the Creative Commons Attribution 4.0 License.
the Creative Commons Attribution 4.0 License.
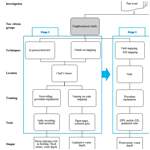
Reconstituting past flood events: the contribution of citizen science
Corine Frischknecht
Hy Dao
David Consuegra
Gregory Giuliani
Information gathered on past flood events is essential for understanding and assessing flood hazards. In this study, we present how citizen science can help to retrieve this information, particularly in areas with scarce or no authoritative measurements of past events. The case study is located in Yeumbeul North (YN), Senegal, where flood impacts represent a growing concern for the local community. This area lacks authoritative records on flood extent and water depth as well as information on the chain of causative factors. We developed a framework using two techniques to retrieve information on past flood events by involving two groups of citizens who were present during the floods. The first technique targeted the part of the citizens' memory that records information on events, recalled through narratives, whereas the second technique focused on scaling past flood event intensities using different parts of the witnesses' bodies. These techniques were used for three events that occurred in 2005, 2009 and 2012. They proved complementary by providing quantitative information on flood extents and water depths and by revealing factors that may have contributed to all three flood events.
- Article
(6350 KB) - Full-text XML
-
Supplement
(189 KB) - BibTeX
- EndNote
Together with Asia, Africa is the continent most affected by floods (UNISDR-CRED, 2015). Between 2000 and 2018, there were 698 flood disasters in Africa, killing more than 14 250, affecting 45 million people and causing USD 6.8 million of economic loss (EM-DAT, 2018). West African countries, such as Burkina Faso or Senegal, appear to be experiencing an increase in flood disasters due to population growth and the urbanisation of flood-prone areas (Di Baldassarre et al., 2010). Between 1990 and 2014, floods were responsible for 86 % of the economic loss from natural disasters in Senegal alone (Preventionweb, 2018). During that period, the years 2005, 2009 and 2012 were marked by severe urban floods, particularly affecting the capital of Senegal, Dakar, causing human casualties and impairing socioeconomic conditions (GFDRR, 2014). The country is facing enormous challenges in flood risk management, exacerbated by climate change (Douglas et al., 2008; Urama and Ozor, 2010), rapid and uncontrolled urbanisation, a lack of drainage infrastructure, and rapid changes in land use that worsen drainage patterns (Chen et al., 2015; Ahiablame and Shakya, 2016).
The government and local authorities of Senegal have tried several strategies to mitigate urban floods, such as developing emergency plans, relocating inhabitants and building water retention basins. However, two key aspects, required for these measures to work, have not yet been considered. First, it is necessary to understand the causes and characteristics of floods, and, secondly, the local population must be involved in the process of risk management. Information on the magnitude and intensity of flood events, as well as on processes controlling the flood, is at the core of flood hazard assessment and zoning (EXCIMAP, 2007). This fundamental information is scarcely available in the region (GFDRR, 2014; Sy et al., 2016). The absence of an organised data acquisition system during floods has led to the absence of a comprehensive catalogue on past flood events and consequently on flood hazard maps.
Without records of past events and without the possibility of capturing the temporal dimension in terms of the frequency of occurrence, accurate flood hazard assessment is impossible to achieve. Moreover, floods are not only triggered by natural factors, but they are frequently influenced by man-made processes (WMO, 2012; DAEC, 2016), which are not easily recorded by ground-based instruments (Townsend and Walsh, 1998) or remote sensing (RS; Sanyal and Lu, 2004). Consequently, new alternatives must be explored. Citizen science is a form of collaborative research involving citizens in scientific projects (Wiggins and Crowston, 2011). Citizen science has attracted much attention from scientists in many fields such as ecology (Dickinson et al., 2010; Silvertown, 2009), astronomy (Raddick et al., 2007) and more recently hydrology (Buytaert et al., 2014; Paul et al., 2018). Rapid advancements in various modern technologies – the internet, web 2.0, virtual globe, location-based services, social media, mobile devices, interactive geo-visualisation interfaces such as OpenStreetMap, Google Earth and Geo-Wiki (Fritz et al., 2009; Mooney and Minghini, 2017; Yu and Gong, 2012) – as well as the rise of participatory research characterised by greater user interactivity and collaboration, have increased the number of studies and subjects investigated by citizen science projects. The use of citizen science has also emerged in flood analysis in recent years. The existing works can be classified according to which phase of flood risk management they are dealing with, i.e. before, during or after the flood event. For example, Sy et al. (2019a) reviewed the use of citizen science in flood hazard assessments, discussing its potential to gather information needed to develop realistic scenarios and provide flood hazard parameters, such as extent and water depth, that could help understanding the hazard level at a site. Assumpção et al. (2018) focused on the role citizen science could play in flood modelling and demonstrated its value to provide data for informing, calibrating and validating flood models, particularly where data are scare. It is notable that most of the existing studies have dealt with fluvial flooding; fewer studies have considered pluvial or groundwater flooding (See, 2019). Moreover, none of those citizen science projects have studied the reconstruction of past events using citizen memory, unlike the field of wildlife conservation where Zhang et al. (2018) demonstrated the value of citizen data for mapping past phenomena that were not otherwise recorded.
The objective of this work is twofold: (1) retrieve flood extents and water depths for different past events and (2) determine whether citizens can clarify the causal chain of flood events. We also assessed the reliability of these data by comparing them against independent methods, such as remote sensing.
Our citizen science approach was applied to the suburbs of Yeumbeul North (YN), one of the municipal districts of Pikine in Dakar, Senegal, western Africa (Fig. 1). YN covers an area of about 9 km2. It is one of the most populated districts of Senegal, with 168 379 inhabitants (ANDS, 2015) and a population density of approximately 18 700 inhabitants km−2. YN is characterised by lowlands with elevation less than 20 m above sea level and is highly urbanised with more than 80 % of its territory covered with buildings, critical facilities and roads (Sy et al., 2016). It is one of the suburbs most affected by flooding. Figure 1 displays the state of the permanent water bodies (Lake Warouwaye and Lake Wouye), which existed before retention basins were implemented as a mitigation measure after the 2012 floods (GFDRR, 2014; Sy et al., 2016).
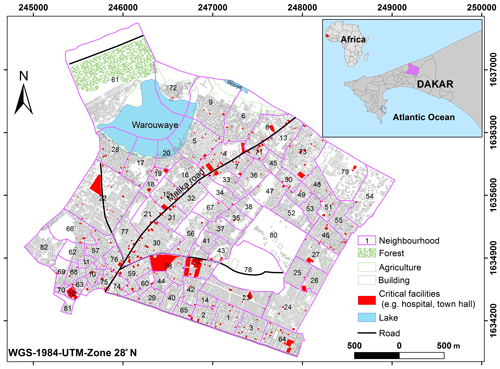
Figure 1Location of the study area. The insert on the right corner locates our study area in the city of Dakar in Senegal. The central map represents our study area Yeumbeul North without the retention basins that were constructed after the 2012 flood. The 82 neighbourhoods are designated by a number from 1 to 82. The corresponding names are provided in the Supplement Table S1.
