the Creative Commons Attribution 4.0 License.
the Creative Commons Attribution 4.0 License.
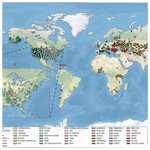
The International Soil Moisture Network: serving Earth system science for over a decade
Irene Himmelbauer
Daniel Aberer
Lukas Schremmer
Ivana Petrakovic
Luca Zappa
Wolfgang Preimesberger
Angelika Xaver
Frank Annor
Jonas Ardö
Dennis Baldocchi
Marco Bitelli
Günter Blöschl
Heye Bogena
Luca Brocca
Jean-Christophe Calvet
J. Julio Camarero
Giorgio Capello
Minha Choi
Michael C. Cosh
Nick van de Giesen
Istvan Hajdu
Jaakko Ikonen
Karsten H. Jensen
Kasturi Devi Kanniah
Ileen de Kat
Gottfried Kirchengast
Pankaj Kumar Rai
Jenni Kyrouac
Kristine Larson
Suxia Liu
Alexander Loew
Mahta Moghaddam
José Martínez Fernández
Cristian Mattar Bader
Renato Morbidelli
Jan P. Musial
Elise Osenga
Michael A. Palecki
Thierry Pellarin
George P. Petropoulos
Isabella Pfeil
Jarrett Powers
Alan Robock
Christoph Rüdiger
Udo Rummel
Michael Strobel
Zhongbo Su
Ryan Sullivan
Torbern Tagesson
Andrej Varlagin
Mariette Vreugdenhil
Jeffrey Walker
Jun Wen
Fred Wenger
Jean Pierre Wigneron
Mel Woods
Kun Yang
Yijian Zeng
Xiang Zhang
Marek Zreda
Stephan Dietrich
Alexander Gruber
Peter van Oevelen
Wolfgang Wagner
Klaus Scipal
Matthias Drusch
Roberto Sabia
In 2009, the International Soil Moisture Network (ISMN) was initiated as a community effort, funded by the European Space Agency, to serve as a centralised data hosting facility for globally available in situ soil moisture measurements (Dorigo et al., 2011b, a). The ISMN brings together in situ soil moisture measurements collected and freely shared by a multitude of organisations, harmonises them in terms of units and sampling rates, applies advanced quality control, and stores them in a database. Users can freely retrieve the data from this database through an online web portal (https://ismn.earth/en/, last access: 28 October 2021). Meanwhile, the ISMN has evolved into the primary in situ soil moisture reference database worldwide, as evidenced by more than 3000 active users and over 1000 scientific publications referencing the data sets provided by the network. As of July 2021, the ISMN now contains the data of 71 networks and 2842 stations located all over the globe, with a time period spanning from 1952 to the present. The number of networks and stations covered by the ISMN is still growing, and approximately 70 % of the data sets contained in the database continue to be updated on a regular or irregular basis. The main scope of this paper is to inform readers about the evolution of the ISMN over the past decade, including a description of network and data set updates and quality control procedures. A comprehensive review of the existing literature making use of ISMN data is also provided in order to identify current limitations in functionality and data usage and to shape priorities for the next decade of operations of this unique community-based data repository.
- Article
(4181 KB) - Full-text XML
- BibTeX
- EndNote
Ground-based soil moisture measurements of land surface variables are indispensable in the process of developing, validating, and advancing spatially contiguous data sets derived from satellites or models (Loew et al., 2017; Gruber et al., 2020). Although the first systematic measurements of soil moisture started well before the satellite era in the former Soviet Union to support agricultural decision-making (Robock et al., 2000), it was not until the early 2000s that soil moisture monitoring networks started being widely established as part of hydrological and meteorological observing capacities. Particularly, the launch of the Soil Moisture Ocean Salinity (SMOS) mission of the European Space Agency (ESA) in 2009 (Kerr et al., 2016), and the launch of the Soil Moisture Active Passive (SMAP) mission of the National Aeronautics and Space Administration (NASA) in 2015 (Entekhabi et al., 2010), boosted the establishment of new research networks (Colliander et al., 2017).
While all networks are valuable assets for assessing the skill of soil moisture products under various conditions and scales, their usage is hampered by the diversity of sensors, data formats, quality control, and accessibility mechanisms. The need to bring together and to harmonise available soil moisture data was recognised by the international soil moisture community and expedited by the Global Energy and Water cycle Exchanges (GEWEX) project of the World Climate Research Programme (WCRP) with support of the Committee on Earth Observation Satellites (CEOS), the Global Climate Observing System (GCOS), and the Group on Earth Observations (GEO). In the advent of the SMOS mission, ESA decided to provide the financial impetus to establish a global reference database of in situ soil moisture measurements for the purpose of satellite product development and validation. As a result, the International Soil Moisture Network (ISMN) went online in 2010 (Dorigo et al., 2011b, a).
The primary objective of the ISMN is to collect in situ soil moisture data sets, shared by various data organisations on a voluntary basis, and make them available in a harmonised format through a centralised free and open web portal (https://ismn.earth/en/, last access: 28 October 2021). While 10 years after its launch the core objective of the ISMN remains valid, its functionality has expanded since then. This new functionality includes the integration of advanced quality control methods (Dorigo et al., 2013; Sect. 3), the provision of additional metadata and ancillary variables (e.g. precipitation and soil and air temperature), ongoing automation, the provision of software code to users, and the implementation of various tools to promote the information exchange between users, the ISMN, and the data providers. Moreover, the ISMN has substantially grown in terms of networks, stations, and data sets.
Data from the ISMN has supported hundreds of scientific papers on soil moisture, satellite product, and land surface model validation in particular (e.g. Al-Yaari et al., 2019b; Brocca et al., 2014a; Beck et al., 2021). Several operational data producing services routinely access the ISMN data for repeated quality assurance, including the ESA's Climate Change Initiative (Dorigo et al., 2017), the Copernicus Global Land Service (Bauer-Marschallinger et al., 2018), and the Copernicus Climate Change Service (C3S; Dorigo et al., 2017). Other domains have also exploited the ISMN data, e.g. in meteorology, drought monitoring, or land–atmosphere coupling (Sect. 4).
Despite the valuable contribution of the ISMN to satellite and climate communities, multiple challenges have yet to be mastered, including the heterogeneous availability in space and time (Dorigo et al., 2015), scale differences between in situ measurements and satellite or model sampling (Gruber et al., 2013), full characterisation and traceability of uncertainties, and differences in spatiotemporal support of the observations caused by different measurement techniques and landscape heterogeneity (Ochsner et al., 2013). New scientific avenues to improve the spatial coverage could be the inclusion of soil moisture data sets from low-cost sensors collected by citizens (Sect. 5.1.4. For climate applications, stable long-term reference data are required, calling for the coordinated establishment and maintenance of Fiducial Reference Measurement (FRM) stations, as outlined by the GEO/CEOS Quality Assurance framework for Earth Observation (QA4EO) (GCOS, 2016; Montzka et al., 2020; Gruber et al., 2020).
The scope of this paper is to inform readers about the evolution of the ISMN over the past decade, including a description of network and data set updates, new quality control procedures, and new functionality of the data portal. We also review scientific literature making use of ISMN data to assess the achievements facilitated by the ISMN and to identify current limitations in data availability and functionality and challenges in data provision and use. Based on this review, prerequisites and priorities needed to ensure another decade of this unique community-based data repository are defined.
Although the ISMN may be considered a mere data repository, there is much more to it. Its core functionality includes collecting data from participating data providing networks, harmonising them in terms of units, sampling rates, naming, and metadata, performing automated quality control, storing the data and metadata in a searchable database, and making them available through a web interface. And, from a system perspective, it entails even more, e.g. communication with (potential) data providers and users (Fig. B1).
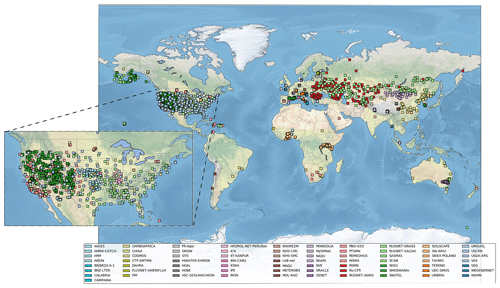
Figure 1Locations of ISMN networks and sites plotted with the ISMN package described in the code and data availability section (status in July 2021).
2.1 Data and metadata summary
As of July 2021, the ISMN contains 71 networks providing access to a total of 2842 stations, approximately 10 000 soil moisture data sets, and an additional 10 000 data sets of other meteorological variables (collocated with the soil moisture measurements). Although the ISMN is a global network, most networks and stations are located in North America (15), Europe (28), Australia (3), and Asia (19; Fig. 1). New networks are continuously being added, while many existing networks are still being upgraded with additional stations, soil moisture sensors, and meteorological variables. The diversity of networks is large, ranging from networks with a single station to networks comprising more than 400 stations covering different landscape types as well as periods. The distribution of stations per Köppen–Geiger climate class is given in Fig. 2.
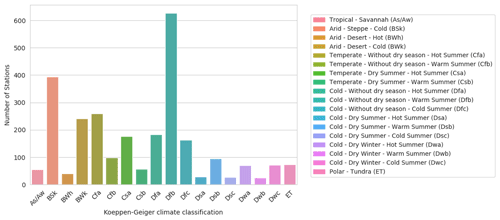
Figure 2Distribution of ISMN sites per Köppen–Geiger climate classification. Only categories with >20 sites are considered (status in June 2021).
Most of the networks originate from scientific initiatives in various disciplines (e.g. remote sensing, soil sciences, agriculture, and meteorology), and only a few are run by operational entities like national weather or environmental services. Consequently, a lack of sustainable project funding has forced several scientific networks to close after some time. As a result, 18 out of the 71 networks contained in the ISMN have become inactive and will no longer provide data set updates (Fig. 3). Data go back as far as 1952, but none of the data sets spans the entire ∼70 year period. The longest available time series (∼40 years) is provided by the RUSWET-AGRO network in the former Soviet Union, while the longest operating network still active is SNOTEL in the USA.
Most networks provide data set updates at yearly or irregular intervals. Data sets from six networks (ARM, COSMOS, FMI, SCAN, SNOTEL, U.S. Climate Reference Network (USCRN), and WegenerNet), comprising approximately 900 stations, are updated in near-real time (NRT; status in July 2021), which is currently defined as once per day. While the earliest networks were sampled manually at weekly, fortnightly, or even monthly intervals, most current networks take their measurements using electronic devices at daily, hourly, or even more frequent sampling rates. For more details on the networks, see Sect. A, Dorigo et al. (2011b, 2013), or the individual references herein.
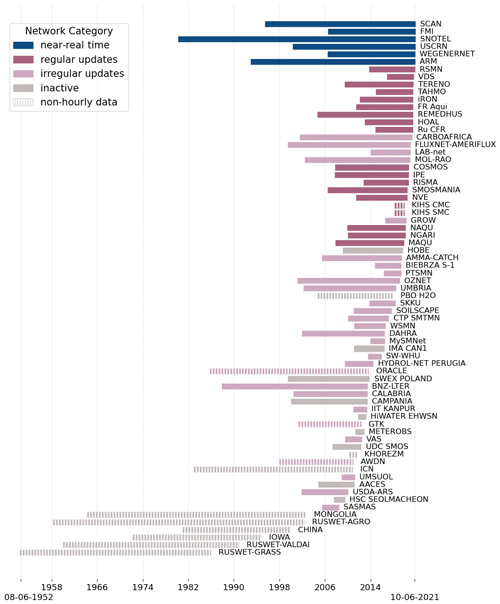
Figure 3Overview of all available networks, the individual time span of the data availability within the ISMN, their operational status, and their updating frequency (status in June 2021).
Table 1Overview of all available temporally dynamic variables stored in the ISMN database. * Note that, for precipitation and air temperature, the measurement height above the ground surface is indicated.
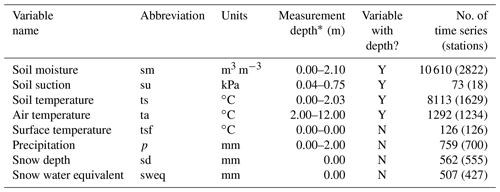
The variables contained in the ISMN (Tables 1 and 2) originate from networks that were built for various purposes, which consequently do not all contribute the same information. Since the ISMN was initially founded as a validation database for satellite (surface) soil moisture data, each station in the database provides soil moisture for the upper soil layer (≤0.10 m depth). Soil moisture data sets in the ISMN can go as deep as 2 m, but generally with a decreasing number of measurements locations with depth (Table C1). Some stations deploy more than one sensor at a certain depth, either as replacement in case of failure of one of the sensors or to characterise local soil moisture variability. The availability of meteorological data, like precipitation and soil and air temperature, is even more heterogeneous, depending on the scope of the network or the data sharing policy of the data providing organisation. It is also quite common that single time series in the database are composed of the consecutive measurements of two or more different sensors when a sensor is replaced after failure.
Metadata information can be divided into two categories, i.e. mandatory metadata, which allow for an unambiguous identification of each network, station, and measurement in the ISMN database (Fig. D1), and optional metadata, shared by data providers to allow more in-depth analysis of their data sets. To be consistent between sites, the mandatory variables of climate, land cover, and soil characteristics are taken from external databases. Climate classification is taken from the Köppen–Geiger database, with a resolution of 0.1∘ (Peel et al., 2007). Dynamically evolving land cover for the years 2000, 2005, and 2010 is obtained from ESA's Climate Change Initiative (CCI) land cover v1.6.1 with 300 m resolution. Soil information is retrieved from the Harmonized World Soil Database (HWSD v1.1; FAO/IIASA/ISRIC/ISS-CAS/JRC, 2009) with a 30′′ (1 km) sampling, although the actual resolution may strongly vary from location to location.
If available, data providers can optionally share their own, more detailed, characterisations of land cover, soil, and quality flags with the ISMN. These are stored in addition to the same variables from external sources. All static variables per measurement site and depth are listed in Table 2.
2.2 Data collection and harmonisation
Data collection is done either manually (mostly by email) or is automated, depending on the degree of automation at the data provider side. Although standards for in situ soil moisture data collection are available (Colliander et al., 2017; Montzka et al., 2020), there is no general agreement within the community, and it is not prescribed for participation in the ISMN. Thus, the data being contributed to the ISMN are heterogeneous with regard to units (e.g. volumetric soil moisture, water depth, mass, soil saturation, and plant-available water), installation depth, integration length, and positioning of the sensors (vertical and horizontal), the metrical system, the sampling interval, and the time zones used for the measurement time stamps.
The first harmonisation step for all data and metadata involves the conversion of units to internationally agreed scientific units (e.g. metres and degrees Celsius). Then, following the recommendations of the World Meteorological Organization for weather observation and forecasting (Williams, 2010), all data are resampled to hourly Coordinated Universal Time (UTC) reference time steps. Data that are available at higher sampling rates are thinned by selecting the individual measurements at the hourly UTC reference time step or the ones that are closest in time within a window of ±0.5 h. If there is no measurement available within this interval, the respective time step is not stored in the database but can be recreated and filled with a no-data value upon download. The temporal resampling scheme is valid for all included dynamic variables, except precipitation. Since precipitation is a flux and not a state variable, all measurements within the hourly interval are summed to represent the full amount of precipitation within the preceding hour (Dorigo et al., 2011b).
All soil moisture measurements provided to the ISMN are converted to volumetric soil moisture in cubic metres by cubic metre (hereafter m3 m−3). Since the majority of networks already shares their measurements in volumetric soil moisture units, often no unit conversion is needed. For the other (mostly historical) networks, measurements are converted to volumetric units using metadata on soil properties (in case measurements are provided as saturation level or plant-available water) and/or the thickness of the soil layer represented by the measurement (in case measurements are provided as water height or mass; Dorigo et al., 2011b). Note that, even if all measurements are harmonised in terms of units, differences in sampling volumes related to the sensor design and installation are not accounted for.
The harmonisation of measurement depths is particularly challenging, as different networks adopt different measurement depths, similar sensors are positioned differently (horizontally vs. vertically), or their measurements represent different observation volumes, which may even differ according to the soil wetness (as for cosmic-ray probes). Thus, harmonising soil moisture measurements to one or several reference depths would require either assumptions on the measurements and soil properties or supplemental soil modelling. Additionally, since there are lots of potential uses for the data, there is no common agreement on the optimum sampling depths. For example, satellite calibration–validation generally requires observations of the 0–5 cm layer, while land surface model evaluation requires reference measurements that are representative for the layers defined in the model (Dorigo et al., 2011b). Consequently, the ISMN does not harmonise measurement depths.
After data harmonisation, the data sets are submitted to extensive quality control procedures (Sect. 3. After quality control, all data sets of soil moisture and other variables, metadata information on networks, responsible organisations, sites, sensors, and static soil attributes for each station are stored in a relational database.
2.3 Data portal
The ISMN can be accessed at https://ismn.earth/ (last access: 28 October 2021) and consists of a project website containing, e.g., information about networks, data, quality control, and partners and a data interface where users can view, query, and download the data contained in the database.
The data interface displays the location and information of networks and single stations and allows plotting of the available data to gain an impression of data availability and quality. Data can be selected for time period, area, single networks, or entire continents. Alternatively, the data download can be selected via an advanced SQL query, which allows users to make more specific selections (e.g. for a sensor brand or a certain depth range).
The selected data are directly extracted from the database, and downloads are organised per network. For each network, the download contains (i) the measurements and their quality flags, (ii) information about the file data organisation, (iii) a description of the ISMN quality flags, (iv) a metadata file compliant with ISO 19115 and INSPIRE (Infrastructure for Spatial Information in the European Community) metadata standards, and (v) information about static site characteristics (e.g. land cover, climate class, and soil characteristics).
The extracted data set files are formatted according to either the CEOP (Coordinated Energy and Water Cycle Observations Project; Williams, 2010) standard or a slightly modified version of the CEOP format which improves data usability (Dorigo et al., 2011b). These standards use the ascii format, but a NetCDF format is foreseen for the near future.
2.4 Data provider and user involvement
The ISMN is entirely built on the voluntary, free-of-charge contributions from scientific and operational providers. This prevents the ISMN from being too prescriptive towards the data providers in terms of delivery intervals, automation, and formatting. Hence, a careful balance is needed between inclusiveness, on the one hand, and data quality standards, on the other. The ISMN facilitates between users and data providers by reporting data quality issues and user feedback to the providers every 6 months. This is done by means of a report on data usage statistics for each individual network, e.g. on the number of downloads, the usage of their data in scientific publications, and flagging statistics. Together with obtaining visibility and citations, obtaining feedback on data usage and data quality is one of the primary motivations for data providers to join the ISMN.
More than 3000 active users have registered since 2009 (status in July 2021). Data download is free of charge but user registration (compliant with the latest European Union General Data Protection Regulation, EU GDPR, privacy standards) is required to prevent illegal redistribution of the data or theft of ground equipment and to track (undisclosed) statistics on data usage that are fed back to the data providers, e.g. by regular reports.
News feeds on the ISMN web page and a biannual newsletter inform the users about new networks, new data sets, data quality issues, important publications, workshops, and more. In addition, a dedicated forum and classical email exchange allow users to raise and receive response to issues. Moreover, open-source software packages are available for reading and plotting the data (see the data availability section).
3.1 Quality flagging methodology
The wide variety of sensor types and installations, measurement protocols, calibration methods, preprocessing, and quality control procedures adopted by the data providers result in data sets with large differences in quality and filtering. In an attempt to harmonise the reliability of the data from different networks and sensors and to allow for the marking of spurious observations in near-real time, the ISMN has adopted automated quality procedures which are applied to all observations feeding into the ISMN (Dorigo et al., 2013). It uses several approaches to detect dubious soil moisture measurements, which can be subdivided into geophysical dynamic range verification, geophysical consistency methods, and spectrum-based methods (Table 3). While the first category of methods applies simple threshold checks directly to the measurements, the geophysical consistency methods make use of ancillary data, which are either observed in situ at the same site or derived from model data (i.e. Global Land Data Assimilation System (GLDAS)-Noah). The spectrum-based flags are the result of a series of conditions applied to the soil moisture measurement time series and their first and second derivatives. The geophysical consistency and spectrum-based methods are only applied to the soil moisture observations, while geophysical dynamic range verification is applied to all dynamic variables in the database.
Recently, the following refinements of the original flagging procedures, as described and assessed in Dorigo et al. (2013), were implemented to increase the flagging accuracy:
-
The outlier detection method (flag D06) now allows spikes to last 2 consecutive time steps instead of the initial 1 h. This occurs when all conditions of Eqs. (4)–(6) in Dorigo et al. (2013) are met, but the peak value remains unchanged for an additional hour. The overall impact is small (flagging numbers increase from 0.31 % to 0.34 %), but its impact on extreme values can be significant.
-
Flag D07 (negative breaks or drops) was extended with an extra possibility, which detects drops from values greater than 0.05 to exactly 0 m3 m−3 soil moisture as follows:
Since a spurious soil moisture drop is a precondition for a low plateau (D09; constant low values following a negative break), the latter is also affected. Adding the extra drop detection increased flagging numbers for D07 from 0.03 % to 0.05 % and for D09 from 1.1 % to 1.4 %.
-
In case more than one soil temperature, air temperature, or precipitation sensor is available at a site, a flag is raised for the soil moisture measurement if the conditions of flags D01, D02, and D04, respectively, are met at least for one of these sensors. This has led to a small overall increase in flags D01, D02, and D04 (<0.8 %).
All quality control procedures adopted by the ISMN have been made available under the open-source license agreement (see the data availability section; https://github.com/TUW-GEO/flagit, last access: 28 October 2021).
We assessed the refined flagging procedures by applying them to 10 networks with hourly data that include stations with multiple soil temperature, air temperature, or precipitation sensors. Despite the very low overall impact of the refined flags, for some networks they are substantial (Fig. 4).
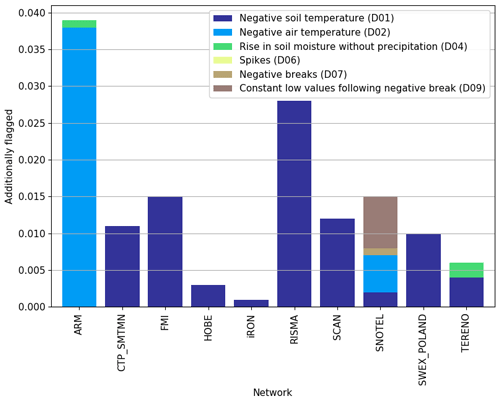
Figure 4Additionally flagged observations by the network shown as fraction of total number of observations. Note that, in principle, the cumulative sum of fractions can be >1 as the occurrence of each flag ranges between 0 and 1.
Measurements that are detected as erroneous by the quality control procedures are not deleted from the database but tagged as such (Table 3). The flag is provided as additional attribute (ISMN quality flag) to the observation upon download and can take one of the main categories, namely C (exceeding plausible geophysical range), D (questionable/dubious), M (missing), or G (good). The D flag is raised when either a geophysical consistency or a spectrum-based check is positive. An additional number indicates the actual cause for flagging (Table 3). A soil moisture observation may receive multiple C- and D-type flags but the good and missing flags are exclusive. Seven networks provide their own soil moisture quality flags, which are added to the ISMN database in addition to the ISMN flags common to all time series. Examples are flags for data quality (without further methodological description) or simple thresholds. For instance, Real-time In-Situ Soil Monitoring for Agriculture (RISMA) tags soil moisture observations for frozen soils when the average temperature of two adjacent soil layers is below 0 ∘C (Pacheco et al., 2014). By contrast, the ISMN flag D01 (soil temperature < 0 ∘C) only considers the corresponding depth. For RISMA, the frozen soil flags provided by the network and those computed by the ISMN (D01; soil temperature < 0 ∘C) agree for 87.8 %.
3.2 Flagging occurrence
The most commonly raised flag is when one of the ancillary temperature observations, i.e. in situ soil temperature (D01), in situ air temperature (D02), or GLDAS soil temperature (D03), is <0 ∘C (Table 3; Fig. 5). Since in situ temperature measurements are not available for all networks, and to keep consistency between networks, flags D01 and D02 are not shown in Fig. 5. The number of observations flagged as frozen soil are not an indicator of the site in general but show which networks are located in areas with a pronounced cold season, e.g. stations from the FMI, RISMA, MAQU, SCAN, and SNOTEL networks.
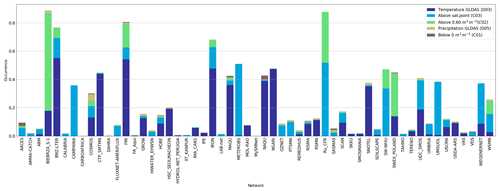
Figure 5Fractions of geophysical dynamic range and consistency quality flags per network. Note that, in principle, the cumulative sum of fractions can be >1 as the occurrence of each flag ranges between 0 and 1.
The second most common flag is C03 (soil moisture above the site-specific saturation point), which is computed from the HWSD soil properties. The site-specific saturation point is usually lower than the globally adopted, less conservative threshold of 0.6 m3 m−3 (flag C02) and, thus, raised more often (Fig. 5). However, the HWSD soil properties are uncertain, and consequently, the C03 flag should be considered as being indicative rather than as an absolute quality indicator. Values exceeding the saturation point are often an indication of calibration biases or atypical site conditions. For example, the large number of C03 flags obtained for the BIEBRZA-S-1 network is because it is installed in a temporarily flooded marshlands with peat porosity exceeding 80 %.
Constant values as a result of saturation plateaus (D10) or after a negative break (D09) are the most common spectrum-based flags (Fig. 6). The latter are often a sign of sensor drop outs and mostly limited to the GROW, ARM, HYDROL-Net Perugia, and the U.S. Department of Agriculture (USDA) Agricultural Research Service (ARS) networks. For example, Xaver et al. (2020) evaluated the sensors used in the GROW network and found occasional drops in soil moisture to zero, which may be the result of corroding contacts. Spikes and breaks are, by nature, isolated events that do not occur over an extended period of time and, thus, appear less frequent in the flagging statistics. The relatively large number of spikes for the network SNOTEL of 0.4 % is due to some extremely noisy time series.
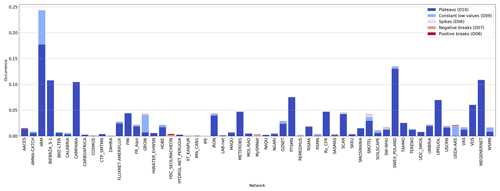
Figure 6Fractions of spectrum-based quality flags per network. Notice that, in principle, the cumulative sum of fractions can be >1 as the occurrence of each flag ranges between 0 and 1.
Table 4Global validation results of surface soil moisture of ERA and ESA CCI soil moisture against ISMN (masked and unmasked for quality flags) for the period 2001–2019. The results for ESA CCI were produced with the QA4SM validation service (https://qa4sm.eu/, last access: 28 October 2021). The results are accessible at https://doi.org/10.5281/zenodo.4288919 (Aberer, 2020a) (absolute values – no flags), https://doi.org/10.5281/zenodo.4288921 (Aberer, 2020b) (absolute values – with flags), https://doi.org/10.5281/zenodo.4288915 (Aberer, 2020c) (anomalies – no flags), and https://doi.org/10.5281/zenodo.4288913 (Aberer, 2020d) (anomalies – with flags). Note: ubRMSD is the unbiased root mean squared difference.
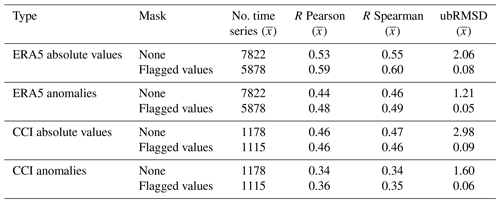
3.3 Effect of flagging on applications
For a selected ISMN site (SCAN and Mayday station), Dorigo et al. (2013) showed that masking flagged values has a small but positive impact on the validation of GLDAS-Noah v1 modelled surface soil moisture and the remotely sensed VUA-NASA Advanced Microwave Scanning Radiometer for Earth Observing System (AMSR-E) soil moisture product. Here, we performed a more extensive assessment of the impact of excluding automatically detected spurious observations by the revised flagging methods (Sect. 3.1) by using ISMN observations available in the period 2001–2019 to validate both ERA5 top layer (0–0.07 m) water content (Hersbach et al., 2020) and ESA CCI Soil Moisture (v5.2; Gruber et al., 2019b, 2017; Dorigo et al., 2017). While the impact of flagging is positive for temporal agreement (R Pearson and R Spearman) between the ISMN and ERA5, the effect is negligible for ESA CCI (Table 4). On the other hand, the ISMN flagging reduces the unbiased root mean squared difference (ubRMSD) for both comparisons. The benefit of excluding spurious values is also obvious in Fig. F1a, where points are located below the 1:1 line. Again, the benefit is less clear for ESA CCI (Fig. F1c). Although validations of a satellite and a model-based are not directly comparable, one reason for the different impact is that the ESA CCI retrievals were already flagged in the production process for values outside valid geophysical range, inconsistencies, dense vegetation, freezing, and snow cover, while this is not the case for the ERA5 model data. Consequently, a positive effect of the ISMN flags is more effective for data sets that were not a priori masked than for data sets that were already filtered for spurious observations.
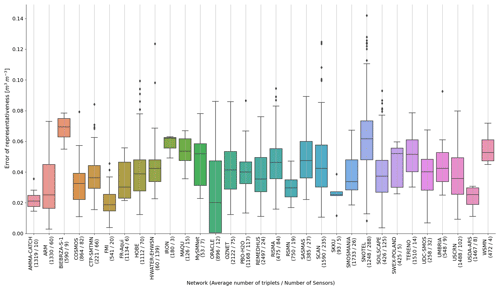
Figure 7Representativeness errors of ISMN networks calculated with triple collocation analysis using top-layer ERA5 volumetric soil water and the ESA CCI SM v05.2 passive product. Values in parentheses show the average number of triplets per time series and the total number of sensors, respectively, for each network. Following Dorigo et al. (2010), we only used triplets with a Spearman correlation > 0.2 between each respective data set pair in the calculation.
3.4 Other quality indicators
The automated quality control algorithms offer insight into the quality of the respective measurement time series but not necessarily of the usability of the data sets for specific applications. Gruber et al. (2013) adopted a triple collocation approach (TCA) to characterise the representativeness errors of ISMN data for coarse-scale (∼25 km) use. TCA is a statistical analysis using a combination of three data sets with independent error structures to estimate the random error variance in each of these data sets. Here, we apply the TCA to estimate the representativeness errors of the ISMN data of all networks with sufficient sampling in the period 2001–2019 for application at the coarse scale. ESA CCI SM passive soil moisture (v5.2; Gruber et al., 2019b; Dorigo et al., 2017) and top-layer ERA5 volumetric soil water content (Hersbach et al., 2020) are used to complement the triplets. Spatial collocation is carried out using a nearest-neighbour method, with a maximum distance of 30 km, while the temporal collocation uses a maximum time difference of 1:20 h between the triplets. An exception was solely made for the PBO_H2O network because its observations are provided daily at 12:00 UTC, while ESA CCI SM is given at 00:00 UTC daily. All measurements that cover, at least partly, the 0.00–0.07 m depth interval are used. Systematic differences between the data sets, i.e. multiplicative and additive biases, are removed by scaling ERA5 and ERA CCI SM soil moisture data sets to the in situ data prior to the TCA.
The results for different networks are quite diverse (Fig. 7). For example, the spread of errors is relatively large for ORACLE but small for others (e.g. African Monsoon Multidisciplinary Analysis – Coupling the Tropical Atmosphere and the Hydrological Cycle (AMMA-CATCH) or Sungkyunkwan University (SKKU)). The median errors per network vary between 0.03 and 0.05 m3 m−3, with some outliers in both directions. Note that the triple collocation analysis estimates the combined representativeness and sensor errors, although the latter are assumed to be small compared to the natural spatiotemporal variability (Gruber et al., 2013).
There is a clear trend of decreasing mean errors with increasing sensor depths (Fig. 8a), which is likely due to a reduction in high-frequency signals and sensor perturbations with depth. Note that this decreasing trend is observed despite the increasing discrepancy in depth support between the ISMN data and the two surface soil moisture data sets (ERA5 and ESA CCI SM). Thus, theoretically, in situ representativeness errors are expected to be even lower than computed. A potential explanation for the slight increase in the median error for the deepest layer (100–255 cm) may be small sample size and the poor soil parameterisation of the land surface model at this depth. Similar patterns were observed by Gruber et al. (2013).
