the Creative Commons Attribution 4.0 License.
the Creative Commons Attribution 4.0 License.
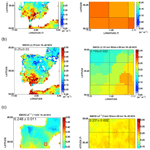
An improved perspective in the spatial representation of soil moisture: potential added value of SMOS disaggregated 1 km resolution “all weather” product
Samiro Khodayar
Amparo Coll
Ernesto Lopez-Baeza
This study uses the synergy of multi-resolution soil moisture (SM) satellite estimates from the Soil Moisture Ocean Salinity (SMOS) mission, a dense network of ground-based SM measurements, and a soil–vegetation–atmosphere transfer (SVAT) model, SURFEX (externalized surface), module ISBA (interactions between soil, biosphere and atmosphere), to examine the benefits of the SMOS level 4 (SMOS-L4) version 3.0, or “all weather” high-resolution soil moisture disaggregated product (SMOS-L43.0; ∼1 km). The added value compared to SMOS level 3 (SMOS-L3; ∼25 km) and SMOS level 2 (SMOS-L2; ∼15 km) is investigated. In situ SM observations over the Valencia anchor station (VAS; SMOS calibration and validation – Cal/Val – site in Europe) are used for comparison. The SURFEX (ISBA) model is used to simulate point-scale surface SM (SSM) and, in combination with high-quality atmospheric information data, namely from the European Centre for Medium-Range Weather Forecasts (ECMWF) and the Système d'analyse fournissant des renseignements atmosphériques à la neige (SAFRAN) meteorological analysis system, to obtain a representative SSM mapping over the VAS. The sensitivity to realistic initialization with SMOS-L43.0 is assessed to simulate the spatial and temporal distribution of SSM. Results demonstrate the following: (a) All SMOS products correctly capture the temporal patterns, but the spatial patterns are not accurately reproduced by the coarser resolutions, probably in relation to the contrast with point-scale in situ measurements. (b) The potential of the SMOS-L43.0 product is pointed out to adequately characterize SM spatio-temporal variability, reflecting patterns consistent with intensive point-scale SSM samples on a daily timescale. The restricted temporal availability of this product dictated by the revisit period of the SMOS satellite compromises the averaged SSM representation for longer periods than a day. (c) A seasonal analysis points out improved consistency during December–January–February and September–October–November, in contrast to significantly worse correlations in March–April–May (in relation to the growing vegetation) and June–July–August (in relation to low SSM values < 0.1 m3 m−3 and low spatial variability). (d) The combined use of the SURFEX (ISBA) SVAT model with the SAFRAN system, initialized with SMOS-L43.0 1 km disaggregated data, is proven to be a suitable tool for producing regional SM maps with high accuracy, which could be used as initial conditions for model simulations, flood forecasting, crop monitoring and crop development strategies, among others.
- Article
(4840 KB) - Full-text XML
- BibTeX
- EndNote
The reliability of climate and hydrological models is constrained by associated uncertainties, such as input parameters. Among them, soil moisture is a variable of pivotal importance controlling the exchanges of water and energy at the surface–atmosphere interface (Entekhabi et al., 1996). Thus, it is a highly relevant variable for climate, hydrology, meteorology and related disciplines (e.g. Seneviratne et al., 2010).
Soil moisture is greatly variable spatially, temporally and across scales. The spatial heterogeneity of soil, vegetation, topography, land cover, rainfall and evapotranspiration are considered responsible for this (Western et al., 2002; Bosch et al., 2007; Rosenbum et al., 2012).
The response of soil moisture to precipitation changes largely depends on soil's water capacity and climatic zones. Particularly, in dry climates such as the Iberian Peninsula (IP), soil moisture reacts quickly to changes in precipitation (Li and Rodell, 2013). Precipitation variability and mean are positively correlated, thus, an increase in precipitation yields wetter soils, which in turn results in higher spatial variability in soil moisture. An adequate representation of the high spatio-temporal variability in soil moisture is needed to improve climate and hydrological modelling (Koster et al., 2004; Brocca et al., 2010). Its impact has been seen on timescales from hours to years (e.g. ∼20 km scale – Taylor and Lebel, 1998; droughts – Schubert and Boche, 2004; decadal drying of the Sahel – Walker and Rowntree, 1977; hot extremes – and Hirschi et al., 2011; decadal simulations – Khodayar et al., 2015b). To obtain an appropriate representation of this variable, especially at high-resolution, is not an easy task, mainly because of its high variability. Methods for the estimation of soil moisture can be divided into three main categories, (i) measurement of soil moisture in the field, (ii) estimation via simulation models, and (iii) measurement using remote sensing. In general, in situ measurements are far from global (e.g. Robock et al., 2000), and model simulations present important biases. Therefore, we have to rely on space-borne sensors to provide such measurements, but until recent times no dedicated, long-term space mission for measuring moisture was attempted (Kerr, 2007).
Nowadays, by means of remote-sensing technology surface soil moisture is available at global scale (Wigneron et al., 2003). The best estimations result from microwave remote sensing at low frequencies (e.g. Kerr, 2007; Jones et al., 2011), and several global soil moisture products have been produced, such as those resulting from the European Space Agency's Climate Change Initiative (ESA CCI, Liu et al., 2011), the Soil Moisture Active Passive satellite (SMAP; Entekhabi et al., 2010), the Advanced Microwave Scanning Radiometer-EOS (AMSR-E; Owe et al., 2008), the advanced scatterometer (ASCAT; Naeimi et al., 2009) and the Soil Moisture and Ocean Salinity (SMOS; Kerr et al., 2001) missions.
The SMOS mission is the first space-borne passive L-band microwave (1.4 GHz) radiometer measuring soil moisture over continental surfaces, as well as ocean salinity, at low frequency (Kerr et al., 2001, 2010). SMOS delivers global surface soil moisture measurements (∼0–5 cm depth) at 06:00 LT and 18:00 LT in a revisit of less than 3 days at a spatial resolution of ∼44 km. The benchmark of the mission is to reach accuracy better than 0.04 m3 m−3 for the provided global maps of soil moisture (Kerr et al., 2001).
SMOS data are not exempt from biases. Validating remote-sensing-derived soil moisture products is difficult, e.g. due to scale differences between the satellite footprints and the point measurements on the ground (Cosh et al., 2004). However, in previous years a huge effort has been made to validate the SMOS algorithm and its associated products. For this purpose, in situ measurements across a range of climate regions were used, assessing the reliability and accuracy of these products using independent measurements (Delwart et al., 2008; Juglea et al., 2010a; Bircher et al., 2012; Dente et al., 2012; Gherboudj et al., 2012; Sánchez et al., 2012; Wigneron et al., 2012). The strategy adapted by the European Space Agency (ESA) was to develop specific land product validation activities over well-equipped monitoring sites. An example of this is the Valencia anchor station (VAS; Lopez-Baeza et al., 2005) in eastern Spain, which was chosen as one of the two main test sites in Europe for the SMOS calibration and validation (Cal/Val) activities. The validation sites were chosen to be slightly larger than the actual pixel (3dB footprint), thus, VAS covers a 50 km×50 km area. Within this area, a limited number of ground stations were installed, relying on spatialized soil moisture information using the SVAT (soil–vegetation–atmosphere transfer) SURFEX (externalized surface) model. Worldwide validation results reveal a coefficient of determination (R2) of about 0.49 when comparing the ∼5 cm in situ soil moisture averages with the SMOS level 2 (SMOS-L2) soil moisture (∼15 km). For example, validation results by Bircher et al. (2012) in western Denmark show an R2 of 0.49–0.67 (SMOS retrieved initial soil moisture) and 0.97 (SMOS-retrieved initial temperature). Besides this, significant under- or overrepresentation of the network data (biases of – 0.092–0.057 m3 m−3) is also found. Over the Maqu (China) and Twente (the Netherlands) regions, the validation analysis resulted in an R2 of 0.55 and 0.51, respectively, for the ascending pass observations, and of 0.24 and 0.41, respectively, for the descending pass observations. Furthermore, Dente et al. (2012) pointed out a systematic SMOS soil moisture (ascending pass observations) dry bias of about 0.13 m3 m−3 for the Maqu region and 0.17 m3 m−3 for the Twente region. Validation of the SMOS level 3 (SMOS-L3) product (∼35 km) shows that the general dry bias in SMOS-L2 is also present in SMOS-L3 SM. This bias is markedly present in the ascending products and shorter time series as described in Sanchez et al. (2012) and Gonzalez-Zamora et al. (2015). In this case, the presence of dense vegetation is seen to increase root-mean-square error (RMSE) scores, whereas in low vegetated areas a lower dry bias is found (Louvet et al., 2015).
Since the launch of the SMOS satellite, the processing prototypes of the SMOS L2 soil moisture have evolved, and their quality has improved. Furthermore, efforts have been made to cover the need for a reliable product with finer resolution for hydrological and climatic studies where the spatial variability in soil moisture plays a crucial role, e.g. in the estimation of land surface fluxes (evapotranspiration – ET – and runoff). Piles et al. (2011) presented a downscaling approach to optimally combine SMOS' soil moisture estimates with MODIS (Moderate Resolution Imaging Spectroradiometer) visible and infrared (VIS/IR) satellite data into 1 km soil moisture maps over the IP without significant degradation of the RMSE. This product has been evaluated using the REMEDHUS (REd de MEDición de la HUmedad del Suelo) soil moisture network in the semi-arid area of the Duero Basin, Zamora, Spain (Piles et al., 2014). Results show that downscaling maintains temporal correlation and root-mean-squared differences with ground-based measurements, hence capturing the soil moisture dynamics. Complementary studies after Piles et al. (2011) have produced similar downscaled high-resolution SMOS level 4 (SMOS-L4) soil moisture products (e.g. Malbéteau et al., 2018; Djamai et al., 2016). Being similar, however, the algorithms creating them are totally different from those of SMOS-L4 products used in this study. Whereas SMOS-L4 products in this study proceed from the original SMOS-L2 (15 km resolution soil moisture) disaggregated by 1 km MODIS LST and the normalized difference vegetation index (NDVI), Malbéteau et al. (2018) and Djamai et al. (2016) products proceed from the original SMOS-L1 (15 km resolution brightness temperature).