Administratively, YN is divided into 82 major neighbourhoods. In each of these, a delegate, chosen among the inhabitants of the neighbourhoods, represents the municipal administration (decree no. 86-761, Republic of Senegal, Republic of Senegal) (GDS, 1986). The delegate should be from the neighbourhood and at least 35 years old. One of the delegate's tasks is to inform the neighbourhood inhabitants about how to face disasters. In this paper, we refer to the delegate as a neighbourhood chief (NC; Tall, 1998), an appellation employed by the local population.
Flooding in this area is mainly due to runoff and rainwater, which are not absorbed by impermeable surfaces, made worse by rapid urbanisation and the ineffective drainage network and combined with the rise of groundwater at some locations. Therefore, our area is characterised by multiple types of floods. Flooding occurs during the rainy season, which usually starts in July and ends in October. The three events considered here occurred in 2005, 2009 and 2012. Their timeframe and the peak rainfall intensity are provided in Table 1. The timeframe was retrieved from the Emergency Events Database (EM-DAT, 2018), whereas the rainfall intensity values were registered at the station of Dakar-Yoff, located 20 km away from the study area.
3.1 Investigation of past flood events
Since there is currently no catalogue of past flood events available for the Dakar region, we decided to investigate the potential of citizen science in the retrieval of this information. We developed a framework combining different participatory approaches together in the field of citizen science (Fig. 2).
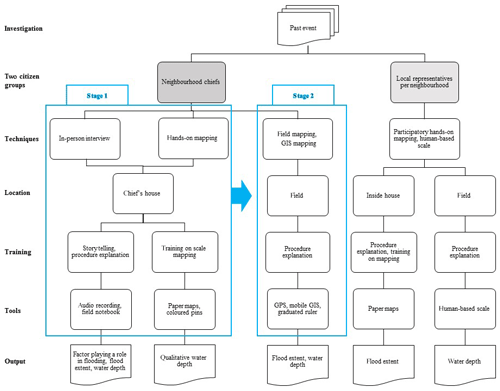
Figure 2Framework for retrieving past flood information by citizen-based methodology. The data regarding this article are available online at: https://doi.org/10.26037/yareta:excgdpysdfadtcyffr4dclt3mm (Sy, 2019b).
The field campaign was carried out from July to August 2017. Our approach involved two different groups of citizens. Participants were selected based on three criteria: they (1) witnessed the three flood events, (2) have a good spatial knowledge of both the study and flooded areas and (3) have a specific social standing. Therefore, the first group consisted of the chiefs of the 82 neighbourhoods in the municipal district of YN. A chief is the qualification given to an official delegate (Tall, 1986) representing the municipal administration (GDS, 1986) and is therefore the focal point for the inhabitants, also in case of disasters. The majority of this group was male (98 %) and their ages varied from 40 to 90 years old, with an average of 66 years old. The second group was composed of 182 people, two or three per neighbourhood. Of these, 72 % were men, with ages varying between 35 and 60. The average was 38 years old. The underrepresentation of women in the study was not by choice, but it is instead due to the sociocultural context of the country (Creevey, 1996).
3.1.1 Neighbourhood chiefs: from episodic memory to flood information
For this group of citizens, we used a two-stage approach to optimise the validity, reliability and utility of the data collected and to transform memories of past floods into temporal and spatial information. The first stage is based on the use of episodic memory through in-person interviews conducted in the chief's house. Episodic memory is the process by which humans remember events in context: date, place and emotional state (Tulving, 1972, 1993, 2002) and is part of long-term memory (Zacks et al., 2000). The second stage involves participatory mapping (IFAD, 2009) and on-site visits.
Face-to-face interviews were conducted with each chief of the 82 neighbourhoods. These persons are nominated by the local population because of their reputation, as they are considered senior and among the oldest inhabitants of the neighbourhood. Each interview was expected to last between 45 and 60 min, but it varied according to the narrative told, and no time limit was imposed. Ultimately, interviews lasted from 30 to 60 min. In some cases it was possible to record the narrative digitally using a smartphone. The information obtained from the narrative allowed the neighbourhoods that were flooded to be identified. Then the chiefs of flooded neighbourhoods were involved in participatory mapping in the house and in the field, together with manual/GIS mapping for the latter case. The purpose of this second step was to formalise and express the chiefs' memories of the floods (as witnesses or victims) in an explicit form in order to obtain past information useable for flood hazard assessment, such as flood extent and water depth. Tools such as paper land-use maps of the area with footprints of houses and different land-use categories (see Fig. 1), handheld GPS and mobile GIS, pins on the map were used.
Stage 1: Investigation of past flood information in the neighbourhood chief's house
The methodology of this stage was derived from techniques used in police investigations (Fisher, 2010; Perfect et al., 2008). Compared to other forms of interviews, it allows the witness (here the neighbourhood chief) to play a more active role, by expressing freely their own narrative without being interrupted or influenced by questions, which could distort the memory (Loftus and Palmer, 1974). First, neighbourhood chiefs were put into a relaxed state, allowing them to focus their thoughts and cognitive and emotional states by closing their eyes (Perfect, 2008) and avoiding physical and psychological distraction (e.g. telephone calls) during this phase, as it requires intense concentration (Fisher, 2010). Some neighbourhood chiefs felt uncomfortable when closing their eyes. In such cases, they were told to focus on a blank surface, like a table or the floor. Once ready, they expressed their memories of the event in the form of descriptive stories, as they came to their mind, using their own words and language (Wolof) in order to avoid misunderstandings. They were instructed to describe in detail anything that may be related to the event, such as (a) processes that accompanied the flood (e.g. the rupture of a water drainage pipe, man-made obstacles); (b) important political or public events that could act as time indicators (e.g. proximity to a presidential election, football game); (c) notable flood-related measures taken by the authorities enabling the event to be dated; (d) spatial indicators such as place and street names allowing reconstruction of the flooded areas; and (e) the event itself, including information allowing for the deduction of the water depth (e.g. “the water reached our knees”).
Following the narrative, only chiefs who indicated having been confronted with floods went through participatory mapping using maps at the scale of the neighbourhood (62 out of 82 chiefs, see Table S2). This phase required training on how to read and use a map. Therefore, the concerned neighbourhood chiefs were first familiarised with a land-use map of their neighbourhood locating their house and other features in their area including main and secondary roads as well as houses. After this introductory explanation, the neighbourhood chiefs used the map to describe their spatial perception of the different flood events, using a distinctive colour pencil to draw the flood contours of each year. Coloured pins were used for indicating the water depth at different locations on the map; red for a high level of water, green for medium and yellow for low. This method allowed a qualitative indication of the water depth as well as its spatial distribution to be obtained.
