), accessible at https://isotope.bot.unibas.ch/PisoAI/ (UNIBAS, 2024). Monthly streamflow isotopes for the 12 Austrian sites were obtained from the WISA “H2O Fachdatenbank” database (Umweltbundesamt, 2022b); for the 8 Swiss stations, this information was sourced from the NAQUA ISOT (Nationalen Grundwasserbeobachtung – Isotopendaten) database (FOEN, 2022b). Streamflow isotopes for 12 additional stations across the Swiss Alps were obtained from the CH-IRP database (Staudinger et al., 2020).
The supplement related to this article is available online at: https://doi.org/10.5194/hess-28-3675-2024-supplement.
MF conceptualized the study, collected the data from the aforementioned sources, ran the formal analysis, visualized the results, and prepared the original draft of the manuscript. All co-authors provided feedback during the analysis and reviewed and edited the manuscript.
The contact author has declared that none of the authors has any competing interests.
Publisher's note: Copernicus Publications remains neutral with regard to jurisdictional claims made in the text, published maps, institutional affiliations, or any other geographical representation in this paper. While Copernicus Publications makes every effort to include appropriate place names, the final responsibility lies with the authors.
The authors would like to acknowledge the institutions and individuals that collected the long-term isotope data that made this study possible: WISA (Wasserinformationssystem Austria), ISOT – FOEN (Swiss Federal Office of the Environment), and the team at the University of Zurich and University of Freiburg (Maria Staudinger and colleagues).
This paper was edited by Yue-Ping Xu and reviewed by two anonymous referees.
Allen, S. T., Keim, R. F., Barnard, H. R., McDonnell, J. J., and Renée Brooks, J.: The role of stable isotopes in understanding rainfall interception processes: a review, WIREs Water, 4, e1187, https://doi.org/10.1002/wat2.1187, 2017.
Allen, S. T., Kirchner, J. W., and Goldsmith, G. R.: Predicting Spatial Patterns in Precipitation Isotope δ2H and δ18O Seasonality Using Sinusoidal Isoscapes, Geophys. Res. Lett., 45, 4859–4868, https://doi.org/10.1029/2018GL077458, 2018.
Berghuijs, W. R. and Kirchner, J. W.: The relationship between contrasting ages of groundwater and streamflow: Connecting Storage and Streamflow Ages, Geophys. Res. Lett., 44, 8925–8935, https://doi.org/10.1002/2017GL074962, 2017.
Brantley, S. L., Eissenstat, D. M., Marshall, J. A., Godsey, S. E., Balogh-Brunstad, Z., Karwan, D. L., Papuga, S. A., Roering, J., Dawson, T. E., Evaristo, J., Chadwick, O., McDonnell, J. J., and Weathers, K. C.: Reviews and syntheses: on the roles trees play in building and plumbing the critical zone, Biogeosciences, 14, 5115–5142, https://doi.org/10.5194/bg-14-5115-2017, 2017.
Briffa, K. R., van der Schrier, G., and Jones, P. D.: Wet and dry summers in Europe since 1750: evidence of increasing drought, Int. J. Climatol., 29, 1894–1905, https://doi.org/10.1002/joc.1836, 2009.
Browne, T. J.: Derivation of a geological index for low flow studies, Catena, 8, 265–280, https://doi.org/10.1016/0341-8162(81)90010-2, 1981.
Ceperley, N., Zuecco, G., Beria, H., Carturan, L., Michelon, A., Penna, D., Larsen, J., and Schaefli, B.: Seasonal snow cover decreases young water fractions in high Alpine catchments, Hydrol. Process., 34, 4794–4813, https://doi.org/10.1002/hyp.13937, 2020.
Cochand, M., Christe, P., Ornstein, P., and Hunkeler, D.: Groundwater Storage in High Alpine Catchments and its Contribution to Streamflow, Water Resour. Res., 55, 2613–2630, 2019.
Cornes, R. C., van der Schrier, G., van den Besselaar, E. J. M., and Jones, P. D.: An Ensemble Version of the E-OBS Temperature and Precipitation Data Sets, J. Geophys. Res.-Atmos., 123, 9391–9409, https://doi.org/10.1029/2017JD028200, 2018.