A big limitation for the downscaling approach used in Piles et al. (2011) is the lack of information in cloudy conditions of the SMOS level 4 2.0 (hereafter named SMOS-L42.0), which significantly limits the availability and usefulness of this product. In this study, we examine a new version of the SMOS-L4 product, the SMOS level 4 3.0 “all weather” disaggregated ∼1 km SM (SMOS-L43.0), which was developed and has been recently made available by SMOS-BEC (Barcelona Expert Center). In this advanced high-resolution soil moisture product, the limitation on clouds is modulated by the use of ERA-Interim LST data, thus providing soil moisture measurements independently of the cloud conditions.
Contrary to SMOS-L3 and SMOS-L2 products, which have been extensively validated as described above and used for assimilation purposes in models (e.g. De Lannoy et al., 2016), few studies deal with the disaggregated 1 km SMOS-L42.0 and SMOS-L43.0 products (mostly in relation to wildfire activity), and validation efforts have only concentrated on the REMEDHUS soil moisture network in Zamora (north-western Spain; e.g. Piles et al., 2014). The objective of this paper is to provide information about the advantages and drawbacks and the added value of the disaggregated 1 km SMOS-L43.0 “all weather” soil moisture product with respect to coarser resolution products. The proposed investigation covers a 1-year period (a complete hydrological cycle) and focuses on the semi-arid VAS area (eastern Spain) and the IP, where water availability and fire risk are big environmental issues, and knowledge of soil moisture conditions is thus of pivotal importance. Furthermore, as springtime soil moisture anomalies over the IP are believed to be a precursor to droughts and heat waves in Europe (Vautard et al., 2007; Zampieri et al., 2009), accurate monitoring and prediction of surface states in this region may be key for improvements in seasonal forecasting systems.
The following objectives are then pursued: (a) the examination of soil moisture temporal and spatial distribution with SMOS-derived soil moisture products over the investigation domain using a multi-resolution approach for L3 (∼25 km), L2 (∼15 km) and L43.0 (∼1 km), (b) validation with the in situ soil moisture measurements' network (VAS) to estimate the reliability of the SMOS SM products, and (c) evaluation of the impact of realistic SM initialization using SMOS-L43.0 on point-scale and regional SURFEX (ISBA) model simulations over the VAS area.
This investigation is structured as follows. In Sect. 2, the study area and the data sets are presented, including the in situ network measurements, the SMOS data products, and the SURFEX (ISBA) model and related atmospheric forcings used. Section 3 summarizes the methodology applied. The results are discussed in Sect. 4. Finally, conclusions are drawn in Sect. 5.
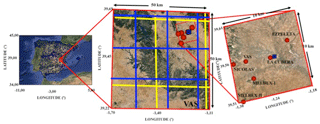
Figure 1Area of investigation and orography. Location of rain gauges from AEMET (Spanish State Meteorological Agency) is shown over the Iberian Peninsula (blue squares). The positions of the soil moisture network stations within the 10 km ×10 km (OBS area) in the VAS (50 km ×50 km) area are indicated by red circles.
2.1 Investigation domain and in situ measurements over the VAS
The main investigation areas in this study are the Iberian Peninsula and the VAS site located in eastern Spain (39.69–39.22∘ N, −1.7–(−1.11∘) W). The VAS site covering an approximately a 50 km ×50 km area was established in December 2001 by the University of Valencia as a Cal/Val site for different low-resolution earth observation data products (Bolle et al., 2006). The extension and homogeneity of the area as well as the mostly flat conditions (slopes lower than 2 %) make it an ideal reference site. Nevertheless, the small variations in the area, 750 to 950 m, influence the climate of the region, which oscillates between semiarid to dry–subhumid. Most of the area is dedicated to vineyards (65 %), followed by trees, shrubs, forest, and industrial and urban cover types. Mostly bare soil conditions are observed beside the vineyard growing season (March or April to September or October). Mean temperatures in the region are between 12 and 14 ∘C, with an annual mean precipitation of about 450 mm and maxima in spring and autumn. Within the VAS, a network consisting of eight ThetaProbe ML2x soil moisture stations was deployed by the Climatology from Satellites Group from the Earth Physics and Thermodynamics Department at the University of Valencia. The eight in situ stations are distributed over a 10 km ×10 km area (Fig. 1), according to land use, soil type and other environmental conditions. Details about the characteristics of each station are summarized in Table 1. Soil moisture measurements every 10 min, mostly from 2006, were carried out for the first 5 cm on the top of the surface. More details about the VAS characteristics and soil moisture measurements could be found in Juglea et al. (2010b). Precipitation measurements over the IP and the VAS are from the AEMET (Agencia Estatal de Meteorología; Spanish State Meteorological Agency) network. Measurements are available for every 10 min.
2.2 The SMOS surface soil moisture products
ESA's derived SMOS Soil Moisture level 2 (SMOS-L2) data product, ∼15 km, contains the retrieved soil moisture and optical thickness as well as complementary parameters such as atmospheric water vapour content, radio frequency interferences and other flags. The SMOS-L2 algorithms have been refined since the launch of SMOS, resulting in more precise SM retrievals (ARRAY, 2014). The SMOS-L3 product was obtained from the operational CATDS (Centre Aval de Traitement des Données) SMOS archive. This is a daily product that contains filtered data. The best estimation of SM is selected for each node when several multi-orbit retrievals are available for a given day. A detection of particular events is also performed in order to flag the data. The processing of the data separates morning and afternoon orbits. The aggregated products are generated from this fundamental product. The SMOS-L4 2.0 data (SMOS-L42.0) with 1 km spatial resolution are provided by BEC and cover the IP, Balearic Islands, Portugal, southern France and northern Morocco (34–45∘ N and 10∘ W–5∘ E). A downscaling method that combines highly accurate, but low-resolution, SMOS radiometric information (SMOS-L2 data) with high-resolution (brightness temperature measurements), but low sensitivity, visible-to-infrared imagery (NDVI) and LST (land surface temperature, from Aqua MODIS) to point-scale surface SM (SSM) across spatial scales is used to derive the SMOS-L42.0 data (Piles et al., 2011). The impact of using different vegetation indices from MODIS with higher spatial and temporal resolution in the downscaling method was explored in Sanchez-Ruiz et al. (2014), showing that the use of more frequent and higher spatial-resolution vegetation information leads to improved SM estimates. The latest SMOS-L4 product is the version 3.0, or “all weather” (SMOS-L43.0), which is the product used and examined in this study. The downscaling approach is based on Piles et al. (2014) and Sanchez-Ruiz et al. (2014), with the novelty of introducing ERA-Interim LST data in the MODIS LST and NDVI scape, thus providing soil moisture measurements independently of the cloud conditions. ERA-Interim provides a resolution of about 0.125∘, whereas MODIS is a ∼1 km product. The evaluation of the SMOS-L4 2.0 and 3.0 products support the use of the “all weather” version, since it does not depend on cloud cover, and the accuracy of the estimates with respect to in situ data is improved or preserved (Piles et al., 2015; SMOS-BEC Team, 2016).
In this study, the SMOS-L2 V5.51 data coming from a L1C input product (obtained from MIRAS measurements), the SMOS-L3 V2.72 and the SMOS-L4 V3.0 are employed.
2.3 The SURFEX (ISBA) SVAT model
The SVAT model SURFEX (Le Moigne et al., 2009), module ISBA (Noilhan and Planton, 1989), is used to generate point-scale and spatially distributed SM at 1 km grid spacing and temporal fields from initial conditions and atmospheric forcing. SURFEX (ISBA) was developed at the National Centre for Meteorological Research (CNRM) in Météo, France, and it has been widely validated over vegetated and bare surfaces (e.g. Calvet et al., 1998). The ISBA scheme uses the Clapp and Hornberger (1978) soil water model and Darcy's law for the estimation of the diffusion of water in the soil, and it allows for 12 land use and related vegetation parameterization types. Crops are considered for the VAS area, since the region is mainly composed of vineyards, almond and olive trees, and shrubs.
The surface characteristics are considered in the SVAT input, roughness and the fraction of vegetation are adopted from ECOCLIMAP (Masson et al., 2003), topography is obtained from GTOPO (GTOPO30 Documentation, 1996), and soil types are defined using FAO (FAO, 2014).
To obtain an accurate simulation of soil moisture in the study area, the model was originally calibrated by Juglea et al. (2010b) to be applied over the entire site for any season or year. Particularly relevant to this study is the specific definition of the soil hydraulic parameters which they made for the VAS area, since most of the hydrological parameters are site dependent and are not available from SMOS observations. A new set of empirical equations as a function of the percentages of sand and clay was defined using Cosby et al. (1984) and Boone et al. (1999). New definitions and recommendations by Juglea et al. (2010b) for the VAS area were adopted in this investigation.
Atmospheric forcing information: ECMWF and SAFRAN
High-quality atmospheric forcing is needed to carry out accurate simulations. To run the SURFEX (ISBA) model, the following atmospheric forcing data are needed: air temperature and humidity at screen level, atmospheric pressure, precipitation, wind speed and direction, and solar and atmospheric radiation. Three different sets of atmospheric forcing information are used in this study as input forcing for the SURFEX (ISBA) simulations: (a) SURFEX-OBS, which consists of meteorological data from three fully equipped stations in the OBS area, MELBEX-I, MELBEX-II and VAS, (b) SURFEX-ECMWF, which consists of ECMWF (European Centre for Medium-Range Weather Forecasts) data, and (c) SURFEX-SAFRAN, information from the SAFRAN (Système d'Analyse Fournissant des Renseignements Atmosphériques à la Neige) meteorological analysis system (Quintana-Seguí et al., 2008; Vidal et al., 2010).