Stage 2: Investigation of past flood information with neighbourhood chiefs in the field
The objective of stage 2 was to corroborate the chiefs' responses from stage 1 by cross-checking the information leading to the map from stage 1 with the on-site mapping. To do this, neighbourhood chiefs brought us to the places they previously described. This is important because memory retrieval is facilitated when the context of the event is recreated, and neighbourhood chiefs can also use their other senses (sight, hearing, smell) to better remember the event (Rubin, 2005). We drew the polygon of the spatial extent using a mobile GIS, with a GPS receiver automatically recording the site location. Furthermore, we measured the water level as indicated by 49 neighbourhood chiefs with a graduated ruler (Tables S3, S4 and S5) at 64 sites and recorded the GPS coordinates. Post-processing treatments include merging the contours of flooded areas obtained on the paper map with the ones obtained in the field as well as checking the correspondence between qualitative water levels obtained with the coloured pins to the quantitative water level measurements. The objective of the latter was to verify if the sites indicated as having had very high water levels on the paper map from stage 1 (red pin) corresponded to a high water level measured in the field. Since we assume that memory retrieval is facilitated when one is present at the site, we consider the field value to be more reliable.
3.1.2 Local representatives: participatory mapping on flood extent and water level of past flood events
The second group involved in investigating past flood events was composed of 182 people, selected by local associations (e.g. “Réseau d'Information d'Education de Communication”, “Association des Relais Communautaires de Yeumbeul”) that deal with the development of the neighbourhood and awareness of health issues. The selection was based on the previously mentioned criteria. As these associations operate locally, they personally know residents and the choice of the inhabitants to be the representatives of the neighbourhood was based on a consensus among the associations. From here on, we use the term “local representatives” to refer to these selected people. The aim of involving local representatives is to integrate their information with that provided from the neighbourhood chiefs in order to check the consistency between the two sources. Two or three local representatives were selected per flooded neighbourhood, accounting for 130 out of 182 representatives, in order for them to recall their memories and reach a common agreement (Swanson et al., 2016) before providing information on flood extent and water depths for the different flood events. Data on flood extent were retrieved by participatory mapping using hands-on techniques. For this, representatives were trained the same way as the chiefs. These maps were then digitised. Regarding water level, local representatives went to the same 64 sites as indicated by the chiefs; they did not have any prior knowledge of the depths given by the chiefs, and depth information was given using the different parts of the human body, e.g. ankle, knee or shoulder. This strategy was proposed to provide local representatives with a visual resource to describe the water level more easily. Then, the pre-defined tags were converted into quantitative data by using average body segment lengths expressed as a fraction of body height, as defined in the field of physical anthropometry (Drillis and Contini, 1966; Winter, 2009). The bottom-up dimensionless coefficients applied for each anthropometric segment (Table S6) are (Winter, 2009; Contini, 1972) ankle (0.039), knee (0. 285), wrist (0.485), elbow (0.63), chest (0.72), shoulder (0.818) and chin (0.870). Finally, the water depth was obtained by multiplying the value of the appropriate coefficient by the contributor's (local representative; LR) height, as measured on site with a tape measure (Tables S3, S4, S5 and S6). As we used two different approaches to obtain the same information, we needed to assess the level of agreement instead of the correlation between the two datasets. We used the Bland–Altman method (1986), which determines the level of agreement between data acquired with two different techniques, even if there is no information about the “true” values (Bland and Altman, 1986). In our case, we assessed depth values that could not be measured authoritatively during the flood events under study. The Bland–Altman method calculates the differences between the results obtained with two different approaches and plots them against the average of the two approaches.
3.1.3 Remote sensing analysis
We used data from a remote sensing analysis to assess the reliability of the extents of flooded areas provided by the two citizens groups. Our requirements were (1) availability of images for the years considered, (2) free access to the data, (3) sufficient resolution for the size of our study area (9 km2) and (4) minimum cloud cover. Radar images such as TerraSAR-X, Radarsat-2 or COSMO-SkyMed can provide information with high resolution (Schubert et al., 2012) and can capture flooded areas in cloudy conditions at day and at night (Mason et al., 2014; Schumann and Moller, 2015), but they are not free of charge, and, most importantly for our case, no images were available for the periods of interest. Consequently, we only used available optical satellite images from different sensors and from different sources. Indeed, optical images were not available on Google Earth for the 2005 flood event. The main characteristics of these products we used are given in Table 2. Flooded areal extents were obtained following the process chain described in Fig. 3.
For the 2005 event, we used two SPOT images (23 October) and (7 September) provided by the applied Remote Sensing Laboratory (LTA) of the Institute of Earth Sciences (IST) of the Université Cheikh Anta Diop (UCAD) (Table 2).
Table 2Remote sensing data. Dates are given the dd/mm/yyyy format. SPOT: Satellite pour l’Observation de la Terre (https://earth.esa.int/web/guest/glossary#s); HRV: high-resolution visible.
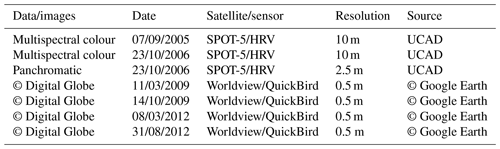
It should be noted that we did not find an image from before the flooding and hence we used an image obtained during a dry period. These two multispectral SPOT 5 images of 10 m resolution were merged with a SPOT 5 panchromatic image with a spatial resolution of 2.5 m to increase the spatial accuracy. We then applied the normalised difference water index (NDWI; Khajuria et al., 2017) to the water signature from other land-use types. The NDWI is calculated following the method of McFeeters (1996), using the green and the near-infrared (NIR) bands.
An unsupervised classification was then performed to cluster pixels having similar NDWI values, using the ISODATA (Iterative Self-Organizing Data Analysis Technique) clustering algorithm provided through the software ERDAS IMAGINE© 2014. The classes were then coded to highlight only the water areas. These areas were then digitised on both images. Finally, both layers were compared and only areas corresponding to flooded areas were kept. An area is considered as flooded if water can be detected only on the image after the flood.
For the 2009 and 2012 events, we used images available from Google Earth. Google launched Google Earth in 2005 (Cha and Pak, 2007), and it provides free online aerial and satellite images covering many parts of the world, with various resolutions and sensors. The highest resolution, about 0.5 m, is provided by Worldwide and QuickBird satellite imagery operated by Digital Globe. For each flood event, we examined the historical true colour composite imagery from Google Earth using the time slider bar of Google to find one image as close as possible to the flood event and another one in a dry period after the event. These images were then photo-interpreted to identify areas of water. These areas were digitised and then compared to extract only areas considered as flooded.