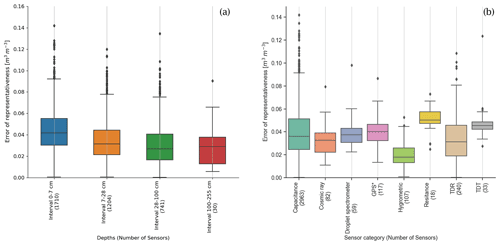
Figure 8Representativeness errors for different sensor depths (a) and sensor types (b) derived with triple collocation. Summary of triple collocation characterisation of random errors per sensor type. Values in parentheses show the total number of sensors for each depth interval and type, respectively.
Concerning the sensors used, there is a large spread in computed representativeness errors for time domain reflectometry (TDR) and capacitance sensors. While the costly hygrometric sensors have the lowest mean error, the mean error of resistance probes is the highest (Fig. 8b). Since cosmic-ray and Global Navigation Satellite System (GNSS)/GPS reflectometry sensors integrate over larger horizontal and vertical domains, one would expect lower representativeness errors for these sensors compared to the point observations. However, this is not confirmed by our triple collocation results. Possibly, the advantage of the larger spatial support of these systems is counteracted by their lower signal-to-noise ratio. Error information at the site, sensor, or data set level is currently not routinely available for the stations in the ISMN but would be required for a proper weighting of individual stations in large-scale validations (Gruber et al., 2018, 2019b).
4.1 User uptake
As mentioned earlier, over 3000 users have registered to the ISMN, while 20–30 new users register each month. Most users are based in the USA, China, India, and Europe (Fig. 9). When asked for the intended use of the data, the four main GEO benefit areas are water, disaster, agriculture, and climate sectors, all with a similar share between 16 % and 30 %. Most users come from research organisations (41 %), higher or secondary education (32 %), and non-profit organisations (19 %). Only few users come from public bodies (6 %) or private companies (2 %).
The large uptake of the ISMN for soil moisture studies is particularly due to the simplicity of accessing and using multiple data sets from a wide variety of networks. Initially, the ISMN was established to facilitate the calibration and validation of SMOS-based soil moisture products (Dorigo et al., 2011b) and, still, satellite soil moisture calibration, validation, and algorithm improvement are the primary applications served by the ISMN (Table 5). In the following, we discuss the major purposes the ISMN has been used for, based on a comprehensive review of all studies that used and cited the ISMN in peer-reviewed scientific publications.
4.2 Satellite product evaluation and development
4.2.1 Scientific studies
Soil moisture measurements from the ISMN have been widely used as reference data sets for the development and evaluation of satellite soil moisture products, which are mostly global coarse-scale surface soil moisture products (Table E1; n=212). Although initially the goal was to support algorithm development and validation of the SMOS satellite, which was indeed the case for 85 studies, many other satellite missions profited from the ISMN data, most notably SMAP (n=52), Advanced Scatterometer (ASCAT; n=50), AMSR-E (n=46), and ESA CCI (n=34). Fortuitously, the data have also been discovered for the evaluation of soil moisture products from less used sensors, including the Chinese Feng-Yun 3B, HY-2, and Gaofen-1 satellites (Parinussa et al., 2014a, 2018; Zhao et al., 2014; Xing et al., 2017), Meteosat Second Generation (MSG) Spinning Enhanced Visible and Infrared Imager (SEVIRI; Leng et al., 2015, 2017), MODIS (Gumbricht et al., 2017; Gumbricht, 2018), Aquarius (González-Zamora et al., 2016), and Landsat (Zhao et al., 2017; Pradhan, 2019).
Recently, the ISMN was recognised as validation source for testing algorithms to derive soil moisture from Global Navigation Satellite Systems (GNSS; e.g. Kim and Lakshmi, 2018; Chew and Small, 2020). Similarly, there is an increasing trend in the use of ISMN data for the validation of novel high-resolution satellite soil moisture products, which either downscale coarse-resolution products through the use of other finer-resolution satellite or ancillary data (e.g. Sheng et al., 2019; Helgert and Khodayar, 2020) or directly derive soil moisture from high-resolution synthetic aperture satellites like Sentinel-1 (e.g. Rodionova, 2019b; Foucras et al., 2020). It should be noted that the ISMN and its contributing networks are mostly designed for analysing time series, thus lacking reference data to assess spatial patterns in the data, particularly in high-resolution products (de Jeu and Dorigo, 2016).
Soil moisture measurements from the ISMN have been used as a training set for various data-driven approaches. In situ observations were ingested into machine learning frameworks together with several ancillary predictor variables, either to simulate soil moisture at a very high spatial resolution (Zappa et al., 2019) or to create long-term records (O and Orth, 2021). Greifeneder et al. (2021) developed a machine learning approach to estimate surface soil moisture from Landsat optical and thermal and Sentinel-1 SAR imagery in the Google Earth Engine, using ISMN soil moisture as target variable. Large-scale monitoring networks are necessary to build reliable models for spatially wide analysis, while dense networks are ideal for accurate localised models (Senanayake et al., 2019; Abbaszadeh et al., 2019).
Usage-oriented evaluation studies have focussed on the intercomparison of multiple coarse-scale satellite products, using the ISMN data as a reference, either to select the best performing sensor for a specific application or geographic region (e.g. Beck et al., 2021; Karthikeyan et al., 2017) or to combine them in an optimal way to build a superior product (e.g. Liu et al., 2011; Hagan et al., 2020).
Since direct satellite observations of soil moisture are only possible for the surface layer, most studies concentrate on the evaluation of surface soil moisture. Yet, various studies also focus on products derived from surface soil moisture that represent moisture in deeper (root zone) layers, either through exponential filtering (e.g. Paulik et al., 2014; Tobin et al., 2017), empirical models (Pradhan, 2019), or by assimilating them in land surface models (e.g. Pablos et al., 2018; Blyverket et al., 2019a). Occasionally, the ISMN is used for the evaluation of other satellite data sets, e.g. soil temperature data to validate the freeze/thaw state (Rautiainen et al., 2016; Hu et al., 2019).
4.2.2 ISMN as part of operational services
Because of its operational nature and advanced quality control procedures, the ISMN has been identified as the primary reference data source for future operational validation systems for global satellite-based soil moisture products (Bayat et al., 2021). But, already today, the ISMN is an integrative part of several operational satellite soil moisture production chains.
-
In 2005, the Satellite Application Facility on support to operational Hydrology and water management (H SAF) started to operationally produce and validate precipitation, soil moisture, and snow products from satellites operated by EUMETSAT (Rinollo et al., 2013). ISMN data are used for the calibration and validation of various soil moisture products produced in H SAF from the Metop Advanced Scatterometers, including global climate data records and near-real time products of surface soil moisture and a root zone soil moisture product over Europe.
-
The Climate Change Initiative of the European Space Agency (ESA CCI) uses data from the ISMN to assess, each year, the quality of new soil moisture climate data record releases and their improvements with respect to forerunner versions (Gruber et al., 2019b; Dorigo et al., 2015). Within ESA CCI, ISMN data were also used to quantify the spatial representativeness of ESA CCI satellite and climate model data sets (Nicolai-Shaw et al., 2015b). The validation is systematically performed through the use of QA4SM (see below) and the results and validation settings are published (e.g. https://doi.org/10.5281/zenodo.4120205; Scanlon, 2020).
-
C3S produces authoritative, quality-assured climate data records of soil moisture and other essential climate variables (ECVs). The satellite soil moisture products produced within C3S are routinely updated every 10 d with the latest available satellite observations. C3S uses the ISMN soil moisture data in combination with the metadata provided to categorise product performance per land cover and climate type (Scanlon et al., 2019). The validation is systematically performed through the use of QA4SM, and the results are transparently published (e.g. https://doi.org/10.5281/zenodo.4736927; Preimesberger, 2021).
-
Copernicus Global Land Service (CGLS) produces, within 1–2 d after satellite overpass, soil moisture data sets from Sentinel-1 and from a combination of Sentinel-1 and ASCAT (SCATSAR; Bauer-Marschallinger et al., 2018, 2019). The latter propagates the surface observations to deeper layers by means of the so-called soil water index (SWI). Both products are provided at 1 km resolution for Europe. ISMN data are used for validating moisture in surface and deeper soil layers.
Recently, the Quality Assurance for Soil Moisture (QA4SM) service (https://qa4sm.eu, last access: 28 October 2021) was initiated to bring together validation methodologies, community protocols, reference data, and satellite observations to evaluate and intercompare soil moisture data products in a coherent, standardised, and transparent way. In situ data sourced from the ISMN are an integral part of this validation system (Scanlon et al., 2019; Gruber et al., 2020). The combination of ISMN, QA4SM, and enhanced quality control protocols and selection procedures to establish a set of fiducial reference measurements is expected to become the standard for satellite soil moisture validation in the next few years (Bayat et al., 2021).
4.3 Model development and validation
In situ measurements are the most important reference source when assessing the performance of land surface models, reanalyses products, and hydrological models. Although also satellite observations are a valuable validation source (e.g. Szczypta et al., 2014), these measure only the upper ∼5 cm of the soil and, hence, do not allow for the validation of deeper layers. Besides, state-of-the-art reanalysis products like ERA5 Hersbach et al., 2020 assimilate satellite soil moisture observations so that, for these products, in situ data remain the only truly independent validation reference. Since the sampling rate (hourly) of the ISMN observations is generally higher, and their spatial support lower than that of most models (Reichle et al., 2004), model evaluation is, in principle, not limited by the spatial and temporal resolution of the in situ data, although representativeness issues often remain.
In particular for global assessments, the availability of harmonised data over multiple networks makes the ISMN a preferred reference source over data from individual networks (Xia et al., 2019). Table E2 shows that many well-established, state-of-the-art land surface model and reanalysis products have been evaluated against data from the ISMN, not only by users of these products (e.g. P. Li et al., 2020) but also by the developer teams themselves, e.g. Reichle et al. (2017) for MERRA-2, Albergel et al. (2020) for the Land Data Assimilation System (LDAS)-Monde, and Balsamo et al. (2015) and Fairbairn et al. (2019) for various European Centre for Medium-Range Weather Forecasts (ECMWF) products.
Also, a suite of new products have been assessed against the ISMN, including multi-model ensembles (Schellekens et al., 2017; Cammalleri et al., 2015), data-driven evaporation models (Martens et al., 2017; Lievens et al., 2017), and statistical infiltration models Pal and Maity (2019). As for remote sensing products, a trend towards higher-resolution regional to global model-based products can be observed. Among others, ISMN data have been used to validate new high-resolution reanalysis products over the USA (McDonough et al., 2018) and Europe (Naz et al., 2020). Apart from soil moisture, soil temperature data have also been drawn from the ISMN for model validation purposes (e.g. Wang et al., 2016; Albergel et al., 2015).
Besides the evaluation of hydrological or land surface model improvements, the ISMN has also frequently served model development in a more fundamental way. For example, Hartmann et al. (2015) developed a large-scale karstic groundwater recharge model over Europe and the Mediterranean and calibrated and evaluated this model with observations of actual evapotranspiration from FLUXNET (Baldocchi et al., 2001) and soil water content data from the ISMN. Martínez-Fernández et al. (2021) used data from the ISMN to assess the fraction of precipitation that is stored in the soil profile. Calvet et al. (2016) used soil moisture and temperature data from the SMOSMANIA (soil moisture observing system – meteorological automatic network integrated application) network contained in the ISMN to derive pedotransfer functions for the soil quartz fraction in southern France. Pal et al. (2016) developed a statistical model to estimate the vertical soil moisture profile using SCAN data of the ISMN as source, while, on a similar note, Shin et al. (2018) developed a non-parametric evolutionary algorithm to predict soil moisture dynamics using ISMN data over Oklahoma and Illinois. Similarly, Jalilvand et al. (2018) estimated the drainage rate from surface soil moisture drydowns from ISMN data. A bridge between soil moisture and vegetation dynamics was made by Sawada (2018), who used ISMN data to validate a new ecohydrological land reanalysis to better simulate the link between sub-surface soil moisture and vegetation dynamics. Finally, Brocca et al. (2015) developed a water balance approach to estimate rainfall from soil moisture observations based on reverse modelling and evaluated this at several ISMN sites in Europe. Later refinements of this approach were also evaluated against data from the ISMN (Hoang and Lu, 2019).
4.4 Meteorological applications
Soil moisture from the ISMN has often been used to validate the land surface representations of meteorological forecasting models. However, as meteorological forecasts often rely on the latest generation of land surface models, in practice there is often no strict distinction between meteorological and land surface model development as described in the previous section. Notable examples are the various generations of the Tiled ECMWF Scheme for Surface Exchanges over Land (TESSEL) models used both in the Integrated Forecasting Systems and reanalysis products of ECMWF, the development of which greatly profited from soil moisture and temperature data from the ISMN (Albergel et al., 2012a, 2015). Dirmeyer et al. (2016) assessed the skill and soil moisture memory effects of various weather and climate models with ISMN data over the USA. Similarly, Angevine et al. (2014) assessed the soil moisture skill of the Weather Research and Forecasting Model (WRF; Table E2).
Several studies have used the ISMN data to assess the forecast skill or new implementations of numerical weather prediction models. For example, de Rosnay et al. (2019) and Rodriguez-Fernandez et al. (2019) used in situ soil moisture observations from several ISMN networks to validate the impact of assimilating SMOS brightness temperatures and soil moisture, respectively, to predict soil moisture up to 5 d ahead. Similarly, Lin and Pu (2019, 2020) used ISMN data to examine the impact of MAP soil moisture assimilation in WRF for near-surface, short-range weather forecasts. Boussetta et al. (2015) used over 500 ISMN sites to assess the impact of assimilating surface albedo and vegetation states from satellite observations on numerical weather prediction.
From a more methodological, land–atmosphere perspective, Lee (2018) used the ISMN data to study the role of soil moisture in triggering rainfall over West Africa. Conversely, S. Zhang et al. (2019) studied the role of rainfall on soil cooling using data from the SMOSMANIA network in southern France.
4.5 Drought monitoring
In a drought monitoring context, ISMN data have frequently been used in a convergence of evidence approach in combination with other drought-related variables or indicators. For example, Scaini et al. (2015) compared the variability in the in situ soil moisture measurements from the ISMN, SMOS surface soil moisture, and two drought indices based on climatic information to study droughts in Spain. Mu et al. (2019) used visible and near-infrared (VNIR) satellite data and soil moisture distributed by the ISMN to monitor drought in the southern USA. On a more technical level, Gruber et al. (2018) assessed the use of spatially sparse ISMN in combination with a continuous model for operational agricultural drought monitoring over the USA.
ISMN data have also been used to classify new and more traditional drought indices, such as the Standardised Precipitation (Evaporation) Index and the Palmer drought severity index (Vicente-Serrano et al., 2012; Krueger et al., 2019). Sadri et al. (2020) used the ISMN data from the RISMA network to assess a global near-real time soil moisture index monitor for food security using SMOS and SMAP data. Likewise, Fang et al. (2021a) used ISMN data to evaluate a new drought monitoring approach based on high spatial resolution soil moisture data from downscaled SMOS and SMAP data over Australia. Chen et al. (2019) used precipitation distributed through the ISMN to evaluate a drought index derived from Sentinel-2 in Spain. Due to relatively good coverage over Europe, Cammalleri et al. (2015) used ISMN data to assess various model soil moisture products as input to the European Drought Observatory (EDO; http://edo.jrc.ec.europa.eu, last access: 28 October 2021). Also, Mishra et al. (2018) used data from the ISMN to assess a suite of land surface models used to reconstruct drought events in India since the mid-1900s.
4.6 Other applications
ISMN data have been used for various other purposes, going beyond what the ISMN was originally developed for. In addition to supporting satellite and land surface model soil moisture product development, the ISMN has played a fundamental role in the validation of a wide range of hybrid observation-based products, including a Soil Moisture Saturation Index (Campo et al., 2011), apparent thermal inertia surface estimates (Notarnicola et al., 2012), an improved antecedent precipitation index (API) formulation (Ramsauer et al., 2021), data-driven surface and root zone soil moisture predictions (Kornelsen and Coulibaly, 2014; Manfreda et al., 2014; L. Wang et al., 2020; O and Orth, 2021), improved satellite albedo products (Liu et al., 2014), and estimates of effective permittivity and brightness temperature of organic soils (Park et al., 2019).
The ISMN has frequently been used to assess the impact of assimilating satellite observations into hydrological models (Khaki et al., 2019; Nair et al., 2020; Gruber et al., 2015; Shin et al., 2016; P. Li et al., 2020; Y. Wang et al., 2020), land surface models (Nair and Indu, 2016; Zhao and Yang, 2018; Nair et al., 2020), and carbon models (Scholze et al., 2016). On a more methodological level, Q. Zhang et al. (2019) assessed a new data assimilation scheme against ISMN observations. Gruber et al. (2018) even assimilated spatially sparse ISMN observations directly into a spatially continuous land surface model over the continental USA and tested the preconditions (e.g. requirements for the signal-to-noise ratio and number of sites) for having a significant positive impact.
The wealth of data covering a wide range of surface and climate conditions has been frequently exploited to study soil water dynamics at various spatial and temporal scales and their (climatic) drivers (Brocca et al., 2014b; Hirschi et al., 2014; Ojha et al., 2014; Qin et al., 2018; Kumar et al., 2019a; J. Tian et al., 2019; Nicolai-Shaw et al., 2015b; Dong et al., 2020; T. Wang et al., 2017; Verrier, 2020; Deng et al., 2020a), flood dynamics (Esposito et al., 2018), or the impact of soil moisture on plant growth (Hottenstein et al., 2015).
The ISMN has been used to develop and test new statistical validation approaches (Zwieback et al., 2013; Gruber et al., 2016; Afshar et al., 2019). Finally, the standards and quality control procedures adopted by the ISMN have served as a guideline for establishing and benchmarking new networks (Kim et al., 2019; Skierucha et al., 2012b, a; Petropoulos and McCalmont, 2017), soil moisture metadatabases (Liao et al., 2019; Xia et al., 2015), or validation services (Kumar et al., 2012) and to assess or improve alternative sensing techniques and constellations (Xaver et al., 2020; Kapilaratne and Lu, 2017; Nguyen et al., 2017; Mahecha et al., 2017).
4.7 Added scientific value of ISMN over other data sources
Although the ISMN has facilitated hundreds of scientific studies, it is impossible to quantify precisely to what degree the ISMN has contributed to product improvements and new insights that could not have obtained otherwise. Admittedly, data from several networks are also distributed through other portals, typically providing access to a single network (e.g. SNOTEL data through https://www.wcc.nrcs.usda.gov/snow/, last access: 28 October 2021). The major contribution of the ISMN to scientific advances is primarily given by the access to the many networks and data sets that are uniquely distributed through the ISMN and in the easy access to thousands of harmonised data sets with a single click.
The tracked download statistics reveal that approximately one-third (34 %) of the studies making use of the ISMN use multiple networks, the choice of which depends on the scope of the study, the geographical region, period of interest, and the year the study was performed (with more networks becoming available over time). Examples of such studies using data from more than 20 different networks are Paulik et al. (2014) (23 ISMN networks used), Dorigo et al. (2015) (28), Beck et al. (2021) (27), Grillakis et al. (2021) (27), O and Orth (2021) (51), van der Schalie et al. (2021) (46), Li et al. (2021) (20), and Wu et al. (2021) (20).
Although it is impossible to pin down the exact contribution of the ISMN to process understanding and product improvements, it is very likely that, mainly, studies have discovered flaws in satellite products that would not have been detected without the use of the ISMN. The main reason is that the large number of networks give insight into the product skill in a large variety of climate zones, land cover types, and so on, which no single network could have provided. This allows for the development of, globally, more robust models and products. Second, the data harmonisation and unified quality control minimise the chance that differences in skill potentially observed between locations with different environmental characteristics is an artefact of a different treatment of the data.
Several studies were identified that not only used the multitude of ISMN data to expose flaws in existing products but also to directly improve data products and models, e.g. by calibrating hydrological model parameters for local site conditions (Beck et al., 2021; Kang et al., 2019; Moradizadeh and Srivastava, 2021; Grillakis et al., 2021), by merging them with other observations and models (Gruber et al., 2018; Xu et al., 2018), or using them as training data in machine learning approaches (Pan et al., 2017; Eroglu et al., 2019; O and Orth, 2021; Greifeneder et al., 2021).
5.1 Scientific challenges
5.1.1 Diversity of measurements
The in situ measurements of soil moisture data in the ISMN have been collected by a large variety of sampling techniques. The early networks contained in the ISMN (e.g. RUSWET, CHINA, and MONGOLIA) are based on gravimetric sampling, which is still considered the most accurate approach (Romano, 2014). However, it is labour intensive and invasive and, thus, measurements are infrequent (weekly or even coarser sampling), taken from a slightly different location every time, and may contain systematic errors between sampling dates.
Nowadays, the most commonly used techniques for systematic in situ sampling are based on the contrasting dielectric properties of soil and water and comprise time domain reflectometry (TDR) and frequency domain reflectometry (FDR; Robinson et al., 2008), e.g. AMMA-CATCH, BNZ LTER, CARBOAFRICA, FMI, GTK, HYDROL-Net Perugia, IPE, LAB-net, ORACLE, OzNet, SASMAS, SWEX_POLAND, Upper Danube Catchment (UDC) SMOS, WSMN, and UMSOL networks). In particular, capacitance sensors based on FDR are becoming more and more widespread because of their lower cost compared to TDR sensors, despite their lower accuracy (Romano, 2014; Brocca et al., 2017). However, great technical improvements are being achieved with capacitance sensors, thus improving their reliability. Yet, TDR and FDR techniques only provide point measurements, i.e. they are representative of small volumes of soil.
Slightly larger soil volumes (diameter of 15 to 60 cm) are observed with the neutron scattering method, where the density of thermal neutrons produced by scattering of fast neutrons on soil hydrogen can be related can be related to soil moisture through a calibration curve (Gardner and Kirkham, 1952; Romano, 2014) (e.g. IOWA network). The cosmic-ray method has been developed based on similar principles of neutron scattering, (Zreda et al., 2012, 2008). Cosmic-ray neutron sensors (CRNSs) are located on or above the soil surface and measure and count the number of cosmogenic neutrons in air above the land surface and that are in equilibrium with the soil. CRNS measurements are representative of significantly larger volumes compared to neutron probes (i.e. radius of a few 100 m and depths between 0.12 and 0.70 m, depending on the soil wetness), and thus can be used to bridge the scales between point observations and coarse resolutions of satellite observations and models. CRNS measurements, which are used in the COSMOS network, are sensitive to vegetation and have relatively high noise levels. To reduce this noise, the data are resampled to daily mean values.
Also, the measurements of the PBO_H2O network, which uses global positioning system (GPS) receivers, can be used to bridge the scales between point and satellite sensors. GPS sensors, initially used for geophysical and geodetic applications, have been found to be well suited for measuring soil moisture (Larson et al., 2008). The GPS signals are representative for an area of approximately 300 m2 and are L band, hence making them ideal for comparison against satellite missions like SMAP and SMOS (Larson et al., 2008).
As shown, all measurement techniques have their strengths and limitations (Bogena et al., 2015; Dorigo et al., 2011b), which complicates their combined, bulk use. Depending on the application and process scales, the user needs to carefully consider which networks to use or exclude and how to interpret the results obtained. A task of the ISMN could be to translate community-based guidelines (Gruber et al., 2020; Montzka et al., 2020) to recommendations for the use of individual data sets.
5.1.2 Spatial and temporal representativeness and scaling
Soil moisture is highly variable in space as a result of complex interactions between soil characteristics, topography, vegetation, and meteorological conditions. Depending on the spatial scale considered, the dominant controlling factor(s) can be different (Crow et al., 2012; Western et al., 2002). Hence, validation of satellite-derived products is hindered by the spatial mismatch between ground observations and satellite footprints (Gruber et al., 2020; Molero et al., 2018; Gruber et al., 2013). Ideally, to reduce the representativeness error of in situ references, enough stations should be deployed within a satellite footprint to develop robust areal soil moisture estimates (Brocca et al., 2007; Famiglietti et al., 2008; Colliander et al., 2017). However, this is a costly solution, and therefore, only a limited number of sites provide such a set-up (Crow et al., 2012; Colliander et al., 2017). In the ISMN, the networks of VDS, BIEBRZA_S-1, RSMN, UDC_SMOS, LAB-net, FMI, and USDA-ARS have been set up in this way. When available, in situ stations within the same satellite pixel should be averaged, either through arithmetic mean or weighted average. Higher weights should be given to stations expected to be more representative of the satellite grid average, e.g. by using Voronoi diagrams (Colliander et al., 2017), the inverse footprint method (Nicolai-Shaw et al., 2015a), the time stability concept (Vachaud et al., 1985), or landscape properties such as land cover and/or soil texture (Bircher et al., 2012). Alternatively, triple collocation analysis can be used to quantify and correct for spatial sampling errors of in situ stations (Miralles et al., 2010; Gruber et al., 2013). The resulting pixel-scale soil moisture ground reference, i.e. the averaged value from dense networks with several stations per satellite grid or the original time series in the case of sparse networks with a single station per pixel, should undergo a statistical rescaling (Gruber et al., 2020). Indeed, a direct comparison of in situ and satellite products would be subject to representativeness errors, which may dominate the total soil moisture retrieval errors (Chen et al., 2017; Gruber et al., 2013; Molero et al., 2018). Rescaling accounts for systematic representativeness errors arising from different spatial resolution and different vertical measurement support, i.e. penetration depths of microwave sensors and in situ sensor placement depths (Gruber et al., 2013, 2020), but does not correct for random representativeness errors. One way to address this is the triple collocation described above. Also, systematic representation errors may have a time-varying (e.g. seasonal) component, which, unless explicitly accounted for, may lead to temporally aliased results. In any case, even though differences in spatial representativeness between ground and satellite measurements impact the evaluation metrics, single stations are still a valuable source for assessing the relative skill of soil moisture products with a similar footprint (Dong et al., 2020).
Also, temporal representativeness issues may exist, but due to the hourly sampling of most data sets, the ISMN usually have a denser sampling than most remote sensing or model data sets. Thus, for most applications, the ISMN can be downsampled to the process or observation timescale of interest. However, some of the older, manually sampled data sets have sampling intervals of about 2 weeks and, thus, may miss many higher-frequency wet or dry spells. On a similar note, data sets with a daily sampling or averaging (e.g. cosmic-ray or GPS reflectometry observations) may miss rainfall peaks and are unsuitable for studying sub-daily variability.
5.1.3 Integration of low-cost sensors
The development and use of low-cost sensing technologies, especially in the environmental sciences, have seen a pronounced increase during the last decade. Such a rise is driven by several factors, e.g. the reduced cost of micro-controllers, electric components, and sensors (Mao et al., 2019). Even though a rich variety of low-cost soil moisture sensors based on different measurement principles has been developed (Chawla et al., 2019; González-Teruel et al., 2019; Kumar et al., 2016), capacitance sensors gained the most popularity because they are relatively inexpensive, easy to operate, and provide reliable observations (Kojima et al., 2016).
The considerably lower cost of these sensors compared to traditional probes makes them suitable for high-density and/or large-scale monitoring of soil moisture. The possibility to map soil moisture (and other environmental variables) with an unprecedented spatial coverage can generate new insights into its dynamics and create new opportunities. For instance, high-density networks of low-cost sensors can be used to reduce spatial representativeness errors by providing numerous observations within a satellite footprint (Teuling et al., 2006). Similarly, one can deploy a temporary low-cost sensors network to identify the most suitable location(s) for long-term monitoring. Such locations could then be equipped with professional sensors, while moving the low-cost network to other sites (Zappa et al., 2019). Another exciting opportunity offered by low-cost sensors is the deployment of networks in low-income countries.
The ISMN has integrated low-cost sensor measurements from the GROW observatory (see Sect. 5.1.4), but a number of practical challenges arise when integrating such data. A key aspect to consider is the lifetime. Depending on the robustness of the sensor (of both electronic components, such as micro-controllers, and sensor housing), reliable measurements can be recorded for a period ranging from a few months to years (Xaver et al., 2020) but even be shorter in extreme environments. Another aspect, particularly affecting automation, is data storage and transmission. Some low-cost sensors allow for wireless communication with a main server (Bogena et al., 2007; Majone et al., 2013), while other sensors have a limited internal storage, and data should be collected persistently over time (e.g. every 80 d; Xaver et al., 2020). Furthermore, it is necessary to assess their accuracy and robustness (Castell et al., 2017). Therefore, low-cost sensors should always undergo thorough evaluation to (i) quantify the agreement of low-cost sensor measurements with gravimetric samples and/or professional probes, ideally considering a wide range of soil and climatic conditions, (ii) assess the inter-sensor variability, and (iii) test the suitability for usage in field conditions (Domínguez-Niño et al., 2019; Kizito et al., 2008; Mittelbach et al., 2012; Adla et al., 2020).
5.1.4 Integration of citizen observations
Citizen science is defined as the involvement of non-experts in collecting data (Bonney et al., 2009). Crowd-sourced measurements of soil moisture are now possible because of the development of low-cost sensors (Sect. 5.1.3). Crowd-sourcing has the potential to overcome some of the most challenging issues of soil moisture monitoring, such as the use of many sensors to address scaling issues, and the fact that the observations can be carried out anywhere, as long as there are citizens willing to collect the data.
An outstanding example of a citizen science project focusing on soil moisture is the GROW Observatory (https://growobservatory.org/, last access: 28 October 2021), from which data sets spanning at least a full year of observations have been added to the ISMN. Within GROW, thousands of low-cost sensors have been distributed to farmers, gardeners, and growers across Europe (Kovács et al., 2019). Focus areas have been identified based on a number of scientific criteria and the presence of active and engaged communities. Within each focus area, covering spatial scales from 20 to 200 km, hundreds of sensors have been distributed. Some sensors served as back-ups for potential failures, so that malfunctioning sensors could be promptly replaced, enabling long-term continuity of observations. In order to ensure high standards of the measurements, citizens have been trained in the selection of the correct locations in which to install sensors and how to properly install and maintain them through field manuals, online courses, meet-ups, and remote support (Kovács et al., 2019). Overall, more than 6000 sensors were deployed to provide soil moisture measurements across 13 European countries, demonstrating that citizen observatories can be integrated in Earth observation activities and contribute to validation of remotely sensed products (Zappa et al., 2020).
The integration of citizen observations in the ISMN is challenging for multiple reasons. Crucial is the long-term engagement of citizens, which needs to be thoroughly addressed from the early stages of designing a citizen observatory. It is necessary to create long-lasting communities that go beyond the duration of the contributory projects (Grainger, 2017). Successful citizen observatories have been those where citizens were able to benefit directly from the data collected by others (Fritz et al., 2017). Additionally, education and resources are essential to boost individual motivation to continuously participate and to sustain membership renewal as natural participation cycles change (Zappa et al., 2020). Even if proper training is provided to citizens, uncertainty in the quality of the data is the main limitation for the use of crowd-sourced observations in science (Lukyanenko et al., 2020). The reliability of measurements from individual sensors is unknown because of, e.g., the selection of unsuitable locations, incorrect sensor installation, and existence of defective sensors. However, increasing the number of sensors within the same satellite pixel reduces this uncertainty. Additionally, a visual inspection of the time series and the application of automated quality flagging controls, such as those developed within the ISMN, could be used to mask suspicious observations (Xaver et al., 2020). Overall, it has been shown that well-organised citizen science projects can provide trustworthy contributions to the scientific community (Kosmala et al., 2016; Palmer et al., 2017).
5.2 Operational challenges
5.2.1 Automation
Part of the contributions to the ISMN (7 networks with around 900 stations) are inserted into the ISMN in NRT. This process is fully automated, including data downloaded from data providers, harmonisation, flagging, insertion into the database, and updating metadata tables. However, because of different data recording and handling mechanisms at the provider side and differences in data sharing mechanisms and policies, the ISMN is also partially manually operated and will probably also have to continue in this way in the future. The differences between the fully automated and the manual approach are confined to the data download and data ingestion into the processing chain. Data harmonisation and quality control are automated for all data sets (see Fig. B1).
A major challenge in the automation process is the enormous heterogeneity of input data formats. Moreover, these change over time for individual networks, stations, and even sensors, as sensors may fail or the method for data logging is changed. Thus, error detection and handling is of utmost importance, and frequent adaptation of the system is required to cover changes in input data.
A potential way to promote the automated insertion of new data is to allow only data that comply with a strict, yet to be defined, data standard. At the same time, this may be bear the risk of raising the barrier to contribute to the ISMN too high for several scientists.
5.2.2 Including new networks
Since data sharing with the ISMN is entirely built upon a voluntary basis and data usage is open and free, new networks may be reluctant to join. The ISMN is in contact with several network providers who are happy to collaborate but are restrained by data sharing policies, which does not allow data sharing at all or only after a certain time. Furthermore, for governmentally operated networks it is often not allowed to share data as open-access or it is unclear who can make these decisions.