Precipitation, air temperature, surface pressure, air-specific humidity, wind speed and direction, downward longwave radiation, diffuse shortwave radiation, downward direct shortwave radiation, snowfall rate and CO2 concentration are used as input data from the aforementioned meteorological stations in the OBS area. A temporal resolution of 10 min is available. From ECMWF, the dew point and temperature at 2 m, pressure, precipitation, and wind components are used as forcing data, with a 6 h temporal resolution and spatial resolution. Precipitation, air temperature, surface pressure, air specific humidity, wind speed, and downward shortwave and longwave radiation from SAFRAN are used as input information, with a spatial resolution of 8 km ×8 km and an hourly temporal resolution. In the last case, we have an optimal spatial and temporal distribution of the atmospheric forcing over the VAS area (∼50 km ×50 km) and a rarely found complete database to force the land surface model. More details about the SAFRAN system and its validation in north-eastern Spain can be found in Quintana-Seguí et al. (2016).
In order to investigate the characteristics and potential added values of fine-scale SMOS-derived soil moisture, the spatial variability, the temporal evolution and the probability distribution are investigated. For this purpose, SMOS-derived soil moisture products at different spatial resolutions, in situ measurements and model simulations are jointly evaluated.
The spatial distribution and temporal evolution of precipitation and SMOS-derived soil moisture over the IP and the VAS area are assessed for the time period from December 2011 to December 2012, also considering hydrological seasons (DJF: December–January–February, MAM: March–April–May, JJA: June–July–August, SON: September–October–November). Special attention is paid to the autumn season, since in this period the western Mediterranean is characterized by a large thermal gradient between the atmosphere and the sea (Duffourg and Ducrocq, 2011, 2013), resulting in intense precipitation extremes (Raveh-Rubin and Wernli, 2015). Furthermore, during 2012, the Hydrological Cycle in the Mediterranean Experiment (HyMeX; Dobrinski et al., 2014) took place in the western Mediterranean, with the IP and particularly the Valencia region as target areas. During the SON period of 2012, the special observation period (SOP1; Ducrocq et al., 2014) took place with intensive experimental deployment over the area. This provides us with valuable information about the environmental conditions as well as the occurrence of precipitation events in the investigation area. Particularly, precipitation in the IP during the autumn (SON) period of 2012 was above average (Khodayar et al., 2016). It was also the hydrological season in which higher variability in the soil moisture was observed as a result of the precipitation distribution. Two unique events, one at the end of September (27–29) affecting southern and eastern Spain and the other at the end of November (19–20) affecting the Ebro Valley (Jansà et al., 2014), largely determined the positive anomaly in precipitation and soil moisture in this period.
SMOS-L3 (∼25 km), SMOS-L2 (∼15 km), and SMOS-L43.0 (∼1 km) are used for the evaluation of soil moisture distribution at different grid spacing. Piles et al. (2014) pointed out that differences may exist between SMOS-L3 and SMOS-L2 and the 1 km disaggregated soil moisture SMOS-L4 because of the distinct methodology used to obtain these products. Only SMOS descending passes or a mean between ascending and descending passes are used to calculate mean daily values of SMOS-derived soil moisture. Soil moisture derived from the afternoon orbits was found to be more accurate than the morning passes (Piles et al., 2014). The fine temporal resolution of the model simulations (1 h) and the observations (10 min) allow comparisons at the time of the SMOS overpasses. Because of the 3-day revisit period of the SMOS swath, the IP is not fully covered by the satellite on daily basis. However, despite identified difficulties (radio frequency interferences, missing data, etc.), the IP is well observed, with 1.5 days being the average observation frequency over the IP. Only those images with coverage higher than 50 % are considered in our calculations. A conservative remapping to coarser resolutions is applied, when required, to make comparisons among each other or with respect to ground-based observations on equal terms. Remapping allows point-to-point comparisons between these data sets. In addition to the yearly and seasonal approach, an exemplary short time period, 19 to 20 October 2012, is considered. This corresponds to one of the periods in which an extreme precipitation event occurred in the Ebro Valley (at the end of November; Jansà et al., 2014). Therefore, high variability in the soil moisture distribution is expected.
The coefficient of variation (CV), defined as the ratio of the standard deviation to the mean, of the precipitation and soil moisture fields over the IP, the VAS (50 km ×50 km) and the OBS (10 km ×10 km) areas are examined for the analysis of the spatial variability and its evolution in time. The soil moisture daily index (SMindex,i) is calculated to assess the evolution pattern, allowing the study of daily variations.
SM (SMi+1 -SMi)/ SMi, where SMi+1 is the soil moisture of the day i+1, and SMi is the soil moisture of the day before i.
For these calculations, SMOS afternoon (descending; Piles et al., 2014) orbits as well as observations at the time of the SMOS overpasses are selected. For the IP and VAS, SMOS-L2 and SMOS–L43.0 have been remapped to the coarser grid spacing for an adequate comparison. Ground-based observations are aggregated using a mean over all stations for comparison with the corresponding SMOS-L43.0 data (the closest grid point is selected).
The reliability of SMOS-L3, SMOS-L2 and SMOS-L43.0 soil moisture products is evaluated by comparison with in situ soil moisture measurements in the OBS area. The spatial and temporal variability are considered as well as the probability distribution. Different approaches are applied: (a) the nearest grid point is selected for point-like comparisons between SMOS-L2 and SMOS-L43.0 against in situ soil moisture stations, to reduce sampling biases in this region of diverse soil characteristics (Table 1), and (b) SMOS-L43.0 soil moisture grid cells are averaged over the 10 km ×10 km area and compared to the mean from the soil moisture network stations to address the issue related to spatial averaging due to the high spatial and temporal variability in the uppermost SSM. For the comparison between the SMOS-L2 and the in situ observations, when single ground-based stations are considered, the closest SMOS pixel is selected, and in the case of considering the OBS (10 km ×10 km) or VAS (50 km ×50 km) areas, the mean over all pixels whose centre falls within the area is used. For the comparison with SMOS descending passes the corresponding values from in situ measurements are considered. Additionally, a separation between wet days (precipitation over 1 mm d−1) and dry days is applied to consider possible implications of wet and dry soils for SMOS measurements.
Linear regression, the coefficient of determination (R2), the mean bias (MB) and the root-mean-square deviation (RMSD) are used to predefine the accuracy. A de-biased or centred RMSD (CRMSD) is applied to discriminate the systematic and random error components, removing the overall bias before calculating the RMSD.
Soil moisture modelling is performed by the use of the SVAT SURFEX (externalized surface), module ISBA, from Météo-France. Configuration and specifications described in Juglea et al. (2010b), which proved successful in adequately simulating the associated soil moisture heterogeneity over the wide VAS surface (50 km ×50 km), are adapted in this study. Simulations start on 1 December 2011 at 00:00 UTC and cover the whole investigation period until 31 December 2012, with an hourly output time resolution. Point-scale SURFEX (ISBA) simulations over the soil moisture network stations in the VAS domain are validated with the in situ measurements to assess the usefulness of the model in further investigation, illustrating the potential of the model in simulating the upper-level soil moisture variability of different soil characteristics (Table 1).
To try to simulate the spatial and temporal heterogeneity of the soil moisture fields over the VAS surface, the SURFEX (ISBA) scheme is used in combination with high-quality forcing data from ECMWF (hereafter SURFEX-ECMWF) and the SAFRAN system (hereafter SURFEX-SAFRAN) for spatialization purposes. Soil moisture initialization in spatialized SURFEX (ISBA) simulations requires a single representative value for the whole simulation area. The benefit of initializing the simulations with SMOS-L43.0 data in comparison to climatological means is discussed. In-situ soil moisture observations over the VAS area are considered for verification. A comparison between SURFEX-SAFRAN point-scale and 10 km ×10 km mean simulations initialized with SMOS-L43.0 data is made against ground measurements to assess the accuracy of the simulated SSM maps.
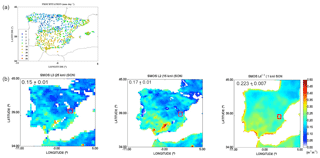
Figure 2(a) spatial distribution of precipitation over the Iberian Peninsula from the network of rain gauges of AEMET. The period of September to November (SON) 2012 is shown. (b) spatial distribution of SMOS-derived soil moisture over the Iberian Peninsula (merged product: ascending and descending orbits, days with areal coverage higher than 50 % are considered).
4.1 SMOS-derived soil moisture at different resolutions
4.1.1 Spatial variability on seasonal and sub-seasonal timescales
Figure 2a shows the north–south precipitation gradient for the SON period mean. The SSM satisfactorily reflects this gradient (Fig. 2b), but it does this more markedly for the SMOS-L3 and SMOS-L2 than the higher-resolution SMOS-L43.0, showing lower standard deviation in SMOS-L3 (), SMOS-L2 () and SMOS-L4 (). The same performance is seen over the VAS domain (not shown). The SSM variability associated with the extreme precipitation events in this period is not well represented in the SMOS-L43.0 seasonal mean. Table 2 shows the number of days (percentage) in which there is more than 50 % of data for the IP for each SMOS product. These periods have been used as basis for the calculation of the spatial distributions in Fig. 2b. SMOS-L3 (88 %) and SMOS-L2 (84 %) show good coverage and a similar number of days. However, a large difference is observed with respect to the SMOS-L42.0 product with only 28 days (32 %) of adequate coverage for the period of SON 2012. This is due to the problem associated to the downscaling approach used to obtain the 1 km soil moisture maps, in which the lack of LST information from MODIS VIS/IR satellite data in cloudy conditions (Sect. 2.2) constrains derived-SSM information. The availability and usefulness of this product is therefore significantly reduced. The new product L43.0 used in this study, in which the previous limitation is resolved using ERA-Interim-derived LST information, shows a coverage percentage of the order of 92 %, even higher than the SMOS-L3 and SMOS-L2 products. However, Fig. 2b demonstrates that the spatial representation of the seasonal mean does not improve with this product, as a consequence of the limited temporal availability of the SMOS-derived SSM product dictated by the revisit period of the satellite.
Table 2Number of days (percentage) in which the SMOS (ascending and descending swaths) coverage is higher than 50 %.