Craig, H. and Gordon, L. I.: Deuterium and Oxygen 18 Variations in the Ocean and the Marine Atmosphere, Spoleto Meeting on Nuclear Geology, Stable Isotopes in Oceanography Studies and Paleo Temperature, https://api.semanticscholar.org/CorpusID:126843232 (last access: 15 August 2024), 1965.
DeWalle, D. R., Edwards, P. J., Swistock, B. R., Drimmie, R. J., and Aravena, R.: Seasonal isotope hydrology of Appalachian forest catchments, in: Proceedings, 10th Central Hardwood Forest Conference, 5–8 March 1995, Morgantown, WV, Gen. Tech. Rep. NE-197, US Department of Agriculture, Forest Service, Northeastern Forest Experiment Station. Radnor, PA, edited by: Gottschalk, K. W. and Fosbroke, S. L. C., p. 296, https://research.fs.usda.gov/treesearch/12769 (last access: 15 August 2024), 1995.
Fleckenstein, J. H., Niswonger, R. G., and Fogg, G. E.: River-Aquifer Interactions, Geologic Heterogeneity, and Low-Flow Management, Ground Water, 44, 837–852, https://doi.org/10.1111/j.1745-6584.2006.00190.x, 2006.
Floriancic, M. G., Meerveld, I., Smoorenburg, M., Margreth, M., Naef, F., Kirchner, J. W., and Molnar, P.: Spatio-temporal variability in contributions to low flows in the high Alpine Poschiavino catchment, Hydrol. Process., 32, 3938–3953, https://doi.org/10.1002/hyp.13302, 2018.
Floriancic, M. G., Spies, D., van Meerveld, I. H. J., and Molnar, P.: A multi-scale study of the dominant catchment characteristics impacting low-flow metrics, Hydrol. Process., 36, e14462, https://doi.org/10.1002/hyp.14462, 2022.
Floriancic, M. G., Allen, S. T., and Kirchner, J. W.: Young and new water fractions in soil and hillslope waters, EGUsphere [preprint], https://doi.org/10.5194/egusphere-2024-437, 2024.
FOEN: Hydrological data and forecasts, FOEN [data set], https://www.hydrodaten.admin.ch/en/stations-and-data.html (last access: 5 December 2022), 2022a.
FOEN: NAQUA ISOT, FOEN [data set], https://www.bafu.admin.ch/bafu/en/home/topics/water/info-specialists/state-of-waterbodies/state-of-groundwater/naqua-national-groundwater-monitoring/isot-module.html (last access: 5 December 2022), 2022b.
Gentile, A., Canone, D., Ceperley, N., Gisolo, D., Previati, M., Zuecco, G., Schaefli, B., and Ferraris, S.: Towards a conceptualization of the hydrological processes behind changes of young water fraction with elevation: a focus on mountainous alpine catchments, Hydrol. Earth Syst. Sci., 27, 2301–2323, https://doi.org/10.5194/hess-27-2301-2023, 2023.
Gleeson, T. and Manning, A. H.: Regional groundwater flow in mountainous terrain: Three-dimensional simulations of topographic and hydrogeologic controls: Regional groundwater flow, Water Resour. Res., 44, W10403, https://doi.org/10.1029/2008WR006848, 2008.
Gleeson, T., Marklund, L., Smith, L., and Manning, A. H.: Classifying the water table at regional to continental scales: Water Table At Continental Scales, Geophys. Res. Lett., 38, L05401, https://doi.org/10.1029/2010GL046427, 2011a.
Gleeson, T., Smith, L., Moosdorf, N., Hartmann, J., Dürr, H. H., Manning, A. H., van Beek, L. P. H., and Jellinek, A. M.: Mapping permeability over the surface of the Earth: Mapping Global Permeability, Geophys. Res. Lett., 38, L02401, https://doi.org/10.1029/2010GL045565, 2011b.
Gleeson, T., Befus, K. M., Jasechko, S., Luijendijk, E., and Cardenas, M. B.: The global volume and distribution of modern groundwater, Nat. Geosci., 9, 161–167, https://doi.org/10.1038/ngeo2590, 2016.
Grant, G. E. and Dietrich, W. E.: The frontier beneath our feet, Water Resour. Res., 53, 2605–2609, https://doi.org/10.1002/2017WR020835, 2017.