Solving such issues can be supported by collaborative data-hosting facilities, like the Global Terrestrial Network – Hydrology (GTN-H; https://www.gtn-h.info/, last access: 28 October 2021), which have a strong connection to governmental bodies like the International Centre for Water Resources and Global Change under the auspices of UNESCO, the United Nations Environment Programme, the International Science Council, and World Meteorological Organization (WMO).
5.2.3 Appropriate recognition of data providers
Not all users correctly cite the ISMN and the involved networks, as stated in the terms and conditions for ISMN data use (ISMN, 2020). Proper citation of single networks is important for giving data providers the recognition that is required to convince funding agencies to continue supporting the maintenance and operation of these networks. In the end, this not only affects the networks themselves but also the open-access availability of data through the ISMN as a whole. Some network providers have raised their concerns in this matter and, hence, continuous efforts are needed to maintain a strong visibility of network data providers towards users.
5.2.4 Transparency and traceability
The ISMN data collection is constantly evolving. New data records are added, and existing ones are extended or, if necessary, reprocessed and corrected. Not only the data but also the underlying code changes. These updates and any retroactive changes made to the data archive are tracked within the ISMN but not yet readily passed on to the users. The updates can lead to differences in analyses on the user side, e.g. when considering obvious changes such as temporal extensions or new stations, and lead to non-reproducible results. Therefore, any update must be traceable and clearly communicated, requiring a system to track and store these changes. Version tracking and digital object identifiers (DOIs) are ways of identifying each database access and, therefore, allow tracing back to past states of the ISMN. Such a mechanism is required to make the ISMN compliant with the FAIR (Findability, Accessibility, Interoperability, and Reusability) data principles (Wilkinson et al., 2016).
In this study, we reviewed the first decade of operations of the ISMN. Besides satisfactorily fulfilling its initial target, i.e. supporting satellite soil moisture product validation and calibration, many additional more or less foreseen uses have emerged. In addition, an increasing number of services and product development chains have routinely included the use of ISMN data in their operational structure. The ISMN started as research activity funded by ESA, and ever since, ESA have provided continuous financial support for ongoing research, development, and operations. In spring 2021, a milestone was achieved when the German Ministry of Transport and Digital Infrastructure announced that it will commit to permanently fund the ISMN operations and development from late 2021. The execution will be with the German Federal Institute of Hydrology (BfG) and the International Centre for Water Resources and Global Change (ICWRGC) based in Koblenz, Germany. At the same time, all network data sets have always been freely contributed by dedicated researchers. To guarantee the availability of these resources for climate and environmental monitoring also for the next decade, we plead with governments and international bodies for systematic funding of its participating data-providing networks too.
To maximise geographic coverage and data usage, the policy of the ISMN has always been to integrate data sets without strict requirements on sensors, sampling protocol, or data quality. The resulting strongly heterogeneous data set characteristics call for far-reaching quantification and traceability of errors, from sensor calibration and data download to the point measurement and the spatiotemporal support of the application. Thus, besides expanding its coverage to data-poor regions and landscapes, the ISMN shall spend the next few years focusing on developing methodologies to fully characterise the uncertainties and usability associated with the individual data sets. An important step in this direction will be made within the ESA-funded Fiducial Reference Measurements for Soil Moisture initiative. With the foreseen developments, the ISMN will reach a new level of maturity in the coming decade.
A summary of each network is given in Table A1, while more details are given in the subsequent paragraphs.
A1 AACES
AACES stands for the Australian Airborne Cal/val Experiments for SMOS. This campaign network covers a 500×100 km2 study area located in southeastern Australia, covering a variety of topography, vegetation, and climate classes (Rüdiger et al., 2007; Peischl et al., 2012). Measurements of soil moisture, soil temperature, and precipitation were taken between May 2009 and September 2010. The AACES calibration and validation campaigns were a temporary project; therefore, no further data sets will be available.
A2 AMMA-CATCH
The AMMA-CATCH observatory gathered data from densely instrumented mesoscale sites in West Africa (Benin, Niger, and Mali). The network is devoted to long-term regional monitoring of global change impacts on the critical zone. Height stations in Benin and four stations in Niger of the network are included in the ISMN from 2006 to the present, including surface soil moisture and root zone soil moisture until 1 m depth. For more information, see Galle et al. (2018).
A3 ARM
The Atmospheric Radiation Measurement (ARM) programme has three soil sensor networks across north-central Oklahoma and southern Kansas in the USA, including the Soil Water And Temperature System (SWATS), through 2016, and, presently, the Soil Temperature and Moisture Profile (STAMP), and Surface Energy Balance System (SEBS). The SWATS and STAMP have profiles at 5–8 depths up to 175 cm, while the SEBS measure at 2.5 cm. All sites are co-located with a suite of meteorological and radiative measurements available from https://arm.gov/ (last access: 28 October 2021; Cook, 2016a; Cook and Sullivan, 2018).
A4 AWDN
The Automated Weather Data Network (AWDN) network is located in Nebraska, USA, and consists of 50 stations. The data sets were collected by the High Plains Regional Climate Center, and data availability is from 1998 to 2010 but varies per station.
A5 BIEBRZA_S-1
The dense soil moisture sites suited for the validation of high-resolution Sentinel-1 soil moisture products were established in the Biebrza Wetlands in northeastern Poland in May 2015 (Musial et al., 2016). One site is located across drained grassland and the second one across natural temporarily flooded marshland. They are located within 7 km distance; therefore, weather conditions are similar but soil moisture regimes differ. Both sites are equipped with nine soil moisture stations with five soil probes each and a weather station. The sites are homogeneous regarding vegetation cover. The organic peat soils feature porosity values up to 82 %. The sites are maintained by the Remote Sensing Centre of the Institute of Geodesy and Cartography (IGiK).
A6 BNZ LTER
The Bonanza Creek Long-Term Ecological Research (BNZ LTER) network consists of 12 stations located in the boreal forest near Fairbanks, Alaska (Van Cleve et al., 2015). Soil moisture measurements start around the year 2000 for each station. In addition to soil moisture at several depths, observations are available of soil temperature, air temperature, precipitation, snow depth, and snow water equivalent.
A7 CALABRIA
The CALABRIA network operates five TDR stations measuring volumetric soil moisture at 30, 60, and 90 cm depths. The stations were installed in 2000 by the Centro Funzionale Decentrato of the Calabria region for hydrometeorological monitoring for flood and landslide risk mitigation. For more information on the performance of the network, see Brocca et al. (2011).
A8 CAMPANIA
The CAMPANIA network consisted of two stations located near the city of Naples in southern Italy. It was managed by the Centro Funzionale per la Previsione Meteorologica e il Monitoraggio Meteo-Pluvio-Idrometrico e delle Frane. The ISMN contains data from the operational start in 2000 until the end of 2008. The data sets include soil moisture measured at a depth of 0.30 m, precipitation, and air temperature. For more information on the performance of the network, see Brocca et al. (2011).
A9 CARBOAFRICA (recently renamed SD_DEM)
CARBOAFRICA/SD_DEM is located outside El Obeid in Kordofan, Sudan, and has been active since February 2002. It is operated by the Department of Physical Geography and Ecosystem Science at Lund University, Sweden, in cooperation with the Agricultural Research Corporation (ARC) in El Obeid, Sudan. From 2007–2009, eddy covariance measurements were undertaken, which are available from FLUXNET (https://fluxnet.org/, last access: 28 October 2021). For more information, see Ardö (2013).
A10 CHINA
This agricultural monitoring campaign from 1981 to 1999 includes 40 stations with soil moisture measurements up to 1 m depth and was hosted by the Institute of Geographical Sciences and Natural Resources Research at the Chinese Academy of Sciences in Beijing (Liu et al., 2001). The data set was transferred to the ISMN from the Global Soil Moisture Data Bank (Robock et al., 2000) and contains measurements made on the 8th, 18th, and 28th of each month.
A11 COSMOS
The Cosmic-ray Soil Moisture Observing System (COSMOS; Zreda et al., 2012) started in 2009 with a grant from the U.S. National Science Foundation as a 4-year project for demonstration of the then-new technology of sensing soil moisture with cosmogenic neutrons (Zreda et al., 2008). On the completion of the project, the network had 60 sites, most of them in the USA and a few in South America, Europe, and Africa. The network produces hourly soil moisture data, available in real time, to all, without restrictions. After the project funding ended in 2013, the network operations continued with the support of Quaesta Instruments, a private company. The current status is active, but the sensors are being relocated and repurposed.
A12 CTP_SMTMN
CTP_SMTMN is a multiscale Soil Moisture and Temperature Monitoring Network on the central Tibetan Plateau (Yang et al., 2013). The network, with an average elevation of 4650 m a.s.l., consists of 56 stations that measure soil moisture and soil freeze/thaw status at three spatial scales (100, 25, and 9 km). The terrain is relatively flat and covered by sparse and short grasses; the annual precipitation is about 400–500 mm. The network has been in operation since 2010.
A13 DAHRA
The DAHRA field site is located in a typical low tree and shrub savanna environment in Senegal. To limit the uncertainty in the comparison of remote sensing products and models, the site was selected to be flat, with homogeneous vegetation cover within a radius of at least 3 km. The site is equipped with two towers, i.e. a meteorological tower with meteorological, hydrological, and radiation sensors and an eddy covariance flux tower. More information can be found in Tagesson et al. (2014).
A14 FLUXNET-AMERIFLUX
AMERIFLUX is the North American contribution to the global FLUXNET. At this moment, two sites close to Sacramento, California, i.e. Tonzi and Vaira ranches, are distributed through the ISMN. Both stations provide soil moisture measurements at eight different depths down to 0.60 m. Additionally, soil temperature, air temperature, and precipitation are provided.
A15 FMI
This distributed network of in situ measurement stations gathering information on soil moisture and soil temperature has been set up in recent years at the Finnish Meteorological Institute's (FMI) Sodankylä Arctic research station in northern Finland. Between 2010 and 2017, 16 stations were installed around Sodankylä and 3 further north at Saariselkä. Each station covers a vertical measuring profile and two additional horizontal measuring points. The vertical profiles have five sensors placed close to the station at the following depths: 5, 10, 20, 40, and 80 cm in mineral and semi-organic soils and at 5, 10, 20, 30, 40 cm in organic soils. The two additional horizontal measuring points, at depths of 5 and 10 cm, have been installed approximately 10 m from the station in opposing directions to catch small-scale variations in topsoil moisture. A more detailed description is provided in Ikonen et al. (2016, 2018).
A16 FR_AQUI
The FR_AQUI network was set up by INRAE in the Bordeaux–Aquitaine region (southwestern France) in 2012 (Wigneron et al., 2018; Al-Yaari et al., 2018a). Measurements taken at this five-station network (plus the nearby Integrated Carbon Observation System (ICOS) Bilos site), include soil moisture and temperature at various depths and the height of the groundwater table. There are four sites installed in the Landes forest, which is one of the largest coniferous forests in Europe, and one site is installed close to vineyards of the Bordeaux Graves region.
A17 GROW
GROW gathered crowd-sourced observations to assess the temporal and spatial consistency of various satellite-derived soil moisture products. In total, 6500 low cost sensors were deployed in 24 GROW places in 13 countries across Europe. A subset of 150 sensors was transferred to the ISMN (Zappa et al., 2019, 2020) and contains measurements of soil moisture and air temperature. The complete data set is licensed and available at https://doi.org/10.15132/10000156. More information on the quality of the data can be found in Xaver et al. (2020).
A18 GTK
This network is operated by the Geological Survey of Finland (GTK) and contains seven stations throughout the country, with one station north of the polar circle. Measurements are taken from the upper soil layer until 0.9 m depth (soil moisture and soil temperature, as well as air temperature). The data are available from the years 2001 to 2012, but the availability varies per station.
A19 HiWATER_EHWSN
The HiWATER_EHWSN network is located on an irrigated farmland in the middle stream of the Heihe River basin close to the Gobi Desert, China. It consists of short time series between April and September 2012 collected at 174 stations by the Cold and Arid Regions Environmental and Engineering Research Institute (CAREERI) of the Chinese Academy of Science (Kang et al., 2014; Jin et al., 2014).
A20 HOAL
The Hydrological Open Air Laboratory – Soil Network (HOAL SoilNet) was set up in Petzenkirchen, Austria, as part of a concerted effort to advance the understanding of water-related flow and transport processes in a 66 ha agricultural catchment (Blöschl et al., 2016). Soil moisture has been monitored since 2013 at about 30 locations, at four depths, and at time intervals of 30 min using time domain transmission sensors. Measurements are taken from permanent stations, located in grassland, forest, or at field boundaries, as well as from stations that are temporarily installed in cropland (Vreugdenhil et al., 2013).
A21 HOBE
HOBE is a hydrological observatory established in the western part of Denmark in the Skjern River catchment (Jensen and Refsgaard, 2018). Within the sub-catchment of Ahlergaarde, data have been collected from a network of 30 soil moisture stations distributed within the sub-catchment, according to respective fractions of classes representing mainly land cover and soil type (Bircher et al., 2012). At each station, Decagon 5TE capacitance sensors have been installed at 2.5, 22.5, and 52.5 cm depths. The sensors are logged every 30 min.
A22 HSC_SEOLMACHEON
HSC_SELMACHEON was a single station located in South Korea. Data were collected by the Hydrological Survey Center (HSC) and Water Resource and Remote Sensing Laboratory (WRRSL) and are available for the period August–September 2011.
A23 HYDROL-Net Perugia
The HYDROL-Net Perugia network (Morbidelli et al., 2014a, 2017; Flammini et al., 2018a, b; Morbidelli et al., 2014b, 2011) consists of one station (called WEEF – Water Engineering Experimental Field) located near the city of Perugia in central Italy. Measurements of soil moisture at four different depths (0.05, 0.15, 0.25, and 0.35 m), soil temperature at two depths (0.05 and 0.30 m), precipitation, and air temperature from January 2010 to December 2013 are included in the ISMN. HYDROL-Net Perugia is operated by the Department of Civil and Environmental Engineering of the University of Perugia.
A24 ICN
The former Illinois Climate Network (ICN) was operated by the Water and Atmospheric Resources Program of the Illinois State Water Survey and formerly integrated in the Global Soil Moisture Data Bank (Hollinger and Isard, 1994). Between 1983 and 2010, ICN covered 19 stations measuring soil moisture and soil temperature down to 2 m depth, as well as precipitation.
A25 IIT_KANPUR
The network IIT_KANPUR network consisted of a single station and was managed by the Hydraulics and Water Resources Laboratory at the Indian Institute of Technology Kanpur, India. Soil moisture measurements were made at four depths (10, 25, 50, and 80 cm) between from June 2011 to November 2012. The station was situated in the Ganges River basin, which is the largest river basin in India, and the soil type at the observation site is clayey silt.
A26 IMA_CAN1
The IMA_CAN1 network is operated by the Institute for Agricultural and Earthmoving Machines (IMAMOTER) of the Italian National Research Council (CNR), now part of STEMS-CNR. It is located in an experimental vineyard in Carpeneto, in the hilly Alto Monferrato region, which is a valuable vine growing and wine production area in the Piedmont region in northwestern Italy. The monitored vineyards are part of the Experimental Vine and Wine Centre of Agrion Foundation. The stations in the network provide measurements of soil moisture, precipitation, air temperature and humidity, hourly runoff, and event soil losses. Hourly volumetric soil moisture was measured by 12 Decagon 5TM sensors in the period 2011–2015, both in grassed and tilled vineyards, in correspondence with the track and no-track position, both down and up the hill (Biddoccu et al., 2016; Capello et al., 2019; Raffelli et al., 2018).
A27 IOWA
The IOWA network was located in two catchments in the southwestern part of Iowa, USA. Soil moisture observations from six stations, until 2.6 m depth, with an interval of twice a month during 1972 to 1994 (April to October) are included in the ISMN (Robock et al., 2000). This network was formerly included in the Global Soil Moisture Data Bank.
A28 IPE
The Instituto Pirenaico de Ecologia (IPE) network runs two stations located in Aragon, northeastern Spain. The stations have been collecting meteorological data with at least an hourly time resolution (air and soil temperatures, soil moisture, relative humidity, radiation, and wind speed) since 2008 in a Mediterranean oak forest (Agüero) and a semi-arid pine forest (Peñaflor). These measurements are related to dendrometer hourly records of changes in the root and stem (Agüero; see Alday et al., 2020) or stem (Peñaflor) increment of the main tree species.
A29 iRON
The interactive Roaring Fork Observation Network (iRON) is a series of 10 stations operated by the Aspen Global Change Institute spread across the elevations of the Roaring Fork Watershed, located in the Southern Rocky Mountains of the USA. This data set includes soil moisture at 5, 20, and 50 cm, soil temperature at 20 cm, and additional weather measurements variable by station. Further information can be found in Osenga et al. (2019) or at https://www.agci.org/iron/about (last access: 28 October 2021).
A30 KHOREZM
The KHOREZM network in Uzbekistan is located between the Amu Darya river and the border with Turkmenistan and was part of a project conducted by the University of Würzburg, Germany (Patrick Knöfel). There were seven stations that made soil moisture, soil temperature, air temperature, and surface temperature measurements from 2010 April to 2011 September, and these data are included in the ISMN. Although soil moisture was not observed continuously, the measurements are still a valuable contribution since no other recent observations are available in this region.
A31 KIHS_CMC
The Korea Institute of Hydrological Survey (KIHS) has been running the Cheongmicheon (CMC) network since 2009, with annually returning measurements from March till December. It comprises 56 TDR Buriable Waveguide soil moisture sensors at 18 stations located on an area of approximately 50×50 m2. All stations have a sensor installed at 0.1 m and additional sensors at varying depths, i.e. at 0.3, 0.4, 0.6, and 0.9 m.
A32 KIHS_SMC
KIHS_SMC is operated by the Environmental and Remote Sensing Lab of the Korea Institute of Hydrological Survey. The 51 soil moisture sensors (depths from 0.1 to 0.6 m) are located on a mountain slope distributed over 19 stations in close proximity to each other.
A33 LAB-net
LAB-net was created as the first soil moisture network in Chile to support remote sensing research on drought and water use conflicts (Mattar et al., 2016, 2014). The three stations measuring soil moisture, soil temperature, precipitation, and air temperature between 2014 and 2017 over various land cover types have been included in the ISMN.
A34 MAQU
The MAQU monitoring network (Su et al., 2011; Dente et al., 2012) is situated on the northeastern fringe of the Tibetan Plateau, covering an area of approximately 40×80 km, with the elevation varying from 3200 m to 4200 m a.s.l. The network provides access to soil moisture and temperature profiles (5, 10, 20, 40, and 80 cm) measured at 15 min intervals. Soil moisture and temperature data from 2008 to 2018 are included in the ISMN.
A35 METEROBS
The METEROBS (MET European Research OBServations) network measured soil moisture at a single site between October 2011 October and May 2012. It was located in the Apennines mountains in the rural area of Benevento, Southern Italy. Soil Moisture measurements from five layers until 0.5 m depth have been included in the ISMN.
A36 MOL-RAO
The MOL-RAO network is operated by the German Meteorological Service (DWD) and is part of the operational measuring programme of the MOL-RAO (Meteorological Observatory Lindenberg – Richard Aßmann Observatory). The network is situated in the northeast of Germany and consists of two stations. While the station at Falkenberg has a grass-type vegetation, Kehrigk is situated in a pine forest (Beyrich and Adam, 2007). Volumetric soil moisture, soil temperature, air temperature, and precipitation have been provided in a half-hourly resolution since 2003.
A37 MONGOLIA
Soil moisture data sets for 44 stations were collected by the National Agency of Meteorology, Hydrology, and Environment Monitoring in Ulaanbaatar. All observations were taken using the gravimetric technique and initially provided as volumetric plant-available water (in percent). Volumetric soil moisture (m3 m−3) was calculated by first extracting texture properties of all sites from the Harmonized World Soil Database and subsequently calculating the wilting levels for all stations using the equations of Saxton and Rawls (2006). Soil moisture measurements are provided 3 times a month (on the 8th, 18th, and 28th) from 1964 to 2002 during the warm period of the year, which runs from April until the end of October (e.g. Robock et al., 2000).
A38 MySMNet
The Malaysian Soil Moisture Network (MySMNet) has been operational since 2014. It deploys seven stations (four on an oil palm plantation, two on shrubland, and one on an orchard) that collect soil moisture at 5, 50, and 100 cm depth, soil temperature at 5 cm, air temperature, and relative humidity, all on an hourly basis. The soil moisture sensors used are the WaterScout SM100 (Kang et al., 2019).
A39 NAQU
The NAQU network is part of the Tibetan Plateau observatory of plateau-scale soil moisture and soil temperature (Tibet-Obs) and consists of 11 stations located in a cold semi-arid climate in Tibet at elevations over 4500 m. Soil moisture and soil temperature have been measured at five different depths (5, 10, 20, 40, and 80 cm) from 2010 onwards (Su et al., 2011).
A40 NGARI
The NGARI network is part of the Tibetan Plateau observatory of plateau-scale soil moisture and soil temperature (Tibet-Obs) and consists of 23 stations located in a cold arid climate in Tibet at elevations between 4200–4700 m. Soil moisture and soil temperature have been measured at five different depths (5, 10, 20, 40, and 80 cm) from 2010 onwards (Su et al., 2011).
A41 NVE
This is a network of monitoring stations of the Norwegian Water Resources and Energy Directorate (NVE). Soil moisture, soil temperature, and air temperature are measured. Currently, three out of eight stations are accessible through the ISMN. The stations are situated in the Trøndelag region in eastern Norway. Data are available from 2012 to 2019 at more than five different depth layers and down to 1.5 to 2 m depth.
A42 ORACLE
The ORACLE network includes six stations. The data sets reach back to the year 1985 and are available until 2013. ORACLE is a research observatory east of Paris used to study the Grand Morin and Petit Morin river catchments, particularly floods, low water periods, water quality, and the impact of human activities on the environment.
A43 OzNet
OzNet was established in 2001 with eight sites across the Murrumbidgee River catchment and a cluster of five further sites in the Adelong and Kyeamba catchments. This was further extended in 2003 to include a total of 11 sites at Kyeamba, for a GRACE validation experiment, and 13 sites at Yanco, for SMOS pre-launch algorithm development and post-launch calibration-validation. The Yanco site was further extended to have clusters of stations across 9 and 3 km grids in crop and grassland areas for SMAP algorithm development, calibration, and validation (Smith et al., 2012; Young et al., 2008).
A44 PBO_H2O
The PBO_H2O network is a former near-real time network of the ISMN hosted by the University of Colorado Boulder, USA. It consisted of 159 stations distributed in the west of the USA, the Bahamas, the Dominican Republic, Puerto Rico, Colombia, South Africa, and Saudi Arabia. Soil moisture (measured using GPS reflections), precipitation, air temperature, and snow depth from 2004 to 2017 is stored in the ISMN database (Larson et al., 2008). The network was discontinued in 2018 because of lacking financial support.
A45 PTSMN
The Patitapu Soil Moisture Network (PTSMN) was established in 2016 on the hill country landscapes of the east coast of New Zealand's North Island. PTSMN was deployed to capture spatiotemporal soil moisture trends on various topographical positions distributed over a 13.8 km2 area. The network is composed of 20 multi-sensor probes that were calibrated to the site-specific soils (Hajdu et al., 2019). The sensors provide readings at four consecutive depths down to 0.43 m.
A46 REMEDHUS
REMEDHUS is the University of Salamanca Soil Moisture Measurement Stations Network and was installed in March 1999 with a set of old TDR stations and manual measurements. It is one of the first soil moisture networks in Europe. The network was automated and upgraded with capacitance probes in 2005. The REMEDHUS data available in the ISMN cover the period since its automation. REMEDHUS is located in an agricultural area in the central part of the Duero basin (Spain). The network currently has 20 stations that measure soil moisture and soil temperature hourly in the 0–5 cm layer (González-Zamora et al., 2018).
A47 RISMA
The Real-time In-Situ Soil Monitoring for Agriculture (RISMA) network was established in 2011 by Agriculture and Agri-Food Canada at agricultural locations in Ontario, Manitoba, and Saskatchewan (Ojo et al., 2015; L'Heureux, 2011; Canisius, 2011). There are currently 23 RISMA stations collecting hourly soil moisture/temperature data at depths to 1–1.5 m in combination with meteorological data. Calibrated, quality-controlled soil moisture and weather data are provided to the ISMN on an annual basis.
A48 RSMN
The Romanian Soil Moisture Network (RSMN) consists of 19 stations homogeneously distributed over Romania. The network is managed by the Romanian National Meteorological Administration and is part of the ASSIMO project, which aims to create a framework for the evaluation of current and future satellite microwave-derived soil moisture products.
A49 Ru_CFR
The Ru_CFR network includes two stations located on the territory of the Central Forest Reserve (CFR), Tver region, Russia. Since 2015, half-hourly continuous measurements of soil moisture have been carried out. Both stations provide measurements of soil moisture at four different depths and measurements of soil temperature, air temperature, and precipitation.
A50 RUSWET-AGRO, RUSWET-GRASS, and RUSWET-VALDAI
The three historical RUSWET networks were agricultural prediction campaigns conducted by the State Hydrological Institute of the former Soviet Union within the area of present-day Russia. Measurements were taken from 1952 until 2002 and initially distributed through the Global Soil Moisture Data Bank. Altogether, the networks operated 337 sites at which soil moisture was measured 3 times per month via gravimetric sampling. At RUSWET-VALDAI, soil temperature, precipitation, and air temperature were also collected. RUSWET contributes both the northernmost (on McClintock Island) and the earliest (8 June 1952) observations to the ISMN (Robock et al., 2000).
A51 SASMAS
The Scaling and Assimilation of Soil Moisture and Streamflow (SASMAS) monitoring network commenced commissioning in late 2002, with a total of 26 stations in operation by 2003 across a 6500 km2 catchment (Rüdiger et al., 2007). Soil moisture is observed up to a depth of 90 cm (where possible) at depth intervals of 0–30, 30–60, and 60–90 cm. The network was developed as a nested catchment with three different types of scales (low-resolution across the entire catchment, medium density across to smaller subcatchment, and very high density across a 175 ha single reach). This site also hosted the second National Airborne Field Experiment (NAFE) campaign, for which additional data were collected.
A52 SCAN
The Natural Resources Conservation Service (NRCS) operates the comprehensive, USA-wide Soil Climate Analysis Network (SCAN). SCAN supports natural resource assessments and conservation activities through its network of automated climate monitoring and data collection sites. SCAN focuses primarily on agricultural areas of the USA, Puerto Rico, and the Virgin Islands. The network consists of 216 stations located across the USA and reports soil moisture, soil temperature, precipitation, temperature, and other climatic variables hourly. Soil sensors are situated at 5, 10, 20, 50, and 100 cm depths (Schaefer et al., 2007).
A53 SKKU
The SKKU network was located at an evenly and moderately vegetated botanical garden in South Korea. It was operated by Sungkyunkwan University (SKKU) from 2014 to 2016, as part of a project for evaluating Cosmic-Ray Neutron Probe (CRNP) soil moisture (Nguyen et al., 2017). The network consisted of 10 stations, and soil moisture measurements were taken at four different depths (i.e. 10, 20, 30, and 40 cm).
A54 SMOSMANIA
The SMOSMANIA network was installed in southern France by Météo-France, the French national meteorological service, in order to monitor in situ soil moisture and soil temperature in contrasting soil and climatic conditions at operational automatic weather stations (Calvet et al., 2007). The SMOSMANIA network is composed of 21 stations, forming an Atlantic–Mediterranean transect, over a large variety of mineral soils ranging from sand and clay to silt loam (Calvet et al., 2016).
A55 SNOTEL
NRCS instals, operates, and maintains an extensive, automated data collection network called SNOTEL (short for snow telemetry; Leavesley et al., 2008). SNOTEL is part of the Snow Survey and Water Supply Forecasting (SSWSF) programme and is designed to collect snowpack and related climatic data in the western USA and Alaska. The programme operates under technical guidance from the NRCS National Water and Climate Center (NWCC). With the majority of the water supply in the west arriving in the form of snow, data on snowpack provide critical information to decision-makers and water managers. SNOTEL currently consists of a network of over 860 automated SNOTEL stations, of which 463 stations have soil moisture and soil temperature sensors.
A56 SoilSCAPE
The Soil moisture Sensing Controller And oPtimal Estimator (SoilSCAPE) is a wireless soil moisture sensor network for measurements of surface-to-root-zone profiles of soil moisture (Moghaddam et al., 2011, 2016; Shuman et al., 2010). It is designed for long-term, ultra-efficient, unattended field operations. Several networks are deployed in the USA, including in the states of California, Arizona, Colorado, Alaska, and New York. Additional deployments are planned in New Mexico, USA, and in New Zealand. The SoilSCAPE architecture includes a local coordinator (LC) and multiple end devices (EDs). The LC is the central command centre of the network, receiving data from the several soil moisture and temperature sensors connected to each ED. The sensors include Decagon 5TM and Teros 12, depending on how recently they were deployed.
A57 SWEX_POLAND
The Soil Water and Energy exchange – Poland (SWEX_POLAND) network was operated between 2000 and 2013 by the Institutes of Agrophysics, Polish Academy of Sciences, in Lublin. The network consisted of six stations located in the wetlands of Poleski Park Krajobrazowy to support SMOS product calibration and validation. Soil moisture and temperature measurements were taken down to 1 m depth, along with precipitation observations (Marczewski et al., 2010).
A58 SW-WHU
The SW-WHU network was hosted by Wuhan University (Chen et al., 2015a, b). SW-WHU is a high-density network, with nearly 100 soil moisture and temperature sensors within 1 km2. It adopts a Narrowband Internet of things (NB-IoT) technique for data transmission at low power consumption. Therefore, the data of SW-WHU are particularly valuable for soil moisture validation, monitoring, and application at a very high spatiotemporal resolution (X. Zhang et al., 2018).
A59 TAHMO
The Trans-African Hydro-Meteorological Observatory (TAHMO; https://tahmo.org/, last access: 28 October 2021) presently runs a network of over 600 meteorological weather stations in more than 20 African countries. The stations measure all standard weather parameters, such as barometric pressure, wind speed, rainfall, and radiation. Each station also has five open ports that can be used for additional sensors, such as soil moisture sensors. Presently, 70 stations have soil moisture sensors, some of them at several depths. Ideally, many stations will be upgraded by soil moisture sensors in the near future.
A60 TERENO
TERENO consists of four terrestrial observatories that represent typical landscapes in Germany and central Europe and are considered to be highly vulnerable to the effects of global and climate change (Zacharias et al., 2011; Bogena et al., 2012). TERENO combines observations with comprehensive large-scale experiments, integrated modelling, remote sensing, and novel measurement technologies to increase our understanding of the functioning and feedbacks of terrestrial ecosystems (Bogena, 2016). The long-term observation platform of TERENO is composed of various measurement systems, including networks of climate and lysimeter stations, eddy covariance towers, and networks of soil, surface water, and groundwater sensors. Almost all online measurements are freely accessible via the TERENO data portal (http://www.tereno.net/ddp/, last access: 28 October 2021).
A61 UDC_SMOS
The former German UDC_SMOS network was hosted by the Department of Geography at the University of Munich, in cooperation with the Bavarian State Research Center for Agriculture, and funded by the German Aerospace Centre (DLR). It was located in grassland in the Bavarian region around Munich as an official European SMOS calibration/validation test site. In total, 11 stations provided soil moisture data from 2007 until 2011, up to 40 cm depth, as measured by several types of sensors (Loew et al., 2009; Schlenz et al., 2012a).
A62 UMBRIA
This soil moisture monitoring network in the north of the Umbria region, in the upper Tiber River basin, operates three stations in real time (Torre dell'Olmo, Petrelle, and Cerbara). Each station measures at 10, 20, and 40 cm depth. Additional stations of the network have not been operational since 2015 due to a lack of resources for their maintenance. Provided financial resources become available, the stations that are no longer functioning will be restored (Brocca et al., 2011, 2009, 2008).
A63 UMSUOL
UMSUOL (Umidita del Suolo) is a one-station network located close to Bologna, northern Italy. Soil moisture measurements at seven different depths are provided by the Agenzia Regionale Prevenione Ambiente (ARPA). The ISMN contains data from the years 2009 and 2010.
A64 USCRN
The U.S. Climate Reference Network (USCRN) contains 114 stations sparsely distributed across the contiguous USA. Each station has three sets of soil moisture/temperature probes at five depths (5, 10, 20, 50, and 100 cm), in addition to air and surface temperature, precipitation, relative humidity, and solar radiation (Bell et al., 2013). The stations were installed between 2009 and 2011 and are still in operation in 2021. The stations are maintained by the National Oceanic and Atmospheric Administration Atmospheric Turbulence and Diffusion Division (NOAA ATDD), and their data are maintained by NOAA National Centers for Environmental Information (NOAA NCEI).