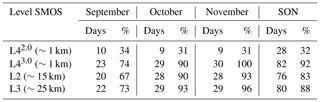
In Fig. 3, only common available days from all different operational levels are selected for an inter-SMOS product comparison. When remapped to the same resolution (coarser grid spacing), comparable values are identified between SMOS-L3, SMOS-L2 and SMOS-L43.0 for the JJA and SON period, whereas relevant differences are pointed out from December to May. In this last period, we identify higher means for the SMOS-L43.0 product and SMOS-L3 with respect to SMOS-L2, which is in agreement with a systematic dry bias also identified for SMOS-L2 in previous studies (Sect. 1).
At sub-seasonal scales, e.g. event scale on the 19–20 November 2012 (Fig. 4), the SMOS-L43.0 product shows SSM mean and variability in the same range as the SMOS-L2 and SMOS-L3 products, but with a finer improved resolution representation of the spatial distribution. Comparisons with the mean ground-based SSM at the VAS (OBS area: 0.25±0.0002) show better agreement with the mean SSM from the SMOS-L43.0 1 km disaggregated product (0.23±0.002) and poorer correlation with SMOS-L2 (0.20±0.002). The problem of SMOS-L43.0 on seasonal timescales vanishes at sub-seasonal (event) scales where the potential added value of the 1 km product is manifest.
4.1.2 Temporal evolution of surface soil moisture data sets
The SMOS and in situ measured SSM time series are investigated and compared in this section in Figs. 5 and 6 over the IP, the VAS (50 km ×50 km) and the OBS (10 km ×10 km) areas. Overall, the averaged SMOS-L2 and SMOS-L43.0 data over the IP are much more variable than the SMOS-L3, showing a more extreme daily index (SMOS-L2: −1 to 2; SMOS-L43.0: −0.7 to 1.45). Over the VAS, SMOS-L2 is clearly more variable than the higher resolution SMOS-L43.0. But the last one shows a wider range of values as well as more extreme daily index values when compared to the averaged in situ soil moisture measurements. The CVs of the spatially averaged SMOS-L43.0 are lower than those of SMOS-L3, SMOS-L2 and in situ observations, indicating that this data are less scattered. Despite detected differences within in situ observations, SMOS responds well to soil moisture variations over time.
Table 3Statistics of the comparisons between SMOS-L2 and SMOS-L43.0 soil moisture and ground-based measurements in the VAS network (the area covering the ground-based network has been called OBS; Fig. 1). SMOS descending orbits are selected for the comparison. Characteristics of the individual stations are given in Table 1. The acronyms for the names of the stations are as follows. M-I: Melbex-I, M-II: Melbex-II, VAS: VAS, NIC: Nicolas, EZ: Ezpeleta, LC: La Cubera. The period December 2011 to December 2012 is evaluated. The seasonal analysis follows the hydrological cycle. OBS stands for the average of (i) SMOS-L2 and/or SMOS-L43.0 soil moisture values within the 10x10 km2 where the ground-based network is placed, and (ii) in the case of the in situ observations, it refers to the mean of all stations. (a) shows a seasonal comparison between the mean of all in situ stations and the corresponding mean of SMOS-L2 and/or SMOS-L43.0 soil moisture values within the 10 km ×10 km area. In (b) SMOS-L2 and SMOS-L43.0 soil moisture observations are compared to point-like ground measurements using the closest grid point. The column on the right shows the mean of all stations.
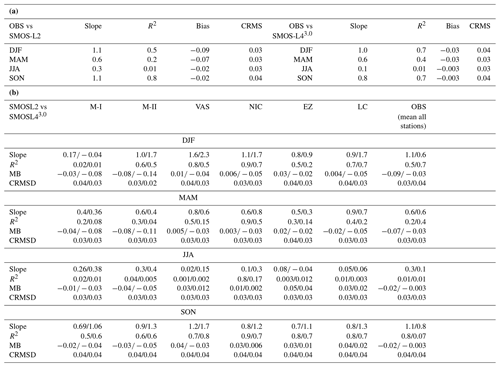
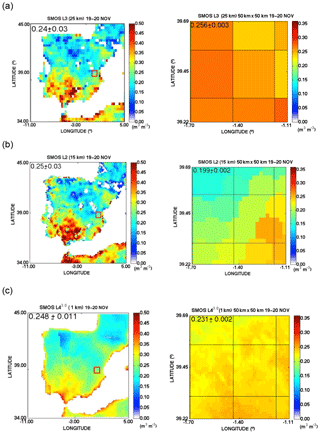
Figure 4Spatial distribution of SMOS-derived soil moisture (merged product: ascending and descending orbits are considered) over the Iberian Peninsula (left) and the VAS (right) as a mean for the 19–20 November 2012 (a) SMOS-L3 (∼25 km), (b) SMOS-L2 (∼15 km) and (c) SMOS-L43.0 (∼1 km). Empty pixels in (a) and (b) are indicative of a lack of data. Please be aware of the different colour scale used for the IP and VAS.
Although absolute values are not totally captured, all three SMOS products adequately reproduce the temporal dynamics at a regional scale. The systematic dry bias present in SMOS-L2 data (Piles et al., 2014) is evident, particularly in the first half of the year. A mean bias of the order of −0.09 to −0.07 m3 m−3 is identified for the DJF–MAM period; this difference is reduced to −0.02 m3 m−3 for the JJA–SON period (Table 3). During the DJF–MAM period the vineyards are bare, and only the vine stocks are present. The water content of the vine stocks negatively impacts the SMOS measurements (Schwank et al., 2012).
Good agreement is found between the SMOS-L43.0 product and the mean of the in situ observations (the network's variability (Fig. 6c; shaded grey contains the SMOS-L43.0 data). Scores confirm this result, particularly for the periods DJF and SON (slope ∼1, R2∼0.7). Poorer correlation is found for the MAM (slope ∼0.6, R2∼0.4). In this period, immediately after the precipitation events, soil moisture maxima are not always well captured by the SMOS-L43.0 data, additionally showing a drying after this that is too rapid. This observation agrees with the SMOS mission's inability of correctly measuring in situations when liquid water is present at the soil. The measured signal is perturbed during the vegetation growing season, which could explain the worse statistics. On the other hand, during JJA, a low slope of ∼0.1 and R2of∼0.01 could be in relation to SSM values close to or lower than 0.1 m3 m−3 with very low spatial variability, which was found to be necessary for an adequate performance of the algorithm used for the derivation of the SMOS-L4 1 km product in Molero et al. (2016).
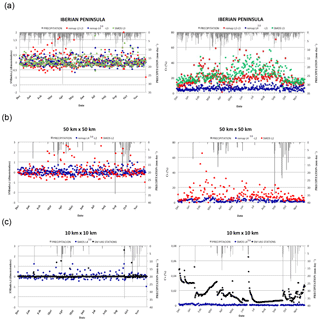
Figure 5Averaged SMOS products and averaged ground-based observations of soil moisture evolution over the Iberian Peninsula (IP; a), the VAS area (b) and the OBS area (c). Descending orbits are used. Precipitation from AEMET rain gauges are shown at the top of each plot. The soil moisture daily index (Ov index,i; dimensionless) is shown in the left-hand plots, and the coefficient of variation (Cv, %) is shown in the right-hand plots.
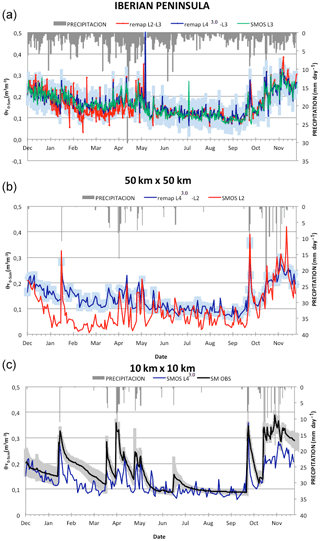
Figure 6Temporal evolution of surface soil moisture time series averaged over the Iberian Peninsula (a), the VAS area (50 km ×50 km; b) and the OBS area (10 km ×10 km; c). SMOS afternoon orbits are considered. Daily mean precipitation from the AEMET stations is shown on the top of each plot. SMOS and remapped SMOS products are indicated in the plots. Shaded areas show standard deviations.
4.2 Spatial comparison at high-resolution: SMOS-L43.0 versus ground measurements
High-resolution spatio-temporal correlations are assessed by spatial comparison with in situ observations. Characteristics of each of the in situ stations are presented in Table 1. A seasonal analysis is performed focusing on the selected year of measurements covering a complete hydrological cycle (from 1 December 2011 to 31 December 2012). Comparisons between SMOS-L2 and ground measurements are additionally included. Statistics for individual comparisons at all stations are summarized in Table 3. Comparisons between SMOS-L3 and ground measurements were similarly performed, evidencing the expected bad correlations (R2∼0.002, not shown). In Fig. 7, the scatter plots display (a) possible differences between dry and wet days (> 1 mm d−1) and (b, c) the agreement between remotely sensed and in situ soil moisture measurements from the OBS network using the seasonal classification. To consider any uncertainties arising from spatial averaging, ground measurements are compared to point-like and 10 km ×10 km SSM means. The 10 km ×10 km area used covers the OBS area, i.e. the network of in situ measurements within the VAS. For comparison, all grid points from SMOS-L43.0 and SMOS-L2 included within the area are considered.
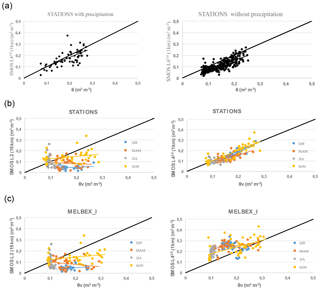
Figure 7Results of the seasonal analysis for the hydrological year starting in December 2011. Scatter plots of (a) SMOS-L43.0 SSM (ascending and descending orbits) versus averaged 10 km ×10 km in situ soil moisture measurements for days with precipitation (left) and for days without precipitation (right; < 1 mm d−1). (b) SMOS-L2 and SMOS-L43.0 SSM (descending orbits) versus averaged 10 km ×10 km in situ soil moisture measurements. (c) SMOS-L2 and SMOS-L43.0 SSM (descending orbits) versus point-like ground measurements from MELBEX-I station, using the closest grid point. Segments are linear fit of seasonal data (3 months of data). Statistics for individual comparisons at all stations are summarized in Table 3.