Hartmann, A., Jasechko, S., Gleeson, T., Wada, Y., Andreo, B., Barberá, J. A., Brielmann, H., Bouchaou, L., Charlier, J.-B., Darling, W. G., Filippini, M., Garvelmann, J., Goldscheider, N., Kralik, M., Kunstmann, H., Ladouche, B., Lange, J., Lucianetti, G., Martín, J. F., Mudarra, M., Sánchez, D., Stumpp, C., Zagana, E., and Wagener, T.: Risk of groundwater contamination widely underestimated because of fast flow into aquifers, P. Natl. Acad. Sci. USA, 118, e2024492118, https://doi.org/10.1073/pnas.2024492118, 2021.
Hartmann, J. and Moosdorf, N.: The new global lithological map database GLiM: A representation of rock properties at the Earth surface, Geochem. Geophy. Geosy., 13, Q12004, https://doi.org/10.1029/2012GC004370, 2012.
Hayashi, M.: Alpine Hydrogeology: The Critical Role of Groundwater in Sourcing the Headwaters of the World, Groundwater, 58, 498–510, https://doi.org/10.1111/gwat.12965, 2019.
Hood, J. L. and Hayashi, M.: Characterization of snowmelt flux and groundwater storage in an alpine headwater basin, J. Hydrol., 521, 482–497, https://doi.org/10.1016/j.jhydrol.2014.12.041, 2015.
Hrachowitz, M., Soulsby, C., Tetzlaff, D., Dawson, J. J. C., and Malcolm, I. A.: Regionalization of transit time estimates in montane catchments by integrating landscape controls, Water Resour. Res., 45, W05421, https://doi.org/10.1029/2008WR007496, 2009.
Hrachowitz, M., Stockinger, M., Coenders-Gerrits, M., van der Ent, R., Bogena, H., Lücke, A., and Stumpp, C.: Reduction of vegetation-accessible water storage capacity after deforestation affects catchment travel time distributions and increases young water fractions in a headwater catchment, Hydrol. Earth Syst. Sci., 25, 4887–4915, https://doi.org/10.5194/hess-25-4887-2021, 2021.
IAEA: Global Network of Isotopes in Precipitation (GNIP), IAEA [data set], https://www.iaea.org/services/networks/gnip (last access: 25 October 2022), 2022a.
IAEA: Global Network of Isotopes in Rivers (GNIR), IAEA [data set], http://www-naweb.iaea.org/napc/ih/IHS_resources_gnir.html (last access: 25 October 2022), 2022b.
Jasechko, S., Kirchner, J. W., Welker, J. M., and McDonnell, J. J.: Substantial proportion of global streamflow less than three months old, Nat. Geosci., 9, 126–130, 2016.
Jasechko, S., Perrone, D., Befus, K. M., Bayani Cardenas, M., Ferguson, G., Gleeson, T., Luijendijk, E., McDonnell, J. J., Taylor, R. G., Wada, Y., and Kirchner, J. W.: Global aquifers dominated by fossil groundwaters but wells vulnerable to modern contamination, Nat. Geosci., 10, 425–429, https://doi.org/10.1038/ngeo2943, 2017.
Kirchner, J. W.: A double paradox in catchment hydrology and geochemistry, Hydrol. Process., 17, 871–874, https://doi.org/10.1002/hyp.5108, 2003.
Kirchner, J. W.: Aggregation in environmental systems – Part 1: Seasonal tracer cycles quantify young water fractions, but not mean transit times, in spatially heterogeneous catchments, Hydrol. Earth Syst. Sci., 20, 279–297, https://doi.org/10.5194/hess-20-279-2016, 2016a.
Kirchner, J. W.: Aggregation in environmental systems – Part 2: Catchment mean transit times and young water fractions under hydrologic nonstationarity, Hydrol. Earth Syst. Sci., 20, 299–328, https://doi.org/10.5194/hess-20-299-2016, 2016b.
Kirchner, J. W.: Quantifying new water fractions and transit time distributions using ensemble hydrograph separation: theory and benchmark tests, Hydrol. Earth Syst. Sci., 23, 303–349, https://doi.org/10.5194/hess-23-303-2019, 2019.
Kirchner, J. W. and Knapp, J. L. A.: Ensemble hydrograph separation scripts – EnviDat, EnviDat [code], https://doi.org/10.16904/envidat.182, 2020a.