4.1 Identifying the chain of events
The chain of events which triggered floods in YN was retrieved from the narrative obtained from 82 neighbourhood chiefs. For the 2005, 2009 and 2012 events, all the 82 neighbourhood chiefs identified rainfall as the primary factor. Of the 29 chiefs (neighbourhood numbers 1, 2, 3, 7, 9, 17, 19, 20, 28, 29, 31, 32, 33, 34, 35, 36, 39, 40, 41, 44, 49, 50, 56, 57, 62, 67, 70, 72, 76; see Fig. 1) also pointed out the rise of the water table, substantiated by the wet ground, greening of walls due to the water infiltration and removal of paint from walls.
The neighbourhood chiefs identified different processes that worsened the flood, by either increasing the quantity of water or obstructing the typical flow, for both different locations and events. For example, for the 2005 event, four neighbourhood chiefs (13, 36, 46, 67) mentioned the failure of the pipeline in the road of Malika, used for water drainage, as increasing the intensity of the flood event. Eight neighbourhood chiefs (7, 17, 18, 20, 21, 28, 45, 77) mentioned the overflow of Lake Warouwaye. Additionally, 15 neighbourhood chiefs (7, 13, 17, 18, 19, 20, 21, 28, 36, 45, 46, 67, 72, 76, 77) mentioned actions performed by the local population, such as the emptying of household septic tanks, which aggravated this event and also had direct consequences on health (e.g. cholera epidemics) (Wade et al., 2009). Pipeline failure and the emptying of septic tanks also occurred during the 2009 event, but at different locations, e.g. near the municipal hospital of YN for the pipeline failure. For the 2012 event, the 82 chiefs did not recall any processes that worsened the floods.
4.2 Flood extent mapping
Flood extents for the 2005, 2009 and 2012 events were obtained from the two citizen groups using the methodologies described in Fig. 2 and then compared to the results derived from the remote sensing analysis (Fig. 4).
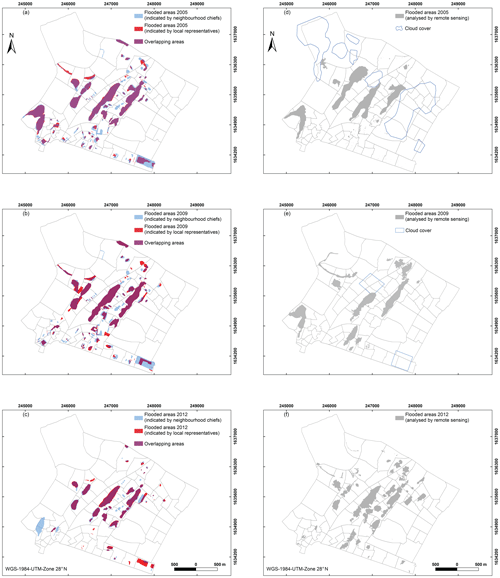
Figure 4Spatial distribution of flooded areas based on citizen science techniques in (a) 2005, (b) 2009 and (c) 2012. Flooded areas based on remote sensing data in (d) 2005, (e) 2009 and (f) 2012.
Data obtained through the citizen science approach revealed that the 2005 event was the most widespread, whereas the 2012 event was the smallest (Table 3). Flooded areas provided by local representatives are slightly smaller than those indicated by neighbourhood chiefs (Table 3), showing variations from 1.8 % in to 2005 to 0.6 % in 2012 (Table 3).
Table 3Comparison of flooding areas from citizen science techniques deployed in Yeumbeul North (neighbourhood chiefs and local representatives) and remote sensing analyses.
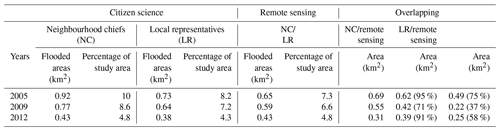
In terms of mapping, slight differences appear between the extents identified by the two citizen groups (Fig. 4), but the areas overlap reasonably well (Fig. 5).
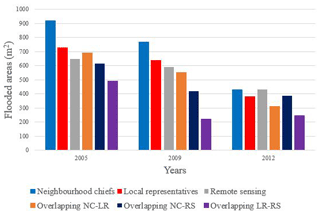
Figure 5Flooded areas obtained by the two citizen groups and remote sensing with the surface of overlapping areas between the two results of the citizen groups (orange), neighbourhood chiefs and remote sensing (dark blue), and local representatives and remote sensing (purple).
The remote sensing analysis confirms that the main flooded areas were in the central part of the study area (Fig. 4), but some discrepancies occur at the edges. The total surface area is smaller than that provided by citizen science for all years (Table 3), but it shows the same tendency of decreasing surfaces from 2005 to 2012. We also find that flood extents provided by neighbourhood chiefs agreed better with the remote sensing than those provided by local representatives for all events.
4.3 Water depth information
Water depth is one of the key parameters considered in describing flood intensity and mapping hazard (Van Alphen et al., 2007), but it is difficult to record during flood events. Therefore, retrieving flood depths from past events is of prime interest. Figure 6 displays scatter diagrams of depth values obtained from the two different groups of citizens using the techniques described in the methods (see Fig. 2) at 64 sites, sampled over 49 neighbourhoods. We have two measurements from each site. The maximum retrieved flood depth is 2.5 m for the 2005 event, 1.5 m for the 2009 event and 1.2 m for the 2012 event.
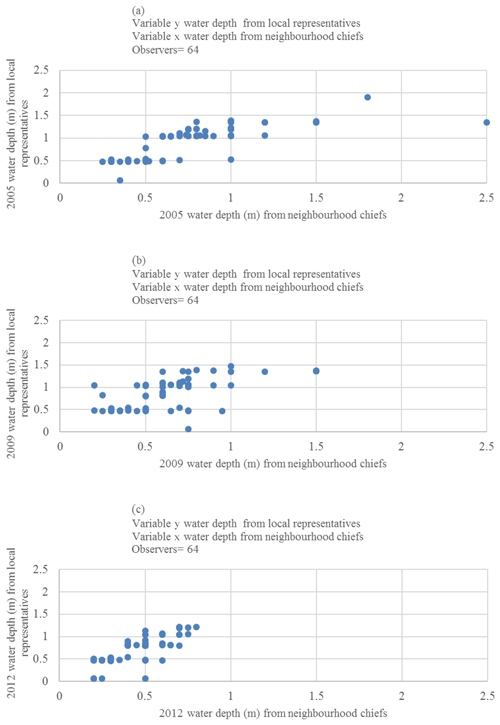
Figure 6Scatter diagram of water depth information provided by techniques used with neighbourhood chiefs and local representatives for three different flooding events in (a) 2005, (b) 2009 and (c) 2012.
Figure 7 shows the data obtained by applying the Bland–Altman method for the 2005, 2009 and 2012 events for the 64 measurement sites. The value of the mean differences in water depth, indicated by the blue line, is 0.16 m for the 2005 event, 0.23 m for 2009 and 0.26 m for 2012. The limits of agreement, also displayed, are set at 95 % confidence intervals. Assuming the differences are normally distributed, these limits are defined by the mean difference ±1.96 multiplied by the standard deviation σ of the differences. For the 2005 event, this range is from 0.68 to −0.37 m, with two values falling outside these limits. For 2009 and 2012, three values are outside the 95 % confidence interval which is from 0.78 to −0.32 m for 2009 and 0.62 to −0.11 m for 2012.