In Fig. 7a, the separation between days with and without precipitation (< 1 mm d−1) points out more similar correlations during dry days than wet days (RMSD ∼0.015, R2∼0.7) for SMOS-L43.0, whereas a slightly better agreement is found for the dry days (not shown) for SMOS-L2. A systematic mean dry bias of about 0.05 (dry days) to 0.08 (wet days) m3 m−3 is assessed for SMOS-L2, while a lower bias with changing sign is identified for the L43.0 product (∼0.005 for wet days; for dry days). Comparisons using the corresponding mean over the 10 km ×10 km OBS area, shown in Fig. 7b and Table 3, show good agreement with respect to the SMOS-L43.0 and poorer scores for SMOS-L2 (only one grid point of SMOS-L2 is located within the OBS area). Worse consistency is found in both cases for the MAM and JJA periods. CRMSD is, in all cases, in the required range of ≤0.04 m3 m−3. Point-like comparisons with the individual in situ stations, shown in Fig. 7c and Table 3, show that spatial patterns are captured at 1km with RMSD ∼0.007 to 0.1 m3 m−3, but in most cases, accuracy for the SMOS-L43.0 1 km disaggregated product is within the required range of less than 0.04 m3 m−3 (not shown). Higher RMSD is found for SMOS-L2, ∼0.008 to 0.13 m3 m−3, accounting for the previously identified dry bias ()–(−0.02)) reduced in SMOS-L43.0 ()–(−0.01)). The CRMSD is, in all cases, ≤ 0.04 m3 m−3. For all stations, better correlations are found in DJF and SON and poorer scores in JJA and MAM, in agreement with the areal mean comparisons (Sect. 4.1.3). Best scores are obtained for the Nicolas, VAS and La Cubera stations, probably in relation to their common soil type distribution over vineyards and homogeneous conditions over a plain (Fig. 8a, Table 3). The SON time period reveals the best agreement; at this time the vineyards are completely grown (however, senescent thus containing less water), and the SSM exhibits substantial spatial variability driven by precipitation and irrigation, thus improving spatio-temporal correlations. Worse statistics are found for Melbex-I, Melbex-II and Ezpeleta, probably in relation to the location of the soil moisture probes in rockier and orographically more complex areas, which are also in proximity to forest and man-made construction areas.
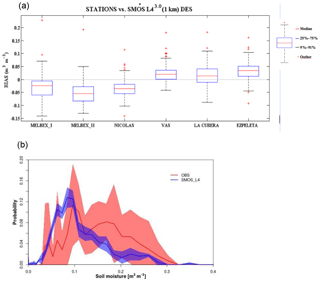
Figure 8(a) box plot of the comparison between point-like ground measurements at all stations over the VAS area and closest SMOS-L43.0 SSM data. (b) probability distribution function (PDF) of SSM from in situ observations and SMOS-L43.0 SSM measurements. The standard deviations are indicated with shaded areas. Solid lines represent the mean over all ground stations and over the 10 km ×10 km of the OBS area in VAS, where the in SSM network is located.
The soil moisture probability distribution function (PDF; Fig. 8b) of all in situ measurements versus SMOS-L43.0 data reveals that the latter overestimates SSM below 0.1 m3 m−3, values mainly observed during the JJA period. But an underestimation occurs in the range between 0.1 and 0.3 m3 m−3, which is consistent with the identified underestimation of maximum soil moisture reached after a precipitation event and the rapid drying of the soil in comparison to the much slower response seen in the observations during the MAM period (Fig. 6c).
4.3 SURFEX model simulations and realistic initialization with 1 km soil moisture data
4.3.1 SURFEX model simulations of selected stations and realistic initialization
As a first step, the performance of the SURFEX (ISBA) SVAT model is evaluated. SURFEX (ISBA) point-like simulations are performed for all in situ soil moisture stations at the VAS area to assess the usefulness of the model for further investigation (Table 4).
SURFEX (ISBA) simulations show good agreement with soil moisture ground-based observations at all stations, adequately capturing the associated spatio-temporal variability (slope ∼1, R2∼0.7 to 0.9; MB ∼0.1 m3 m−3; CRMSD ∼0.02 m3 m−3). It can be concluded that the model performs well and is therefore suitable for further investigation. The seasonal analysis points out the best simulations in the SON period (R2∼0.9 for all stations), but CRMSD is ≤0.04 m3 m−3 for all stations at all periods.
Using the mean of the ground-based measurement on the day of the model simulation initialization (realistic initialization; REAL-I) the temporal mean comparison for each station presented in Fig. 9 and Table 4 reveals mean R2∼0.8 when the whole hydrological year is considered.
4.3.2 Spatialization
As a first step, point-scale SURFEX-ECMWF and SURFEX-SAFRAN simulations covering the whole investigation period are performed for all in situ soil moisture stations to examine their ability to reproduce soil moisture dynamics. Ground measurements at each station are used for initialization. Scores clearly indicate better agreement with all in situ observations for the SURFEX-SAFRAN simulations (slopes ∼1, R2∼0.9, RMSD < 0.1 m3 m−3), rather than the SURFEX-ECMWF simulations (slopes > 1, R2∼0.6, and RMSD > 0.1 m3 m−3).
In a second step, SURFEX-ECMWF and SURFEX-SAFRAN simulations are spatialized to obtain maps of soil moisture over the investigation area. In our CTRL simulations, the daily soil moisture from the mean of the in situ measurements on the initialization day is used for model initialization. The mean SSM from in situ measurements for the whole investigation period is of the order of 0.14±0.005, whereas the SURFEX-ECMWF-derived SSM field is about 0.18±0.007 and the SURFEX-SAFRAN-derived SSM field is 0.15±0.002, thus being closer to ground-based observations. Performing a seasonal analysis, we demonstrate that this consistency is maintained for all seasons (not shown). The higher resolution of the SAFRAN atmospheric forcing better reproduces the high spatial heterogeneity over the VAS area, resulting in improved mapping of simulated SSM.
To exemplify the importance and implications of soil moisture initialization, several experiments are performed. Initialization of the SURFEX-SAFRAN simulation using SMOS-L43.0 (EXP-SMOS) is examined against a sensitivity simulation using the climatological soil moisture from observations for the initial soil moisture scenario (daily mean over 10 years, which has been selected to be far from observations; EXP-CLIM). These experiments are initialized in dry periods, following Khodayar et al. (2015) recommendations, to maximize the impact and run for about 3–4 months. In the first case, initialization is performed in a winter month (December), and the whole simulation period remains almost dry. In the second case, a summer month (July) is chosen for the initialization, and it is followed by a wet autumn period with frequent heavy precipitation events in the area.
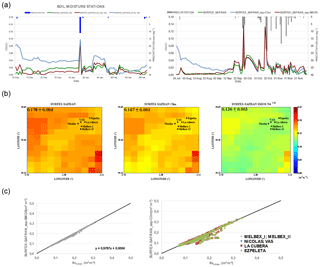
Figure 10(a) RMSD for the daily mean SSM from the three SURFEX (ISBA) simulations with perturbed initial SSM scenarios (details in Sect. 4.3.2). (b) spatial distribution of mean SSM for the winter simulation (left-hand side, a) for the three simulations. (c) scatter plot depicting the comparison between in situ SSM observations and SURFEX–SAFRAN–SMOSL43.0 simulations, as a mean over all stations (left) and for each of the stations (right).
The temporal evolution of the RMSD (Fig. 10a) demonstrates that the initial soil moisture scenario influences its evolution until the end of the simulation, in agreement with previous results in Sect. 4.3.1. Larger deviations occur during dry periods in both scenarios. Longer spin-up times, defined as the time that soil needs to re-establish quasi-equilibrium, characterize the dry scenario. It is after heavy precipitation events that deviations decrease. Soil quickly reacts to changes in the precipitation field in the semi-arid IP. When the upper-level soil gets close to saturation soil, memory is almost lost. Before the high precipitation events, SSM evolves following the direction of the initial perturbation, i.e. higher initial SSM yields higher SSM; however, a stochastic behaviour is identified afterwards.
As an example, differences in the spatial distribution of soil moisture for the winter or dry-period simulation are discussed (Fig. 10b). A relevant difference in the mean is identified when compared to the CTRL simulation (0.17±0.004): EXP-CLIM (0.014±0.003) and EXP_SMOS (0.17±0.003). Clearly, better agreement is found in the last case.
Considering the EXP-SMOS initialization scenario simulation, a comparison between simulated point-like and the 10 km ×10 km mean against corresponding ground measurements was done for verification (Fig. 10c). Correlations of the order of R2∼0.9 confirm that the combined use of SURFEX-SAFRAN and SMOS-L43.0 for initialization successfully reproduces soil moisture spatial and temporal variability becoming an optimal tool for mapping soil moisture heterogeneity over a study region for diverse purposes.