Kirchner, J. W. and Knapp, J. L. A.: Technical note: Calculation scripts for ensemble hydrograph separation, Hydrol. Earth Syst. Sci., 24, 5539–5558, https://doi.org/10.5194/hess-24-5539-2020, 2020b.
Kirchner, J. W., Benettin, P., and Meerveld, I. van: Instructive Surprises in the Hydrological Functioning of Landscapes, Annu. Rev. Earth Planet. Sci., 51, 277–299, https://doi.org/10.1146/annurev-earth-071822-100356, 2023.
Klaus, J. and McDonnell, J. J.: Hydrograph separation using stable isotopes: Review and evaluation, J. Hydrol., 505, 47–64, https://doi.org/10.1016/j.jhydrol.2013.09.006, 2013.
Knapp, J. L. A., Neal, C., Schlumpf, A., Neal, M., and Kirchner, J. W.: New water fractions and transit time distributions at Plynlimon, Wales, estimated from stable water isotopes in precipitation and streamflow, Hydrol. Earth Syst. Sci., 23, 4367–4388, https://doi.org/10.5194/hess-23-4367-2019, 2019.
Mastrotheodoros, T., Pappas, C., Molnar, P., Burlando, P., Manoli, G., Parajka, J., Rigon, R., Szeles, B., Bottazzi, M., Hadjidoukas, P., and Fatichi, S.: More green and less blue water in the Alps during warmer summers, Nat. Clim. Change, 10, 155–161, https://doi.org/10.1038/s41558-019-0676-5, 2020.
McCormick, E. L., Dralle, D. N., Hahm, W. J., Tune, A. K., Schmidt, L. M., Chadwick, K. D., and Rempe, D. M.: Widespread woody plant use of water stored in bedrock, Nature, 597, 225–229, https://doi.org/10.1038/s41586-021-03761-3, 2021.
McDonnell, J. J. and Beven, K.: Debates – The future of hydrological sciences: A (common) path forward? A call to action aimed at understanding velocities, celerities and residence time distributions of the headwater hydrograph, Water Resour. Res., 50, 5342–5350, https://doi.org/10.1002/2013WR015141, 2014.
McDonnell, J. J., McGuire, K., Aggarwal, P., Beven, K. J., Biondi, D., Destouni, G., Dunn, S., James, A., Kirchner, J., Kraft, P., Lyon, S., Maloszewski, P., Newman, B., Pfister, L., Rinaldo, A., Rodhe, A., Sayama, T., Seibert, J., Solomon, K., Soulsby, C., Stewart, M., Tetzlaff, D., Tobin, C., Troch, P., Weiler, M., Western, A., Wörman, A., and Wrede, S.: How old is streamwater? Open questions in catchment transit time conceptualization, modelling and analysis, Hydrol. Process., 24, 1745–1754, https://doi.org/10.1002/hyp.7796, 2010.
Neal, C. and Rosier, P. T. W.: Chemical studies of chloride and stable oxygen isotopes in two conifer afforested and moorland sites in the British uplands, J. Hydrol., 115, 269–283, https://doi.org/10.1016/0022-1694(90)90209-G, 1990.
Nelson, D. B., Basler, D., and Kahmen, A.: Precipitation isotope time series predictions from machine learning applied in Europe, P. Natl. Acad. Sci. USA, 118, e2024107118, https://doi.org/10.1073/pnas.2024107118, 2021.
Pinos, J., Llorens, P., and Latron, J.: High-resolution temporal dynamics of intra-storm isotopic composition of stemflow and throughfall in a Mediterranean Scots pine forest, Hydrol. Process., 36, e14641, https://doi.org/10.1002/hyp.14641, 2022.
Schmieder, J., Hanzer, F., Marke, T., Garvelmann, J., Warscher, M., Kunstmann, H., and Strasser, U.: The importance of snowmelt spatiotemporal variability for isotope-based hydrograph separation in a high-elevation catchment, Hydrol. Earth Syst. Sci., 20, 5015–5033, https://doi.org/10.5194/hess-20-5015-2016, 2016.