A65 USDA-ARS
The U.S. Department of Agriculture Agricultural Research Service (USDA ARS) operates a number of Long-Term Agroecosystem Research (LTAR) sites, some of which have spatial coverage of soil moisture and soil temperature. As experimental sites, the locations and configuration of the stations can change depending on the current scientific questions being addressed. A description of the sites can be found in Jackson et al. (2011).
A66 VAS
The Valencia Anchor Station (VAS) network is operated by the Climatology from Satellites Group and Jucar River Basin Authority of the University of Valencia, Spain. The network is located in Spain and consists of three stations. The data sets are available for the years 2010 and 2011.
A67 VDS
The VDS network is run by VanderSat, a Dutch company that specialises in providing global satellite-observed data and services over land. The VDS network consists of four stations located near the city of Bago in Myanmar. The network was installed to validate satellite soil moisture products in the tropics. The network has two measurement periods, i.e. one from June 2017 to July 2018 and one from March 2020 onwards.
A68 WegenerNet
The WegenerNet, located in the foreland of the southeastern Austrian Alps, is a long-term weather and climate monitoring facility comprising 155 hydrometeorological stations in a dense grid, with one station every about 2 km2 (Kirchengast et al., 2014; Fuchsberger et al., 2021). Together with a range of meteorological variables, such as temperature, humidity, precipitation, and wind, it also measures soil moisture and temperature at 12 stations. These variables are measured at 0.2–0.3 m depth in diverse soil types representative for the region.
A69 WSMN
The Wales Soil Moisture Network (WSMN) was founded in July 2011. It consists of a total of nine monitoring sites located in mid-Wales representing a range of conditions typical of the Welsh environment, with climate ranging from oceanic to temperate and the most typical land use/cover types. The data set acquired in the network is composed of 0–5 (or 0–10) cm soil moisture, soil temperature, precipitation, as well as other ancillary data (Petropoulos and McCalmont, 2017).
Table E1Overview of scientific studies using ISMN data for satellite product evaluation and development.
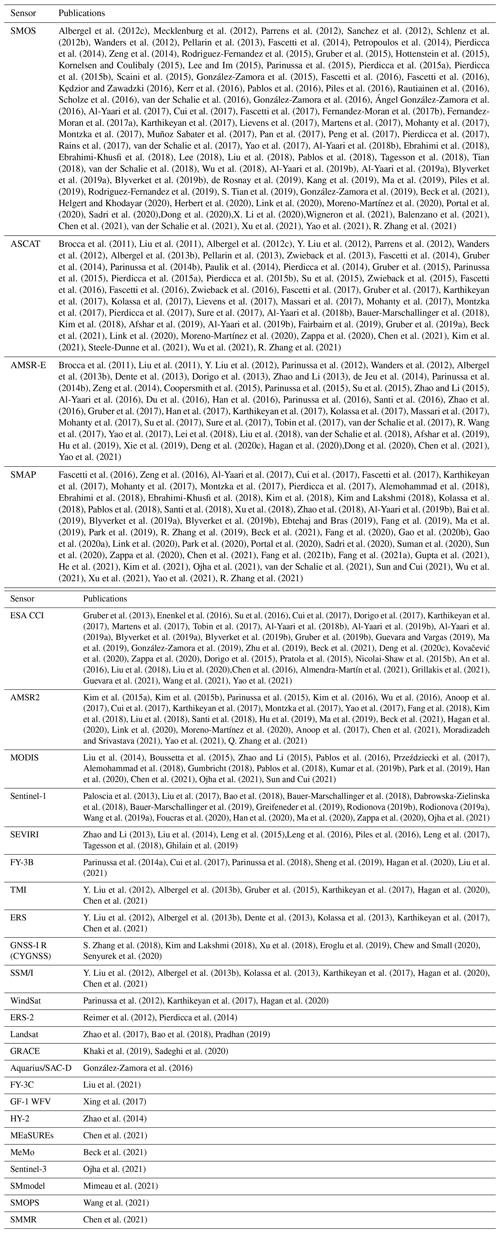
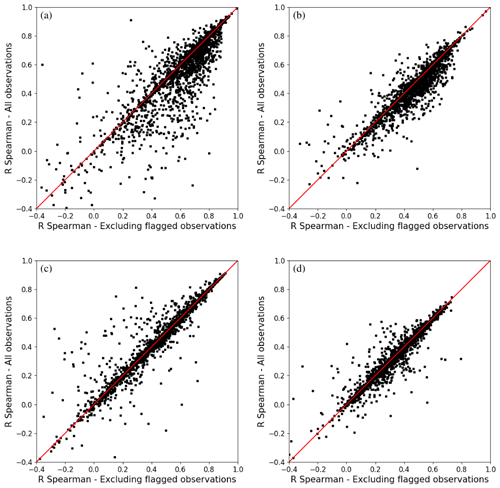
Figure F1Scatterplots of R Spearman between ISMN and ERA5 and ISMN and ESA CCI v05.2 combined for absolute values and anomalies. A 35 d moving average window was used to calculate anomalies. Each dot represents a single time series. (a) ERA5 absolute values. (b) ERA5 anomalies. (c) ESA CCI absolute values. (d) ESA CCI anomalies.
Upon registration, all data and metadata described in this paper can be downloaded for free from https://ismn.earth (ISMN, 2021). A Python package to read and plot the data and metadata (TUW-GEO/ismn: v1.1) can be accessed at https://doi.org/10.5281/zenodo.855308 (Preimesberger et al., 2021). An example of output of this package is shown in Fig. 1. A Python package containing the ISMN quality control procedures for in situ soil moisture is available on GitHub (https://github.com/TUW-GEO/flagit; Aberer et al., 2021).
WD conceived the paper and wrote it together with IH, DA, LS, IP, LZ, and WP. All other authors contributed data to the ISMN and provided feedback on the paper.
The authors declare that they have no conflict of interest.
Publisher's note: Copernicus Publications remains neutral with regard to jurisdictional claims in published maps and institutional affiliations.
The authors greatly acknowledge the financial support provided by ESA through various projects including the following: SMOSnet International Soil Moisture Network (grant no. 4000102722/10/NL/FF/fk) and Operations (grant no. 3-13185/NL/FF/fk), IDEAS+ (grant no. TVUK/AG/18/02082), QA4EO (grant no. TPZV/UK/AG/19/02321), and QA4SM (grant no. 4000135204/21//I-BG). Additional funding for the methodological advances has been received from the EU FP7 EartH2Observe project (grant no. 603608), the EU H2020 GROW project (grant no. 690199), and the QA4SM project funded by the Austrian Space Applications Programme 14 (grant no. 866004). Network funding has been provided by TWIGA (grant no. 776691). Andrej Varlagin has been supported by the Russian Foundation for Basic Research (grant no. 19-04-01234-a). Alexander Gruber also received funding (grant nos. FWO-1224320N and FWO-1530019N). We acknowledge the endorsement of various international bodies, including CEOS, WCRP GEWEX, GCOS, GTN-H, and GEO. We greatly thank all staff members from the participating networks for their continued technical support. The authors acknowledge TU Wien Bibliothek for financial support through its open-access funding programme.
The authors greatly acknowledge the financial support provided by ESA through various projects including the following: SMOSnet International Soil Moisture Network (grant no. 4000102722/10/NL/FF/fk) and Operations (grant no. 3-13185/NL/FF/fk), IDEAS+ (grant no. TVUK/AG/18/02082), QA4EO (grant no. TPZV/UK/AG/19/02321), and QA4SM (grant no. 4000135204/21//I-BG). Additional funding for the methodological advances has been received from the EU FP7 EartH2Observe project (grant no. 603608), the EU H2020 GROW project (grant no. 690199), and the QA4SM project funded by the Austrian Space Applications Programme 14 (grant no. 866004). Network funding has been provided by TWIGA (grant no. 776691). Andrej Varlagin has been supported by the Russian Foundation for Basic Research (grant no. 19-04-01234-a). Alexander Gruber also received funding (grant nos. FWO-1224320N and FWO-1530019N). TU Wien Bibliothek has provided financial support through its open-access funding programme.
This paper was edited by Thom Bogaard and reviewed by Jan Friesen and Mirko Mälicke.
Abbaszadeh, P., Moradkhani, H., and Zhan, X.: Downscaling SMAP Radiometer Soil Moisture Over the CONUS Using an Ensemble Learning Method, Water Resour. Res., 55, 324–344, https://doi.org/10.1029/2018WR023354, 2019. a
Aberer, D.: Validation of ESA CCI SM combined v05.2 vs ISMN 20191211 global – without anomalies and without ISMN flags, Zenodo [data set], https://doi.org/10.5281/zenodo.4288919, 2020a. a
Aberer, D.: Validation of ESA CCI SM combined v05.2 vs ISMN 20191211 global – without Anomalies and ISMN flagged, Zenodo [data set], https://doi.org/10.5281/zenodo.4288921, 2020b. a
Aberer, D.: Validation of ESA CCI SM combined v05.2 vs ISMN 20191211 global – Anomalies and no ISMN flags, Zenodo [data set], https://doi.org/10.5281/zenodo.4288915, 2020c. a
Aberer, D.: Validation of ESA CCI SM combined v05.2 vs ISMN 20191211 global – Anomalies and ISMN flagged, Zenodo [data set], https://doi.org/10.5281/zenodo.4288913, 2020d. a
Aberer, D., Xaver, A., and Preimesberger, W.: TUW-GEO/flagit, GitHub [code], https://github.com/TUW-GEO/flagit, last access: 8 November 2021. a
Adla, S., Rai, N. K., Karumanchi, S. H., Tripathi, S., Disse, M., and Pande, S.: Laboratory Calibration and Performance Evaluation of Low-Cost Capacitive and Very Low-Cost Resistive Soil Moisture Sensors, Sensors, 20, 363, https://doi.org/10.3390/s20020363, 2020. a
Afshar, M., Yilmaz, M., and Crow, W.: Impact of rescaling approaches in simple fusion of soil moisture products, Water Resour. Res., 55, 7804–7825, 2019. a, b, c, d
Albergel, C., Calvet, J.-C., de Rosnay, P., Balsamo, G., Wagner, W., Hasenauer, S., Naeimi, V., Martin, E., Bazile, E., Bouyssel, F., and Mahfouf, J.-F.: Cross-evaluation of modelled and remotely sensed surface soil moisture with in situ data in southwestern France, Hydrol. Earth Syst. Sci., 14, 2177–2191, https://doi.org/10.5194/hess-14-2177-2010, 2010. a, b
Albergel, C., Balsamo, G., de Rosnay, P., Muñoz Sabater, J., and Boussetta, S.: A bare ground evaporation revision in the ECMWF land-surface scheme: evaluation of its impact using ground soil moisture and satellite microwave data, Hydrol. Earth Syst. Sci., 16, 3607–3620, https://doi.org/10.5194/hess-16-3607-2012, 2012a. a, b
Albergel, C., de Rosnay, P., Balsamo, G., Isaksen, L., and Muñoz-Sabater, J.: Soil Moisture Analyses at ECMWF: Evaluation Using Global Ground-Based In Situ Observations, J. Hydrometeorol., 13, 1442–1460, https://doi.org/10.1175/JHM-D-11-0107.1, 2012b. a
Albergel, C., de Rosnay, P., Gruhier, C., Muñoz-Sabater, J., Hasenauer, S., Isaksen, L., Kerr, Y., and Wagner, W.: Evaluation of remotely sensed and modelled soil moisture products using global ground-based in situ observations, Remote Sens. Environ., 118, 215–226, https://doi.org/10.1016/j.rse.2011.11.017, 2012c. a, b, c
Albergel, C., Dorigo, W., Balsamo, G., Muñoz-Sabater, J., de Rosnay, P., Isaksen, L., Brocca, L., de Jeu, R., and Wagner, W.: Monitoring multi-decadal satellite earth observation of soil moisture products through land surface reanalyses, Remote Sens. Environ., 138, 77–89, https://doi.org/10.1016/j.rse.2013.07.009, 2013a. a
Albergel, C., Dorigo, W., Reichle, R. H., Balsamo, G., de Rosnay, P., Muñoz-Sabater, J., Isaksen, L., de Jeu, R., and Wagner, W.: Skill and Global Trend Analysis of Soil Moisture from Reanalyses and Microwave Remote Sensing, J. Hydrometeorol., 14, 1259–1277, https://doi.org/10.1175/JHM-D-12-0161.1, 2013b. a, b, c, d, e, f, g
Albergel, C., Dutra, E., Muñoz-Sabater, J., Haiden, T., Balsamo, G., Beljaars, A., Isaksen, L., de Rosnay, P., Sandu, I., and Wedi, N.: Soil temperature at ECMWF: An assessment using ground-based observations, J. Geophys. Res.-Atmos., 120, 1361–1373, https://doi.org/10.1002/2014JD022505, 2015. a, b, c, d
Albergel, C., Munier, S., Bocher, A., Bonan, B., Zheng, Y., Draper, C., Leroux, D., and Calvet, J.-C.: LDAS-Monde Sequential Assimilation of Satellite Derived Observations Applied to the Contiguous US: An ERA-5 Driven Reanalysis of the Land Surface Variables, Remote Sens., 10, 1627, https://doi.org/10.3390/rs10101627, 2018. a
Albergel, C., Zheng, Y., Bonan, B., Dutra, E., Rodríguez-Fernández, N., Munier, S., Draper, C., de Rosnay, P., Muñoz Sabater, J., Balsamo, G., Fairbairn, D., Meurey, C., and Calvet, J.-C.: Data assimilation for continuous global assessment of severe conditions over terrestrial surfaces, Hydrol. Earth Syst. Sci., 24, 4291–4316, https://doi.org/10.5194/hess-24-4291-2020, 2020. a, b
Alday, J. G., Camarero, J. J., Revilla, J., and Dios, V. R.: Similar diurnal, seasonal and annual rhythms in radial root expansion across two coexisting Mediterranean oak species, Tree Physiol., 40, 956–968, 2020. a
Alemohammad, S. H., Kolassa, J., Prigent, C., Aires, F., and Gentine, P.: Global downscaling of remotely sensed soil moisture using neural networks, Hydrol. Earth Syst. Sci., 22, 5341–5356, https://doi.org/10.5194/hess-22-5341-2018, 2018. a, b
Almendra-Martín, L., Martínez-Fernández, J., Piles, M., and González-Zamora, Á.: Comparison of gap-filling techniques applied to the CCI soil moisture database in Southern Europe, Remote Sens. Environ., 258, 112377, https://doi.org/10.1016/j.rse.2021.112377, 2021. a
Al-Yaari, A., Wigneron, J., Kerr, Y., de Jeu, R., Rodriguez-Fernandez, N., van der Schalie, R., Al Bitar, A., Mialon, A., Richaume, P., Dolman, A., and Ducharne, A.: Testing regression equations to derive long-term global soil moisture datasets from passive microwave observations, Remote Sens. Environ., 180, 453–464, https://doi.org/10.1016/j.rse.2015.11.022, 2016. a
Al-Yaari, A., Wigneron, J.-P., Kerr, Y., Rodriguez-Fernandez, N., O'Neill, P., Jackson, T., De Lannoy, G., Al Bitar, A., Mialon, A., Richaume, P., Walker, J., Mahmoodi, A., and Yueh, S.: Evaluating soil moisture retrievals from ESA's SMOS and NASA's SMAP brightness temperature datasets, Remote Sens. Environ., 193, 257–273, https://doi.org/10.1016/j.rse.2017.03.010, 2017. a, b
Al-Yaari, A., Dayau, S., Chipeaux, C., Aluome, C., Kruszewski, A., Loustau, D., and Wigneron, J.-P.: The AQUI Soil Moisture Network for Satellite Microwave Remote Sensing Validation in South-Western France, Remote Sens., 10, 1105, https://doi.org/10.3390/rs10111839, 2018a. a
Al-Yaari, A., Dayau, S., Chipeaux, C., Aluome, C., Kruszewski, A., Loustau, D., and Wigneron, J.-P.: The AQUI Soil Moisture Network for Satellite Microwave Remote Sensing Validation in South-Western France, Remote Sens., 10, 1839, https://doi.org/10.3390/rs10111839, 2018b. a, b, c
Al-Yaari, A., Ducharne, A., Cheruy, F., Crow, W. T., and Wigneron, J.-P.: Satellite-based soil moisture provides missing link between summertime precipitation and surface temperature biases in CMIP5 simulations over conterminous United States, Scient. Rep., 9, 1657, https://doi.org/10.1038/s41598-018-38309-5, 2019a. a, b
Al-Yaari, A., Wigneron, J.-P., Dorigo, W., Colliander, A., Pellarin, T., Hahn, S., Mialon, A., Richaume, P., Fernandez-Moran, R., Fan, L., Kerr, Y., and Lannoy, G. D.: Assessment and inter-comparison of recently developed/reprocessed microwave satellite soil moisture products using ISMN ground-based measurements, Remote Sens. Environ., 224, 289–303, https://doi.org/10.1016/j.rse.2019.02.008, 2019b. a, b, c, d, e
An, R., Zhang, L., Wang, Z., Quaye-Ballard, J. A., You, J., Shen, X., Gao, W., Huang, L., Zhao, Y., and Ke, Z.: Validation of the ESA CCI soil moisture product in China, Int. J. Appl. Earth Obs. Geoinf., 48, 28–36, https://doi.org/10.1016/j.jag.2015.09.009, 2016. a
Ángel González-Zamora, Sánchez, N., Martínez-Fernández, J., and Wagner, W.: Root-zone plant available water estimation using the SMOS-derived soil water index, Adv. Water Resour., 96, 339–353, https://doi.org/10.1016/j.advwatres.2016.08.001, 2016. a
Angevine, W. M., Bazile, E., Legain, D., and Pino, D.: Land surface spinup for episodic modeling, Atmos. Chem. Phys., 14, 8165–8172, https://doi.org/10.5194/acp-14-8165-2014, 2014. a, b, c, d
Anoop, S., Maurya, D. K., Rao, P. V. N., and Sekhar, M.: Validation and Comparison of LPRM Retrieved Soil Moisture Using AMSR2 Brightness Temperature at Two Spatial Resolutions in the Indian Region, IEEE Geosci. Remote Sens. Lett., 14, 1561–1564, https://doi.org/10.1109/LGRS.2017.2722542, 2017. a, b
Ardö, J.: A 10-Year Dataset of Basic Meteorology and Soil Properties in Central Sudan, Dataset Papers in Geosciences [data set], https://doi.org/10.7167/2013/297973/dataset, 2013. a
Bai, L., Lv, X., and Li, X.: Evaluation of Two SMAP Soil Moisture Retrievals Using Modeled- and Ground-Based Measurements, Remote Sens., 11, 2891, https://doi.org/10.3390/rs11242891, 2019. a
Baldocchi, D., Falge, E., Gu, L., Olson, R., Hollinger, D., Running, S., Anthoni, P., Bernhofer, C., Davis, K., Evans, R., Fuentes, J., Goldstein, A., Katul, G., Law, B., Lee, X., Malhi, Y., Meyers, T., Munger, J., Oechel, W., and Richardson, F.: FLUXNET: A New Tool to Study the Temporal and Spatial Variability of Ecosystem–Scale Carbon Dioxide, Water Vapor, and Energy Flux Densities, B. Am. Meteorol. Soc., 82, 2415–2434, https://doi.org/10.1175/1520-0477(2001)082<2415:FANTTS>2.3.CO;2, 2001. a
Balenzano, A., Mattia, F., Satalino, G., Lovergine, F. P., Palmisano, D., Peng, J., Marzahn, P., Wegmüller, U., Cartus, O., Da̧browska-Zielińska, K., Musial, J. P., Davidson, M. W., Pauwels, V. R., Cosh, M. H., McNairn, H., Johnson, J. T., Walker, J. P., Yueh, S. H., Entekhabi, D., Kerr, Y. H., and Jackson, T. J.: Sentinel-1 soil moisture at 1 km resolution: a validation study, Remote Sens. Environ., 263, 112554, https://doi.org/10.1016/j.rse.2021.112554, 2021. a
Balsamo, G., Albergel, C., Beljaars, A., Boussetta, S., Brun, E., Cloke, H., Dee, D., Dutra, E., Muñoz Sabater, J., Pappenberger, F., de Rosnay, P., Stockdale, T., and Vitart, F.: ERA-Interim/Land: a global land surface reanalysis data set, Hydrol. Earth Syst. Sci., 19, 389–407, https://doi.org/10.5194/hess-19-389-2015, 2015. a, b
Bao, Y., Lin, L., Wu, S., Deng, K. A. K., and Petropoulos, G. P.: Surface soil moisture retrievals over partially vegetated areas from the synergy of Sentinel-1 and Landsat 8 data using a modified water-cloud model, Int. J. Appl. Earth Obs. Geoinf., 72, 76–85, https://doi.org/10.1016/j.jag.2018.05.026, 2018. a, b
Barbu, A. L., Calvet, J.-C., Mahfouf, J.-F., and Lafont, S.: Integrating ASCAT surface soil moisture and GEOV1 leaf area index into the SURFEX modelling platform: a land data assimilation application over France, Hydrol. Earth Syst. Sci., 18, 173–192, https://doi.org/10.5194/hess-18-173-2014, 2014. a
Bauer-Marschallinger, B., Paulik, C., Hochstöger, S., Mistelbauer, T., Modanesi, S., Ciabatta, L., Massari, C., Brocca, L., and Wagner, W.: Soil Moisture from Fusion of Scatterometer and SAR: Closing the Scale Gap with Temporal Filtering, Remote Sens., 10, 1030, https://doi.org/10.3390/rs10071030, 2018. a, b, c, d
Bauer-Marschallinger, B., Freeman, V., Cao, S., Paulik, C., Schaufler, S., Stachl, T., Modanesi, S., Massari, C., Ciabatta, L., Brocca, L., and Wagner, W.: Toward Global Soil Moisture Monitoring With Sentinel-1: Harnessing Assets and Overcoming Obstacles, IEEE T. Geosci. Remote, 57, 520–539, https://doi.org/10.1109/TGRS.2018.2858004, 2019. a, b
Bayat, B., Camacho, F., Nickeson, J., Cosh, M., Bolten, J., Vereecken, H., and Montzka, C.: Toward operational validation systems for global satellite-based terrestrial essential climate variables, Int. J. Appl. Earth Obs. Geoinf., 95, 102240, https://doi.org/10.1016/j.jag.2020.102240, 2021. a, b
Beck, H. E., Pan, M., Miralles, D. G., Reichle, R. H., Dorigo, W. A., Hahn, S., Sheffield, J., Karthikeyan, L., Balsamo, G., Parinussa, R. M., van Dijk, A. I. J. M., Du, J., Kimball, J. S., Vergopolan, N., and Wood, E. F.: Evaluation of 18 satellite- and model-based soil moisture products using in situ measurements from 826 sensors, Hydrol. Earth Syst. Sci., 25, 17–40, https://doi.org/10.5194/hess-25-17-2021, 2021. a, b, c, d, e, f, g, h, i, j, k, l, m, n
Bell, J., Palecki, M., Baker, B., Collins, W., Lawrimore, J., Leeper, R., Hall, M., Kochendorfer, J., Meyers, T., Wilson, T., and Diamond, H.: U.S. Climate Reference Network Soil Moisture and Temperature Observations, J. Hydrometeorol., 14, 977–988, https://doi.org/10.1175/JHM-D-12-0146.1, 2013. a
Beyrich, F. and Adam, W.: Site and Data Report for the Lindenberg Reference Site in CEOP – Phase 1, 230, Berichte des Deutschen Wetterdienstes, Offenbach am Main, 2007. a
Bi, H., Ma, J., Zheng, W., and Zeng, J.: Comparison of soil moisture in GLDAS model simulations and in situ observations over the Tibetan Plateau, J. Geophys. Res.-Atmos., 121, 2658–2678, https://doi.org/10.1002/2015JD024131, 2016. a, b, c, d
Biddoccu, M., Ferraris, S., Opsi, F., and Cavallo, E.: Long-term monitoring of soil management effects on runoff and soil erosion in sloping vineyards in Alto Monferrato (North West Italy), Soil Till. Res., 155, 176–189, https://doi.org/10.1016/j.still.2015.07.005, 2016. a
Bircher, S., Skou, N., Jensen, K. H., Walker, J. P., and Rasmussen, L.: A soil moisture and temperature network for SMOS validation in Western Denmark, Hydrol. Earth Syst. Sci., 16, 1445–1463, https://doi.org/10.5194/hess-16-1445-2012, 2012. a, b
Blöschl, G., Blaschke, A. P., Broer, M., Bucher, C., Carr, G., Chen, X., Eder, A., Exner-Kittridge, M., Farnleitner, A., Flores-Orozco, A., Haas, P., Hogan, P., Kazemi Amiri, A., Oismüller, M., Parajka, J., Silasari, R., Stadler, P., Strauss, P., Vreugdenhil, M., Wagner, W., and Zessner, M.: The Hydrological Open Air Laboratory (HOAL) in Petzenkirchen: a hypothesis-driven observatory, Hydrol. Earth Syst. Sci., 20, 227–255, https://doi.org/10.5194/hess-20-227-2016, 2016. a
Blyverket, J., Hamer, P., Bertino, L., Albergel, C., Fairbairn, D., and Lahoz, W.: An Evaluation of the EnKF vs. EnOI and the Assimilation of SMAP, SMOS and ESA CCI Soil Moisture Data over the Contiguous US, Remote Sens., 11, 478, https://doi.org/10.3390/rs11050478, 2019a. a, b, c, d
Blyverket, J., Hamer, P., Bertino, L., Albergel, C., Fairbairn, D., and Lahoz, W.: An Evaluation of the EnKF vs. EnOI and the Assimilation of SMAP, SMOS and ESA CCI Soil Moisture Data over the Contiguous US, Remote Sens., 11, 012046, https://doi.org/10.3390/rs11050478, 2019b. a, b, c
Bogena, H., Huisman, J., Oberdörster, C., and Vereecken, H.: Evaluation of a low-cost soil water content sensor for wireless network applications, J. Hydrol., 344, 32–42, https://doi.org/10.1016/j.jhydrol.2007.06.032, 2007. a
Bogena, H., Kunkel, R., Pütz, T., Vereecken, H., Kruger, E., Zacharias, S., Dietrich, P., Wollschläger, U., Kunstmann, H., Papen, H., Schmid, H., Munch, J., Priesack, E., Schwank, M., Bens, O., Brauer, A., Borg, E., and Hajnsek, I.: TERENO – Long-term monitoring network for terrestrial environmental research, Hydrol. Wasserbewirt., 56, 138–143, 2012. a
Bogena, H. R.: TERENO: German network of terrestrial environmental observatories, J. Large-scale Res. Facil., 2, A52, https://doi.org/10.17815/jlsrf-2-98, 2016. a
Bogena, H. R., Huisman, J. A., Güntner, A., Hübner, C., Kusche, J., Jonard, F., Vey, S., and Vereecken, H.: Emerging methods for noninvasive sensing of soil moisture dynamics from field to catchment scale: a review: Emerging methods for noninvasive sensing of soil moisture dynamics, Wiley Interdisciplin. Rev.: Water, 2, 635–647, https://doi.org/10.1002/wat2.1097, 2015. a
Bonney, R., Cooper, C. B., Dickinson, J., Kelling, S., Phillips, T., Rosenberg, K. V., and Shirk, J.: Citizen Science: A Developing Tool for Expanding Science Knowledge and Scientific Literacy, BioScience, 59, 977–984, https://doi.org/10.1525/bio.2009.59.11.9, 2009. a
Boussetta, S., Balsamo, G., Dutra, E., Beljaars, A., and Albergel, C.: Assimilation of surface albedo and vegetation states from satellite observations and their impact on numerical weather prediction, Remote Sens. Environ., 163, 111–126, https://doi.org/10.1016/j.rse.2015.03.009, 2015. a, b
Brocca, L., Morbidelli, R., Melone, F., and Moramarco, T.: Soil moisture spatial variability in experimental areas of central Italy, J. Hydrol., 333, 356–373, https://doi.org/10.1016/j.jhydrol.2006.09.004, 2007. a
Brocca, L., Melone, F., and Moramarco, T.: On the estimation of antecedent wetness condition in rainfall-runoff modeling, Hydrol. Process., 22, 629–642, https://doi.org/10.1002/hyp.6629, 2008. a
Brocca, L., Melone, F., Moramarco, T., and Morbidelli, R.: Antecedent wetness conditions based on ERS scatterometer data, J. Hydrol., 364, 73–87, 2009. a
Brocca, L., Hasenauer, S., Lacava, T., Melone, F., Moramarco, T., Wagner, W. A. D., Matgen, P., Martínez-Fernández, J., Llorens, P., Latron, J., Martin, C., and Bittelli, M.: Soil moisture estimation through ASCAT and AMSR-E sensors: An intercomparison and validation study across Europe, Remote Sens. Environ., 115, 3390–3408, https://doi.org/10.1016/j.rse.2011.08.003, 2011. a, b, c, d, e
Brocca, L., Ciabatta, L., Massari, C., Moramarco, T., Hahn, S., Hasenauer, S., Kidd, R., Dorigo, W., Wagner, W., and Levizzani, V.: Soil as a natural rain gauge: Estimating global rainfall from satellite soil moisture data, J. Geophys. Res.-Atmos., 119, 5128–5141, https://doi.org/10.1002/2014jd021489, 2014a. a
Brocca, L., Zucco, G., Mittelbach, H., Moramarco, T., and Seneviratne, S. I.: Absolute versus temporal anomaly and percent of saturation soil moisture spatial variability for six networks worldwide, Water Resour. Res., 50, 5560–5576, https://doi.org/10.1002/2014WR015684, 2014b. a
Brocca, L., Massari, C., Ciabatta, L., Moramarco, T., Penna, D., Zuecco, G., Pianezzola, L., Borga, M., Matgen, P., and Martínez-Fernández, J.: Rainfall estimation from in situ soil moisture observations at several sites in Europe: an evaluation of the SM2RAIN algorithm, J. Hydrol. Hydromech., 63, 201–209, https://doi.org/10.1515/johh-2015-0016, 2015. a
Brocca, L., Ciabatta, L., Massari, C., Camici, S., and Tarpanelli, A.: Soil Moisture for Hydrological Applications: Open Questions and New Opportunities, Water, 9, 140, https://doi.org/10.3390/w9020140, 2017. a
Calvet, J.-C., Fritz, N., Froissard, F., Suquia, D., Petitpa, A., and Piguet, B.: In situ soil moisture observations for the CAL/VAL of SMOS: the SMOSMANIA network, in: 2007 IEEE International Geoscience and Remote Sensing Symposium, 1196–1199, https://doi.org/10.1109/IGARSS.2007.4423019, 2007. a
Calvet, J.-C., Fritz, N., Berne, C., Piguet, B., Maurel, W., and Meurey, C.: Deriving pedotransfer functions for soil quartz fraction in southern France from reverse modeling, SOIL, 2, 615–629, https://doi.org/10.5194/soil-2-615-2016, 2016. a, b
Cammalleri, C., Micale, F., and Vogt, J.: On the value of combining different modelled soil moisture products for European drought monitoring, J. Hydrol., 525, 547–558, https://doi.org/10.1016/j.jhydrol.2015.04.021, 2015. a, b, c, d, e
Campo, L., Castelli, F., Caparrini, F., and Entekhabi, D.: Production of a 5-years Dataset of Soil Moisture Maps on Italian Territory with an Operational Algorithm, Eur. Water, 35, 45–56, 2011. a
Canisius, F.: Calibration of Casselman, Ontario Soil Moisture Monitoring Network, Agriculture and Agri-Food, Ottawa, ON, Canada, 37 pp., 2011. a
Capello, G., Biddoccu, M., Ferraris, S., and Cavallo, E.: Effects of Tractor Passes on Hydrological and Soil Erosion Processes in Tilled and Grassed Vineyards, Water, 11, 2118, https://doi.org/10.3390/w11102118, 2019. a
Castell, N., Dauge, F. R., Schneider, P., Vogt, M., Lerner, U., Fishbain, B., Broday, D., and Bartonova, A.: Can commercial low-cost sensor platforms contribute to air quality monitoring and exposure estimates?, Environ. Int., 99, 293–302, https://doi.org/10.1016/j.envint.2016.12.007, 2017. a
Chawla, S., Bachhtey, S., Gupta, V., Sharma, S., Seth, S., Gandhi, T., Varshney, S., Mehta, S., and Jha, R.: Low Cost Soil Moisture Sensors and their Application in Automatic Irrigation System, p. 4, available at: https://www.researchgate.net/publication/308742451_Low_Cost_Soil_Moisture_Sensors_and_their_Application_in_Automatic_Irrigation_System (last access: 28 October 2021), 2019. a
Chen, F., Crow, W. T., Colliander, A., Cosh, M. H., Jackson, T. J., Bindlish, R., Reichle, R. H., Chan, S. K., Bosch, D. D., Starks, P. J., Goodrich, D. C., and Seyfried, M. S.: Application of Triple Collocation in Ground-Based Validation of Soil Moisture Active/Passive (SMAP) Level 2 Data Products, IEEE J. Select. Top. Appl. Earth Obs. Remote Sens., 10, 489–502, https://doi.org/10.1109/JSTARS.2016.2569998, 2017. a
Chen, N., Xiang, Z., and Wang, C.: Integrated open geospatial web service enabled cyber-physical information infrastructure for precision agriculture monitoring, Comput. Electron. Agricult., 111, 78–91, https://doi.org/10.1016/j.compag.2014.12.009, 2015a. a