High-resolution soil moisture products are essential for our understanding of hydrological and climatic processes as well as improvement of model skills. Due to its high spatial and temporal variability, it is a complicated variable to assess. Mapping high-resolution soil moisture fields using intensively collected in situ measurements is infeasible. Thus, state-of-the-art high-resolution modelling and satellite-derived products have to fill this gap, although verification is needed. In this study, we examine the potential of the state of the art SMOS-L43.0 1 km “all weather” disaggregated product for the assessment of soil moisture variability and improvement of the SVAT SURFEX (ISBA) simulations, in combination with the SAFRAN meteorological analysis system (SURFEX-SAFRAN), through realistic initialization. A dense network of ground-based soil moisture measurements over the Valencia anchor station (VAS; one of the SMOS test sites in Europe) is used for verification. The proposed analysis focuses on the semi-arid IP and covers the 1-year period of 2012 (from December 2011 to December 2012). The comparison of the SMOS-L43.0 1km product to different grid spacing soil moisture data products from SMOS, namely SMOS-L3 (∼25 km) and SMOS-L2 (∼15 km), shows that on seasonal timescales, SMOS-L43.0 does not accurately capture the spatial variability in the soil moisture field, contrary to SMOS-L3 and SMOS-L2, despite the novelty of introducing ERA-Interim LST data to the MODIS LST and NDVI space (Piles et al., 2014; Sanchez-Ruiz et al., 2014). This is probably in relation to the very different spatial resolution of ERA-Interim and MODIS. This new downscaling approach greatly enhances the potential applicability of the data for the days or periods in which measurements are available, but cannot accurately fill in those periods without measurements dictated by the revisit period of the SMOS satellite, hence compromising the soil moisture representation as a mean for longer periods than a day. On sub-seasonal timescales, when SMOS images are available, the SMOS-L43.0 high-resolution product shows its potential. It adequately captures the surface soil moisture variability in association with the precipitation field, also capturing this variability when extreme precipitation takes place.
Mean and single-station comparisons with in situ measurements reveal that characteristics of SMOS-L43.0 soil moisture fields are closer to in situ observations than SMOS-L3 and SMOS-L2 products. Point-like and 10 km ×10 km comparisons show good agreement with respect to the SMOS-L43.0 and poorer scores for SMOS-L2 (e.g. in the DJF period, for SMOS-L3 and SMOS-L2, the slope was 1.1 and 1.0, the R2 was 0.5 and 0.7, and the bias was −0.09 and −0.03, respectively). Generally, all three SMOS products adequately reproduce the soil moisture temporal dynamics meeting the desired accuracy of the mission (0.04 m3 m−3); however, the spatial patterns did not always reach the expected precision in agreement with former studies in other regions (Gonzalez-Zamora et al., 2015). Comparisons with ground soil moisture measurements from the eight stations in the OBS network (10 km ×10 km) over the VAS area show that the spatial patterns are captured at 1 km with an RMSD of ∼0.007 to 0.1 m3 m−3. The best correlations are in DJF and SON, and poorer scores in MAM and JJA, in agreement with the areal-mean comparisons. SMOS-L43.0 data show better agreement at those stations over plain areas and those with uniform conditions (vineyards), compared to those over more complex and less homogeneous terrains (rocky soils and areas close to forest and man-made constructions). The SMOS-L43.0 soil moisture probability distribution function (PDF), in comparison to that of the in situ measurements, reveals a SMOS overestimation below 0.1 m3 m−3 and an underestimation in the range of 0.1 to 0.3 m3 m−3. A seasonal analysis points out better scores for the DJF and SON periods, whereas poorer correlation is found for the MAM and JJA periods. In the MAM period, an under-representation of the rainy events, as well as faster and stronger drying changes coinciding with the vegetation growth season, is found. In JJA, the very low soil moisture values (< 0.1 m3 m−3) with associated low spatial variability result in low R2. No significant differences are found during dry and wet days (> 0.1 mm d−1).
SURFEX (ISBA) SVAT simulations covering the whole investigation period over all in situ measurement stations at the VAS area show good agreement with ground-based observations. Mean values are well reproduced for all stations, and the temporal variability is well captured (R2∼0.7 to 0.95; RMSD ∼0.02). The synergetic use of SURFEX (ISBA) simulations with SAFRAN atmospheric forcing information initialized with realistic SSM values from the SMOS-L43.0 data set was a successful combination for obtaining soil moisture maps over the VAS domain. Good agreement was reached when comparisons between point-like and 10 km ×10 km simulations with SURFEX-SAFRAN initialized with SMOS-L43.0 data and in situ soil moisture measurements were made (R2∼0.9 and RMSD < 0.04 m3 m
In this study, the comparison and suitability of different operational satellite products from the SMOS platform is investigated to provide realistic information on the water content of the soil. The comparison carried out helps with drawing guidelines on best practices for the sensible use of these products. Currently, there is not a consensus about what the “best” SMOS product is. Different users utilize different products depending on their application, rather than based on performance arguments. This study and the conclusions obtained from the comparison are important in providing information on the advantages and drawbacks of these data sets. The high temporal and spatial resolution soil moisture maps obtained in this study could be of use for hydrological and agronomical applications, for building climatologies of SSM, as initial condition for convective system modelling, for flood forecasting, and for downstream local applications such for as crop monitoring and crop development strategies as well as for irrigation data sets, among others. Additionally, an accurate representation of SSM will permit the calculation of SM profiles by the application of exponential filters, for example, which has been demonstrated to be a successful technique. Furthermore, the added value of the SMOS-L43.0 1 km disaggregated product for initialization purposes is demonstrated, which suggests its potential for assimilation purposes. These two last aspects are out of the scope of this paper, but they are investigated in detail in a follow-up study. Important aspects of the SMOS-L43.0 SSM product must still be improved, namely its temporal availability (e.g. successful investigations on the increase of SMOS-L3 temporal resolution to 3 h are available; Louvet et al., 2015) and its spatio-temporal correlation with in situ measurements over complex topographic areas, in areas or periods with low spatial variability, and in rainy periods when an under-representation and rapid decay of SSM has been identified. This study also points out that, in order to more accurately examine the reproducibility of the high spatial variability in this variable by the newly available satellite-derived downscaled high-resolution soil moisture observations, large and dense networks of in situ soil moisture measurements covering different soil types and land uses as well as considering different soil depths are needed. In an effort to step forward in this direction, dedicated long-term networks with the previously described characteristics should be established permanently in different regions around the world.
In situ observations from La Cubera, Ezpeleta, Melbex_I, Melbex_II, VAS and Nicolas stations in the Valencia Anchor Station can be obtained under request to the Climatology from Satellites Group at the Earth Physics and Thermodynamics Department at the University of Valencia. SMOS level 1 and level 3 data can be accessed at http://bec.icm.csic.es/data/data-access/ (Barcelona Expert Center, 2017). SMOS level 2 data are available under request at CATS (Centre Aval de Traitement des Donneés SMOS). AEMET precipitation data have been obtained from http://mistrals.sedoo.fr/HyMeX/ (SEDOO, OMP, Toulouse and ESPRI, IPSL, 2018). SAFRAN data are available under request to Pere Quintana Seguí (pquintana@obsebre.es). ECMWF data have been obtained from https://www.ecmwf.int/en/forecasts/datasets (European Centre for Medium-Range, 2017).
The authors declare that they have no conflict of interest.
The authors acknowledge AEMET for supplying the precipitation data and the
HyMeX database teams (ESPRI – IPSL, SEDOO – Observatoire
Midi-Pyrénées) for their help in accessing the data. SMOS L1 and L3 data were produced by the Barcelona Expert Center (http://www.smos-bec.icm.csic.es), a joint initiative of the Spanish Research Council (CSIC) and the Technical University of Catalonia (UPC), mainly funded by the Spanish National Program on Space. The SMOS L2 data were obtained from CATDS (Centre Aval de Traitement des Données SMOS) and SMOS-BEC (Barcelona Expert Center). We acknowledge the support of
the SURFEX-web team members. The ECMWF data were obtained from
http://www.ecmwf.int (last access: 3 February 2017). Special thanks go to Pere Quintana for providing the
SAFRAN atmospheric forcing data. Amparo Coll's work was supported
by both the National Spanish Space Research Programme projects MIDAS-6
(MIDAS-6/UVEG; SMOS Ocean Salinity and Soil Moisture products – Improvements
and Application Demonstration) and MIDAS-7 (MIDAS-7/UVEG; SMOS and Future
Missions Advanced Products and Applications). The first author's research is
supported by the Bundesministerium für Bildung und Forschung (BMBF;
German Federal Ministry of Education and Research).
The article processing charges for this open-access
publication were
covered by a research
centre of the Helmholtz Association.
Edited by: Pierre Gentine
Reviewed by: two anonymous referees
ARRAY Systems Computing Inc.: CESBIO, IPSL-Service d'Aèronomie, INRA-EPHYSE, Reading University, Tor Vergata University. Algorithm Theoretical Basis Document (ATBD) for the SMOS Level 2 Soil Moisture Processor Development Continuation Project. ESA No.: SO-TN-ARR-L2PP-0037 Issue: 3.9 Array No.: ASC_SMPPD_037 Date: 24 October 2014.
Barcelona Expert Center: Remote sensing research, data distribution and visualization services, available at: http://bec.icm.csic.es/data/data-access/, last access: 4 March 2017
Bircher, S., Skou, N., Jensen, K. H., Walker, J. P., and Rasmussen, L.: A soil moisture and temperature network for SMOS validation in Western Denmark, Hydrol. Earth Syst. Sci., 16, 1445–1463, https://doi.org/10.5194/hess-16-1445-2012, 2012.
Bolle, H.-J., Eckardt, M., Koslowsky, D., Maselli, F., Meliá Miralles, J., Menenti, M., Olesen, F.-S., Petkov, L., Rasool, I., and Van de Griend, A. A. (Eds.); Contributing Authors: Billing, H., Gitelson, A., Göttsche, F., Jochum-Osann, A., Lopez-Baeza, E., Meneguzzo, F., Moreno, J., Nerry, F., Rossini, P., Veroustraete, F., Vogt, R., and Van Oeleven, P. J.: Mediterranean Landsurface Processes Assessed From Space, Chapter 6 From Research to Application, Regional Climate Studies Series, Springer-Verlag Berlin Heidelberg, ISBN: 978-3-540-40151-3, Print: 978-3-540-45310-9, 2006.
Boone, A., Calvet, J.-C., and Noilhan, J.: Inclusion of a Third Soil Layer in a Land Surface Scheme Using the Force–Restore Method, J. Appl. Meteorol., 38, 1611–1630, https://doi.org/10.1175/1520-0450(1999)038<1611:IOATSL>2.0.CO;2, 1999.