In this study, we have used citizen science to retrieve information on three past flood events that impacted the region of Dakar during the past 10 years. Our approach provides quantitative information on water depth, helps retrieve the flood extents and provides insights into factors that aggravate the intensity of floods.
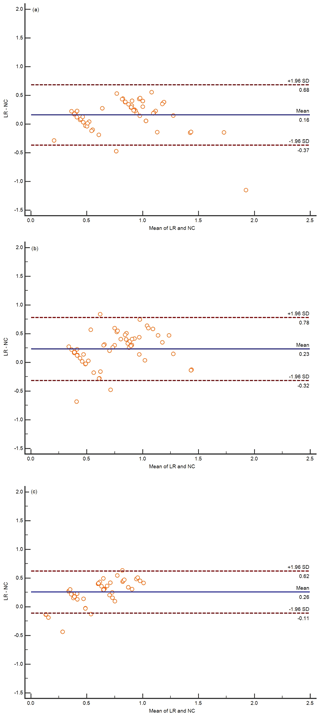
Figure 7Bland–Altman plots for different flooding events in (a) 2005, (b) 2009 and (c) 2012. These graphs show differences between water depth provided by neighbourhood chiefs (NC) and local representatives (LR) in metres against averaged values of NC and LR. Blue line is the mean difference value, and the red dotted lines show the ±1.96 standard deviation (SD) water depth differences for all observations.
Our methodology consisted of a set of techniques designed to gather the most complete spectrum of information. These techniques are unusual in the field of flood hazard assessment, and we had to resolve some challenges associated with the time that has elapsed since the events and participants' understanding of maps. One technique is based on people's episodic memory; we used face-to-face interviews with neighbourhood chiefs, applying specific tools in order to limit external influence and memory distortion. The procedure was then completed by a site visit with each neighbourhood chief involved in order to consolidate the verbally provided information. The site visit is very important because the time elapsed between the oldest event and the date of this study is about 12 years. As time goes by, memories can become vague (Lacy and Stark, 2013). However, people who have experienced traumatic and stressful events, like floods, tend to retain a more accurate, detailed and time-persistent memory of the event (Sotgiu and Galati, 2007).
Another technique involved participatory hands-on mapping. Mapping can represent a challenging task for laypeople (Handmer, 1985; Żyszkowska, 2015, 2017), as they may have difficulties understanding and locating themselves on a map. Moreover, maps are usually constructed by applying standard rules of graphic semiology (Thomas, 2001) that does not necessary take into account the cultural background or knowledge of the citizen (Fuchs et al., 2009). Therefore, if a citizen has no experience in reading or producing maps, information can be incorrectly reported. To overcome this problem, we trained people on how to read a map and locate themselves to ensure they understood the map, and we explained what they should be doing and how to do it.
Developing the quality and reliability of citizen science data is a growing research field (Crall et al., 2011; Flanagin and Metzger, 2008; Silvertown et al., 2015). In our study, we developed different strategies in order to improve these two aspects. We decided to work with two different target groups according to the context and the purpose of the study. The objective was to check the consistency of information obtained from the two groups. If the same area is described as flooded by both groups, there is a good chance that the area was indeed flooded. Due to the social organisation of the Dakar region, we limited issues regarding source credibility (Flanagin and Metzger, 2008) by involving neighbourhood chiefs. Indeed, these chiefs are appointed by local citizens, according to the trust placed in them and on their long-lasting presence in the area. Usually, they have a good memory and good verbal abilities. Moreover, as a witness or sometimes as a victim, they were at the forefront of the flood scene, therefore representing a valuable source of information on the chain of events. The second group was composed of local representatives, selected with the support of local and well-implemented associations.
Identifying the chain of processes generating flooding is very important for flood hazard assessment (DAEC, 2016) as it enables analysis of more realistic flood scenarios. Citizens living in flood affected areas are not frequently included in post-event or flood hazard assessments, even though they could provide useful insights as they have a good understanding of their surroundings (Tran et al., 2009) and in-depth local knowledge. Our study demonstrates this as the neighbourhood chiefs identified both natural and man-made factors that contributed to flooding, such as the rise of ground water, the Lake Warouwaye overflow and the emptying of septic tanks.
In terms of flooded areas, the results obtained from the two groups of citizens are similar for each event, although some spatial differences can be observed regarding the extent. Reasons for the differences could be related to (a) a more in-depth knowledge of the neighbourhood and their surroundings by the chiefs, as they have the confidence (Tall, 1998) of the inhabitants and therefore have access to more detailed information and (b) the techniques used in mapping the areas. With neighbourhood chiefs, we used a two-stage procedure to retrieve the flood extent, involving hands-on mapping and GIS mapping in the field, whilst the local representatives only produced hands-on maps that were then digitised.
A good spatial agreement exists between flood extents determined from remote sensing and citizen science, with better agreement from the data provided by the neighbourhood chiefs. However, areas provided by remote sensing are smaller. This discrepancy can be explained by various factors. One could be the different spatial resolutions of the selected images, which varied from 0.5 to 2.5 m; the larger were probably not being small enough to capture all flooded areas (Grimaldi et al., 2016) at the scale at which we worked. A second factor concerns the different time lapses between images. Post-event images from Google Earth were captured at intervals from 1 to 15 d, and therefore they may not have captured the maximum extent. Furthermore, for the 2005 event, one image was obtained during the flooding, with the second image taken one year after with the assumption it was captured during a dry period. A third factor is related to technical limitations of the capability of optical satellites to detect flooded areas, which is reduced when clouds are present (Malinowski et al., 2017; Mallinis et al., 2013). A fourth factor could be linked with the heterogeneity of sensors used in this study. A way to remove this source of discrepancy would be to use satellite images obtained with the same sensor. Finally, the efficiency of the NDWI index used to detect water areas could be altered by noise (Xu, 2006).
One of the techniques used to retrieve water depths was inspired from studies expressing flood hazard levels on maps using a body scale (e.g. EXCIMAP, 2007; Luke et al., 2018). Therefore, quantitative data on water depth were retrieved using a proportion of the size of the human body borrowed from the field of physiology (Winter, 2009). These values represent an average (Drillis and Contini, 1966), since the length of human body segments depends on body structure (Contini, 1972), gender and racial groups, and therefore it could be a source of uncertainties. However, when comparing the two approaches used for water depth investigation, we find a fairly good agreement, with average differences less than 0.3 m, which is within the range of other comparisons between observed and simulated methods (Kutija et al., 2014).