Chen, N., Xiao, C., Pu, F., Wang, X., Wang, C., Wang, Z., and Gong, J.: Cyber-Physical Geographical Information Service-Enabled Control of Diverse In-Situ Sensors, Sensors (Basel, Switzerland), 15, 2565–2592, https://doi.org/10.3390/s150202565, 2015b. a
Chen, X., Su, Y., Liao, J., Shang, J., Dong, T., Wang, C., Liu, W., Zhou, G., and Liu, L.: Detecting significant decreasing trends of land surface soil moisture in eastern China during the past three decades (1979–2010), J. Geophys. Res.-Atmos., 121, 5177–5192, https://doi.org/10.1002/2015JD024676, 2016. a
Chen, Y., Sun, L., Wang, W., and Pei, Z.: Application of Sentinel 2 data for drought monitoring in Texas, America, in: 2019 8th International Conference on Agro-Geoinformatics (Agro-Geoinformatics), 1–4, https://doi.org/10.1109/Agro-Geoinformatics.2019.8820491, 2019. a
Chen, Y., Feng, X., and Fu, B.: An improved global remote-sensing-based surface soil moisture (RSSSM) dataset covering 2003–2018, Earth Syst. Sci. Data, 13, –31, https://doi.org/10.5194/essd-13-1-2021, 2021. a, b, c, d, e, f, g, h, i, j, k
Chew, C. and Small, E.: Estimating inundation extent using CYGNSS data: A conceptual modeling study, Remote Sens. Environ., 246, 111869, https://doi.org/10.1016/j.rse.2020.111869, 2020. a, b
Colliander, A., Jackson, T., Bindlish, R., Chan, S., Das, N., Kim, S., Cosh, M., Dunbar, R., Dang, L., Pashaian, L., Asanuma, J., Aida, K., Berg, A., Rowlandson, T., Bosch, D., Caldwell, T., Caylor, K., Goodrich, D., al Jassar, H., Lopez-Baeza, E., Martínez-Fernández, J., González-Zamora, A., Livingston, S., McNairn, H., Pacheco, A., Moghaddam, M., Montzka, C., Notarnicola, C., Niedrist, G., Pellarin, T., Prueger, J., Pulliainen, J., Rautiainen, K., Ramos, J., Seyfried, M., Starks, P., Su, Z., Zeng, Y., van der Velde, R., Thibeault, M., Dorigo, W., Vreugdenhil, M., Walker, J., Wu, X., Monerris, A., O'Neill, P., Entekhabi, D., Njoku, E., and Yueh, S.: Validation of SMAP surface soil moisture products with core validation sites, Remote Sens. Environ., 191, 215–231, https://doi.org/10.1016/j.rse.2017.01.021, 2017. a, b, c, d, e
Cook, D. R.: Soil temperature and moisture profile (STAMP) system handbook, Tech. rep., DOE Office of Science Atmospheric Radiation Measurement (ARM) Program, available at: https://www.osti.gov/biblio/1332724 (last access: 1 November 2021), 2016a. a
Cook, D. R. and Sullivan, R. C.: Surface Energy Balance System (SEBS) Instrument Handbook, Tech. rep., DOE Office of Science Atmospheric Radiation Measurement (ARM) Program, available at: https://www.osti.gov/biblio/1004944 (last access: 1 November 2021), 2018. a
Coopersmith, E. J., Cosh, M. H., Bindlish, R., and Bell, J.: Comparing AMSR-E soil moisture estimates to the extended record of the U.S. Climate Reference Network (USCRN), Adv. Water Resour., 85, 79–85, https://doi.org/10.1016/j.advwatres.2015.09.003, 2015. a
Crow, W. T., Berg, A. A., Cosh, M. H., Loew, A., Mohanty, B. P., Panciera, R., de Rosnay, P., Ryu, D., and Walker, J. P.: Upscaling sparse ground-based soil moisture observations for the validation of coarse-resolution satellite soil moisture products: Upscaling Soil Moisture, Rev. Geophys., 50, RG2002, https://doi.org/10.1029/2011RG000372, 2012. a, b
Cui, C., Xu, J., Zeng, J., Chen, K.-S., Bai, X., Lu, H., Chen, Q., and Zhao, T.: Soil Moisture Mapping from Satellites: An Intercomparison of SMAP, SMOS, FY3B, AMSR2, and ESA CCI over Two Dense Network Regions at Different Spatial Scales, Remote Sens., 10, 33, https://doi.org/10.3390/rs10010033, 2017. a, b, c, d, e
Dabrowska-Zielinska, K., Musial, J., Malinska, A., Budzynska, M., Gurdak, R., Kiryla, W., Bartold, M., and Grzybowski, P.: Soil Moisture in the Biebrza Wetlands Retrieved from Sentinel-1 Imagery, Remote Sens., 10, 1979, https://doi.org/10.3390/rs10121979, 2018. a
de Jeu, R. and Dorigo, W.: On the importance of satellite observed soil moisture, Int. J. Appl. Earth Obs. Geoinf., 45, 107–109, https://doi.org/10.1016/j.jag.2015.10.007, 2016. a
de Jeu, R. A., Holmes, T. R., Parinussa, R. M., and Owe, M.: A spatially coherent global soil moisture product with improved temporal resolution, J. Hydrol., 516, 284–296, https://doi.org/10.1016/j.jhydrol.2014.02.015, 2014. a
Deng, Y., Wang, S., Bai, X., Luo, G., Wu, L., Chen, F., Wang, J., Li, C., Yang, Y., Hu, Z., Tian, S., and Lu, Q.: Vegetation greening intensified soil drying in some semi-arid and arid areas of the world, Agr. Forest Meteorol., 292–293, 108103, https://doi.org/10.1016/j.agrformet.2020.108103, 2020a. a
Deng, Y., Wang, S., Bai, X., Luo, G., Wu, L., Chen, F., Wang, J., Li, Q., Li, C., Yang, Y., Hu, Z., and Tian, S.: Spatiotemporal dynamics of soil moisture in the karst areas of China based on reanalysis and observations data, J. Hydrol., 585, 124744, https://doi.org/10.1016/j.jhydrol.2020.124744, 2020b. a
Deng, Y., Wang, S., Bai, X., Wu, L., Cao, Y., Li, H., Wang, M., Li, C., Yang, Y., Hu, Z., Tian, S., and Lu, Q.: Comparison of soil moisture products from microwave remote sensing, land model, and reanalysis using global ground observations, Hydrol. Process., 34, 836–851, https://doi.org/10.1002/hyp.13636, 2020c. a, b, c, d
Dente, L., Su, Z., and Wen, J.: Validation of SMOS soil moisture products over the Maqu and Twente regions, Sensors, 12, 9965–9986, 2012. a
Dente, L., Vekerdy, Z., de Jeu, R., and Su, Z.: Seasonality and autocorrelation of satellite-derived soil moisture products, Int. J. Remote Sens., 34, 3231–3247, https://doi.org/10.1080/01431161.2012.716923, 2013. a, b
de Rosnay, P., Muñoz, J., Sabater, C. A., Lawrence, H., Isaksen, L., and English, S.: ECMWF Final Report on SMOS brightness temperature activities over land: Monitoring and Data Assimilation, ESA Contract Report, available at: https://www.ecmwf.int/node/19531 (last access: 8 November 2021), 2019. a, b
Dirmeyer, P. A., Wu, J., Norton, H. E., Dorigo, W. A., Quiring, S. M., Ford, T. W., Santanello, J. A., Bosilovich, M. G., Ek, M. B., Koster, R. D., Balsamo, G., and Lawrence, D. M.: Confronting Weather and Climate Models with Observational Data from Soil Moisture Networks over the United States, J. Hydrometeorol., 17, 1049–1067, https://doi.org/10.1175/JHM-D-15-0196.1, 2016. a, b, c, d, e
Domínguez-Niño, J. M., Bogena, H. R., Huisman, J. A., Schilling, B., and Casadesús, J.: On the Accuracy of Factory-Calibrated Low-Cost Soil Water Content Sensors, Sensors, 19, 3101, https://doi.org/10.3390/s19143101, 2019. a
Dong, J., Crow, W. T., Tobin, K. J., Cosh, M. H., Bosch, D. D., Starks, P. J., Seyfried, M., and Collins, C. H.: Comparison of microwave remote sensing and land surface modeling for surface soil moisture climatology estimation, Remote Sens. Environ., 242, 111756, https://doi.org/10.1016/j.rse.2020.111756, 2020. a, b, c, d, e, f, g, h
Dorigo, W., van Oevelen, P., Wagner, W., Drusch, M., Mecklenburg, S., Robock, A., and Jackson, T.: A New International Network for in Situ Soil Moisture Data, EOS Trans. Am. Geophys. Union, 92, 141–142, https://doi.org/10.1029/2011EO170001, 2011a. a, b
Dorigo, W. A., Wagner, W., Hohensinn, R., Hahn, S., Paulik, C., Xaver, A., Gruber, A., Drusch, M., Mecklenburg, S., van Oevelen, P., Robock, A., and Jackson, T.: The International Soil Moisture Network: a data hosting facility for global in situ soil moisture measurements, Hydrol. Earth Syst. Sci., 15, 1675–1698, https://doi.org/10.5194/hess-15-1675-2011, 2011b. a, b, c, d, e, f, g, h, i
Dorigo, W., Xaver, A., Vreugdenhil, M., Gruber, A., Dostálová, A., Sanchis-Dufau, A. D., Zamojski, D., Cordes, C., Wagner, W., and Drusch, M.: Global Automated Quality Control of In Situ Soil Moisture Data from the International Soil Moisture Network, Vadose Zone J., 12, vzj2012.0097, https://doi.org/10.2136/vzj2012.0097, 2013. a, b, c, d, e, f, g, h
Dorigo, W., Gruber, A., Jeu, R. D., Wagner, W., Stacke, T., Loew, A., Albergel, C., Brocca, L., Chung, D., Parinussa, R., and Kidd, R.: Evaluation of the ESA CCI soil moisture product using ground-based observations, Remote Sens. Environ., 162, 380–395, https://doi.org/10.1016/j.rse.2014.07.023, 2015. a, b, c, d
Dorigo, W., Wagner, W., Albergel, C., Albrecht, F., Balsamo, G., Brocca, L., Chung, D., Ertl, M., Forkel, M., Gruber, A., Haas, E., Hamer, P. D., Hirschi, M., Ikonen, J., de Jeu, R., Kidd, R., Lahoz, W., Liu, Y. Y., Miralles, D., Mistelbauer, T., Nicolai-Shaw, N., Parinussa, R., Pratola, C., Reimer, C., van der Schalie, R., Seneviratne, S. I., Smolander, T., and Lecomte, P.: ESA CCI Soil Moisture for improved Earth system understanding: State-of-the art and future directions, Remote Sens. Environ., 203, 185–215, https://doi.org/10.1016/j.rse.2017.07.001, 2017. a, b, c, d, e
Dorigo, W. A., Scipal, K., Parinussa, R. M., Liu, Y. Y., Wagner, W., de Jeu, R. A. M., and Naeimi, V.: Error characterisation of global active and passive microwave soil moisture datasets, Hydrol. Earth Syst. Sci., 14, 2605–2616, https://doi.org/10.5194/hess-14-2605-2010, 2010. a
Draper, C. and Reichle, R. H.: Assimilation of Satellite Soil Moisture for Improved Atmospheric Reanalyses, Mon. Weather Rev., 147, 2163–2188, https://doi.org/10.1175/MWR-D-18-0393.1, 2019. a
Du, J., Kimball, J. S., and Jones, L. A.: Passive Microwave Remote Sensing of Soil Moisture Based on Dynamic Vegetation Scattering Properties for AMSR-E, IEEE T. Geosci. Remote, 54, 597–608, 2016. a
Ebrahimi, M., Alavipanah, S. K., Hamzeh, S., Amiraslani, F., Neysani Samany, N., and Wigneron, J.-P.: Exploiting the synergy between SMAP and SMOS to improve brightness temperature simulations and soil moisture retrievals in arid regions, J. Hydrol., 557, 740–752, https://doi.org/10.1016/j.jhydrol.2017.12.051, 2018. a, b
Ebrahimi-Khusfi, M., Alavipanah, S. K., Hamzeh, S., Amiraslani, F., Neysani Samany, N., and Wigneron, J.-P.: Comparison of soil moisture retrieval algorithms based on the synergy between SMAP and SMOS-IC, Int. J. Appl. Earth Obs. Geoinf., 67, 148–160, https://doi.org/10.1016/j.jag.2017.12.005, 2018. a, b
Ebtehaj, A. and Bras, R. L.: A physically constrained inversion for high-resolution passive microwave retrieval of soil moisture and vegetation water content in L-band, Remote Sens. Environ., 233, 111346, https://doi.org/10.1016/j.rse.2019.111346, 2019. a
Enenkel, M., Reimer, C., Dorigo, W., Wagner, W., Pfeil, I., Parinussa, R., and De Jeu, R.: Combining satellite observations to develop a global soil moisture product for near-real-time applications, Hydrol. Earth Syst. Sci., 20, 4191–4208, https://doi.org/10.5194/hess-20-4191-2016, 2016. a
Entekhabi, D., Njoku, E. G., O'Neill, P. E., Kellogg, K. H., Crow, W. T., Edelstein, W. N., Entin, J. K., Goodman, S. D., Jackson, T. J., Johnson, J., Kimball, J., Piepmeier, J. R., Koster, R. D., Martin, N., McDonald, K. C., Moghaddam, M., Moran, S., Reichle, R., Shi, J. C., Spencer, M. W., Thurman, S. W., Tsang, L., and Van Zyl, J.: The Soil Moisture Active Passive (SMAP) Mission, Proc. IEEE, 98, 704–716, https://doi.org/10.1109/JPROC.2010.2043918, 2010. a
Erlingis, J. M., Rodell, M., Peters-Lidard, C. D., Li, B., Kumar, S. V., Famiglietti, J. S., Granger, S. L., Hurley, J. V., Liu, P.-W., and Mocko, D. M.: A High-Resolution Land Data Assimilation System Optimized for the Western United States, J. Am. Water Resour. Assoc., https://doi.org/10.1111/1752-1688.12910, in press, 2021. a, b, c, d, e, f, g
Eroglu, O., Kurum, M., Boyd, D., and Gurbuz, A. C.: High spatio-temporal resolution CYGNSS soil moisture estimates using artificial neural networks, Remote Sens., 11, 2272, https://doi.org/10.3390/rs11192272, 2019. a, b
Esposito, G., Matano, F., and Scepi, G.: Analysis of Increasing Flash Flood Frequency in the Densely Urbanized Coastline of the Campi Flegrei Volcanic Area, Italy, Front. Earth Sci., 6, 63, https://doi.org/10.3389/feart.2018.00063, 2018. a
Fairbairn, D., Barbu, A. L., Mahfouf, J.-F., Calvet, J.-C., and Gelati, E.: Comparing the ensemble and extended Kalman filters for in situ soil moisture assimilation with contrasting conditions, Hydrol. Earth Syst. Sci., 19, 4811–4830, https://doi.org/10.5194/hess-19-4811-2015, 2015. a
Fairbairn, D., de Rosnay, P., and Browne, P. A.: The New Stand-Alone Surface Analysis at ECMWF: Implications for Land–Atmosphere DA Coupling, J. Hydrometeorol., 20, 2023–2042, https://doi.org/10.1175/JHM-D-19-0074.1, 2019. a, b, c
Famiglietti, J. S., Ryu, D., Berg, A. A., Rodell, M., and Jackson, T. J.: Field observations of soil moisture variability across scales: Soil Moisture Variabilty Across Scales, Water Resour. Res., 44, W01423, https://doi.org/10.1029/2006WR005804, 2008. a
Fang, B., Lakshmi, V., Bindlish, R., and Jackson, T.: AMSR2 Soil Moisture Downscaling Using Temperature and Vegetation Data, Remote Sens., 10, 1575, https://doi.org/10.3390/rs10101575, 2018. a
Fang, B., Lakshmi, V., Bindlish, R., Jackson, T. J., and Liu, P.-W.: Downscaling and Validation of SMAP Radiometer Soil Moisture in CONUS, in: IEEE, 39th annual IGARSS symposium, 28 July–2 August 2019, Yokohama, Japan, 6194–6197, 2019. a
Fang, B., Lakshmi, V., Bindlish, R., Jackson, T. J., and Liu, P.-W.: Evaluation and validation of a high spatial resolution satellite soil moisture product over the Continental United States, J. Hydrol., 588, 125043, https://doi.org/10.1016/j.jhydrol.2020.125043, 2020. a
Fang, B., Kansara, P., Dandridge, C., and Lakshmi, V.: Drought monitoring using high spatial resolution soil moisture data over Australia in 2015–2019, J. Hydrol., 594, 125960, https://doi.org/10.1016/j.jhydrol.2021.125960, 2021a. a, b
Fang, B., Lakshmi, V., Cosh, M. H., and Hain, C.: Very High Spatial Resolution Downscaled SMAP Radiometer Soil Moisture in the CONUS Using VIIRS/MODIS Data, IEEE J. Select. Top. App. Earth Obs. Remote Sens., 14, 4946–4965, https://doi.org/10.1109/JSTARS.2021.3076026, 2021b. a
FAO/IIASA/ISRIC/ISS-CAS/JRC: Harmonized World Soil Database (version 1.1), FAO, Rome, Italy and IIASA, Laxenburg, Austria, available at: http://www.fao.org/3/a-aq361e.pdf (last access: 1 November 2021), 2009. a
Fascetti, F., Pierdicca, N., Pulvirenti, L., Crapolicchio, R., and Sabater, J. M.: Soil moisture comparison through triple and quadruple collocation between: Metop, ERA, SMOS and in-situ data, in: 34th annual IGARSS symposium, 13–18 July 2014, Quebec City, Canada, 2451–2454, 2014. a, b
Fascetti, F., Pierdicca, N., Crapolicchio, R., Pulvirenti, L., and Muoz-Sabater, J.: An assessment of SMOS version 6.20 products through Triple and Quadruple Collocation techniques considering ASCAT, ERA/Interim LAND, ISMNand SMAP soil moisture data, in: 14th Specialist Meetingon Microwave Radiometry and Remote Sensing of the Environment (MicroRad 2016), 11–14 April 2016, Espoo, Finland, 91–94, 2016. a, b, c
Fascetti, F., Pierdicca, N., Pulvirenti, L., Crapolicchio, R., and Muñoz-Sabater, J.: A comparison of ASCAT and SMOS soil moisture retrievals over Europe and Northern Africa from 2010 to 2013, Int. J. Appl. Earth Obs. Geoinf., 45, 135–142, https://doi.org/10.1016/j.jag.2015.09.008, 2016. a, b
Fascetti, F., Pierdicca, N., Pulvirenti, L., and Crapolicchio, R.: Error characterization of SMOS, ASCAT, SMAP, ERA and ISMN soil moisture products: Automatic detection of cross-correlation error through extended quadruple collocation, in: 37th annual IGARSS symposium, 23–28 July 2017, Fort Worth, TX, USA, 4154–4157, https://doi.org/10.1109/IGARSS.2017.8127916, 2017. a, b, c
Fernandez-Moran, R., Al-Yaari, A., Mialon, A., Mahmoodi, A., Al Bitar, A., De Lannoy, G., Rodriguez-Fernandez, N., Lopez-Baeza, E., Kerr, Y., and Wigneron, J.-P.: SMOS-IC: An Alternative SMOS Soil Moisture and Vegetation Optical Depth Product, Remote Sens., 9, 457, https://doi.org/10.3390/rs9050457, 2017a. a
Fernandez-Moran, R., Wigneron, J.-P., De Lannoy, G., Lopez-Baeza, E., Parrens, M., Mialon, A., Mahmoodi, A., Al-Yaari, A., Bircher, S., Al Bitar, A., Richaume, P., and Kerr, Y.: A new calibration of the effective scattering albedo and soil roughness parameters in the SMOS SM retrieval algorithm, Int. J. Appl. Earth Obs. Geoinf., 62, 27–38, https://doi.org/10.1016/j.jag.2017.05.013, 2017b. a
Flammini, A., Corradini, C., Morbidelli, R., Saltalippi, C., Picciafuoco, T., and Giráldez, J. V.: Experimental analyses of the evaporation dynamics in bare soils under natural conditions, Water Resour. Manage., 32, 1153–1166, 2018a. a
Flammini, A., Morbidelli, R., Saltalippi, C., Picciafuoco, T., Corradini, C., and Govindaraju, R. S.: Reassessment of a semi-analytical field-scale infiltration model through experiments under natural rainfall events, J. Hydrol., 565, 835–845, 2018b. a
Foucras, M., Zribi, M., Albergel, C., Baghdadi, N., Calvet, J.-C., and Pellarin, T.: Estimating 500-m Resolution Soil Moisture Using Sentinel-1 and Optical Data Synergy, Water, 12, 866, https://doi.org/10.3390/w12030866, 2020. a, b
Fritz, S., Fonte, C., and See, L.: The Role of Citizen Science in Earth Observation, Remote Sens., 9, 357, https://doi.org/10.3390/rs9040357, 2017. a
Fuchsberger, J., Kirchengast, G., and Kabas, T.: WegenerNet high-resolution weather and climate data from 2007 to 2020, Earth Syst. Sci. Data, 13, 1307–1334, https://doi.org/10.5194/essd-13-1307-2021, 2021. a
Galle, S., Manuela, G., Peugeot, C., Bouzou-Moussa, I., Cappelaere, B., Demarty, J., Mougin, E., Panthou, G., Adjomayi, P., Agbossou, E., Abdramane, B., Boucher, M., Cohard, J.-M., Descloitres, M., Descroix, L., Diawara, M., Do, M., Favreau, G., Fabrice, G., and Wilcox, C.: AMMA-CATCH, a critical zone observatory in West Africa monitoring a region in transition, Vadose Zone J., 17, 1–24, https://doi.org/10.2136/vzj2018.03.0062, 2018. a
Gao, L., Sadeghi, M., and Ebtehaj, A.: Microwave retrievals of soil moisture and vegetation optical depth with improved resolution using a combined constrained inversion algorithm: Application for SMAP satellite, Remote Sens. Environ., 239, 111662, https://doi.org/10.1016/j.rse.2020.111662, 2020a. a
Gao, L., Sadeghi, M., Feldman, A. F., and Ebtehaj, A.: A Spatially Constrained Multichannel Algorithm for Inversion of a First-Order Microwave Emission Model at L-Band, IEEE T. Geosci. Remote, 58, 8134–8146, https://doi.org/10.1109/TGRS.2020.2987490, 2020b. a
Gardner, W. and Kirkham, D.: Determination of soil moisture by neutron scattering, Soil Sci., 73, 391–401, 1952. a
GCOS: The Global Climate Observing System, available at: https://gcos.wmo.int/en/gcos-implementation-plan (last access: 1 November 2021), 2016. a
Ghilain, N., Arboleda, A., Batelaan, O., Ardö, J., Trigo, I., Barrios, J.-M., and Gellens-Meulenberghs, F.: A new retrieval algorithm for soil moisture index from thermal infrared sensor on-board geostationary satellites over Europe and Africa and its validation, Remote Sens., 11, 1968, https://doi.org/10.3390/rs11171968, 2019. a
González-Teruel, J., Torres-Sánchez, R., Blaya-Ros, P., Toledo-Moreo, A., Jiménez-Buendía, M., and Soto-Valles, F.: Design and Calibration of a Low-Cost SDI-12 Soil Moisture Sensor, Sensors, 19, 491, https://doi.org/10.3390/s19030491, 2019. a
González-Zamora, A., Sánchez, N., Gumuzzio, A., Piles, M., Olmedo, E., and Martínez-Fernández, J.: Validation of SMOS L2 and L3 soil moisture products over the Duero Basin at different spatial scales, ISPRS - International Archives of the Photogrammetry, Remote Sensing and Spatial Information Sciences, XL-7/W3, 1183–1188, https://doi.org/10.5194/isprsarchives-XL-7-W3-1183-2015, 2015. a
González-Zamora, A., Sánchez, N., and Martínez-Fernández, J.: Validation of Aquarius Soil Moisture Products Over the Northwest of Spain: A Comparison With SMOS, IEEE J. Select. Top. Appl. Earth Obs. Remote Sens., 9, 2763–2769, 2016. a, b, c
González-Zamora, Á., Sanchez, N., Pablos, M., and Martínez-Fernández, J.: CCI soil moisture assessment with SMOS soil moisture and in situ data under different environmental conditions and spatial scales in Spain, Remote Sens. Environ., 225, 469–482, https://doi.org/10.1016/j.rse.2018.02.010, 2018. a
González-Zamora, Á., Sánchez, N., Pablos, M., and Martínez-Fernández, J.: CCI soil moisture assessment with SMOS soil moisture and in situ data under different environmental conditions and spatial scales in Spain, Remote Sens. Environ., 225, 469–482, https://doi.org/10.1016/j.rse.2018.02.010, 2019. a, b
Grainger, A.: Citizen Observatories and the New Earth Observation Science, Remote Sens., 9, 153, https://doi.org/10.3390/rs9020153, 2017. a
Greifeneder, F., Khamala, E., Sendabo, D., Wagner, W., Zebisch, M., Farah, H., and Notarnicola, C.: Detection of soil moisture anomalies based on Sentinel-1, Phys. Chem. Earth Pt. A/B/C, 112, 75–82, https://doi.org/10.1016/j.pce.2018.11.009, 2019. a
Greifeneder, F., Notarnicola, C., and Wagner, W.: A Machine Learning-Based Approach for Surface Soil Moisture Estimations with Google Earth Engine, Remote Sens., 13, 2099, https://doi.org/10.3390/rs13112099, 2021. a, b
Grillakis, M. G., Koutroulis, A. G., Alexakis, D. D., Polykretis, C., and Daliakopoulos, I. N.: Regionalizing Root-Zone Soil Moisture Estimates From ESA CCI Soil Water Index Using Machine Learning and Information on Soil, Vegetation, and Climate, Water Resour. Res., 57, e2020WR029249, https://doi.org/10.1029/2020WR029249, 2021. a, b, c
Gruber, A., Dorigo, W., Zwieback, S., Xaver, A., and Wagner, W.: Characterizing Coarse-Scale Representativeness of in situ Soil Moisture Measurements from the International Soil Moisture Network, Vadose Zone J., 12, vzj2012.0170, https://doi.org/10.2136/vzj2012.0170, 2013. a, b, c, d, e, f, g, h, i, j
Gruber, A., Paloscia, S., Santi, E., Notarnicola, C., Pasolli, L., Smolander, T., Pulliainen, J., Mittelbach, H., Dorigo, W., and Wagner, W.: Performance inter-comparison of soil moisture retrieval models for the MetOp-A ASCAT instrument, in: 34th annual IGARSS symposium, 13–18 July 2014, Quebec City, Canada, 2455–2458, https://doi.org/10.1109/IGARSS.2014.6946969, 2014. a
Gruber, A., Crow, W., Dorigo, W., and Wagner, W.: The potential of 2D Kalman filtering for soil moisture data assimilation, Remote Sens. Environ., 171, 137–148, https://doi.org/10.1016/j.rse.2015.10.019, 2015. a, b, c, d
Gruber, A., Su, C.-H., Crow, W. T., Zwieback, S., Dorigo, W. A., and Wagner, W.: Estimating error cross-correlations in soil moisture data sets using extended collocation analysis, J. Geophys. Res.-Atmos., 121, 1208–1219, https://doi.org/10.1002/2015JD024027, 2016. a
Gruber, A., Dorigo, W. A., Crow, W., and Wagner, W.: Triple Collocation-Based Merging of Satellite Soil Moisture Retrievals, IEEE T. Geosci. Remote, 55, 6780–6792, https://doi.org/10.1109/TGRS.2017.2734070, 2017. a, b, c
Gruber, A., Crow, W. T., and Dorigo, W. A.: Assimilation of Spatially Sparse In Situ Soil Moisture Networks into a Continuous Model Domain, Water Resour. Res., 54, 1353–1367, https://doi.org/10.1002/2017WR021277, 2018. a, b, c, d
Gruber, A., De Lannoy, G., and Crow, W.: A Monte Carlo based adaptive Kalman filtering framework for soil moisture data assimilation, Remote Sens. Environ., 228, 105–114, 2019a. a
Gruber, A., Scanlon, T., van der Schalie, R., Wagner, W., and Dorigo, W.: Evolution of the ESA CCI Soil Moisture climate data records and their underlying merging methodology, Earth Syst. Sci. Data, 11, 717–739, https://doi.org/10.5194/essd-11-717-2019, 2019b. a, b, c, d, e
Gruber, A., De Lannoy, G., Albergel, C., Al-Yaari, A., Brocca, L., Calvet, J.-C., Colliander, A., Cosh, M., Crow, W., Dorigo, W., Draper, C., Hirschi, M., Kerr, Y., Konings, A., Lahoz, W., McColl, K., Montzka, C., Muñoz-Sabater, J., Peng, J., Reichle, R., Richaume, P., Rüdiger, C., Scanlon, T., van der Schalie, R., Wigneron, J.-P., and Wagner, W.: Validation practices for satellite soil moisture retrievals: What are (the) errors?, Remote Sens. Environ., 244, 111806, https://doi.org/10.1016/j.rse.2020.111806, 2020. a, b, c, d, e, f, g
Guevara, M. and Vargas, R.: Downscaling satellite soil moisture using geomorphometry and machine learning, PloS One, 14, e0219639, https://doi.org/10.1371/journal.pone.0219639, 2019. a
Guevara, M., Taufer, M., and Vargas, R.: Gap-free global annual soil moisture: 15 km grids for 1991–2018, Earth Syst. Sci. Data, 13, 1711–1735, https://doi.org/10.5194/essd-13-1711-2021, 2021. a
Gumbricht, T.: Detecting Trends in Wetland Extent from MODIS Derived Soil Moisture Estimates, Remote Sens., 10, 611, https://doi.org/10.3390/rs10040611, 2018. a, b
Gumbricht, T., Roman-Cuesta, R. M., Verchot, L., Herold, M., Wittmann, F., Householder, E., Herold, N., and Murdiyarso, D.: An expert system model for mapping tropical wetlands and peatlands reveals South America as the largest contributor, Global Change Biol., 23, 3581–3599, https://doi.org/10.1111/gcb.13689, 2017. a
Gupta, D. K., Srivastava, P. K., Singh, A., Petropoulos, G. P., Stathopoulos, N., and Prasad, R.: SMAP Soil Moisture Product Assessment over Wales, U.K., Using Observations from the WSMN Ground Monitoring Network, Sustainability, 13, 11, https://doi.org/10.3390/su13116019, 2021. a
Hagan, D. F. T., Wang, G., Kim, S., Parinussa, R. M., Liu, Y., Ullah, W., Bhatti, A. S., Ma, X., Jiang, T., and Su, B.: Maximizing Temporal Correlations in Long-Term Global Satellite Soil Moisture Data-Merging, Remote Sens., 12, 2164, https://doi.org/10.3390/rs12132164, 2020. a, b, c, d, e, f, g
Hajdu, I., Yule, I., Bretherton, M., Singh, R., and Hedley, C.: Field performance assessment and calibration of multi-depth AquaCheck capacitance-based soil moisture probes under permanent pasture for hill country soils, Agr. Water Manage., 217, 332–345, https://doi.org/10.1016/j.agwat.2019.03.002, 2019. a
Han, M., Lu, H., and Yang, K.: Development of passive microwave retrieval algorithm for estimation of surface soil temperature from AMSR-E data, in: 36th annual IGARSS symposium, 10–15 July 2016, Beijing, China, 1671–1674, 2016. a
Han, M., Lu, H., Yang, K., Qin, J., Chen, Y., Zhao, L., and Lazhu Lab: A surface soil temperature retrieval algorithm based on AMSR-E multi-frequency brightness temperatures, Int. J. Remote Sens., 38, 6735–6754, https://doi.org/10.1080/01431161.2017.1363438, 2017. a
Han, Y., Bai, X., Shao, W., and Wang, J.: Retrieval of Soil Moisture by Integrating Sentinel-1A and MODIS Data over Agricultural Fields, Water, 12, 1726, https://doi.org/10.3390/w12061726, 2020. a, b
Hartmann, A., Gleeson, T., Rosolem, R., Pianosi, F., Wada, Y., and Wagener, T.: A large-scale simulation model to assess karstic groundwater recharge over Europe and the Mediterranean, Geosci. Model Dev., 8, 1729–1746, https://doi.org/10.5194/gmd-8-1729-2015, 2015. a
He, L., Chen, J. M., Mostovoy, G., and Gonsamo, A.: Soil Moisture Active Passive Improves Global Soil Moisture Simulation in a Land Surface Scheme and Reveals Strong Irrigation Signals Over Farmlands, Geophys. Res. Lett., 48, e2021GL092658, https://doi.org/10.1029/2021GL092658, 2021. a, b