Bosch, D. D., Sheridan, J. M., and Marshall, L. K.: Precipitation, soil moisture, and climate database, Little River Experimental Watershed, Georgia, United States, Water Resour. Res., 43, W09472, https://doi.org/10.1029/2006WR005834, 2007.
Brocca, L., Melone, F., Moramarco, T., Wagner, W., and Hasenauer, S.: ASCAT soil wetness index validation through in situ and modeled soil moisture data in central Italy, Remote Sens. Environ., 114, 2745–2755, 2010.
Calvet, J.-C., Noilhan, J., and Bessemoulin, P.: Retrieving the Root-Zone Soil Moisture from Surface Soil Moisture or Temperature Estimates: A Feasibility Study Based on Field Measurements, J. Appl. Meteorol., 37, 371–386, https://doi.org/10.1175/1520-0450(1998)037<0371:RTRZSM>2.0.CO;2, 1998.
Clapp, R. B. and Hornberger, G. M.: Empirical equations for some soil hydraulic properties, Water Resour. Res., 14, 601–604, 1978.
Cosby, B. J., Hornberger, G. M., Clapp, R. B., and Ginn, T.: A statistical exploration of the relationships of soil moisture characteristics to the physical properties of soils, Water Resour. Res., 20, 682–690, 1984.
Cosh, M. H., Jackson, T. J., Bindlish, R., and Prueger, J. H.: Watershed scale temporal and spatial stability of soil moisture and its role in validating satellite estimates, Remote Sens. Environ., 92, 427–435, 2004.
Djamai, N., Magagi, R., Goïta, K., Merlin, O., Kerr, Y., and Roy, A.: A combination of DISPATCH downscaling algorithm with CLASS land surface scheme for soil moisture estimation at fine scale during cloudy days, Remote Sens. Environ., 184, 1–14, 2016.
De Lannoy, G. J. and Reichle, R. H.: Global assimilation of multiangle and multipolarization SMOS brightness temperature observations into the GEOS-5 catchment land surface model for soil moisture estimation, J. Hydrometeorol., 17, 669–691, 2016.
Delwart, S., Bouzinac, C., Wursteisen, P., Berger, M., Drinkwater, M., Martín-Neira, M., and Kerr, Y. H.: SMOS validation and the COSMOS campaigns, IEEE T. Geosci. Remote, 46, 695–704, 2008.
Dente, L., Su, Z., and Wen, J.: Validation of SMOS soil moisture products over the Maqu and Twente regions, Sensors, 12, 9965–9986, 2012.
Drobinski P., Ducrocq, V., Alpert, P., Anagnostou, E., Béranger, K., Borga, M., Braud, I., Chanzy, A., Davolio, S., Delrieu, G., Estournel, C., Filali, N., Boubrahmi, J., Font, V., Grubišić, S., Gualdi, V., Homar, B., Ivančan-Picek, C., Kottmeier, V., Kotroni, K., Lagouvardos, P., Lionello, M., Llasat, C., Ludwig, W., Lutoff, C., Mariotti, A., Richard, E., Romero, R., Rotunno, R., Roussot, O., Ruin, I., Somot, S., Taupier-Letage, I., Tintore, J., Uijlenhoet, R., and Wernli, H.: HyMeX: A 10-year multidisciplinary program on the Mediterranean water cycle, B. Am. Meteorol. Soc., 95, 1063–1082, https://doi.org/10.1175/BAMS-D-12-00242.1, 2014.
Ducrocq V., Braud, I., Davolio, S., Ferretti, R., Flamant, C., Jansa, A., Kalthoff, N., Richard, E., Taupier-Letage, I., Ayral,P.-A., Belamari, S., Berne, A., Borga, M., Boudevillain, B., Bock, O., Boichard, J.-L., Bouin, M.-N., Bousquet, O., Bouvier, C., Chiggiato, J., Cimini, D., Corsmeier, U., Coppola, L., Cocquerez, P., Defer, E., Delanoë, J., Di Girolamo, P., Doerenbecher, A., Drobinski, P., Dufournet, Y., Fourrié, N., Gourley, J. J., Labatut, L., Lambert, D., Le Coz, J., Marzano, F. S., Molinié, G., Montani, A., Nord, G., Nuret, M., Ramage, K., Rison, W., Roussot, O., Said, F., Schwarzenboeck, A., Testor, P., Van Baelen, J., Vincendon, B., Aran, M., and Tamayo, J.: HyMeX-SOP1: The field campaign dedicated to heavy precipitation and flash flooding in the Northwestern Mediterranean, B. Am. Meteorol. Soc., 95, 1083–1100, https://doi.org/10.1175/BAMS-D-12-00244.1, 2014.
Duffourg, F. and Ducrocq, V.: Origin of the moisture feeding the Heavy Precipitating Systems over Southeastern France, Nat. Hazards Earth Syst. Sci., 11, 1163–1178, https://doi.org/10.5194/nhess-11-1163-2011, 2011.
Duffourg, F. and Ducrocq, V.: Assessment of the water supply to Mediterranean heavy precipitation: a method based on finely designed water budgets, Atmos. Sci. Lett., 14, 133–138, 2013.
Entekhabi, D., Rodriguez-Iturbe, I., and Castelli, F.: Mutual interaction of soil moisture state and atmospheric processes, J. Hydrol., 184, 3–17, 1996.
Entekhabi, D., Njoku, E. G., Neill, P. E., Kellogg, K. H., Crow, W. T., Edelstein, W. N., Entin, J. K., Goodman, S. D., Jackson, T. J., and Johnson, J.: The soil moisture active passive (SMAP) mission, P. IEEE, 98, 704–716, 2010.
European Centre for Medium-Range: Weather Forecasts, available at: https://www.ecmwf.int/en/forecasts/datasets, last access 3 February 2017.
FAO: World reference base for soil resources 2014 international soil classification system for naming soils and creating legends for soil maps, Rome, FAO, 2014.
Gherboudj, I., Magagi, R., Goïta, K., Berg, A. A., Toth, B., and Walker, A.: Validation of SMOS data over agricultural and boreal forest areas in Canada, IEEE T. Geosci. Remote, 50, 1623–1635, 2012.
GTOPO30 Documentation: U.S. Geological Survey, Global 30 Arc-Second Elevation, 1996.
Gonzalez-Zamora, A., Sánchez, N., Martinez-Fernandez, J., Gumuzzio, A., Piles, M., and Olmedo, E.: Long-Term SMOS Soil Moisture Products: A Comprehensive Evaluation across Scales and Methods in the Duero Basin, Phys. Chem. Earth, 83–84, 123–136, https://doi.org/10.1016/j.pce.2015.05.009, 2015.
Hirschi, M., Seneviratne, S. I., Alexandrov, V., Boberg, F., Boroneant, C., Christensen, O. B., and Stepanek, P.: Observational evidence for soil-moisture impact on hot extremes in southeastern Europe, Nat. Geosci., 4, https://doi.org/10.1038/ngeo1032, 2011.
Jansa, J., Erb, A., Oberholzer, H. R., Šmilauer, P., and Egli, S.: Soil and geography are more important determinants of indigenous arbuscular mycorrhizal communities than management practices in Swiss agricultural soils, Molecul. Ecol., 23, 2118–2135, 2014.
Jones, M. O., Jones, L. A., Kimball, J. S., and McDonald, K. C.: Satellite passive microwave remote sensing for monitoring global land surface phenology, Remote Sens. Environ., 115, 1102–1114, https://doi.org/10.1016/j.rse.2010.12.015, 2011.
Juglea, S., Kerr, Y., Mialon, A., Lopez-Baeza, E., Braithwaite, D., and Hsu, K.: Soil moisture modelling of a SMOS pixel: interest of using the PERSIANN database over the Valencia Anchor Station, Hydrol. Earth Syst. Sci., 14, 1509–1525, https://doi.org/10.5194/hess-14-1509-2010, 2010a.
Juglea, S., Kerr, Y., Mialon, A., Wigneron, J.-P., Lopez-Baeza, E., Cano, A., Albitar, A., Millan-Scheiding, C., Carmen Antolin, M., and Delwart, S.: Modelling soil moisture at SMOS scale by use of a SVAT model over the Valencia Anchor Station, Hydrol. Earth Syst. Sci., 14, 831–846, https://doi.org/10.5194/hess-14-831-2010, 2010b.
Kerr, Y. H.: Soil moisture from space: Where are we?, Hydrogeol. J., 15, 117–120, 2007.
Kerr, Y. H., Waldteufel, P., Wigneron, J. P., Delwart, S., Cabot, F., Boutin, J., and Juglea, S. E.: The SMOS mission: New tool for monitoring key elements ofthe global water cycle, P. IEEE, 98, 666–687, 2010.
Kerr, Y. H., Waldteufel, P., Wigneron, J. P., Martinuzzi, J. A. M. J., Font, J., and Berger, M.: Soil moisture retrieval from space: The Soil Moisture and Ocean Salinity (SMOS) mission, IEEE T. Geosci. Remote, 39, 1729–1735, 2001.
Khodayar, S., Sehlinger, A., Feldmann, H., and Kottmeier, C.: Sensitivity of soil moisture initialization for decadal predictions under different regional climatic conditions in Europe, Int. J. Climatol., 35, 1899–1915, https://doi.org/10.1002/joc.4096, 2015.
Khodayar, S., Raff, F., Kalthoff, N., and Bock, O.: Diagnostic study of a high precipitation event in the Western Mediterranean: adequacy of current operational networks, Q. J. Roy. Meteor. Soc., 142, 72–85, https://doi.org/10.1002/qj.2600, 2016.
Koster, R. D., Dirmeyer, P. A., Guo, Z., Bonan, G., Chan, E., Cox, P., and Liu, P.: Regions of strong coupling between soil moisture and precipitation, Science, 305, 1138–1140, 2004.
Le Moigne, P., Boone, A., Calvet, J. C., Decharme, B., Faroux, S., Gibelin, A. L., and Mironov, D.: SURFEX scientific documentation, Note de centre (CNRM/GMME), Météo-France, Toulouse, France, 2009.