Both involvement and motivation from citizens are necessary for the success of citizen science projects (Rotman et al., 2012). As Facebook was one of the most used social media in YN at the time of the study (Sy, 2019a), we first created a page to interact with local citizens and motivate them to be part of the project. Secondly, we designed and presented the project in a way to convince contributors that their contribution will be beneficial for them and their neighbours. Thirdly, we worked with community leaders (Bénit-Gbaffou and Katsaura, 2014) and local associations to ensure a better acceptance of the project.
Citizen science requires involvement and time, compared to a remote sensing analysis which can now also take advantage of the free availability of radar images such as Sentinel (Malenovský et al., 2012). However, at the scale at which we worked, these images offer neither the required spatial resolution (Twele et al., 2016) nor information on the depth of the flood, which is a critical datum for flood hazard assessment that we were able to obtain with citizen science.
In conclusion, our study shows the potential of citizen science in retrieving quantitative and reliable information on past flood events, especially in areas where no or few records of past events are available. Our investigation strategy, involving two different groups of citizens, increases the reliability of the obtained data. Provided that the functioning of the society subject to floods is well understood, such an approach can be replicated in other parts of the world. Moreover, the citizens that have been involved in the various steps of this project have developed skills in flood data acquisition and an understanding of flood processes. They can thus better integrate into a decision-making process regarding flood risk.
Raw Google images are accessible from the Google Earth portal. SPOT images are accessible on request to the owning institution, i.e. Institute of Science of Earth (IST) of the Université Cheikh Anta Diop in Dakar. All other related data are available at https://doi.org/10.26037/yareta:excgdpysdfadtcyffr4dclt3mm (Sy, 2019b).
The supplement related to this article is available online at: https://doi.org/10.5194/hess-24-61-2020-supplement.
BS conceived the study and carried out citizen science project in the field. BS analysed the results and compiled the figures with input from CF. The outline of the paper was drafted by BS, HD, DC, GG and CF. BS and CF prepared the paper with contributions from all co-authors. All the authors reviewed the paper.
The authors declare that they have no conflict of interest.
The authors are grateful to Prof. Souleye Wade from the Applied Remote Sensing Laboratory (LTA) of the Institute of Earth Sciences (IST) of the Université Cheikh Anta Diop in Dakar (UCAD) for providing them with the SPOT satellite images.
This field campaigns were supported by the Augustin Lombard grant from the Société de physique et d'histoire naturelle de Genève and Plantamour-Prévost grant from the Faculty of Science of the University of Geneva.
This paper was edited by Elena Toth and reviewed by Linda See and one anonymous referee.
Ahiablame, L. and Shakya, R.: Modeling flood reduction effects of low impact development at a watershed scale, J. Environ. Manage., 171, 81–91, https://doi.org/10.1016/j.jenvman.2016.01.036, 2016.
ANDS: Situation économique et sociale régionale 2013: Agence Nationale de la Statistique et de la Démographie, 1–129, 2015.
Assumpção, T. H., Popescu, I., Jonoski, A., and Solomatine, D. P.: Citizen observations contributing to flood modelling: opportunities and challenges, Hydrol. Earth Syst. Sci., 22, 1473–1489, https://doi.org/10.5194/hess-22-1473-2018, 2018.
Bénit-Gbaffou, C. and Katsaura, O.: Community Leadership and the Construction of Political Legitimacy: Unpacking Bourdieu's “Political Capital” in Post-Apartheid Johannesburg, Int. J. Urban Regional, 38, 1807–1832, https://doi.org/10.1111/1468-2427.12166, 2014.
Bland, J. M. and Altman, D.: Statistical methods for assessing agreement between two methods of clinical measurement, The Lancet, 327, 307–310, 1986.
Buytaert, W., Zulkafli, Z., Grainger, S., Acosta, L., Alemie, T. C., Bastiaensen, J., De Bièvre, B., Bhusal, J., Clark, J., Dewulf, A. Foggin, M., Hannah, D. M., Hergarten, C., Isaeva, A., Karpouzoglou, T., Pandeya, B., Paudel, D., Sharma, K., Steenhuis, T., Tilahun, S., Van Hecken, G., and Zhumanova, M: Citizen science in hydrology and water resources: opportunities for knowledge generation, ecosystem service management, and sustainable development, Front. Earth Sci., 2, 1–21, https://doi.org/10.3389/feart.2014.00026, 2014.
Cha, S.-Y. and Park, C.-H.: The utilization of Google Earth images as reference data for the multitemporal land cover classification with MODIS data of North Korea, Korean Journal of Remote Sensing, 23, 483–491, 2007.
Chen, Y., Zhou, H., Zhang, H., Du, G., and Zhou, J.: Urban flood risk warning under rapid urbanization, Environ. Res., 139, 3–10, https://doi.org/10.1016/j.envres.2015.02.028, 2015.
Contini, R.: Body segment parameters, Part II, Artificial Limbs, 16, 1–19, 1972.
Crall, A. W., Newman, G. J., Stohlgren, T. J., Holfelder, K. A., Graham, J., and Waller, D. M.: Assessing citizen science data quality: an invasive species case study, Conserv. Lett., 4, 433–442, https://doi.org/10.1111/j.1755-263X.2011.00196.x, 2011.
Creevey, L.: Islam, Women and the Role of the State in Senegal, J. Relig. Afr., 327, 268–307, https://doi.org/10.2307/1581646, 1996.
DAEC: Cartographie intégrale des dangers naturels liés aux crues sur le plateau fribourgeois, Direction de l'aménagement, de l'environnement et des constructions, 1–25, 2016.
Di Baldassarre, G., Montanari, A., Lins, H., Koutsoyiannis, D., Brandimarte, L., and Blöschl, G.: Flood fatalities in Africa: from diagnosis to mitigation, Geophys. Res. Lett., 37, L22402, https://doi.org/10.1029/2010GL045467, 2010.
Dickinson, J. L., Zuckerberg, B., and Bonter, D. N.: Citizen science as an ecological research tool: challenges and benefits, Annu. Rev. Ecol. Syst., 41, 149–172, 2010.
Douglas, I., Alam, K., Maghenda, M., Mcdonnell, Y., McLean, L., and Campbell, J.: Unjust waters: climate change, flooding and the urban poor in Africa, Environ. Urban., 20, 187–205, https://doi.org/10.1177/0956247808089156, 2008.
Drillis, R. and Contini, R.: Body segment parameters. Office of Vocational Rehabilitation, Department of Health, Education and Welfare: New York, Scholl of Engineering and Science, New York University, Report No. 1166-03, 1966.
EM-DAT: The OFDA/CRED International Disaster Database, available at: http://www.emdat.be/disaster_trends/index.html, last access: 14 August 2018.
EXCIMAP: Handbook on good practices for flood mapping in Europe. (European exchange circle on flood mapping), 1–60, available at: https://ec.europa.eu/environment/water/flood_risk/flood_atlas/pdf/handbook_goodpractice.pdf (last access: 14 August 2018), 2007.
Fisher, R. P.: Interviewing cooperative witnesses, Legal Criminol. Psych., 15, 25–38, https://doi.org/10.1348/135532509X441891, 2010.