Helgert, S. and Khodayar, S.: Improvement of the soil-atmosphere interactions and subsequent heavy precipitation modelling by enhanced initialization using remotely sensed 1 km soil moisture information, Remote Sens. Environ., 246, 111812, https://doi.org/10.1016/j.rse.2020.111812, 2020. a, b
Herbert, C., Pablos, M., Vall-llossera, M., Camps, A., and Martínez-Fernández, J.: Analyzing Spatio-Temporal Factors to Estimate the Response Time between SMOS and In-Situ Soil Moisture at Different Depths, Remote Sens., 12, 2614, https://doi.org/10.3390/rs12162614, 2020. a
Hersbach, H., Bell, B., Berrisford, P., Hirahara, S., Horányi, A., Muñoz-Sabater, J., Nicolas, J., Peubey, C., Radu, R., Schepers, D., Simmons, A., Soci, C., Abdalla, S., Abellan, X., Balsamo, G., Bechtold, P., Biavati, G., Bidlot, J., Bonavita, M., De Chiara, G., Dahlgren, P., Dee, D., Diamantakis, M., Dragani, R., Flemming, J., Forbes, R., Fuentes, M., Geer, A., Haimberger, L., Healy, S., Hogan, R. J., Hóm, E., Janisková, M., Keeley, S., Laloyaux, P., Lopez, P., Lupu, C., Radnoti, G., de Rosnay, P., Rozum, I., Vamborg, F., Villaume, S., and Thépaut, J.-N.: The ERA5 Global Reanalysis, Q. J. Roy. Meteorol. Soc., 146, 1999–2049, https://doi.org/10.1002/qj.3803, 2020. a, b, c
Hirschi, M., Mueller, B., Dorigo, W., and Seneviratne, S.: Using remotely sensed soil moisture for land–atmosphere coupling diagnostics: The role of surface vs. root-zone soil moisture variability, Remote Sens. Environ., 154, 246–252, https://doi.org/10.1016/j.rse.2014.08.030, 2014. a
Hoang, K. O. and Lu, M.: Impacts of temperature effect removal on rainfall estimation from soil water content by using SM2RAIN algorithm, IOP Conf. Ser.: Earth Environ. Sci., 344, 012046, https://doi.org/10.1088/1755-1315/344/1/012046, 2019. a
Hollinger, S. and Isard, S.: A Soil Moisture Climatology of Illinois, J. Climate, 7, 822–833, https://doi.org/10.1175/1520-0442(1994)007<0822:ASMCOI>2.0.CO;2, 1994. a
Hottenstein, J. D., Ponce-Campos, G. E., Moguel-Yanes, J., and Moran, M. S.: Impact of Varying Storm Intensity and Consecutive Dry Days on Grassland Soil Moisture, J. Hydrometeorol., 16, 106–117, https://doi.org/10.1175/JHM-D-14-0057.1, 2015. a, b
Hu, T., Zhao, T., Zhao, K., and Shi, J.: A continuous global record of near-surface soil freeze/thaw status from AMSR-E and AMSR2 data, Int. J. Remote Sens., 40, 6993–7016, https://doi.org/10.1080/01431161.2019.1597307, 2019. a, b, c
Ikonen, J., Vehviläinen, J., Rautiainen, K., Smolander, T., Lemmetyinen, J., Bircher, S., and Pulliainen, J.: The Sodankylä in situ soil moisture observation network: an example application of ESA CCI soil moisture product evaluation, Geosci. Instrum. Method. Data Syst., 5, 95–108, https://doi.org/10.5194/gi-5-95-2016, 2016. a
Ikonen, J., Smolander, T., Rautiainen, K., Cohen, J., Lemmetyinen, J., Salminen, M., and Pulliainen, J.: Spatially distributed evaluation of ESA CCI Soil Moisture products in a northern boreal forest environment, Geosciences, 8, 51, https://doi.org/10.3390/geosciences8020051, 2018. a
ISMN: ISMN stated terms and conditions for data use, available at: https://ismn.geo.tuwien.ac.at/en/terms-and-conditions/, last access: 20 August 2020. a
ISMN: Welcome to the International Soil Moisture Network, available at: https://ismn.earth, last access: 28 October 2021. a
Jackson, T., Cosh, M., Bindlish, R., Starks, P., Bosch, D., Seyfried, M., Goodrich, D., Moran, M., and Du, J.: Validation of Advanced Microwave Scanning Radiometer Soil Moisture Products, IEEE T. Geosci. Remote, 48, 4256–4272, https://doi.org/10.1109/TGRS.2010.2051035, 2011. a
Jalilvand, E., Tajrishy, M., Brocca, L., Massari, C., Ghazi Zadeh Hashemi, S., and Ciabatta, L.: Estimating the drainage rate from surface soil moisture drydowns: Application of DfD model to in situ soil moisture data, J. Hydrol., 565, 489–501, https://doi.org/10.1016/j.jhydrol.2018.08.035, 2018. a
Jensen, K. and Refsgaard, J.: HOBE: The Danish Hydrological Observatory, Vadose Zone J., 17, 180059, https://doi.org/10.2136/vzj2018.03.0059, 2018. a
Ji, P., Yuan, X., and Liang, X.-Z.: Do Lateral Flows Matter for the Hyperresolution Land Surface Modeling?, J. Geophys. Res.-Atmos., 122, 12077–12092, https://doi.org/10.1002/2017JD027366, 2017. a
Jin, R., Li, X., Yan, B., Li, X., Luo, W., Ma, M., Guo, J., Kang, J., Zhu, Z., and Zhao, S.: A Nested Ecohydrological Wireless Sensor Network for Capturing the Surface Heterogeneity in the Midstream Areas of the Heihe River Basin, China, IEEE Geosci. Remote Sens. Lett., 11, 2015–2019, https://doi.org/10.1109/LGRS.2014.2319085, 2014. a
Kang, C. S., Kanniah, K. D., and Kerr, Y. H.: Calibration of SMOS Soil Moisture Retrieval Algorithm: A Case of Tropical Site in Malaysia, IEEE T. Geosci. Remote, 57, 3827–3839, 2019. a, b, c
Kang, J., Li, X., Jin, R., Ge, Y., Wang, J., and Wang, J.: Hybrid Optimal Design of the Eco-Hydrological Wireless Sensor Network in the Middle Reach of the Heihe River Basin, China, Sensors, 14, 19095–19114, https://doi.org/10.3390/s141019095, 2014. a
Kapilaratne, R. J. and Lu, M.: Automated general temperature correction method for dielectric soil moisture sensors, J. Hydrol., 551, 203–216, https://doi.org/10.1016/j.jhydrol.2017.05.050, 2017. a
Karthikeyan, L., Pan, M., Wanders, N., Kumar, D. N., and Wood, E. F.: Four decades of microwave satellite soil moisture observations: Part 2. Product validation and inter-satellite comparisons, Adv. Water Resour., 109, 236–252, https://doi.org/10.1016/j.advwatres.2017.09.010, 2017. a, b, c, d, e, f, g, h, i, j, k
Kędzior, M. and Zawadzki, J.: Comparative study of soil moisture estimations from SMOS satellite mission, GLDAS database, and cosmic-ray neutrons measurements at COSMOS station in Eastern Poland, Geoderma, 283, 21–31, https://doi.org/10.1016/j.geoderma.2016.07.023, 2016. a, b
Kerr, Y., Al-Yaari, A., Rodriguez-Fernandez, N., Parrens, M., Molero, B., Leroux, D., Bircher, S., Mahmoodi, A., Mialon, A., Richaume, P., Delwart, S., Al Bitar, A., Pellarin, T., Bindlish, R., Jackson, T., Rüdiger, C., Waldteufel, P., Mecklenburg, S., and Wigneron, J.-P.: Overview of SMOS performance in terms of global soil moisture monitoring after six years in operation, Remote Sens. Environ., 180, 40–63, https://doi.org/10.1016/j.rse.2016.02.042, 2016. a, b
Khaki, M., Hoteit, I., Kuhn, M., Forootan, E., and Awange, J.: Assessing data assimilation frameworks for using multi-mission satellite products in a hydrological context, Sci. Total Environ., 647, 1031–1043, https://doi.org/10.1016/j.scitotenv.2018.08.032, 2019. a, b
Kim, H. and Lakshmi, V.: Use of Cyclone Global Navigation Satellite System (CyGNSS) Observations for Estimation of Soil Moisture, Geophys. Res. Lett., 45, 8272–8282, https://doi.org/10.1029/2018GL078923, 2018. a, b, c
Kim, H., Parinussa, R., Konings, A. G., Wagner, W., Cosh, M. H., Lakshmi, V., Zohaib, M., and Choi, M.: Global-scale assessment and combination of SMAP with ASCAT (active) and AMSR2 (passive) soil moisture products, Remote Sens. Environ., 204, 260–275, https://doi.org/10.1016/j.rse.2017.10.026, 2018. a, b, c
Kim, K., Jung, S., and Lee, Y.: A Study for establishment of soil moisture station in mountain terrain (1): the representative analysis of soil moisture for construction of Cosmic-ray verification system, J. Korea Water Resour. Assoc., 52, 51–60, 2019. a
Kim, S., Liu, Y., Johnson, F. M., Parinussa, R. M., and Sharma, A.: A global comparison of alternate AMSR2 soil moisture products: Why do they differ?, Remote Sens. Environ., 161, 43–62, https://doi.org/10.1016/j.rse.2015.02.002, 2015a. a
Kim, S., Parinussa, R. M., Liu, Y. Y., Johnson, F. M., and Sharma, A.: A framework for combining multiple soil moisture retrievals based on maximizing temporal correlation, Geophys. Res. Lett., 42, 6662–6670, https://doi.org/10.1002/2015GL064981, 2015b. a
Kim, S., Parinussa, R., Liu, Y., Johnson, F., and Sharma, A.: Merging Alternate Remotely-Sensed Soil Moisture Retrievals Using a Non-Static Model Combination Approach, Remote Sens., 8, 518, https://doi.org/10.3390/rs8060518, 2016. a, b, c
Kim, S., Sharma, A., Liu, Y., and Young, S.: Rethinking Satellite Data Merging: From Averaging to SNR Optimization, IEEE T. Geosci. Remote, https://doi.org/10.36227/techrxiv.14214035, in press, 2021. a, b
Kirchengast, G., Kabas, T., Leuprecht, A., Bichler, C., and Truhetz, H.: WegenerNet: A Pioneering High-Resolution Network for Monitoring Weather and Climate, B. Am. Meteorol. Soc., 95, 227–242, https://doi.org/10.1175/BAMS-D-11-00161.1, 2014. a
Kizito, F., Campbell, C., Campbell, G., Cobos, D., Teare, B., Carter, B., and Hopmans, J.: Frequency, electrical conductivity and temperature analysis of a low-cost capacitance soil moisture sensor, J. Hydrol., 352, 367–378, https://doi.org/10.1016/j.jhydrol.2008.01.021, 2008. a
Kojima, Y., Shigeta, R., Miyamoto, N., Shirahama, Y., Nishioka, K., Mizoguchi, M., and Kawahara, Y.: Low-Cost Soil Moisture Profile Probe Using Thin-Film Capacitors and a Capacitive Touch Sensor, Sensors, 16, 1292, https://doi.org/10.3390/s16081292, 2016. a
Kolassa, J., Aires, F., Polcher, J., Prigent, C., Jimenez, C., and Pereira, J. M.: Soil moisture retrieval from multi-instrument observations: Information content analysis and retrieval methodology, J. Geophys. Res.-Atmos., 118, 4847–4859, https://doi.org/10.1029/2012JD018150, 2013. a, b
Kolassa, J., Gentine, P., Prigent, C., Aires, F., and Alemohammad, S.: Soil moisture retrieval from AMSR-E and ASCAT microwave observation synergy. Part 2: Product evaluation, Remote Sens. Environ., 195, 202–217, https://doi.org/10.1016/j.rse.2017.04.020, 2017. a, b
Kolassa, J., Aires, F., Polcher, J., Prigent, C., Jimenez, C., and Pereira, J. M.: Estimating surface soil moisture from SMAP observations using a Neural Network technique, Remote Sens. Environ., 204, 43–59, https://doi.org/10.1016/j.rse.2017.10.045, 2018. a
Kornelsen, K. C. and Coulibaly, P.: Root-zone soil moisture estimation using data-driven methods, Water Resour. Res., 50, 2946–2962, https://doi.org/10.1002/2013WR014127, 2014. a
Kornelsen, K. C. and Coulibaly, P.: Reducing multiplicative bias of satellite soil moisture retrievals, Remote Sens. Environ., 165, 109–122, https://doi.org/10.1016/j.rse.2015.04.031, 2015. a
Kosmala, M., Wiggins, A., Swanson, A., and Simmons, B.: Assessing data quality in citizen science, Front. Ecol. Environ., 14, 551–560, https://doi.org/10.1002/fee.1436, 2016. a
Kovačević, J., Cvijetinović, Z., Stančić, N., Brodić, N., and Mihajlović, D.: New Downscaling Approach Using ESA CCI SM Products for Obtaining High Resolution Surface Soil Moisture, Remote Sens., 12, 1119, https://doi.org/10.3390/rs12071119, 2020. a
Kovács, K. Z., Hemment, D., Woods, M., van der Velden, N. K., Xaver, A., Giesen, R. H., Burton, V. J., Garrett, N. L., Zappa, L., Long, D., Dobos, E., and Skalsky, R.: Citizen observatory based soil moisture monitoring – the GROW example, Hungar. Geogr. Bull., 68, 119–139, https://doi.org/10.15201/hungeobull.68.2.2, 2019. a, b
Krueger, E. S., Ochsner, T. E., and Quiring, S. M.: Development and Evaluation of Soil Moisture-Based Indices for Agricultural Drought Monitoring, Agron. J., 111, 1392–1406, https://doi.org/10.2134/agronj2018.09.0558, 2019. a
Kumar, M. S., Chandra, T. R., Kumar, D. P., and Manikandan, M. S.: Monitoring moisture of soil using low cost homemade Soil moisture sensor and Arduino UNO, in: IEEE 2016 3rd International Conference on Advanced Computing and Communication Systems (ICACCS), Coimbatore, India, 1–4, https://doi.org/10.1109/ICACCS.2016.7586312, 2016. a
Kumar, S., Newman, M., Wang, Y., and Livneh, B.: Potential Reemergence of Seasonal Soil Moisture Anomalies in North America, Journal of Climate, 32, 2707–2734, https://doi.org/10.1175/JCLI-D-18-0540.1, 2019a. a
Kumar, S. V., M. Mocko, D., Wang, S., Peters-Lidard, C. D., and Borak, J.: Assimilation of Remotely Sensed Leaf Area Index into the Noah-MP Land Surface Model: Impacts on Water and Carbon Fluxes and States over the Continental United States, J. Hydrometeorol., 20, 1359–1377, https://doi.org/10.1175/JHM-D-18-0237.1, 2019b. a
Kumar, S. V., Peters-Lidard, C. D., Santanello, J., Harrison, K., Liu, Y., and Shaw, M.: Land surface Verification Toolkit (LVT) – a generalized framework for land surface model evaluation, Geosci. Model Dev., 5, 869–886, https://doi.org/10.5194/gmd-5-869-2012, 2012. a
Larson, K., Small, E., Gutmann, E., Bilich, A., Braun, J., Zavorotny, V., and Larson, C.: Use of GPS receivers as a soil moisture network for water cycle studies, Geophys. Res. Lett., 35, L24405, https://doi.org/10.1029/2008GL036013, 2008. a, b, c
Leavesley, G., David, O., Garen, D., Lea, J., Marron, J., Pagano, T., Perkins, T., and Strobel, M.: A Modeling Framework for Improved Agricultural Water Supply Forecasting, in: AGU Fall Meeting Abstracts, 2008. a
Lee, J.: The consecutive dry days to trigger rainfall over West Africa, J. Hydrol., 556, 934–943, https://doi.org/10.1016/j.jhydrol.2016.06.003, 2018. a, b, c
Lee, J. and Im, J.: A Novel Bias Correction Method for Soil Moisture and Ocean Salinity (SMOS) Soil Moisture: Retrieval Ensembles, Remote Sens., 7, 16045–16061, https://doi.org/10.3390/rs71215824, 2015. a
L'Heureux, J. L.: 2011 Installation Report for AAFC- SAGES Soil Moisture Stations in Kenaston, SK, Agriculture, 2011. a
Lei, F., Crow, W. T., Shen, H., Su, C.-H., Holmes, T. R., Parinussa, R. M., and Wang, G.: Assessment of the impact of spatial heterogeneity on microwave satellite soil moisture periodic error, Remote Sens. Environ., 205, 85–99, https://doi.org/10.1016/j.rse.2017.11.002, 2018. a
Leng, P., Song, X., Li, Z.-L., Wang, Y., and Wang, R.: Toward the Estimation of Surface Soil Moisture Content Using Geostationary Satellite Data over Sparsely Vegetated Area, Remote Sens., 7, 4112–4138, https://doi.org/10.3390/rs70404112, 2015. a, b
Leng, P., Song, X., Duan, S.-B., and Li, Z.-L.: Preliminary validation of two temporal parameter-based soil moisture retrieval models using a satellite product and in situ soil moisture measurements over the REMEDHUS network, Int. J. Remote Sens., 37, 5902–5917, https://doi.org/10.1080/01431161.2016.1253896, 2016. a
Leng, P., Song, X., Duan, S.-B., and Li, Z.-L.: Generation of continuous surface soil moisture dataset using combined optical and thermal infrared images, Hydrol. Process., 31, 1398–1407, https://doi.org/10.1002/hyp.11113, 2017. a, b
Li, M., Wu, P., and Ma, Z.: A comprehensive evaluation of soil moisture and soil temperature from third-generation atmospheric and land reanalysis data sets, Int. J. Climatol., 40, 5744–5766, https://doi.org/10.1002/joc.6549, 2020. a, b, c, d, e
Li, M., Wu, P., Sexton, D. M., and Ma, Z.: Potential shifts in climate zones under a future global warming scenario using soil moisture classification, Clim. Dynam., 56, 2071–2092, https://doi.org/10.1007/s00382-020-05576-w, 2021. a, b
Li, P., Zha, Y., Tso, C.-H. M., Shi, L., Yu, D., Zhang, Y., and Zeng, W.: Data assimilation of uncalibrated soil moisture measurements from frequency-domain reflectometry, Geoderma, 374, 114432, https://doi.org/10.1016/j.geoderma.2020.114432, 2020. a, b
Li, X., Al-Yaari, A., Schwank, M., Fan, L., Frappart, F., Swenson, J., and Wigneron, J.-P.: Compared performances of SMOS-IC soil moisture and vegetation optical depth retrievals based on Tau-Omega and Two-Stream microwave emission models, Remote Sens. Environ., 236, 111502, https://doi.org/10.1016/j.rse.2019.111502, 2020. a
Liao, W., Wang, D., Wang, G., Xia, Y., and Liu, X.: Quality Control and Evaluation of the Observed Daily Data in the North American Soil Moisture Database, J. Meteorol. Res., 33, 501–518, https://doi.org/10.1007/s13351-019-8121-2, 2019. a
Lievens, H., Martens, B., Verhoest, N., Hahn, S., Reichle, R., and Miralles, D.: Assimilation of global radar backscatter and radiometer brightness temperature observations to improve soil moisture and land evaporation estimates, Remote Sens. Environ., 189, 194–210, https://doi.org/10.1016/j.rse.2016.11.022, 2017. a, b, c, d
Lin, L.-F. and Pu, Z.: Examining the Impact of SMAP Soil Moisture Retrievals on Short-Range Weather Prediction under Weakly and Strongly Coupled Data Assimilation with WRF-Noah, Mon. Weather Rev., 147, 4345–4366, https://doi.org/10.1175/MWR-D-19-0017.1, 2019. a, b
Lin, L.-F. and Pu, Z.: Improving Near-Surface Short-Range Weather Forecasts Using Strongly Coupled Land–Atmosphere Data Assimilation with GSI-EnKF, Mon. Weather Rev., 148, 2863–2888, https://doi.org/10.1175/MWR-D-19-0370.1, 2020. a
Link, M., Drusch, M., and Scipal, K.: Soil Moisture Information Content in SMOS, SMAP, AMSR2, and ASCAT Level-1 Data Over Selected In Situ Sites, IEEE Geosci. Remote Sens. Lett., 17, 1213–1217, 2020. a, b, c, d
Liu, G., Chen, J.-M., Ji, L.-R., and Sun, S.-Q.: Relationship of summer soil moisture with early winter monsoon and air temperature over eastern China, Int. J. Climatol., 32, 1513–1519, https://doi.org/10.1002/joc.2352, 2012. a, b
Liu, S., Mo, X., Li, H., Peng, G., and Robock, A.: Spatial Variation of Soil Moisture in China: Geostatistical Characterization, J. Meteorol. Soc. Jpn., 79, 555–574, https://doi.org/10.2151/jmsj.79.555, 2001. a
Liu, S., Roujean, J.-L., Kaptue Tchuente, A. T., Ceamanos, X., and Calvet, J.-C.: A parameterization of SEVIRI and MODIS daily surface albedo with soil moisture: Calibration and validation over southwestern France, Remote Sens. Environ., 144, 137–151, https://doi.org/10.1016/j.rse.2014.01.016, 2014. a, b, c
Liu, Y., Dorigo, W., Parinussa, R., de Jeu, R., Wagner, W., McCabe, M., Evans, J., and van Dijk, A.: Trend-preserving blending of passive and active microwave soil moisture retrievals, Remote Sens. Environ., 123, 280–297, https://doi.org/10.1016/j.rse.2012.03.014, 2012. a, b, c, d, e
Liu, Y., Yang, Y., and Yue, X.: Evaluation of Satellite-Based Soil Moisture Products over Four Different Continental In-Situ Measurements, Remote Sens., 10, 1161, https://doi.org/10.3390/rs10071161, 2018. a, b, c, d
Liu, Y., Jing, W., Wang, Q., and Xia, X.: Generating high-resolution daily soil moisture by using spatial downscaling techniques: a comparison of six machine learning algorithms, Adv. Water Resour., 141, 103601, https://doi.org/10.1016/j.advwatres.2020.103601, 2020. a
Liu, Y., Zhou, Y., Lu, N., Tang, R., Liu, N., Li, Y., Yang, J., Jing, W., and Zhou, C.: Comprehensive assessment of Fengyun-3 satellites derived soil moisture with in-situ measurements across the globe, J. Hydrol., 594, 125949, https://doi.org/10.1016/j.jhydrol.2020.125949, 2021. a, b
Liu, Y. Y., Parinussa, R. M., Dorigo, W. A., De Jeu, R. A. M., Wagner, W., van Dijk, A. I. J. M., McCabe, M. F., and Evans, J. P.: Developing an improved soil moisture dataset by blending passive and active microwave satellite-based retrievals, Hydrol. Earth Syst. Sci., 15, 425–436, https://doi.org/10.5194/hess-15-425-2011, 2011. a, b, c, d
Liu, Z., Li, P., and Yang, J.: Soil Moisture Retrieval and Spatiotemporal Pattern Analysis Using Sentinel-1 Data of Dahra, Senegal, Remote Sens., 9, 1197, https://doi.org/10.3390/rs9111197, 2017. a
Loew, A., dall'Amico, J., Schlenz, F., and Mauser, W.: The Upper Danube soil moisture validation site: measurements and activities, in: Earth Observation and Water Cycle Science, edited by: Lacoste, H., vol. 674 of ESA Special Publication, p. 56, 2009. a
Loew, A., Bell, W., Brocca, L., Bulgin, C. E., Burdanowitz, J., Calbet, X., Donner, R. V., Ghent, D., Gruber, A., Kaminski, T., Kinzel, J., Klepp, C., Lambert, J.-C., Schaepman-Strub, G., Schröder, M., and Verhoelst, T.: Validation practices for satellite-based Earth observation data across communities, Rev. Geophys., 55, 779–817, https://doi.org/10.1002/2017RG000562, 2017. a
Lu, Y., Jin, J., and Kueppers, L.: Crop growth and irrigation interact to influence surface fluxes in a regional climate-cropland model (WRF3.3-CLM4crop), Clim. Dynam., 45, 3347–3363, https://doi.org/10.1007/s00382-015-2543-z, 2015. a
Lukyanenko, R., Wiggins, A., and Rosser, H. K.: Citizen Science: An Information Quality Research Frontier, Inform. Syst. Front., 22, 961–983, https://doi.org/10.1007/s10796-019-09915-z, 2020. a
Ma, C., Li, X., and McCabe, M. F.: Retrieval of High-Resolution Soil Moisture through Combination of Sentinel-1 and Sentinel-2 Data, Remote Sens., 12, 2303, https://doi.org/10.3390/rs12142303, 2020. a
Ma, H., Zeng, J., Chen, N., Zhang, X., Cosh, M. H., and Wang, W.: Satellite surface soil moisture from SMAP, SMOS, AMSR2 and ESA CCI: A comprehensive assessment using global ground-based observations, Remote Sens. Environ., 231, 111215, https://doi.org/10.1016/j.rse.2019.111215, 2019. a, b, c, d
Mahecha, M., Gans, F., Sippel, S., Donges, J., Kaminski, T., Metzger, S., Migliavacca, M., Papale, D., Rammig, A., and Zscheischler, J.: Detecting impacts of extreme events with ecological in-situ monitoring networks, Biogeosciences, 14, 4255–4277, https://doi.org/10.5194/bg-14-4255-2017, 2017. a
Majone, B., Viani, F., Filippi, E., Bellin, A., Massa, A., Toller, G., Robol, F., and Salucci, M.: Wireless Sensor Network Deployment for Monitoring Soil Moisture Dynamics at the Field Scale, Proced. Environ. Sci., 19, 426–435, https://doi.org/10.1016/j.proenv.2013.06.049, 2013. a
Manfreda, S., Brocca, L., Moramarco, T., Melone, F., and Sheffield, J.: A physically based approach for the estimation of root-zone soil moisture from surface measurements, Hydrol. Earth Syst. Sci., 18, 1199–1212, https://doi.org/10.5194/hess-18-1199-2014, 2014. a
Mao, F., Khamis, K., Krause, S., Clark, J., and Hannah, D. M.: Low-Cost Environmental Sensor Networks: Recent Advances and Future Directions, Front. Earth Sci., 7, 221, https://doi.org/10.3389/feart.2019.00221, 2019. a
Marczewski, W., Slominski, J., Slominska, E., Usowicz, B., Usowicz, J., Romanov, S., Maryskevych, O., Nastula, J., and Zawadzki, J.: Strategies for validating and directions for employing SMOS data, in the Cal-Val project SWEX (3275) for wetlands, Hydrol. Earth Syst. Sci. Discuss., 7, 7007–7057, https://doi.org/10.5194/hessd-7-7007-2010, 2010. a
Martens, B., Miralles, D., Lievens, H., van der Schalie, R., de Jeu, R. A., Fernández-Prieto, D., Beck, H. E., Dorigo, W., and Verhoest, N.: GLEAM v3: satellite-based land evaporation and root-zone soil moisture, Geosci. Model Dev., 10, 1903–1925, https://doi.org/10.5194/gmd-10-1903-2017, 2017. a, b, c, d
Martens, B., de Jeu, R., Verhoest, N., Schuurmans, H., Kleijer, J., and Miralles, D.: Towards Estimating Land Evaporation at Field Scales Using GLEAM, Remote Sens., 10, 1720, https://doi.org/10.3390/rs10111720, 2018. a
Martínez-Fernández, J., González-Zamora, A., and Almendra-Martín, L.: Soil moisture memory and soil properties: An analysis with the stored precipitation fraction, J. Hydrol., 593, 125622, https://doi.org/10.1016/j.jhydrol.2020.125622, 2021. a
Massari, C., Su, C.-H., Brocca, L., Sang, Y.-F., Ciabatta, L., Ryu, D., and Wagner, W.: Near real time de-noising of satellite-based soil moisture retrievals: An intercomparison among three different techniques, Remote Sens. Environ., 198, 17–29, https://doi.org/10.1016/j.rse.2017.05.037, 2017. a, b
Mattar, C., Santamaría-Artigas, A., Durán-Alarcón, C., Olivera-Guerra, L., and Fuster, R.: LAB-net the first Chilean soil moisture network for remote sensing applications, in: Quantitative Remote Sensing Symposium (RAQRS), 22–26, 2014. a
Mattar, C., Santamaría-Artigas, A., Durán-Alarcón, C., Olivera-Guerra, L., Fuster, R., and Borvarán, D.: The LAB-Net Soil Moisture Network: Application to Thermal Remote Sensing and Surface Energy Balance, Data, 1, 6, https://doi.org/10.3390/data1010006, 2016. a
McDonough, K. R., Hutchinson, S. L., Hutchinson, J. S., Case, J. L., and Rahmani, V.: Validation and assessment of SPoRT-LIS surface soil moisture estimates for water resources management applications, J. Hydrol., 566, 43–54, https://doi.org/10.1016/j.jhydrol.2018.09.007, 2018. a, b
Mecklenburg, S., Drusch, M., Kerr, Y. H., Font, J., Martin-Neira, M., Delwart, S., Buenadicha, G., Reul, N., Daganzo-Eusebio, E., Oliva, R., and Crapolicchio, R.: ESA's Soil Moisture and Ocean Salinity Mission: Mission Performance and Operations, IEEE T. Geosci. Remote, 50, 1354–1366, https://doi.org/10.1109/TGRS.2012.2187666, 2012. a, b
Mimeau, L., Tramblay, Y., Brocca, L., Massari, C., Camici, S., and Finaud-Guyot, P.: Modeling the response of soil moisture to climate variability in the Mediterranean region, Hydrol. Earth Syst. Sci., 25, 653–669, https://doi.org/10.5194/hess-25-653-2021, 2021. a
Miralles, D. G., Crow, W. T., and Cosh, M. H.: Estimating Spatial Sampling Errors in Coarse-Scale Soil Moisture Estimates Derived from Point-Scale Observations, J. Hydrometeorol., 11, 1423–1429, https://doi.org/10.1175/2010JHM1285.1, 2010. a
Mishra, V., Shah, R., and Thrasher, B.: Soil Moisture Droughts under the Retrospective and Projected Climate in India, J. Hydrometeorol., 15, 2267–2292, https://doi.org/10.1175/JHM-D-13-0177.1, 2014. a
Mishra, V., Shah, R., Azhar, S., Shah, H., Modi, P., and Kumar, R.: Reconstruction of droughts in India using multiple land-surface models (1951–2015), Hydrol. Earth Syst. Sci., 22, 2269–2284, https://doi.org/10.5194/hess-22-2269-2018, 2018. a, b, c, d
Mittelbach, H., Lehner, I., and Seneviratne, S. I.: Comparison of four soil moisture sensor types under field conditions in Switzerland, J. Hydrol., 430–431, 39–49, https://doi.org/10.1016/j.jhydrol.2012.01.041, 2012. a
Moghaddam, M., Entekhabi, D., Goykhman, Y., Li, K., Liu, M., Mahajan, A., Nayyar, A., Shuman, D., and Teneketzis, D.: A Wireless Soil Moisture Smart Sensor Web Using Physics-Based Optimal Control: Concept and Initial Demonstrations, IEEE J. Select. Top. Appl. Earth Obs. Remote Sens., 3, 522–535, https://doi.org/10.1109/JSTARS.2010.2052918, 2011. a
Moghaddam, M., Silva, A., Clewley, D., Akbar, R., Hussaini, S., Whitcomb, J., Devarakonda, R., Shrestha, R., Cook, R., Prakash, G., Santhana Vannan, S., and Boyer, A.: Soil Moisture Profiles and Temperature Data from SoilSCAPE Sites, USA, ORNL DAAC, Oak Ridge, Tennessee, USA, https://doi.org/10.3334/ORNLDAAC/1339, 2016. a
Mohanty, B. P., Cosh, M. H., Lakshmi, V., and Montzka, C.: Soil Moisture Remote Sensing: State-of-the-Science, Vadose Zone J., 16, vzj2016.10.0105, https://doi.org/10.2136/vzj2016.10.0105, 2017. a, b, c, d
Molero, B., Leroux, D. J., Richaume, P., Kerr, Y. H., Merlin, O., Cosh, M. H., and Bindlish, R.: Multi-Timescale Analysis of the Spatial Representativeness of In Situ Soil Moisture Data within Satellite Footprints: Soil Moisture Time and Spatial Scales, J. Geophys. Res.-Atmos., 123, 3–21, https://doi.org/10.1002/2017JD027478, 2018. a, b