Li, B. and Rodell, M.: Spatial variability and its scale dependency of observed and modeled soil moisture over different climate regions, Hydrol. Earth Syst. Sci., 17, 1177–1188, https://doi.org/10.5194/hess-17-1177-2013, 2013.
Liu, Y. Y., Parinussa, R. M., Dorigo, W. A., De Jeu, R. A. M., Wagner, W., van Dijk, A. I. J. M., McCabe, M. F., and Evans, J. P.: Developing an improved soil moisture dataset by blending passive and active microwave satellite-based retrievals, Hydrol. Earth Syst. Sci., 15, 425–436, https://doi.org/10.5194/hess-15-425-2011, 2011.
Lopez-Baeza, E., Domenech, C., Gimeno-Ferrer, J., and Velazquez, A.: Proposal of a Water Cycle Observatory: The Reference Valencia and Alacant Anchor Stations for Remote Sensing Data and Products, XI Spanish Remote Sensing Congress, Puerto de la Cruz, Tenerife, 2005.
Louvet, S., Pellarin, T., al Bitar, A., Cappelaere, B., Galle, S., Grippa, M., Gruhier, C., Kerr, Y., Lebel, T., Mialon, A., Mougin, E., Quantin, G., Richaume, P., and de Rosnay, P.: SMOS soil moisture product evaluation over West-Africa from local to regional scale, Remote Sens. Environ., 156, 383–394, https://doi.org/10.1016/j.rse.2014.10.005, 2015.
Malbéteau, Y., Merlin, O., Balsamo, G., Er-Raki, S., Khabba, S.,Walker, J. P., and Jarlan, L.: Toward a Surface Soil Moisture Product at High Spatiotemporal Resolution: Temporally Interpolated, Spatially Disaggregated SMOS Data, J. Hydrometeorol., 19, 183–200, 2018.
Masson, V., Champeaux, J. L., Chauvin, F., Meriguet, C., and Lacaze, R.: A global database of land surface parameters at 1-km resolution in meteorological and climate models, J. Climate, 16, 1261–1282, https://doi.org/10.1175/1520-0442-16.9.1261, 2003.
Molero, B., Merlin,O., Malbéteau, Y., Al Bitar, A., Cabot, F., Stefan, V., Kerr, Y., Bacon, S., Cosh, M., and Bindlish, R.: SMOS disaggregated soil moisture product at 1 km resolution: Processor overview and first validation results, Remote Sens. Environ., 180, 361–376, 2016.
Naeimi, V., Scipal, K., Bartalis, Z., Hasenauer, S., and Wagner, W.: An improved soil moisture retrieval algorithm for ers and metop scatterometer observations, IEEE T. Geosci. Remote, 47, 1999–2013, 2009.
Noilhan, J. and Planton, S.: A Simple Parameterization of Land Surface Processes for Meteorological Models, Mon. Weather Rev., 137, 536–549, https://doi.org/10.1175/1520-8560493(1989)117<0536:ASPOLS>2.0.CO;2, 1989.
Owe, M., de Jeu, R., and Walker, J.: A methodology for surface soil moisture and vegetation optical depth retrieval using the microwave polarization difference index, IEEE T. Geosci. Remote, 39, 1643–1654, 2001.
Owe, M., de Jeu, R., and Holmes, T.: Multisensor historical climatology of satellite-derived global land surface moisture, J. Geophys. Res., 113, F01002, https://doi.org/10.1029/2007JF000769, 2008.
Piles, M., Camps, A., Vall-Llossera, M., Corbella, I., Panciera, R., Rudiger, C., and Walker, J.: Downscaling SMOS-derived soil moisture using MODIS visible/infrared data, IEEE T. Geosci. Remote, 49, 3156–3166, https://doi.org/10.1109/TGRS.2011.2120615, 2011.
Piles, M., Sánchez, N., Vall-Llossera, M., Camps, A., Martínez-Fernandez, J., Martinez, J., and Gonzalez-Gambau, V.: A downscaling approach for SMOS land observations: Evaluation of high-resolution soil moisture maps over the Iberian peninsula, IEEE J. Sel. Top. Appl., 7, 3845–3857, https://doi.org/10.1109/JSTARS.2014.2325398, 2014.
Piles, M., Pou, X., Camps, A., and Vall-llosera, M.: Quality report: Validation of SMOS BEC L4 high resolution soil moisture products, version 3.0 or “all-weather”, Technical report, available at: http://bec.icm.csic.es/doc/BEC-SMOS-L4SMv3-QR.pdf (last access: 8 September 2018), 2015.
Quintana-Segui, P., Le Moigne, P., Durand, Y., Martin, E., Habets, F., Baillon, M., and Morel, S.: Analysis of near-surface atmospheric variables: Validation of the SAFRAN analysis over France, J. Appl. Meteorol. Clim., 47, 92–107, 2008.
Quintana-Seguí, P., Peral, C., Turco, M., Llasat, M. C., and Martin, E.: Meteorological Analysis Systems in North-East Spain: Validation of SAFRAN and SPAN, J. Environ. Inform., 27, 116–130, https://doi.org/10.3808/jei.201600335, 2016.
Raveh-Rubin, S. and Wernli, H.: Large-scale wind and precipitation extremes in the Mediterranean: a climatological analysis for 1979–2012, Q. J. Roy. Meteor. Soc., 141, 2404–2417, 2015.
Robock, A., Vinnikov, K. Y., Srinivasan, G., Entin, J. K., Hollinger, S. E., Speranskaya, N. A., and Namkhai, A.: The global soil moisture data bank, B. Am. Meteorol. Soc., 81, 1281–1299, 2000.
Rosenbaum, U., Bogena, H. R., Herbst, M., Huisman, J. A., Peterson, T. J., Weuthen, A., Western, A. W., and Vereecken, H.: Seasonal and event dynamics of spatial soil moisture patterns at the small catchment scale, Water Resour. Res., 48, W10544, https://doi.org/10.1029/2011WR011518, 2012.
Sanchez, N., Martinez-Fernandez, J., Scaini, A., and Perez-Gutierrez, C.: Validation of the SMOS L2 Soil Moisture Data in the REMEDHUS Network (Spain), IEEE T. Geosci. Remote, 50, 1602–1611, https://doi.org/10.1109/TGRS.2012.2186971, 2012.
Sánchez-Ruiz, S., Piles, M., Sánchez, N., Martínez-Fernández, J., Vall-llossera, M., and Camps, A.: Combining SMOS with visible and near/shortwave/thermal infrared satellite data for high resolution soil moisture estimates, J. Hydrol., 516, 273–283, https://doi.org/10.1016/j.jhydrol.2013.12.047, 2014.
Schubert, M. and Boche, H.: Solution of the multiuser downlink beamforming problem with individual SINR constraints, IEEE T. Veh. Technol., 53, 18–28, 2004.
Schwank, M., Wigneron, J. P., Lopez-Baeza, E., Volksch, I., Matzler, C., and Kerr, Y. H.: L-band radiative properties of vine vegetation at the MELBEX III SMOS cal/val site, IEEE T. Geosci. Remote, 50, 1587–1601, 2012.
Seneviratne, S. I., Corti, T., Davin, E. L., Hirschi, M., Jaeger, E. B., Lehner, I., and Teuling, A. J.: Investigating soil moisture–climate interactions in a changing climate: A review, Earth-Sci. Rev., 99, 125–161, 2010.
SEDOO, OMP, Toulouse and ESPRI, IPSL: Paris, available at: http://mistrals.sedoo.fr/HyMeX/, last access: 17 April 2018.
SMOS-BEC Team: SMOS-BEC Ocean and Land Products Description. Technical report, vailable at: http://bec.icm.csic.es/doc/BEC-SMOS-0001-PD.pdf (last access: 1 September 2018), 2016.
Taylor, C. M. and Lebel, T.: Observational evidence of persistent convective-scale rainfall patterns, Mon. Weather Rev., 126, 1597–1607, 1998.
Vautard, R., Yiou, P., D'andrea, F., De Noblet, N., Viovy, N., Cassou, C., and Fan, Y.: Summertime European heat and drought waves induced by wintertime Mediterranean rainfall deficit, Geophys. Res. Lett., 34, L07711, https://doi.org/10.1029/2006GL028001, 2007.
Vidal, J.-P., Martin, E., Franchistéguy, L., Baillon, M., and Soubeyroux, J.-M.: A 50-year high-resolution atmospheric reanalysis over France with the Safran system, Int. J. Climatol., 30, 1627–1644, 2010.
Walker, J. and Rowntree, P. R.: The effect of soil moisture on circulation and rainfall in a tropical model, Q. J. Roy. Meteor. Soc., 103, 29–46, 1977.
Western, A. W., Grayson, R. B., and Blöschl, G.: Scaling of soil moisture: A hydrologic perspective, Annu. Rev. Earth Planet. Sc., 30, 149–180, 2002.
Wigneron, J. P., Calvet, J. C., Pellarin, T., Van de Griend, A. A., Berger, M., and Ferrazzoli, P.: Retrieving near-surface soil moisture from microwave radiometric observations: current status and future plans, Remote Sens. Environ., 85, 489–506, 2003.
Wigneron, J.-P., Schwank, M., Lopez Baeza, E., Kerr, Y., Novello, N., Millan, C., Moisy, C., Richaume, P., Mialon, A., Al Bitar, A., Cabot, F., Lawrence, H., Guyon, D., Calvet, J.-C., Grant, J. P., de Rosnay, P., Mahmoodi, A., Delwart, S., and Mecklenburg, S.: First Evaluation of the Simultaneous SMOS and ELBARA-II Observations in the Mediterranean Region, Remote Sens. Environ., 124, 26–37, 2012.
Zampieri, M., D'Andrea, F., Vautard, R., Ciais, P., de Noblet-Ducoudré, N., and Yiou, P.: Hot European Summers and the Role of Soil Moisture in the Propagation of Mediterranean Drought, J. Climate, 22, 4747–4758, https://doi.org/10.1175/2009JCLI2568.1, 2009.