Flanagin, A. J. and Metzger, M. J.: The credibility of volunteered geographic information, GeoJournal, 72, 137–148, https://doi.org/10.1007/s10708-008-9188-y, 2008.
Fritz, S., McCallum, I., Schill, C., Perger, C., Grillmayer, R., Achard, F., Kraxner, F., and Obersteiner, M.: Geo-Wiki. Org: The use of crowdsourcing to improve global land cover, Remote Sensing, 1, 345–354, 2009.
Fuchs, S., Spachinger, K., Dorner, W., Rochman, J., and Serrhini, K.: Evaluating cartographic design in flood risk mapping, Environmental Hazards, 8, 52–70, 2009.
GDS: Decret N 86-761 du 30 juin 1986, Gouvernement du Sénégal, available at: http://www.servicepublic.gouv.sn/assets/textes/deleg-quartier.pdf (last access: 24 September 2018), 1986.
GFDRR: Senegal: urban floods: recovery and reconstruction since 2009, World Bank's Global facility for Disaster Reduction and Recovery, 1–48, 2014.
Grimaldi, S., Li, Y., Pauwels, V. R., and Walker, J. P.: Remote sensing-derived water extent and level to constrain hydraulic flood forecasting models: opportunities and challenges, Surv. Geophys., 37, 977–1034, https://doi.org/10.1007/s10712-016-9378-y, 2016.
Handmer, J.: Floodplain maps: uses and limitations as public information, in: proceedings of the 13th New Zealand Geographical Society Conference, Hamilton, New Zealand, 1985.
IFAD: Good practices in participatory mapping: a review prepared for the International Fund for Agricultural Development, 1–59, available at: https://www.ifad.org/documents/38714170/39144386/PM_web.pdf (last access: 18 December 2019), 2009.
Khajuria, A., Farooq, M., and Prashar, P: Flood inundation mapping of Srinagar city using geospatial techniques, in: Proceedings of the National Conference on Advances in Water Ressource and Environment Research, Tamilnadu, India 29–30 June 2017, 1–236, 2017.
Kutija, V., Bertsch, R., Glenis, V., Alderson, D., Parkin, G., Walsh, C., Robinson, J., and Kilsby, C.: Model validation using crowd-sourced data from a large pluvial flood, in: Proceedings of the 11th International conference on hydroinformatics, New York City, USA, 17–21 August 2014, 2014.
Lacy, J. W. and Stark, C. E.: The neuroscience of memory: implications for the courtroom, Nat. Rev. Neurosci., 14, 649–658, https://doi.org/10.1038/nrn3563, 2013.
Loftus, E. F. and Palmer, J. C.: Reconstruction of automobile destruction: An example of the interaction between language and memory, J. Verb. Learn. Verb. Be., 13, 585–589, 1974.
Luke, A., Sanders, B. F., Goodrich, K. A., Feldman, D. L., Boudreau, D., Eguiarte, A., Serrano, K., Reyes, A., Schubert, J. E., AghaKouchak, A., Basolo, V., and Matthew, R. A.: Going beyond the flood insurance rate map: insights from flood hazard map co-production, Nat. Hazards Earth Syst. Sci., 18, 1097–1120, https://doi.org/10.5194/nhess-18-1097-2018, 2018.
Malenovský, Z., Rott, H., Cihlar, J., Schaepman, M. E., García-Santos, G., Fernandes, R., and Berger, M.: Sentinels for science: Potential of Sentinel-1, -2, and -3 missions for scientific observations of ocean, cryosphere, and land, Remote Sens. Environ., 120, 91–101, https://doi.org/10.1016/j.rse.2011.09.026, 2012.
Malinowski, R., Groom, G. B., Heckrath, G., and Schwanghart, W.: Do Remote Sensing Mapping Practices Adequately Address Localized Flooding? A Critical Overview: Springer Science Reviews, 5, 1–17, https://doi.org/10.1007/s40362-017-0043-8, 2017.
Mallinis, G., Gitas, I. Z., Giannakopoulos, V., Maris, F., and Tsakiri-Strati, M.: An object-based approach for flood area delineation in a transboundary area using ENVISAT ASAR and LANDSAT TM data, Int. J. Digit. Earth, 6, 124–136, https://doi.org/10.1080/17538947.2011.641601, 2013.
Mason, D. C., Giustarini, L., Garcia-Pintado, J., and Cloke, H. L.: Detection of flooded urban areas in high resolution Synthetic Aperture Radar images using double scattering, Int. Journée d'animation Earth Obs., 28, 150–159, https://doi.org/10.1016/j.jag.2013.12.002, 2014.
McFeeters, S. K.: The use of the Normalized Difference Water Index (NDWI) in the delineation of open water features, Int. J. Remote S., 17, 1425–1432, https://doi.org/10.1080/01431169608948714, 1996.
Mooney, P. and Minghini, M.: A review of OpenStreetMap data, in: Mapping and the Citizen Sensor, edited by: Foody, G., See, L., Fritz, S., Mooney, P., Olteanu-Raimond, A.-M., Fonte, C. C., and Antoniou, V., 37–59, Ubiquity Press, London, https://doi.org/10.5334/bbf.c, 2017.
Paul, J. D., Buytaert, W., Allen, S., Ballesteros-Cánovas, J. A., Bhusal, J., Cieslik, K., Clark, J., Dugar, S., Hannah, D. M., and Stoffel, M.: Citizen science for hydrological risk reduction and resilience building, Wiley Interdisciplinary Reviews: Water, 5, e1262, https://https://doi.org/10.1002/wat2.1262, 2018.
Perfect, T. J., Wagstaff, G. F., Moore, D., Andrews, B., Cleveland, V., Newcombe, S., Brisbane, K.-A., and Brown, L.: How can we help witnesses to remember more? It's an (eyes) open and shut case, Law Human Behav., 32, 314–324, 2008.
Preventionweb; Disaster and Risk Profile, Africa, Senegal, Nationally Reported Losses 1990–2014, available at: http://www.preventionweb.net/countries/sen/data/, last access: 14 August 2018.
Raddick, J., Lintott, C., Schawinski, K., Thomas, D., Nichol, R., Andreescu, D., Bamford, S., Land, K., Murray, P., and Slosar, A.: Galaxy Zoo: an experiment in public science participation, Bulletin of the American Astronomical Society, 39, p. 892, 2007.
Rotman, D., Preece, J., Hammock, J., Procita, K., Hansen, D., Parr, C., Lewis, D., and Jacobs, D.: Dynamic changes in motivation in collaborative citizen-science projects, in: Proceedings of the ACM 2012 conference on computer supported cooperative work, Washington, USA, 11–15 February 2012, 217–226, available at: https://dl.acm.org/citation.cfm?id=2145238 (last access: 25 January 2019), 2012.
Rubin, D. C.: A basic-systems approach to autobiographical memory, Curr. Dir. Psychol. Sci., 14, 79–83, 2005.