Montzka, C., Bogena, H., Zreda, M., Monerris, A., Morrison, R., Muddu, S., and Vereecken, H.: Validation of Spaceborne and Modelled Surface Soil Moisture Products with Cosmic-Ray Neutron Probes, Remote Sens., 9, 103, https://doi.org/10.3390/rs9020103, 2017. a, b, c, d
Montzka, C., Cosh, M., Bayat, B., Bitar, A. A., Berg, A., Bindlish, R., Bogena, H. R., Bolton, J. D., Cabot, F., Caldwell, T., Chan, S., Colliander, A., Crow, W., Das, N., Lannoy, G. D., Dorigo, W., Evett, S. R., Gruber, A., Hahn, S., Jagdhuber, T., Jones, S., Kerr, Y., Kim, S., Koyama, C., Kurum, M., Lopez-Baeza, E., Mattia, F., McColl, K., Mecklenburg, S., Mohanty, B., O'Neill, P., Or, D., Pellarin, T., Petropoulos, G. P., Piles, M., Reichle, R. H., Rodriguez-Fernandez, N., Rüdiger, C., Scanlon, T., Schwartz, R. C., Spengler, D., Srivastava, P., Suman, S., van der Schalie, R., Wagner, W., Wegmüller, U., Camacho, F., and Nickeson, J.: Soil Moisture Product Validation Best Practice Protocol, Version 1.0, available at: https://pubs.er.usgs.gov/publication/70216425 (last access: 1 November 2021), 2020. a, b, c
Moradizadeh, M. and Srivastava, P. K.: A new model for an improved AMSR2 satellite soil moisture retrieval over agricultural areas, Comput. Electron. Agricult., 186, 106205, https://doi.org/10.1016/j.compag.2021.106205, 2021. a, b
Morbidelli, R., Corradini, C., Saltalippi, C., Flammini, A., and Rossi, E.: Infiltration-soil moisture redistribution under natural conditions: experimental evidence as a guideline for realizing simulation models, Hydrol. Earth Syst. Sci., 15, 2937–2945, https://doi.org/10.5194/hess-15-2937-2011, 2011. a
Morbidelli, R., Saltalippi, C., Flammini, A., Rossi, E., and Corradini, C.: Soil water content vertical profiles under natural conditions: matching of experiments and simulations by a conceptual model, Hydrol. Process., 28, 4732–4742, https://doi.org/10.1002/hyp.9973, 2014a. a
Morbidelli, R., Saltalippi, C., Flammini, A., Rossi, E., and Corradini, C.: Soil water content vertical profiles under natural conditions: Matching of experiments and simulations by a conceptual model, Hydrol. Process., 28, 4732–4742, 2014b. a
Morbidelli, R., Saltalippi, C., Flammini, A., Cifrodelli, M., Picciafuoco, T., Corradini, C., and Govindaraju, R. S.: In situ measurements of soil saturated hydraulic conductivity: Assessment of reliability through rainfall–runoff experiments, Hydrol. Process., 31, 3084–3094, https://doi.org/10.1002/hyp.11247, 2017. a
Moreno-Martínez, Á., Piles, M., Muñoz-Marí, J., Campos-Taberner, M., Adsuara, J. E., Mateo, A., Perez-Suay, A., Javier García-Haro, F., Camps-Valls, G., Prasad, S., and Chanussot, J.: Machine Learning Methods for Spatial and Temporal Parameter Estimation, Springer International Publishing, Cham, 5–35, https://doi.org/10.1007/978-3-030-38617-7_2, 2020. a, b, c
Mu, L., Li, S., Qin, B., and Liu, K.: Drought Monitoring Using Tiangong-2 Wide-Band Spectrometer Data, in: Proceedings of the Tiangong-2 Remote Sensing Application Conference, edited by: Gu, Y., Gao, M., and Zhao, G., Springer, Singapore, 277–285, https://doi.org/10.1007/978-981-13-3501-3_26, 2019. a
Muñoz Sabater, J., Rodriguez-Fernandez, N., Sabater, J. M., Richaume, P., De Rosnay, P., Kerr, Y., Albergel, C., Drusch, M., and Mecklenburg, S.: SMOS near-real-time soil moisture product: processor overview and first validation results, Hydrol. Earth Syst. Sci., 21, 5201–5216, https://doi.org/10.5194/hess-21-5201-2017, 2017. a
Musial, J. P., Dabrowska-Zielinska, K., Kiryla, W., Oleszczuk, R., Gnatowski, T., and Jaszczynski, J.: Derivation and validation of the high resolution satellite soil moisture products: a case study of the Biebrza Sentinel-1 validation sites, Geoinform. Issues, 8, 37–53, 2016. a
Nair, A. and Indu, J.: Enhancing Noah Land Surface Model Prediction Skill over Indian Subcontinent by Assimilating SMOPS Blended Soil Moisture, Remote Sens., 8, 976, https://doi.org/10.3390/rs8120976, 2016. a
Nair, A. S., Mangla, R. P. T., and Indu, J.: Remote sensing data assimilation, Hydrolog. Sci. J., 0, 1–33, https://doi.org/10.1080/02626667.2020.1761021, 2020. a, b
Naz, B. S., Kollet, S., Franssen, H.-J. H., Montzka, C., and Kurtz, W.: A 3 km spatially and temporally consistent European daily soil moisture reanalysis from 2000 to 2015, Scient. Data, 7, 111, https://doi.org/10.1038/s41597-020-0450-6, 2020. a, b
Nguyen, H. H., Kim, H., and Choi, M.: Evaluation of the soil water content using cosmic-ray neutron probe in a heterogeneous monsoon climate-dominated region, Adv. Water Resour., 108, 125–138, https://doi.org/10.1016/j.advwatres.2017.07.020, 2017. a, b
Nicolai-Shaw, N., Hirschi, M., Mittelbach, H., and Seneviratne, S. I.: Spatial representativeness of soil moisture using in situ, remote sensing, and land reanalysis data, J. Geophys. Res.-Atmos., 120, 9955–9964, https://doi.org/10.1002/2015JD023305, 2015a. a
Nicolai-Shaw, N., Hirschi, M., Mittelbach, H., and Seneviratne, S. I.: Spatial representativeness of soil moisture using in situ, remote sensing, and land reanalysis data, J. Geophys. Res.-Atmos., 120, 9955–9964, https://doi.org/10.1002/2015JD023305, 2015b. a, b, c, d
Notarnicola, C., Caporaso, L., Giuseppe, F. D., Temimi, M., Ventura, B., and Zebisch, M.: Inferring soil moisture variability in the Mediterrean Sea area using infrared and passive microwave observations, Can. J. Remote Sens., 38, 46–59, https://doi.org/10.5589/m12-011, 2012. a
O, S. and Orth, R.: Global soil moisture data derived through machine learning trained with in-situ measurements, Scient. Data, 8, 1–14, https://doi.org/10.1038/s41597-021-00964-1, 2021. a, b, c, d
Ochsner, T., Cosh, M., Cuenca, R., Dorigo, W., Draper, C., Hagimoto, Y., Kerr, Y., Larson, K., Njoku, E., Small, E., and Zreda, M.: State of the Art in Large-Scale Soil Moisture Monitoring, Soil Sci. Soc. Am. J., 77, 1888–1919, https://doi.org/10.2136/sssaj2013.03.0093, 2013. a
Ojha, N., Merlin, O., Suere, C., and Escorihuela, M. J.: Extending the Spatio-Temporal Applicability of DISPATCH Soil Moisture Downscaling Algorithm: A Study Case Using SMAP, MODIS and Sentinel-3 Data, Front. Environ. Sci., 9, 40, https://doi.org/10.3389/fenvs.2021.555216, 2021. a, b, c, d
Ojha, R., Morbidelli, R., Saltalippi, C., Flammini, A., and Govindaraju, R. S.: Scaling of surface soil moisture over heterogeneous fields subjected to a single rainfall event, J. Hydrol., 516, 21–36, https://doi.org/10.1016/j.jhydrol.2014.01.057, 2014. a
Ojo, E. R., Bullock, P., L'Heureux, J., Powers, J., McNairn, H., and Pacheco, A.: Calibration and Evaluation of a Frequency Domain Reflectometry Sensor for Real-Time Soil Moisture Monitoring, Vadose Zone J., 14, vzj2014.08.0114, https://doi.org/10.2136/vzj2014.08.0114, 2015. a
Osenga, E., Arnott, J., Endsley, K., and Katzenberger, J.: Bioclimatic and Soil Moisture Monitoring Across Elevation in a Mountain Watershed: Opportunities for Research and Resource Management, Water Resour. Res., 2493–2503, https://doi.org/10.1029/2018WR023653, 2019. a
Pablos, M., Martínez-Fernández, J., Piles, M., Sánchez, N., Vall-llossera, M., and Camps, A.: Multi-Temporal Evaluation of Soil Moisture and Land Surface Temperature Dynamics Using in Situ and Satellite Observations, Remote Sens., 8, 587, https://doi.org/10.3390/rs8070587, 2016. a, b
Pablos, M., González-Zamora, Á., Sánchez, N., and Martínez-Fernández, J.: Assessment of Root Zone Soil Moisture Estimations from SMAP, SMOS and MODIS Observations, Remote Sens., 10, 981, https://doi.org/10.3390/rs10070981, 2018. a, b, c, d
Pacheco, A., L'Heureux, J., McNairn, H., Powers, J., Howard, A., Geng, X., Rollin, P., Gottfried, K., Freeman, J., and Ojo, R.: Real-time in-situ soil monitoring for agriculture (RISMA) network metadata, Science and Technology Branch Agriculture and Agri-Food Canada, Edmonton, AB, Canada, 2014. a
Pal, M. and Maity, R.: Development of a spatially-varying Statistical Soil Moisture Profile model by coupling memory and forcing using hydrologic soil groups, J. Hydrol., 570, 141–155, 2019. a, b
Pal, M., Maity, R., and Dey, S.: Statistical Modelling of Vertical Soil Moisture Profile: Coupling of Memory and Forcing, Water Resour. Manage., 30, 1973–1986, https://doi.org/10.1007/s11269-016-1263-4, 2016. a
Palmer, J. R. B., Oltra, A., Collantes, F., Delgado, J. A., Lucientes, J., Delacour, S., Bengoa, M., Eritja, R., and Bartumeus, F.: Citizen science provides a reliable and scalable tool to track disease-carrying mosquitoes, Nat. Commun., 8, 916, https://doi.org/10.1038/s41467-017-00914-9, 2017. a
Paloscia, S., Pettinato, S., Santi, E., Notarnicola, C., Pasolli, L., and Reppucci, A.: Soil moisture mapping using Sentinel-1 images: Algorithm and preliminary validation, Remote Sens. Environ., 134, 234–248, https://doi.org/10.1016/j.rse.2013.02.027, 2013. a
Pan, X., Kornelsen, K. C., and Coulibaly, P.: Estimating Root Zone Soil Moisture at Continental Scale Using Neural Networks, J. Am. Water Resour. Assoc., 53, 220–237, https://doi.org/10.1111/1752-1688.12491, 2017. a, b, c
Parinussa, R., Wang, G., Holmes, T., Liu, Y., Dolman, A., de Jeu, R., Jiang, T., Zhang, P., and Shi, J.: Global surface soil moisture from the Microwave Radiation Imager onboard the Fengyun-3B satellite, Int. J. Remote Sens., 35, 7007–7029, https://doi.org/10.1080/01431161.2014.960622, 2014a. a, b
Parinussa, R. M., Yilmaz, M. T., Anderson, M. C., Hain, C. R., and de Jeu, R. A. M.: An intercomparison of remotely sensed soil moisture products at various spatial scales over the Iberian Peninsula, Hydrol. Process., 28, 4865–4876, https://doi.org/10.1002/hyp.9975, 2014b. a, b
Parinussa, R., de Jeu, R., van der Schalie, R., Crow, W., Lei, F., and Holmes, T.: A Quasi-Global Approach to Improve Day-Time Satellite Surface Soil Moisture Anomalies through the Land Surface Temperature Input, Climate, 4, 50, https://doi.org/10.3390/cli4040050, 2016. a
Parinussa, R. M., Holmes, T. R. H., and de Jeu, R. A. M.: Soil Moisture Retrievals From the WindSat Spaceborne Polarimetric Microwave Radiometer, IEEE T. Geosci. Remote, 50, 2683–2694, https://doi.org/10.1109/TGRS.2011.2174643, 2012. a, b
Parinussa, R. M., Holmes, T. R. H., Wanders, N., Dorigo, W. A., and de Jeu, R. A. M.: A Preliminary Study toward Consistent Soil Moisture from AMSR2, J. Hydrometeorol., 16, 932–947, https://doi.org/10.1175/JHM-D-13-0200.1, 2015. a, b, c, d
Parinussa, R. M., Wang, G., Liu, Y., Lou, D., Hagan, D. F. T., Zhan, M., Su, B., and Jiang, T.: Improved surface soil moisture anomalies from Fengyun-3B over the Jiangxi province of the People's Republic of China, Int. J. Remote Sens., 39, 8950–8962, https://doi.org/10.1080/01431161.2018.1500729, 2018. a, b
Park, C.-H., Montzka, C., Jagdhuber, T., Jonard, F., De Lannoy, G., Hong, J., Jackson, T. J., and Wulfmeyer, V.: A Dielectric Mixing Model Accounting for Soil Organic Matter, Vadose Zone J., 18, 190036, https://doi.org/10.2136/vzj2019.04.0036, 2019. a, b, c
Park, C.-H., Jagdhuber, T., Colliander, A., Lee, J., Berg, A., Cosh, M., Kim, S.-B., Kim, Y., and Wulfmeyer, V.: Parameterization of Vegetation Scattering Albedo in the Tau-Omega Model for Soil Moisture Retrieval on Croplands, Remote Sens., 12, https://doi.org/10.20944/preprints202007.0717.v1, 2020. a
Parrens, M., Zakharova, E., Lafont, S., Calvet, J.-C., Kerr, Y., Wagner, W., and Wigneron, J.-P.: Comparing soil moisture retrievals from SMOS and ASCAT over France, Hydrol. Earth Syst. Sci., 16, 423–440, https://doi.org/10.5194/hess-16-423-2012, 2012. a, b, c
Paulik, C., Dorigo, W., Wagner, W., and Kidd, R.: Validation of the ASCAT Soil Water Index using in situ data from the International Soil Moisture Network, Int. J. Appl. Earth Obs. Geoinf., 30, 1–8, https://doi.org/10.1016/j.jag.2014.01.007, 2014. a, b, c
Peel, M. C., Finlayson, B. L., and McMahon, T. A.: Updated world map of the Köppen-Geiger climate classification, Hydrol. Earth Syst. Sci., 11, 1633–1644, https://doi.org/10.5194/hess-11-1633-2007, 2007. a
Peischl, S., Walker, J. P., Rüdiger, C., Ye, N., Kerr, Y. H., Kim, E., Bandara, R., and Allahmoradi, M.: The AACES field experiments: SMOS calibration and validation across the Murrumbidgee River catchment, Hydrol. Earth Syst. Sci., 16, 1697–1708, https://doi.org/10.5194/hess-16-1697-2012, 2012. a
Pellarin, T., de Rosnay, P., Albergel, C., Abdalla, S., and al Bitar, A.: Root-zone soil moisture index complementary validation at global scale based on triple collocation method. Comparison with State-OfThe-Art global scale root-zone soil moisture products, in: H-SAF Visiting Scientist Program HSAF_CDOP2_VS12_02, semanticscholar, available at: https://www.semanticscholar.org/paper/CDOP-2-_-VS-12-_-02-Root-zone-soil-moisture-index-.-Pellarin-Rosnay/b6b862c72ebd8862798516e4b3ce089d55a9b083 (last access: 1 November 2021), 2013. a, b, c, d
Peng, J., Loew, A., Merlin, O., and Verhoest, N. E. C.: A review of spatial downscaling of satellite remotely sensed soil moisture: Downscale Satellite-Based Soil Moisture, Rev. Geophys., 55, 341–366, https://doi.org/10.1002/2016RG000543, 2017. a
Petropoulos, G. P. and McCalmont, J. P.: An operational in situ soil moisture & soil temperature monitoring network for West Wales, UK: The WSMN network, Sensors, 17, 1481, https://doi.org/10.3390/s17071481, 2017. a, b
Petropoulos, G. P., Ireland, G., Srivastava, P. K., and Ioannou-Katidis, P.: An appraisal of the accuracy of operational soil moisture estimates from SMOS MIRAS using validated in situ observations acquired in a Mediterranean environment, Int. J. Remote Sens., 35, 5239–5250, https://doi.org/10.1080/2150704X.2014.933277, 2014. a
Pierdicca, N., Pulvirenti, L., and Fascetti, F.: Multitemporal soil moisture retrieval from 3-days ERS-2 data: Comparison with ASCAT, SMOS and in situ measurements, in: 34th annual IGARSS symposium, 13–18 July 2014, Quebec City, Canada, 2459–2462, https://doi.org/10.1109/IGARSS.2014.6946970, 2014. a, b, c
Pierdicca, N., Fascetti, F., Pulvirenti, L., Crapolicchio, R., and Muñoz Sabater, J.: Quadruple Collocation Analysis for Soil Moisture Product Assessment, IEEE Geosci. Remote Sens. Lett., 12, 1595–1599, https://doi.org/10.1109/LGRS.2015.2414654, 2015a. a, b, c
Pierdicca, N., Fascetti, F., Pulvirenti, L., Crapolicchio, R., and Muñoz-Sabater, J.: Analysis of ASCAT, SMOS, in-situ and land model soil moisture as a regionalized variable over Europe and North Africa, Remote Sens. Environ., 170, 280–289, https://doi.org/10.1016/j.rse.2015.09.005, 2015b. a, b, c, d
Pierdicca, N., Fascetti, F., Pulvirenti, L., and Crapolicchio, R.: Error Characterization of Soil Moisture Satellite Products: Retrieving Error Cross-Correlation Through Extended Quadruple Collocation, IEEE J. Select. Top. Appl. Earth Obs. Remote Sens., 10, 4522–4530, https://doi.org/10.1109/JSTARS.2017.2714025, 2017. a, b, c
Piles, M., Petropoulos, G. P., Sánchez, N., Ángel González-Zamora, and Ireland, G.: Towards improved spatio-temporal resolution soil moisture retrievals from the synergy of SMOS and MSG SEVIRI spaceborne observations, Remote Sens. Environ., 180, 403–417, https://doi.org/10.1016/j.rse.2016.02.048, 2016. a, b
Piles, M., Ballabrera-Poy, J., and Muñoz-Sabater, J.: Dominant features of global surface soil moisture variability observed by the SMOS satellite, Remote Sens., 11, 95, https://doi.org/10.3390/rs11010095, 2019. a
Portal, G., Jagdhuber, T., Vall-llossera, M., Camps, A., Pablos, M., Entekhabi, D., and Piles, M.: Assessment of Multi-Scale SMOS and SMAP Soil Moisture Products across the Iberian Peninsula, Remote Sens., 12, 570, https://doi.org/10.3390/rs12030570, 2020. a, b
Pradhan, N. R.: Estimating growing-season root zone soil moisture from vegetation index-based evapotranspiration fraction and soil properties in the Northwest Mountain region, USA, Hydrolog. Sci. J., 64, 771–788, 2019. a, b, c
Pratola, C., Barrett, B., Gruber, A., and Dwyer, E.: Quality Assessment of the CCI ECV Soil Moisture Product Using ENVISAT ASAR Wide Swath Data over Spain, Ireland and Finland, Remote Sens., 7, 15388–15423, https://doi.org/10.3390/rs71115388, 2015. a
Preimesberger, W.: Validation of C3S v202012 vs C3S v201912 vs ISMN 20210131 global, Zenodo [data set], https://doi.org/10.5281/zenodo.4736927, 2021. a
Preimesberger, W., Buttinger, P., Paulik, C., Scanlon, T., Aberer, D., Schmitzer, M., Baum, D., Zappa, L., Hahn, S., and Crezee, B.: TUW-GEO/ismn: v1.1.0, Zenodo [data set], https://doi.org/10.5281/zenodo.5095509, 2021. a
Przeździecki, K., Zawadzki, J., Cieszewski, C., and Bettinger, P.: Estimation of soil moisture across broad landscapes of Georgia and South Carolina using the triangle method applied to MODIS satellite imagery, Silva Fennica, 51, 1683, https://doi.org/10.14214/sf.1683, 2017. a
Qin, M., Giménez, D., and Miskewitz, R.: Temporal dynamics of subsurface soil water content estimated from surface measurement using wavelet transform, J. Hydrol., 563, 834–850, https://doi.org/10.1016/j.jhydrol.2018.06.023, 2018. a
Raffelli, G., Id, M., Previati, M., Id, D., Canone, D., Gisolo, D., Bevilacqua, I., Capello, G., Biddoccu, M., Cavallo, E., Id, R., Deiana, R., Id, G., Cassiani, G., and Ferraris, S.: Local-and Plot-Scale Measurements of Soil Moisture: Time and Spatially Resolved Field Techniques in Plain, Hill and Mountain Sites, Water, 9, 706, https://doi.org/10.3390/w9090706, 2018. a
Rains, D., Han, X., Lievens, H., Montzka, C., and Verhoest, N.: SMOS brightness temperature assimilation into the Community Land Model, Hydrol. Earth Syst. Sci., 21, 5929–5951, https://doi.org/10.5194/hess-21-5929-2017, 2017. a
Ramsauer, T., Weiß, T., Löw, A., and Marzahn, P.: RADOLAN_API: An Hourly Soil Moisture Data Set Based on Weather Radar, Soil Properties and Reanalysis Temperature Data, Remote Sens., 13, 1712, https://doi.org/10.3390/rs13091712, 2021. a
Raoult, N., Ottlé, C., Peylin, P., Bastrikov, V., and Maugis, P.: Evaluating and Optimizing Surface Soil Moisture Drydowns in the ORCHIDEE Land Surface Model at In Situ Locations, J. Hydrometeorol., 22, 1025–1043, https://doi.org/10.1175/JHM-D-20-0115.1, 2021. a
Rautiainen, K., Parkkinen, T., Lemmetyinen, J., Schwank, M., Wiesmann, A., Ikonen, J., Derksen, C., Davydov, S., Davydova, A., Boike, J., Langer, M., Drusch, M., and Pulliainen, J.: SMOS prototype algorithm for detecting autumn soil freezing, Remote Sens. Environ., 180, 346–360, https://doi.org/10.1016/j.rse.2016.01.012, 2016. a, b
Reichle, R. H., Koster, R. D., Dong, J., and Berg, A. A.: Global Soil Moisture from Satellite Observations, Land Surface Models, and Ground Data: Implications for Data Assimilation, J. Hydrometeorol., 5, 430–442, https://doi.org/10.1175/1525-7541(2004)005<0430:GSMFSO>2.0.CO;2, 2004. a
Reichle, R. H., Draper, C. S., Liu, Q., Girotto, M., Mahanama, S. P. P., Koster, R. D., and De Lannoy, G. J. M.: Assessment of MERRA-2 Land Surface Hydrology Estimates, J. Climate, 30, 2937–2960, https://doi.org/10.1175/JCLI-D-16-0720.1, 2017. a, b
Reimer, A. C., Melzer, B. T., Kidd, C. R., and Wagner, D. W.: Validation of the enhanced resolution ERS-2 scatterometer soil moisture product, in: 2012 IEEE International Geoscience and Remote Sensing Symposium, 22–27 July 2012, Munich, Germany, 1208–1211, https://doi.org/10.1109/IGARSS.2012.6351330, 2012. a
Rinollo, A., Vulpiani, G., Puca, S., Pagliara, P., Kaňák, J., Labo, E., Okon, L., Roulin, E., Baguis, P., Cattani, E., Laviola, S., and Levizzani, V.: Definition and impact of a quality index for radar-based reference measurements in the H-SAF precipitation product validation, Nat. Hazards Earth Syst. Sci., 13, 2695–2705, https://doi.org/10.5194/nhess-13-2695-2013, 2013. a
Robinson, D. A., Campbell, C. S., Hopmans, J. W., Hornbuckle, B. K., Jones, S. B., Knight, R., Ogden, F., Selker, J., and Wendroth, O.: Soil Moisture Measurement for Ecological and Hydrological Watershed-Scale Observatories: A Review, Vadose Zone J., 7, 358–389, https://doi.org/10.2136/vzj2007.0143, 2008. a
Robock, A., Vinnikov, K., Srinivasan, G., Entin, J., Hollinger, S., Speranskaya, N., Liu, S., and Namkhai, A.: The Global Soil Moisture Data Bank, B. Am. Meteoro. Soc., 81, 1281–1300, https://doi.org/10.1175/1520-0477(2000)081<1281:TGSMDB>2.3.CO;2, 2000. a, b, c, d, e
Rodionova, N.: Correlation of the Sentinel 1 Radar Data with Ground-Based Measurements of the Soil Temperature and Moisture, Izvest. Atmos. Ocean. Phys., 55, 939–948, 2019a. a
Rodionova, N. V.: Backscattering from the near-surface layer of Thawed/Frozen Soils of Alaska from Sentinel 1 Radar Data, Rensit, 11, 21–30, https://doi.org/10.17725/rensit.2019.11.021, 2019b. a, b
Rodriguez-Fernandez, N., Aires, F., Richaume, P., Kerr, Y., Prigent, C., Kolassa, J., Cabot, F., Jimenez, C., Mahmoodi, A., and Drusch, M.: Soil Moisture Retrieval Using Neural Networks: Application to SMOS, IEEE T. Geosci. Remote, 53, 5991–6007, https://doi.org/10.1109/TGRS.2015.2430845, 2015. a, b
Rodriguez-Fernandez, N., de Rosnay, P., Albergel, C., Richaume, P., Aires, F., Prigent, C., and Kerr, Y.: SMOS Neural Network Soil Moisture Data Assimilation in a Land Surface Model and Atmospheric Impact, Remote Sens., 11, 1334, https://doi.org/10.3390/rs11111334, 2019. a, b
Romano, N.: Soil moisture at local scale: Measurements and simulations, J. Hydrol., 516, 6–20, https://doi.org/10.1016/j.jhydrol.2014.01.026, 2014. a, b, c
Rüdiger, C., Hancock, G., Hemakumara, H., Jacobs, B., Kalma, J., Martinez, C., Thyer, M., Walker, J., Wells, T., and Willgoose, G.: Goulburn River experimental catchment data set, Water Resour. Res., 43, W10403, https://doi.org/10.1029/2006WR005837, 2007. a, b
Sadeghi, M., Gao, L., Ebtehaj, A., Wigneron, J.-P., Crow, W. T., Reager, J. T., and Warrick, A. W.: Retrieving global surface soil moisture from GRACE satellite gravity data, J. Hydrol., 584, 124717, https://doi.org/10.1016/j.jhydrol.2020.124717, 2020. a
Sadri, S., Pan, M., Wada, Y., Vergopolan, N., Sheffield, J., Famiglietti, J. S., Kerr, Y., and Wood, E.: A global near-real-time soil moisture index monitor for food security using integrated SMOS and SMAP, Remote Sens. Environ., 246, 111864, https://doi.org/10.1016/j.rse.2020.111864, 2020. a, b, c
Sanchez, N., Martinez-Fernandez, J., Scaini, A., and Perez-Gutierrez, C.: Validation of the SMOS L2 Soil Moisture Data in the REMEDHUS Network (Spain), IEEE T. Geosci. Remote, 50, 1602–1611, https://doi.org/10.1109/TGRS.2012.2186971, 2012. a
Santi, E., Paloscia, S., Pettinato, S., Brocca, L., and Ciabatta, L.: Robust Assessment of an Operational Algorithm for the Retrieval of Soil Moisture From AMSR-E Data in Central Italy, IEEE J. Select. Top. Appl. Earth Obs. Remote Sens., 9, 2478–2492, 2016. a
Santi, E., Paloscia, S., Pettinato, S., Brocca, L., Ciabatta, L., and Entekhabi, D.: Integration of microwave data from SMAP and AMSR2 for soil moisture monitoring in Italy, Remote Sens. Environ., 212, 21–30, https://doi.org/10.1016/j.rse.2018.04.039, 2018. a, b
Sawada, Y.: Quantifying Drought Propagation from Soil Moisture to Vegetation Dynamics Using a Newly Developed Ecohydrological Land Reanalysis, Remote Sens., 10, 1197, https://doi.org/10.3390/rs10081197, 2018. a
Scaini, A., Sánchez, N., Vicente-Serrano, S. M., and Martínez-Fernández, J.: SMOS-derived soil moisture anomalies and drought indices: a comparative analysis using in situ measurements, Hydrol. Process., 29, 373–383, https://doi.org/10.1002/hyp.10150, 2015. a, b
Scanlon, T.: Validation of ESA CCI SM combined v04.7 vs ESA CCI SM combined v05.2 vs ISMN 20191211 global, Zenodo [data set], https://doi.org/10.5281/zenodo.4120205, 2020. a
Scanlon, T., Dorigo, W., Baum, D., Preimesberger, W., Buttinger, P., Bakcsa, Z., van der Schalie, R., Reimer, C., Hirschi, M., Kidd, R., de Jeu, R., and Boresch, A.: ESA CCI and C3S Soil Moisture-Recent advances and quality assurance based on the online validation platform QA4SM, in: EGU General Assembly Conference Abstracts, EGU General Assembly Conference Abstracts, p. 14629, available at: https://ui.adsabs.harvard.edu/abs/2019EGUGA..2114629S (last access: 1 November 2021), 2019. a, b
Schaefer, G., Cosh, M., and Jackson, T.: The USDA natural resources conservation service soil climate analysis network (SCAN), J. Atmos. Ocean. Tech., 24, 2073–2077, https://doi.org/10.1175/2007JTECHA930.1, 2007. a
Schellekens, J., Dutra, E., Martínez-de la Torre, A., Balsamo, G., van Dijk, A., Sperna Weiland, F., Minvielle, M., Calvet, J.-C., Decharme, B., Eisner, S., Fink, G., Flörke, M., Peßenteiner, S., van Beek, R., Polcher, J., Beck, H., Orth, R., Calton, B., Burke, S., Dorigo, W., and Weedon, G. P.: A global water resources ensemble of hydrological models: the eartH2Observe Tier-1 dataset, Earth Syst. Sci. Data, 9, 389–413, https://doi.org/10.5194/essd-9-389-2017, 2017. a
Schlenz, F., dall'Amico, J. T., Loew, A., and Mauser, W.: Uncertainty Assessment of the SMOS Validation in the Upper Danube Catchment, IEEE T. Geosci. Remote, 50, 1517–1529, 2012a. a
Schlenz, F., dall'Amico, J. T., Mauser, W., and Loew, A.: Analysis of SMOS brightness temperature and vegetation optical depth data with coupled land surface and radiative transfer models in Southern Germany, Hydrol. Earth Syst. Sci., 16, 3517–3533, https://doi.org/10.5194/hess-16-3517-2012, 2012b. a
Scholze, M., Kaminski, T., Knorr, W., Blessing, S., Vossbeck, M., Grant, J., and Scipal, K.: Simultaneous assimilation of SMOS soil moisture and atmospheric CO2 in-situ observations to constrain the global terrestrial carbon cycle, Remote Sens. Environ., 180, 334–345, https://doi.org/10.1016/j.rse.2016.02.058, 2016. a, b
Senanayake, I., Yeo, I.-Y., Tangdamrongsub, N., Willgoose, G., Hancock, G., Wells, T., Fang, B., Lakshmi, V., and Walker, J.: An in-situ data based model to downscale radiometric satellite soil moisture products in the Upper Hunter Region of NSW, Australia, J. Hydrol., 572, 820–838, https://doi.org/10.1016/j.jhydrol.2019.03.014, 2019. a
Senyurek, V., Lei, F., Boyd, D., Kurum, M., Gurbuz, A. C., and Moorhead, R.: Machine Learning-Based CYGNSS Soil Moisture Estimates over ISMN sites in CONUS, Remote Sens., 12, 1168, https://doi.org/10.3390/rs12071168, 2020. a
Sheng, J., Rao, P., and Ma, H.: Spatial Downscaling of the FY3B Soil Moisture Using Random Forest Regression, in: 2019 8th International Conference on Agro-Geoinformatics (Agro-Geoinformatics), 16–19 July 2019, Istanbul, Turkey, 1–6, 2019. a, b
Shin, Y., Lim, K., Park, K., and Jung, Y.: Development of Dynamic Ground Water Data Assimilation for Quantifying Soil Hydraulic Properties from Remotely Sensed Soil Moisture, Water, 8, 311, https://doi.org/10.3390/w8070311, 2016. a