Schmieder, J., Garvelmann, J., Marke, T., and Strasser, U.: Spatio-temporal tracer variability in the glacier melt end-member – How does it affect hydrograph separation results?, Hydrol. Process., 32, 1828–1843, https://doi.org/10.1002/hyp.11628, 2018.
Schürch, M., Kozel, R., Schotterer, U., and Tripet, J.-P.: Observation of isotopes in the water cycle – the Swiss National Network (NISOT), Environ. Geol., 45, 1–11, https://doi.org/10.1007/s00254-003-0843-9, 2003.
Seeger, S. and Weiler, M.: Reevaluation of transit time distributions, mean transit times and their relation to catchment topography, Hydrol. Earth Syst. Sci., 18, 4751–4771, https://doi.org/10.5194/hess-18-4751-2014, 2014.
Segura, C., James, A. L., Lazzati, D., and Roulet, N. T.: Scaling relationships for event water contributions and transit times in small-forested catchments in Eastern Quebec, Water Resour. Res., 48, W07502, https://doi.org/10.1029/2012WR011890, 2012.
Sklash, M. G. and Farvolden, R. N.: The role of groundwater in storm runoff, J. Hydrol., 43, 45–65, https://doi.org/10.1016/0022-1694(79)90164-1, 1979.
Soulsby, C., Malcolm, R., Helliwell, R., Ferrier, R. C., and Jenkins, A.: Isotope hydrology of the Allt a' Mharcaidh catchment, Cairngorms, Scotland: implications for hydrological pathways and residence times, Hydrol. Process., 14, 747–762, https://doi.org/10.1002/(SICI)1099-1085(200003)14:4<747::AID-HYP970>3.0.CO;2-0, 2000.
Staudinger, M., Seeger, S., Herbstritt, B., Stoelzle, M., Seibert, J., Stahl, K., and Weiler, M.: The CH-IRP data set: a decade of fortnightly data on δ2H and δ18O in streamflow and precipitation in Switzerland, Earth Syst. Sci. Data, 12, 3057–3066, https://doi.org/10.5194/essd-12-3057-2020, 2020.
Stockinger, M. P., Bogena, H. R., Lücke, A., Stumpp, C., and Vereecken, H.: Time variability and uncertainty in the fraction of young water in a small headwater catchment, Hydrol. Earth Syst. Sci., 23, 4333–4347, https://doi.org/10.5194/hess-23-4333-2019, 2019.
Trabucco, A. and Zomer, R.: Global Aridity Index and Potential Evapotranspiration (ET0) Climate Database v2, figshare [data set], https://doi.org/10.6084/m9.figshare.7504448.v3, 2019.
Umweltbundesamt: Hydrographisches Jahrbuch – WISA, Umweltbundesamt [data set], https://wasser.umweltbundesamt.at/hydjb/ (last access: 5 December 2022), 2022a.
Umweltbundesamt: WISA H2O Fachdatenbank, Umweltbundesamt [data set], https://wasser.umweltbundesamt.at/h2odb/ (last access: 5 December 2022), 2022b.
UNIBAS: Piso.AI, https://isotope.bot.unibas.ch/PisoAI/ (last access: 15 August 2024), 2024.
von Freyberg, J., Studer, B., and Kirchner, J. W.: A lab in the field: high-frequency analysis of water quality and stable isotopes in stream water and precipitation, Hydrol. Earth Syst. Sci., 21, 1721–1739, https://doi.org/10.5194/hess-21-1721-2017, 2017.
von Freyberg, J., Allen, S. T., Seeger, S., Weiler, M., and Kirchner, J. W.: Sensitivity of young water fractions to hydro-climatic forcing and landscape properties across 22 Swiss catchments, Hydrol. Earth Syst. Sci., 22, 3841–3861, https://doi.org/10.5194/hess-22-3841-2018, 2018.
Weiler, M., McDonnell, J. J., Tromp-van Meerveld, I., and Uchida, T.: Subsurface Stormflow, in: Encyclopedia of Hydrological Sciences, John Wiley & Sons, Ltd, https://doi.org/10.1002/0470848944.hsa119, 2006.
Weingartner, R., Viviroli, D., and Schädler, B.: Water resources in mountain regions: a methodological approach to assess the water balance in a highland-lowland-system, Hydrol. Process., 21, 578–585, https://doi.org/10.1002/hyp.6268, 2007.