Sanyal, J. and Lu, X.: Application of remote sensing in flood management with special reference to monsoon Asia: a review, Nat. Hazards, 33, 283–301, 2004.
Schubert, A., Small, D., Jehle, M., and Meier, E.: COSMO-SkyMed, TerraSAR-X, and RADARSAT-2 geolocation accuracy after compensation for earth-system effects, in: 2012 IEEE International Geoscience and Remote Sensing Symposium, 3301–3304, 2012.
Schumann, G. J.-P. and Moller, D. K.: Microwave remote sensing of flood inundation: Physics and Chemistry of the Earth, Parts A/B/C, 83–84, 84–95, 2015
See, L. M.: A Review of Citizen Science and Crowdsourcing in Applications of Pluvial Flooding, Front. Earth Sci., 7, p. 44, https://doi.org/10.3389/feart.2019.00044, 2019.
Silvertown, J.: A new dawn for citizen science, Trends Ecol. Evol., 24, 467–471, 2009.
Silvertown, J., Harvey, M., Greenwood, R., Dodd, M., Rosewell, J., Rebelo, T., Ansine, J., and McConway, K.: Crowdsourcing the identification of organisms: A case-study of iSpot, ZooKeys, 480, 125–146, https://doi.org/10.3897/zookeys.480.8803, 2015.
Sotgiu, I. and Galati, D.: Long-term memory for traumatic events: experiences and emotional reactions during the 2000 flood in Italy, J. Psychol., 141, 91–108, 2007.
Swanson, A., Kosmala, M., Lintott, C., and Packer, C.: A generalized approach for producing, quantifying, and validating citizen science data from wildlife images, Conserv. Biol., 30, 520–531, https://doi.org/10.1111/cobi.12695, 2016.
Sy, B.: Approche multidisciplinaire de l'évaluation de l'aléa d'inondation à Yeumbeul Nord, Dakar, Sénégal : la contribution de la science citoyenne. Université de Genève, Thèse, https://doi.org/10.13097/archive-ouverte/unige:126388, 2019a.
Sy, B.: data_BSy_etal_HESS, https://doi.org/10.26037/yareta:exc, 2019b.
Sy, B., Frischknecht, C., Dao, H., Giuliani, G., Consuegra, D., Wade, S., and Kêdowidé, C.: Participatory approach for flood risk assessment: the case of Yeumbeul Nord (YN), Dakar, Senegal, WIT Trans. Built Env., 165, 331–342, https://doi.org/10.2495/UW160291, 2016.
Sy, B., Frischknecht, C., Dao, H., Consuegra, D., and Giuliani, G.: Flood hazard assessment and the role of citizen science, J. Flood Risk Manag., e12519, https://doi.org/10.1111/jfr3.12519, 2019.
Tall, S. M.: La Décentralisation et le Destin des Délégués de Quartier à Dakar (Sénégal). Plaidoyer pour les délégués de quartier de Dakar après la loi de décentralisation de 1996, Bulletin de l'APAD, 15, 1–13, available at: http://journals.openedition.org/apad/567 (last access: 28 November 2018), 1998.
Thomas, I.: Cartographie d'aujourd'hui et de demain: rappels et perspectives: Cybergeo: Revue européenne de géographie, document 189, https://doi.org/10.4000/cybergeo.3812, 2001.
Townsend, P. A. and Walsh, S. J.: Modeling floodplain inundation using an integrated GIS with radar and optical remote sensing, Geomorphology, 21, 295–312, 1998.
Tran, P., Shaw, R., Chantry, G., and Norton, J.: GIS and local knowledge in disaster management: a case study of flood risk mapping in Viet Nam, Disasters, 33, 152–169, 2009.
Tulving, E.: Episodic and semantic memory, Organization of memory, 1, 381–403, 1972.
Tulving, E.: What is episodic memory?, Curr. Dir. Psychol. Sci., 2, 67–70, 1993.
Tulving, E.: Episodic memory: From mind to brain, Annu. Rev. Psychol., 53, 1–25, 2002.
Twele, A., Cao, W., Plank, S., and Martinis, S.: Sentinel-1-based flood mapping: a fully automated processing chain, Int. J. Remote Sens., 37, 2990–3004, 2016.
UNISDR-CRED: The human cost of weather-related disasters 1995–2015, The United Nations office for Disaster Risk Reduction (UNISDR) and Centre for Research on the Epidemiology of Disasters (CRED), 1–30, 2015.
Urama, K. C. and Ozor, N.: Impacts of climate change on water resources in Africa: the role of adaptation, African Technology Policy Studies Network, 29, 1–29, 2010.
Van Alphen, J., Passchier, R., and Martini, F.: Atlas of Flood Maps: Examples from 19 European Countries, USA and Japan, available at: http://www.mko.gov.si/.../atlas_primerov_kartiranja_poplavne_nevarn... (last access: 1 December 2018), 2007.
Wade, S., Faye, S., Dieng, M., Kaba, M., and Kane, N.: Télédétection des catastrophes d'inondation urbaine: le cas de la région de Dakar (Sénégal), Journées dÁnimation Scientifique (JAS09) de lAUF Alger, 2009.
Wiggins, A. and Crowston, K.: From conservation to crowdsourcing: A typology of citizen science, in: Proceedings of the 44th Hawaii International Conference on System Science (HICSS), 1–10, 2011.
Winter, D. A.: Biomechanics and motor control of human movement, fourth edition, John Wiley & Sons, Inc., 82–106, ISBN 978-0-470-39818-0, 2009.
WMO: Integrated flood management tools series: Urban flood management in a changing climate, World Meteorological Organization, 14, 1–54, 2012.
Xu, H.: Modification of normalised difference water index (NDWI) to enhance open water features in remotely sensed imagery, Int. J. Remote Sens., 27, 3025–3033, https://doi.org/10.1080/01431160600589179, 2006.
Yu, L. and Gong, P.: Google Earth as a virtual globe tool for Earth science applications at the global scale: progress and perspectives, Int. J. Remote Sens., 33, 3966–3986, 2012.
Zacks, R. T., Hasher, L., and Li, K. Z.: Human memory, in: the handbook of aging and cognition, edited by: Craik, F. I. M. and Salthouse, T. A., Mahwah, NJ: Erlbaum, 293–357, 2000.
Zhang, G., Zhu, A. X., Huang, Z. P., Ren, G., Qin, C. Z., and Xiao, W.: Validity of historical volunteered geographic information: Evaluating citizen data for mapping historical geographic phenomena, T. GIS, 149–164, 2018.
Żyszkowska, W.: Map perception: theories and research in the second half of the twentieth century, Polish Cartographical Review, 47, 179–190, https://doi.org/10.1515/pcr-2015-0017, 2015.
Żyszkowska, W.: Levels and properties of map perception, Polish Cartographical Review, 49, 17–26, 2017.