Shin, Y., Mohanty, B. P., and Ines, A. V.: Development of non-parametric evolutionary algorithm for predicting soil moisture dynamics, J. Hydrol., 564, 208–221, https://doi.org/10.1016/j.jhydrol.2018.07.003, 2018. a
Shuman, D. I., Nayyar, A., Mahajan, A., Goykhman, Y., Li, K., Liu, M., Teneketzis, D., Moghaddam, M., and Entekhabi, D.: Measurement Scheduling for Soil Moisture Sensing: From Physical Models to Optimal Control, Proc. IEEE, 98, 1918–1933, https://doi.org/10.1109/JPROC.2010.2052532, 2010. a
Skierucha, W., Sławiński, C., Wilczek, A., Żyromski, A., and Biniak-Pieróg, M.: Telemetric system for the measurement of soil moisture based on the TDR technique, Woda Środowisko Obszary Wiejskie, 12, 257–267, 2012a. a
Skierucha, W., Wilczek, A., Szypłowska, A., Sławiński, C., and Lamorski, K.: A TDR-Based Soil Moisture Monitoring System with Simultaneous Measurement of Soil Temperature and Electrical Conductivity, Sensors, 12, 13545–13566, https://doi.org/10.3390/s121013545, 2012b. a
Smith, A., Walker, J., Western, A., Young, R., Ellett, K., Pipunic, R., Grayson, R., Siriwardena, L., Chiew, F., and Richter, H.: The Murrumbidgee Soil Moisture Monitoring Network data set, Water Resour. Res., 48, W07701, https://doi.org/10.1029/2012WR011976, 2012. a
Solander, K. C., Newman, B. D., Carioca de Araujo, A., Barnard, H. R., Berry, Z. C., Bonal, D., Bretfeld, M., Burban, B., Antonio Candido, L., Célleri, R., Chambers, J. Q., Christoffersen, B. O., Detto, M., Dorigo, W. A., Ewers, B. E., José Filgueiras Ferreira, S., Knohl, A., Leung, L. R., McDowell, N. G., Miller, G. R., Terezinha Ferreira Monteiro, M., Moore, G. W., Negron-Juarez, R., Saleska, S. R., Stiegler, C., Tomasella, J., and Xu, C.: The pantropical response of soil moisture to El Niño, Hydrol. Earth Syst. Sci., 24, 2303–2322, https://doi.org/10.5194/hess-24-2303-2020, 2020. a, b, c
Steele-Dunne, S. C., Hahn, S., Wagner, W., and Vreugdenhil, M.: Towards Including Dynamic Vegetation Parameters in the EUMETSAT H SAF ASCAT Soil Moisture Products, Remote Sens., 13, 1463, https://doi.org/10.3390/rs13081463, 2021. a
Su, C.-H., Narsey, S. Y., Gruber, A., Xaver, A., Chung, D., Ryu, D., and Wagner, W.: Evaluation of post-retrieval de-noising of active and passive microwave satellite soil moisture, Remote Sens. Environ., 163, 127–139, https://doi.org/10.1016/j.rse.2015.03.010, 2015. a, b
Su, C.-H., Ryu, D., Dorigo, W., Zwieback, S., Gruber, A., Albergel, C., Reichle, R. H., and Wagner, W.: Homogeneity of a global multisatellite soil moisture climate data record, Geophys. Res. Lett., 43, 11245–11252, https://doi.org/10.1002/2016GL070458, 2016. a
Su, Y., Chen, X., Su, H., Liu, L., and Liao, J.: Digitizing the thermal and hydrological parameters of land surface in subtropical China using AMSR-E brightness temperatures, Int. J. Digit. Earth, 10, 687–700, https://doi.org/10.1080/17538947.2016.1247472, 2017. a
Su, Z., Wen, J., Dente, L., van der Velde, R., Wang, L., Ma, Y., Yang, K., and Hu, Z.: The Tibetan Plateau observatory of plateau scale soil moisture and soil temperature (Tibet-Obs) for quantifying uncertainties in coarse resolution satellite and model products, Hydrol. Earth Syst. Sci., 15, 2303–2316, https://doi.org/10.5194/hess-15-2303-2011, 2011. a, b, c
Suman, S., Srivastava, P. K., Petropoulos, G. P., Pandey, D. K., and O'Neill, P. E.: Appraisal of SMAP Operational Soil Moisture Product from a Global Perspective, Remote Sens., 12, 1977, https://doi.org/10.3390/rs12121977, 2020. a
Sun, H. and Cui, Y.: Evaluating Downscaling Factors of Microwave Satellite Soil Moisture Based on Machine Learning Method, Remote Sens., 13, 133, https://doi.org/10.3390/rs13010133, 2021. a, b
Sun, H., Zhou, B., Zhang, C., Liu, H., and Yang, B.: DSCALE_mod16: A Model for Disaggregating Microwave Satellite Soil Moisture with Land Surface Evapotranspiration Products and Gridded Meteorological Data, Remote Sens., 12, 980, https://doi.org/10.3390/rs12060980, 2020. a
Sure, A., Varade, D., and Dikshit, O.: Factors determining spatio-temporal variations of soil moisture using microwave data, in: 2017 International Conference on Emerging Trends in Computing and Communication Technologies (ICETCCT), 17–18 November 2017, Dehradun, India, 1–5, https://doi.org/10.1109/ICETCCT.2017.8280301, 2017. a, b
Szczypta, C., Calvet, J.-C., Maignan, F., Dorigo, W., Baret, F., and Ciais, P.: Suitability of modelled and remotely sensed essential climate variables for monitoring Euro-Mediterranean droughts, Geosci. Model Dev., 7, 931–946, https://doi.org/10.5194/gmd-7-931-2014, 2014. a
Tagesson, T., Fensholt, R., Guiro, I., Rasmussen, M., Huber, S., Mbow, C., Garcia, M., Horion, S., Sandholt, I., Holm-Rasmussen, B., Göttsche, F.-M., Ridler, M., Boke-Olén, N., Olsen, J., Ehammer, A., Madsen, M., Olesen, F., and Ardö, J.: Ecosystem properties of semi-arid savanna grassland in West Africa and its relationship to environmental variability, Global Change Biol., 21, 250–264, https://doi.org/10.1111/gcb.12734, 2014. a
Tagesson, T., Horion, S., Nieto, H., Zaldo Fornies, V., Mendiguren González, G., Bulgin, C., Ghent, D., and Fensholt, R.: Disaggregation of SMOS soil moisture over West Africa using the Temperature and Vegetation Dryness Index based on SEVIRI land surface parameters, Remote Sens. Environ., 206, 424–441, https://doi.org/10.1016/j.rse.2017.12.036, 2018. a, b
Teuling, A. J., Uijlenhoet, R., Hupet, F., van Loon, E. E., and Troch, P. A.: Estimating spatial mean root-zone soil moisture from point-scale observations, Hydrol. Earth Syst. Sci., 10, 755–767, https://doi.org/10.5194/hess-10-755-2006, 2006. a
Tian, J., Zhang, B., He, C., Han, Z., Bogena, H. R., and Huisman, J. A.: Dynamic response patterns of profile soil moisture wetting events under different land covers in the Mountainous area of the Heihe River Watershed, Northwest China, Agr. Forest Meteorol., 271, 225–239, 2019. a
Tian, S.: Monitoring and forecasting drought through the assimilation of satellite water observations, PhD thesis, Research School of Earth Sciences, The Australian National University, available at: https://openresearch-repository.anu.edu.au/handle/1885/157222 (last access: 1 November 2021), 2018. a
Tian, S., Renzullo, L. J., van Dijk, A. I. J. M., Tregoning, P., and Walker, J. P.: Global joint assimilation of GRACE and SMOS for improved estimation of root-zone soil moisture and vegetation response, Hydrol. Earth Syst. Sci., 23, 1067–1081, https://doi.org/10.5194/hess-23-1067-2019, 2019. a
Tobin, K. J., Torres, R., Crow, W. T., and Bennett, M. E.: Multi-decadal analysis of root-zone soil moisture applying the exponential filter across CONUS, Hydrol. Earth Syst. Sci., 21, 4403–4417, https://doi.org/10.5194/hess-21-4403-2017, 2017. a, b, c
Vachaud, G., Passerat De Silans, A., Balabanis, P., and Vauclin, M.: Temporal Stability of Spatially Measured Soil Water Probability Density Function, Soil Sci. Soc. Am. J., 49, 822–828, https://doi.org/10.2136/sssaj1985.03615995004900040006x, 1985. a
Van Cleve, K., Chapin, F. S. S., and Ruess, R. W.: Bonanza Creek Long Term Ecological Research Project Climate Database, University of Alaska, Fairbanks, available at: http://www.lter.uaf.edu/ (last access: 3 November 2021), 2015. a
van der Schalie, R., Kerr, Y., Wigneron, J., Rodríguez-Fernández, N., Al-Yaari, A., and Jeu, R.: Global SMOS Soil Moisture Retrievals from The Land Parameter Retrieval Model, Int. J. Appl. Earth Obs. Geoinf., 45, 125–134, https://doi.org/10.1016/j.jag.2015.08.005, 2016. a
van der Schalie, R., de Jeu, R., Kerr, Y., Wigneron, J., Rodríguez-Fernández, N., Al-Yaari, A., Parinussa, R., Mecklenburg, S., and Drusch, M.: The merging of radiative transfer based surface soil moisture data from SMOS and AMSR-E, Remote Sens. Environ., 189, 180–193, https://doi.org/10.1016/j.rse.2016.11.026, 2017. a, b
van der Schalie, R., de Jeu, R., Parinussa, R., Rodríguez-Fernández, N., Kerr, Y., Al-Yaari, A., Wigneron, J.-P., and Drusch, M.: The Effect of Three Different Data Fusion Approaches on the Quality of Soil Moisture Retrievals from Multiple Passive Microwave Sensors, Remote Sens., 10, 107, https://doi.org/10.3390/rs10010107, 2018. a, b
van der Schalie, R., van der Vliet, M., Rodríguez-Fernández, N., Dorigo, W. A., Scanlon, T., Preimesberger, W., Madelon, R., and de Jeu, R. A. M.: L-Band Soil Moisture Retrievals Using Microwave Based Temperature and Filtering. Towards Model-Independent Climate Data Records, Remote Sens., 13, 2480, https://doi.org/10.3390/rs13132480, 2021. a, b, c
Van doninck, J., Peters, J., Lievens, H., De Baets, B., and Verhoest, N.: Accounting for seasonality in a soil moisture change detection algorithm for ASAR Wide Swath time series, Hydrol. Earth Syst. Sci., 16, 773–786, https://doi.org/10.5194/hess-16-773-2012, 2012. a
Verrier, S.: Multifractal and multiscale entropy scaling of in-situ soil moisture time series: Study of SMOSMANIA network data, southwestern France, J. Hydrol., 585, 124821, https://doi.org/10.1016/j.jhydrol.2020.124821, 2020. a
Vicente-Serrano, S. M., Beguería, S., Lorenzo-Lacruz, J., Camarero, J. J., López-Moreno, J. I., Azorin-Molina, C., Revuelto, J., Morán-Tejeda, E., and Sanchez-Lorenzo, A.: Performance of Drought Indices for Ecological, Agricultural, and Hydrological Applications, Earth Interact., 16, 1–27, https://doi.org/10.1175/2012EI000434.1, 2012. a
Vreugdenhil, M., Dorigo, W., Broer, M., Haas, P., Eder, A., Hogan, P., Bloeschl, G., and Wagner, W.: Towards a high-density soil moisture network for the validation of SMAP in Petzenkirchen, Austria, in: 2013 IEEE International Geoscience and Remote Sensing Symposium – IGARSS, 21–26 July 2013, Melbourne, VIC, Australia, 1865–1868, https://doi.org/10.1109/IGARSS.2013.6723166, 2013. a
Wanders, N., Karssenberg, D., Bierkens, M., Parinussa, R., de Jeu, R., van Dam, J., and de Jong, S.: Observation uncertainty of satellite soil moisture products determined with physically-based modeling, Remote Sens. Environ., 127, 341–356, https://doi.org/10.1016/j.rse.2012.09.004, 2012. a, b, c
Wang, L., Li, X., Chen, Y., Yang, K., Chen, D., Zhou, J., Liu, W., Qi, J., and Huang, J.: Validation of the global land data assimilation system based on measurements of soil temperature profiles, Agr. Forest Meteorol., 218–219, 288–297, https://doi.org/10.1016/j.agrformet.2016.01.003, 2016. a
Wang, L., He, B., Bai, X., and Xing, M.: Assessment of different vegetation parameters for parameterizing the coupled water cloud model and advanced integral equation model for soil moisture retrieval using time series Sentinel-1A data, Photogram. Eng. Remote Sens., 85, 43–54, 2019a. a
Wang, L., Xie, Z., Jia, B., Xie, J., Wang, Y., Liu, B., Li, R., and Chen, S.: Contributions of climate change and groundwater extraction to soil moisture trends, Earth Syst. Dynam., 10, 599–615, https://doi.org/10.5194/esd-10-599-2019, 2019b. a
Wang, L., Fang, S., Pei, Z., Zhu, Y., Khoi, D. N., and Han, W.: Using FengYun-3C VSM Data and Multivariate Models to Estimate Land Surface Soil Moisture, Remote Sens., 12, 1038, https://doi.org/10.3390/rs12061038, 2020. a
Wang, R., Zhu, Q., Ma, H., and Wang, Y.: Spatial and temporal variations of the surface soil moisture in the source re-gion of the Yellow River from 2003 to 2010 based on AMSR-E[J], Sci. Soil Water Conserv., 15, 22–32, https://doi.org/10.16843/j.sswc.2017.01.004, 2017, 2017. a
Wang, T., Franz, T. E., Li, R., You, J., Shulski, M. D., and Ray, C.: Evaluating climate and soil effects on regional soil moisture spatial variability using EOFs, Water Resour. Res., 53, 4022–4035, https://doi.org/10.1002/2017WR020642, 2017. a
Wang, Y., Yang, J., Chen, Y., Fang, G., Duan, W., Li, Y., and De Maeyer, P.: Quantifying the Effects of Climate and Vegetation on Soil Moisture in an Arid Area, China, Water, 11, 767, https://doi.org/10.3390/w11040767, 2019. a
Wang, Y., Shi, L., Lin, L., Holzman, M., Carmona, F., and Zhang, Q.: A robust data-worth analysis framework for soil moisture flow by hybridizing sequential data assimilation and machine learning, Vadose Zone J., 19, e20026, https://doi.org/10.1002/vzj2.20026, 2020. a
Wang, Y., Leng, P., Peng, J., Marzahn, P., and Ludwig, R.: Global assessments of two blended microwave soil moisture products CCI and SMOPS with in-situ measurements and reanalysis data, Int. J. Appl.Earth Obs. Geoinf., 94, 102234, https://doi.org/10.1016/j.jag.2020.102234, 2021. a, b
Western, A. W., Grayson, R. B., and Blöschl, G.: Scaling of Soil Moisture: A Hydrologic Perspective, Annu. Rev. Earth Planet. Sci., 30, 149–180, https://doi.org/10.1146/annurev.earth.30.091201.140434, 2002. a
Wigneron, J.-P., Dayan, S., Kruszewski, A., Aluome, C., Al-Yaari, A., Fan, L., Guven, S., Chipeaux, C., Moisy, C., Guyon, D., and Loustau, D.: The Aqui Network: Soil Moisture Sites in the “Les Landes” Forest and Graves Vineyards (Bordeaux Aquitaine Region, France), in: IGARSS 2018 – 2018 IEEE International Geoscience and Remote Sensing Symposium, 22–27 Juy 2018, Valencia, Spain, 3739–3742, https://doi.org/10.1109/IGARSS.2018.8517392, 2018. a
Wigneron, J.-P., Li, X., Frappart, F., Fan, L., Al-Yaari, A., De Lannoy, G., Liu, X., Wang, M., Le Masson, E., and Moisy, C.: SMOS-IC data record of soil moisture and L-VOD: Historical development, applications and perspectives, Remote Sens. Environ., 254, 112238, https://doi.org/10.1016/j.rse.2020.112238, 2021. a
Wilkinson, M. D., Dumontier, M., Aalbersberg, I. J., Appleton, G., Axton, M., Baak, A., Blomberg, N., Boiten, J.-W., da Silva Santos, L. B., Bourne, P. E., Bouwman, J., Brookes, A. J., Clark, T., Crosas, M., Dillo, I., Dumon, O., Edmunds, S., Evelo, C. T., Finkers, R., Gonzalez-Beltran, A., Gray, A. J., Groth, P., Goble, C., Grethe, J. S., Heringa, J., 't Hoen, P. A., Hooft, R., Kuhn, T., Kok, R., Kok, J., Lusher, S. J., Martone, M. E., Mons, A., Packer, A. L., Persson, B., Rocca-Serra, P., Roos, M., van Schaik, R., Sansone, S.-A., Schultes, E., Sengstag, T., Slater, T., Strawn, G., Swertz, M. A., Thompson, M., van der Lei, J., van Mulligen, E., Velterop, J., Waagmeester, A., Wittenburg, P., Wolstencroft, K., Zhao, J., and Mons, B.: The FAIR Guiding Principles for scientific data management and stewardship, Scient. Data, 3, 160018, https://doi.org/10.1038/sdata.2016.18, 2016. a
Williams, S.: The Coordinated Energy and Water cycle Observations Project (CEOP) Data Integration Approach, EMS, p. 798, available at: https://ui.adsabs.harvard.edu/abs/2010ems..confE.798W (last access: 3 November 2021), 2010. a, b
Wu, K., Ryu, D., Nie, L., and Shu, H.: Time-variant error characterization of SMAP and ASCAT soil moisture using Triple Collocation Analysis, Remote Sens. Environ., 256, 112324, https://doi.org/10.1016/j.rse.2021.112324, 2021. a, b, c, d
Wu, M., Scholze, M., Voßbeck, M., Kaminski, T., and Hoffmann, G.: Simultaneous Assimilation of Remotely Sensed Soil Moisture and FAPAR for Improving Terrestrial Carbon Fluxes at Multiple Sites Using CCDAS, Remote Sens., 11, 27, https://doi.org/10.3390/rs11010027, 2018. a
Wu, Q., Liu, H., Wang, L., and Deng, C.: Evaluation of AMSR2 soil moisture products over the contiguous United States using in situ data from the International Soil Moisture Network, Int. J. Appl. Earth Obs. Geoinf., 45, 187–199, https://doi.org/10.1016/j.jag.2015.10.011, 2016. a
Xaver, A., Zappa, L., Rab, G., Pfeil, I., Vreugdenhil, M., Hemment, D., and Dorigo, W. A.: Evaluating the suitability of the consumer low-cost Parrot Flower Power soil moisture sensor for scientific environmental applications, Geosci. Instrum. Method. Data Syst., 9, 117–139, https://doi.org/10.5194/gi-9-117-2020, 2020. a, b, c, d, e, f
Xia, Y., Ford, T. W., Wu, Y., Quiring, S. M., and Ek, M. B.: Automated Quality Control of In Situ Soil Moisture from the North American Soil Moisture Database Using NLDAS-2 Products, J. Appl. Meteorol. Clim., 54, 1267–1282, https://doi.org/10.1175/JAMC-D-14-0275.1, 2015. a
Xia, Y., Hao, Z., Shi, C., Li, Y., Meng, J., Xu, T., Wu, X., and Zhang, B.: Regional and Global Land Data Assimilation Systems: Innovations, Challenges, and Prospects, J. Meteorol. Res., 33, 159–189, https://doi.org/10.1007/s13351-019-8172-4, 2019. a, b
Xie, Q., Menenti, M., and Jia, L.: Improving the AMSR-E/NASA Soil Moisture Data Product Using In-Situ Measurements from the Tibetan Plateau, Remote Sens., 11, 2748, https://doi.org/10.3390/rs11232748, 2019. a
Xing, C., Chen, N., Zhang, X., and Gong, J.: A Machine Learning Based Reconstruction Method for Satellite Remote Sensing of Soil Moisture Images with In Situ Observations, Remote Sens., 9, 484, https://doi.org/10.3390/rs9050484, 2017. a, b
Xu, H., Yuan, Q., Li, T., Shen, H., Zhang, L., and Jiang, H.: Quality Improvement of Satellite Soil Moisture Products by Fusing with In-Situ Measurements and GNSS-R Estimates in the Western Continental U.S., Remote Sens., 10, 1351, https://doi.org/10.3390/rs10091351, 2018. a, b, c
Xu, L., Chen, N., Zhang, X., Moradkhani, H., Zhang, C., and Hu, C.: In-situ and triple-collocation based evaluations of eight global root zone soil moisture products, Remote Sens. Environ., 254, 112248, https://doi.org/10.1016/j.rse.2020.112248, 2021. a, b, c, d, e, f, g
Yang, K., Qin, J., Zhao, L., Chen, Y., Tang, W., Han, M., Zhu, l., Chen, Z., Lv, N., Ding, B., Wu, H., and Lin, C.: A Multi-Scale Soil Moisture and Freeze-Thaw Monitoring Network on the Third Pole, B. Am. Meteorol. Soc., 94, 1907–1916, https://doi.org/10.1175/BAMS-D-12-00203.1, 2013. a
Yao, P., Shi, J., Zhao, T., Lu, H., and Al-Yaari, A.: Rebuilding Long Time Series Global Soil Moisture Products Using the Neural Network Adopting the Microwave Vegetation Index, Remote Sens., 9, 35, https://doi.org/10.3390/rs9010035, 2017. a, b, c
Yao, P., Lu, H., Shi, J., Zhao, T., Yang, K., Cosh, M. H., Gianotti, D. J. S., and Entekhabi, D.: A long term global daily soil moisture dataset derived from AMSR-E and AMSR2 (2002–2019), Scient. Data, 8, 1–16, https://doi.org/10.1038/s41597-021-00925-8, 2021. a, b, c, d, e
Yin, Z., Ottlé, C., Ciais, P., Guimberteau, M., Wang, X., Zhu, D., Maignan, F., Peng, S., Piao, S., Polcher, J., Zhou, F., Kim, H., and other China-Trend-Stream project members: Evaluation of ORCHIDEE-MICT-simulated soil moisture over China and impacts of different atmospheric forcing data, Hydrol. Earth Syst. Sci., 22, 5463–5484, https://doi.org/10.5194/hess-22-5463-2018, 2018. a
Young, R., Walker, J., Yeoh, N., Smith, A., Ellett, K., Merlin, O., and Western, A.: Soil moisture and meteorological observations from the Murrumbidgee catchment, Department of Civil and Environmental Engineering, The University of Melbourne, Melbourne, 2008. a
Zacharias, S., Bogena, H., Samaniego, L., Mauder, M., Fuß, R., Pütz, T., Frenzel, M., Schwank, M., Baessler, C., Butterbach-Bahl, K., Bens, O., Borg, E., Brauer, A., Dietrich, P., Hajnsek, I., Helle, G., Kiese, R., Kunstmann, H., Klotz, S., and Vereecken, H.: A Network of Terrestrial Environmental Observatories in Germany, Vadose Zone J., 10, 955–973, https://doi.org/10.2136/vzj2010.0139, 2011. a
Zappa, L., Forkel, M., Xaver, A., and Dorigo, W.: Deriving Field Scale Soil Moisture from Satellite Observations and Ground Measurements in a Hilly Agricultural Region, Remote Sens., 11, 2596, https://doi.org/10.3390/rs11222596, 2019. a, b, c
Zappa, L., Woods, M., Hemment, D., Xaver, A., and Dorigo, W.: Evaluation of Remotely Sensed Soil Moisture Products using Crowdsourced Measurements, in: Eighth International Conference on Remote Sensing and Geoinformation of Environment, SPIE, Cyprus, 2020. a, b, c, d, e, f, g
Zawadzki, J. and Kędzior, M.: Soil moisture variability over Odra watershed: Comparison between SMOS and GLDAS data, Int. J. Appl. Earth Obs. Geoinf., 45, 110–124, https://doi.org/10.1016/j.jag.2015.03.005, 2016. a
Zeng, J., Li, Z., Quan, C., and Bi, H.: A simplified physically-based algorithm for surface soil moisture retrieval using AMSR-E data, Front. Earth Sci., 8, 427–438, https://doi.org/10.1007/s11707-014-0412-4, 2014. a, b
Zeng, J., Chen, K., Bi, H., and Chen, Q.: A Preliminary Evaluation of the SMAP Radiometer Soil Moisture Product Over United States and Europe Using Ground-Based Measurements, IEEE T. Geosci. Remote, 54, 4929–4940, 2016. a
Zhang, Q., Shi, L., Holzman, M., Ye, M., Wang, Y., Carmona, F., and Zha, Y.: A dynamic data-driven method for dealing with model structural error in soil moisture data assimilation, Adv. Water Resour., 132, 103407, https://doi.org/10.1016/j.advwatres.2019.103407, 2019. a
Zhang, Q., Yuan, Q., Li, J., Wang, Y., Sun, F., and Zhang, L.: Generating seamless global daily AMSR2 soil moisture (SGD-SM) long-term products for the years 2013–2019, Earth Syst. Sci. Data, 13, 1385–1401, https://doi.org/10.5194/essd-13-1385-2021, 2021. a
Zhang, R., Kim, S., and Sharma, A.: A comprehensive validation of the SMAP Enhanced Level-3 Soil Moisture product using ground measurements over varied climates and landscapes, Remote Sens. Environ., 223, 82–94, 2019. a
Zhang, R., Kim, S., Sharma, A., and Lakshmi, V.: Identifying relative strengths of SMAP, SMOS-IC, and ASCAT to capture temporal variability, Remote Sens. Environ., 252, 112126, https://doi.org/10.1016/j.rse.2020.112126, 2021. a, b, c
Zhang, S., Calvet, J.-C., Darrozes, J., Roussel, N., Frappart, F., and Bouhours, G.: Deriving surface soil moisture from reflected GNSS signal observations from a grassland site in southwestern France, Hydrol. Earth Syst. Sci., 22, 1931–1946, https://doi.org/10.5194/hess-22-1931-2018, 2018. a
Zhang, S., Meurey, C., and Calvet, J.-C.: Identification of soil-cooling rains in southern France from soil temperature and soil moisture observations, Atmos. Chem. Phys., 19, 5005–5020, https://doi.org/10.5194/acp-19-5005-2019, 2019. a
Zhang, X., Chen, N., Chen, Z., Wu, L., Li, X., Zhang, L., Di, L., Gong, J., and Li, D.: Geospatial sensor web: A cyber-physical infrastructure for geoscience research and application, Earth-Sci. Rev., 185, 684–703, 2018. a
Zhao, L. and Yang, Z.-L.: Multi-sensor land data assimilation: Toward a robust global soil moisture and snow estimation, Remote Sens. Environ., 216, 13–27, https://doi.org/10.1016/j.rse.2018.06.033, 2018. a, b
Zhao, L., Yang, Z.-L., and Hoar, T. J.: Global Soil Moisture Estimation by Assimilating AMSR-E Brightness Temperatures in a Coupled CLM4–RTM–DART System, J. Hydrometeorol., 17, 2431–2454, https://doi.org/10.1175/JHM-D-15-0218.1, 2016. a
Zhao, T., Shi, J., Lin, M., Yin, X., Liu, Y., Lan, H., and Xiong, C.: Potential soil moisture product from the Chinese HY-2 scanning microwave radiometer and its initial assessment, J. Appl. Remote Sens., 8, 1–12, https://doi.org/10.1117/1.JRS.8.083560, 2014. a, b
Zhao, W. and Li, A.: A Downscaling Method for Improving the Spatial Resolution of AMSR-E Derived Soil Moisture Product Based on MSG-SEVIRI Data, Remote Sens., 5, 6790–6811, https://doi.org/10.3390/rs5126790, 2013. a, b
Zhao, W. and Li, A.: A comparison study on empirical microwave soil moisture downscaling methods based on the integration of microwave-optical/IR data on the Tibetan Plateau, Int. J. Remote Sens., 36, 4986–5002, https://doi.org/10.1080/01431161.2015.1041178, 2015. a, b
Zhao, W., Li, A., Jin, H., Zhang, Z., Bian, J., and Yin, G.: Performance Evaluation of the Triangle-Based Empirical Soil Moisture Relationship Models Based on Landsat-5 TM Data and In Situ Measurements, IEEE T. Geosci. Remote, 55, 2632–2645, https://doi.org/10.1109/TGRS.2017.2649522, 2017. a, b
Zhao, W., Sánchez, N., Lu, H., and Li, A.: A spatial downscaling approach for the SMAP passive surface soil moisture product using random forest regression, J. Hydrol., 563, 1009–1024, https://doi.org/10.1016/j.jhydrol.2018.06.081, 2018. a
Zhu, L., Wang, H., Tong, C., Liu, W., and Du, B.: Evaluation of ESA Active, Passive and Combined Soil Moisture Products Using Upscaled Ground Measurements, Sensors, 19, 2718, https://doi.org/10.3390/s19122718, 2019. a
Zreda, M., Desilets, D., Ferré, T., and Scott, R.: Measuring soil moisture content non-invasively at intermediate spatial scale using cosmic-ray neutrons, Geophys. Res. Lett., 35, L21402, https://doi.org/10.1029/2008GL035655, 2008. a, b
Zreda, M., Shuttleworth, W. J., Zeng, X., Zweck, C., Desilets, D., Franz, T., and Rosolem, R.: COSMOS: the COsmic-ray Soil Moisture Observing System, Hydrol. Earth Syst. Sci., 16, 4079–4099, https://doi.org/10.5194/hess-16-4079-2012, 2012. a, b
Zwieback, S., Dorigo, W., and Wagner, W.: Estimation of the temporal autocorrelation structure by the collocation technique with an emphasis on soil moisture studies, Hydrolog. Sci. J., 58, 1729–1747, https://doi.org/10.1080/02626667.2013.839876, 2013. a, b, c
Zwieback, S., Paulik, C., and Wagner, W.: Frozen Soil Detection Based on Advanced Scatterometer Observations and Air Temperature Data as Part of Soil Moisture Retrieval, Remote Sens., 7, 3206–3231, https://doi.org/10.3390/rs70303206, 2015. a, b
Zwieback, S., Su, C.-H., Gruber, A., Dorigo, W. A., and Wagner, W.: The Impact of Quadratic Nonlinear Relations between Soil Moisture Products on Uncertainty Estimates from Triple Collocation Analysis and Two Quadratic Extensions, J. Hydrometeorol., 17, 1725–1743, https://doi.org/10.1175/JHM-D-15-0213.1, 2016. a
- Abstract
- Introduction
- The ISMN data hosting facility
- Quality control
- Impact of the ISMN on Earth system sciences
- Challenges and opportunities
- Conclusions
- Appendix A: Network overview
- Appendix B: Detailed system overview
- Appendix C: Soil moisture sensor availability per soil depth interval
- Appendix D: Metadata structure
- Appendix E: Scientific studies using ISMN data for satellite and model product evaluation and development.
- Appendix F: Effect of quality flagging on validation of ERA5 and ESA CCI soil moisture
- Code and data availability
- Author contributions
- Competing interests
- Disclaimer
- Acknowledgements
- Financial support
- Review statement
- References
- Abstract
- Introduction
- The ISMN data hosting facility
- Quality control
- Impact of the ISMN on Earth system sciences
- Challenges and opportunities
- Conclusions
- Appendix A: Network overview
- Appendix B: Detailed system overview
- Appendix C: Soil moisture sensor availability per soil depth interval
- Appendix D: Metadata structure
- Appendix E: Scientific studies using ISMN data for satellite and model product evaluation and development.
- Appendix F: Effect of quality flagging on validation of ERA5 and ESA CCI soil moisture
- Code and data availability
- Author contributions
- Competing interests
- Disclaimer
- Acknowledgements
- Financial support
- Review statement
- References